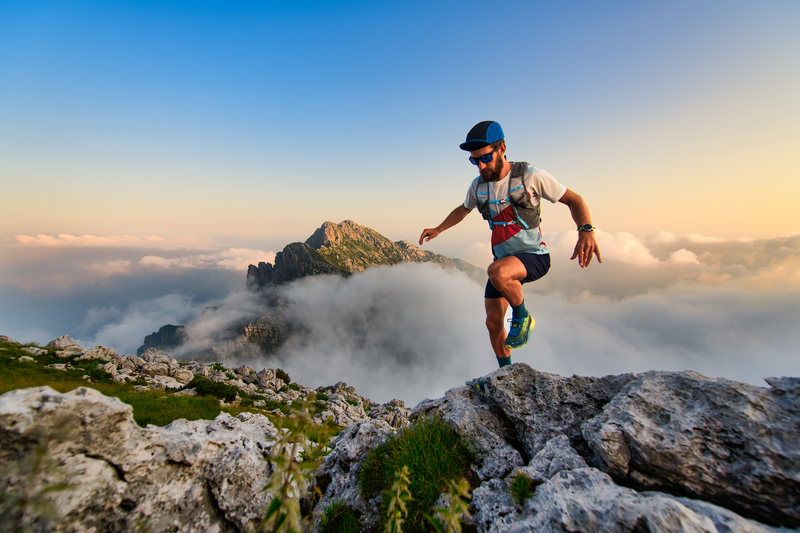
94% of researchers rate our articles as excellent or good
Learn more about the work of our research integrity team to safeguard the quality of each article we publish.
Find out more
MINI REVIEW article
Front. Neurol. , 07 January 2021
Sec. Applied Neuroimaging
Volume 11 - 2020 | https://doi.org/10.3389/fneur.2020.629463
This article is part of the Research Topic Multi-Modal Imaging in Neurological Conditions: Translational Applications View all 9 articles
In recent decades, the field of neuroimaging has experienced a surge of popularity and innovation which has led to significant advancements in the understanding of neurological disease, if not immediate clinical translation. In the case of Down's syndrome, a complex interplay of neurodevelopmental and neurodegenerative processes occur as a result of the trisomy of chromosome 21. The substantial potential impact of improved clinical intervention and the limited research under-taken to date make it a prime candidate for longitudinal neuroimaging-based study. However, as with a multitude of other multifaceted brain-based disorders, singular utilization of lone modality imaging has limited interpretability and applicability. Indeed, a present challenge facing the neuroimaging community as a whole is the methodological integration of multi-modal imaging to enhance clinical understanding. This review therefore aims to assess the current literature in Down's syndrome utilizing a multi-modal approach with regards to improvement upon consideration of a single modality. Additionally, we discuss potential avenues of future research that may effectively combine structural, functional and molecular-based imaging techniques for the significant benefit of the understanding of Down's syndrome pathology.
Down syndrome (DS) is the leading genetic cause of intellectual disability, with an approximate incidence of 1 in 750 live births (1). The extra copy of chromosome 21 is associated with a 4–5-fold overexpression of the amyloid precursor protein (APP) gene and increased accumulation of cerebral beta-amyloid (Aβ) deposition in the brain and subsequent neurofibrillary tau formation, metabolic changes and neurodegeneration (2). The processing of APP generates Aβ, the abnormal accumulation of which leads to the formation of amyloid plaques and the clinical manifestation of early onset (in the forties) Alzheimer's disease (AD) and progressive cognitive decline among individuals with DS. As our understanding of DS has increased and clinical care has improved, the lifespan of individuals with DS in developed countries has improved dramatically (3). However, there are limited and only symptomatic treatments available for AD dementia. Nevertheless, the near ubiquity of AD progression in DS means that multi-modal neuroimaging studies in DS may help delineate the natural history of biomarker changes, and in the process identify early neural substrates of AD pathogenesis. In addition, a multi-modal neuroimaging approach would be especially beneficial due to the genetics of DS and an expected predictable trajectory of development of disease and disorder.
Advances in our understanding in both the some aspects of the molecular basis and the pathogenesis of AD have resulted in novel opportunities to study potential therapeutic targets (4). Neuroimaging contributes to these rising developments by providing biomarkers that could improve comorbid AD diagnosis, inform prognosis, aid in deep phenotyping, allow for risk stratification, and track therapeutic efficacy in future clinical trials for people with DS. Models of AD pathophysiology propose a sequential progression of brain changes that are reflected by neuroimaging abnormalities, beginning with an early increase in Aβ PET tracer binding, followed by a gradual progression of neurofibrillary tau tangles, deficits in cerebral glucose metabolism (i.e., [18F]-fluorodeoxyglucose-PET (FDG-PET) and gray matter atrophy and white matter dysfunction as seen with structural T1-weighted MRI, resting-state connectivity differences (5) and diffusion imaging (6). Indeed, as we will summarize in the next section, these stereotypical AD biomarker changes have often been recapitulated in the small but growing number of neuroimaging studies in DS.
However, there is still a considerable gap between research findings and translatability into clinical practice for DS. Much progress is still needed to understand the optimal use of these imaging markers and how they could be jointly evaluated during the different stages of the disease. The majority of the literature has hitherto relied on single-modality designs to study pathological processes in isolation, despite the growing appreciation that dementia and DS is a multifactorial disease that likely emerges as a phenotypic spectrum from the dynamics between multiple pathological processes. Further studies that are able to integrate other biomarkers known to play a role in the physiopathology of AD (tau, inflammation, etc.) within a longitudinal design would be useful to unravel their relative roles, sequence, and causal relationships in the context of DS. To this end, large-scale initiatives such has ABC-DS have begun and the research community would be to synthesize the vast amounts of data meaningfully to help us understand better the neuropathological processes and clinical manifestations.
To continue advancement of understanding in the field of Down's syndrome neurodegeneration, it is key that recent innovations in neuroimaging technology are harnessed. As integration of more than one imaging modality leverages significantly more pathology-relevant information that standard methodologies, it is of importance that approaches for doing so are put forward and discussed. In this brief review, we highlight prominent existing research that utilizes such multimodal neuroimaging methods and consider future directions in a methodological context.
Post-mortem studies have indicated that virtually all persons with DS over age 40 harbor abnormal degrees of Aβ accumulation (7). This post-mortem evidence has since been borne out by in vivo evidence using [11C]-PiB PET imaging (8, 9). In addition, several reports have highlighted the striatum as a possible nidus of amyloid accumulation, as it is commonly associated with the earliest and most prominent signal retention (8, 10), similar to that observed in a [11C]-PiB PET study of presenilin-1 (PS1) mutation carriers (11). The clinical relevance of Aβ in DS has been highlighted through its associations with brain atrophy (12) and mild cognitive impairment in DS (13). While longitudinal data is scarce, Aβ accumulation in DS rates are similar to that observed in late-onset AD (14), despite an earlier onset by ~15–20 years (8, 10). More recently, tau accumulation in adults with DS has been studied using the PET tracer [18F]-AV-1451. The well-documented coupling between tau and amyloid has also led to proposals that elevated tau, particularly its propagation beyond the medial temporal cortices, is predicated upon Aβ positivity (15), consistent with that noted in late onset AD (16). Further studies to investigate the extent to which tau is associated with downstream brain atrophy (17) and cognitive decline is a subject of future research.
Glucose metabolism is one of the most robust biomarkers of AD, and [18F]-FDG PET is widely recognized as a sensitive tool to measure neuronal activity on glucose metabolism. In DS, the spatial distribution of hypometabolism appears to resemble that of sporadic AD, encompassing key regions such as the posterior cingulate and other regions that are key nodes of the default mode network (18, 19). Decreased metabolic activity has also been related to elevated Aβ burden and cognitive function (9, 20–23). Recently, the relative tracer delivery (R1) from [11C]-PiB PET imaging has been validated by Mak et al. (24) as a surrogate index of cerebral perfusion, showing marked deficits that were associated with amyloid deposition and longitudinal cognitive decline.
The cortical signature of DS has been a subject of extensive investigations using T1-weighted MRI (9, 12, 19, 25). A common finding in the literature points is that of Aβ-associated atrophy in temporo-parietal cortices and subcortical atrophy, hallmarks of atrophy patterns in sporadic AD (26). Interestingly, there are also several reports of increased cortical thickness in DS individuals without amyloid burden (25). Taken together, atrophy appears to be predicated on the presence of amyloid, a notion that is supported by a previous study demonstrating a correlation between Aβ and atrophy in DS (15).
Diffusion tensor imaging (DTI) is a non-invasive in vivo technique for characterizing the microstructural properties of white matter tracts by quantifying changes in both the rate and directionality of water molecules (27). However, this topic has received relatively little attention compared to gray matter analyses. One study showed that persons with DS and dementia have decreased white matter integrity compared to non-demented DS subjects in a similar topography seen in AD (28, 29). Given that the role of amyloid and tau accumulation underpinning white matter dysfunction is still unclear, our group is pursuing this topic via a joint-analysis of [11C]-PiB, [18F]-AV1451 and DTI datasets.
The intersection of the clinical understanding of brain pathology and technological innovation in neuroimaging has yielded significant progress in the fields of psychiatry and neurology. However, single modality imaging is inherently limited in the information it can reveal, restricting the understanding of complex and multifaceted disease processes. The complexity problem of neuroimaging datasets in neurological study and the dynamic nature of pathology is difficult to address. The successful and meaningful integration, therefore, of multiple brain imaging modalities is key to clinical progress, whether that be rooted in biomarker identification or translation to therapeutics.
Machine learning is emerging as a significantly useful technique which can both reduce and utilize the high dimensionality of neuroimaging data (30). Thus, supervised machine learning approaches are excellent candidates for the integration of multiple imaging modalities and data types. The basis of machine learning is that a data-driven methodology is applied to predictively model either a discrete or continuous outcome variable. For instance, a well-performing model based on medically non-invasive data, such as structural or functional MRI, which effectively predicts a variable only identifiable to date in an invasive or costly manner, such as amyloid burden in Alzheimer's disease, is of considerable use in clinical practice. In Down's syndrome, such advances are particularly warranted given the need for careful ethical considerations of patient vulnerability and burden. It should be noted however, that unbiased and large datasets are required for such machine learning approaches, as smaller data with sample bias may risk models that are ungeneralizable to the wider population.
Supervised machine learning algorithms are commonly applied using either a classification or regression approach, where the outcome variable of interest is either binary or continuous, respectively (31, 32). These methodologies allow for a tailored hypothesis-driven approach to the data. In Down's syndrome, an important example of the utility of a classifier would be using brain MRI data to define a patient as positive or negative for amyloid burden, thus removing the need for expensive and potentially distressing PET scanning. Given that amyloid positive status is an early indicator of the definitive development of Alzheimer's pathology, this methodology may be utilized as a biomarker for the indication of need for therapeutic intervention. Similarly, the training of regression machine learning algorithms on multimodal brain imaging data may infer accurate predictions of CSF-based biomarkers without the need for invasive procedures and laboratory cost. Importantly, machine learning represents a significantly useful technique for the integration of different types of brain imaging modalities, which among other benefits, such as predictive power, provides a wider and more accurate insight into brain pathology, leveraging multiple data types meaningful to underlying biology (33). Additionally, such implementation of machine learning for multimodal neuroimaging datasets would allow for the assessment of models for predicting pertinent clinical states and, where longitudinal training data is available, prognostic value. The limitations of machine learning techniques however should also be acknowledged, such as to-date minimal uptake of published algorithmic diagnostic approaches into clinical communities and the necessity of large and generalizable datasets for training. DS-AD is a promising candidate for such methodology as on the whole, very high disease penetrance means that the clinical population is relatively homogenous compared to other psychiatric disorders and dementias. To address the issue of translation into the clinic, neuroimaging research employing machine learning tools should carefully consider both the representative nature of the dataset and test hypotheses that address significant clinical need.
Additionally, when considering brain structure and connectivity, statistically integrating multiple MRI techniques can also be advantageous in understanding fundamental biological mechanisms. Two of the most commonly acquired types of structural data are T1-weighted and diffusion-weighted images, which rely on the time taken for the proton spin to realign with the static B0 magnetic field and the anisotropy of water diffusion along multiple magnetic gradient b-vectors, respectively (34). Therefore, the combination of T1-weighted and diffusion-weighted imaging allows for tissue macrostructure and the tissue integrity to be analyzed in tandem. One example of such an approach is the using statistical covariance of regional brain structural metrics and diffusivity metrics like fractional anisotropy to produce a morphometry similarity index, which has been previously used to demonstrate significant underlying pathology in disorders such as psychosis (35). Application of such integration between structural modalities gives enhanced understanding of alterations to brain tissue during disease states and would be particularly useful in mapping AD development in Down's syndrome.
When considering PET modalities, atlas-based parcellations of T1-weighted structural images are routinely used to label anatomical brain regions for the extraction of degree of tracer binding. However, other modalities may be leveraged in this approach to assess neuropathology in tandem with other metrics, such as functional connectivity, as demonstrated by Franzmeier et al. (36). Through the application of linear regression, with the vectorized functional connectivity matrix serving as a predictor for degree of longitudinal tau change as measured by AV1451, the results showed a functional connectivity and tau-spread coupling, which supports the hypothesis of trans-neuronal tau propagation in sporadic AD. Hence, the multimodal approach to data analysis in this case yielded important results for understanding the pathological development mechanisms of AD. In the case of DS-AD, connectomic approaches may be used in a similar way, in tandem with PET-based neuropathology data, for example to assess tau propagation with respect to white matter structural density. The outline of such a methodological approach is summarized in Figure 1.
Figure 1. Theoretic example of how connectomic approaches may be integrated with PET-based neuropathology data. Diffusion-based white matter connectivity can be statistically modeled with PET ligand binding using matrix methodology.
The increasingly common usage of ultra-high field MRI in clinical research is also a valuable asset for the advancement of methodologies that integrate multiple brain imaging modalities. The enhanced signal-to-noise ratio and spatial resolution of high field scanners, along with the application of Bayesian probabilistic approaches to structural data has allowed for the automated segmentation of small brain structures with previously unattainable accuracy (37). The hippocampal subfields (dentate gyrus, cornu ammonis 1–4 and subiculum) can now be isolated and volumetrically analyzed from T1- and T2-weighted acquisitions (38, 39), with the potential to provide valuable insight in Down's syndrome given the prominent role of the hippocampus in Alzheimer's neuropathological development. However, to gain a fuller understanding of how AD development affects the hippocampal subfields, a multimodal approach should be leveraged. The treatment of the subfields as regions of interest for tau or amyloid PET analysis would yield significant insight if utilized cross-sectionally across age groups, enabling trajectory-mapping of AD pathology within the hippocampal structure. Additionally, the integration of the hippocampal subfields with structural or functional brain connectivity data as seed regions of interests would progress past basic whole brain analyses and demonstrate hippocampal-specific changes in connectivity and its relevance to clinical phenotypic expression that may further understanding of the pathological role of these granular regions during the development of DS-AD.
The statistical joint analysis of multi-modal imaging data is also a subject of future interest (40). The recently proposed non-parametric combination (NPC) technique could be employed to investigate how multiple imaging measurements are simultaneously associated with dementia or amyloid burden in DS (i.e., “Is amyloid burden associated with both gray matter and perfusion deficits in the same regions?”). For instance, it has been shown that NPC could yield additional information about patterns of group differences not visible in each modality alone (41). To the best of our knowledge, no study has applied this technique to investigate joint changes in multiple modalities and thus should be an area of future research, particularly in the early stages such as DS persons who are cognitively normal.
In conclusion, we present in this review the trajectory of innovation regarding the integration of multimodal MRI in the clinical research of DS-AD. Whilst this review is not a comprehensive review of all neuroimaging-based methodologies, importantly, we highlight how broad approaches to neuroimaging should continue to develop how modalities can be combined to improve current knowledge of AD pathological development. A benefit of acquiring multimodal neuroimaging data is that different data types can infer different biological mechanisms—for example regional brain metabolism can be inferred from fluorodeoxyglucose (FDG)-PET, broad neurotransmitter and metabolite levels can be extracted from spectroscopy methods and brain structural analyses can examine how regional volumetrics and form associate with neurocognitive measures. The synergistic advantages of combination of these techniques mean that longitudinal imaging studies are valuable for the understanding of pathology and trajectory of disease. Building the evidence base of the development of neuropathology in DS-AD is crucial therefore, not just for more efficient diagnoses, but for identifying pertinent disease stages for effective intervention, potentially modifiable risks and mechanisms or patterns of brain alteration that can be therapeutically targeted. Gaining such knowledge as the degree of involvement of the noradrenergic and cholinergic systems in DS-AD through regional analyses of specialized brain nuclei is one example of how this may be achieved. In this review, we also put forward potential methodological approaches for future research in the field, with the aims of demonstrating that novel advancements in how data is combined can yield pertinent insights into neuropathology and begin to translate research more effectively into patient benefit.
SB and EM contributed equally to manuscript writing and conception. SZ contributed to manuscript conception and provided manuscript feedback. All authors contributed to the article and approved the submitted version.
EM was supported by the Alzheimer's Society Junior Research Fellowship (RG9611; 443 JF-18-017).
The authors declare that the research was conducted in the absence of any commercial or financial relationships that could be construed as a potential conflict of interest.
1. Antonarakis SE, Lyle R, Dermitzakis ET, Reymond A, Deutsch S. Chromosome 21 and down syndrome: from genomics to pathophysiology. Nat Rev Genet. (2004) 5:725–38. doi: 10.1038/nrg1448
2. Wiseman FK, Al-Janabi T, Hardy J, Karmiloff-Smith A, Nizetic D, Tybulewicz VL, et al. A genetic cause of Alzheimer disease: mechanistic insights from Down syndrome. Nat Rev Neurosci. (2015) 16:564–74. doi: 10.1038/nrn3983
3. Zigman WB, Lott IT. Alzheimer's disease in Down syndrome: neurobiology and risk. Ment Retard Dev Disabil Res Rev. (2007) 13:237–46. doi: 10.1002/mrdd.20163
4. Caraci F, Iulita MF, Pentz R, Flores Aguilar L, Orciani C, Barone C, et al. Searching for new pharmacological targets for the treatment of Alzheimer's disease in Down syndrome. Eur J Pharmacol. (2017) 817:7–19. doi: 10.1016/j.ejphar.2017.10.004
5. Wilson LR, Vatansever D, Annus T, Williams GB, Hong YT, Fryer TD, et al. Differential effects of Down's syndrome and Alzheimer's neuropathology on default mode connectivity. Hum Brain Mapp. (2019) 40:4551–63. doi: 10.1002/hbm.24720
6. Mak E, Su L, Williams GB, Watson R, Firbank M, Blamire A, et al. Differential atrophy of hippocampal subfields: a comparative study of dementia with lewy bodies and Alzheimer disease. Am J Geriatr Psychiatry. (2016) 24:136–43. doi: 10.1016/j.jagp.2015.06.006
7. Mann DM, Yates PO, Marcyniuk B. Alzheimer's presenile dementia, senile dementia of Alzheimer type and Down's syndrome in middle age form an age related continuum of pathological changes. Neuropathol Appl Neurobiol. (1984) 10:185–207. doi: 10.1111/j.1365-2990.1984.tb00351.x
8. Annus T, Wilson LR, Hong YT, Acosta-Cabronero J, Fryer TD, Cardenas-Blanco A, et al. The pattern of amyloid accumulation in the brains of adults with Down syndrome. Alzheimers Dement. (2016) 12:538–45. doi: 10.1016/j.jalz.2015.07.490
9. Lao PJ, Handen BL, Betthauser TJ, Mihaila I, Hartley SL, Cohen AD, et al. Longitudinal changes in amyloid positron emission tomography and volumetric magnetic resonance imaging in the nondemented Down syndrome population. Alzheimers Dement. (2017) 9:1–9. doi: 10.1016/j.dadm.2017.05.001
10. Handen BL, Cohen AD, Channamalappa U, Bulova P, Cannon SA, Cohen WI, et al. Imaging brain amyloid in nondemented young adults with Down syndrome using Pittsburgh compound B. Alzheimers Dement. (2012) 8:496–501. doi: 10.1016/j.jalz.2011.09.229
11. Klunk WE, Price JC, Mathis CA, Tsopelas ND, Lopresti BJ, Ziolko SK, et al. Amyloid deposition begins in the striatum of presenilin-1 mutation carriers from two unrelated pedigrees. J Neurosci. (2007) 27:6174–84. doi: 10.1523/JNEUROSCI.0730-07.2007
12. Mak E, Padilla C, Annus T, Wilson LR, Hong YT, Fryer TD, et al. Delineating the topography of amyloid-associated cortical atrophy in Down syndrome. Neurobiol Aging. (2019) 80:196–202. doi: 10.1016/j.neurobiolaging.2019.02.018
13. Keator DB, Phelan MJ, Taylor L, Doran E, Krinsky-McHale S, Price J, et al. Down syndrome: distribution of brain amyloid in mild cognitive impairment. Alzheimers Dement. (2020) 12:e12013. doi: 10.1002/dad2.12013
14. Zammit MD, Laymon CM, Betthauser TJ, Cody KA, Tudorascu DL, Minhas DS, et al. Amyloid accumulation in Down syndrome measured with amyloid load. Alzheimers Dement. (2020) 12:e12020. doi: 10.1002/dad2.12020
15. Mak E, Bickerton A, Padilla C, Walpert MJ, Annus T, Wilson LR, et al. Longitudinal trajectories of amyloid deposition, cortical thickness, and tau in Down syndrome: a deep-phenotyping case report. Alzheimers Dement. (2019) 11:654–8. doi: 10.1016/j.dadm.2019.04.006
16. Scholl M, Lockhart SN, Schonhaut DR, O'Neil JP, Janabi M, Ossenkoppele R, et al. PET imaging of Tau deposition in the aging human brain. Neuron. (2016) 89:971–82. doi: 10.1016/j.neuron.2016.01.028
17. Mak E, Bethlehem RAI, Romero-Garcia R, Cervenka S, Rittman T, Gabel S, et al. In vivo coupling of tau pathology and cortical thinning in Alzheimer's disease. Alzheimers Dement. (2018) 10:678–87. doi: 10.1016/j.dadm.2018.08.005
18. Minoshima S, Giordani B, Berent S, Frey KA, Foster NL, Kuhl DE. Metabolic reduction in the posterior cingulate cortex in very early Alzheimer's disease. Ann Neurol. (1997) 42:85–94. doi: 10.1002/ana.410420114
19. Matthews DC, Lukic AS, Andrews RD, Marendic B, Brewer J, Rissman RA, et al. Dissociation of Down syndrome and Alzheimer's disease effects with imaging. Alzheimers Dement. (2016) 2:69–81. doi: 10.1016/j.trci.2016.02.004
20. Haier RJ, Alkire MT, White NS, Uncapher MR, Head E, Lott IT, et al. Temporal cortex hypermetabolism in Down syndrome prior to the onset of dementia. Neurology. (2003) 61:1673–9. doi: 10.1212/01.WNL.0000098935.36984.25
21. Haier RJ, Head K, Head E, Lott IT. Neuroimaging of individuals with Down's syndrome at-risk for dementia: evidence for possible compensatory events. Neuroimage. (2008) 39:1324–32. doi: 10.1016/j.neuroimage.2007.09.064
22. Rafii MS, Wishnek H, Brewer JB, Donohue MC, Ness S, Mobley WC, et al. The down syndrome biomarker initiative (DSBI) pilot: proof of concept for deep phenotyping of Alzheimer's disease biomarkers in down syndrome. Front Behav Neurosci. (2015) 9:239. doi: 10.3389/fnbeh.2015.00239
23. Sabbagh MN, Chen K, Rogers J, Fleisher AS, Liebsack C, Bandy D, et al. Florbetapir PET, FDG PET, and MRI in Down syndrome individuals with and without Alzheimer's dementia. Alzheimers Dement. (2015) 11:994–1004. doi: 10.1016/j.jalz.2015.01.006
24. Mak E, Grigorova M, Beresford-Webb J, Malpetti M, Walpert M, Brown S, et al. Measuring cerebral perfusion with [11C]-PiB R1 in down syndrome: associations with amyloid burden and longitudinal cognitive decline. Brain Commun. (2020) fcaa198, doi: 10.1093/braincomms/fcaa198
25. Annus T, Wilson LR, Acosta-Cabronero J, Cardenas-Blanco A, Hong YT, Fryer TD, et al. The Down syndrome brain in the presence and absence of fibrillar beta-amyloidosis. Neurobiol Aging. (2017) 53:11–19. doi: 10.1016/j.neurobiolaging.2017.01.009
26. Dickerson BC, Bakkour A, Salat DH, Feczko E, Pacheco J, Greve DN, et al. The cortical signature of Alzheimer's disease: regionally specific cortical thinning relates to symptom severity in very mild to mild AD dementia and is detectable in asymptomatic amyloid-positive individuals. Cereb Cortex. (2009) 19:497–510. doi: 10.1093/cercor/bhn113
27. Le Bihan D. Diffusion MRI: what water tells us about the brain. EMBO Mol Med. (2014) 6:569–73. doi: 10.1002/emmm.201404055
28. Acosta-Cabronero J, Williams GB, Pengas G, Nestor PJ. Absolute diffusivities define the landscape of white matter degeneration in Alzheimer's disease. Brain. (2010) 133:529–39. doi: 10.1093/brain/awp257
29. Powell D, Caban-Holt A, Jicha G, Robertson W, Davis R, Gold BT, et al. Frontal white matter integrity in adults with Down syndrome with and without dementia. Neurobiol Aging. (2014) 35:1562–9. doi: 10.1016/j.neurobiolaging.2014.01.137
30. Schmidt-Erfurth U, Bogunovic H, Sadeghipour A, Schlegl T, Langs G, Gerendas BS, et al. Machine learning to analyze the prognostic value of current imaging biomarkers in neovascular age-related macular degeneration. Ophthalmol Retina. (2018) 2:24–30. doi: 10.1016/j.oret.2017.03.015
31. Qi C, Li Y, Fan X, Jiang Y, Wang R, Yang S, et al. A quantitative SVM approach potentially improves the accuracy of magnetic resonance spectroscopy in the preoperative evaluation of the grades of diffuse gliomas. Neuroimage Clin. (2019) 23:101835. doi: 10.1016/j.nicl.2019.101835
32. Kassraian-Fard P, Matthis C, Balsters JH, Maathuis MH, Wenderoth Promises N. Pitfalls, and basic guidelines for applying machine learning classifiers to psychiatric imaging data, with autism as an example. Front Psychiatry. (2016) 7:177. doi: 10.3389/fpsyt.2016.00177
33. Zacharaki EI, Wang S, Chawla S, Soo Yoo D, Wolf R, Melhem ER, et al. Classification of brain tumor type and grade using MRI texture and shape in a machine learning scheme. Magn Reson Med. (2009) 62:1609–18. doi: 10.1002/mrm.22147
34. Jackson GD, Calamante F, Tournier JD, Abbott DF, Connelly A. New anatomic MRI techniques. Epilepsia. (2010) 1:80–2. doi: 10.1111/j.1528-1167.2009.02455.x
35. Morgan SE, Seidlitz J, Whitaker KJ, Romero-Garcia R, Clifton NE, Scarpazza C, et al. Cortical patterning of abnormal morphometric similarity in psychosis is associated with brain expression of Schizophrenia-related genes. Proc Natl Acad Sci USA. (2019) 116:9604–9. doi: 10.1073/pnas.1820754116
36. Franzmeier N, Neitzel J, Rubinski A, Smith R, Strandberg O, Ossenkoppele R, et al. Alzheimer's Disease Neuroimaging, Functional brain architecture is associated with the rate of tau accumulation in Alzheimer's disease. Nat Commun. (2020) 11:347. doi: 10.1038/s41467-019-14159-1
37. Trattnig S, Springer E, Bogner W, Hangel G, Strasser B, Dymerska B, et al. Key clinical benefits of neuroimaging at 7T. Neuroimage. (2018) 168:477–89. doi: 10.1016/j.neuroimage.2016.11.031
38. Giuliano A, Donatelli G, Cosottini M, Tosetti M, Retico A, Fantacci ME. Hippocampal subfields at ultra high field MRI: an overview of segmentation and measurement methods. Hippocampus. (2017) 27:481–94. doi: 10.1002/hipo.22717
39. Suthana NA, Donix M, Wozny DR, Bazih A, Jones M, Heidemann RM, et al. High-resolution 7T fMRI of human hippocampal subfields during associative learning. J Cogn Neurosci. (2015) 27:1194–206. doi: 10.1162/jocn_a_00772
40. Winkler AM, Webster MA, Brooks JC, Tracey I, Smith SM, Nichols TE. Non-parametric combination and related permutation tests for neuroimaging. Hum Brain Mapp. (2016) 37:1486–511. doi: 10.1002/hbm.23115
Keywords: Down's syndrome (DS), Alzheimer's disease, multi-modal, MRI, PET, neuroimaging
Citation: Brown SSG, Mak E and Zaman S (2021) Multi-Modal Imaging in Down's Syndrome: Maximizing Utility Through Innovative Neuroimaging Approaches. Front. Neurol. 11:629463. doi: 10.3389/fneur.2020.629463
Received: 14 November 2020; Accepted: 08 December 2020;
Published: 07 January 2021.
Edited by:
Volker Rasche, University of Ulm, GermanyReviewed by:
Yann Quidé, University of New South Wales, AustraliaCopyright © 2021 Brown, Mak and Zaman. This is an open-access article distributed under the terms of the Creative Commons Attribution License (CC BY). The use, distribution or reproduction in other forums is permitted, provided the original author(s) and the copyright owner(s) are credited and that the original publication in this journal is cited, in accordance with accepted academic practice. No use, distribution or reproduction is permitted which does not comply with these terms.
*Correspondence: Stephanie S. G. Brown, c2IyNDAzQG1lZHNjaGwuY2FtLmFjLnVr; Elijah Mak, ZmttMjRAbWVkc2NobC5jYW0uYWMudWs=
†These authors have contributed equally to this work
Disclaimer: All claims expressed in this article are solely those of the authors and do not necessarily represent those of their affiliated organizations, or those of the publisher, the editors and the reviewers. Any product that may be evaluated in this article or claim that may be made by its manufacturer is not guaranteed or endorsed by the publisher.
Research integrity at Frontiers
Learn more about the work of our research integrity team to safeguard the quality of each article we publish.