- 1Department of Psychiatry, Western Psychiatric Hospital, University of Pittsburgh, Pittsburgh, PA, United States
- 2Department of Orthopaedic Surgery, University of Pittsburgh Medical Center (UPMC) Sports Concussion Program-University of Pittsburgh, Pittsburgh, PA, United States
- 3Department of Radiology, Magnetic Resonance Research Center, University of Pittsburgh, Pittsburgh, PA, United States
Background: Concussion symptoms in adolescents typically resolve within 4 weeks. However, 20 – 30% of adolescents experience a prolonged recovery. Abnormalities in tracts implicated in visuospatial attention and emotional regulation (i.e., inferior longitudinal fasciculus, ILF; inferior fronto-occipital fasciculus, IFOF; uncinate fasciculus; UF) have been consistently reported in concussion; yet, to date, there are no objective markers of prolonged recovery in adolescents. Here, we evaluated the utility of diffusion MRI in outcome prediction. Forty-two adolescents (12.1 – 17.9 years; female: 44.0%) underwent a diffusion Magnetic Resonance Imaging (dMRI) protocol within the first 10 days of concussion. Based on days of injury until medical clearance, adolescents were then categorized into SHORT (<28 days; N = 21) or LONG (>28 days; N = 21) recovery time. Fractional anisotropy (FA) in the ILF, IFOF, UF, and/or concussion symptoms were used as predictors of recovery time (SHORT, LONG). Forty-two age- and sex-matched healthy controls served as reference. Higher FA in the ILF (left: adjusted odds ratio; AOR = 0.36, 95% CI = 0.15 – 0.91, P = 0.030; right: AOR = 0.28, 95% CI = 0.10 – 0.83, P = 0.021), IFOF (left: AOR = 0.21, 95% CI = 0.07 – 0.66, P = 0.008; right: AOR = 0.30, 95% CI = 0.11 – 0.83, P = 0.020), and UF (left: AOR = 0.26, 95% CI = 0.09 – 0.74, P = 0.011; right: AOR = 0.28, 95% CI = 0.10 – 0.73, P = 0.010) was associated with SHORT recovery. In additional analyses, while adolescents with SHORT recovery did not differ from HC, those with LONG recovery showed lower FA in the ILF and IFOF (P < 0.014). Notably, inclusion of dMRI findings increased the sensitivity and specificity (AUC = 0.93) of a prediction model including clinical variables only (AUC = 0.75). Our findings indicate that higher FA in long associative tracts (especially ILF) might inform a more objective and accurate prognosis for recovery time in adolescents following concussion.
Introduction
Sport-related concussion represents a major public health problem in children and adolescents (1, 2). Typically, concussion symptoms resolve within the first 4 weeks of injury without any prolonged effects in this population (3). In some cases, however, symptoms persist beyond this time frame and affect functioning and academic performance (4–10). Studies investigating risk factors for persistence of concussion symptoms have identified that reporting higher severity of concussion symptoms (11–14), neurocognitive impairment (15, 16), being female (17), having a delay in the first clinical visit (11, 18), and/or history of previous concussions (17) are associated with prolonged recovery after injury. Yet, these factors cannot help quantifying the extent of the injury and if this is associated with different outcomes.
Neuroimaging can detect neural anatomical abnormalities following concussion. In recent years, studies have shown structural and functional abnormalities in the brain following concussion (19). Diffusion Magnetic Resonance Imaging (dMRI), in particular, has been used to show micro- and macro-structural abnormalities in white matter tracts (20, 21). Most dMRI studies focused on adult populations and showed that adults who sustained a concussion have lower fractional anisotropy (FA, an index of microstructural directionality of the water, reflecting the collinearity and/or integrity of the fibers in the brain) (22) in key tracts of visuospatial attention (23–26), including the inferior longitudinal fasciculus (ILF) (23), inferior fronto-occipital fasciculus (IFOF) (23, 24), and uncinate fasciculus (UF) (25, 26) in comparison to healthy controls. In addition, research showed that FA positively correlates with better cognitive performance, as measured by the Standard Assessment of Concussion, in collegiate football players (mean age [SD] = 19.2 [1.0]) in the acute stages of concussion (27). Research also demonstrated that other dMRI measurements are affected by concussion. For example, after accounting for pre-injury abnormalities in thirteen collegiate athletes, Cubon et al. showed that concussion is associated with increases in radial diffusivity (RD, a metric that reflects the displacement of water perpendicular to the principal direction) (22) in the IFOF and UF tracts, reflecting local edema, disorganization of the fiber architecture and/or abnormal white matter integrity (28). In children and adolescents, however, concussion has been associated with both increases and decreases in FA. For example, even if in pediatric populations most studies have shown higher FA and lower RD in initial stages of concussion (29–33), lower FA in the UF has been associated with poor outcomes, including prolonged recovery time and persistence of symptoms months after injury (26, 34). In one of these studies (34), King et al. showed that children reporting a persistent symptomatology 1 month after injury showed lower FA in the UF when compared to healthy controls (34). Altogether, these findings suggest that lower FA in white matter tracts implicated in visuospatial attention and emotional regulation may be associated with a persistent symptomatology. Recent dMRI methods allow the detection of collinearity and/or integrity along the entire tract (tractometry/tract-profile) and identify both focal and widespread abnormalities (35, 36), likely increasing the sensitivity of these techniques.
The aim of the present study was to expand on the extant literature and test if dMRI close to the injury can help predict recovery time up to 13 months after concussion in 12–17-year-olds. To this end, dMRI data were collected within the first 10 days of concussion. Based on days of injury until medical clearance, concussed adolescents were categorized into those with short (<4 weeks) or long (>4 weeks) recovery time. dMRI data were also collected in a sample of age- and sex-match healthy controls to aid interpretation of dMRI findings distinguishing adolescents with short from those with long recovery time. To the best of our knowledge, this is the first study to evaluate recovery time after concussion in a pediatric population followed up for 13 months after injury using a combination of tractography and tract-profile approaches. Based on the extant literature, our main hypothesis was that, in adolescents who sustained a recent concussion (up to 10 days), lower fiber collinearity in major white matter tracts previously reported to be abnormal following concussion, namely ILF, IFOF, and UF, would be associated with prolonged recovery (symptoms persisting after 4 weeks of injury). We further hypothesized that the inclusion of dMRI findings would increase the predictive value of clinical prediction models (secondary hypothesis).
Materials and Methods
Participants
The study was approved under an expedited protocol by the University of Pittsburgh Institutional Review Board. An initial sample of fifty adolescents (12.1 – 17.9 years; 19 [44.0%] female) with a recently (range between concussion and study entry = 1–10, mean [SD]: 7.2 [2.4] days) diagnosed concussion were recruited through the longitudinal Investigating Concussion in Adolescents at Risk for Emotion dysregulation (iCARE) study (R01MH11488101; PIs: Versace, Kontos). Eight (16%) adolescents were excluded from the analysis due to incomplete neuroimaging acquisition or loss of follow-up (for more information, see Supplementary Table 1), leaving a total of 42 (84%) concussed participants (age range = 12.1–17.9 years old; 42.9% female). The vast majority of the 42 participants showed sport-related concussion (N = 35, 83.3%). The remaining participants reported fall/accident (N = 6, 14.3%) and direct blow to the head (N = 1, 2.4%) as the cause of concussion. Forty-two age- and sex- matched adolescent healthy controls (HC) with no Axis-I diagnoses were recruited through a local recruitment website (https://pittplusme.org) and an ongoing neuroimaging study (i.e., Mood and Brain Circuitry in Adolescence [MBA] study [R01 MH111600, PIs: Ladouceur, Diler]). Demographic characteristics of the sample are detailed in Table 1. Exclusion criteria are described in the Supplementary Methods.
Clinical Assessments
Recovery Time After Concussion
Recovery time was defined as the number of days between the most recent concussion and the medical clearance (3). Participants, who were follow-up of up to 13 months (mean [SD] = 5.4 [4.9]), received medical clearance if they met the following criteria: (1) No symptoms at rest for a minimum of 24 h, (2) No provocation of symptoms with typical physical and cognitive activities, (3) Neurocognitive functioning at typical baseline, (4) Normal vestibular and oculomotor functioning, and (5) No other related medical complaints. Based on recovery time and the fact that concussion symptoms usually resolve within 4 weeks in this population (3), two concussion groups were derived: (1) SHORT, including participants who received medical clearance within the first 4 weeks (28 days) after injury, and (2) LONG, including participants who did not receive clearance in the same period.
Measures of Concussion
Concussion-related symptoms and impairments were assessed at study entry using the Immediate Post-concussion Assessment and Cognitive Testing (ImPACT) (37), Post-Concussion Symptom Scales (PCSS) (37), and Vestibular/Ocular-Motor Screening (VOMS) (38). ImPACT is a computerized battery that includes six neurocognitive tests (e.g., attention, verbal recognition, visual working memory, visual processing speed, reaction time, and learning) and the PCSS for symptom inventory. VOMS is a screening tool that assess vestibular and ocular motor impairments. The interviews were conducted by research staff trained to reliably administer these assessments.
Post-Concussion Symptoms
The PCSS for symptom inventory rated symptoms using a 0–6 Likert scale, with 0 indicating no difficulty and 1–6 indicting mild-to-severe difficulty with the symptom. As previously done (39), the scores of seventeen symptoms were combined to derive four main symptom factors: (1) cognitive-migraine-fatigue (headache, dizziness, fatigue, drowsiness, sensitivity to light, sensitivity to noise, feeling slowed down, mentally foggy, difficulty concentrating, and difficulty remembering), (2) affective (sadness, nervousness, and feeling more emotional), (3) somatic (vomiting and numbness) and (4) sleep (sleeping more than usual and sleeping less than usual).
Neurocognitive Tests
Four composite scores were derived using the ImPACT neurocognitive tests: (1) verbal memory (percent correct), (2) visual memory (percent correct), (3) visual motor processing speed (numerical score), and (4) reaction time (seconds). Better performance is associated with higher values in the first three composite scores and lower reaction time.
Vestibular and Ocular Motor Impairment
The following domains were assessed with VOMS: smooth pursuit, horizontal and vertical saccades, convergence, horizontal vestibular ocular reflex, and visual motion sensitivity. Participants used a scale of 0 (none) to 10 (severe) to report the intensity of four symptoms after the assessment of each domain: headache, dizziness, nausea, and fogginess (maximum total for each item = 40). A total symptom score was calculated by combining the score reported for each of these four symptoms (maximum total = 270).
Additional Clinical Measures
History of previous concussions was collected at study entry. Information regarding the presence of headaches and nausea 6 months before injury was also collected. In addition to demographic information and concussion related symptoms, psychiatric symptoms (e.g., impulsivity, anxiety, depression) were assessed with rating scales and questionnaires administered on the day of scan (40–43).
Neuroimaging Data
MRI data were acquired up to 10 days after concussion (mean time [SD]: 7.0 [2.6] days). MRI images were reviewed by clinical board-certified radiologist to rule out major structural abnormalities. dMRI acquisition and preprocessing steps are described in the Supplementary Methods. In brief, after eddy current, subject motion, and Echo Planar Imaging (EPI) distortions correction (44, 45), dMRI bundle-specific tractography was performed using TractSeg (35, 36). TractSeg is a convolutional neural network approach that directly segments white matter tracts using fiber orientation distribution function (fODF) peaks, based on Multi-Shell-Multi-Tissue Constrained Spherical Deconvolution (46, 47), as proposed in Mrtrix-3 (48). Six a priori major white matter tracts were selected for this study: left/right ILF, left/right IFOF, and left/right UF. The contribution of other major white matter tracts (anterior thalamic radiation, cingulum bundle, fronto-pontine tract, optic radiation, parieto-occipital pontine, superior longitudinal fasciculus I-III, arcuate fasciculus, superior thalamic radiation, corticospinal tract, thalamic-premotor, thalamic-parietal, thalamic-occipital, striato-frontal, striato-premotor, and seven main bundles of the corpus callosum) to the time to recover after concussion was also explored. FA maps were calculated using Mrtrix-3 (48) tools (dwi2tensor and tensor2metric). Axial Diffusivity (AD) and Radial Diffusivity (RD) reflect the displacement of water molecules along or perpendicular to the principal diffusion direction and can help interpret FA findings (22). Thus also these measures were similarly calculated.
For each tract, overall mean and nodal values were extracted to depict the collinearity of the fibers across (mean) and along (tractometry/tract-profile) the entire tract. Tract-profile analyses allow for the characterization of dMRI properties along each tract (5 consecutive nodes, each node covering 20% of the entire tract) and thus for the identification of focal abnormalities in tracts of interest.
Statistical Analyses
Level 1 Analysis: Main Hypothesis Testing (Neural Correlates of Recovery Groups)
To test our main hypothesis, we used logistic regression models in SPSS V24.0. Using six parallel regression models, we examined the main effect of mean FA in six white matter tracts of interest (left/right ILF, left/right IFOF, and left/right UF) on recovery groups (SHORT, LONG). Given the known effect of age (49–51) and sex (52) on white matter, these variables were included as covariates. In addition, the number of days between the most recent concussion and scan was used as covariate. To account for multiple testing (six tracts of interest), False Discovery Rate (FDR, p < 0.05) (53) was used.
Tract-Profile Analyses
For those white matter tracts in which, after FDR correction, there was a main effect of FA (mean FA across the entire tract) on recovery time, nodal FA values (tract-profile; 5 consecutive nodes) were used to identify if the FA differences between adolescents with SHORT and LONG recovery were focal or widespread. In the case of two or more consecutive nodes in the same tract (>40%) surviving FDR correction, mean FA was used to reflect the focal abnormality in further analyses. Mean AD and RD were also extracted from node clusters to help further interpret main FA findings.
Between-Group Comparisons
Additional analyses explored the group differences between LONG (or SHORT) and our healthy control group (LONG vs. HC; SHORT vs. HC) on main FA findings identified above. Analysis of covariance (ANCOVA) evaluated differences between HC and Concussed groups (SHORT combined with LONG) using the mean FA of each white matter tract of interest (left/right ILF, IFOF, and UF).
Recovery Time as a Continuous Measure
As opposed to using a dichotomic approach to recovery time (SHORT, LONG), number of days to recover after concussion (continuous variable) was used in linear regression models.
Level 2 Analysis: Secondary Hypothesis Testing (Predictive Models)
Forward stepwise logistic regression was used to build predictive models that combined clinical, demographic, and/or neuroimaging characteristics to differentiate our main groups (SHORT, LONG). Two models were tested: (1) A model that included clinical and demographic variables significantly different between the two main groups, and (2) A second model that combined clinical, demographic, and FA abnormalities identified in Level 1 analysis. Receiver Operating Characteristic (ROC) curve and Area Under the Curve (AUC) were used to assess the diagnostic ability of the models (54).
Recovery Time as a Continuous Measure
Linear regression was used to assess the ability of the combined model to predict time to recover as a continuous variable.
Exploratory Analyses
In the concussion group, Pearson or Spearman correlations, as appropriate, were used to explore the relationships between mean FA of abnormalities identified in Level 1 analysis and composite scores, post-concussion symptom factors, and VOMS total symptom score. Two-sample Kolmogorov-Smirnov tests were used to compare the distribution of FA in these node clusters (55). In addition, analysis of variance (ANOVA) was used to evaluate the effect of previous concussions or history of headaches and nausea over the past 6 months on mean FA in each node cluster.
Given the known effect of movements on dMRI measures, the main effect of average translation and average rotation was also explored in each node cluster.
Results
All concussed participants received medical clearance (range = 9–150 days, mean [SD] = 43.3 [38.1] days), with 50% (N = 21) showing recovery within 4 weeks of injury (SHORT). There were no significant differences between SHORT and LONG recovery groups regarding demographic characteristics, time between injury and scan, previous concussions, history of headaches, or nausea (Table 1). Compared to SHORT, those with LONG recovery showed lower percentage of correct answers in two out of the four neurocognitive measures (i.e., verbal and visual memory composite scores), higher severity in three out of the four post-concussion symptom factors (i.e., affective, sleep, and cognitive-migraine-fatigue symptoms), and higher VOMS total symptom score (Table 1). For additional information regarding demographic and clinical characteristics, see Supplementary Tables 2, 3.
Level 1 Analysis: Main Hypothesis Testing (Neural Correlates of Recovery Groups)
Logistic regression models revealed that there was a significant main effect of mean FA on recovery groups (SHORT, LONG) in all white matter tracts of interest (Supplementary Table 4): (1) left ILF (adjusted odd ration; AOR = 0.36, 95% CI = 0.15–0.91, P = 0.030, FDR P = 0.030), (2) right ILF (AOR = 0.28, 95% CI = 0.10–0.83, P = 0.021, FDR P = 0.025), (3) left IFOF (AOR = 0.21, 95% CI = 0.07–0.66, P = 0.008, FDR P = 0.022), (4) right IFOF (AOR = 0.30, 95% CI = 0.11–0.83, P = 0.020, FDR P = 0.025), (5) left UF (AOR = 0.26, 95% CI = 0.09–0.74, P = 0.011, FDR P = 0.022), and (6) right UF (AOR = 0.28, 95% CI = 0.10–0.73, P = 0.010, FDR P = 0.022).
Focal Abnormalities in Tracts of Interest
Tract-profile analyses revealed that the FA abnormalities differentiating SHORT and LONG groups were focal (Figures 1–3): temporal portions of the left ILF (Size = 40% of the tract, AOR = 0.13, 95% CI = 0.03–0.54, P = 0.005, FDR P = 0.011), right ILF (Size = 20% of the tract, AOR = 0.16, 95% CI = 0.04–0.63, P = 0.009, FDR P = 0.011), left UF (Size = 20% of the tract, AOR = 0.25, 95% CI = 0.09–0.66, P = 0.005, FDR P = 0.011), and right UF (Size = 20% of the tract, AOR = 0.28, 95% CI = 0.11–0.70, P = 0.006, FDR P = 0.011); the middle portions of the left IFOF (Size = 20% of the tract, AOR = 0.14, 95% CI = 0.03–0.55, P = 0.005, FDR P = 0.011) and right IFOF (Size = 60% of the tract, AOR = 0.22, 95% CI = 0.07–0.67, P = 0.008, FDR P = 0.011); and the frontal portion of the left IFOF (Size = 20% of the tract, AOR = 0.30, 95% CI = 0.11–0.80, P = 0.016, FDR P = 0.016). Analyses using RD and AD measures further revealed that the lower FA in concussed participants with LONG vs. those with SHORT recovery was associated with higher RD. AD did not differ (Supplementary Table 5).
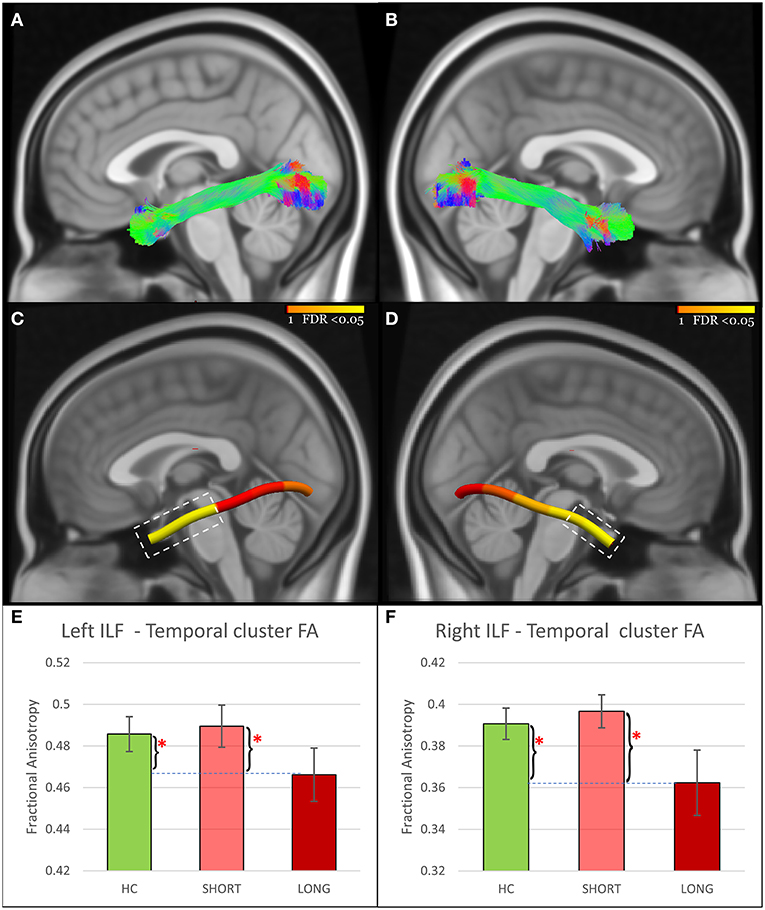
Figure 1. ILF findings. (A,B) Show the reconstructed left and right ILF using TractSeg. (C,D) Show node clusters in the left and right ILF. The background is the standard MNI-152 1 mm brain. Red-Yellow color bar represents the range of p values used in node-wise statistics after FDR correction. Error-bar plots in (E,F) depict the group difference upon FA in the 42 HC (green color), 21 adolescents with short recovery (SHORT, light red color), and 21 adolescents with prolonged recovery (LONG, red color) after concussion. Braces and asterisks show p-values that survived FDR correction. ILF, Inferior Longitudinal Fasciculus; HC, healthy control; FA, Fractional Anisotropy; FDR, False Discovery Rate.
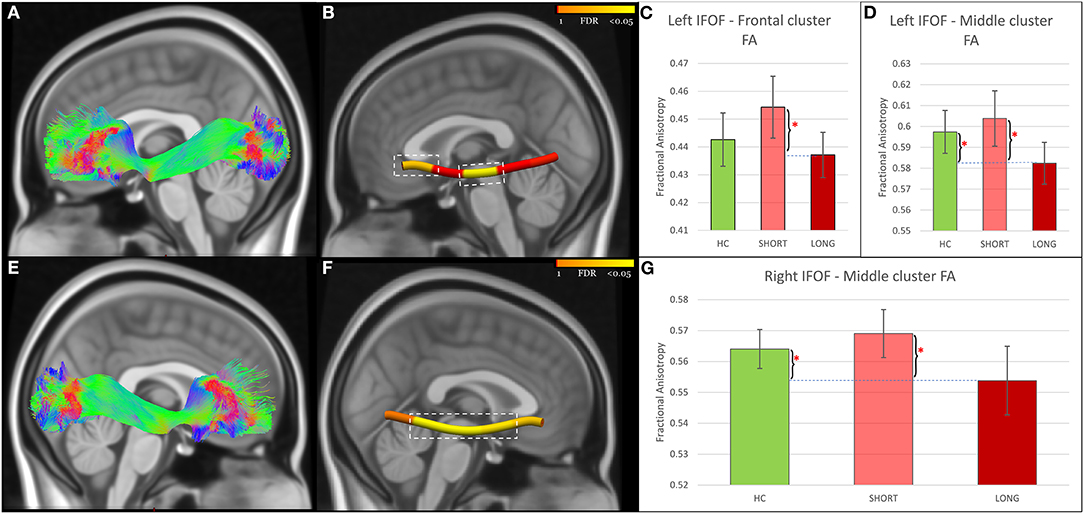
Figure 2. IFOF findings. (A,E) Show the reconstructed left and right IFOF using TractSeg. (B,F) Show node clusters in the left and right IFOF. The background is the standard MNI-152 1 mm brain. Red-Yellow color bar represents the range of p values used in node-wise statistics after FDR correction. Error-bar plots in (C,D,G) depict the group difference upon FA in the 42 HC (green color), 21 adolescents with short recovery (SHORT, light red color), and 21 adolescents with prolonged recovery (LONG, red color) after concussion. Braces and asterisks show p-values that survived FDR correction. IFOF, Inferior Fronto-Occipital Fasciculus; HC, healthy control; FA, Fractional Anisotropy; FDR, False Discovery Rate.
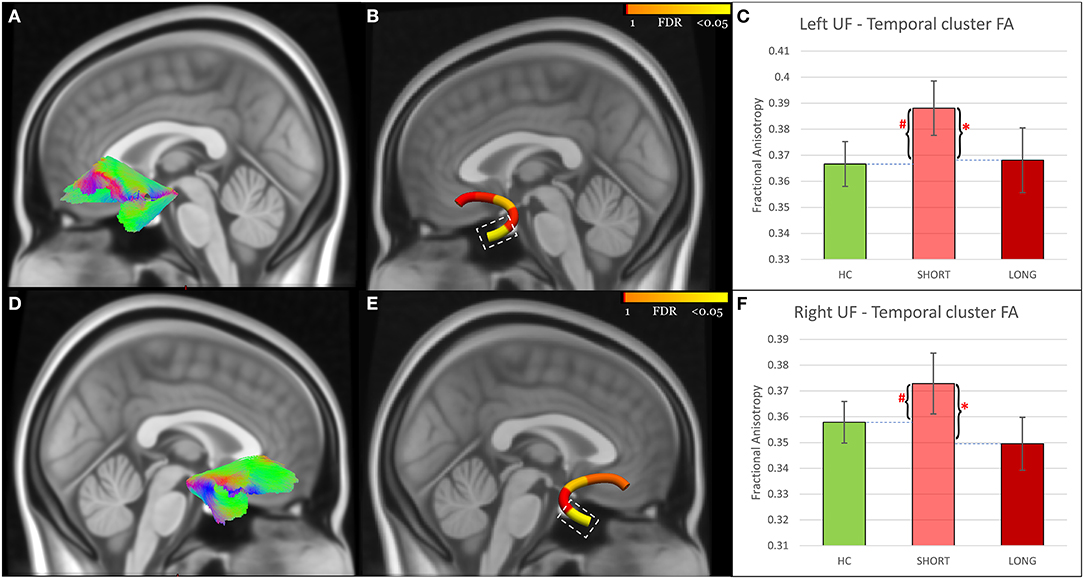
Figure 3. UF findings. (A,D) Show the reconstructed left and right UF using TractSeg. (B,E) show node clusters in the left and right UF. The background is the standard MNI-152 1 mm brain. Red-Yellow color bar represents the range of p values used in node-wise statistics after FDR correction. Error-bar plots in (C,F) depict the group difference upon FA in the 42 HC (green color), 21 adolescents with short recovery (SHORT, light red color), and 21 adolescents with prolonged recovery (LONG, red color) after concussion. Braces and asterisks show p-values that survived FDR correction. Pound signs indicate significant p-values that did not survive FDR correction. UF, Uncinate Fasciculus; HC, healthy control; FA, Fractional Anisotropy; FDR, False Discovery Rate.
Between-Group Comparisons
Participants in the LONG recovery group showed lower FA in all seven node clusters in comparison to those with SHORT recovery. When compared to HC, those in the LONG recovery group showed lower FA in the temporal clusters of the left and right ILF and in the middle clusters of the left and right IFOF. There were no differences between LONG and HC in the other node clusters. However, participants in the SHORT recovery group showed higher FA than HC in the left and right UF node clusters, but these findings did not survive FDR correction. There were no differences between SHORT and HC in the other node clusters (Figures 1–3 and Supplementary Table 6).
Recovery Time as a Continuous Measure
Mean FA in main node clusters was negatively associated with the number of days to recover after concussion (Supplementary Table 7).
Level 2 Analysis: Secondary Hypothesis Testing—Predictive Models
For the model that included only clinical and demographic variables, six predictors were tested: two neurocognitive measures—verbal and visual memory composite scores, three post-concussion symptom factors—affective, sleep, and cognitive-migraine-fatigue, and the VOMS total symptom score. Only the cognitive-migraine-fatigue factor was identified. This model showed an AUC of 0.75 (Table 2 and Figure 4).
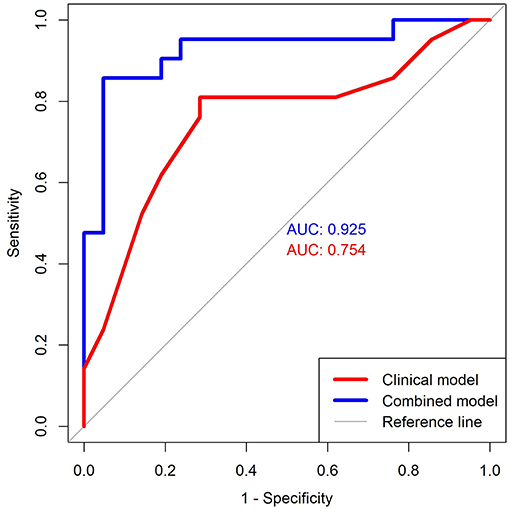
Figure 4. ROC curves. This figure shows the curve for the Clinical model (red color) and Combined model (blue color). The first model includes only the Cognitive-migraine-fatigue symptom factor and the second model combines the Cognitive-migraine-fatigue symptom factor with the FA of Left ILF—Temporal cluster FA and Right ILF—Temporal cluster FA. These curves were created by plotting data pairs of sensitivity/specificity and the AUC represents the discriminative ability of the test. ROC, Receiver Operating Characteristic; AUC, Area Under the Curve; ILF, Inferior Longitudinal Fasciculus.
For the model that combined clinical, demographic, and neuroimaging variables, thirteen predictors were tested (the same six included in the first model, in addition to the mean FA of the seven node clusters identified in Level 1 analysis). Three predictors were identified: (1) Left ILF temporal cluster FA, (2) Right ILF temporal cluster FA, and (3) cognitive-migraine-fatigue factor. This second model showed an AUC of 0.93 (Table 2 and Figure 4). The difference between ROC curves was statistically significant (P = 0.011).
Recovery Time as a Continuous Measure
There was a main effect of the combined model on the number of days to recover after concussion (F = 6.7, P = 0.001).
Exploratory Analyses
Across all concussed participants, there was no relationship between neurocognitive measures, post-concussion symptom factors, VOMS total score, history of previous concussions, headaches, or nausea with mean FA of the main findings identified in Level 1 analysis (for additional information, see Supplementary Table 9). In addition, recovery groups showed no differences in the distribution of FA in most of the clusters (for additional information, see Supplementary Table 9).
There was no significant effect of dMRI movements on our main findings (for additional information, see Supplementary Table 10).
Analyses involving other tracts did not yield significant findings, after accounting for multiple comparisons (for additional information, see Supplementary Table 11).
Discussion
Findings from this study show that abnormal indices of tract microstructure—reflecting white matter integrity/organization of the fiber architecture—(i.e., lower FA and higher RD) in the ILF, IFOF, and UF are associated with higher probability of prolonged recovery (more than 4 weeks after concussion). Further analyses revealed that lower FA in the temporal ILF and middle IFOF distinguished concussed participants with a prolonged recovery from healthy participants. Notably, the inclusion of the dMRI findings in a clinical prediction model significantly increased the overall sensitivity and specificity.
In support of our main hypothesis, findings indicate that focal changes in the microstructural architecture of ILF, IFOF, and UF can help differentiate adolescents with prolonged recovery from those with short recovery (and healthy controls). The ILF is a long associative tract that connects occipital to anterior temporal regions of the brain and has been associated mainly with visuospatial attention, as well as high-order cognitive functions such as reading fluency and comprehension (56). Studies have shown that this tract develops earlier and faster than other associative tracts, with a peak around 11 years (50, 56–58). Thus, it is possible that when concussion-related lesions occur after this age (as in our sample; mean age [SD] = 15.5 [1.7] years), adolescents might need more time to recover from concussion, especially for those brain functions associated with this tract. Intervention strategies aiming to strengthen attentional processes might be able to promote/reverse plasticity of the ILF, as shown by lesion studies (59–61). The IFOF is also a long associative tract that, bypassing the temporal lobe, connects occipital to frontal cortical regions. Similar to ILF, IFOF has been implicated in visual processing, spatial attention, and higher cognitive functions, such as reading fluency and comprehension (62–64). The UF connects frontal and temporal regions of the brain and plays an important role in emotional regulation (65) (e.g., monitoring emotional distractors and redirecting attention in the context of goal-directed behavior). Within this network, the IFOF can be considered a direct path that, by promptly feeding visual information to the prefrontal cortex, carries context-relevant information for higher cognitive functioning (e.g., decision making). The ILF and UF can be considered the indirect path by which the anterior temporal pole upgrades these visual stimuli with salience (salience attribution) to then feed-forward this “enriched” information to the prefrontal cortex for additional context. Feed-back inputs (from the prefrontal cortical to temporo-occipital regions) is also mediated by these tracts. Supplementary analyses also showed that other white matter tracts involved with visual processing, visuospatial attention and executive functions (e.g., superior longitudinal fasciculus, optic radiation) (66–68) were associated with prolonged recovery, but did not survive multiple comparison correction. Studies have shown that white matter abnormalities are associated with different outcomes in concussion (26, 34, 69); however, to the best of our knowledge, this is the first study to use tractography to identify tract-specific abnormalities that are associated with prolonged recovery in adolescents and show that concussion might not affect white matter tracts uniformly. Understanding the properties along the tract is particularly important in children and adolescents, as brain maturation also does not happen uniformly (70). For example, a recent study in collegiate athletes (18.9 [0.9] years) using tract-based spatial statistical (TBSS) showed that having higher RD in the 24–48 h after concussion was associated with prolonged recovery (up to 27 days) after concussion. However, no tract-profile approach was used to inform on possible pathophysiologic mechanisms following concussion (71). Our findings indicate that abnormalities near the gray-white matter boundary in frontal and temporal regions are particularly important for recovery after concussion. This interface appears to be especially vulnerable to injury due to the different physical properties of gray and white matter and distinct responses to forces secondary to the impact (72, 73). In addition, previous studies have reported that perfusion abnormalities (e.g., reduced blood flow and volume) in frontal and temporal lobes after concussion are associated with worse outcomes (74). Interestingly, our findings in the medial portion of the IFOF sits in proximity of the temporal lobe, suggesting that—depending on the magnitude of the injury—altered perfusion in this region might also affect tracts that pass by this region to connect other lobes.
In support of our secondary hypothesis, we found that indices of white matter abnormalities did improve the performance of our clinical prediction model. Symptoms clustering in the cognitive-migraine-fatigue factor are common following concussion and have been associated with prolonged recovery in pediatric population (39, 75, 76). The ILF, as previously mentioned, is associated with visuospatial attention (56). In this study, each predictor (i.e., cognitive-migraine-fatigue factor, left ILF temporal cluster, and right ILF temporal cluster) individually showed high sensitivity and specificity to differentiate adolescents with short from those with prolonged recovery; however, the inclusion of the left/right ILF increased the predictive value of the clinical model, suggesting a potential role for dMRI in the prediction of different clinical outcomes in concussion.
There were limitations in this study. (1) Although we employed a combination of tractography and tract-profile approaches to assess white matter correlates of prolonged recovery following concussion in adolescents, as noted above, this study did not include pre-injury dMRI data. To address this limitation, we included a healthy control group as normative reference, but this approach cannot rule out the possibility that these white matter abnormalities existed before concussion. (2) Although this study yielded compelling evidence of neural correlates of prolonged recovery following concussion, future studies with larger samples are needed to replicate these findings and further show the extent to which dMRI can help predict recovery time in clinical practice. (3) Future studies should include a neuroimaging longitudinal design with repeated dMRI scans to evaluate the effect of concussion on developmental trajectories of different white matter tracts. (4) This study included adolescents in the acute/subacute stages of concussion. As such, the white matter characteristics identified during this period might not apply when including asymptomatic participants in later stages of recovery. Continued follow-up of the present sample and the inclusion of a follow-up scan will allow us to better understand the relationship between white matter and concussion in acute/subacute and chronic stages of concussion.
To our knowledge, this is the first study to identify focal abnormalities in white matter tracts that can help to distinguish between short and prolonged recovery. Our findings indicate that the integrity of white matter tracts involved in visuospatial attention is particularly important for a prompt recovery after injury. Identification of these neural correlates deepens our understanding of the pathophysiological mechanisms associated with concussion and the potential role of dMRI as an objective measure in concussion recovery. In addition, our findings suggest that the combination of clinical and dMRI measures might improve prediction and be useful for clinical decision-making and early intervention strategies.
Data Availability Statement
The raw data supporting the conclusions of this article will be made available by the authors, without undue reservation.
Ethics Statement
The studies involving human participants were reviewed and approved by the Institutional Review Board at the University of Pittsburgh. Written informed consent to participate in this study was provided by the participants' legal guardian/next of kin.
Author Contributions
JL, AK, SM, CH, SS, HA-w, RS, HB, NB, AC, CS, and AV contributed to the conception, design, acquisition, and analysis of the data. JL, AK, and AV conceptualized and designed the article. JL, AK, SM, SE, CH, SS, HA-w, RS, HB, NB, AC, CS, AB, TK, SI, AS, RD, CL, MP, DB, MC, and AV contributed to manuscript revision and approved the submitted version. All authors contributed to the article and approved the submitted version.
Funding
The present study was supported by the National Institute of Mental Health (R01MH114881, PIs: Versace, Kontos; R01MH111600, PIs: Ladouceur, Diler), National Institute of Health (1S10OD023405-01; PI: Hetherington), and the Chuck Noll Foundation for Brain Injury Research (FP00004146). These funding agencies were not involved in the design or conduct of the study, the collection, management, analysis, or interpretation of the data, or the preparation, review, or approval of the manuscript.
Conflict of Interest
MP declares a one-time honorarium from Sunovion in November 2016. DB receives research support from NIMH, AFSP, the Once Upon a Time Foundation, and the Beckwith Foundation, receives royalties from Guilford Press, from the electronic self-rated version of the C-SSRS from eRT, Inc., and from performing duties as an UptoDate Psychiatry Section Editor, receives consulting fees from Healthwise, and receives Honoraria from the Klingen-stein Third Generation Foundation for scientific board membership and grant review. MC is a former Co-owner and Board Member of ImPACT Applications (relationship ended 12/16/19).
The remaining authors declare that the research was conducted in the absence of any commercial or financial relationships that could be construed as a potential conflict of interest.
Acknowledgments
We thank the participants and their families for their contributions to this study.
Supplementary Material
The Supplementary Material for this article can be found online at: https://www.frontiersin.org/articles/10.3389/fneur.2021.681467/full#supplementary-material
References
1. Bryan MA, Rowhani-Rahbar A, Comstock RD, Rivara F. Sports-and recreation-related concussions in US youth. Pediatrics. (2016) 138:e20154635. doi: 10.1542/peds.2015-4635
2. Veliz P, McCabe SE, Eckner JT, Schulenberg JE. Prevalence of concussion among US adolescents and correlated factors. JAMA. (2017) 318:1180–2. doi: 10.1001/jama.2017.9087
3. McCrory P, Meeuwisse W, Dvorak J, Aubry M, Bailes J, Broglio S, et al. Consensus statement on concussion in sport—the 5th international conference on concussion in sport held in Berlin, October 2016. Br J Sports Med. (2017) 51:838–47. doi: 10.1136/bjsports-2017-097699
4. Kontos AP, Covassin T, Elbin R, Parker T. Depression and neurocognitive performance after concussion among male and female high school and collegiate athletes. Arch Phys Med Rehabil. (2012) 93:1751–6. doi: 10.1016/j.apmr.2012.03.032
5. Barlow KM, Crawford S, Stevenson A, Sandhu SS, Belanger F, Dewey D. Epidemiology of post-concussion syndrome in pediatric mild traumatic brain injury. Pediatrics. (2010) 126:e374–81. doi: 10.1542/peds.2009-0925
6. Iverson GL, Gardner AJ, Terry DP, Ponsford JL, Sills AK, Broshek DK, et al. Predictors of clinical recovery from concussion: a systematic review. Br J Sports Med. (2017) 51:941–8. doi: 10.1136/bjsports-2017-097729
7. Zuckerman SL, Lee YM, Odom MJ, Solomon GS, Forbes JA, Sills AK. Recovery from sports-related concussion: days to return to neurocognitive baseline in adolescents versus young adults. Surg Neurol Int. (2012) 3:130. doi: 10.4103/2152-7806.102945
8. Crowe L, Collie A, Hearps S, Dooley J, Clausen H, Maddocks D, et al. Cognitive and physical symptoms of concussive injury in children: a detailed longitudinal recovery study. Br J Sports Med. (2016) 50:311–6. doi: 10.1136/bjsports-2015-094663
9. Kostyun RO, Milewski MD, Hafeez I. Sleep disturbance and neurocognitive function during the recovery from a sport-related concussion in adolescents. Am J Sports Med. (2015) 43:633–40. doi: 10.1177/0363546514560727
10. Barlow KM. Postconcussion syndrome: a review. J Child Neurol. (2016) 31:57–67. doi: 10.1177/0883073814543305
11. Kowalczyk CL, Eagle SR, Holland CL, Collins MW, Kontos AP. Average symptom severity and related predictors of prolonged recovery in pediatric patients with concussion. Appl Neuropsychol Child. (2020) 1–5. doi: 10.1080/21622965.2020.1774376. [Epub ahead of print].
12. Davis-Hayes C, Gossett JD, Levine WN, Shams T, Harada J, Mitnick J, et al. Sex-specific outcomes and predictors of concussion recovery. J Am Acad Orthop Surg. (2017) 25:818–28. doi: 10.5435/JAAOS-D-17-00276
13. Teel EF, Marshall SW, Shankar V, McCrea M, Guskiewicz KM. Predicting recovery patterns after sport-related concussion. J Athl Train. (2017) 52:288–98. doi: 10.4085/1062-6050-52.1.12
14. McCrea M, Guskiewicz K, Randolph C, Barr WB, Hammeke TA, Marshall SW, et al. Incidence, clinical course, and predictors of prolonged recovery time following sport-related concussion in high school and college athletes. J Int Neuropsychol Soc. (2013) 19:22. doi: 10.1017/S1355617712000872
15. Lau B, Lovell MR, Collins MW, Pardini J. Neurocognitive and symptom predictors of recovery in high school athletes. Clin J Sport Med. (2009) 19:216–21. doi: 10.1097/JSM.0b013e31819d6edb
16. Pellman EJ, Viano DC, Casson IR, Arfken C, Powell J. Concussion in professional football: injuries involving 7 or more days out—Part 5. Neurosurgery. (2004) 55:1100–19. doi: 10.1227/01.NEU.0000147063.12873.F5
17. Thomas DJ, Coxe K, Li H, Pommering TL, Young JA, Smith GA, et al. Length of recovery from sports-related concussions in pediatric patients treated at concussion clinics. Clin J Sport Med. (2018) 28:56–63. doi: 10.1097/JSM.0000000000000413
18. Eagle SR, Puligilla A, Fazio-Sumrok V, Kegel N, Collins MW, Kontos AP. Association of time to initial clinic visit with prolonged recovery in pediatric patients with concussion. J Neurosurg Pediatr. (2020) 26:1–6. doi: 10.3171/2020.2.PEDS2025
19. Fong A, Allen M, Waltzman D, Sarmiento K, Yeates KO, Suskauer SJ, et al. Neuroimaging in pediatric patients with mild traumatic brain injury: relating the current. CDC guideline and the potential of advanced neuroimaging modalities for research and clinical biomarker development. J Neurotr. (2018) 38:44–52. doi: 10.1089/neu.2020.7100
20. Khong E, Odenwald N, Hashim E, Cusimano MD. Diffusion tensor imaging findings in post-concussion syndrome patients after mild traumatic brain injury: a systematic review. Front Neurol. (2016) 7:156. doi: 10.3389/fneur.2016.00156
21. Shenton ME, Hamoda H, Schneiderman J, Bouix S, Pasternak O, Rathi Y, et al. A review of magnetic resonance imaging and diffusion tensor imaging findings in mild traumatic brain injury. Brain Imaging Behav. (2012) 6:137–92. doi: 10.1007/s11682-012-9156-5
22. Jones DK, Leemans A. Diffusion tensor imaging. Methods Mol Biol. (2011) 711:127–44. doi: 10.1007/978-1-61737-992-5_6
23. Murugavel M, Cubon V, Putukian M, Echemendia R, Cabrera J, Osherson D, et al. A longitudinal diffusion tensor imaging study assessing white matter fiber tracts after sports-related concussion. J Neurotr. (2014) 31:1860–71. doi: 10.1089/neu.2014.3368
24. Smits M, Houston GC, Dippel DW, Wielopolski PA, Vernooij MW, Koudstaal PJ, et al. Microstructural brain injury in post-concussion syndrome after minor head injury. Neuroradiology. (2011) 53:553–63. doi: 10.1007/s00234-010-0774-6
25. D'souza MM, Trivedi R, Singh K, Grover H, Choudhury A, Kaur P, et al. Traumatic brain injury and the post-concussion syndrome: a diffusion tensor tractography study. Indian J Radiol Imaging. (2015) 25:404–14. doi: 10.4103/0971-3026.169445
26. Fakhran S, Yaeger K, Collins M, Alhilali L. Sex differences in white matter abnormalities after mild traumatic brain injury: localization and correlation with outcome. Radiology. (2014) 272:815–23. doi: 10.1148/radiol.14132512
27. Mustafi SM, Harezlak J, Koch KM, Nencka AS, Meier TB, West JD, et al. Acute white-matter abnormalities in sports-related concussion: a diffusion tensor imaging study from the NCAA-DoD CARE Consortium. J Neurotr. (2018) 35:2653–64. doi: 10.1089/neu.2017.5158
28. Cubon VA, Murugavel M, Holmes KW, Dettwiler A. Preliminary evidence from a prospective DTI study suggests a posterior-to-anterior pattern of recovery in college athletes with sports-related concussion. Brain Behav. (2018) 8:e01165. doi: 10.1002/brb3.1165
29. Virji-Babul N, Borich MR, Makan N, Moore T, Frew K, Emery CA, et al. Diffusion tensor imaging of sports-related concussion in adolescents. Pediatr Neurol. (2013) 48:24–9. doi: 10.1016/j.pediatrneurol.2012.09.005
30. Babcock L, Yuan W, Leach J, Nash T, Wade S. White matter alterations in youth with acute mild traumatic brain injury. J Pediatr Rehabil Med. (2015) 8:285–96. doi: 10.3233/PRM-150347
31. Chu Z, Wilde E, Hunter J, McCauley S, Bigler E, Troyanskaya M, et al. Voxel-based analysis of diffusion tensor imaging in mild traumatic brain injury in adolescents. AJNR Am J Neuroradiol. (2010) 31:340–6. doi: 10.3174/ajnr.A1806
32. Bazarian JJ, Zhu T, Blyth B, Borrino A, Zhong J. Subject-specific changes in brain white matter on diffusion tensor imaging after sports-related concussion. Magn Reson Imaging. (2012) 30:171–80. doi: 10.1016/j.mri.2011.10.001
33. Mayer AR, Ling JM, Yang Z, Pena A, Yeo RA, Klimaj S. Diffusion abnormalities in pediatric mild traumatic brain injury. J Neurosci. (2012) 32:17961–9. doi: 10.1523/JNEUROSCI.3379-12.2012
34. King R, Grohs MN, Kirton A, Lebel C, Esser MJ, Barlow KM. Microstructural neuroimaging of white matter tracts in persistent post-concussion syndrome: a prospective controlled cohort study. Neuroimage Clin. (2019) 23:101842. doi: 10.1016/j.nicl.2019.101842
35. Wasserthal J, Neher P, Maier-Hein KH. Tractseg-fast and accurate white matter tract segmentation. NeuroImage. (2018) 183:239–53. doi: 10.1016/j.neuroimage.2018.07.070
36. Wasserthal J, Neher PF, Maier-Hein KH. International Conference on Medical Image Computing and Computer-Assisted Intervention. Springer (2018). p. 36–44. doi: 10.1007/978-3-030-00931-1_5
37. Covassin T, Elbin RJ, Stiller-Ostrowski JL, Kontos AP. Immediate post-concussion assessment and cognitive testing (ImPACT) practices of sports medicine professionals. J Athl Train. (2009) 44:639–44. doi: 10.4085/1062-6050-44.6.639
38. Mucha A, Collins MW, Elbin R, Furman JM, Troutman-Enseki C, DeWolf RM, et al. A brief vestibular/ocular motor screening (VOMS) assessment to evaluate concussions: preliminary findings. Am J Sports Med. (2014) 42:2479–86. doi: 10.1177/0363546514543775
39. Kontos AP, Elbin R, Schatz P, Covassin T, Henry L, Pardini J, et al. A revised factor structure for the post-concussion symptom scale: baseline and postconcussion factors. Am J Sports Med. (2012) 40:2375–84. doi: 10.1177/0363546512455400
40. Patton JH, Stanford MS, Barratt ES. Factor structure of the Barratt impulsiveness scale. J Clin Psychol. (1995) 51:768–74. doi: 10.1002/1097-4679(199511)51:6<768::aid-jclp2270510607>3.0.co;2-1
41. Birmaher B, Brent DA, Chiappetta L, Bridge J, Monga S, Baugher M. Psychometric properties of the screen for child anxiety related emotional disorders (SCARED): a replication study. J Am Acad Child Adolesc Psychiatry. (1999) 38:1230–6. doi: 10.1097/00004583-199910000-00011
42. Gerson AC, Gerring JP, Freund L, Joshi PT, Capozzoli J, Brady K, et al. The Children's affective lability scale: a psychometric evaluation of reliability. Psychiatry Res. (1996) 65:189–98. doi: 10.1016/S0165-1781(96)02851-X
43. Poznanski EO, Mokros HB. Children's Depression Rating Scale, Revised (CDRS-R). Western Psychological Services Los Angeles (1996).
44. Andersson JL, Skare S, Ashburner J. How to correct susceptibility distortions in spin-echo echo-planar images: application to diffusion tensor imaging. Neuroimage. (2003) 20:870–88. doi: 10.1016/S1053-8119(03)00336-7
45. Smith SM, Jenkinson M, Woolrich MW, Beckmann CF, Behrens TE, Johansen-Berg H, et al. Advances in functional and structural MR image analysis and implementation as FSL. Neuroimage. (2004) 23:S208–19. doi: 10.1016/j.neuroimage.2004.07.051
46. Tournier J-D, Calamante F, Connelly A. Robust determination of the fibre orientation distribution in diffusion MRI: non-negativity constrained super-resolved spherical deconvolution. Neuroimage. (2007) 35:1459–72. doi: 10.1016/j.neuroimage.2007.02.016
47. Jeurissen B, Tournier J-D, Dhollander T, Connelly A, Sijbers J. Multi-tissue constrained spherical deconvolution for improved analysis of multi-shell diffusion MRI data. NeuroImage. (2014) 103:411–26. doi: 10.1016/j.neuroimage.2014.07.061
48. Tournier J-D, Smith R, Raffelt D, Tabbara R, Dhollander T, Pietsch M, et al. MRtrix3: A fast, flexible and open software framework for medical image processing and visualisation. NeuroImage. (2019) 2019:116137. doi: 10.1016/j.neuroimage.2019.116137
49. Kochunov P, Williamson DE, Lancaster J, Fox P, Cornell J, Blangero J, et al. Fractional anisotropy of water diffusion in cerebral white matter across the lifespan. Neurobiol Aging. (2012) 33:9–20. doi: 10.1016/j.neurobiolaging.2010.01.014
50. Lebel C, Gee M, Camicioli R, Wieler M, Martin W, Beaulieu C. Diffusion tensor imaging of white matter tract evolution over the lifespan. Neuroimage. (2012) 60:340–52. doi: 10.1016/j.neuroimage.2011.11.094
51. Westlye LT, Walhovd KB, Dale AM, Bjornerud A, Due-Tonnessen P, Engvig A, et al. Life-span changes of the human brain white matter: diffusion tensor imaging (DTI) and volumetry. Cereb Cortex. (2010) 20:2055–68. doi: 10.1093/cercor/bhp280
52. Simmonds DJ, Hallquist MN, Asato M, Luna B. Developmental stages and sex differences of white matter and behavioral development through adolescence: a longitudinal diffusion tensor imaging (DTI) study. Neuroimage. (2014) 92:356–68. doi: 10.1016/j.neuroimage.2013.12.044
53. Benjamini Y, Hochberg Y. Controlling the false discovery rate: a practical and powerful approach to multiple testing. J R Stat Soc. (1995) 57:289–300. doi: 10.1111/j.2517-6161.1995.tb02031.x
54. Robin X, Turck N, Hainard A, Tiberti N, Lisacek F, Sanchez J-C, et al. pROC: an open-source package for R and S+ to analyze and compare ROC curves. BMC Bioinformatics. (2011) 12:1–8. doi: 10.1186/1471-2105-12-77
55. Core Team R. R: A Language and Environment for Statistical Computing. R Foundation for Statistical Computing. Vienna, Austria. Available online at: https://www R-project org/[Google Scholar]. (2017).
56. Herbet G, Zemmoura I, Duffau H. Functional anatomy of the inferior longitudinal fasciculus: from historical reports to current hypotheses. Front Neuroanat. (2018) 12:77. doi: 10.3389/fnana.2018.00077
57. Lebel C, Walker L, Leemans A, Phillips L, Beaulieu C. Microstructural maturation of the human brain from childhood to adulthood. Neuroimage. (2008) 40:1044–55. doi: 10.1016/j.neuroimage.2007.12.053
58. Dubois J, Dehaene-Lambertz G, Perrin M, Mangin JF, Cointepas Y, Duchesnay E, et al. Asynchrony of the early maturation of white matter bundles in healthy infants: quantitative landmarks revealed noninvasively by diffusion tensor imaging. Hum Brain Mapp. (2008) 29:14–27. doi: 10.1002/hbm.20363
59. Lambon Ralph MA, Cipolotti L, Manes F, Patterson K. Taking both sides: do unilateral anterior temporal lobe lesions disrupt semantic memory? Brain. (2010) 133:3243–55. doi: 10.1093/brain/awq264
60. Rice GE, Hoffman P, Ralph MAL. Graded specialization within and between the anterior temporal lobes. Ann N Y Acad Sci. (2015) 1359:84–97. doi: 10.1111/nyas.12951
61. McKinnon ET, Fridriksson J, Glenn GR, Jensen JH, Helpern JA, Basilakos A, et al. Structural plasticity of the ventral stream and aphasia recovery. Ann Neurol. (2017) 82:147–51. doi: 10.1002/ana.24983
62. Duffau H, Gatignol P, Mandonnet E, Peruzzi P, Tzourio-Mazoyer N, Capelle L. New insights into the anatomo-functional connectivity of the semantic system: a study using cortico-subcortical electrostimulations. Brain. (2005) 128:797–810. doi: 10.1093/brain/awh423
63. Martino J, Brogna C, Robles SG, Vergani F, Duffau H. Anatomic dissection of the inferior fronto-occipital fasciculus revisited in the lights of brain stimulation data. Cortex. (2010) 46:691–9. doi: 10.1016/j.cortex.2009.07.015
64. Wu Y, Sun D, Wang Y, Wang Y. Subcomponents and connectivity of the inferior fronto-occipital fasciculus revealed by diffusion spectrum imaging fiber tracking. Front Neuroanat. (2016) 10:88. doi: 10.3389/fnana.2016.00088
65. Von Der Heide RJ, Skipper LM, Klobusicky E, Olson IR. Dissecting the uncinate fasciculus: disorders, controversies and a hypothesis. Brain. (2013) 136:1692–707. doi: 10.1093/brain/awt094
66. Sherbondy AJ, Dougherty RF, Napel S, Wandell BA. Identifying the human optic radiation using diffusion imaging and fiber tractography. J Vis. (2008) 8:12.1–11. doi: 10.1167/8.10.12
67. Urger SE, De Bellis MD, Hooper SR, Woolley DP, Chen SD, Provenzale J. The superior longitudinal fasciculus in typically developing children and adolescents: diffusion tensor imaging and neuropsychological correlates. J Child Neurol. (2015) 30:9–20. doi: 10.1177/0883073813520503
68. Klarborg B, Skak Madsen K, Vestergaard M, Skimminge A, Jernigan TL, Baaré WF. Sustained attention is associated with right superior longitudinal fasciculus and superior parietal white matter microstructure in children. Hum Brain Mapp. (2013) 34:3216–32. doi: 10.1002/hbm.22139
69. Wu T, Merkley TL, Wilde EA, Barnes A, Li X, Chu ZD, et al. A preliminary report of cerebral white matter microstructural changes associated with adolescent sports concussion acutely and subacutely using diffusion tensor imaging. Brain Imaging Behav. (2018) 12:962–73. doi: 10.1007/s11682-017-9752-5
70. Lynch KM, Cabeen RP, Toga AW, Clark KA. Magnitude and timing of major white matter tract maturation from infancy through adolescence with NODDI. NeuroImage. (2020) 212:116672. doi: 10.1016/j.neuroimage.2020.116672
71. Wu Y-C, Harezlak J, Elsaid NM, Lin Z, Wen Q, Mustafi SM, et al. Longitudinal white-matter abnormalities in sports-related concussion: a diffusion MRI study. Neurology. (2020) 95:e781–92. doi: 10.1212/WNL.0000000000009930
72. Liu J, Kou Z, Tian Y. Diffuse axonal injury after traumatic cerebral microbleeds: an evaluation of imaging techniques. Neural Regen Res. (2014) 9:1222–30. doi: 10.4103/1673-5374.135330
73. Meaney DF, Smith DH. Biomechanics of concussion. Clin Sports Med. (2011) 30:19–31. doi: 10.1016/j.csm.2010.08.009
74. Yuh EL, Hawryluk GW, Manley GT. Imaging concussion: a review. Neurosurgery. (2014). 75(suppl_4):S50–63. doi: 10.1227/NEU.0000000000000491
75. Schilling S, Mansour A, Sullivan L, Ding K, Pommering T, Yang J. Symptom burden and profiles in concussed children with and without prolonged recovery. Int J Environ Res Public Health. (2020) 17:351. doi: 10.3390/ijerph17010351
76. Sufrinko AM, Marchetti GF, Cohen PE, Elbin R, Re V, Kontos AP. Using acute performance on a comprehensive neurocognitive, vestibular, and ocular motor assessment battery to predict recovery duration after sport-related concussions. Am J Sports Med. (2017) 45:1187–94. doi: 10.1177/0363546516685061
Keywords: adolescence, concussion, predictors, recovery, diffusion MRI
Citation: Lima Santos JP, Kontos AP, Mailliard S, Eagle SR, Holland CL, Suss SJ Jr, Abdul-waalee H, Stiffler RS, Bitzer HB, Blaney NA, Colorito AT, Santucci CG, Brown A, Kim T, Iyengar S, Skeba A, Diler RS, Ladouceur CD, Phillips ML, Brent D, Collins MW and Versace A (2021) White Matter Abnormalities Associated With Prolonged Recovery in Adolescents Following Concussion. Front. Neurol. 12:681467. doi: 10.3389/fneur.2021.681467
Received: 16 March 2021; Accepted: 27 May 2021;
Published: 24 June 2021.
Edited by:
Bridgette D. Semple, Monash University, AustraliaReviewed by:
Ralph George Depalma, United States Department of Veterans Affairs, United StatesCarlo Augusto Mallio, Campus Bio-Medico University, Italy
Copyright © 2021 Lima Santos, Kontos, Mailliard, Eagle, Holland, Suss Jr., Abdul-waalee, Stiffler, Bitzer, Blaney, Colorito, Santucci, Brown, Kim, Iyengar, Skeba, Diler, Ladouceur, Phillips, Brent, Collins and Versace. This is an open-access article distributed under the terms of the Creative Commons Attribution License (CC BY). The use, distribution or reproduction in other forums is permitted, provided the original author(s) and the copyright owner(s) are credited and that the original publication in this journal is cited, in accordance with accepted academic practice. No use, distribution or reproduction is permitted which does not comply with these terms.
*Correspondence: João Paulo Lima Santos, c2FudG9zanBAdXBtYy5lZHU=