- 1Department of Neurology, Affiliated Brain Hospital of Nanjing Medical University, Nanjing, China
- 2Department of Neurology, Affiliated to Nanjing Medical University, Nanjing, China
- 3Nanjing Medical University, Nanjing, China
Background: Recent studies have discovered that functional connections are impaired among patients with Alzheimer's disease (AD), even at the preclinical stage. The cerebellum has been implicated as playing a role in cognitive processes. However, functional connectivity (FC) among cognitive sub-regions of the cerebellum in patients with AD and mild cognitive impairment (MCI) remains to be further elucidated.
Objective: Our study aims to investigate the FC changes of the cerebellum among patients with AD and MCI, compared to healthy controls (HC). Additionally, we explored the role of cerebellum FC changes in the cognitive performance of all subjects.
Materials: Resting-state functional magnetic resonance imaging (rs-fMRI) data from three different groups (28 AD patients, 26 MCI patients, and 30 HC) was collected. We defined cerebellar crus II and lobule IX as seed regions to assess the intragroup differences of cortico-cerebellar connectivity. Bias correlational analysis was performed to investigate the relationship between changes in FC and neuropsychological performance.
Results: Compared to HC, AD patients had decreased FC within the caudate, limbic lobe, medial frontal gyrus (MFG), middle temporal gyrus, superior frontal gyrus, parietal lobe/precuneus, inferior temporal gyrus, and posterior cingulate gyrus. Interestingly, MCI patients demonstrated increased FC within inferior parietal lobe, and MFG, while they had decreased FC in the thalamus, inferior frontal gyrus, and superior frontal gyrus. Further analysis indicated that FC changes between the left crus II and the right thalamus, as well as between left lobule IX and the right parietal lobe, were both associated with cognitive decline in AD. Disrupted FC between left crus II and right thalamus, as well as between left lobule IX and right parietal lobe, was associated with attention deficit among subjects with MCI.
Conclusion: These findings indicate that cortico-cerebellar FC in MCI and AD patients was significantly disrupted with different distributions, particularly in the default mode networks (DMN) and fronto-parietal networks (FPN) region. Increased activity within the fronto-parietal areas of MCI patients indicated a possible compensatory role for the cerebellum in cognitive impairment. Therefore, alterations in the cortico-cerebellar FC represent a novel approach for early diagnosis and a potential therapeutic target for early intervention.
Introduction
Alzheimer's disease (AD), the most common neurodegenerative dementia, is characterized by a progressive deterioration of cognitive functions, as well as changes in behavior and personality (1, 2). Amnesic mild cognitive impairment (aMCI) has been recognized as a transition stage between normal cognitive function and AD-type dementia, which has a chance of progression to AD up to 25% per year (3). Resting-state functional magnetic resonance imaging (fMRI) is reflecting the synchronization of functional activity between distant brain regions by observing the brain low frequency fluctuations in the blood-oxygen level-dependent (BOLD) signals, which is widely used in diagnosis and predict the disease progression of AD (4–6). The application of resting-state fMRI techniques has revealed imaging features of AD with regards to brain structure (7, 8). Studies have shown that functional connections and brain networks are impaired as early as the aMCI stage (5). In the preclinical stage of AD, the hippocampus, visual cortex and frontal lobe have been decoupled, and the enhanced connections between the middle cingulate gyrus (MCC), the precuneus gyrus (PCU), the posterior cingulate gyrus (PCC), and the cerebellum are the internal mechanisms of ad functional compensation. After entering MCI stage, PCU can no longer compensate for the decompensation of AD susceptible areas such as hippocampus, but the connection between cerebellum, MCC and PCC is enhanced, which can be maintained until dementia stage (9, 10). AD progression can be delayed via early diagnosis and intervention. Resting state fMRI can be more sensitive to explore the brain network changes of early AD (11, 12). Previous studies have focused on the cerebral cortex, and less research has been focused on the role of the cerebellum in cognitive regulation of the AD spectrum (13, 14).
Recent studies have implicated the role of cerebellum in cognitive processes (15–19). Cerebellar cognitive affective syndrome (CCAS) is characterized by executive dysfunction, spatial cognitive impairment, language deficits, and personality changes (20–22). The human cerebellar cortex is a complicated structure, as the surface of it is more tightly folded than the cerebral cortex, and has almost 80% of the surface area of the neocortex, and the nerve fiber connections to the brain's cognitive network is extensive (23), indicating that the cerebellum plays an important role in the evolution of behavior and cognition. Furthermore, the cerebellar lobular volumes, as well as the cortico-cerebellar FC, was found to decrease with age, leading to cognitive decline among the healthy elderly people (24, 25). Previous studies have suggested that the cerebellum is a survivor of preclinical AD stage process and remains virtually unaffected (26, 27). However, recent studies have found that as the disease progresses, the structure, as well as the function of the cerebellum also changes (28).
With the progression of AD, the cerebellar gray matter volume changes in a continuum with posterior-to-anterior cerebellar lobe development. The vermis and paravermian lobes of anterior lobe (I-V) and posterior lobe (VI) were mainly involved in aMCI, and the hemispheric part of posterior lobe (VI lobule) and Crus I were involved in AD. GM atrophy of Crus I will cause functional damage, which becomes more obvious with the increase of disease severity (29). Another study shows that the FC between the dentate nucleus (DN) and lateral temporal regions was increased in AD patients compared to controls, when using cerebellar DN as a region of interest, which suggests that FC changes within specific cerebellar-cortical functional modules is involved in cognitive impairment among AD patients (30). The DN is involved in planning and execution of random movements, as well as higher cognitive and sensory processing. It is also a key area involved in integration and regulating cerebral-cerebellar networks. The dorsolateral prefrontal lobe of the cerebral cortex is involved in working memory, decision-making, time processing, and other cognitive functions (31). After an injection of a trans-neuronal retrograde tracer to the dorsolateral prefrontal lobe, a small number of Purkinje cells were labeled in the lateral region of Crus II (32) (along with Crus I, a hemispheric extension of lobule VIIa), as well as in the normal portions of lobule X and lobule VII. This suggests the existence of a “cognitive” loop between dorsolateral prefrontal lobe and specific cerebellar cortical areas (33).
An increasing number of imaging studies have confirmed that cerebellar Crus II and lobular IX are associated with cognitive networks (32, 34, 35), particularly with the default mode network (DMN) and fronto-parietal network (FPN) (36–38). The anterior cerebellum and lobule VIII are associated with movement, whereas the posterior cerebellum (i.e., lobule VI-Crus I, lobule Crus II-VIIB, and lobule IX) are critical for cognitive representation (39–41). Lobule VI, VIIB, and Crus I are specifically involved in executive functions, including working memory, planning, organizing, and strategy formation, all of which are important for creative divergent thinking (42–45). Visual divergent thinking is an approach to a situation or concept that focuses on exploring as many aspects of the visual concept as possible, and it is a primary component of fields such as photography, drawing, architecture and sculpture, which is significantly associated with activity in the left lobule VI, VIIB, Crus I, and Crus II, and is associated with executive function (46). Previous studies have established that working memory task processing and front-oparietal network connectivity simultaneously engaged lobule VI/Crus I, Crus II/lobule VIIB, and lobule IX with the DMN (32, 44, 47). Bai et al. used a resting-state fMRI to explore spontaneous activation of the cerebellum and found significant differences in lobule IX and Crus II in the posterior cerebellum of aMCI patients compared to controls (36). Therefore, bilateral Crus II and lobule IX were chosen herein as regions of interest to study FC characteristics of the cerebellum in patients with aMCI and AD.
However, the characteristics and differences of cerebellar FC in MCI and AD patients remains unknown. This present study was conducted to analyze alterations in cerebellar-cortical FC and whether these alterations were associated with clinical cognitive impairment in MCI and AD patients.
Materials and Methods
Participants
All study subjects were recruited from the Nanjing Brain Hospital between June 2018 to October 2020. All subjects were right-handed, and included 27 AD subjects (11 males and 16 females), 25 MCI subjects (9 males and 16 females), and 13 healthy controls were not different statistically in terms of age and sex (7 males and 6 females). The diagnosis of AD and MCI was carried out according to the National Institute on Aging and the Alzheimer's Association working group (NIA-A) in 2011 (3, 48). All patients underwent clinical and neuropsychological assessment, MRI scans, and cerebrospinal fluid (CSF) analysis. The inclusion criteria for health controls (HC) included (1) no current cognitive issues, (2) no neurological or psychiatric diseases, and (3) a clinical dementia rating (CDR) score of 0 (49). Exclusion criteria for both groups included (1) other known causes of dementia (i.e., frontotemporal dementia, dementia with Lewy bodies, vascular dementia, severe depression, cerebrovascular disease, tumors, poisoning, metabolic diseases, and infections), and (2) contraindications to undergoing an MRI, such as claustrophobia or pacemaker implantation.
All participants provided a written informed consent and the study was granted approval by the Medical Research Ethical Committee of Nanjing Brain Hospital in Nanjing, China.
Clinical and Neuropsychological Assessments
All participants underwent comprehensive and standard neuropsychological assessments in order to evaluate their cognitive function. Global cognition was evaluated using the mini-mental state examination (MMSE) (50), the Montreal cognitive assessment (MoCA) (51), and the CDR (52). Episodic memory was assessed using the auditory verbal learning test (AVLT) (53). Visuospatial abilities were evaluated with the clock-drawing test (CDT) (54). Language function was determined using the Boston naming test (BNT) and the verbal fluency test (VFT-animals). Executive function was assessed using part A and B of the trail making test (TMT), as well as the symbol digit modalities test (SDMT). Verbal working memory was determined using the digit span test (DST) (55). The emotional condition of the subjects were determined using the Hamilton Depression (HAMD) (56). These scales were validated by senior neuropsychologists and evaluated by experienced clinicians.
Cerebrospinal Fluid Biomarkers
CSF Aβ1–42, t-tau, and p-tau were measured using INNOBIA AlzBio3 immunoassay kit-based reagents (Innotest, Fujirebio, Ghent, Belgium). Notably, not all participants had CSF sample data since lumbar puncture is an invasive operation. In this study, 25AD subjects and 27 MCI subjects had CSF sample data available.
Magnetic Resonance Imaging Data Acquisition
Magnetic resonance imaging (MRI) was acquired utilizing a Siemens 3.0 T singer scanner (Siemens, Verio, Germany) with an 8-channel radio frequency coil at the Affiliated Brain Hospital of Nanjing Medical University. Participants were asked to remain as still as possible, close their eyes, remain awake, and to not think of anything. T1WI was acquired through application of a three-dimensional magnetization prepared rapid gradient echo (3D-MPRAGE). The parameters included time repetition (TR) = 1,900 ms, echo time (TE) = 2.48 ms, inversion time (TI) = 900 ms, number of slices = 176, thickness = 1.0 mm, gap = 0.5 mm, matrix = 256 × 256, flip angle (FA) = 9?, field of view (FOV) = 256 × 256 mm, and voxel size = 1 × 1 × 1 mm3. Resting-state fMRI acquisition was applied using single echo planar imaging (EPI). The gradient echo-echo planar imaging (GRE-EPI) sequence included 240 time points. TE = 30 ms; TR = 2,000 ms; number of slices = 36, FOV = 220 × 220 mm2; matrix = 64 × 64; FA = 90°; thickness = 4.0 mm, gap = 0 mm. The imaging for each subject took ~ 14 min.
Data Preprocessing
The fMRI data were processed using the Data Processing and Analysis for Brain Imaging (DPABI, http://www.rest.restfmri.net) (57). The first 10 volumes of the rest session were discarded for each subject. The remaining images were corrected utilizing slice timing and motion (head motion ≤ 3 mm, head motion angle ≤ 3°). Next, resting-state fMRI images were co-registered to high-resolution 3D-T1 structural images. Normalization of 3D-T1 structural MRI images to Montreal Neurological Institute (MNI) space was undertaken via non-linear warping based on Diffeomorphic Anatomical Registration Through Exponentiated Lie Algebra (DARTEL). After spatial normalization to T1 space, all images were resampled into 3 × 3 × 3 mm3 voxels and spatially smoothed using a Gaussian filter of 6 mm full-width at half-maximum (FWHM). Data was then temporally band-pass-filtered (0.01–0.08 Hz) in order to eliminate low-frequency drifts and physiological high-frequency noise. Furthermore, to reduce the confounding artifacts of resting head movements and physiological noise (respiration and cardiac fluctuations), nuisance covariates were regressed out, including the Friston 24-motion parameter model, global mean, white matter, and cerebrospinal fluid signals.
Functional Connectivity Analysis
The bilateral cerebellar Crus II and lobule IX were extracted as regions of interest (ROI) utilizing the DPABI software package template (anatomical automatic labeling, AAL) in order to localize the two ROIs, respectively (Figure 1).
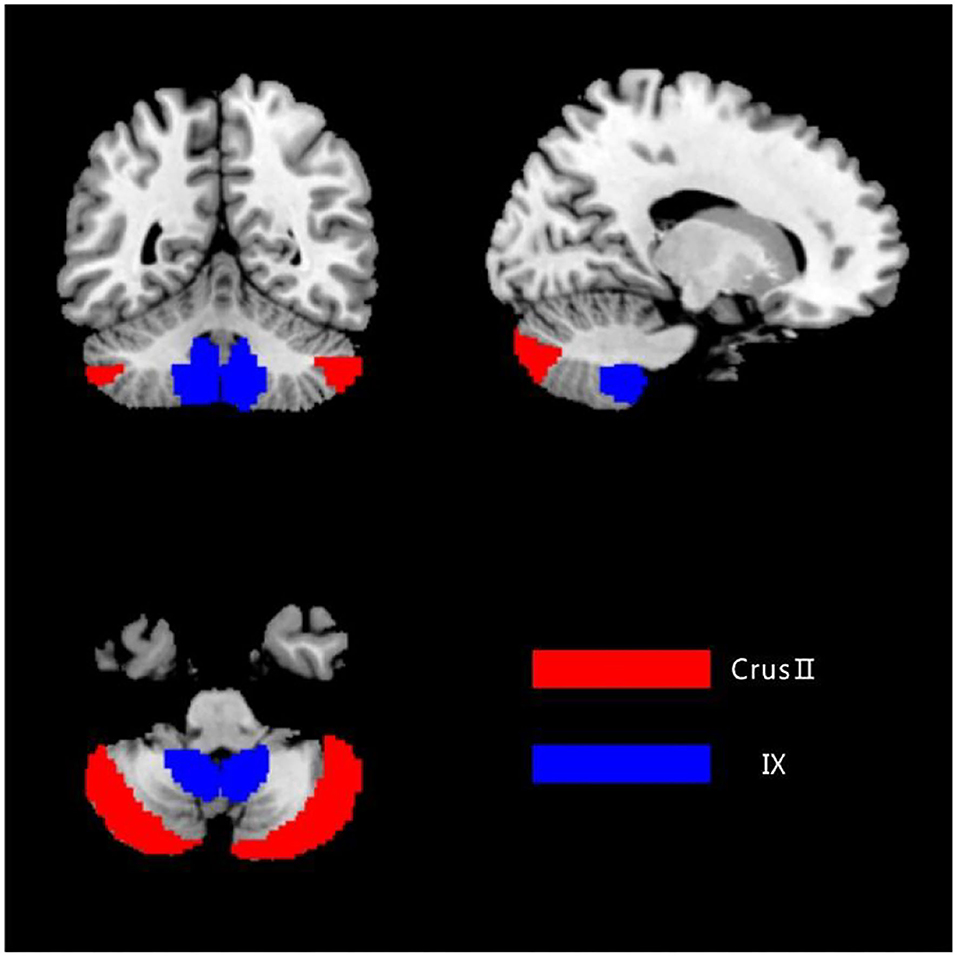
Figure 1. The ROIs of the cerebellum. The image was transformed into the space of the SUIT atlas and was overlapped by the seeds. Red color represents the Crus II, and blue color represents the posterior lobule IX.
FC analysis was performed between each seed region, as well as the whole brain, in a voxel-wise manner using the DPABI software. The voxels of each seed region of every subject were extracted and averaged in order to obtain the reference time series of seed points. Then, we calculated the correlation coefficient between the reference time series and the time series involving all other brain voxels. The correlation coefficients were transformed into z-values using the Fisher r-to-z transformation, leading to an improvement in normality.
Statistical Analysis
All data was tested for normality and variance congruence. Normally distributed data was expressed as mean ± standard deviation. The ANOVA and chi-square test were performed to compare the demographic and neurocognitive data among the groups (AD, MCI, and HC). Bonferroni correction was used for post-hoc comparisons. Statistical analyses were performed using IBM SPSS 25.0 software (SPSS Inc., Chicago, Illinois, USA). A two-sided P < 0.05 represented statistical significance.
In order to determine the differences of whole-brain resting-state FC of each cerebellar seed region, we conducted statistical analyses across the three groups was conducted utilizing ANOVA, with age, sex, years of education, and gray matter volume used as covariates. Gray matter volume sequence was extracted by REST Toolkit (http://www.restfmri.net). The multiple comparisons of ANOVA results were corrected using AlphaSim with a significance threshold of P < 0.05 (cluster size > 100 voxels, and voxel-level P < 0.05; determined by a Monte Carlo simulation). Post-hoc two-sample t tests were corrected by Bonferroni. P < 0.05 was statistically significant.
Then, partial bias correlation analysis was utilized to explore the relationship between resting-state FC among different brain regions with the clinical neuropsychological score, using gender, age, and education level as covariates (P < 0.05, Bonferroni-corrected).
Results
Demographic and Clinical Characteristics
The demographic and clinical characteristics of each participant are indicated in Table 1. There were no significant differences with regards to age, gender, or education among the three groups. In contrast, there were significant differences for each cognitive domain. Overall cognitive levels, episodic memory ability, executive ability, verbal ability, and visuospatial function were significantly lower in the AD group compared to both the MCI and HC group. There was also a significant decrease in the above cognitive domains in the MCI group compared to the HC group (p < 0.001).
Functional Connectivity
Functional Connectivity Changes of the Left Crus II
Using left crus II as the ROI, whole-brain FC analysis revealed that FC values of the right caudate (CAU), left limbic lobe (LIM), and right medial frontal gyrus (MFG) were reduced in AD patients compared to HC. Furthermore, FC with left thalamus (THAL) was decreased in the MCI group, but increased in the right cerebellum posterior lobe (CPL) compared to the HC group. There were no significant differences in left-sided crus II and whole brain FC between the AD and MCI groups (Table 2 and Figure 2).
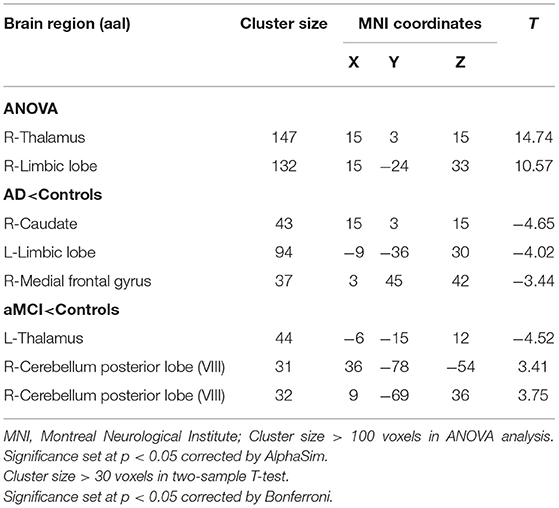
Table 2. Regions showing resting state functional connectivity (rs-FC) changes in cerebellum left crus II.
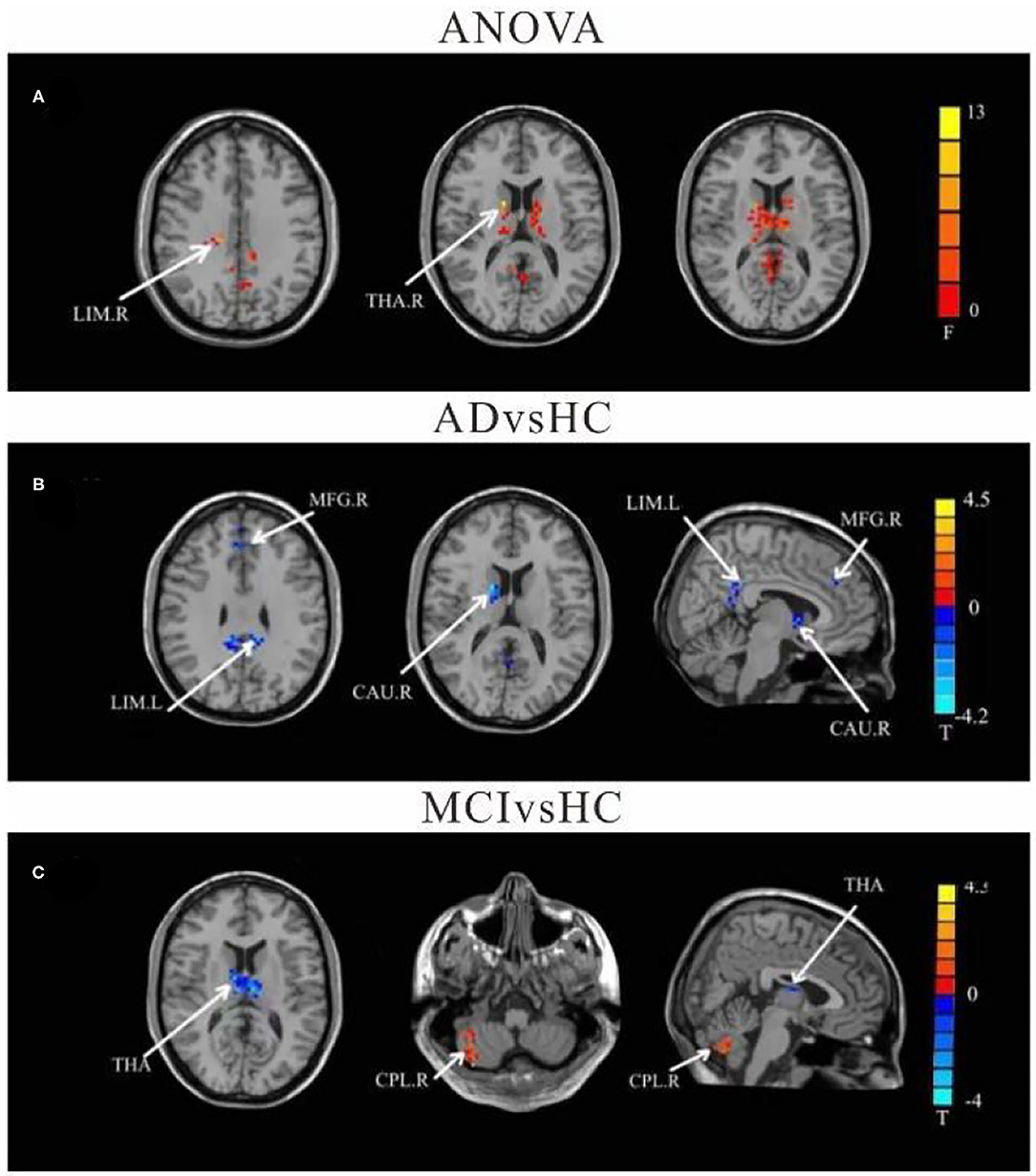
Figure 2. Differences of FC values in the cerebellum left crus II. (A) Brain regions showing significant differences in FC of the left cerebellum crus II based on analysis of variance (ANOVA) analysis between HC, AD, and aMCI, p < 0.05, the cluster size > 100 voxels). (B,C) Results of post-hoc two-sample T-tests in voxel-wise analysis (Bonferroni corrected, cluster size ≥ 30 voxels, p < 0.05). AD, Alzheimer's disease; aMCI, amnestic mild cognitive impairment; HC, healthy controls; LIM, limbic lobe; THA, Thalamus; MFG, Middle Frontal Gyrus; CAU, Caudate; CPL, cerebellum posterior lobe; L, left; R, right.
Functional Connectivity Changes of the Right Crus II
Compared to HC, the FC values in AD between right crus II and left middle temporal gyrus (MTG), left MFG, right CAU, left superior frontal gyrus (SFG), and left LIM were significantly decreased. Among individuals with MCI, decreased FC was indicated between the right crus II with left inferior frontal gyrus (IFG) and left superior frontal gyrus (SFG), while the right CPL FC were increased among individuals with MCI. The FC changes in right crus II and inferior parietal lobe (IPL) was decreased in AD and MCI patients (Table 3 and Figure 3).
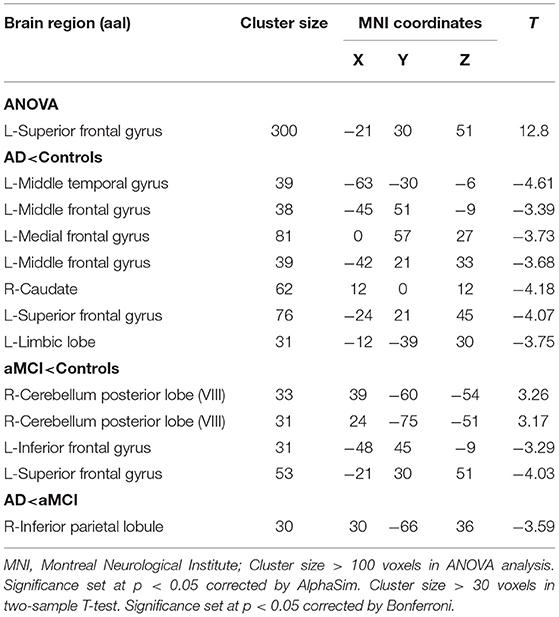
Table 3. Regions showing resting state functional connectivity (rs-FC) changes in cerebellum right crus II.
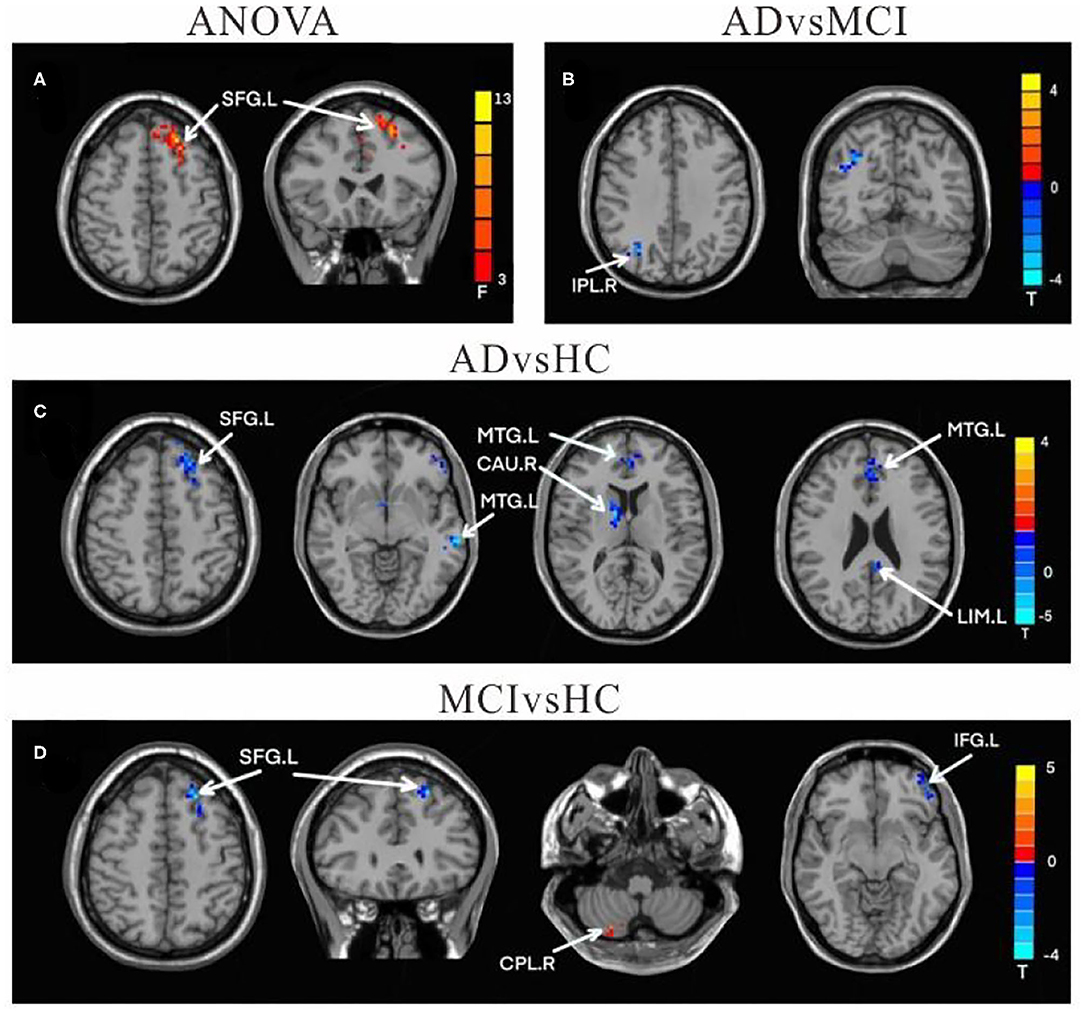
Figure 3. Differences of FC values in the right cerebellum Crus II. (A) Brain regions showing significant differences in FC of the right cerebellum Crus II based on analysis of variance (ANOVA) analysis between HC, AD, and aMCI patients, p < 0.05, the cluster size > 100 voxels). (B–D) The results of post-hoc two-sample T-tests in voxel-wise analysis (Bonferroni corrected, cluster size ≥ 30 voxels, p < 0.05). AD, Alzheimer's disease; aMCI, amnestic mild cognitive impairment; HC, healthy controls; SFG, superior frontal gyrus; IPL, inferior parietal lobe; MFG, Middle Frontal Gyrus; CAU, Caudate; LIM, limbic lobe; CPL, cerebellum posterior lobe; IFG, inferior frontal lobe; L, left; R, right.
Functional Connectivity Changes of the Left Lobule IX
In comparison to HC, the FC value between cerebellum lobule IX and right parietal lobe/precuneus (PCU) was remarkably reduced in the AD group. In contrast, FC values in left lobule IX and right cerebellum anterior lobe (CAL) were significantly increased in MCI patients compared to HC. There were no significant differences in FC values of left-sided lobule IX and whole brain between the AD and MCI groups (Table 4 and Figure 4).
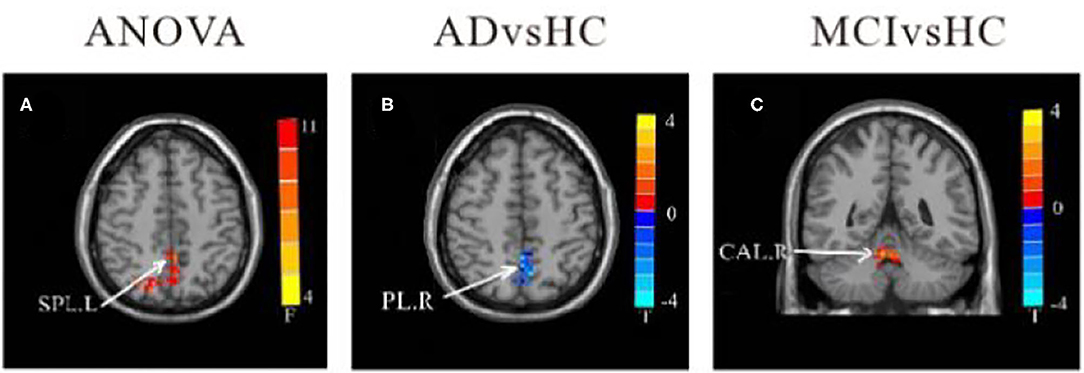
Figure 4. Differences of FC values in the cerebellum left lobule IX. (A) Brain regions showing significant differences in FC of the cerebellum left lobule IX based on analysis of variance (ANOVA) analysis between HC, AD, and aMCI (p < 0.05; the cluster size > 100 voxels). (B,C) The results of post-hoc two-sample T-tests in voxel-wise analysis (Bonferroni corrected, cluster size ≥ 30 voxels, p < 0.05). AD, Alzheimer's disease; aMCI, amnestic mild cognitive impairment; HC, healthy controls; SPL, superior parietal lobe; PL, parietal lobe; CAL, cerebellum Anterior lobe; L, left; R, right.
Functional Connectivity Changes of the Right Lobule IX
Compared to HC, the FC between right-sided lobule IX and left inferior temporal gyrus (ITG), left LIM/Posterior Cingulate Gyrus (PCG), left MFG, and right SFG was decreased among all AD patients. However, in comparison to HC, the MCI group had increased FC between the right-sided lobule IX and right parietal lobe/postcentral gyrus, right MFG, right CPL, and right CAL, while there was decreased FC between the right lobule IX and left SFG FC values (Table 5 and Figure 5).
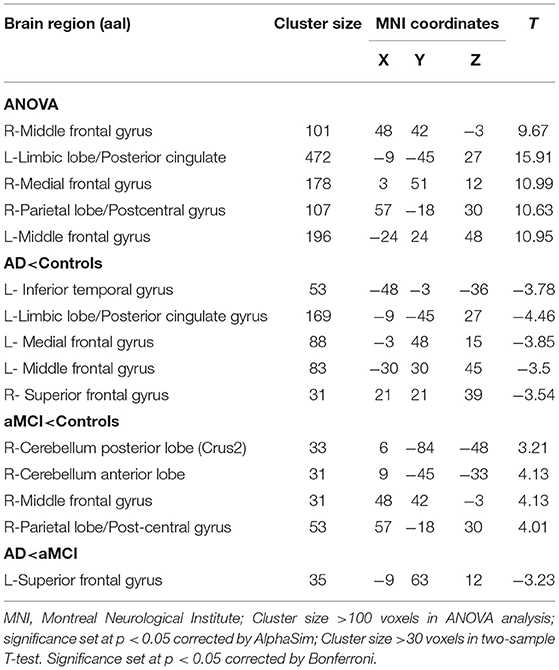
Table 5. Regions showing resting state functional connectivity (rsFC) in cerebellum right lobule IX.
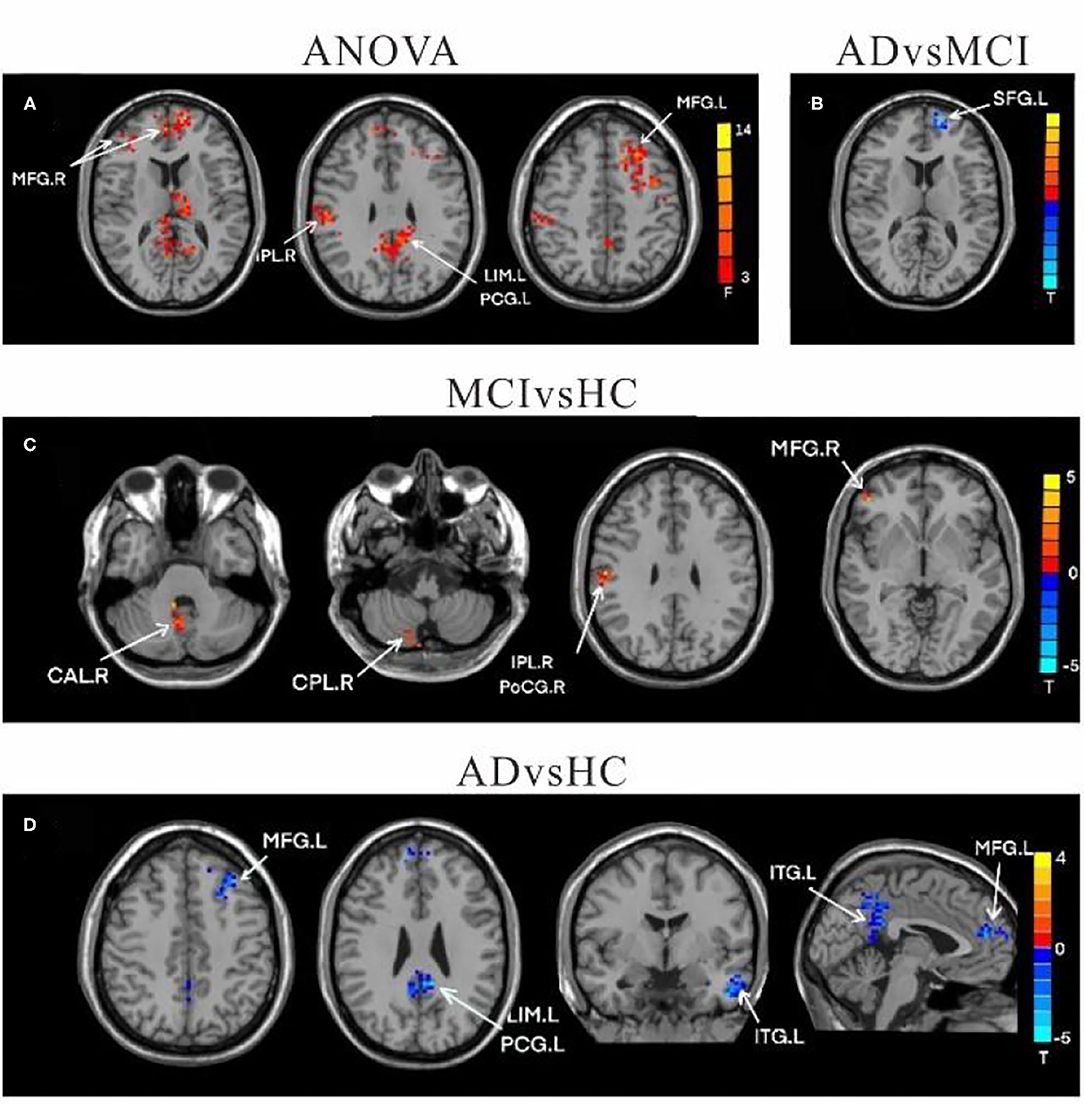
Figure 5. Differences of FC values in the cerebellum right lobule IX. (A) Brain regions showing significant differences in FC of the right lobule IX based on analysis of variance (ANOVA) analysis between HC, AD, and aMCI; p < 0.05, the cluster size > 100 voxels. (B–D) The results of post-hoc two-sample T-tests in voxel-wise analysis (Bonferroni corrected, cluster size ≥ 30 voxels, p < 0.05). AD, Alzheimer's disease; aMCI, amnestic mild cognitive impairment; HC, healthy controls; MFG, Middle Frontal Gyrus; IPL, inferior parietal lobe; LIM, limbic lobe; PCG, posterior cingulate gyrus; SFG, superior frontal gyrus; CAL, cerebellum Anterior lobe; CPL, cerebellum posterior lobe; THA, Thalamus; ITG, inferior temporal gyrus; CAU, Caudate; L, left; R, right.
Correlation Analysis With Clinical Behavior Scores and CSF Biomarkers
As shown in Figure 6, MOCA scores negatively correlate with FC values between right crus II and right THAL values (r = −0.2285, p = 0.005), as well as between left lobule IX and right parietal lobe FC values in AD patients (r = −0.3517, p = 0.043). In contrast, TMT-A scores positively correlate with FC values between left lobule IX and right parietal lobe/post-central gyrus FC values in AD patients (r = 0.3981, p = 0.026). In the MCI group, DST scores were positively correlated with FC values between left crus II and right THAL values (r = 0.3961, p = 0.017), as well as between right crus II and right frontal lobe (r = 0.3961, p = 0.048). Moreover, VFT scores were also positively correlated with FC values between right lobule IX and left frontal lobe in MCI patients (r = 0.4431, p = 0.014). There was no significant correlation between CSF biomarkers and functional connectivity (P > 0.05).
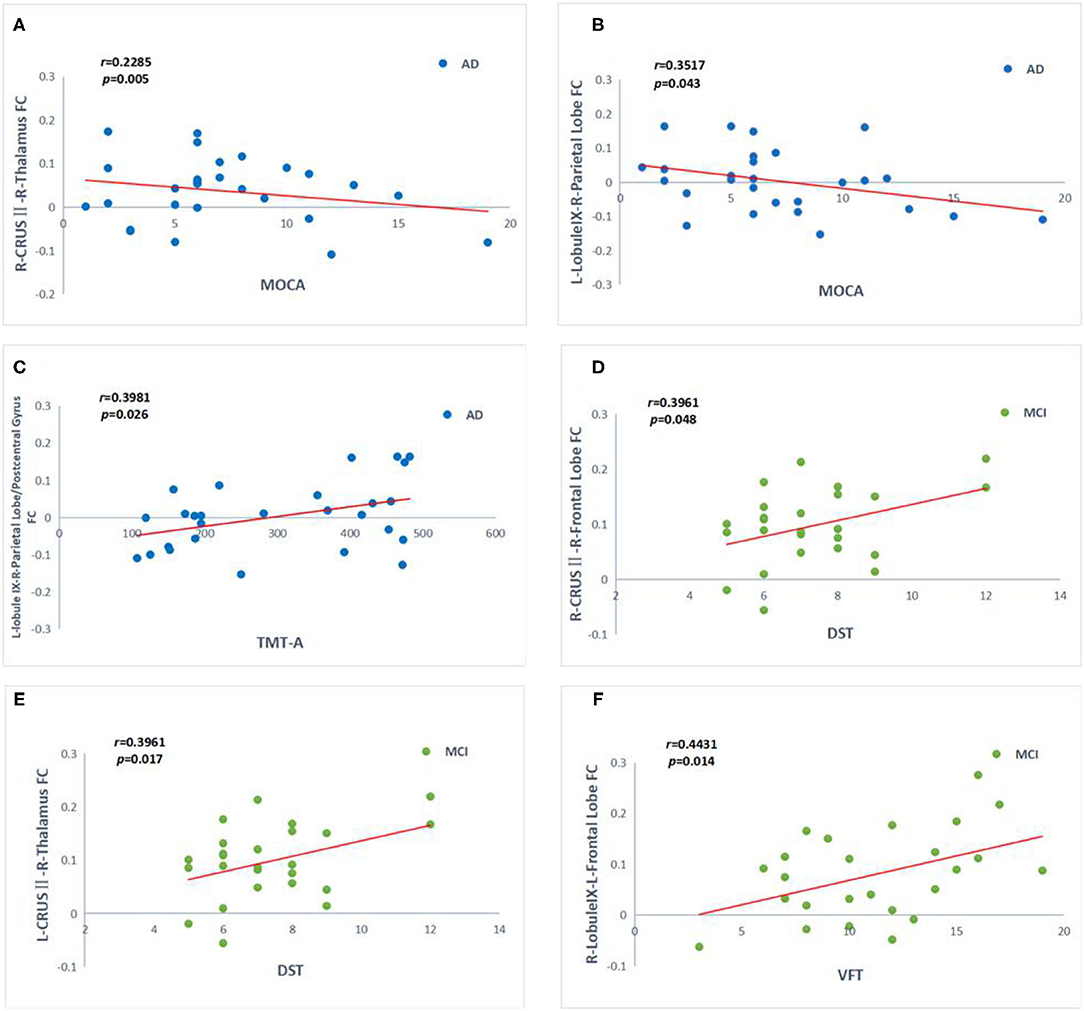
Figure 6. Correlation between the functional connectivity (FC) and cognitive function scores in the AD (blue point) and aMCI (green point) patients. (A–F) Significant correlation between FC changes and cognitive function scales include MOCA, STT-A, DST, VFT in cerebellum crus II and lobule IX (Bonferroni corrected, p < 0.05). Age, gender, and years of education were used to control variables of the results. AD, Alzheimer's disease; aMCI, amnesic mild cognitive impairment; MOCA, Montreal Cognitive Assessment; DST, Digit Span Test; VTF, Verbal Fluency Test; TMT, Trail Making Test; R, right; L, left.
Discussion
In the present study, alterations in FC between the cerebellum cognitive sub-region and whole brain were investigated among patients with AD and MCI. We also explored the relevance of this change to cognitive function. Firstly, we found that after adjusting for age, gender, education level and gray matter volume, FC changed brain regions were distributed in temporal region and front-oparietal lobe region, which were important brain regions of DMN and FPN, respectively. Secondly, we identified that FC changes was associated with impaired cognitive function, especially in attention, executive, and memory tasks.
The current research indicates that there are significant changes with regards to the FC of cerebellar cognitive subregions within the AD and MCI groups. Compared to the HC group, FC in frontal lobe, temporal lobe, and parietal lobe were decreased in AD group. Interestingly, the FC between cerebellum and superior frontal gyrus and inferior frontal gyrus in the MCI group was partially weakened, while some connections in the middle frontal gyrus and parietal lobe were strengthened. The research on brain functional connections of AD is now a hot topic, but there are a few studies that have evaluated the effect of the cerebellum on cognitive function connections.
Functional Connectivity Changes in the AD Patients
In the present study, decreased FC in the AD group were mainly in frontal, temporal, parietal and precuneus cortex, which were closely related to DMN and FPN (38).
It was found in other longitudinal studies that the evolution of AD spectrum is characterized by progressive loss of functional connectivity in the neocortical association area (58, 59). Compared with HC subjects, the hyper connection found in MCI subjects may be a compensation mechanism for the low efficiency of memory network, especially in the temporoparietal region (60, 61). Previous studies have found that the prefrontal and temporoparietal connectivity of MCI patients is stronger than that of normal people (13, 62), With the progression of the disease, the connectivity is weakened in AD (58, 63, 64).
Furthermore, a strong relationship between DMN, lobule IX, and crus II have been reported previously (7, 32, 47, 65). DMN is a brain resting network that is activated when individuals are not engaged in attending to or responding to external stimuli, and is involved in regulating self-reflection and memory processes (66–68). The DMN network, which consists of the PCUN/PCC, medial prefrontal cortex, lateral temporal and parietal cortices and hippocampus, has been proposed to be the most vulnerable brain network in patients with AD (8, 69, 70). Thus, it can be inferred that diminished cerebellar connectivity to the DMN network in AD patients may be one of the causes involved in cognitive impairment. FPN is a network that is involved in attention and working memory function and consists of lateral prefrontal and parietal cortex. It has also been reported that the FPN is remarkably impaired in AD patients, as reported herein (38, 71).
It is important to note that compared to HC, the connections between cognitive subregions of the cerebellum and the limbic lobe were significantly reduced in AD patients. This result is consistent with a recent study that reported that FC in the cerebellar-limbic network was significantly more vulnerable in AD patients compared to aMCI patients (72).
Functional Connectivity Changes in the MCI Patients
In comparison to HC, patients with MCI show right cerebellar crus II hyper-connectivity with bilateral IPL and MFG, which is functionally associated with FPN. Moreover, the FC between the cerebellum and thalamus, superior frontal gyrus, and inferior frontal gyrus were decreased in MCI patients.
Previous studies have shown that there is less structural and pathological damage at the aMCI stage (5). It has also been reported that increased cerebellar activity was positively correlated with memory enhancement, and served as a compensatory process (58). Thus, we can speculate that an increase in connectivity here may underline some of the compensatory mechanism of the cerebellum for sites of weakened connectivity.
While the FC in IPL sites were impaired in AD patients, they were actually enhanced in aMCI patients, suggesting that enhanced connectivity between the cerebellum and IPL in preclinical AD may have functional compensatory mechanisms (5). The IPL is an important node in DMN and FPN, and is considered a heterogeneous brain area with functions in episodic memory, semantic processing, and spatial cognition (73, 74). Consistent with our results, hyperactivity in the inferior and superior parietal lobes in MCI patients has been reported within the present study (14). Therefore, these findings indicate that IPL may be one of the brain areas responsible for episodic memory. It is worth noting that the aMCI group showed increased FC between the cerebellum and IPL in the DMN, while it was decreased in the AD group, compared to the HC group. This proves that changes in FC may serve as markers for identifying patients with AD and aMCI.
In the present study, decreased FC between the cerebellum and MFG is indicated in AD patients. However, the FC between the right-sided lobule IX and MFG was significantly increased in MCI patients, which may compensate for the impaired memory that is often seen in MCI patients. Previous studies have indicated that decreased DMN connectivity is associated with increased prefrontal connectivity and that this increased connectivity may be a compensatory effect on cognition of the prefrontal lobes (5, 75). In addition, some studies found that FC increased in anterior DMN and FC decreased in posterior DMN; The increase of FC in anterior DMN is considered to be a compensatory increase of cognitive function to maintain task performance (76, 77).
Hypo-connectivity between the cerebellum crus II, lobule IX, and THAL was also investigated in the present study. The cerebellum is involved in cognitive processes through cerebellum-thalamo-cortical pathways to cognitive functions regions, including the prefrontal and parietal cortices, and the cingulate and para-hippocampal gyri (14, 21). The thalamic nuclei are important intermediate stations in the cerebrocerebellar feedback limb of the cerebrocerebellar circuit (33). It is significant for the cerebellum-thalamo-cortical, and cortical-ponto-cerebellar pathways to modulate cognitively-relevant prefrontal and parietal activities (46). Therefore, reduced connectivity between cerebellum and thalamus in AD patients may directly interrupt the connectivity of the cerebellum to cognitive networks, including the DMN and the limbic lobe network, which leads to cognitive impairment.
Further correlation analysis with clinical score revealed that compensation and decompensation in AD and MCI patients were clinically distinguishable. The FC values between the right crus II and the left SFG were positively correlated with DST scores in MCI patients, while the resting-state FC values between the right crus II and the right THAL were negatively correlated with MOCA scores in AD patients. These results indicate that remarkable changes occur in the attentive networks involving lobule VI, crus I, and crus II, implying a fundamental role for these cerebellar areas in attention (78). FC between the cerebellum and fronto-parietal cortex was proposed to be strongly associated with magnitude of cerebellar activation through working memory and attention (79). No correlation with HDAS was found in this study, which may be due to the limited range of the dependent variable in our cohort, which has been added to the revised comments.
We also correlated signals that were extracted from areas of significant FC with cerebrospinal fluid biomarkers, including Aβ1-40, Aβ1-42, Aβ1-40/Aβ1-42, t-tau, and p-tau, which be used as one of the differentiations and diagnostic criteria for Alzheimer's disease (80). However, no statistically significant difference was identified.
Di Lorenzo et al. used the neurophysiological method of short latency afferent inhibition (SAI) and found that stimulating the cerebellum θ Wave group (TBS) can activate cerebello thalamic cortical pathway and regulate central cholinergic function (81). In subsequent study, they used repetitive and paired pulse transcranial magnetic stimulation in patients with different degrees of AD and were followed up for 3 years. The results showed that the loss of LTP like cortical plasticity in AD patients was more severe than that in MCI patients (82). After the study of cerebellum iTBS, they found that the plasticity mechanism of cerebellum cortex was damaged in Alzheimer disease (83). A previous study that applied continuous theta burst stimulation (cTBS) (a non-invasive stimulus in which cTBS can inhibits brain excitability) to the lateral part of the cerebellum found that FC between the frontal and parietal cognitive areas was significantly attenuated, while FC between cTBS and motor areas remained unchanged (84). This also proves that cerebellar TBS can promote the reorganization of cerebellar cortex and has a potential role in improving motor and learning (85). The results of this study demonstrate the role of the cerebellum in AD progression and pathogenesis, and provide a novel target for non-pharmacological interventions. Our findings are able to help explain the mechanism by which non-invasive stimulation improves cognitive impairment in AD, and provides new targets and ideas for non-pharmacological interventions.
Limitations
There were three major limitations of this study that need to be addressed in the future. Firstly, some patients with ad also have emotional symptoms. The relationship between cerebellum and emotion has been confirmed. Therefore, in the next study, we will add CCAS scale to evaluate more comprehensively. Secondly, in this study, the result of cluster sizes was relatively small, it may be related to the small sample size. We will continue to increase the sample size in the follow-up study. Thirdly, the study was a cross-sectional study, longitudinal studies are needed in the future to study the dynamic changes of cerebellum in the development of AD.
Conclusion
In conclusion, FC between the cerebellar and other cognitively relevant sub-regions was found to be significantly reduced in AD patients. In MCI patients, the FC between the cerebellum and cortex, including the superior and inferior frontal gyri, was also disrupted. On the other hand, the FC between the cerebellum and the middle frontal gyrus, as well as the parietal regions, was enhanced. These results suggest that MCI may be in the preclinical stage of structural damage and functional compensation of AD, and is associated with cognitive function. And the FC changes in specific cerebello-cortical functional modules may play an important role in the pathogenesis of AD.
Data Availability Statement
The raw data supporting the conclusions of this article will be made available by the authors, without undue reservation.
Ethics Statement
The studies involving human participants were reviewed and approved by Medical Research Ethical Committee of Nanjing Brain Hospital in Nanjing, China. The patients/participants provided their written informed consent to participate in this study.
Author Contributions
JS, FT, and QY designed this study. FT, WM, and QL analyzed the data. FT and DZ wrote the manuscript. All authors contributed to the article and approved the submitted version.
Funding
This work was supported by Nanjing Medical Science and Technology Development key project (No. ZKX19029).
Conflict of Interest
The authors declare that the research was conducted in the absence of any commercial or financial relationships that could be construed as a potential conflict of interest.
References
1. Jack CR Jr, Knopman DS, Jagust WJ, Petersen RC, Weiner MW, et al. Tracking pathophysiological processes in Alzheimer's disease: an updated hypothetical model of dynamic biomarkers. Lancet Neurol. (2013) 12:207–16. doi: 10.1016/S1474-4422(12)70291-0
2. Alzheimer's A. 2016 Alzheimer's disease facts and figures. Alzheimers Dement. (2016) 12:459–509. doi: 10.1016/j.jalz.2016.03.001
3. Knopman DS, Petersen RC. Mild cognitive impairment and mild dementia: a clinical perspective. Mayo Clin Proc. (2014) 89:1452–9. doi: 10.1016/j.mayocp.2014.06.019
4. Sheline YI, Raichle ME, Snyder AZ, Morris JC, Head D, Wang S, et al. Amyloid plaques disrupt resting state default mode network connectivity in cognitively normal elderly. Biol Psychiatry. (2010) 67:584–7. doi: 10.1016/j.biopsych.2009.08.024
5. Cai S, Chong T, Peng Y, Shen W, Li J, von Deneen KM, et al. Altered functional brain networks in amnestic mild cognitive impairment: a resting-state fMRI study. Brain Imaging Behav. (2017) 11:619–31. doi: 10.1007/s11682-016-9539-0
6. Li H, Jia X, Qi Z, Fan X, Ma T, Ni H, et al. Altered functional connectivity of the basal nucleus of meynert in mild cognitive impairment: a resting-state fMRI study. Front Aging Neurosci. (2017) 9:127. doi: 10.3389/fnagi.2017.00127
7. Guo CC, Tan R, Hodges JR, Hu X, Sami S, Hornberger M. Network-selective vulnerability of the human cerebellum to Alzheimer's disease and frontotemporal dementia. Brain. (2016) 139(Pt 5):1527–38. doi: 10.1093/brain/aww003
8. Zhu H, Zhou P, Alcauter S, Chen Y, Cao H, Tian M, et al. Changes of intranetwork and internetwork functional connectivity in Alzheimer's disease and mild cognitive impairment. J Neural Eng. (2016) 13:046008. doi: 10.1088/1741-2560/13/4/046008
9. Wang L, Zang Y, He Y, Liang M, Zhang X, Tian L, et al. Changes in hippocampal connectivity in the early stages of Alzheimer's disease: evidence from resting state fMRI. Neuroimage. (2006) 31:496–504. doi: 10.1016/j.neuroimage.2005.12.033
10. Skouras S, Falcon C, Tucholka A, Rami L, Sanchez-Valle R, Llado A, et al. Mechanisms of functional compensation, delineated by eigenvector centrality mapping, across the pathophysiological continuum of Alzheimer's disease. Neuroimage Clin. (2019) 22:101777. doi: 10.1016/j.nicl.2019.101777
11. Fox MD, Greicius M. Clinical applications of resting state functional connectivity. Front Syst Neurosci. (2010) 4:19. doi: 10.3389/fnsys.2010.00019
12. Lombardi G, Crescioli G, Cavedo E, Lucenteforte E, Casazza G, Bellatorre AG, et al. Structural magnetic resonance imaging for the early diagnosis of dementia due to Alzheimer's disease in people with mild cognitive impairment. Cochrane Database Syst Rev. (2020) 3:CD009628. doi: 10.1002/14651858.CD009628.pub2
13. Delli Pizzi S, Punzi M, Sensi SL. Alzheimer's disease neuroimaging I. Functional signature of conversion of patients with mild cognitive impairment. Neurobiol Aging. (2019) 74:21–37. doi: 10.1016/j.neurobiolaging.2018.10.004
14. Gu L, Zhang Z. Exploring structural and functional brain changes in mild cognitive impairment: a whole brain ALE meta-analysis for multimodal MRI. ACS Chem Neurosci. (2019) 10:2823–9. doi: 10.1021/acschemneuro.9b00045
15. Rapoport M, van Reekum R, Mayberg H. The role of the cerebellum in cognition and behavior: a selective review. J Neuropsychiatr Clin Neurosci. (2000) 12:193–8. doi: 10.1176/jnp.12.2.193
16. Schmahmann JD, Caplan D. Cognition, emotion and the cerebellum. Brain. (2006) 129(Pt 2):290–2. doi: 10.1093/brain/awh729
17. Balsters JH, Whelan CD, Robertson IH, Ramnani N. Cerebellum and cognition: evidence for the encoding of higher order rules. Cereb Cortex. (2013) 23:1433–43. doi: 10.1093/cercor/bhs127
18. Fan L, Hu J, Ma W, Wang D, Yao Q, Shi JJN, et al. Altered baseline activity and connectivity associated with cognitive impairment following acute cerebellar infarction: a resting-state fMRI study. Neurosci Lett. (2019) 692:199–203. doi: 10.1016/j.neulet.2018.11.007
19. Wang D, Yao Q, Yu M, Xiao C, Fan L, Lin X, et al. Topological disruption of structural brain networks in patients with cognitive impairment following cerebellar infarction. Front Neurol. (2019) 10:759. doi: 10.3389/fneur.2019.00759
20. Schmahmann JD, Sherman JC. The cerebellar cognitive affective syndrome. Brain. (1998) 121 (Pt 4):561–79. doi: 10.1093/brain/121.4.561
21. Hoche F, Guell X, Vangel MG, Sherman JC, Schmahmann JD. The cerebellar cognitive affective/Schmahmann syndrome scale. Brain. (2018) 141:248–70. doi: 10.1093/brain/awx317
22. Ahmadian N, van Baarsen K, van Zandvoort M, Robe PA. The cerebellar cognitive affective syndrome-a meta-analysis. Cerebellum. (2019) 18:941–50. doi: 10.1007/s12311-019-01060-2
23. Sereno MI, Diedrichsen J, Tachrount M, Testa-Silva G, d'Arceuil H, De Zeeuw. C. The human cerebellum has almost 80% of the surface area of the neocortex. Proc Natl Acad Sci USA. (2020) 117:19538–43. doi: 10.1073/pnas.2002896117
24. Uwisengeyimana JD, Nguchu BA, Wang Y, Zhang D, Liu Y, Qiu B, et al. Cognitive function and cerebellar morphometric changes relate to abnormal intra-cerebellar and cerebro-cerebellum functional connectivity in old adults. Exp Gerontol. (2020) 140:111060. doi: 10.1016/j.exger.2020.111060
25. Gellersen HM, Guell X, Sami S. Differential vulnerability of the cerebellum in healthy ageing and Alzheimer's disease. Neuroimage Clin. (2021) 30:102605. doi: 10.1016/j.nicl.2021.102605
26. Harper L, Bouwman F, Burton EJ, Barkhof F, Scheltens P, O'Brien JT, et al. Patterns of atrophy in pathologically confirmed dementias: a voxelwise analysis. J Neurol Neurosurg Psychiatry. (2017) 88:908–16. doi: 10.1136/jnnp-2016-314978
27. Tabatabaei-Jafari H, Walsh E, Shaw ME, Cherbuin N, Alzheimer's Disease Neuroimaging I the cerebellum shrinks faster than normal ageing in Alzheimer's disease but not in mild cognitive impairment. Hum Brain Mapp. (2017) 38:31413150. doi: 10.1002/hbm.23580
28. Bruchhage MMK, Correia S, Malloy P, Salloway S, Deoni S. Machine learning classification identifies cerebellar contributions to early and moderate cognitive decline in Alzheimer's disease. Front Aging Neurosci. (2020) 12:524024. doi: 10.3389/fnagi.2020.524024
29. Toniolo S, Serra L, Olivito G, Marra C, Bozzali M, Cercignani M. Patterns of cerebellar gray matter atrophy across Alzheimer's disease progression. Front Cell Neurosci. (2018) 12:430. doi: 10.3389/fncel.2018.00430
30. Olivito G, Serra L, Marra C, Di Domenico C, Caltagirone C, Toniolo S, et al. Cerebellar dentate nucleus functional connectivity with cerebral cortex in Alzheimer's disease and memory: a seed-based approach. Neurobiol Aging. (2020) 89:32–40. doi: 10.1016/j.neurobiolaging.2019.10.026
31. Barbey AK, Koenigs M, Grafman J. Dorsolateral prefrontal contributions to human working memory. Cortex. (2013) 49:1195–205. doi: 10.1016/j.cortex.2012.05.022
32. Buckner RL, Krienen FM, Castellanos A, Diaz JC, Yeo BT. The organization of the human cerebellum estimated by intrinsic functional connectivity. J Neurophysiol. (2011) 106:2322–45. doi: 10.1152/jn.00339.2011
33. Kelly RM, Strick PL. Cerebellar loops with motor cortex and prefrontal cortex of a nonhuman primate. J Neurosci. (2003) 23:8432–44. doi: 10.1523/JNEUROSCI.23-23-08432.2003
34. Toniolo S, Serra L, Olivito G, Caltagirone C, Mercuri N, Marra C, et al. Cerebellar white matter disruption in Alzheimer's disease patients: a diffusion tensor imaging study. (2020) 74:615–24. doi: 10.3233/JAD-191125
35. Zhang L, Ni H, Yu Z, Wang J, Qin J, Hou F, et al. Investigation on the alteration of brain functional network and its role in the identification of mild cognitive impairment. Front Neurosci. (2020) 14:558434. doi: 10.3389/fnins.2020.558434
36. Bai F, Liao W, Watson DR, Shi Y, Yuan Y, Cohen AD, et al. Mapping the altered patterns of cerebellar resting-state function in longitudinal amnestic mild cognitive impairment patients. J Alzheimers Dis. (2011) 23:87–99. doi: 10.3233/JAD-2010-101533
37. Li W, Han T, Qin W, Zhang J, Liu H, Li Y, et al. Altered functional connectivity of cognitive-related cerebellar subregions in well-recovered stroke patients. Neural Plast. (2013) 2013:452439. doi: 10.1155/2013/452439
38. Zheng W, Liu X, Song H, Li K, Wang Z. Altered functional connectivity of cognitive-related cerebellar subregions in Alzheimer's disease. Front Aging Neurosci. (2017) 9:143. doi: 10.3389/fnagi.2017.00143
39. Sveljo O, Culic M, Koprivsek K, Lucic M. The functional neuroimaging evidence of cerebellar involvement in the simple cognitive task. Brain Imaging Behav. (2014) 8:480–6. doi: 10.1007/s11682-014-9290-3
40. Kuper M, Kaschani P, Thurling M, Stefanescu MR, Burciu RG, Goricke S, et al. Cerebellar fMRI activation increases with increasing working memory demands. Cerebellum. (2016) 15:322–35. doi: 10.1007/s12311-015-0703-7
41. Schmahmann JD. Cerebellum in Alzheimer's disease and frontotemporal dementia: not a silent bystander. Brain. (2016) 139(Pt 5):1314–8. doi: 10.1093/brain/aww064
42. Stoodley CJ, Schmahmann JD. Functional topography in the human cerebellum: a meta-analysis of neuroimaging studies. Neuroimage. (2009) 44:489–501. doi: 10.1016/j.neuroimage.2008.08.039
43. Sokolov AA, Miall RC, Ivry RB. The cerebellum: adaptive prediction for movement and cognition. Trends Cogn Sci. (2017) 21:313–32. doi: 10.1016/j.tics.2017.02.005
44. Guell X, Gabrieli JDE, Schmahmann JD. Triple representation of language, working memory, social and emotion processing in the cerebellum: convergent evidence from task and seed-based resting-state fMRI analyses in a single large cohort. Neuroimage. (2018) 172:437–49. doi: 10.1016/j.neuroimage.2018.01.082
45. Shipman ML, Green JT. Cerebellum and cognition: does the rodent cerebellum participate in cognitive functions? Neurobiol Learn Mem. (2019) 106996. doi: 10.1016/j.nlm.2019.02.006
46. Gao Z, Liu X, Zhang D, Liu M, Hao N. The indispensable role of the cerebellum in visual divergent thinking. Sci Rep. (2020) 10:16552. doi: 10.1038/s41598-020-73679-9
47. Habas C, Kamdar N, Nguyen D, Prater K, Beckmann CF, Menon V, et al. Distinct cerebellar contributions to intrinsic connectivity networks. J Neurosci. (2009) 29:8586–94. doi: 10.1523/JNEUROSCI.1868-09.2009
48. McKhann GM, Knopman DS, Chertkow H, Hyman BT, Jack CR Jr, et al. The diagnosis of dementia due to Alzheimer's disease: recommendations from the National Institute on Aging-Alzheimer's Association workgroups on diagnostic guidelines for Alzheimer's disease. Alzheimers Dement. (2011) 7:263–9. doi: 10.1016/j.jalz.2011.03.005
49. Chen J, Shu H, Wang Z, Zhan Y, Liu D, Liao W, et al. Convergent and divergent intranetwork and internetwork connectivity patterns in patients with remitted late-life depression and amnestic mild cognitive impairment. Cortex. (2016) 83:194–211. doi: 10.1016/j.cortex.2016.08.001
50. Tombaugh TN, McIntyre NJ. The mini-mental state examination: a comprehensive review. J Am Geriatr Soc. (1992) 40:922–35. doi: 10.1111/j.1532-5415.1992.tb01992.x
51. Horton DK, Hynan LS, Lacritz LH, Rossetti HC, Weiner MF, Cullum CM. An Abbreviated montreal cognitive assessment (MoCA) for dementia screening. Clin Neuropsychol. (2015) 29:413–25. doi: 10.1080/13854046.2015.1043349
52. Morris JC. The Clinical Dementia Rating (CDR): current version and scoring rules. Neurology. (1993) 43:2412–4. doi: 10.1212/WNL.43.11.2412-a
53. Vakil E, Blachstein H. Rey auditory-verbal learning test: structure analysis. J Clin Psychol. (1993) 49:883–90. doi: 10.1002/1097-4679(199311)49:6<883::AID-JCLP2270490616>3.0.CO;2-6
54. Mainland BJ, Amodeo S, Shulman KI. Multiple clock drawing scoring systems: simpler is better. Int J Geriatr Psychiatr. (2014) 29:127–36. doi: 10.1002/gps.3992
55. Bowden SC, Petrauskas VM, Bardenhagen FJ, Meade CE, Simpson LC. Exploring the dimensionality of digit span. Assessment. (2013) 20:188–98. doi: 10.1177/1073191112457016
56. Bagby RM, Ryder AG, Schuller DR, Marshall MB. The hamilton depression rating scale: has the gold standard become a lead weight? Am J Psychiatr. (2004) 161:2163–77. doi: 10.1176/appi.ajp.161.12.2163
57. Yan CG, Wang XD, Zuo XN, Zang YF. DPABI: data processing and analysis for (Resting-State) brain imaging. Neuroinformatics. (2016) 14:339–51. doi: 10.1007/s12021-016-9299-4
58. McLaren DG, Sreenivasan A, Diamond EL, Mitchell MB, Van Dijk KR, Deluca AN, et al. Tracking cognitive change over 24 weeks with longitudinal functional magnetic resonance imaging in Alzheimer's disease. Neurodegener Dis. (2012) 9:176–86. doi: 10.1159/000335876
59. Zhan Y, Ma J, Alexander-Bloch AF, Xu K, Cui Y, Feng Q, et al. Longitudinal study of impaired intra- and inter-network brain connectivity in subjects at high risk for Alzheimer's disease. J Alzheimers Dis. (2016) 52:913–27. doi: 10.3233/JAD-160008
60. Bajo R, Castellanos NP, Cuesta P, Aurtenetxe S, Garcia-Prieto J, Gil-Gregorio P, et al. Differential patterns of connectivity in progressive mild cognitive impairment. Brain Connect. (2012) 2:21–4. doi: 10.1089/brain.2011.0069
61. Jacobs HI, Van Boxtel MP, Heinecke A, Gronenschild EH, Backes WH, Ramakers IH, et al. Functional integration of parietal lobe activity in early Alzheimer disease. Neurology. (2012) 78:352–60. doi: 10.1212/WNL.0b013e318245287d
62. Sheline YI, Raichle ME. Resting state functional connectivity in preclinical Alzheimer's disease. Biol Psychiatr. (2013) 74:340–7. doi: 10.1016/j.biopsych.2012.11.028
63. Grady CL, McIntosh AR, Beig S, Keightley ML, Burian H, Black SE. Evidence from functional neuroimaging of a compensatory prefrontal network in Alzheimer's disease. J Neurosci. (2003) 23:986–93. doi: 10.1523/JNEUROSCI.23-03-00986.2003
64. Zhou B, Yao H, Wang P, Zhang Z, Zhan Y, Ma J, et al. Aberrant functional connectivity architecture in Alzheimer's disease and mild cognitive impairment: a whole-brain, data-driven analysis. Biomed Res Int. (2015) 2015:495375. doi: 10.1155/2015/495375
65. Krienen FM, Buckner RL. Segregated fronto-cerebellar circuits revealed by intrinsic functional connectivity. Cereb Cortex. (2009) 19:2485–97. doi: 10.1093/cercor/bhp135
66. Raichle ME. The brain's default mode network. Annu Rev Neurosci. (2015) 38:433–47. doi: 10.1146/annurev-neuro-071013-014030
67. Davey CG, Pujol J, Harrison BJ. Mapping the self in the brain's default mode network. Neuroimage. (2016) 132:390–7. doi: 10.1016/j.neuroimage.2016.02.022
68. Davey CG, Harrison BJ. The brain's center of gravity: how the default mode network helps us to understand the self. World Psychiatr. (2018) 17:278–9. doi: 10.1002/wps.20553
69. Greicius MD, Srivastava G, Reiss AL, Menon V. Default-mode network activity distinguishes Alzheimer's disease from healthy aging: evidence from functional MRI. Proc Natl Acad Sci USA. (2004) 101:4637–42. doi: 10.1073/pnas.0308627101
70. Dillen KNH, Jacobs HIL, Kukolja J, Richter N, von Reutern B, Onur OA, et al. Functional disintegration of the default mode network in prodromal Alzheimer's disease. J Alzheimers Dis. (2017) 59:169–87. doi: 10.3233/JAD-161120
71. Zhao Q, Sang X, Metmer H, Swati Z, Lu J, Alzheimer's disease neuroimaging I. Functional segregation of executive control network and frontoparietal network in Alzheimer's disease. Cortex. (2019) 120:36–48. doi: 10.1016/j.cortex.2019.04.026
72. Qi Z, An Y, Zhang M, Li HJ, Lu J. Altered cerebro-cerebellar limbic network in ad spectrum: a resting-state fMRI study. Front Neural Circuits. (2019) 13:72. doi: 10.3389/fncir.2019.00072
73. Bzdok D, Hartwigsen G, Reid A, Laird AR, Fox PT, Eickhoff SB. Left inferior parietal lobe engagement in social cognition and language. Neurosci Biobehav Rev. (2016) 68:319–34. doi: 10.1016/j.neubiorev.2016.02.024
74. Wang J, Xie S, Guo X, Becker B, Fox PT, Eickhoff SB, et al. Correspondent functional topography of the human left inferior parietal lobule at rest and under task revealed using resting-state fMRI and coactivation based parcellation. Hum Brain Mapp. (2017) 38:1659–75. doi: 10.1002/hbm.23488
75. Wang Y, Risacher SL, West JD, McDonald BC, Magee TR, Farlow MR, et al. Altered default mode network connectivity in older adults with cognitive complaints and amnestic mild cognitive impairment. J Alzheimers Dis. (2013) 35:751–60. doi: 10.3233/JAD-130080
76. Dunn CJ, Duffy SL, Hickie IB, Lagopoulos J, Lewis SJ, Naismith SL, et al. Deficits in episodic memory retrieval reveal impaired default mode network connectivity in amnestic mild cognitive impairment. Neuroimage Clin. (2014) 4:473–80. doi: 10.1016/j.nicl.2014.02.010
77. Xue C, Yuan B, Yue Y, Xu J, Wang S, Wu M, et al. Distinct disruptive patterns of default mode subnetwork connectivity across the spectrum of preclinical Alzheimer's disease. Front Aging Neurosci. (2019) 11:307. doi: 10.3389/fnagi.2019.00307
78. Castellazzi G, Bruno SD, Toosy AT, Casiraghi L, Palesi F, Savini G, et al. Prominent changes in cerebro-cerebellar functional connectivity during continuous cognitive processing. Front Cell Neurosci. (2018) 12:331. doi: 10.3389/fncel.2018.00331
79. Brissenden JA, Levin EJ, Osher DE, Halko MA, Somers DC. Functional evidence for a cerebellar node of the dorsal attention network. J Neurosci. (2016) 36:6083–96. doi: 10.1523/JNEUROSCI.0344-16.2016
80. Molinuevo JL, Gispert JD, Dubois B, Heneka MT, Lleo A, Engelborghs S, et al. The AD-CSF-index discriminates Alzheimer's disease patients from healthy controls: a validation study. J Alzheimers Dis. (2013) 36:67–77. doi: 10.3233/JAD-130203
81. Di Lorenzo F, Martorana A, Ponzo V, Bonni S, D'Angelo E, Caltagirone C, et al. Cerebellar theta burst stimulation modulates short latency afferent inhibition in Alzheimer's disease patients. Front Aging Neurosci. (2013) 5:2. doi: 10.3389/fnagi.2013.00002
82. Di Lorenzo F, Motta C, Casula EP, Bonni S, Assogna M, Caltagirone C, et al. LTP-like cortical plasticity predicts conversion to dementia in patients with memory impairment. Brain Stimul. (2020) 13:1175–82. doi: 10.1016/j.brs.2020.05.013
83. Di Lorenzo F, Bonni S, Picazio S, Motta C, Caltagirone C, Martorana A, et al. Effects of cerebellar theta burst stimulation on contralateral motor cortex excitability in patients with Alzheimer's disease. Brain Topogr. (2020) 33:613–7. doi: 10.1007/s10548-020-00781-6
84. Rastogi A, Cash R, Dunlop K, Vesia M, Kucyi A, Ghahremani A, et al. Modulation of cognitive cerebello-cerebral functional connectivity by lateral cerebellar continuous theta burst stimulation. Neuroimage. (2017) 158:48–57. doi: 10.1016/j.neuroimage.2017.06.048
Keywords: cerebellum, Azheimer's disease, mild cognitive impairment, functional connectivity, resting-state functional MRI
Citation: Tang F, Zhu D, Ma W, Yao Q, Li Q and Shi J (2021) Differences Changes in Cerebellar Functional Connectivity Between Mild Cognitive Impairment and Alzheimer's Disease: A Seed-Based Approach. Front. Neurol. 12:645171. doi: 10.3389/fneur.2021.645171
Received: 05 January 2021; Accepted: 24 May 2021;
Published: 17 June 2021.
Edited by:
Wen Qin, Tianjin Medical University General Hospital, ChinaReviewed by:
Sofia Toniolo, University of Oxford, United KingdomHossein Tabatabaei Jafari, Australian National University, Australia
Copyright © 2021 Tang, Zhu, Ma, Yao, Li and Shi. This is an open-access article distributed under the terms of the Creative Commons Attribution License (CC BY). The use, distribution or reproduction in other forums is permitted, provided the original author(s) and the copyright owner(s) are credited and that the original publication in this journal is cited, in accordance with accepted academic practice. No use, distribution or reproduction is permitted which does not comply with these terms.
*Correspondence: Jingping Shi, cHJvZnNoaWpwQDE2My5jb20=