- Department of Neurology, The First Affiliated Hospital of Wenzhou Medical University, Wenzhou, China
Objective: Explore Chinese patients' risk-benefit preferences and willingness-to-pay (WTP) for antiepileptic drugs (AEDs) treatment through the discrete choice experiment (DCE).
Method: Six attributes including the efficacy of AEDs, adverse reactions (digestive system, neuropsychic systems, and the effects on the fetus), dosing frequency and drug costs (to estimate patient WTP) were included in the DCE questionnaire based on results collected from literature reviews, expert consultation, and patient survey. The alternative-specific conditional logit model was used to analyze patient preference and WTP for each attribute and its level and to assess the sociodemographic impact and clinical characteristics.
Results: A total of 151 valid questionnaires were collected. The result shows that five out of the six attributes are significant, except the dosing frequency. Among the six attributes, the efficacy of AEDs (10.0; 95% CI 8.9–11.1) is mostly concerned by patients, followed by the effects of AEDs on the fetus (8.9; 95% CI 7.7–10.1), duration of side effects in the neuropsychic system (4.9; 95% CI 3.7–6.0) and adverse reactions of the digestive system (3.2; 95% CI 1.5–4.2). The patients surveyed are willing to spend ¥ 1,246 (95% CI, ¥ 632- ¥ 1,861) per month to ensure 100% seizure control, and ¥ 1,112 (95% CI, ¥ 586–¥ 1,658) to reduce the risk of the drug affecting the fetus to 3%. Besides, it was found that personal characteristics including the intention for conception and AEDs treatment regimens have statistical significance.
Conclusion: Improving the drug's efficacy and reducing its side effects are predominant considerations for patients with epilepsy in China, especially for those who are concerned about the seizure control and the drug effect on the fetus. This finding is useful to physicians and can encourage shared decision-making between the patients and their doctors in the clinic.
Introduction
Epilepsy is a chronic brain disorder with multiple etiologies. Its primary pathology is a recurrent, paroxysmal, and transient brain dysfunction caused by excessive discharge of cerebral neurons. The incidence rate of epilepsy was 61.44 per 100,000 person-years, and about 70 million people worldwide suffer from this disease (1–3). More than nine million people have epilepsy in China, and about two-thirds of them have active one. What's more, 400,000 new cases of epilepsy are diagnosed in China annually (4). AEDs is the cornerstone of epilepsy management and it helps to reduce the severity and seizures frequency.
Additionally, patients' treatment adherence depends on many factors, including efficacy, adverse effects, safety, ease of use, and costs, especially the adverse effects like nausea, vomiting, dizziness, tremor, skin rash, memory problems, and cognitive impairment (5–7). Furthermore, drugs' seizures and adverse effects can affect health-related quality of life (HRQOL) and patients' satisfaction (8). In practice, drug selection is mainly determined by the doctor without considering patients' opinions that mattered. Hence, doctors need to understand patients' preferences for AEDs and consider patients' opinions when making clinical practice decisions so as to improve treatment adherence, patient's life quality, and epilepsy control.
Discrete choice experiments (DCEs) are effective techniques for eliciting preferences. Hypothesis scenarios (such as scheme A and scheme B) are presented through different attributes and attribute levels, requiring participants to choose their favorite opinions and quantify each different attribute's individual impact on decision-making (9–17). DCEs have been widely used in many fields, such as marketing, transport economics, and environmental economics (18, 19). Besides, DCEs are increasingly important in health economics (20). They have been used to quantify the preference of patients or doctors for treatments on diseases like tumors (9–11), kidney disease (12), anxiety (13), and depression (13). In the field of epilepsy, DCEs are mainly used to study the choice of AEDs. For example, Lloyd et al. (14) and Manjunath et al. (15) used DCEs or conjoint analysis to discover patients' preference and willingness to pay (WTP) of AEDs or add on AEDs. Ettinger et al. (16) conducted a DCE to find the difference between neurologists and patients in choosing AED. Holmes et al. (17) combined the SANAD clinical trial data to estimate the utility of hypothetical AEDs.
However, studies assessing the patient's preference for AEDs were mainly conducted in developed countries such as the U.S. and the U.K., while few have been done in developing countries. China is a developing country with about 6 million people having active epilepsy, and most of them need long-term AEDs treatment. Therefore, revealing patients' preference for AEDs through DCEs may help improve patients' treatment adherence in developing countries so as to reduce the incidence of drug-resistant epilepsy (21–24).
Methods
Identify Attributes and Levels
The primary step in DCEs is to determine appropriate attributes and levels (25). As many factors affect the choice of AEDs, the attributes included must represent the compelling elements of the clinical scenario and facial validity. Methods for selecting attributes include literature reviews, professional recommendations, focus groups, interviews or consultation with physicians or patients, patient surveys, expert reviews, and so on (26–29). Excessive attributes may increase the cognitive burden of responders, while insufficient attributes will make it difficult to obtain convincing results. Therefore, the number of attributes should be appropriate, usually 4–9 in the field of Medicine and Health (30).
Through literature reviews (5, 14–17, 21–23, 31–34), patient survey, and expert consultation in our center, we identified six attributes: the efficacy of AEDs, adverse reactions of the digestive system, the duration of side effects in neuropsychic systems, dosing frequency, effects of AEDs on the fetus and drug cost. This paper's drug costs were used as a value attribute to explore patient trade-off in AEDs costs. The preference of males with epilepsy was also investigated for the attribute relating to AEDs' effects on the fetus. The reason is that males with epilepsy worry that AEDs may be detrimental to the fetus. As reported in the literature, the baby's risk of congenital anomalies will increase slightly if the father uses AEDs 3 months before the mother's pregnancy (35). There are six attributes, with three levels per attribute (Table 1). For this study, all attributes and levels were identified by published literature and calibrated by neurologists.
Construction of the DCE Questionnaire
The study obtained 729 (36) hypothetical scenarios by combining attributes and levels (six attributes with three levels per attribute). It is not realistic for the patient to complete all the choice sets. Therefore, we used D-optimal design (SAS 9.4, the procedure by %ChoicEff Macro) to generate the most optimal scenarios (36). The final experimental design consists of 18 choice sets (An example of a choice set is shown in Figure 1), and each respondent was required to finish 18 trade-off questions. All of them are binary choices, which means that patients must choose between drug A or drug B. In this questionnaire, the choice set that “hypothetical” AED A was superior to B′ was used to explore preferences consistency.
In addition to DCE, the paper also investigated the sociodemographic and patients' clinical characteristics. See Table 2 for details.
Patient Recruitment
Patients were consecutively recruited from the epilepsy center of the First Affiliated Hospital of Wenzhou Medical University, China. All patients were interviewed face to face with neurologists, which may help patients to understand the questionnaire.
The inclusion criteria are as follows: (1) Patients aged 18 years or older; (2) a definite diagnosis of epilepsy according to the 2017 International League Against Epilepsy (ILAE) criteria; (3) no cognitive impairment and able to understand the questionnaire; (4) willing to participate and can provide informed consent. Patients will be excluded if they (1) have incomplete questionnaire information; (2) answer the consistency check incorrectly in the questionnaire.
From 31 May 2020 to 1 August 2020, 176 patients have consented to complete the survey. Twenty-five patients were excluded from the study as they failed to answer the consistency check correctly, meaning that they did not understand the task adequately. The final sample in our study included 151 patients. The number is large enough for reliable statistical analyses according to related studies (37).
Statistical Analysis
Sociodemographic characteristics and clinical variables were evaluated by descriptive analysis.
The alternative-specific conditional logit model (also called McFadden's choice model) was used to analyze the data. Coefficients of the model indicate the importance of each attribute and the direction of choosing AEDs treatment (38–40). In the model, effect coding was adopted to represent a dummy variable to ensure that all attribute levels can be estimated (41). Then, segmented analyses were conducted to assess how preferences varied based on sociodemographic and clinical characteristics and calculated WTP for sub-groups. STATA statistical software was used to analyze the data (version 16.0).
Results
Patient Demographics and Clinical Characteristics
The demographics and clinical characteristics of the respondents were presented in Table 2. This sample consists of 151 patients: the number of female patients is 79 (52.3%). The age of the patients ranges from 19 to 68, and the average age is 32. Their average length of education time is 12 years, and most of them (96.0%) have a secondary education degree or above. 20.5% of them have conception intention. A relatively large portion of patients have focal epilepsy (56.3%), but most of them have no recent seizures (42.4%). The percentage of the patients who receive monotherapy, two AEDs, and more than two AEDs are 51.0, 38.4, and 10.6%, respectively.
Patients Preference for the Treatment of AEDs
The preference weights of the alternative-specific conditional logit model results are shown in Table 3 and Figure 2. In the model, the attribute of drug costs was used as continuous variables, and the other five as categorical variables. The results show that the coefficients are significant (p < 0.05) for five out of the six attributes, except the dosing frequency. That means patients' treatment preferences are not influenced by dosing frequency. Table 3 illustrates that patients prefer better seizure control and fewer side effects of drugs. What's more, they have the strongest desire for achieving 100% seizure control (coefficient = 0.778, p = 0.000) and lower effects (3%) on the fetus (coefficient = 0.700, p = 0.000). In addition, they prefer to having no adverse reactions (0%) in neuropsychic systems (coefficient = 0.378, p = 0.000) and in digestive system (coefficient = 0.247, p = 0.000). The coefficient that is <0 means that respondents have little preference for this attribute. Figure 2 shows that the mean relative importance is 95% CI, and the efficacy of AEDs is the most preferred treatment attribute (10.0; 95% CI 8.9–11.1). Apart from the efficacy of AEDs, patients rank the remaining treatment attributes as follows: effects of AEDs on the fetus (8.9; 95% CI 7.7–10.1), duration of side effects in the neuropsychic system (4.9; 95% CI 3.7–6.0), and adverse reactions of the digestive system (3.2; 95% CI 1.5–4.2).
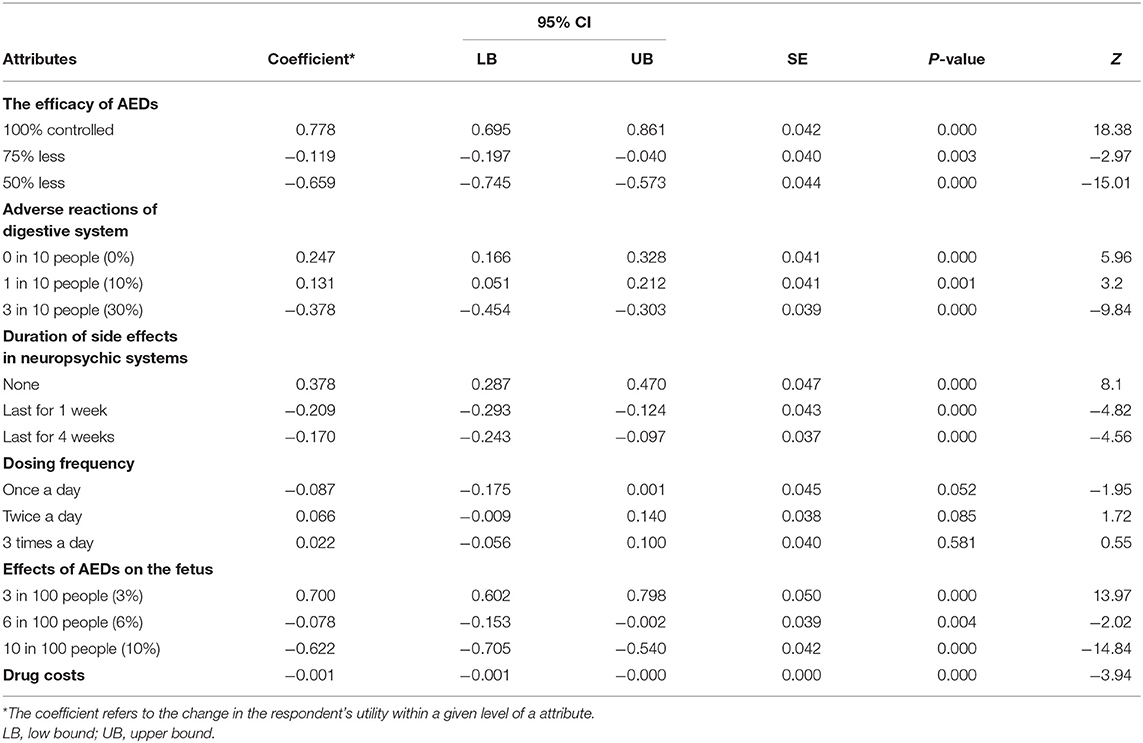
Table 3. The patients' preference for treatment of AEDs: the main effects of alternative-specific conditional logit model results (N = 151).
The Impact of Fertility Preparation on Treatment Preferences
After analyzing the interaction terms between patients' sociodemographic or clinical characteristics and their preferences for different attributes, the authors found that the personal attribute of pregnancy intentions is significant (P < 0.05). As the preference-weight shown in Figure 3, patients who have pregnancy intention have a significantly high coefficient (coefficient = 1.591, 95% CI 1.092–2.090, p = 0.000) in lower effects (3%) on the fetus, which is more than the double of that of the patients who are not (coefficient = 0.571, 95% CI 0.467–0.675, p = 0.000). This result indicates that patients with pregnancy intentions worry more about the effect of AEDs on the fetus than the efficacy of AEDs. Besides, there is little difference between patients who are trying for babies and patients who are not in the coefficients of the other four attributes.
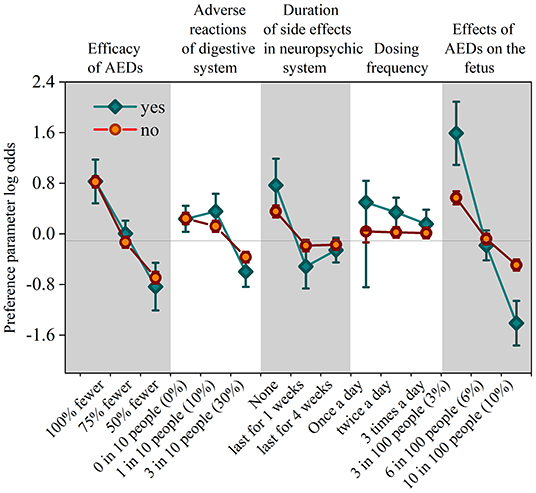
Figure 3. The coefficient of personal attribute in the intention for having babies. Vertical bars denote 95% confidence intervals.
To Estimate the Willingness-to-Pay (WTP)
Physicians can evaluate the economic value of the treatment attributes by analyzing the attribute of drug costs. The comparison of each attribute is demonstrated in the economic value-form, which is easier for doctors to understand.
Table 4 presents the patients' trade-off of drug costs for the treatment of AEDs in each attribute. Among all respondents' WTP, patients are willing to spend ¥ 1,246 (95% CI, ¥ 632–¥ 1,861) per month to ensure 100% seizure control and ¥ 1,112 (95% CI, ¥ 586–¥ 1,658) to reduce the risk of drug effects on the fetus to 3%. The value for no side effects in neuropsychic systems is ¥ 606 (95% CI, ¥ 316–¥ 896) and for no adverse reactions in the digestive system is ¥ 396 (95% CI, ¥ 152–¥ 640).
Then the authors identified the number of AEDs that the respondents were using. As shown in Table 4, the WTP of respondents in various attributes decreases as the number of AED patients increases. For example, patients who are treated with monotherapy are willing to pay ¥ 2,128 to achieve 100% seizure control. However, those who use three or more AEDs are willing to pay ¥ 346 for the same treatment. In general, the result shows that the patients have stronger negative values as the efficacy deteriorates and the risk of side effects increases.
Discussion
This paper is the first study that uses discrete choice experiments to quantify patients' benefit-risk preferences and their trade-off to treat AEDs in China. It is also the first study to investigate the preference of males with epilepsy for the effect of AEDs on the fetus. The research shows that improving drug efficacy or better controlling seizures and reducing the risk of adverse reactions are the predominant considerations for the patients when choosing their treatments. Among all six attributes observed in the paper, the attribute of AEDs efficacy is the most important one for patients to select the treatment, and that is followed by the effects of AEDs on the fetus. Besides, the digestive system's adverse reaction and the duration of side effects in neuropsychic systems have statistical significance. This result is similar to the earlier literature. Ettinger et al. (16) used DCE in patients and physicians and found that both of them consider seizure control as the most critical factor. Manjunath et al. (15) investigated patient preference for add-on AEDs, and they found that the reduction of seizure frequency was concerned more than that of adverse effects. Unexpectedly, dosing frequency does not significantly influence patients' treatment preferences, which indicates that patients may rely on their current treatment options. Many patients have epilepsy for the average course of more than 10 years, and they need to take AEDs at least twice a day. Therefore, the increase of dosing frequency may have little impact on their quality of life. Besides, the patients' WTP is strongest when it comes to being free from seizure, and the WTP degree is followed when it comes to reducing the risk of fetal abnormality to 3%. This proves the value of improving drug efficacy and reducing the risk of side effects.
Furthermore, the study investigates the impact of personal conditions on treatment preferences. It is found that pregnancy intentions and AEDs treatment regimens significantly affect the patients' preference degree.
Firstly, epilepsy patients preparing for pregnancy have a strong positive preference for reducing the risk of AEDs to the fetus. This finding is consistent with the study of Holmes et al. (17), which investigated the women with productive potential but with generalized or unclassified epilepsy. According to the time of treatment failure in the SNADA study (42), AED is ranked as valproate > topiramate > lamotrigine for generalized or unclassified epilepsy patients. But combined with data and clinical evidence of patient preference, the sequence is reversed, i.e., lamotrigine > topiramate > valproate. Therefore, the treatment preference of patients will vary with individual attributes. For example, in this study, epilepsy patients who have pregnant intentions are more concerned about the effects of drugs on the fetus than about the control of epilepsy.
Secondly, there is no difference between males and females in terms of the preference for AEDs, which means that male patients are also concerned about the effect of drugs on the fetus. As there was no enough evidence to confirm that AEDs taken by male patients who are about to father a baby can cause fetal malformation (35), it's worthwhile to study whether hormonal problems can negatively impact patients' fertility in future work.
Thirdly, patients' WTP decreases significantly as the number of AEDs that they need to take increases. For example, the WTP percentages of patients who use three or more AEDs take in monotherapy patients regarding three factors: acquiring 100% seizure control, no side effects in the digestive system, and no adverse reaction in neuropsychic systems, are 1/6, 1/3, 1/13, respectively.
The first possible reason is that the patients who take three or more drugs are more likely to be drug-resistant. Their relatively large total defined daily dose (DDD) can lead to a heavy economic burden on drugs. Therefore, with the same income, patients taking three or more AEDs have lower WTP on controlling seizures and reducing adverse reactions than those who choose monotherapy. According to the literature reported by Hong et al. (33), the overall average annual spending on epilepsy treatment accounts for more than half of patients' mean yearly income, and the economic burden of patients with drug-resistant epilepsy is approximately eight times that of seizure-free patients. We know the main goal of the AEDs treatment is the total seizure control. However, due to the reduction of WTP in patients with drug-resistant epilepsy, the adverse effects of AEDs may negatively impact the patients' quality of life more than the seizure frequency.
The second possible reason is that monotherapy can help many patients achieve seizure-free after. Some literature show that about 47% of newly diagnosed patients can become seizure-free after the first use of AED, while 13% and 1% of them can be seizure-free after the second and third single drug treatment (43, 44). It can be seen that some patients with monotherapy may have ideal epilepsy control, and they may be willing to spend more money on the following treatments. This result is similar to that of Lloyd et al. (14), which showed that participants who experienced seizures every week have a WTP for £27 per month to achieve 100% seizure control, £38 and £89 for monthly and yearly seizures, respectively. The data means that patients with lower seizure frequency are more likely to pay more to improve seizure control. For drug-resistant epilepsy or refractory epilepsy patient, physicians should pay more attention to their economic burden of drugs in the clinic.
This study shows that DCE is an effective and accurate method for acquiring patient preference for AEDs treatment. It can quantify different treatment attributes and their levels, providing useful information to help researchers understand their importance. Epilepsy is a chronic disease that needs individualized treatment. Understanding the patient treatment preference through DCE and taking it into account when making decisions can facilitate decision sharing between doctors and patients, which in turn can improve HRQOL and therapeutic compliance.
Some limitations should also be highlighted. First, as it was infeasible to include all the treatment attributes in the choice set, this study only selected the six most representative indicators. Therefore, these attributes may not accurately reflect their displayed preferences. For example, major side effect of AEDs such as sedation, cognitive deficits, overweight/obesity, and cardiovascular risks were not listed as a separate attribute. Second, in the choice set of DCEs, patients were required to choose from a pair of hypothetical AEDs, but the choice of real AEDs may reveal different findings. Third, the patients were all recruited from the epilepsy center of tertiary hospitals in China, while those from primary and secondary hospitals are not considered.
Data Availability Statement
The raw data supporting the conclusions of this article will be made available by the authors, without undue reservation.
Ethics Statement
The studies involving human participants were reviewed and approved by the Human Research Ethics committee of the First Affiliated Hospital of Wenzhou Medical University. The patients/participants provided their written informed consent to participate in this study.
Author Contributions
Material preparation, data collection, and analysis were performed by YH, XL, JG, JL, XW, ZZ, and HX. The first draft of the manuscript was written by YH and ZZ. All authors commented on previous versions of the manuscript, contributed to the study conception and design, and read and approved the final manuscript.
Funding
This study was supported by Medical Health Science and Technology Project of Zhejiang Provincial Health Commission (Grant Number: 2020KY180) and Wenzhou Science and Technology Plan Project (Grant Number: Y20190096).
Conflict of Interest
The authors declare that the research was conducted in the absence of any commercial or financial relationships that could be construed as a potential conflict of interest.
Acknowledgments
We thank all participating patients.
Abbreviations
WTP, willingness-to-pay; AEDs, antiepileptic drugs; DCEs, discrete choice experiments; ADHD, attention-deficit/hyperactivity disorder; LB, low bound; UB, upper bound.
References
1. Fiest KM, Sauro KM, Wiebe S, Patten SB, Nathalie J. Prevalence and incidence of epilepsy: a systematic review and meta-analysis of international studies. Neurology. (2017) 88:296–303. doi: 10.1212/WNL.0000000000003509
2. Ngugi AK, Kariuki SM, Bottomley C, Kleinschmidt I, Sander JW, Newton CR. Incidence of epilepsy: a systematic review and meta-analysis. Neurology. (2011) 77:1005–12. doi: 10.1212/WNL.0b013e31822cfc90
3. Fisher RS, Acevedo C, Arzimanoglou A, Bogacz A, Cross JH, Elger CE, et al. ILAE official report: a practical clinical definition of epilepsy. Epilepsia. (2014) 55:475–82. doi: 10.1111/epi.12550
4. Song P, Liu Y, Yu X, Wu J, Poon AN, Demaio A, et al. Prevalence of epilepsy in China between 1990 and 2015: a systematic review and meta-analysis. J Glob Health. (2017) 7:020706. doi: 10.7189/jogh.07.020706
5. Gaitatzis A, Sander JW. The long-term safety of antiepileptic drugs. CNS Drugs. (2013) 27:435–55. doi: 10.1007/s40263-013-0063-0
6. Hirsch LJ, Arif H, Nahm EA, Buchsbaum R, Resor SR Jr, Bazil CW. Cross-sensitivity of skin rashes with antiepileptic drug use. Neurology. (2008) 71:1527–34. doi: 10.1212/01.wnl.0000334295.50403.4c
7. Witt JA, Elger CE, Helmstaedter C. Adverse cognitive effects of antiepileptic pharmacotherapy: each additional drug matters. Eur Neuropsychopharmacol. (2015) 25:1954−9. doi: 10.1016/j.euroneuro.2015.07.027
8. Santos AMC, Castro-Lima H, Matos MAA, Brito MB. Quality of life among women with epilepsy during their reproductive years. Epilepsy Behav. (2018) 85:10–3. doi: 10.1016/j.yebeh.2018.04.028
9. Hauber AB, Arellano J, Qian Y, González JM, Posner JD, Mohamed AF, et al. Patient preferences for treatments to delay bone metastases. Prostate. (2014) 74:1488–97. doi: 10.1002/pros.22865
10. Sun H, Wang H, Shi L, Wang M, Li J, Shi J, et al. Physician preferences for chemotherapy in the treatment of non-small cell lung cancer in China: evidence from multicentre discrete choice experiments. BMJ Open. (2020) 10:e032336. doi: 10.1136/bmjopen-2019-032336
11. Smith ML, White CB, Railey E, Sledge GW. Examining and predicting drug preferences of patients with metastatic breast cancer: using conjoint analysis to examine attributes of paclitaxel and capecitabine. Breast Cancer Res Treat. (2014) 145:83–9. doi: 10.1007/s10549-014-2909-7
12. Clark MD, Szczepura A, Gumber A, Howard K, Moro D, Morton RL. Measuring trade-offs in nephrology: a systematic review of discrete choice experiments and conjoint analysis studies. Nephrol Dial Transplant. (2018) 33:348–355. doi: 10.1093/ndt/gfw436
13. Tünneßen M, Hiligsmann M, Stock S, Vennedey V. Patients' preferences for the treatment of anxiety and depressive disorders: a systematic review of discrete choice experiments. J Med Econ. (2020) 23:546–56. doi: 10.1080/13696998.2020.1725022
14. Lloyd A, McIntosh E, Price M. The importance of drug adverse effects compared with seizure control for people with epilepsy: a discrete choice experiment. Pharmacoeconomics. (2005) 23:1167–81. doi: 10.2165/00019053-200523110-00008
15. Manjunath R, Yang JC, Ettinger AB. Patients' preferences for treatment outcomes of add-on antiepileptic drugs: a conjoint analysis. Epilepsy Behav. (2012) 24:474–9. doi: 10.1016/j.yebeh.2012.05.020
16. Ettinger AB, Carter JA, Rajagopalan K. Patient versus neurologist preferences: a discrete choice experiment for antiepileptic drug therapies. Epilepsy Behav. (2018) 80:247253. doi: 10.1016/j.yebeh.2018.01.025
17. Holmes EAF, Plumpton C, Baker GA, Jacoby A, Ring A, Williamson P, et al. Patient-focused drug development methods for benefit-risk assessments: a case study using a discrete choice experiment for antiepileptic drugs. Clin Pharmacol Ther. (2019) 105:672–83. doi: 10.1002/cpt.1231
18. Louviere JJ, Woodworth G. Design and analysis of simulated consumer choice or allocation experiments: an approach based on aggregate data. J Market Res. (1983) 20:350–67. doi: 10.1177/002224378302000403
19. Adamowicz WL, Louviere JJ, Williams M. Combining revealed and stated preference methods for valuing environmental amenities. J Environ Econ Manag. (1992) 26:271–92. doi: 10.1006/jeem.1994.1017
20. Clark MD, Determann D, Petrou S, Moro D, de Bekker-Grob EW. Discrete choice experiments in health economics: a review of the literature. Pharmacoeconomics. (2014) 32:883–902. doi: 10.1007/s40273-014-0170-x
21. Malek N, Heath CA, Greene J. A review of medication adherence in people with epilepsy. Acta Neurol Scand. (2017) 135:507–15. doi: 10.1111/ane.12703
22. Al-Aqeel S, Gershuni O, Al-Sabhan J, Hiligsmann M. Strategies for improving adherence to antiepileptic drug treatment in people with epilepsy. Cochrane Database Syst Rev. (2017) 2:CD008312. doi: 10.1002/14651858.CD008312.pub3
23. O'Rourke G, O'Brien JJ. Identifying the barriers to antiepileptic drug adherence among adults with epilepsy. Seizure. (2017) 45:160–8. doi: 10.1016/j.seizure.2016.12.006
24. Dalic L, Cook MJ Managing drug-resistant epilepsy: challenges and solutions. Neuropsychiatr Dis Treat. (2016) 12:2605–16. doi: 10.2147/NDT.S84852
25. Gallego G, Dew A, Bulkeley K, Veitch C, Lincoln M, Bundy A, et al. Factors affecting retention of allied health professionals working with people with disability in rural New South Wales, Australia: discrete choice experiment questionnaire development. Hum Resour Health. (2015) 13:22. doi: 10.1186/s12960-015-0013-7
26. Hall J, Kenny P, King M, Louviere J, Viney R, Yeoh A. Using stated preference discrete choice modelling to evaluate the introduction of varicella vaccination. Health Econ. (2002) 11:457–65. doi: 10.1002/hec.694
27. Phillips KA, Maddala T, Johnson FR. Measuring preferences for health care interventions using conjoint analysis: an application to HIV testing. Health Serv Res. (2002) 37:1681–705. doi: 10.1111/1475-6773.01115
28. Hundley V, Ryan M, Graham W. Assessing women's preferences for intrapartum care. Birth. (2001) 28:254–63. doi: 10.1046/j.1523-536X.2001.00254.x
29. Coast J, Horrocks S. Developing attributes and levels for discrete choice experiments using qualitative methods. J Health Serv Res Policy. (2007) 12:25–30. doi: 10.1258/135581907779497602
30. Soekhai V, de Bekker-Grob EW, Ellis AR, Vass CM. Discrete choice experiments in health economics: past, present and future. Pharmacoeconomics. (2019) 37:201–26. doi: 10.1007/s40273-018-0734-2
31. Hernández-Díaz S, Smith CR, Shen A, Mittendorf R, Hauser WA, Yerby M, et al. Comparative safety of antiepileptic drugs during pregnancy. Neurology. (2012) 78:1692–9. doi: 10.1212/WNL.0b013e3182574f39
32. Tomson T, Battino D, Bonizzoni E, Craig J, Lindhout D, Perucca E, et al. Comparative risk of major congenital malformations with eight different antiepileptic drugs: a prospective cohort study of the EURAP registry. Lancet Neurol. (2018) 17:530–8. doi: 10.1016/S1474-4422(18)30107-8
33. Hong Z, Qu B, Wu XT, Yang TH, Zhou D. Economic burden of epilepsy in a developing country: a retrospective cost analysis in China. Epilepsia. (2009) 50:2192–8. doi: 10.1111/j.1528-1167.2009.02185.x
34. Sirsi D, Safdieh J E. The safety of levetiracetam. Expert Opin Drug Safety. (2007) 6:241–50. doi: 10.1517/14740338.6.3.241
35. Yang F, Yuan W, Liang H, Song X, Yu Y, Gelaye B, et al. Preconceptional paternal antiepileptic drugs use and risk of congenital anomalies in offspring: a nationwide cohort study. Eur J Epidemiol. (2019) 34:651–60. doi: 10.1007/s10654-019-00509-2
36. World Health Organization. How to Conduct a Discrete Choice Experiment for Health Workforce Recruitment and Retention in Remote and Rural Areas: A User Guide With Case Studies. Geneva: World Health Organization (2012).
37. de Bekker-Grob EW, Donkers B, Jonker MF, Stolk EA. Sample size requirements for discrete-choice experiments in healthcare: a practical guide. Patient. (2015) 8:373–84. doi: 10.1007/s40271-015-0118-z
40. McFadden D. Conditional logit analysis of qualitative choice behavior. In: Zarembka P, editor. Frontiers in Econometrics. New York, NY: Academic Press (1974). p.105–42.
41. Bech M, Gyrd-Hansen D. Effects coding in discrete choice experiments. Health Econ. (2005) 14:1079–83. doi: 10.1002/hec.984
42. Marson AG, Al-Kharusi AM, Alwaidh M, Appleton R, Baker GA, Chadwick DW, et al. The SANAD study of effectiveness of valproate, lamotrigine, or topiramate for generalized and unclassifiable epilepsy: an unblinded randomized controlled trial. Lancet. (2007) 369:1016–26. doi: 10.1016/S0140-6736(07)60461-9
43. National Institute for Health and Clinical Excellence. The Epilepsies: The Diagnosis and Management of the Epilepsies in Adults and Children in Primary and Secondary Care. Clinical Guideline 137, United Kingdom (2012). Available online at: http://www.nice.org.uk/Guidance/cg137
Keywords: antiepileptic drugs, discrete choice experiment, preference, epilepsy, willingness to pay (WTP)
Citation: Hua Y, Zhu Z, Li X, Gong J, Ding S, Lin J, Wang X, Du Y, Xia N, Zheng R and Xu H (2020) Patient Preference for Antiepileptic Drugs Treatment in China: Evidence From the Discrete Choice Experiment. Front. Neurol. 11:602481. doi: 10.3389/fneur.2020.602481
Received: 03 September 2020; Accepted: 11 November 2020;
Published: 03 December 2020.
Edited by:
Fernando Cendes, Campinas State University, BrazilReviewed by:
Christian Brandt, Mara Hospital, GermanyVeriano Alexandre, University of São Paulo, Brazil
Copyright © 2020 Hua, Zhu, Li, Gong, Ding, Lin, Wang, Du, Xia, Zheng and Xu. This is an open-access article distributed under the terms of the Creative Commons Attribution License (CC BY). The use, distribution or reproduction in other forums is permitted, provided the original author(s) and the copyright owner(s) are credited and that the original publication in this journal is cited, in accordance with accepted academic practice. No use, distribution or reproduction is permitted which does not comply with these terms.
*Correspondence: Huiqin Xu, xuhuiqin@wmu.edu.cn
†These authors have contributed equally to this work and share first authorship