- Department of Neurology, Laboratory of Neurodegenerative Disorders, Rare Diseases Center, West China Hospital, Sichuan University, Chengdu, China
Purpose: Falls are common and are frequently accompanied by injuries in patients with spinocerebellar ataxias type 3 (SCA3). We explored which factors could predict falls in a cohort of patients with SCA3 and developed a nomogram model to predict the first fall in non-fallen patients with SCA3.
Method: We conducted a prospective cohort study. Forty-four non-fallen patients with SCA3 were followed up until the first fall or November 5, 2020, whichever came first. Univariate and multivariate Cox proportional hazard regression analyses were applied to explore the predictive factors of falls in patients with SCA3. A nomogram model predicting the no-fall probabilities at 3, 6, 12, and 24 months was formulated based on the results of the multivariate Cox analysis. Internal validation was conducted to assess the discrimination and calibration of the final model using bootstrapping with 1,000 resamples.
Results: Multivariate Cox proportional hazard regression showed that the presence of dystonia, hyperreflexia, urinary incontinence, and hidrosis and the number of abnormal eye movements predicted a more rapid progression to falls in patients with SCA3. The nomogram model showed good discrimination with a concordance index of 0.83 and good calibration.
Conclusion: Patients with dystonia, hyperreflexia, urinary incontinence, and hidrosis, and more types of abnormal eye movement had a more rapid progression to falls in SCA3.
Introduction
Spinocerebellar ataxias (SCAs) are a genetically heterogeneous group of autosomal dominantly inherited progressive disorders characterized neuropathologically by degeneration of the cerebellum and clinically by loss of balance and coordination accompanied by slurred speech. Spinocerebellar ataxias type 3 (SCA3), also known as Machado-Joseph disease, is the most common SCA worldwide and is caused by CAG expansion of the ATXN3 gene. In China, SCA3 is also the most common subtype of SCA, accounting for 48–49% of patients with SCA (1).
Falls are frequent in patients with SCA (2). In a study including 42 patients with autosomal dominant cerebellar ataxias or idiopathic late-onset cerebellar ataxias, up to 93% of patients reported at least one fall in the 12 preceding months. Another study observed that 84.1% of patients experienced at least one fall during a period of 12 months in a cohort of patients with SCA1, SCA2, SCA3, or SCA6 with a mean disease duration of 11.8 ± 6.4 years (3). In addition, falls are frequently accompanied by serious injuries. Eighty-five percent of the fallers reported injuries, and 31% of the fallers suffered a fracture or joint dislocation among patients with degenerative cerebellar ataxias (4). Falls may also induce a fear of falling and can cause a vicious cycle of immobilization.
According to a previous study, falls can occur as early as 2 years after disease onset in SCA (4). A previous retrospective study explored the factors associated with falls in patients with SCA1, SCA2, SCA3, and SCA6 and determined that disease duration, severity of ataxia, presence of pyramidal symptoms, total number of non-ataxia symptoms, and genotype SCA3 were associated with a higher fall frequency (2). However, no prospective study has been conducted as yet to explore the risk factors that could predict the first fall in non-fallen patients with SCA3. Nomograms, visible, and effective tools for multivariate survival analysis, have been widely used in cancer and other diseases (5). To date, no nomogram has been applied to predict falls in patients with SCA3. Therefore, in the current study, we aimed to investigate which factors could predict falls in a cohort of patients with SCA3 and to develop a nomogram model to predict the first fall in non-fallen patients with SCA3.
Materials and Methods
Patients Evaluation
This study was executed in agreement with the Ethics Committee of West China Hospital of Sichuan University. All recruited participants provided written informed consent. A total of forty-four genetically confirmed non-fallen patients with SCA3 (16 males) were consecutively recruited from the Department of Neurology, West China Hospital of Sichuan University, between August 2015 and January 2020.
We performed a prospective study in the current study. All patients received a genetically confirmed diagnosis of SCA3 after testing for trinucleotide repeat expansions of genes causing SCA1, SCA2, SCA3, SCA6, and SCA7 using short tandem repeat (STR) analysis. The CAG repeat lengths of the expanded allele were collected. All patients underwent a face-to-face interview at baseline. Sex, age, weight, height, educational years, age at onset, and disease duration of these patients were collected. The body mass index (BMI) was calculated by body weight (kg) divided by height squared (m2). The Montreal Cognitive Assessment (MoCA) was used to assess global cognitive function (6). Depression was screened using the Hamilton Depression Rating Scale-24 (HDRS-24) (7). Anxiety was screened using the Hamilton Anxiety Rating Scale (HARS) (8). Excessive daytime sleepiness was screened using the Epworth Sleepiness Scale (ESS) (9). Sleep problems were evaluated using the Pittsburgh sleep quality index (PSQI) (10).
Ataxia severity was assessed using the Scale for the Assessment and Rating of Ataxia (SARA), which is composed of eight items—gait, stance, sitting, speech disturbance, finger chase, nose-finger test, fast alternating hand movements, and heel-shin slide—and has been proven to be a reliable and valid clinical scale measuring the severity of ataxia (11). Non-ataxia symptoms, including bradykinesia, spasticity, paresis, muscle atrophy, fasciculation, myoclonus, rigidity, chorea/dyskinesia, dystonia, resting tremor, action tremor, sensory symptoms, dysphagia, hyperreflexia, urinary incontinence, constipation, hidrosis, orthostatic hypotension, diplopia, vision loss, and eye movement abnormalities, were evaluated by neurologists who were experienced in movement disorders. Eye movement abnormalities, including impaired smooth pursuit, increased square-wave jerks (SWJ), gaze-evoked nystagmus (GEN), slowing of saccades, saccadic hypo/hypermetria, and supranuclear gaze palsy, were evaluated using accepted bedside techniques (12). Impaired smooth pursuit, GEN, slowing of saccades, and saccadic hypo/hypermetria were evaluated following the International Cooperative Ataxia Rating Scale (ICARS) procedure (13). SWJ was detected during central fixation, and increased SWJ was defined as SWJ ≥ 10 per minute (14). Pursuit, saccade speed, saccade accuracy, supranuclear gaze palsy, and GEN were evaluated in both the horizontal and vertical planes.,
The 44 patients with SCA3 were followed up with telephone or face-to-face interviews with an irregular interval. November 5, 2020, was the last follow-up time. The endpoint was set as the first fall or November 5, 2020, whichever came first.
Statistical Analysis
Since no continuous variables were normally distributed, they were presented as the mediums (ranges), and all categorical variables were presented as numbers and percentages. Survival analysis was conducted to explore which factors could predict falls as early biomarkers. The fall-free time from enrollment was calculated from the date of enrollment to the first fall for fallers and from the date of enrollment to the date of last follow-up, namely, November 5, 2020, for non-fallers. We used the time from enrollment as the timescale. Thirty-two variables were evaluated for their predictive role for falls using univariate Cox proportional hazard regression models, including age, sex, educational year, disease duration, CAG repeat numbers, BMI, SARA score, MoCA score, HARS score, HDRS-24 score, PSQI score, ESS score, the number of abnormal eye movement, vision loss, bradykinesia, spasticity, paresis, muscle atrophy, fasciculation, rigidity, chorea/dyskinesia, dystonia, resting tremor, action tremor, sensory symptoms, dysphagia, diplopia, orthostatic hypotension, hyperreflexia, urinary incontinence, constipation, and hidrosis. The p-values were false discovery rate (FDR)-corrected for multiple testing to avoid false positive significances. Then, candidates with a FDR-corrected p < 0.20 were entered into the multivariate Cox proportional hazard regression model. In addition, given that age, disease duration, CAG repeat numbers, and abnormal eye movement have been reported to be prognostic factors for the survival of SCA3 patients (15), they were always included in the multivariate Cox proportional hazard regression model regardless of the p value obtained in univariate analysis. Finally, a nomogram model predicting the no-fall probabilities at 3, 6, 12, and 24 months was formulated based on the results of the multivariate Cox analysis. Internal validation was conducted to assess the discrimination and calibration of the nomogram model using bootstrapping with 1,000 resamples. Discrimination was measured with the concordance index (c-index) and the time-dependent receiver operating characteristic curve (t-ROC); the larger the c-index or the areas under the ROC curve (AUC) was, the more accurate the prognostic prediction was. Calibration curves were plotted to assess the calibration of the nomogram. All analyses were performed using the Statistical Package for the Social Sciences (SPSS) version 22.0 and R version 4.0.2. Two-tailed p values of <0.05 were considered statistically significant.
Results
All forty-four patients were followed up until the first fall or November 5, 2020, whichever came first (Table 1). The mean follow-up time was 16.23 ± 12.35 months. The Kaplan-Meier graph is shown in Figure 1. In the univariate Cox proportional hazard regression models applied, four variables were identified to have a FDR-corrected p < 0.20, including dystonia (p = 0.168), hyperreflexia (p = 0.168), urinary incontinence (p < 0.032), and hidrosis (p = 0.112) (Table 2). Combined with age, disease duration, CAG repeat numbers, SARA score, and the number of abnormal eye movement, nine variables were ultimately included in the multivariate Cox proportional hazard regression model. The multivariate Cox proportional hazard regression showed that the number of abnormal eye movements (HR = 1.703, 95% CI: 1.133–2.560, p = 0.011), dystonia (HR = 34.340, 95% CI: 2.514–469.096, p = 0.008), hyperreflexia (HR = 3.051, 95% CI: 1.125–8.274, p = 0.028), urinary incontinence (HR = 14.225, 95% CI: 3.782–53.501, p < 0.001), and hidrosis (HR = 4.006, 95% CI: 1.498–10.711, p = 0.006) predicted a more rapid progression to falls in SCA3 patients (Table 3 and Figure 2).
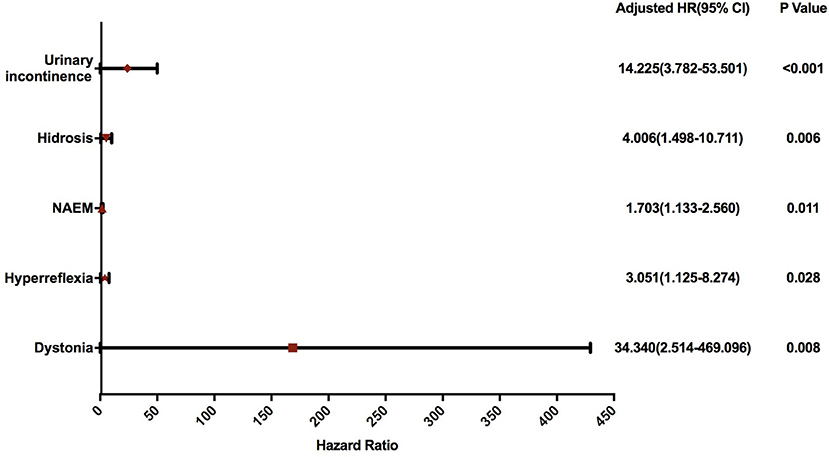
Figure 2. Forest plot of the hazard ratio for fall indicators analyzed using a multivariate Cox proportional hazard regression model. SCA3, spinocerebellar ataxia 3; HR, hazard ratio; NAEM, number of abnormal eye movements.
The presence of dystonia, hyperreflexia, urinary incontinence, and hidrosis and the number of abnormal eye movements were used to build the nomogram model according to the results of the multivariate Cox proportional hazard regression analysis (Figure 3). The c-index of the nomogram model was 0.83. In t-ROC analysis, the AUC for predicting 3, 6, 12, and 24 months no-fall probability were 0.919, 0.919, 0.971, and 0.841, respectively (Supplementary Figure 1). The calibration curves of the nomogram for the prediction of no-fall probability at 3, 6, 12, and 24 months showed good calibration (Supplementary Figure 2).
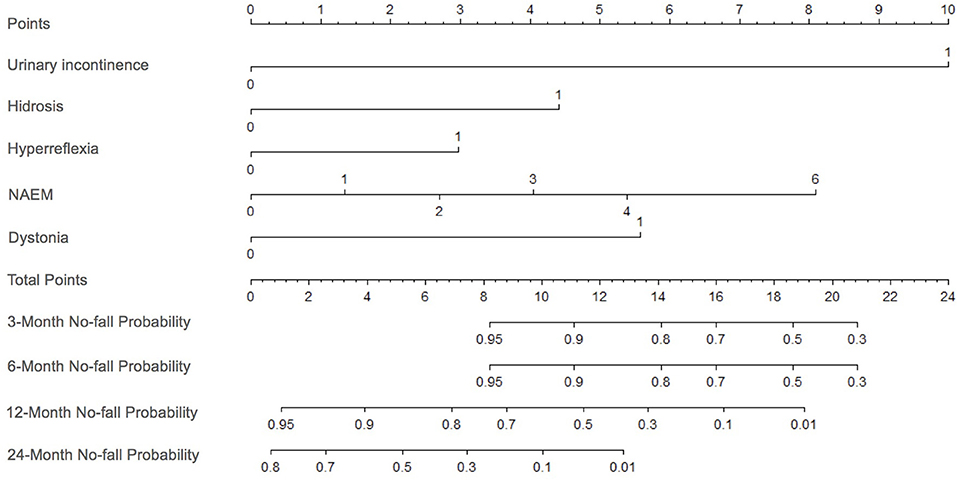
Figure 3. Nomogram plot to predict no-fall probability in patients with SCA3. The no-fall probability is calculated by drawing a line to the point on the axias for each of the following variables: dystonia, hyperreflexia, urinary incontinence, hidrosis, and the number of abnormal eye movements. The points for each variable are summed and located on the total points line. Then, a vertical line is projected from the total point line to the predicted probability bottom scales to obtain the individual no-fall probabilities. SCA3, spinocerebellar ataxia 3; NAEM, number of abnormal eye movements.
Discussion
Fall frequency has been reported to be associated with disease duration, ataxia severity, presence of pyramidal symptoms, total number of non-ataxia symptoms (including hyperreflexia, areflexia, extensor plantar reflex, spasticity, paresis, muscle atrophy, fasciculation, myoclonus, rigidity, chorea/dyskinesia, dystonia, resting tremor, sensory symptoms, urinary dysfunction, cognitive dysfunction, and brainstem oculomotor signs), and the genotype of SCA3 in patients with SCA by correlation analysis (2, 3). The current study established the first nomogram model to predict falls in patients with SCA3. This model showed good discrimination and calibration. In this predictive model, dystonia, hyperreflexia, urinary incontinence, hidrosis, and abnormal eye movements were indicators for falls in patients with SCA3.
Dystonia is one of the most common extrapyramidal manifestations in SCA3. Previous studies have reported a prevalence of dystonia between 3.7 and 33.3% in SCA3 (16, 17). Dystonia and ataxia may share some overlapping pathologies, such as synaptic transmission and neurodevelopment (18). A study found that the presence of dystonia correlated with greater severity of ataxia in SCA3 (19). Another study revealed the predictive value of dystonia on survival in SCA3, which was consistent with our findings (20).
Hyperreflexia is present in 66.7%−78.3% of patients with SCA3 (21, 22). A previous study found that hyperreflexia was associated with longer CAG repeat lengths in SCA3 (23). Another study found that the pyramidal signs could predict a more rapid progression to death using the univariate Cox analysis (20). Our study confirmed the predictive role of hyperreflexia in disease progression.
Autonomic dysfunction is not rare in patients with SCA3 (24). A study reported a urinary incontinence prevalence of 39.3%, an orthostatic hypotension prevalence of 35.7%, and a hidrosis prevalence of 39.3% in patients with SCA3, which were significantly higher than those in healthy controls (25). A study revealed the involvement of Onuf's nucleus in SCA3 using morphometric and immunohistochemical methods, which may account for the autonomic dysfunction in SCA3 (26). However, the relationship between autonomic dysfunction and disease progression in SCA3 has never been studied before. Our study revealed the predictive role of autonomic dysfunction on falls in patients with SCA3 for the first time, emphasizing the importance of autonomic dysfunction screening when handling with patients with SCA3.
Abnormal eye movements are common in SCA3 (27). Several cross-sectional studies have shown the correlation between abnormal eye movements and disease severity in patients with SCA3 (28–30). The brain structures involved in the occurrence of abnormal eye movements are wide, and the neural network involved is complex (31). The flocculonodular lobe (32), the dorsal oculomotor vermis (OMV) (lobule VII and a part of folium VIc), and the caudal fastigial nuclei (CFN) are crucial in controlling smooth pursuit (33–35). The cerebellum plays a role in fixation stability, impairment of which would lead to increased SWJ (36). The brain structures responsible for normal saccades comprise the paramedian pontine reticular formation (PPRF), the rostral interstitial nucleus of the medial longitudinal fasciculus (riMLF), the OMV, and the CFN (37). The neural network that confers the ability to maintain horizontal eccentric eye positions is distributed over the cerebellum and brainstem comprising the flocculus, the nucleus prepositus hypoglossi, and the adjacent medial vestibular nucleus. Damage to one of these structures causes GEN (38). The affected areas are also wide in patients with SCA3: the degenerative process initially involves the cerebellum and cerebellar nuclei, substantia nigra, and vestibular and oculomotor nuclei, while the thalamus, pons, and medulla oblongata, and cranial nerves from III to XII are later involved (39). A previous study found that GEN was observed in 17% of asymptomatic SCA3 carriers but was absent in non-carrier controls, indicating that GEN may appear preceding other signs in patients with SCA3 (40). Another study also found that several oculomotor alterations could be detected in pre-SCA3 carriers, including SWJ and GEN (29). A study compared the degree of lower extremity ataxia with the degree of oculomotor disorder by using eye tracking tests (ETT) and optokinetic pattern tests (OKP) and found that SCA3 patients showed high ETT and low mean slowest phase velocity in OKP, indicating that SCA3 tends to be characterized by oculomotor disorder preceding extremity ataxia (41). Thus, abnormal eye movement might be a sensitive early biomarker of the impairment of neural networks and brain structures. This may be a reasonable explanation for the predictive role of abnormal eye movements on falls or, more generally, on disease progression in patients with SCA3 detected in the current study.
This is the first study to explore the potential predictive factors of falls in a prospective cohort of patients with SCA3 and to establish a nomogram model to predict falls in SCA3 individual patients. The results showed that pyramidal signs, extrapyramidal symptoms, autonomic dysfunction, and abnormal eye movements are all predictors of falls in SCA3, indicating that SCA3 is not a pure cerebellar disease and that multiple systems are involved in its pathogenesis. However, several limitations should be acknowledged in the current study. The first limitation was the lack of electro-oculography or video-oculography to assess abnormal eye movements objectively. Instead, eye movements were assessed by neurologists who were experienced in movement disorders using accepted bedside techniques described before (12). The second limitation was the lack of external validation of the nomogram model due to the small sample size. Further validation in other patient cohorts is required. Therefore, our findings need validation in an external population before they can be used to counsel patients and their families.
In conclusion, our study revealed that patients with dystonia, hyperreflexia, urinary incontinence, hidrosis, and more types of abnormal eye movement had a more rapid progression to falls in SCA3, which emphasizes the importance of clinical assessment of these characteristics in patients with SCA3. Additionally, our study established the first nomogram to predict falls in patients with SCA3.
Data Availability Statement
The original contributions presented in the study are included in the article/Supplementary Materials, further inquiries can be directed to the corresponding author/s.
Ethics Statement
The studies involving human participants were reviewed and approved by the Ethics Committee of West China Hospital of Sichuan University. The patients/participants provided their written informed consent to participate in this study.
Author Contributions
JL contributed with conception, organization and execution, data collection and statistical analysis, and drafting the manuscript. LZ contributed with execution, data collection, and statistical analysis. BC, YH, KL, XX, and XG contributed with execution and data collection. QW contributed with execution and data collection. RO contributed with conception, organization, execution, and data collection. HS contributed with conception and organization, manuscript review and critique, and responsible for the overall content as the guarantor. All authors contributed to the article and approved the submitted version.
Funding
This study was supported by the 1.3.5 project for disciplines of excellence–Clinical Research Incubation Project, West China Hospital, Sichuan University (Grant No. 2019HXFH016).
Conflict of Interest
The authors declare that the research was conducted in the absence of any commercial or financial relationships that could be construed as a potential conflict of interest.
Acknowledgments
The authors thank all the participants of this study.
Supplementary Material
The Supplementary Material for this article can be found online at: https://www.frontiersin.org/articles/10.3389/fneur.2020.602003/full#supplementary-material
Supplementary Figure 1. Time-dependent receiver operating characteristic curves (t-ROC) of the nomogram prediction. X-axis demonstrates the false positive rate of the model, and Y-axia shows the true positive rate of the model. AUC, areas under the ROC curve; TP, true positive; FP, false positive.
Supplementary Figure 2. Calibration curves of the nomogram prediction.
References
1. Klockgether T, Mariotti C, Paulson HL. Spinocerebellar ataxia. Nat Rev Dis Primers. (2019) 5:24. doi: 10.1038/s41572-019-0074-3
2. Fonteyn EM, Schmitz-Hubsch T, Verstappen CC, Baliko L, Bloem BR, Boesch S, et al. Falls in spinocerebellar ataxias: results of the EuroSCA fall study. Cerebellum. (2010) 9:232–9. doi: 10.1007/s12311-010-0155-z
3. Fonteyn EMR, Schmitz-Hubsch T, Verstappen CCP, Baliko L, Bloem BR, Boesch S, et al. Prospective analysis of falls in dominant ataxias. Eur Neurol. (2013) 69:53–7. doi: 10.1159/000342907
4. van de Warrenburg B, Steijns J, Munneke M, Kremer B, Bloem BJM. Falls in degenerative cerebellar ataxias. Movement Disorder. (2005) 20:497–500. doi: 10.1002/mds.20375
5. Hogue O, Fernandez HH, Floden DP. Predicting early cognitive decline in newly-diagnosed Parkinson's patients: a practical model. Parkinsonism Relat Disord. (2018) 56:70–5. doi: 10.1016/j.parkreldis.2018.06.031
6. Nasreddine ZS, Phillips NA, Bedirian V, Charbonneau S, Whitehead V, Collin I, et al. The montreal cognitive assessment, MoCA: a brief screening tool for mild cognitive impairment. J Am Geriatr Soc. (2005) 53:695–9. doi: 10.1111/j.1532-5415.2005.53221.x
7. Hamilton M. Development of a rating scale for primary depressive illness. Br J Soc Clin Psychol. (1967) 6:278–96. doi: 10.1111/j.2044-8260.1967.tb00530.x
8. Hamilton M. The assessment of anxiety states by rating. Br J Med Psychol. (1959) 32:50–5. doi: 10.1111/j.2044-8341.1959.tb00467.x
9. Johns MW. A new method for measuring daytime sleepiness: the Epworth sleepiness scale. Sleep. (1991) 14:540–5. doi: 10.1093/sleep/14.6.540
10. Buysse DJ, Reynolds, 3rd CF, Monk TH, Berman SR, Kupfer DJ. The Pittsburgh Sleep Quality Index: a new instrument for psychiatric practice and research. Psychiatry Res. (1989) 28:193–213. doi: 10.1016/0165-1781(89)90047-4
11. Schmitz-Hubsch T, du Montcel ST, Baliko L, Berciano J, Boesch S, Depondt C, et al. Scale for the assessment and rating of ataxia: development of a new clinical scale. Neurology. (2006) 66:1717–20. doi: 10.1212/01.wnl.0000219042.60538.92
12. Corbett JJ. The bedside and office neuro-ophthalmology examination. Semin Neurol. (2003) 23:63–76. doi: 10.1055/s-2003-40753
13. Trouillas P, Takayanagi T, Hallett M, Currier RD, Subramony SH, Wessel K, et al. International Cooperative Ataxia Rating Scale for pharmacological assessment of the cerebellar syndrome. The Ataxia Neuropharmacology Committee of the World Federation of Neurology. J Neurol Sci. (1997) 145:205–11. doi: 10.1016/S0022-510X(96)00231-6
15. Kieling C, Prestes PR, Saraiva-Pereira ML, Jardim LB. Survival estimates for patients with Machado-Joseph disease (SCA3). Clin Genet. (2007) 72:543–5. doi: 10.1111/j.1399-0004.2007.00910.x
16. Jhunjhunwala K, Netravathi M, Purushottam M, Jain S. Profile of extrapyramidal manifestations in 85 patients with spinocerebellar ataxia type 1, 2 and 3. J Clin Neurosci. (2014) 21, 1002–6. doi: 10.1016/j.jocn.2013.10.021
17. Catai L, Camargo C, Moro A, Ribas G, Raskin S, Teive HJT, et al. Dystonia in patients with spinocerebellar Ataxia 3 - Machado-Joseph disease: an underestimated diagnosis? Open Neurol J. (2018) 12:41–9. doi: 10.2174/1874205X01812010041
18. Nibbeling E, Delnooz C, de Koning T, Sinke R, Jinnah H, Tijssen M, et al. Using the shared genetics of dystonia and ataxia to unravel their pathogenesis. Neurosci Biobehav Rev. (2017) 75:22–39. doi: 10.1016/j.neubiorev.2017.01.033
19. Kuo P, Gan S, Wang J, Lo R, Figueroa K, Tomishon D, et al. Dystonia and ataxia progression in spinocerebellar ataxias. Parkinsonism Relat Disord. (2017) 45:75–80. doi: 10.1016/j.parkreldis.2017.10.007
20. Diallo A, Jacobi H, Cook A, Labrum R, Durr A, Brice A, et al. Survival in patients with spinocerebellar ataxia types 1, 2, 3, and 6 (EUROSCA): a longitudinal cohort study. Lancet. (2018) 17:327–34. doi: 10.1016/S1474-4422(18)30042-5
21. Tang B, Liu C, Shen L, Dai H, Pan Q, Jing L, et al. Frequency of SCA1, SCA2, SCA3/MJD, SCA6, SCA7, and DRPLA CAG trinucleotide repeat expansion in patients with hereditary spinocerebellar ataxia from Chinese kindreds. Arch Neurol. (2000) 57:540–4. doi: 10.1001/archneur.57.4.540
22. Boonkongchuen P, Pongpakdee S, Jindahra P, Papsing C, Peerapatmongkol P, Wetchaphanphesat S, et al. Clinical analysis of adult-onset spinocerebellar ataxias in Thailand. BMC Neurol. (2014) 14:75. doi: 10.1186/1471-2377-14-75
23. Matsumura R, Takayanagi T, Fujimoto Y, Murata K, Mano Y, Horikawa H, et al. The relationship between trinucleotide repeat length and phenotypic variation in Machado-Joseph disease. Res Profiles. (1996) 139:52–7. doi: 10.1016/0022-510X(96)00016-0
24. Takazaki K, D'Abreu A, Nucci A, Lopes-Cendes I, França MJC. Dysautonomia is frequent in Machado-Joseph disease: clinical and neurophysiological evaluation. Cerebellum. (2013) 12, 513–9. doi: 10.1007/s12311-013-0458-y
25. Moro A, Munhoz R, Moscovich M, Arruda W, Raskin S, Silveira-Moriyama L, et al. Nonmotor symptoms in patients with spinocerebellar Ataxia type 10. Cerebellam. (2017) 16:938–44. doi: 10.1007/s12311-017-0869-2
26. Shimizu H, Yamada M, Toyoshima Y, Ikeuchi T, Onodera O, Takahashi HJA, et al. Involvement of Onuf's nucleus in Machado-Joseph disease: a morphometric and immunohistochemical study. Acta Neuropathol. (2010) 120:439–48. doi: 10.1007/s00401-010-0699-5
27. Anderson TJ, MacAskill MR. Eye movements in patients with neurodegenerative disorders. Nat Rev Neurol. (2013) 9:74–85. doi: 10.1038/nrneurol.2012.273
28. Moscovich M, Okun MS, Favilla C, Figueroa KP, Pulst SM, Perlman S, et al. Clinical evaluation of eye movements in spinocerebellar Ataxias. J Neuro-Ophthalmol. (2015) 35:16–21. doi: 10.1097/WNO.0000000000000167
29. Wu C, Chen DB, Feng L, Zhou XX, Zhang JW, You HJ, et al. Oculomotor deficits in spinocerebellar ataxia type 3: potential biomarkers of preclinical detection and disease progression. CNS Neurosci Ther. (2017) 23:321–8. doi: 10.1111/cns.12676
30. Stephen CD, Schmahmann JD. Eye movement abnormalities are ubiquitous in the spinocerebellar Ataxias. Cerebellum. (2019) 18:1130–6. doi: 10.1007/s12311-019-01044-2
31. Strupp M, Hufner K, Sandmann R, Zwergal A, Dieterich M, Jahn K, et al. Central oculomotor disturbances and nystagmus A window into the brainstem and cerebellum. Deutsches Arzteblatt Int. (2011) 108:197–204. doi: 10.3238/arztebl.2011.0197
32. Zee DS, Yamazaki A, Butler PH, Gucer G. Effects of ablation of flocculus and paraflocculus of eye movements in primate. J Neurophysiol. (1981) 46:878–99. doi: 10.1152/jn.1981.46.4.878
33. Suzuki DA, Noda H, Kase M. Visual and pursuit eye movement-related activity in posterior vermis of monkey cerebellum. J Neurophysiol. (1981) 46:1120–39. doi: 10.1152/jn.1981.46.5.1120
34. Suzuki DA, Keller EL. The role of the posterior vermis of monkey cerebellum in smooth-pursuit eye movement control. Eye I and head movement-related activity. J Neurophysiol. (1988) 59:1–18. doi: 10.1152/jn.1988.59.1.1
35. Suzuki DA, Keller EL. The role of the posterior vermis of monkey cerebellum in smooth-pursuit eye movement control II target velocity-related Purkinje cell activity. J Neurophysiol. (1988) 59:19–40. doi: 10.1152/jn.1988.59.1.19
36. Hotson JR. Cerebellar control of fixation eye movements. Neurology. (1982) 32:31–6. doi: 10.1212/WNL.32.1.31
37. Neurology. The Neurology of Eye Movements, 3rd ed. New York, NY: Oxford University Press (2000).
38. Cannon SC, Robinson DA. Loss of the neural integrator of the oculomotor system from brain stem lesions in monkey. J Neurophysiol. (1987) 57:1383–409. doi: 10.1152/jn.1987.57.5.1383
39. Scherzed W, Brunt ER, Heinsen H, de Vos RA, Seidel K, Burk K, et al. Pathoanatomy of cerebellar degeneration in spinocerebellar ataxia type 2 (SCA2) and type 3 (SCA3). Cerebellum. (2012) 11:749–60. doi: 10.1007/s12311-011-0340-8
40. Raposo M, Vasconcelos J, Bettencourt C, Kay T, Coutinho P, Lima M. Nystagmus as an early ocular alteration in Machado-Joseph disease (MJD/SCA3). BMC Neurol. (2014) 14:17. doi: 10.1186/1471-2377-14-17
Keywords: spinocerebellar ataxia type 3, eye movements, falls, nomogram, prospective
Citation: Lin J, Zhang L, Cao B, Wei Q, Ou R, Hou Y, Xu X, Liu K, Gu X and Shang H (2021) Establish a Nomogram to Predict Falls in Spinocerebellar Ataxia Type 3. Front. Neurol. 11:602003. doi: 10.3389/fneur.2020.602003
Received: 02 September 2020; Accepted: 17 December 2020;
Published: 27 January 2021.
Edited by:
Tim Anderson, University of Otago, Christchurch, New ZealandReviewed by:
Elsdon Storey, Monash University, AustraliaZhong Pei, Sun Yat-sen University, China
Susan L. Perlman, Ronald Reagan UCLA Medical Center, United States
Copyright © 2021 Lin, Zhang, Cao, Wei, Ou, Hou, Xu, Liu, Gu and Shang. This is an open-access article distributed under the terms of the Creative Commons Attribution License (CC BY). The use, distribution or reproduction in other forums is permitted, provided the original author(s) and the copyright owner(s) are credited and that the original publication in this journal is cited, in accordance with accepted academic practice. No use, distribution or reproduction is permitted which does not comply with these terms.
*Correspondence: Huifang Shang, hfshang2002@126.com