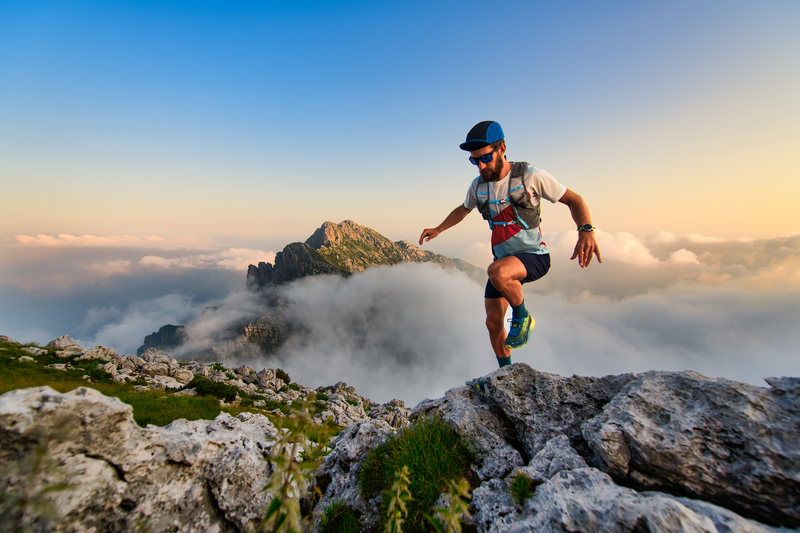
94% of researchers rate our articles as excellent or good
Learn more about the work of our research integrity team to safeguard the quality of each article we publish.
Find out more
ORIGINAL RESEARCH article
Front. Neurol. , 14 January 2021
Sec. Applied Neuroimaging
Volume 11 - 2020 | https://doi.org/10.3389/fneur.2020.606592
This article is part of the Research Topic Multi-Modal Imaging in Neurological Conditions: Translational Applications View all 9 articles
Purpose: Previous studies have shown that HIV affects striato-cortical regions, leading to persisting cognitive impairment in 30–70% of the infected individuals despite combination antiretroviral therapy. This study aimed to investigate brain functional dynamics whose deficits might link to early cognitive decline or immunologic deterioration.
Methods: We applied sliding windows and K-means clustering to fMRI data (HIV patients with asymptomatic neurocognitive impairment and controls) to construct dynamic resting-state functional connectivity (RSFC) maps and identify states of their reoccurrences. The average and variability of dynamic RSFC, and the dwelling time and state transitioning of each state were evaluated.
Results: HIV patients demonstrated greater variability in RSFC between the left pallidum and regions of right pre-central and post-central gyri, and between the right supramarginal gyrus and regions of the right putamen and left pallidum. Greater variability was also found in the frontal RSFC of pars orbitalis of the left inferior frontal gyrus and right superior frontal gyrus (medial). While deficits in learning and memory recall of HIV patients related to greater striato-sensorimotor variability, deficits in attention and working memory were associated with greater frontal variability. Greater striato-parietal variability presented a strong link with immunologic function (CD4+/CD8+ ratio). Furthermore, HIV-infected patients exhibited longer time and reduced transitioning in states typified by weaker connectivity in specific networks. CD4+T-cell counts of the HIV-patients were related to reduced state transitioning.
Conclusion: Our findings suggest that HIV alters brain functional connectivity dynamics, which may underlie early cognitive impairment. These findings provide novel insights into our understanding of HIV pathology, complementing the existing knowledge.
Despite combination antiretroviral therapy (cART) use, 30–70% of the HIV-infected individuals develop neurocognitive disorders (HAND) (1–3). The brain-communicating systems corresponding to cognitive and behavioral functions are susceptible to pathological features of HIV infection (4, 5). Neurocognitive deficits for HIV patients include attention and working memory, motor control, visuospatial processing, and executive functioning (6). The neural mechanisms underlying these impairments remain largely unclear. It has been shown that magnetic resonance imaging (MRI) can be sensitive to brain changes linked to impairments (1, 7–9).
Gray matter loss in sensorimotor cortices and basal ganglia (striatum) has been reported in patients with HIV (10–12). This volume deficit is overly associated with CD4+ lymphocyte counts and HAND in severely immunosuppressed patients (13, 14). Alterations of striato-cortical regional activities (ReHo) and amplitude of low-frequency fluctuations (ALFF) relating to patients' CD4+T-cell counts and waning cognitive learning and memory have also been reported (15, 16). Studies of asymptomatic HIV patients, however, have reported that antiretroviral drugs could delay or improve brain activity (17). For example, switching from efavirenz to rilpivirine appeared to improve functional connectivity of the dorsal attention network along with enhancing working memory, speed of visual processing, and executive function while switching from raltegravir to dolutegravir appeared to improve the dorsal attention and associative visual and sensory-motor networks (17). Studies have also suggested that assessing functional interactions of multiple regions could reveal more brain changes underlying brain functionality losses in HIV patients (18).
Now, studies focused on resting-state functional connectivity (RSFC) analyses have found attenuation in striato-cortical functional connectivity in HIV patients (19, 20). The most consistent impaired functional connectivities involved striatal, lateral prefrontal, and parietal regions, and regions of the default mode network (DMN) (19, 20). In cortical regions, altered connectivity was reported within PFC areas and between PFC and parietal cortex (19–22). The salience and executive networks also demonstrated intrinsic hypo-connectivity, but hyper-connectivity was found between regions of DMN and regions of frontoparietal and sensorimotor networks (23, 24). These changes were related to deteriorating cognitive functions (22, 23). Emerging research suggests that brain interactions are inherently dynamic and thus studying dynamic properties of these interactions could provide more insight into disease pathology (25–27).
A full-scale characterization of complex dynamic processes may be difficult, but low-scale dynamics such as temporal variability and properties of states of reoccurrence of dynamic RSFC may describe disease pathology beyond static RSFC (28, 29). Deficits in these properties have already been reported in patients with major depression (30), Parkinson's disease (31), autism (32), and schizophrenia (33, 34). For example, patients with Parkinson's disease showed within-network sparse connectivity in states with shorter dwelling time (the duration spent by the participant in a certain state) (31). On the other hand, patients with schizophrenia exhibited a longer time in hypo-connected states but a shorter time in hyper-connected states (33, 35). While temporal variability, the standard deviation of correlation across windowed time, is generally considered to reflect levels of brain adaptability and flexibility relating to learning (32), prefrontal variability is also involved in thinking and planning, a property which is altered in major depression (28, 36). Together with other studies (37, 38), these findings suggest that dynamic RSFC could reliably capture cognitively relevant pathogenic features.
In this study, an attempt is made to detect HIV-associated dynamic changes that may underlie persisting cognitive impairments. With sliding windows and K-means clustering applied to the fMRI data of HIV-infected patients adhering to cART but presenting asymptomatic neurocognitive impairment (ANI) and healthy controls, we construct the whole-brain dynamic RSFC maps and further assess the strength (average) and temporal variability of dynamic RSFC, and state properties: state network topology; mean dwelling time; and state transitions—the number of times a switch occurs from one state to the other. The current study is designed to capture a wide range of state transitions, including specific-state-to-specific-state transitioning in an effort to identify cognitively relevant state transitioning changes (39). We speculate that HIV impairs functional dynamics and that dynamic RSFC deficit (temporal variability or state transitioning) may be related to disease severity (the decline of CD4+/CD8+ ratio and CD4+ T-cell counts) and cognitive performances, suggesting their role in early HAND.
Sixteen HIV-infected patients (mean age ± standard deviation = 30.31 ± 7.21 years; 16 male) with asymptomatic neurocognitive impairment (early HAND) and 16 age- and gender-matched healthy controls (33.00 ± 5.51 years; 16 male) participated in the current study. Neuroimaging and clinical assessment data were collected at the Beijing YouAn Hospital, the Capital Medical University between March 2016 and November 2016. HIV-1 seropositivity with ANI in sustained stable cART regimen [tenofovir (TDF) + lamivudine (3TC) + efavirenz (EFV)] for at least 12 weeks was an inclusion criterion for HIV-participants. Any record of illicit drugs and alcohol use, cerebral atrophy, brain lesions, head injury, or neurological disorders was the other factor for exclusion.
The clinical assessment characteristics are summarized in Table 1. Given that CD4+ T-cell counts, CD4+/CD8+ ratio, and plasma viral loads were most closely associated with earlier alterations of the brains of HIV patients and are considered to be potential predictors of HIV disease severity (9, 16, 22, 40), we assessed these parameters.
The evaluation of HAND severity was achieved by referring to the Frascati criteria (2). Each patient received a battery of neuropsychological tests for HAND 2 h before the MRI scan. The performances for six cognitive domains were evaluated: (1) learning and recall was assessed using the Hopkins Verbal Learning Test-Revised (HVLT-R) and the Brief Visuospatial Memory Test-Revised (BVMT-R); (2) motor function using the Grooved Pegboard test; (3) abstract and executive function by the Wisconsin Card Sorting Test-64 (WCST-64); (4) information processing speed by the trail making test part A; (5) verbal and language using category fluency and animal naming test; and (6) attention and working memory using the Continuous Performance Test Identical Pairs (CPT-IP), the Wechsler Memory Scale-III (WMS-III), and Paced Auditory Serial Addition Test (PASAT) (also see Supplementary Table 2). Test scores were standardized in T-score. For a cognitive domain tested by multiple testing methods, T-scores were averaged across tests to obtain a composite T-score for the specific domain. A patient was thus considered to have ANI if at least two cognitive domains were impaired (performance of at least 1 SD below the mean for norms on neuropsychological tests) without decreased everyday functioning (2).
All imaging was conducted on a 3T (Siemens) Scanner (Allegra, Siemens Medical System, Erlangen, Germany). The protocol includes high-resolution T1-weighted anatomical images. fMRI data were collected with an echo-planar imaging (EPI) sequence (see Supplementary Table 1 for imaging parameter settings).
Data were pre-processed using Data Processing and Analysis for Brain Imaging (DPABI V3.0, http://rfmri.org/) (41) and MATLAB (MATLAB and Statistics Toolbox Release 2018a, The Mathworks, Inc., Natick, Massachusetts, United States). Pre-processing steps were as follows: (a) correcting slice-timing; (b) motion correction by realigning images; (b) reorienting structural and functional images manually; (c) co-registering structural images into functional images and segmenting to gray matter, white matter, and cerebrospinal fluid; (d) reducing potential influences of motion-related artifacts from functional data through (i) regressing out 24 Friston-model head-motion estimates and nuisance covariates (white matter and cerebrospinal fluid signals) (42) and (ii) scrubbing outlier volumes with mean framewise displacement (FD) >0.2 mm using spline interpolation (19, 43, 44); and (e) normalizing functional images to Montreal Neurological Institute standard space by Diffeomorphic Anatomical Registration Through Exponentiated Lie algebra Method (45) and reslicing to 3.0 × 3.0 × 3.0 mm3.
(a) Noises associated with machine instability were minimized by removing the first 10 image volumes prior to pre-processing, resulting in 230 volumes. (b) Low-frequency drifts and high-frequency aliasing were band-pass filtered (0.01–0.08 Hz) after data normalization (d). Only data with maximum translation <1 mm and rotation <1.0 degree were included for further analysis. With respect to these confounding factors, further analyses were performed with 16 HIV patients and 16 HCs.
To test whether confounding variables (age and mean FD) present significant differences between the groups, two-sample t-tests were performed using SPSS software [IBM SPSS Statistics for Windows, version 20.0 (IBM Corp., Armonk, N.Y., USA)]. No significant differences in age (p > 0.05; Table 1) was observed; however, HIV patients had a relatively lower mean FD (p < 0.05) compared with controls.
The brain networks were constructed from 90 ROIs defined in an automated anatomical labeling (AAL-90) atlas (46). The AAL-90 atlas does not include the cerebellum and is the most popular atlas widely used to identify brain changes in recent years (47). The regions in the networks were partitioned according to their locations in the brain, yielding six subnetworks, namely prefrontal, frontal, parietal, occipital, temporal, and subcortical subnetworks (48). To test whether there is an intersection between the static and dynamic RSFC or whether they are supplemental to each other in understanding the neural mechanisms underlying early cognitive impairment in HIV, we computed static z-transformed Pearson's correlations over a full range of time (230-image volumes).
For dynamic RSFC analyses, we first constructed dynamic correlation maps by sliding a window (WL = 30 s) to 230-TR fMRI data (data length, 460 s), conforming to the procedures described by Allen et al. (25). The window was designed to detect a wide range of transitions as described in the previous study (39). The proposed window length (WL = 30 s) can separate cognitive process-specific dynamics while keeping better correlation estimates (37, 49). Having slid the window by the 2-s step size (1TR) (25), 216 (230 – 15 + 1) windowed correlation maps were generated for each subject. Two hundred and sixteen Fisher's z-transformed correlation maps (90 × 90 matrices) were produced, regarded as dynamic RSFC maps.
To understand how properties of dynamic RSFC behave in HIV individuals relative to healthy controls, we evaluated the temporal variability (RSFC-SD) and strength (RSFC-STR) of the dynamic RSFC (28, 50, 51). Thus, for each subject, the temporal variability of dynamic RSFC was obtained by computing the standard deviation of each ROI–ROI correlation pair across all dynamic windows (N = 216), reflecting the dynamic reconfiguration of a brain into distinct functional modules at a different time and is indicative of brain flexibility and adaptability (52, 53). The strength of dynamic RSFC was estimated by averaging ROI–ROI correlation pairs across all windows (N = 216) (Figure 1 and Supplementary Table 3).
Figure 1. Illustrations of procedures for dynamic RSFC and state analyses. Ninty ROIs' times series were extracted from fMRI data (230 TR) and sliding windows (WL = 30 s) were used to generate dynamic RSFC maps. Dynamic RSFC properties (average dynamic RSFC, and connectivity variability) were computed across windows. Optimal clusters (states, k = 1–5) were generated using K-means clustering. State properties [the mean dwelling time of the state (MDT), and state transitioning (PT, PSPT)] were further estimated from each state. RSFCwi represents dynamic RSFC map at window wi; MDT and PT denote the mean dwelling and probability of transitioning to statek respectively, whereas PSPT signifies the probability of specific transitioning from statej to statek.
Then, we adopted a k-means algorithm with L1 distance function to estimate the dominant recurrent patterns—regarded as states—of the dynamic RSFC (25, 31, 32). From 216 windows × 4,005 features of each subject, we identified windowed covariance matrices (exemplars) with local maxima to use for clustering initialization (54). This reduces redundancy between windows and computational load during clustering (25). One thousand and sixty-four exemplars were identified and adopted for initial clustering with 500 repetitions to escape local minima (55). The resulting centroids were used to initialize clustering for all data (32 participants × 216 windows = 6,912 instances). Similar to Abrol et al. (54), we estimated the valid optimal number of clusters (from k = 2–20) using the cluster validity index based on the elbow criterion (54). The resulting cluster medians were regarded as the dominant RSFC states. The reproducibility of these states was in accordance with Abrol et al. (54) and Allen et al.'s (25) studies (25, 54).
To investigate the effects that HIV may have on the properties of transient states, we examined three state properties—mean dwelling time, state transitioning, and state network topology. The mean dwelling time measured by the metric MDT reflects the time an individual spends in a particular state (27, 51, 56, 57). State transitioning measured by the metric PT denotes the probability of transitioning to a particular state i from other states (i ≠ k) (33, 58, 59). Further, we extended characterizing state transitioning by quantifying the transition from specific state j to specific state k (j ≠ k), measured by the metric PSPT (the likelihood of the state j to transit to state k and not otherwise) (Figure 1 and Supplementary Table 3). Network configurations of the states demonstrating alterations in MDT, PT, or PSPT were further assessed (31, 33, 60).
The general linear model (GLM) examined the differences in static and dynamic RSFC between HIV patients and healthy controls. Results were further false discovery rate-corrected (p < 0.05, FDR) with age and mean FD as covariates using the Gretna toolbox (61). Permutation tests (p < 0.05) evaluated the differences between groups in state properties (MDT, PT, and PSPT) (56). Pearson's correlations were performed to examine the relationship between imaging markers and clinical measures using SPSS software, controlling for age, and mean FD (p < 0.05). The relationship between altered properties of RSFC and states was further assessed.
Our results showed significantly increased static RSFC between the right caudate nucleus (CAU.R) and left pre-central gyrus (PreG.L), and between the right caudate nucleus (CAU.R) and left inferior parietal gyrus (IPL.L) in HIV patients (Figure 2A and Table 2). The rest of the regions did not report significant differences in static RSFC between the groups.
Figure 2. Static and dynamic functional connectivity. (A) The static RSFC maps for HIV and HC groups, group difference map (HIV-HC), and between-group contrast. (B) The average dynamic RSFC maps for HIV and HC groups, group difference maps (HIV-HC), and between-group contrast in average dynamic RSFC. (C) The mean dynamic temporal variability maps for HIV and HC groups, and group variability-difference maps (HIV-HC). (D) The group difference for temporal variability as depicted in the histogram. PFR, prefrontal regions; FR, other frontal regions; PR, parietal regions; OR, occipital regions; TR, parietal regions; and SUB, subcortical regions. R, right; L, left; CAU, the caudate nucleus; IPL, inferior frontal lobe; and PreCG, precentral gyrus; SMG, supramarginal gyrus, PUT, putamen; PAL, pallidum; PoCG, postcentral gyrus; SFGmed; superior frontal (medial) gyrus; ORBinf, the pars of orbitalis of the inferior frontal gyrus. Pearson's correlation coefficients were all fisher's z-transformed. The group-difference (HIV-HC) connectivity maps are displayed as –log10 (p-value) × sign (t).
A significant increase in the strength (average) of dynamic RSFC was also reported between the right caudate nucleus and left pre-central gyrus, and between the right caudate nucleus and left inferior parietal gyrus in HIV patients, compared to healthy controls (Figure 2B and Table 2). The average dynamic RSFC of other regions did not reach significance.
Compared to healthy controls, temporal variability of dynamic RSFC in HIV patients was greater between the right supramarginal gyrus (SMG.R) and right putamen; the right SMG and left pallidum; the left pallidum and right post-central gyrus; the left pallidum (PAL.L) and right pre-central gyrus (PreCG.R); and the right superior frontal (medial) gyrus (SFGmed.R) and pars orbitalis of the left inferior frontal gyrus (ORBinf.L) (Figures 2C,D and Table 2). No significant differences were observed in the dynamic RSFC variability of other functional connections.
States and their features are shown in Table 3. The clustering analyses suggested five transient states, State 1 (S1) with 34% (N = 2,379) of the proportion of dynamic correlation maps, State 2 (S2) with 20% (N = 1,371), State 3 (S3) with 18% (N = 1,274), State 4 (S4) with 16% (N = 1,072), and State 5 (S5) with 12% (N = 816). State 1 and state 5 were characterized by globally hypo-connected and hyper-connected patterns, respectively. State 2 was typified by weaker occipital connectivity. State 3 had frontal and prefrontal hypo-connectivity. State 4 was associated with weaker subcortical connectivity (Figure 3). Compared with controls, HIV patients had lower transitions (PT) to state 4. Reduced state transitioning (PSPT) from state 4 to state 1 and from state 2 to state 4 was also observed. Furthermore, we found that patients with HIV had higher dwelling time (MDT) in state 2 compared with healthy controls (Figure 3 and Table 3).
Table 3. Group difference in state properties between HIV and HC groups and categorization of state network connectivity.
Figure 3. States properties of HIV infected individuals and healthy controls. (A) Five transient states generated by K-means clustering analysis, displayed with the number of correlation maps constituting to the state. (B) Mean dwelling time (MDT) between groups. (C) The probabilities of transitioning (PT) for each state in HIV and HC groups. (D) The probabilities of specific state-to-state transitioning (PSPT). On top of the figures are the numbers (N=) of dynamic correlation maps [with their percentages (%)] showing the population of correlation maps assigned to those states during clustering. Group differences (HIV-HC) were significant at p < 0.05.
Correlation results are reported in Figure 4. Neither neuropsychological results nor blood results were correlated with static RSFC. However, in dynamic RSFC analyses, altered dynamic RSFC variability and cognitive performances revealed significant relationships. Specifically, greater dynamic RSFC variability of PAL.L-PreCG.R showed a positive relationship with cognitive Leaning and Recalling (memory) (Figure 4A; P = 0.005, r = 0.659). We also observed a strong association between the dynamic variability of SFGmed.R-ORBinf.L and cognitive attention/working memory (Figure 4B; P = 0.022, r = 0.566). While the variability of PAL.L-SMG.R was related to CD4+/CD8+ ratio (Figure 4C; P = 0.015, r = 0.655), reduced state transitioning and CD4+ T-cell counts were negatively correlated (Figure 4D; PT, state 4, P = 0.013, r = −0.622; PSPT, state 2-to-state 4, P = 0.046, r = −0.521).
Figure 4. Correlations of properties of dynamic RSFC and RSFC states with clinical characteristics of HIV patients. (A) Correlation of PAL.L-to-PreCG.R temporal variability with learning and recall score. (B) Association of temporal variability of SFGmed.R-to-ORBinf-L with Attention/working memory performance. (C) The relationship between PAL.L-SMG.R variability and CD4+/CD8+ ratio. (D) Relationships of probabilities of transitioning to state 4, and state-2-to-state-4 transitioning with CD4+ T-cell counts. The displayed relationships were evaluated in Pearson's correlations coefficients, r; p < 0.05. P, p-value; L, left; R, right; PAL, the pallidum; PreCG, precentral gyrus; SFGmed, superior frontal (medial) gyrus; ORBinf, pars orbitalis of the inferior frontal gyrus; and SMG, supramarginal gyrus.
In HIV patients, fewer transitions (PT) to state 4 demonstrated strong correlations with altered static RSFC of CAU.R-to-PreCG.L (P = 0.003, r = −0.686) and CAU.R-IPL.L (P = 0.031, r = −0.518; Supplementary Figure 3A). A similar relationship existed between PT of state 4 and average dynamic RSFC (STR) of CAU.R-to-PreCG.L (Supplementary Figure 3B; P = 0.026, r = −0.553). Results also showed that higher mean dwelling time (MDT) of state 2 was related to average dynamic RSFC of CAU.R-IPL.L (Supplementary Figure 3C; P = 0.031, r = 0.540).
To test the reproducibility of these results at 30–60 s window sizes, we examined properties of dynamic RSFC across different window sizes [30 s (15TR), 44 s (22TR), and 60 s (30TR)]. We found that results of both average dynamic RSFC (Supplementary Figure 1) and temporal variability (Supplementary Figure 2) were reproducible within the specified range of sliding window size, consistent with previous empirical studies (29, 32, 37, 49). We also assessed the consistency of our results using network-based statistics (FDR, corrected) (62) and found that our findings were also consistent.
The present study investigated the dynamic aspects of RSFC, providing insight into understanding HIV pathology. We presented methodological improvements designed to capture low-level state-to-state transitions. To the best of our knowledge, this study is the first to explore the dynamic functional connectivity in HIV patients using fMRI data.
Increased caudate functional connectivity to pre-central and inferior parietal regions observed in both static and dynamic (average) RSFC suggests that average dynamic RSFC estimated across windows complement to static RSFC estimated over the whole BOLD signals over time. Moreover, these results may signify that static RSFC can be deduced from dynamic RSFC and that dynamic RSFC provides a broader characterization of functional changes. Several previous cross-sectional studies have suggested that the caudate nucleus and the primary sensorimotor, pre-motor, and inferior parietal cortices are selectively vulnerable in HIV disease (12, 63, 64). It is worth noting that the caudate nucleus and primary sensorimotor cortex integrate during motor control and learning functioning; thus, the observed alterations in caudate-to-pre-central connectivity suggest a possible HIV-associated brain injury that might impair spatial-motor memory and learning (12, 64). Previous studies have also detected neural infiltration of the inferior parietal lobe in the caudate nucleus (65), implying the existence of communication between these regions. Melrose et al. (66) found that this communication is susceptible to HIV infection. For example, during semantic event sequencing, patients with HIV exhibited greater caudate-to-parietal connectivity (66). Our results demonstrating increased caudate-parietal connectivity support these studies that disrupted communication between subsystems of these regions is attributed to HIV infection. Since neurons of the brain are multimodal (involved in differential brain functions) (67–70), increases in caudate connectivity to IPL and pre-central gyrus may reflect compensatory plasticity necessitated to retain their functions following HIV injury.
Previous studies have demonstrated how brain flexibility and plasticity are important for learning ability, gene expression, and fluid intelligence (29, 32, 71). The brain reconfigures its functional interactions or circuitry over time while learning, a property which is vulnerable to injuries, neurological disease, psychiatric disorders, or cognitive aging (72). Our results illustrating increasing striato-sensorimotor variability with cognitive learning and recall may suggest (i) the striato-sensorimotor connectivity and its flexibility are relevant to human learning and recall. Thus, it can be used for the prediction of learning capacity. This hypothesis could be supported by previous studies that demonstrated how these regions play an important role in early, long-term, and categorical learning or that linked the dynamics of these regions with learning stage (early, middle, or late) predictions (72–77). (ii) These findings also suggest that striato-sensorimotor circuitry may be one of the primary targets of HIV infection, consistent to other previous studies (15). (iii) Moreover, altered striato-sensorimotor dynamics might be a functional basis for cognitive impairments, with the hypothesis that intensively lowered variability reflecting severe learning deficits. (iv) Increases in striato-sensorimotor temporal variability in patients on cART might be a sign of brain compensatory mechanisms or cART-necessitated CN immune improvements triggering plasticity to restore or preserve brain functions. This can also be supported by earlier studies showing that cART improves neural functions (20), and those increasing dynamics are likely to occur after rehabilitation to restore brain functionality (32), or in response to cognitive demands (78).
Our findings demonstrating greater dynamic RSFC variability between the pars orbitalis of the left inferior frontal and right frontal (medial) gyri related to attention and working memory in HIV patients may point to the fact that brain flexibility may not be limited to functions such as injury recovery, adaptive responses, or a growth mindset (79–82) but rather extends to a wide range of roles, including attention and working memory. PFC-system reconfigurations may be required for capturing temporal storage and active manipulation of the stored information (78). Frontal regions' participation in attention and working memory has already been explained previously (83–85). Studies have reported that the execution of attention and working memory highly requires the flexibility of frontal and or frontal-related frontal systems than non-frontal systems (78). This may be the case since the lateral prefrontal cortices (ventrolateral PFC and dorsolateral PFC) are functionally and anatomically connected through arcuate fibers (86, 87), becoming critical nodes that work together for a variety of cognitive functions (87). They are also connected to the executive network and DMN dorsolaterally to control for the dynamic allocation of attention (88). Furthermore, increases in frontal variability observed in HIV patients suggest attenuations HIV might have in temporal reconfigurations of these PFC circuits, agreeing with previous HIV studies demonstrating attenuated activation in PFC during complex attention, and working memory tasks in infected male patients (7, 89).
Greater variability found in the dynamic RSFC between supramarginal and regions of striatum (putamen and pallidum) also suggests abnormal patterns associated with HIV. This could be supported by the observed strong correlation (especially, in PAL.L-SMG.R) with the CD4+/CD8+ ratio. This result confirms the evidence derived from static RSFC studies that HIV pathology includes impaired communication between striato-parietal regions (20, 22, 90–92), consistent with our static results of attenuated caudate–IPL connectivity. The positive relationship between variability and CD4+/CD8+ ratio may also indicate the ability of temporal variability to predict the progress of HIV in the brain or the decline of CNs immune systems. Given that the CD4+/CD8+ ratio is a more accurate hallmark of the status of the body immune system (93, 94), we speculate that monitoring of trends of the CN CD4+/CD8+ ratio balance on differential antiretroviral therapies can be achieved through tracking the temporal variability, supporting earlier studies detecting functional changes with switching antiretroviral drugs (17). Again, since cART repairs innate CNs by reducing inflammation and enhances synoptodentritic plasticity that improves dynamic synaptic communication and neural functions (4, 95), we hypothesize that the observed variability increases in HIV patients on stable cART might also be attributed to cART stabilization; however, further evidence is required to confirm this supposition.
In this study, we observed five hypo/hyper-connected states suggestive of large-scale brain networks being characterized by hypo/hyper-connected patterns, consistent with other dynamic RSFC studies (31, 32, 96). In contrast to a study by Mash et al. (52) who considered clustering the data of patients and healthy controls separately, this study did not cluster data separately. This conformed to a large body of earlier studies (33, 56). It is worth noting that clustering the groups separately could result in mismatched clusters (52). The maximum whole correlation approach often used to estimate the paring clusters is also likely to match two or more clusters to a cluster of another group (52), which would affect the analyses. Furthermore, we hypothesize that clustering groups separately influence group differences fundamentally as clustering foundations such as local minima's are data-dependent, which are likely to be different between groups.
As noted above, state 2 was typified by weaker connectivity within occipital networks, paralleled by a longer dwelling time pattern of the state. Several studies of HIV have identified structural and functional abnormalities in the occipital lobe, suggesting the presence of HIV-associated injuries (3, 12, 97–101). The cortical thickness of the occipital cortex is one of the major domains, which is progressively affected by HIV (12, 101). The neurons of the visual cortex of the occipital lobe are also known to undergo atrophy in HIV patients (98, 99). A study by R. Li et al. found a deficiency in spontaneous activity of the occipital lobe in early HIV infection (100). While a dysfunction of the occipital lobe was not clinically implicated in HAND in earlier studies, sufficient evidence implicated HIV in gray matter loss, supported by their associations to CD4+ T-cell counts (102). Later studies revealed a link between impaired spontaneous activity in the occipital cortex and deficits in executive function or CD4+/CD8 ratio (100). The clinical picture of the relationship between HAND and deficits in the occipital lobe in patients without and with cART is increasingly becoming clear. Emerging evidence suggests that generalized degradation of gray matter, disruption of spontaneous activity, and attenuation of functional activation and connectivity, involving the occipital cortex, could explain deficits seen in visual attention and visuospatial processing in patients with HIV (3, 89). Our observed changes in temporal properties of dynamic RSFC which depict altered dwelling time in states with weaker occipital connectivity may be related to an initial dysfunction of the occipital cortex and are in support of the above hypothesis. In other aspect, increased amount of time spent could be interpreted as a potential compensatory mechanism of the brain networks resulting in prolonged stay in occipitally hypoconnected state.
In HIV patients, state transitioning (PT) of state 4 typified by weaker connectivity in subcortical networks was reduced, paralleled by reduced switching (PSPT) from state 4 to state 1 and from state 2 to state 4. Regions of the subcortical networks are susceptible to HIV (20). Among other effects, gray matter volume loss, activity modulation loss, subcortical network integration loss have been reported (10, 19). The reduction of state transitioning of the state with weaker subcortical connectivity (state 4) was observed to be negatively correlated with CD4+T-cell counts, possibly indicating an association between HIV severity and switching between states. Reduced switching from state 2 to state 4 also presented a similar pattern of association to HIV severity, a finding that can only be detected precisely at low-level of state transitioning analyses. HIV has been postulated to have varying effects on the brain (103). Therefore, among the possibilities of this reversed relationship could be evidence of such variant effects of HIV or a potential compensatory mechanism resulting in a somewhat increasing state transitioning as CD4+ T-cell counts deteriorate following HIV and cART. A similar phenomenon was previously reported (104, 105) between CD4+T-cell counts and static RSFC.
One would like to relate our observed results across diseases. Among the findings worth noting is that while HIV patients appeared to spend a longer time in states typified by weaker connectivity in occipital networks, parallel to patients with schizophrenia (33, 35), patients with Parkinson's disease spent a shorter time in states with weaker connectivity (31). Findings showing patients with schizophrenia spending a shorter time in states with strong connectivity in global networks are inconsistent with those we found in HIV patients, as neither MDT nor PT of globally hyperconnected states (state 5) presented differences between groups. This may suggest that abnormal patterns related to HIV are associated with region-specific network alterations—such as subcortical and occipital regions alterations (3, 12, 97–101)—rather than global network alterations.
We noticed that the mean dwelling time of the state with weaker connectivity in occipital networks was related to the average dynamic RSFC of the caudate nucleus and regions of the left inferior parietal lobe. Moreover, reduced state transitioning of the state with weaker subcortical connectivity also presented a strong relationship with both static and average dynamic RSFC. Together, these findings demonstrate that state properties may supplement both static and dynamic RSFC in delineate HIV pathology, expanding knowledge to the existing literature.
The present study presents some limitations. Although the number of samples might be relatively larger compared with previous studies of HIV (7, 89), large cohorts are required to validate these findings. This study used data from male patients; this may be common in some of the HIV studies (7, 89, 106) as HIV data availability is challenging. The design of our study was based on the hypothesis that HIV can lead to cognitive dysfunction. Thus, the assessment of cognitive performances did not include healthy controls, consistent with earlier studies (100, 101). However, the lack of neuropsychological tests for healthy controls may also limit the study. It is also important to note that the current study is significant for the majority of the population living with HIV nowadays. Nonetheless, other less represented groups in the HIV+ population worldwide should be assessed in future studies. Both static RSFC and dynamic RSFC analyses were performed using ROI pairs of the whole brain regions defined by AAL-90 atlas, future studies are required to validate these findings with data-driven approaches. In correlation analyses, we did not correct for multiple comparisons, in line with other studies (3, 15). We also suggest that future studies should include gender-matched cohorts.
In the present study, we applied dynamic resting-state functional connectivity analyses to investigate dynamic alterations that may underlie early cognitive impairments in HIV patients on cART. We found that HIV patients exhibit greater temporal variability related to learning, attention and working memory, and CD4+/CD8+ ratio. HIV patients also appear to have reduced state transitioning associated with CD4+T cell counts. We conclude that HIV affects brain connectivity dynamics and that these abnormal dynamic patterns may contribute to persisting cognitive impairments in patients with HIV. These findings also indicate that dynamic RSFC analyses may provide an impetus for detecting other pathological dimensions of HIV on the brain in patients receiving antiretroviral therapy.
The raw data supporting the conclusions of this article will be made available by the authors, without undue reservation.
The studies involving human participants were reviewed and approved by the Ethical Committee of the Capital Medical University and the University of Science and Technology of China. The patients/participants provided their written informed consent to participate in this study.
BN, JZ, YL, and XW substantial contributions to the conception or design of the work. BN, JZ, YWa, YWe, JU, and HL contributions to the acquisition, analysis or interpretation of data. BN, XW, HL, and BQ drafting the work or revising it critically for important intellectual content. HL and BQ final approval of the version submitted. All authors contributed to the article and approved the submitted version.
This work was supported by the National Natural Science Foundation of China (61936013, 81771806, 81701665), the Beijing Municipal Administration of Hospitals Clinical Medicine Development of Special Funding Support (No. ZYLX201511), the Capital Medical University Research and Incubation Funding (PYZ2017124), and the Beijing Municipal Administration of Hospitals Incubating Program (PX2016036).
The authors declare that the research was conducted in the absence of any commercial or financial relationships that could be construed as a potential conflict of interest.
The Supplementary Material for this article can be found online at: https://www.frontiersin.org/articles/10.3389/fneur.2020.606592/full#supplementary-material
1. Abidin AZ, Dsouza AM, Nagarajan MB, Wang L, Qiu X, Schifitto G, et al. Alteration of brain network topology in HIV-associated neurocognitive disorder: a novel functional connectivity perspective. Neuroimage Clin. (2018) 17:768–777. doi: 10.1016/j.nicl.2017.11.025
2. Antinori A, Arendt G, Becker JT, Brew BJ, Byrd DA, Cherner M, et al. Updated research nosology for HIV-associated neurocognitive disorders. Neurology. (2007) 69:1789–99. doi: 10.1212/01.WNL.0000287431.88658.8b
3. Wiesman AI, O'Neill J, Mills MS, Robertson KR, Fox HS, Swindells S, et al. Aberrant occipital dynamics differentiate HIV-infected patients with and without cognitive impairment. Brain. (2018) 141:1678–90. doi: 10.1093/brain/awy097
4. Ellis R, Langford D, Masliah E. HIV and antiretroviral therapy in the brain: neuronal injury and repair. Nat Rev Neurosci. (2007) 8:33–44. doi: 10.1038/nrn2040
5. McIntosh RC, Rosselli M, Uddin LQ, Antoni M. Neuropathological sequelae of human immunodeficiency virus and apathy: a review of neuropsychological and neuroimaging studies. Neurosci Biobehav Rev. (2015) 55:147–64. doi: 10.1016/j.neubiorev.2015.04.008
6. Lew BJ, McDermott TJ, Wiesman AI, O'Neill J, Mills MS, Robertson KR, et al. Neural dynamics of selective attention deficits in HIV-associated neurocognitive disorder. Neurology. (2018) 91:E1860–9. doi: 10.1212/WNL.0000000000006504
7. Ernst T, Chang L, Jovicich J, Ames N, Arnold S. Abnormal brain activation on functional MRI in cognitively asymptomatic HIV patients. Neurology. (2002) 59:1343–49. doi: 10.1212/01.WNL.0000031811.45569.B0
8. Janssen MA, Meulenbroek O, Steens SC, Góraj B, Bosch M, Koopmans PP, et al. Cognitive functioning, wellbeing and brain correlates in HIV-1 infected patients on long-term combination antiretroviral therapy. Aids. (2015) 29:2139–48. doi: 10.1097/QAD.0000000000000824
9. Zhao J, Chen F, Ren M, Li L, Li A, Jing B, et al. Low-frequency fluctuation characteristics in rhesus macaques with SIV infection: a resting-state fMRI study. J Neurovirol. (2019) 25:141–9. doi: 10.1007/s13365-018-0694-5
10. Aylward EH, Hendere JD, McArthur JC, Brettschneider PD, Harris GJ, Barta PE, et al. Reduced basal ganglia volume in HIV-1-associated dementia-Results. J Neurol. (1993) 43:2099–104. doi: 10.1212/WNL.43.10.2099
11. Ragin AB, Du H, Ochs R, Wu Y, Sammet CL, Shoukry A, et al. Structural brain alterations can be detected early in HIV infection. Neurology. (2012) 79:2328–34. doi: 10.1212/WNL.0b013e318278b5b4
12. Thompson PM, Dutton RA, Hayashi KM, Toga AW, Lopez OL. Thinning of the cerebral cortex visualized in HIV/AIDS reflects CD4+ T lymphocyte decline. Proc Natl Acad Sci. (2005) 102:15647–52. doi: 10.1073/pnas.0502548102
13. Heaps JM, Sithinamsuwan P, Paul R, Lerdlum S, Pothisri M, Clifford D, et al. Association between brain volumes and HAND in cART-naive HIV+ individuals from Thailand. J Neurovirol. (2015) 21:105–12. doi: 10.1007/s13365-014-0309-8
14. Thompson PM, Jahanshad N. Novel neuroimaging methods to understand how HIV affects the brain. Curr Hiv/Aids Rep. (2015) 12:289–98. doi: 10.1007/s11904-015-0268-6
15. Yadav SK, Gupta RK, Hashem S, Bhat AA, Garg RK, Venkatesh V, et al. Changes in resting-state functional brain activity are associated with waning cognitive functions in HIV-infected children. Neuroimage Clin. (2018) 20:1204–10. doi: 10.1016/j.nicl.2018.10.028
16. Zhao J, Jing B, Chen F, Liu J, Wang Y, Li H. Altered regional homogeneity of brain spontaneous signals in SIV infected rhesus macaque model. Magn Reson Imaging. (2017) 37:56–61. doi: 10.1016/j.mri.2016.10.019
17. Toniolo S, Cercignani M, Mora-Peris B, Underwood J, Alagaratnam J, Bozzali M, et al. Changes in functional connectivity in people with HIV switching antiretroviral therapy. J Neurovirol. (2020) 26:754–63. doi: 10.1007/s13365-020-00853-0
18. Zang YF, Jiang TZ, Lu YL, He Y, Tian LX. Regional homogeneity approach to fMRI data analysis. Neuroimage. (2004) 22:394–400. doi: 10.1016/j.neuroimage.2003.12.030
19. Ipser JC, Brown GG, Bischoff-Grethe A, Connolly CG, Ellis RJ, Heaton RK, et al. HIV infection is associated with attenuated frontostriatal intrinsic connectivity: a preliminary study. J Int Neuropsychol Soc. (2015) 21:203–13. doi: 10.1017/S1355617715000156
20. Ortega M, Brier MR, Ances BM. Effects of HIV and combination antiretroviral therapy on cortico-striatal functional connectivity. Aids. (2015) 29:703–12. doi: 10.1097/QAD.0000000000000611
21. McIntosh RC, Chow DC, Lum CJ, Hidalgo M, Shikuma CM, Kallianpur KJ. Reduced functional connectivity between ventromedial prefrontal cortex and insula relates to longer corrected QT interval in HIV plus and HIV- individuals. Clin Neurophysiol. (2017) 128:1839–50. doi: 10.1016/j.clinph.2017.07.398
22. Samboju V, Philippi CL, Chan P, Cobigo Y, Fletcher JLK, Robb M, et al. Structural and functional brain imaging in acute HIV. Neuroimage Clin. (2018) 20:327–35. doi: 10.1016/j.nicl.2018.07.024
23. Chaganti JR, Heinecke A, Gates TM, Moffat KJ, Brew BJ. Functional connectivity in virally suppressed patients with HIV-associated neurocognitive disorder: a resting-state analysis. Am J Neuroradiol. (2017) 38:1623–9. doi: 10.3174/ajnr.A5246
24. Egbert AR, Biswal B, Karunakaran KD, Pluta A, Wolak T, Rao S, et al. HIV infection across aging: Synergistic effects on intrinsic functional connectivity of the brain. Prog Neuro Psychopharmacol Biol Psychiatry. (2019) 88:19–30. doi: 10.1016/j.pnpbp.2018.06.006
25. Allen EA, Damaraju E, Plis SM, Erhardt EB, Eichele T, Calhoun VD. Tracking Whole-Brain Connectivity Dynamics in the Resting State. Cereb Cortex. (2014) 24:663–76. doi: 10.1093/cercor/bhs352
26. Beaty RE, Benedek M, Silvia PJ, Schacter DL. Creative cognition and brain network dynamics. Trends Cogn Sci. (2016) 20:87–95. doi: 10.1016/j.tics.2015.10.004
27. Calhoun VD, Miller R, Pearlson G, Adali T. The chronnectome: time-varying connectivity networks as the next frontier in fMRI data discovery. Neuron. (2014) 84:262–74. doi: 10.1016/j.neuron.2014.10.015
28. Kaiser RH, Whitfield-Gabrieli S, Dillon DG, Goer F, Beltzer M, Minkel J, et al. Dynamic resting-state functional connectivity in major depression. Neuropsychopharmacology. (2016) 41:1822–30. doi: 10.1038/npp.2015.352
29. Preti MG, Bolton TAW, Van De Ville D. The dynamic functional connectome: state-of-the-art and perspectives. Neuroimage. (2017) 160:41–54. doi: 10.1016/j.neuroimage.2016.12.061
30. Yao Z, Shi J, Zhang Z, Zheng W, Hu T, Li Y, et al. Altered dynamic functional connectivity in weakly-connected state in major depressive disorder. Clin Neurophysiol. (2019) 130:2096–104. doi: 10.1016/j.clinph.2019.08.009
31. Kim J, Criaud M, Cho SS, Diez-Cirarda M, Mihaescu A, Coakeley S, et al. Abnormal intrinsic brain functional network dynamics in Parkinson's disease. Brain. (2017) 140:2955–67. doi: 10.1093/brain/awx233
32. Zhang J, Cheng W, Liu Z, Zhang K, Lei X, Yao Y, et al. Neural, electrophysiological and anatomical basis of brain-network variability and its characteristic changes in mental disorders. Brain. (2016) 139:2307–21. doi: 10.1093/brain/aww143
33. Damaraju E, Allen EA, Belger A, Ford JM, McEwen S, Mathalon DH, et al. Dynamic functional connectivity analysis reveals transient states of dysconnectivity in schizophrenia. Neuroimage Clin. (2014) 5:298–308. doi: 10.1016/j.nicl.2014.07.003
34. Fu Z, Tu Y, Di X, Du Y, Pearlson GD, Turner JA, et al. Characterizing dynamic amplitude of low-frequency fluctuation and its relationship with dynamic functional connectivity: an application to schizophrenia. Neuroimage. (2018) 180:619–31. doi: 10.1016/j.neuroimage.2017.09.035
35. Du Y, Pearlson GD, Yu Q, He H, Lin D, Sui J, et al. Interaction among subsystems within default mode network diminished in schizophrenia patients: a dynamic connectivity approach. Schizophr Res. (2016) 170:55–65. doi: 10.1016/j.schres.2015.11.021
36. Wise T, Marwood L, Perkins AM, Herane-Vives A, Joules R, Lythgoe DJ, et al. Instability of default mode network connectivity in major depression: a two-sample confirmation study. Transl Psychiatry. (2017) 7:e1105. doi: 10.1038/tp.2017.40
37. Xie H, Zheng CY, Handwerker DA, Bandettini PA, Calhoun VD, Mitra S, et al. Efficacy of different dynamic functional connectivity methods to capture cognitively relevant information. Neuroimage. (2019) 188:502–14. doi: 10.1016/j.neuroimage.2018.12.037
38. Zalesky A, Fornito A, Cocchi L, Gollo LL, Breakspear M. Time-resolved resting-state brain networks. Proc Natl Acad Sci USA. (2014) 111:10341–6. doi: 10.1073/pnas.1400181111
39. Shakil S, Lee C-H, Keilholz SD. Evaluation of sliding window correlation performance for characterizing dynamic functional connectivity and brain states. Neuroimage. (2016) 133:111–28. doi: 10.1016/j.neuroimage.2016.02.074
40. Mbugua KK, Holmes MJ, Cotton MF, Ratai E-M, Little F, Hess AT, et al. HIV-associated CD4/8 depletion in infancy is associated with neurometabolic reductions in the basal ganglia at age 5 years despite early antiretroviral therapy. AIDS (London England). (2016) 30:1353. doi: 10.1097/QAD.0000000000001082
41. Yan CG, Wang XD, Zuo XN, Zang YF. DPABI: data processing and analysis for (resting-state) brain imaging. Neuroinformatics. (2016) 14:339–51. doi: 10.1007/s12021-016-9299-4
42. Friston KJ, Williams S, Howard R, Frackowiak RS, Turner R. Movement-related effects in fMRI time-series. Magn Reson Med. (1996) 35:346–55. doi: 10.1002/mrm.1910350312
43. Power JD, Mitra A, Laumann TO, Snyder AZ, Schlaggar BL, Petersen SE. Methods to detect, characterize, and remove motion artifact in resting state fMRI. Neuroimage. (2014) 84:320–41. doi: 10.1016/j.neuroimage.2013.08.048
44. Xie X, Cao Z, Weng X, Jin D. Estimating intrinsic dimensionality of fMRI dataset incorporating an AR (1) noise model with cubic spline interpolation. Neurocomputing. (2009) 72:1042–55. doi: 10.1016/j.neucom.2008.04.003
45. Ashburner J. A fast diffeomorphic image registration algorithm. Neuroimage. (2007) 38:95–113. doi: 10.1016/j.neuroimage.2007.07
46. Tzourio-Mazoyer N, Landeau B, Papathanassiou D, Crivello F, Etard O, Delcroix N, et al. Automated anatomical labeling of activations in SPM using a macroscopic anatomical parcellation of the MNI MRI single-subject brain. Neuroimage. (2002) 15:273–89. doi: 10.1006/nimg.2001
47. Long Z, Huang J, Li B, Li Z, Li Z, Chen H, et al. A comparative atlas-based recognition of mild cognitive impairment with voxel-based morphometry. Front Neurosci. (2018) 12:916. doi: 10.3389/fnins.2018.00916
48. Wang K, Liang M, Wang L, Tian L, Zhang X, Li K, et al. Altered functional connectivity in early Alzheimer's disease: a resting-state fMRI study. Hum Brain Map. (2007) 28:967–78. doi: 10.1002/hbm.20324
49. Leonardi N, Van De Ville D. On spurious and real fluctuations of dynamic functional connectivity during rest. Neuroimage. (2015) 104:430–6. doi: 10.1016/j.neuroimage.2014.09.007
50. Kung YC, Li CW, Chen S, Chen SCJ, Lo CYZ, Lane TJ, et al. Instability of brain connectivity during nonrapid eye movement sleep reflects altered properties of information integration. Hum Brain Map. (2019) 40:3192–202. doi: 10.1002/hbm.24590
51. Liu F, Wang Y, Li M, Wang W, Li R, Zhang Z, et al. Dynamic functional network connectivity in idiopathic generalized epilepsy with generalized tonic-clonic seizure. Hum Brain Map. (2017) 38:957–73. doi: 10.1002/hbm.23430
52. Mash LE, Linke AC, Olson LA, Fishman I, Liu TT, Müller RA. Transient states of network connectivity are atypical in autism: a dynamic functional connectivity study. Hum Brain Map. (2019) 40:2377–89. doi: 10.1002/hbm.24529
53. Sun J, Liu Z, Rolls ET, Chen Q, Yao Y, Yang W, et al. Verbal creativity correlates with the temporal variability of brain networks during the resting state. Cereb Cortex. (2019) 29:1047–58. doi: 10.1093/cercor/bhy010
54. Abrol A, Damaraju E, Miller RL, Stephen JM, Claus ED, Mayer AR, et al. Replicability of time-varying connectivity patterns in large resting state fMRI samples. Neuroimage. (2017) 163:160–76. doi: 10.1016/j.neuroimage.2017.09.020
55. Laumann TO, Snyder AZ, Mitra A, Gordon EM, Gratton C, Adeyemo B, et al. On the stability of BOLD fMRI correlations. Cereb Cortex. (2017) 27:4719–32. doi: 10.1093/cercor/bhw265
56. Shen H, Li Z, Qin J, Liu Q, Wang L, Zeng L-L, et al. Changes in functional connectivity dynamics associated with vigilance network in taxi drivers. Neuroimage. (2016) 124:367–78. doi: 10.1016/j.neuroimage.2015.09.010
57. Vidaurre D, Smith SM, Woolrich MW. Brain network dynamics are hierarchically organized in time. Proc Natl Acad Sci. (2017) 114:12827–32. doi: 10.1073/pnas.1705120114
58. Díez-Cirarda M, Strafella AP, Kim J, Peña J, Ojeda N, Cabrera-Zubizarreta A, et al. Dynamic functional connectivity in Parkinson's disease patients with mild cognitive impairment and normal cognition. NeuroImage Clin. (2018) 17:847–55. doi: 10.1016/j.nicl.2017.12.013
59. Hutchison RM, Womelsdorf T, Allen EA, Bandettini PA, Calhoun VD, Corbetta M, et al. Dynamic functional connectivity: promise, issues, and interpretations. Neuroimage. (2013) 80:360–78. doi: 10.1016/j.neuroimage.2013.05.079
60. Rashid B, Chen J, Rashid I, Damaraju E, Liu J, Miller R, et al. A framework for linking resting-state chronnectome/genome features in schizophrenia: a pilot study. Neuroimage. (2019) 184:843–54. doi: 10.1016/j.neuroimage.2018.10.004
61. Wang J, Wang X, Xia M, Liao X, Evans A, He Y. GRETNA: a graph theoretical network analysis toolbox for imaging connectomics. Front Hum Neurosci. (2015) 9:386. doi: 10.3389/fnhum.2015.00458
62. Zalesky A, Fornito A, Bullmore ET. Network-based statistic: identifying differences in brain networks. Neuroimage. (2010) 53:1197–207. doi: 10.1016/j.neuroimage.2010.06.041
63. Stout JC, Ellis RJ, Jernigan TL, Archibald SL, Abramson I, Wolfson T, et al. Progressive cerebral volume loss in human immunodeficiency virus infection: a longitudinal volumetric magnetic resonance imaging study. Arch Neurol. (1998) 55:161–8. doi: 10.1001/archneur.55.2.161
64. Zhou Y, Li R, Wang X, Miao H, Wei Y, Ali R, et al. Motor-related brain abnormalities in HIV-infected patients: a multimodal MRI study. Neuroradiology. (2017) 59:1133–42. doi: 10.1007/s00234-017-1912-1
65. Petras JM. Connections of parietal lobe. J Psychiat Res. (1971) 8:189. doi: 10.1016/0022-3956(71)90018-5
66. Melrose RJ, Tinaz S, Castelo JMB, Courtney MG, Stern CE. Compromised fronto-striatal functioning in HIV: an fMRI investigation of semantic event sequencing. Behav Brain Res. (2008) 188:337–47. doi: 10.1016/j.bbr.2007.11.021
67. Fogassi L, Luppino G. Motor functions of the parietal lobe. Curr Opin Neurobiol. (2005) 15:626–31. doi: 10.1016/j.conb.2005.10.015
68. Torrey EF. Schizophrenia and the inferior parietal lobule. Schizophr Res. (2007) 97:215–25. doi: 10.1016/j.schres.2007.08.023
69. Wagner AD, Shannon BJ, Kahn I, Buckner RL. Parietal lobe contributions to episodic memory retrieval. Trends Cogn Sci. (2005) 9:445–53. doi: 10.1016/j.tics.2005.07.001
70. Yamazaki Y, Hashimoto T, Iriki A. The posterior parietal cortex and non-spatial cognition. F1000 Biol Rep. (2009) 1:74. doi: 10.3410/B1-74
71. Bassett DS, Wymbs NF, Porter MA, Mucha PJ, Carlson JM, Grafton ST. Dynamic reconfiguration of human brain networks during learning. Proc Natl Acad Sci. (2011) 108:7641–6. doi: 10.1073/pnas.1018985108
72. Barbey AK. Network neuroscience theory of human intelligence. Trends Cogn Sci. (2018) 22:8–20. doi: 10.1016/j.tics.2017.10.001
73. Antzoulatos EG, Miller EK. Increases in functional connectivity between prefrontal cortex and striatum during category learning. Neuron. (2014) 83:216–25. doi: 10.1016/j.neuron.2014.05.005
74. Bassett DS, Yang M, Wymbs NF, Grafton ST. Learning-induced autonomy of sensorimotor systems. Nat Neurosci. (2015) 18:744–51. doi: 10.1038/nn.3993
75. Duncan J, Owen AM. Common regions of the human frontal lobe recruited by diverse cognitive demands. Trends Neurosci. (2000) 23:475–83. doi: 10.1016/S.0166-2236(00)01633-7
76. Floyer-Lea A, Matthews PM. Distinguishable brain activation networks for short-and long-term motor skill learning. J Neurophysiol. (2005) 94:512–8. doi: 10.1152/jn.00717.2004
77. Honda M, Deiber M-P, Ibánez V, Pascual-Leone A, Zhuang P, Hallett M. Dynamic cortical involvement in implicit and explicit motor sequence learning. A PET study. Brain. (1998) 121:2159–73. doi: 10.1093/brain/121.11.2159
78. Braun U, Schaefer A, Walter H, Erk S, Romanczuk-Seiferth N, Haddad L, et al. Dynamic reconfiguration of frontal brain networks during executive cognition in humans. Proc Natl Acad Sci USA. (2015) 112:11678–83. doi: 10.1073/pnas.1422487112
79. Cramer SC, Sur M, Dobkin BH, O'brien C, Sanger TD, Trojanowski JQ, et al. Harnessing neuroplasticity for clinical applications. Brain. (2011) 134:1591–609. doi: 10.1093/brain/awr039
80. Mattson MP, Moehl K, Ghena N, Schmaedick M, Cheng A. Intermittent metabolic switching, neuroplasticity and brain health. Nat Rev Neurosci. (2018) 19:63. doi: 10.1038/nrn.2017.156
81. Sarrasin JB, Nenciovici L, Foisy L-MB, Allaire-Duquette G, Riopel M, Masson S. Effects of teaching the concept of neuroplasticity to induce a growth mindset on motivation, achievement, and brain activity: a meta-analysis. Trends Neurosci Educ. (2018) 12:22–31. doi: 10.1016/j.tine.2018.07.003
82. Tomassini V, Matthews PM, Thompson AJ, Fuglø D, Geurts JJ, Johansen-Berg H, et al. Neuroplasticity and functional recovery in multiple sclerosis. Nat Rev Neurol. (2012) 8:635–46. doi: 10.1038/nrneurol.2012.179
83. Barbey AK, Koenigs M, Grafman J. Dorsolateral prefrontal contributions to human working memory. Cortex. (2013) 49:1195–205. doi: 10.1016/j.cortex.2012.05.022
84. Corbetta M, Shulman GL. Control of goal-directed and stimulus-driven attention in the brain. Nat Rev Neurosci. (2002) 3:201–15. doi: 10.1038/nrn755
85. Levy R, Goldman-Rakic PS. Segregation of working memory functions within the dorsolateral prefrontal cortex. In: Schneider WX, Owen AM, and Duncan J, editors, Executive Control and the Frontal Lobe: Current Issues. Berlin: Springer (2000). p. 23–32.
86. Kinoshita M, Shinohara H, Hori O, Ozaki N, Ueda F, Nakada M, et al. Association fibers connecting the Broca center and the lateral superior frontal gyrus: a microsurgical and tractographic anatomy. J Neurosurg. (2012) 116:323–30. doi: 10.3171/2011.10.JNS11434
87. Li W, Qin W, Liu H, Fan L, Wang J, Jiang T, et al. Subregions of the human superior frontal gyrus and their connections. Neuroimage. (2013) 78:46–58. doi: 10.1016/j.neuroimage.2013.04.011
88. Leech R, Kamourieh S, Beckmann CF, Sharp DJ. Fractionating the default mode network: distinct contributions of the ventral and dorsal posterior cingulate cortex to cognitive control. J Neurosci. (2011) 31:3217–24. doi: 10.1523/JNEUROSCI.5626-10.2011
89. Chang L, Speck O, Miller EN, Braun J, Jovicich J, Koch C, et al. Neural correlates of attention and working memory deficits in HIV patients. Neurology. (2001) 57:1001–7. doi: 10.1212/WNL.57.6.1001
90. Keltner JR, Connolly CG, Vaida F, Jenkinson M, Fennema-Notestine C, Archibald S, et al. HIV distal neuropathic pain is associated with smaller ventral posterior cingulate cortex. Pain Med. (2017) 18:428–40. doi: 10.1093/pm/pnw180
91. Wang X, Foryt P, Ochs R, Chung J-H, Wu Y, Parrish T, et al. Abnormalities in resting-state functional connectivity in early human immunodeficiency virus infection. Brain Connect. (2011) 1:207–17. doi: 10.1089/brain.2011.0016
92. Wilson TW, Heinrichs-Graham E, Becker KM, Aloi J, Robertson KR, Sandkovsky U, et al. Multimodal neuroimaging evidence of alterations in cortical structure and function in HIV-infected older adults. Hum Brain Map. (2015) 36:897–910. doi: 10.1002/hbm.22674
93. McBride JA, Striker R. Imbalance in the game of T cells: what can the CD4/CD8 T-cell ratio tell us about HIV and health? PLoS Pathog. (2017) 13:e1006624. doi: 10.1371/journal.ppat.1006624
94. Sauter R, Huang R, Ledergerber B, Battegay M, Bernasconi E, Cavassini M, et al. CD4/CD8 ratio and CD8 counts predict CD4 response in HIV-1-infected drug naive and in patients on cART. Medicine. (2016) 95:e5094. doi: 10.1097/MD.0000000000005094
95. Zhuang Y, Qiu X, Wang L, Ma Q, Mapstone M, Luque A, et al. Combination antiretroviral therapy improves cognitive performance and functional connectivity in treatment-naive HIV-infected individuals. J Neurovirol. (2017) 23:704–12. doi: 10.1007/s13365-017-0553-9
96. Sanfratello L, Houck JM, Calhoun VD. Relationship between MEG global dynamic functional network connectivity measures and symptoms in schizophrenia. Schizophr Res. (2019) 209:129–34. doi: 10.1016/j.schres.2019.05.007
97. Büttner A, Mehraein P, Weis S. Vascular changes in the cerebral cortex in HIV-1 infection. Acta Neuropathol. (1996) 92:35–41. doi: 10.1007/s004010050486
98. Cardenas V, Meyerhoff D, Studholme C, Kornak J, Rothlind J, Lampiris H, et al. Evidence for ongoing brain injury in human immunodeficiency virus-positive patients treated with antiretroviral therapy. J Neurovirol. (2009) 15:324–33. doi: 10.1080/13550280902973960
99. Everall IP, Luthert PJ, Lantos PL. Neuronal number and volume alterations in the neocortex of HIV infected individuals. J Neurol Neurosurg Psychiatry. (1993) 56:481–486. doi: 10.1136/jnnp.56.5.481
100. Li R, Wang W, Wang Y, Peters S, Zhang X, Li H. Effects of early HIV infection and combination antiretroviral therapy on intrinsic brain activity: a cross-sectional resting-state fMRI study. Neuropsychiatr Dis Treat. (2019) 15:883. doi: 10.2147/NDT.S195562
101. Yu X, Gao L, Wang H, Yin Z, Fang J, Chen J, et al. Neuroanatomical changes underlying vertical HIV infection in adolescents. Front Immunol. (2019) 10:814. doi: 10.3389/fimmu.2019.00814
102. Küper M, Rabe K, Esser S, Gizewski E, Husstedt I, Maschke M, et al. Structural gray and white matter changes in patients with HIV. J Neurol. (2011) 258:1066–75. doi: 10.1007/s00415-010-5883-y
103. Crowell CS, Malee KM, Yogev R, Muller WJ. Neurologic disease in HIV-infected children and the impact of combination antiretroviral therapy. Rev Med Virol. (2014) 24:316–31. doi: 10.1002/rmv.1793
104. Saylor D, Dickens AM, Sacktor N, Haughey N, Slusher B, Pletnikov M, et al. HIV-associated neurocognitive disorder—pathogenesis and prospects for treatment. Nat Rev Neurol. (2016) 12:234. doi: 10.1038/nrneurol.2016.27
105. Toich JTF, Taylor PA, Holmes MJ, Gohel S, Cotton MF, Dobbels E, et al. Functional connectivity alterations between networks and associations with infant immune health within networks in HIV infected children on early treatment: a study at 7 years. Front Hum Neurosci. (2017) 11:635. doi: 10.3389/fnhum.2017.00635
Keywords: fMRI, temporal variability, HIV-associated neurocognitive disorders, brain resting-state functional connectivity dynamics, functional connectivity
Citation: Nguchu BA, Zhao J, Wang Y, Li Y, Wei Y, Uwisengeyimana JdD, Wang X, Qiu B and Li H (2021) Atypical Resting-State Functional Connectivity Dynamics Correlate With Early Cognitive Dysfunction in HIV Infection. Front. Neurol. 11:606592. doi: 10.3389/fneur.2020.606592
Received: 15 September 2020; Accepted: 01 December 2020;
Published: 14 January 2021.
Edited by:
Mohamed Mounir El Mendili, Icahn School of Medicine at Mount Sinai, United StatesReviewed by:
Sofia Toniolo, University of Oxford, United KingdomCopyright © 2021 Nguchu, Zhao, Wang, Li, Wei, Uwisengeyimana, Wang, Qiu and Li. This is an open-access article distributed under the terms of the Creative Commons Attribution License (CC BY). The use, distribution or reproduction in other forums is permitted, provided the original author(s) and the copyright owner(s) are credited and that the original publication in this journal is cited, in accordance with accepted academic practice. No use, distribution or reproduction is permitted which does not comply with these terms.
*Correspondence: Bensheng Qiu, YnFpdUB1c3RjLmVkdS5jbg==; Hongjun Li, bGlob25nanVuMDAxMTNAMTI2LmNvbQ==
†These authors have contributed equally to this work
Disclaimer: All claims expressed in this article are solely those of the authors and do not necessarily represent those of their affiliated organizations, or those of the publisher, the editors and the reviewers. Any product that may be evaluated in this article or claim that may be made by its manufacturer is not guaranteed or endorsed by the publisher.
Research integrity at Frontiers
Learn more about the work of our research integrity team to safeguard the quality of each article we publish.