- 1Department of General and Interventional Radiology and Neuroradiology, Wroclaw Medical University, Wrocław, Poland
- 2Department of Psychiatry, Wroclaw Medical University, Wrocław, Poland
- 3Department of Social Medicine, Wroclaw Medical University, Wrocław, Poland
- 4Department of Angiology, Hypertension and Diabetology, Wroclaw Medical University, Wrocław, Poland
Introduction: The aim of the study was to evaluate the impact of multiple risk factors (age, diabetes, hypertension, hyperlipidemia, BMI, smoking, alcohol) on the gray and white matter volumes as well as on the burden of white matter hyperintensities (WMH).
Material and Methods: The study group consisted of 554 subjects (age range: 50–69 yrs, F/M: 367/187) recruited from the larger cohort of the Polish fraction of the Prospective Urban Rural Epidemiological (PURE) study. The participants answered questionnaires about their lifestyle, underwent physical and psychological examination (MoCA test), laboratory blood tests followed by brain MRI. Volumetric measurements of the total gray matter (GMvol), total white matter (WMvol) and WHM (WMHvol) normalized to the total intracranial volume were performed using the Computational Anatomy Toolbox 12 (CAT12) and Statistical Parametric Maps 12 (SPM12) based on 3D T1-weighted sequence. The influence of risk factors was assessed using multiple regression analysis before and after correction for multiple comparisons.
Results: Older age was associated with lower GMvol and WMvol, and higher WMHvol (p < 0.001). Smaller GMvol volume was associated with higher WMHvol (p < 0.001). Higher WMHvol was associated with hypertension (p = 0.01) and less significantly with hyperlipidemia (only before correction p = 0.03). Diabetes, abnormal BMI, smoking and alcohol intake did not have any significant impact on GMvol, WMvol or WMHvol (p > 0.05). MoCA score was not influenced by any of the factors.
Conclusions: Gray matter loss is strongly associated with the accumulation of WMH which seems to be potentially preventable by maintaining normal blood pressure and cholesterol levels.
Introduction
Brain aging is a complex process affecting both gray and white matter. It is a physiological process that begins in the fourth and peaks in the seventh decade of life (1, 2). Physiological degeneration of the gray and white matters is the result of alterations in their microstructure and biochemistry leading not only to the loss of brain cells but also to the accumulation of iron, amyloid plaques, neurofibrils, lipofuscin and neuromelanin, as well as a decrease in the number of neurotransmitters such as dopamine and serotonin (3, 4). At a molecular level there are also some other factors that can affect the process of brain aging such as dysregulation of calcium metabolism, abnormalities in glucose metabolism or mitochondrial dysfunction (5, 6).
Brain aging is influenced by many factors including genetic, environmental, or coexisting diseases. Cerebral vascular pathology strongly contributes to acceleration of brain degeneration, especially cerebral small vessel disease (CSVD) causing lacunar infarcts, microbleeds and white matter hyperintensities (WMH) leading to white matter damage (7). Recently, WMH have been of a great interest since they are associated with the increased risk of cognitive impairment and vascular dementia (8). Their etiology and pathophysiology are not yet fully understood, however hypoxic-ischemic axonal loss and demyelination, hypoperfusion due to altered cerebrovascular autoregulation as well as blood-brain barrier dysfunction have been established as leading pathophysiological causes (9). Histopathological findings in regions of WMH are myelin paleness, tissue rarefaction with loss of myelin and axons, as well as mild gliosis (10).
It has been shown that several hormones, other comorbidities as well as environmental and genetic factors may also have an impact on gray and white matter degeneration (11–13). Atrial fibrillation, genetic variants in the APOEe4gene, low mental activity and passive lifestyle may accelerate brain atrophy (9). Other studies revealed that hypertension, diabetes, hypercholesterolemia may also have a significant impact on this process while healthy lifestyle, balanced diet, regular physical activity, low or no alcohol consumption and prevention of cardiovascular diseases directly reduce the occurrence of WMH and the risk of white matter atrophy (4, 9, 14, 15).
Pathological acceleration of gray and white matter degeneration is closely linked to cognitive impairment and dementia. In the past decades dementia has become a huge clinical, social and economic problem reaching a status of pandemic affecting 50 million people worldwide with nearly 10 million new cases every year according to the WHO report from 2020. It has been reported that markers of CSVD are not only common in individuals diagnosed with vascular dementia but also in patients with Alzheimer's disease (AD) and, after accounting for non-independence between risk factors, about one-third of AD cases worldwide might be attributed to potentially modifiable factors (16, 17). It is also well-known that brain degeneration in AD and other dementias accumulates sub-clinically over years leading finally to irreversible severe brain damage.
The association of different risk factors with brain atrophy and WMH has already been reported in the literature however the results are often contradictory. Majority of the articles focus on evaluation of the influence of single factors while they usually do not occur isolated but as a combination of multiple risk factors (15, 18, 19).
The aim of this cross-sectional study was to evaluate the impact of multiple common demographic and epidemiological factors such as age, sex, diabetes, hypertension, hyperlipidemia, body weight, smoking and alcohol consumption on the volumes of the gray and white matter and on the presence of WMH in a large cohort of 554 community-based subjects recruited from the Polish sub-study of the large multinational Prospective Urban Rural Epidemiological (PURE) study. To our knowledge this is the first report showing the results of associations between multiple factors and brain volumetry as well as the presence of WMH from a large cohort of subjects representing Central Europe Caucasian population. Since there is no effective treatment available, knowledge of risk factors of lower brain volumes and white matter damage, which may be specific for population and geographic regions, seems to be of great importance in the successful prevention of cognitive impairment and dementia.
Materials and Methods
The study group consisted of 554 subjects (367 women and 187 men) recruited from a larger cohort of 1,269 participants of the Polish sub-study of the multinational Prospective Urban Rural Epidemiological (PURE) study. All participants underwent demographic and risk factor assessment followed by psychological evaluation and magnetic resonance imaging (MRI) of the brain. The study exclusion criteria were: age below 50 and above 69 years, contraindications to MRI (including mainly a pacemaker and other contraindicated body implants, as well as severe claustrophobia), history of stroke or dementia as well as other significant neurological or psychiatric diseases.
An informed consent was obtained from all individual participants involved in the study. The study protocol was approved by the local Bioethical Committee (permission No. KB-32/2016).
Demographic and Risk Factor Assessment
Demographic and medical information including: age, sex, diabetes, hypertension, hyperlipidemia, body max index (BMI), smoking status, alcohol consumption were collected using standardized PURE questionnaires followed by laboratory blood assessment and physical examination. Diabetes was defined as fasting blood glucose ≥126 mg/dl (7.0 mmol/l) or currently treated diabetes while hyperlipidemia as total cholesterol ≥200 mg/dl. Hypertension was defined as a systolic blood pressure (SBP) ≥135 mm Hg or diastolic blood pressure (DBP) ≥85 mmHg or currently treated hypertension. Body mass index was calculated as weight (kg) divided by height (m) squared. Abnormal weight was defined as BMI ≥25 and normal <25. Information on smoking status and alcohol consumption was collected as self-reported answers “yes” or “no” in the questionnaires. The smoking group included current and former smokers. Alcohol intake was negative when no alcohol consumption was reported.
Cognitive Assessment
Cognitive assessment was performed using the Montreal Cognitive Assessment (MoCA) in the Polish version which is tailored to evaluate various cognitive domains such as attention and concentration, executive functions, memory, language, visuo-constructional skills, conceptual thinking, calculations, and orientation. The total possible score was 30 points with 26 points or above considered as cognitive health and scores below 26 points as potential mild cognitive impairment (MCI). The duration of the test was about 10 min. All cognitive tests were conducted by experienced psychologists.
Brain MRI Acquisition and Analysis
Brain MRI was performed using the same 1.5 Tesla MR scanner (GE, Hdx). The scanning protocol included: 3D T1-weighted images [repetition time (TR) = 8.3 ms, echo time (TE) = 3.2 ms, inversion time (TI) = 650 ms, field of view (FOV) = 240 × 240 mm2, slice thickness =1 mm, flip angle = 12°, matrix = 256 x 256, NEX = 1), T2-weighted images (TR = 2720 ms, TE = 88/8.8ms, FOV = 240 × 240 mm2, slice thickness = 3.5 mm, matrix = 256 x 256, NEX = 1), Fluid-attenuated inversion recovery sequences (FLAIR) (TR = 8.800 ms, TE = 145 ms, TI = 2.2 ms, FOV = 240 × 240 mm2, slice thickness = 3.5 mm, matrix = 256 x 256, NEX = 1), diffusion weighted images (DWI) (TR = 1000 ms, echo TE = 107 ms, FOV = 240 x 168 mm2, slice thickness = 0.7 mm, flip angle = 20°, matrix = 256 x 256, NEX = 1), susceptibility-weighted images (SWI) (TR = 73.9 ms, TE = 47.4 ms, FOV= 240 x 168 mm2, slice thickness = 3.5 mm, flip angle = 12°, matrix = 256 × 256, NEX = 1).
Visual Assessment of MR Images
Vascular lesions related to CSVD were classified according to the standard published criteria as lacunar infarcts, microbleeds and WMH (20). Lacunar infarcts were assessed on T2-weighted and FLAIR images as infarcts in basal ganglia, thalami and the white matter smaller than 1.5 cm in size. Presence of microbleeds was assessed on SWI images. White matter hyperintensities were assessed on T2-weighted and FLAIR images separately in the periventricular (periventricular WMH) and subcortical (subcortical WMH) locations and graded based on the Fazekas scale (10) with grades 0 or 1 interpreted as normal and grades 2 or 3 regarded as pathological amount of WMH.
All MR scans were rated by two independent experienced neuroradiologists trained for CSVD assessment using the same criteria. The inter-rater reliability was established with kappa coefficient that ranged between 0.71 and 0.88 depending on the category of the assessed parameters. Despite the good inter-rater reliability, all scans that showed any discrepancy between the two readers were re-evaluated and the final score was established by consensus.
Volumetric Measurements of the MRI Data
Data processing workflow consisted of a voxel-based processing using the Computational Anatomy Toolbox 12 (CAT12, Structural Brain Imaging Group, University of Jena) and the Statistical Parametric Maps 12 (SPM12) software. The initial voxel processing steps included a spatial adaptive non-local means (SANLM) denoising filter (21) then data was bias-corrected and affine-registered followed by the standard SPM “unified segmentation” (22). Further steps included skull-stripping of the brain, parcellation into the left and right hemisphere, subcortical areas, and the cerebellum. To address problems of gray matter intensities a local intensity transformation of all tissue classes was performed. Final steps included adaptive maximum a posteriori (AMAP) segmentation (23), which was then refined by applying a partial volume estimation (24). We have used a CAT12 method to detect and handle WMH based on the 3D T1-weighted sequence which is comparable to existing T2/FLAIR-based methods (25) and uses the region-growing approach to identify all WMH lesions also those in the periventricular location (26).
For quality assurance, a 2-step process was used. First, before preprocessing in CAT12, overall data quality was checked and datasets with artifacts were rejected. Secondly, the quality control measures incorporated in the CAT12 processing pipeline were used to identify the corrupted data after segmentation. Additionally, all segmentations for each subject were manually reviewed for its quality. As a results 23 subjects were rejected due to incomplete or insufficient data quality due to movement artifacts or aborted scanning. Finally 554 subjects were included in the study. Tissue class images in spatial correspondence to the original data have been obtained. This allowed us to calculate native volumes of the gray and white matters, cerebrospinal fluid and WMH for each subject which were exported from CAT12 for statistical analyses. Figure 1 shows the segmented brain tissue images generated using the CAT12 VBM methods.
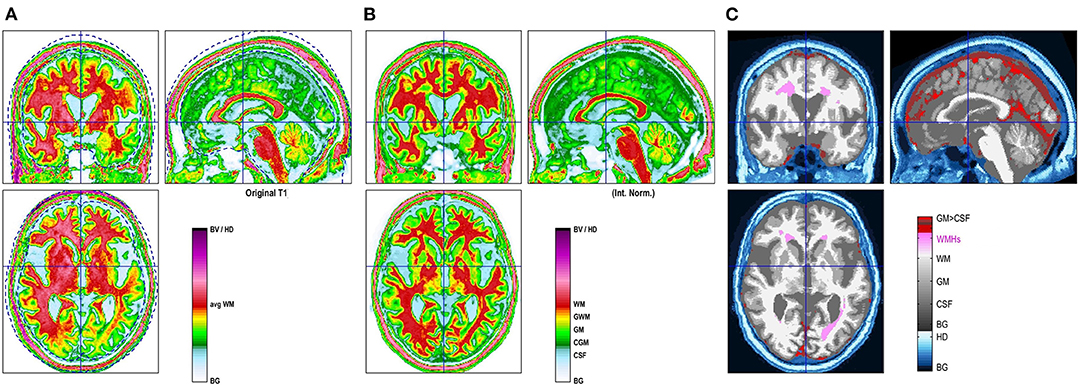
Figure 1. CAT12 VBM images showing segmented brain tissues: original image (A), normalized image with template (B) and WMH segmentation (C).
Final volumetric measurements consisted of the volumes of the total intracranial space, total gray matter (GMvol), total white matter (WMvol), total cerebrospinal fluid and WMH (WMHvol). Volumes of total gray matter, total white matter and total WMH were estimated as direct volumes in mm3 as well as the volumes normalized to the volume of the total intracranial space to reduce the influence of individual and sex-related differences in brain sizes.
Statistical Analysis
Descriptive statistics of the demographic data, risk factors and the results of brain volumetry were presented as mean values with standard deviations and range, or number of cases with percentage.
Analysis of the effect of different risk factors on GMvol, WMvol and WMHvol was performed using multiple linear regression modeling. Statistics presented for those models are regression coefficient and its 95% confidence interval.
Analysis of the influence of different risk factors on pathological MOCA scores (0–25 vs. 26–30 points) as well as pathological accumulation of periventricular and subcortical WMH (Fazekas grades 2–3 vs. Fazekas grades 0–1) was assessed using a binary logistic regression modeling. Statistics presented for those models are odds ratio and its 95% confidence interval.
In each case full models were used adjusted for age, sex and all risk factors. Models for GMvol, WMvol and MOCA score were additionally adjusted for WMHvol. P-values in multivariate regression analyses were corrected for multiple testing using Benjamini-Hochberg correction.
All calculations were made using the R package for Windows (version 4.0.4). Statistical results were considered significant when the p-value was < 0.05.
Results
The largest group of participants consisted of the older population between 60 and 69 years of age (335/554, 60.47%), followed by the group between 50 and 59 years (219/554, 39.43%) (Table 1). Women constituted the majority of the study group (367/554, 66.25%). Hyperlipidemia occurred in about 59.57% of the subjects, followed by hypertension in ~50% of the group, while diabetes was found in 18.6 % of the subjects. Majority of the subjects (70.76%) had normal body weight (BMI <25), 81.41% never smoked and around 24.19% declared no alcohol consumption. Approximately 40% of the participants scored <26 points in the MoCA test (Table 1, Figure 2).
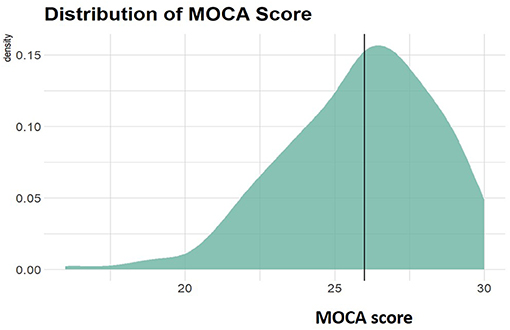
Figure 2. Distribution of MOCA scores among participants. Vertical line indicates 26 points which is a threshold between normal (26–30) and abnormal (0–25) scores.
The results of both visual inspection as well as volumetric analysis of MR images are shown in Table 2. Visual inspection of MR images revealed lacunar infarcts and microbleeds in <30 subjects each. Pathological amount of WMH (Fazekas grades two or three either in the periventricular or subcortical location) was present in 13.36% of the study group, more often in the periventricular (10.83%) than in the subcortical (8.48%) location. Distribution of WMH burden according to Fazekas scale is shown in Figure 3. Parametric results of GMvol, WMvol and WMHvol both direct in cm3 and normalized to the volume of the total intracranial space are shown in Table 2.
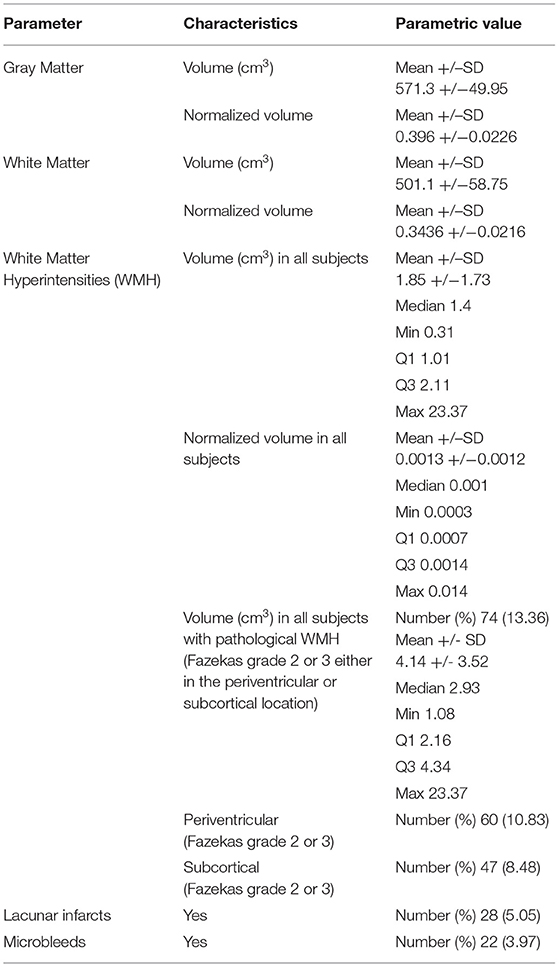
Table 2. Volumetric measurements of the gray and white matters as well as cerebral small vessel disease characteristics derived from brain MRI.
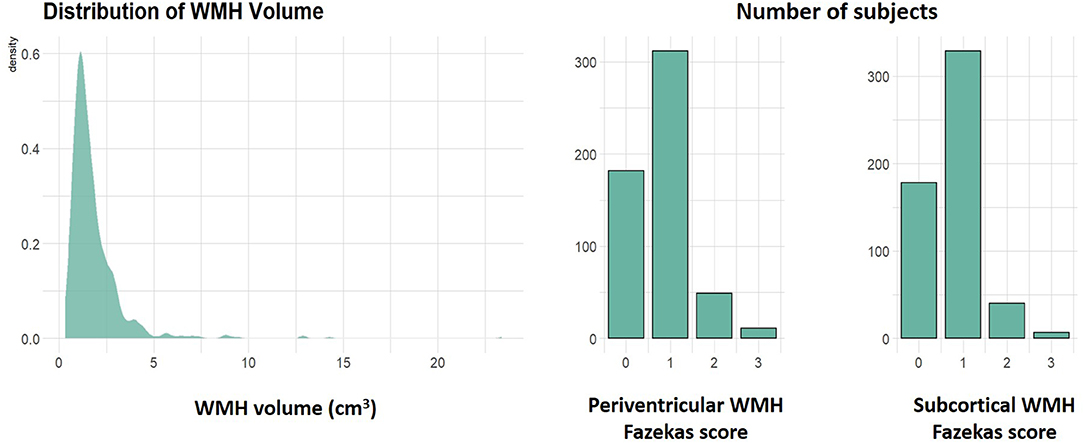
Figure 3. Distribution of white matter hyperintensities (WMH) volumes among participants as well as histograms showing numbers of subjects with normal (scores 0–1) and pathological (scores 2–3) amount of periventricular and subcortical WMH according to the Fazekas scale.
The results of the multivariate regression models used for the evaluation of associations between age, sex and multiple risk factors and brain parameters derived from MRI as well as the results of the MoCA test are shown in Tables 3, 4. Statistical analysis revealed that older age was significantly associated with lower GMvol (p < 0.001, corrected p < 0.001), lower WMvol (p < 0.001, corrected p < 0.001) and higher WMHvol (p < 0.001, corrected p = 0.0003). Age was a significant factor increasing the risk of pathological amount of periventricular (p < 0.001, corrected p = 0.0001) and subcortical (p = 0.005, corrected p = 0.01) WMH, as well as lower scores at MoCA test (p < 0.001, corrected p = 0.005). Even though all volumetric measurements were corrected for the volume of the intracranial space to avoid significant individual or sex-related differences in brain sizes, still male sex was associated with the higher GMvol compared to females (p < 0.001, corrected p < 0.001).
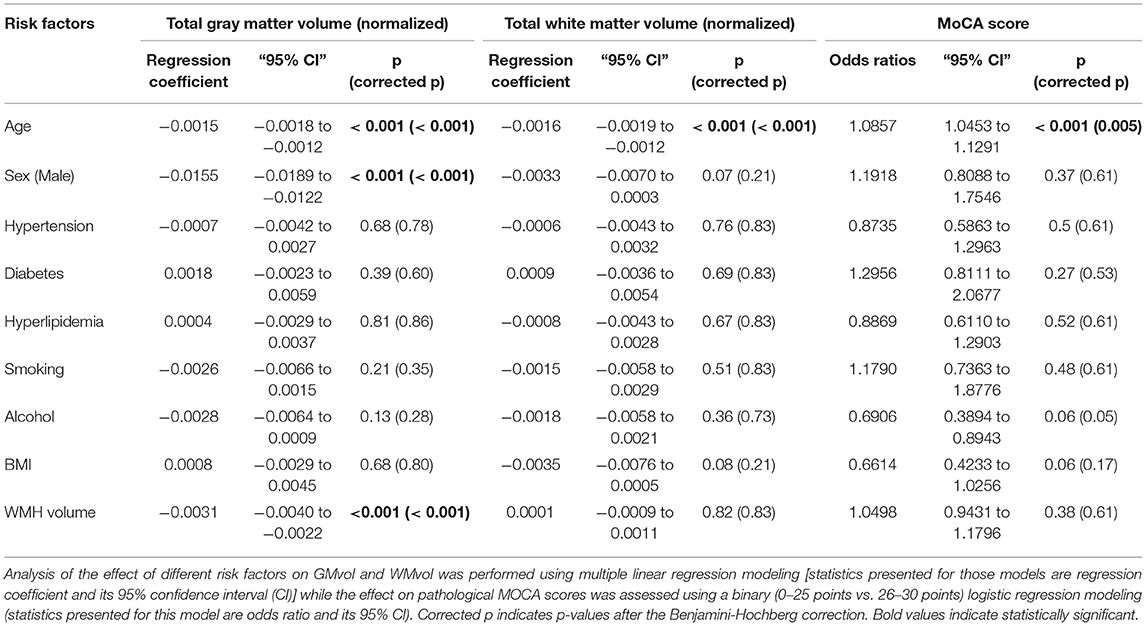
Table 3. Associations between multiple risk factors and the volumes of the gray and white matter as well as MoCA scores.
Evaluation of the impact of multiple risk factors revealed that hypertension had a significant influence on the presence of a pathological amount of WMH in both periventricular and subcortical locations before and after correction for multiple comparisons (before correction p = 0.007 and p = 0.005, respectively, and after correction p = 0.02 and p = 0.01, respectively). Hypertension and hyperlipidemia showed significant impact on WMHvol before correction (p = 0.01 and p = 0.03, respectively), but not after correction (p = 0.06 and p = 0.08, respectively).
On the other hand, risk factors such as diabetes, smoking, alcohol consumption or abnormal BMI showed no significant influence (p > 0.05) on GMvol, WMvol or the presence of WMH before and after correction for multiple comparisons.
The presence of higher WMHvol was significantly associated with lower GMvol before and after correction (p <0.001), without any effect on WMvol or the MOCA score (p > 0.05).
The MoCA score was not associated with GMvol, WMvol and WMHvol or with any other risk factor, except for age (p > 0.05), both before and after correction for multiple comparisons.
Discussion
The main goal of this study was to assess the impact of age, sex and multiple risk factors on aging and degeneration of the gray and white matter characterized by the decrease of their volumes and the presence of a pathological burden of WMH reflecting chronic white matter damage.
Even though the age range in the studied population was only 20 years and all the subjects were between 50 and 70 years of age, still age was found to be one of the most important factors influencing the volumes of the gray and white matter and WMH as well as the presence of a pathological burden of periventricular and subcortical WMH. It is not surprising since all these features are hallmark of both physiological and pathological aging of the brain. Our results are in accordance with many previous histological and neuroimaging reports that established normal aging to be associated with a distinctive atrophy of mainly hemispheric gray and white matter with a reduction of brain volumes at a rate of 5% per decade after 40 years of age, together with the increased accumulation of WMH (2, 4, 10, 20, 27–32).
Sex-related differences in the size of male and female brains have already been reported (33, 34). Both, to account for normal variation in head size and sex-related smaller volumes of the brain in women, all volumetric measurements were normalized to the total volume of the intracranial space. We did not find any influence of male or female sexes on the volumes of the white matter and WMH, though the gray matter volume was significantly increased in men compared to women.
Analysis of the impact of multiple factors on the volume of the gray matter revealed that only higher WMH volume was associated with lower volumes of GMvol. No influence of any other of the evaluated risk factors on GMvol was demonstrated. There are numerous reports in the literature about the indisputable effect of WMH on brain atrophy and gray matter loss (12, 13, 15, 19, 35–38). Moreover, Habes et al. found that individuals with a high burden of WMH displayed significantly higher rate of the cortical atrophy pattern similar to that found in AD, compared to people with a lower burden of WMH exhibiting more age-related patterns of brain atrophy (14). Several mechanisms have been proposed to explain the association between WMH and gray matter atrophy, such as ischemic damage or Wallerian degeneration. It is believed that there are several mechanisms for the formation of WMH, and the most important is the direct injury of the myelin sheaths of axons, oligodendrocytes, glial cells as a result of small vessel damage. Ischemia of the subcortical axons leads to the disappearance of gray matter cells through damage to synapses. As a result of microangiopathy, cerebral circulation is dysregulated leading to hypoxia and secondarily to loss of neurons (35, 36). Advanced neuroimaging methods suggest that WMH particularly affect white matter networks connecting remote brain regions and thus lead to gray matter shrinkage through Wallerian degeneration (39). These results make it particularly important to assess an individual's brain health profile with both the presence of WMH and gray matter loss that is influenced by the coexistence of WMH. Thus, a well-known relation between WMH and cognitive decline or even dementia should be explained by two mechanisms such as direct damage of the white matter and acceleration of the gray matter loss.
In our study evaluation of the white matter consisted of the assessment of its volume and the presence of WMH. Age was found to be the only factor with an impact on the WMvol. On the other hand, hypertension was demonstrated to significantly increase the volume of WMH and the risk of pathological amount of WMH located both in the periventricular and subcortical brain regions. Moreover, hyperlipidemia was found to be associated with the higher volumes of WMH though this relationship was not as strong as with hypertension and only significant before correction for multiple comparisons. Nevertheless, our findings are in accordance with several previous reports from the Western European, North American and Chinese studies (15, 18). Hypertension is one of the most commonly identified factors associated with WMH (14). Several mechanisms, including neuroinflammation, oxidative stress, dendritic shrinkage, and apoptosis, are thought to be implicated in the pathophysiology linking elevated blood pressure and neurodegeneration. It has been postulated that hypertension may increase accumulation of WMH by disrupting the blood-brain barrier integrity what causes a secondary disorder of neuronal nutrition that results in their apoptosis. More often also cardio-vascular factors are thought to be very important contributors. Since high blood pressure increases the risk of arthrosclerosis by 50% or more, it is likely to lead to lower blood perfusion in capillaries, endothelia dysfunction, impaired vasoreactivity, increased pulsatility, vessel stiffening, and changes to the blood brain barrier integrity. Small vessel disease in conjunction with ischemia, inflammation, and myelin loss are then likely to contribute to the development of WMH (14, 39).
Observations of the impact of hyperlipidemia on the human body are numerous, while the literature has no clear data regarding its direct relationship with the process of brain damage. The causes may be related to the accumulation of cholesterol molecules in the inner membrane of the arteries, resulting in damage to the vascular endothelium. It might also lead to a decrease in the level of secreted prostacyclins, which prevent platelets from sticking, therefore clots and stenosis may form, and vessel walls may harden. The above pathogenesis applies to all arterial vessels, including cerebral vessels. These processes lead to secondary ischemia of the nerve cells and their atrophy (40). It has to be stressed that the etiology of WMH is of particular significance, as they impact cognitive function across all domains, probably in a greater extent than brain atrophy (39).
In our study, factors without any influence on the gray or white matter volumes or WMH were diabetes, abnormal BMI, smoking and alcohol intake. Regarding diabetes there is no clear scientific evidence for a significant effect of glycemic disorders on the process of brain atrophy and there are contradictory reports on its influence on brain structures (14, 15, 41–44). The causes of brain damage and accelerated process of volume reduction and WMH accumulation in diabetics may be explained by direct toxic effects of the elevated glucose levels on nerve cells, damage of cerebral vessels, as well as by the disturbance of biochemical processes, among others inappropriate stimulation of receptors by insulin. Increased BMI has already been reported to be associated with a decrease in the total brain volume, though it was not demonstrated in our study (15, 45). The available literature lacks detailed analysis of the impact of abnormal BMI on the atrophy of individual brain structures. The mechanism by which obesity reduces the volume of the brain is not fully understood. Possible mechanisms include the metabolic syndrome that coexists with obesity and factors such as high triglyceride levels, low HDL cholesterol, hypertension, insulin resistance, as well as thrombotic and inflammatory condition that may reduce cerebral perfusion and increase β-amyloid production leading to neuronal degeneration. Leptin, an obesity-related peptide, has also been reported to increases the deposition of β-amyloid (45).
In this study, factors such as smoking or alcohol consumption had no significant impact on the volumes of the evaluated brain regions. It may be related to a relatively small number of active smokers (18%) and only occasional alcohol drinkers in the study material. However, it has to be stressed that there are reports in the literature that showed the effect of a long-term cigarette smoking on generalized brain atrophy and WMH accumulation either through the direct cytotoxic effect of tar substances, e.g., carbon monoxide (CO) on nerve and glial cells leading to their apoptosis through damage to cell membranes or the indirect mechanism associated with lowering the concentration of natural antioxidants, including vitamins A, C, E, which makes nerve cells more prone to oxidative stress and injury (14, 15, 46). Smoking is also associated with a more intense occurrence of atherosclerosis, which leads to secondary ischemia of nerve cells and their death (46).
On the other hand, studies on alcohol consumption showed that it is another important epidemiological factor related with brain atrophy, especially cerebellum (47–49). It has been reported that there is a significant acceleration of physiological brain atrophy in the group of people who consume a large amount of alcohol, ~418.1 g per week. However, moderate or low alcohol consumption below 181.2 g per week has no significant effect on brain volume (50). What is interesting the effect of alcohol consumption on total brain atrophy is represented by the so-called J or U curve since both groups of people non-drinkers and severe abusers show a tendency to higher brain atrophy which increases the risk of cognitive impairment (MCI) and dementia, while low doses of alcohol do not have an effect on brain volume or may even be protective (47–50). In our study no significant impact of the alcohol consumption on the brain volume may be associated with a moderate alcohol intake by the participants which is believed to have the weakest influence on brain atrophy.
In our study the increased risk of cognitive deficits in the MoCA score was only associated with the increased age. The MoCA score was not influenced by any of the evaluated risk factors or the volumes of the total gray and white matter as well as WMH. The MoCA is a brief global cognitive assessment which is sensitive to vascular cognitive impairment (51). The reports on the associations of MoCA and WMH are contradictory. Similarly to us, Smith at al did not report a significant impact of total gray and white matter volumes as well as WMH volume on MoCA in the Canadian cohort concluding that this test may not be sensitive enough to the presence of covert cerebrovascular disease in a population-based setting (19). On the other hand, recent larger Canadian study by Anand et al. demonstrated such associations between MoCA and WMH. Moreover, there have been several reports in the literature revealing significant impact of obesity or hypertension on worse scoring in MoCA as well as a protecting role of not smoking (52–54). It should be stressed that subjects in our study had relatively high scores of MoCA with mean 25.77 (below 26 is regarded as cognitive impairment) and did not report any subjective complaints on cognitive dysfunction. We believe that the larger cohort of participants or an inclusion of subjects with more pronounced cognitive impairment with lower MoCA scores could influence the results of associations between MoCA and WMH.
Major strength of our study is that this is the first large scale study of this kind in Central Europe based on the big cohort of participants recruited in the Polish fraction of the multinational PURE study focused on the evaluation of the socio-demographic and health-related factors and their impact on general health, brain lesions and cognition. Another strength is the parallel assessment of the influence of multiple factors and their association with brain volumes, WMH and the results of a psychological test. All participants underwent exactly the same procedures regarding their physical and psychological health assessment and were scanned on the same MR scanner using the same protocol what provided good quality reproducible data sets.
There are several limitations of the study. First, the cross-sectional observational character of the study and assessment of the volumes of total gray and white matter without detailed evaluation of multiple separate structures of the brain such as basal ganglia or cerebellum. On the other hand what is known from the literature brainstem and basal ganglia seem to be less affected regarding volumetric loss. In those structures changes due to aging are found mostly in their microstructure and thus may be visualized using other more sensitive imaging techniques such as quantitative MRI (qMRI) or tensor-based morphometry (TBM) (55). Another limitation is the use of the 1.5 Tesla MR scanner that allows for reliable assessment of volumetric measurements of the gray and white matters as well as WMH, but does not allow for the application of more advanced techniques such as diffusion tensor imaging or rs-fMRI which may give more detailed insight into subtle microstructural and functional abnormalities due to aging. A further limitation of the study is the use of 3D T1 sequences to assess WMH volume which is not a standardized and generally accepted method, although it was already used by Dahnke et al. (25) on a small sample group and by Dadar et al. (56) who validated this method on large-scale datasets. According to Dahnke et al. this method has a risk of underestimation of WMH volume when the lesion volume is under 10 cm3. On the other hand, Dadar et al. proved that regarding the assessment of clinical outcomes, the WMH segmentation based only on T1-weighted imaging correlates with age, cognitive, and clinical measures as strongly as the WMH volumes determined using the optimal modalities of FLAIR or T2w/PD.
Conclusions
Based on the results of our study apart from age, the main risk factor of the gray matter volume reduction is the presence of WMH. On the other hand degeneration of the white matter reflected by the increased accumulation of WMH is strongly related to hypertension and also to hyperlipidemia. Thus, prevention of gray and white matter damage should be focused especially on potentially modifiable risk factors affecting WMH accumulation of which hypertension and hyperlipidemia seem to be of the greatest importance. Our results from the largest population-based cohort study from Central Europe follow the results of the analogous studies from North America, Western Europe and China. We believe that prevention of pathological accumulation of WMH and gray matter loss may be an important factor reducing signs of pathological aging of the brain which when uncontrolled may lead to cognitive impairment or even dementia.
Data Availability Statement
The original contributions presented in the study are included in the article/supplementary material, further inquiries can be directed to the corresponding authors.
Ethics Statement
The studies involving human participants were reviewed and approved by the local University Ethics Committee for conducting research involving humans. Each participant signed his/her informed consent before inclusion. The patients/participants provided their written informed consent to participate in this study.
Author Contributions
JJ-N conducted all volumetric measurements, analyzed and interpreted data, conducted literature search, and wrote the manuscript. PP contributed to the study design and supervised all volumetric measurements, and prepared all figures. JB contributed to the study design and critically reviewed the manuscript. DS performed psychological assessment of all subjects and critically reviewed the paper. JR performed psychiatric assessment of the subject and critically reviewed the paper. KZ contributed to the study design, provided all epidemiological data, and critically reviewed the paper. KP-Z contributed to the study design, provided epidemiological data, and critically reviewed the paper. MS contributed to the study design and critically reviewed the paper. AS contributed to the study design, critically reviewed the paper, and supervised the research project. AZ contributed to the study design, critically reviewed the paper, supervised the research project, the study design, analyzed and interpreted MRI data, supervised writing of the manuscript, and critically reviewed the paper. All authors contributed to the article and approved the submitted version.
Funding
This study was supported by the National Centre grant (NCN nr 2015/17/B/NZ7-02963) and partially by the Polish Ministry of Science and Higher Education grant No 290/W-PURE/2008/0.
Conflict of Interest
The authors declare that the research was conducted in the absence of any commercial or financial relationships that could be construed as a potential conflict of interest.
References
1. Svennerholm L, Bostrom K, Jungbjer B. Changes in weight and compositions of major membrane components of human brain during the span of adult human life of Swedes. Acta Neuropathol. (1997) 94:345–52. doi: 10.1007/s004010050717
2. Scahill RI, Frost C, Jenkins R, Whitwell JL, Rossor MN, Fox NC. A longitudinal study of brain volume changes in normal ageing using serial registered magnetic resonance imaging. Arch Neurol. (2003) 60:989–94. doi: 10.1001/archneur.60.7.989
3. Anderton B. Ageing of the brain. Mech Ageing Dev. (2002) 123:811–17. doi: 10.1016/S0047-6374(01)00426-2
5. Toescu E, Verkhratsky A, Landfield P. Ca2+ regulation and gene expression in normal brain ageing. Trends Neurosci. (2004) 27:614–20. doi: 10.1016/j.tins.2004.07.010
6. Melov S. Modeling mitochondrial function in ageing neurons. Trends Neurosci. (2004) 27:601–6. doi: 10.1016/j.tins.2004.08.004
7. Dobrynina LA, Gadzhieva ZS, Shamtieva KV, Kremneva EI, Akhmetzyanov BM, Kalashnikova LA, et al. Microstructural predictors of cognitive impairment in cerebral small vessel disease and the conditions of their formation. Diagnostics. (2020) 10:720. doi: 10.3390/diagnostics10090720
8. Garnier-Crussard A, Bougacha S, Wirth M, André C, Delarue M, Landeau B, et al. White matter hyperintensities across the adult lifespan: relation to age, Aβ load, and cognition. Alzheimers Res Ther. (2020) 12:127. doi: 10.1186/s13195-020-00669-4
9. Grosu S, Rospleszcz S, Hartmann F, Habes M, Bamberg F, Schlett CL, et al. Associated factors of white matter hyperintensity volume: a machine-learning approach. Sci Rep. (2021) 11:2325. doi: 10.1038/s41598-021-81883-4
10. Fazekas F, Kleinert R, Offenbacher H, Schmidt R, Kleinert G, Payer F, et al. Pathologic correlates of incidental MRI white matter signal hyperintensities. Neurology. (1993) 43:1683–89. doi: 10.1212/WNL.43.9.1683
11. Compton J, Van Amelsoort T, Murphy D. HRT and its effect on normal ageing of the brain and dementia. Br J Clin Pharmacol. (2001) 52:647–53. doi: 10.1046/j.0306-5251.2001.01492.x
12. Sytze van Dam P, Aleman A. Insulin-like growth factor-I, cognition and brain ageing. Eur J Pharmacol. (2004) 490:87–95. doi: 10.1016/j.ejphar.2004.02.047
13. Lipnicki DM, Crawford J, Kochan NA, Trollor JN, Draper B, Reppermund S, et al. Risk factors for mild cognitive impairment, dementia and mortality: the sydney memory and ageing study. Sydney memory and aging study team. J Am Med Dir Assoc. (2017) 18:388–95. doi: 10.1016/j.jamda.2016.10.014
14. Habes M, Erus G, Toledo JB, Zhang T, Bryan N, Launer LJ, et al. White matter hyperintensities and imaging patterns of brain ageing in the general population. Brain. (2016) 139 (Pt 4):1164–79. doi: 10.1093/brain/aww008
15. Cox SR, Lyall DM, Ritchie SJ, Bastin ME, Harris MA, Buchanan CR, et al. Associations between vascular risk factors and brain MRI indices in UK Biobank. Eur Heart J. (2019) 40:2290–300. doi: 10.1093/eurheartj/ehz100
16. Scott TM, Bhadelia RA, Qiao Qiu W, Folstein MF, Rosenberg IH. Small vessel cerebrovascular pathology identified by magnetic resonance imaging is prevalent in Alzheimer's disease and mild cognitive impairment: a potential target for intervention. J Alzheimers Dis. (2018) 65:293–302. doi: 10.3233/JAD-180366
17. Norton S, Matthews FE, Barnes DE, Yaffe K, Brayne C. Potential for primary prevention of Alzheimer's disease: an analysis of population-based data. Lancet Neurol. (2014) 13:788–94. doi: 10.1016/S1474-4422(14)70136-X
18. Han F, Zhai F, Wang Q, Zhou L, Ni J, Yo M, et al. Prevelence of risk factors of cerebral small vessel disease in a Chinese population-base sample. J Stroke. (2018) 20:239–46. doi: 10.5853/jos.2017.02110
19. Smith EE, O'Donnell M, Dagenais G, Lear SA, Wielgosz A, Sharma M, et al. Early cerebral small vessel disease and brain volume, cognition, and gait. Ann Neurol. (2015) 77:251–61. doi: 10.1002/ana.24320
20. Wardlaw JM, Smith EE, Biessels GJ, Cordonnier C, Fazekas F, Frayne R, et al. Neuroimaging standards for research into small vessel disease and its contribution to ageing and neurodegeneration. Lancet Neurol. (2013) 12:822–38. doi: 10.1016/S1474-4422(13)70124-8
21. Manjon J, Coupe P, Marti-Bonmati L, Collins DL, Robles M. Adaptive non-local means denoising of MR images with spatially varying noise levels. J Magn Reson Imag. (2010) 31:192–203. doi: 10.1002/jmri.22003
22. Ashburner J, Friston KJ. Unified segmentation. Neuroimage. (2005) 26:839–51. doi: 10.1016/j.neuroimage.2005.02.018
23. Rajapakse JC, Giedd JN, Rapoport JL. Statistical approach to segmentation of single-channel cerebral MR images. IEEE Trans Med Imag. (1997) 16:176–86. doi: 10.1109/42.563663
24. Tohka J, Zijdenbos A, Evans A. Fast and robust parameter estimation for statistical partial volume models in brain MRI. Neuroimage. (2004) 23:84–97. doi: 10.1016/j.neuroimage.2004.05.007
25. Dahnke R, Ziegler G, Gaser C. Detection of White Matter Hyperintensities in T1 without FLAIR. Presented at the Human Brain Mapping Conference, Rome (2019).
26. Mangin J-F. From 3D magnetic resonance images to structural representations of the cortex topography using topology preserving deformations. J Math Imag Vision. (1995) 5:318–297. doi: 10.1007/BF01250286
27. McKee AC, Cantu RC, Nowinski CJ, Hedley-Whyte ET, Gavett BE, Budson AE, et al. Chronic traumatic encephalopathy in athletes: progressive tauopathy after repetitive head injury. J Neuro Exp Neurol. (2009) 68:709–35. doi: 10.1097/NEN.0b013e3181a9d503
28. Keller S, Robert N. Measurement of brain volume using MRI: software, techniques, choices and prerequisites. J Anthropol Sci. (2009) 87:127–51.
29. Jernigan TL, Archibald SL, Fennema-Notestine C, Gamst AC, Stout JC, Bonner J, et al. Effects of age on tissues and regions of the cerebrum and cerebellum. Neurobiol Aging. (2001) 22:581–94. doi: 10.1016/S0197-4580(01)00217-2
30. Ueno M, Sakamoto H, Kanenishi K, Onodera M, Akiguchi I, Hosokawa M. Ultrastructural and permeability features of microvessels in the hippocampus, cerebellum and pons of senescence-accelerated mice (SAM). Neurobiol Aging. (2001) 22:469–78. doi: 10.1016/S0197-4580(01)00213-5
31. Ruigrok AN, Salimi-Khorshidi G, Lai MC, Baron-Cohen S, Lombardo MV, Tait RJ, et al. A meta-analysis of sex differences in human brain structure. Neurosci Biobehav Rev. (2014) 39:34–50. doi: 10.1016/j.neubiorev.2013.12.004
32. Giorgio A, Watkins KE, Douaud G, James AC, James S, De Stefano N, et al. Changes in white matter microstructure during adolescence. Neuroimage. (2008) 39:52–6. doi: 10.1016/j.neuroimage.2007.07.043
33. Raz N, Gunning-Dixon F, Head D, Rodrigue KM, Williamson A, Acker JD. Aging, sexual dimorphism, and hemispheric asymmetry of the cerebral cortex: replicability of regional differences in volume. Neurobiol Aging. (2004) 25:377–96. doi: 10.1016/S0197-4580(03)00118-0
34. Wang Y, Xu Q, Luo J, Hu M, Zuo C. Effects of age and sex on subcortical volumes. Front Aging Neurosci. (2019) 11:259–70. doi: 10.3389/fnagi.2019.00259
35. Appelman AP, Exalto LG, van der Graaf Y, Biessels GJ, Mali WP, Geerlings MI. White matter lesions and brain atrophy: more than shared risk factors? A systematic review. Cerebrovasc Dis. (2009) 28:227–42. doi: 10.1159/000226774
36. Nitkunan A, Lanfranconi S, Charlton RA, Barrick TR, Markus HS. Brain atrophy and cerebral small vessel disease a prospective follow-up study. Stroke. (2001) 42:133–38. doi: 10.1161/STROKEAHA.110.594267
37. Petkov CI, Wu CC, Eberling JL, Mungas D, Zrelak PA, Yonelinas AP, et al. Correlates of memory function in community-dwelling elderly: the importance of white matter hyperintensities. J Int Neuropsychol Soc. (2004) 10:371–81. doi: 10.1017/S1355617704103056
38. Schmidt R, Ropele S, Enzinger C, Petrovic K, Smith S, Schmidt H, et al. White matter lesion progression, brain atrophy, and cognitive decline: the Austrian stroke prevention study. Ann Neurol. (2005) 58:491–510. doi: 10.1002/ana.20630
39. Alateeq K, Walsh EI, Cherbuin N. Higher blood pressure is associated with greater white matter lesions and brain atrophy: a systematic review with meta-analysis. J Clin Med. (2021) 10:637. doi: 10.3390/jcm10040637
41. Fontbonne A, Berr C, Ducimetière P, Alpérovitch A. Changes in cognitive abilities over a 4 year period are unfavourably affected in elderly diabetic subjects: results of the epidemiology of vascular ageing study. Diab Care. (2001) 24:366–70. doi: 10.2337/diacare.24.2.366
42. Budge M, Johnston C, Hogervorst E, de Jager C, Milwain E, Iversen SD, et al. Plasma total homocysteine and cognitive performance in a volunteer elderly population. Ann New York Acad Sci. (2000) 903:407–10. doi: 10.1111/j.1749-6632.2000.tb06392.x
43. Biessels GJ, Staekenborg S, Brunner E, Brayne C, Scheltens P. Risk of dementia in diabetes mellitus: a systematic review. Lancet Neurol. (2006) 5:64–74. doi: 10.1016/S1474-4422(05)70284-2
44. Sastre A, Grimley Evan J. Effect of the treatment of type II diabetes mellitus on the development of cognitive impairment and dementia. Cochrane Database Syst Rev. (2003)6:CD003804. doi: 10.1002/14651858.CD003804
45. Fewlass DC, Noboa K, Pi-Sunyer FX, Johnston JM, Yan SD, Tezapsidis N. Obesity-related leptin regulates Alzheimer's Abeta. Faseb J. (2004) 18:1870–78. doi: 10.1096/fj.04-2572com
46. Durazzo TC, Meyerhoff DJ, Nixon SJ. Chronic cigarette smoking: implications for neurocognition and brain neurobiology. Int J Environ Res Public Health. (2010) 7:3760–91. doi: 10.3390/ijerph7103760
47. Mukamal KJ, Kuller LH, Fitzpatrick AL, Longstreth WT Jr, Mittleman MA, Siscovick DS. Prospective study of alcohol consumption and risk of dementia in older adults. JAMA. (2003) 289:1405–13. doi: 10.1001/jama.289.11.1405
48. Mattson M, Chan S, Duan W. Modification of brain ageing and neurodegenerative disorders by genes, diet and behaviour. Physiol Rev. (2002) 82:637–72. doi: 10.1152/physrev.00004.2002
49. Otsuka M, Yamaguchi K, Ueki A. Similarities and differences between Alzheimer's disease and vascular dementia from the viewpoint of nutrition. Ann New York Acad Sci. (2002) 977:155–61. doi: 10.1111/j.1749-6632.2002.tb04811.x
50. Kubota M, Nakazaki S, Hirai S, Saeki N, Yamaura A, Kusaka T. Alcohol consumption and frontal lobe shrinkage: study of 1432 non-alcoholic subjects. J Neurol Neurosurg Psychiatry. (2001) 71:104–6. doi: 10.1136/jnnp.71.1.104
51. Pendlebury ST, Cuthbertson FC, Welch SJ, Mehta Z, Rothwell PM. Underestimation of cognitive impairment by mini-mental state examination versus the montreal cognitive assessment in patients with transient ischemic attack and stroke : a population-based study. Stroke. (2010) 41:1290–93. doi: 10.1161/STROKEAHA.110.579888
52. Singh-Manoux A, Dugravot A, Shipley M, Brunner EJ, Elbaz A, Sabia S, et al. Obesity trajectories and risk of dementia: 28 years of follow-up in the Whitehall II study. Alzheimers Dement. (2018) 14:178–86. doi: 10.1016/j.jalz.2017.06.2637
53. Walker KA, Sharrett AR, Wu A, Schneider ALC, Albert M, Lutsey PL, et al. Association of midlife to late-life blood pressure patterns with incident dementia. JAMA. (2019) 322:535–45. doi: 10.1001/jama.2019.10575
54. Szcześniak D, Rymaszewska J, Zimny A, Sasiadek M, Połtyn-Zaradna K, Smith EE, et al. Cerebral small vessel disease and other influential factors of cognitive impairment in the middle-aged: a long-term observational cohort PURE-MIND study in Poland. Geroscience. (2021) 43:279–95. doi: 10.1007/s11357-020-00271-4
55. Lambert C, Chowdhury R, Fitzgerald TH, Fleming SM, Lutti A, Hutton C, et al. Characterizing aging in the human brainstem using quantitative multimodal MRI analysis. Front Human Neurosci. (2013) 7:462. doi: 10.3389/fnhum.2013.00462
Keywords: brain aging, brain volumetry, white matter hyperintensities, epidemiological factors, prevention of brain degeneration
Citation: Jacków-Nowicka J, Podgórski P, Bladowska J, Szcześniak D, Rymaszewska J, Zatońska K, Połtyn-Zaradna K, Szuba A, Sa̧siadek M and Zimny A (2021) The Impact of Common Epidemiological Factors on Gray and White Matter Volumes in Magnetic Resonance Imaging–Is Prevention of Brain Degeneration Possible? Front. Neurol. 12:633619. doi: 10.3389/fneur.2021.633619
Received: 25 November 2020; Accepted: 07 June 2021;
Published: 13 July 2021.
Edited by:
Dusko Kozic, University of Novi Sad, SerbiaReviewed by:
Daniela Pinter, Medical University of Graz, AustriaRoberto Gasparotti, University of Brescia, Italy
Copyright © 2021 Jacków-Nowicka, Podgórski, Bladowska, Szcześniak, Rymaszewska, Zatońska, Połtyn-Zaradna, Szuba, Sa̧siadek and Zimny. This is an open-access article distributed under the terms of the Creative Commons Attribution License (CC BY). The use, distribution or reproduction in other forums is permitted, provided the original author(s) and the copyright owner(s) are credited and that the original publication in this journal is cited, in accordance with accepted academic practice. No use, distribution or reproduction is permitted which does not comply with these terms.
*Correspondence: Joanna Bladowska, YXNpYS5ibGFkb3dza2EmI3gwMDA0MDtnbWFpbC5jb20=; am9hbm5hLmJsYWRvd3NrYSYjeDAwMDQwO3VtZWQud3JvYy5wbA==