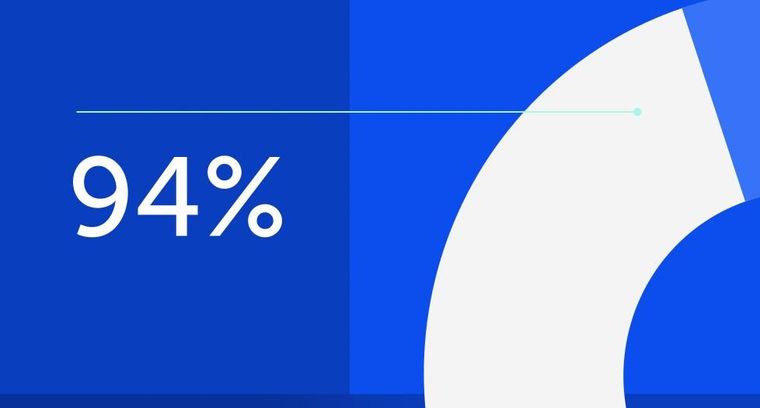
94% of researchers rate our articles as excellent or good
Learn more about the work of our research integrity team to safeguard the quality of each article we publish.
Find out more
ORIGINAL RESEARCH article
Front. Neurol., 30 October 2020
Sec. Multiple Sclerosis and Neuroimmunology
Volume 11 - 2020 | https://doi.org/10.3389/fneur.2020.581700
This article is part of the Research TopicCognition in Multiple SclerosisView all 16 articles
Background: Cognitive reserve (CR) could attenuate the impact of the brain burden on the cognition in people with multiple sclerosis (PwMS).
Objective: To explore the relationship between CR and structural brain connectivity and investigate their role on cognition in PwMS cognitively impaired (PwMS-CI) and cognitively preserved (PwMS-CP).
Methods: In this study, 181 PwMS (71% female; 42.9 ± 10.0 years) were evaluated using the Cognitive Reserve Questionnaire (CRQ), Brief Repeatable Battery of Neuropsychological tests, and MRI. Brain lesion and gray matter volumes were quantified, as was the structural network connectivity. Patients were classified as PwMS-CI (z scores = −1.5 SD in at least two tests) or PwMS-CP. Linear and multiple regression analyses were run to evaluate the association of CRQ and structural connectivity with cognition in each group. Hedges's effect size was used to compute the strength of associations.
Results: We found a very low association between CRQ scores and connectivity metrics in PwMS-CP, while in PwMS-CI, this relation was low to moderate. The multiple regression model, adjusted for age, gender, mood, lesion volume, and graph metrics (local and global efficiency, and transitivity), indicated that the CRQ (β = 0.26, 95% CI: 0.17–0.35) was associated with cognition (adj R2 = 0.34) in PwMS-CP (55%). In PwMS-CI, CRQ (β = 0.18, 95% CI: 0.07–0.29), age, and network global efficiency were independently associated with cognition (adj R2 = 0.55). The age- and gender-adjusted association between CRQ score and global efficiency on having an impaired cognitive status was −0.338 (OR: 0.71, p = 0.036) and −0.531 (OR: 0.59, p = 0.002), respectively.
Conclusions: CR seems to have a marginally significant effect on brain structural connectivity, observed in patients with more severe clinical impairment. It protects PwMS from cognitive decline regardless of their cognitive status, yet once cognitive impairment has set in, brain damage and aging are also influencing cognitive performance.
Cognitive impairment (CI) has been reported in 40–70% of people with multiple sclerosis (PwMS) (1) and it has a negative impact on their quality of life (2). It is associated with the combined effect of both white matter (WM) and gray matter (GM) damage (3). However, magnetic resonance imaging (MRI) metrics like lesion volume (Lv) or GM volume (GMv) only partially explain the cognitive changes of PwMS. Non-conventional MRI techniques, such as diffusion-weighted imaging (DWI) or functional MRI (fMRI), can be used to further explore structural and functional brain connectivity and its associations with CI (4, 5). Moreover, through theoretical graph analysis, it has been suggested that disrupting the optimal balance between local integration and global segregation of network components might hamper information flow, exerting a negative impact on cognition (5, 6).
Some individuals better maintain their cognitive performance despite the presence of substantial brain damage. This clinico-pathological dissociation (7) indicates that certain factors protect against cognitive decline, such as the cognitive reserve (CR), understood to be lifelong intellectual enrichment that attenuates the negative effect of MS disease burden on neuropsychological activity (8, 9). Previous studies in MS and other neurodegenerative diseases, such as Alzheimer's disease, suggested that patients with higher CR displayed better cognitive function regardless of having similar brain damage (7, 10, 11). Indeed, CR seems to preserve brain network functional connectivity counterbalancing the impact of the disruption of WM tracts due to lesions in MS on cognition (12). However, this protective role of the CR diminishes over the MS disease course as the brain burden becomes stronger (13, 14). Before the appearance of CI, the brain probably employs adaptive and compensatory mechanisms, undergoing structural and functional reorganization in response to the pathological changes caused by MS (5, 15). However, the accumulation of brain damage can lead to network dysfunction that may contribute significantly to the development of CI in PwMS (16). As far as we know, the relationship between CR and structural brain connectivity remains unexplored.
We hypothesized that individuals with higher CR would exhibit higher structural connectivity and, consequently, better cognitive performance. Also, the influence of CR on cognitive performance in PwMS may be distinct before and after the emergence of CI. Therefore, we aimed to understand the association between CR and structural connectivity integrity and their impact on cognition in PwMS. For this, we analyzed their role in patients with different cognitive status, thus in PwMS cognitively impaired (PwMS-CI) and in those who remained cognitively preserved (PwMS-CP).
A cohort of 181 PwMS (aged 18–65 years) who fulfilled the 2010 McDonald criteria (17) was consecutively selected at the MS Unit of the Hospital Clinic of Barcelona. To be included, patients had to be free from relapses in the last 30 days and have no significant neurological or psychiatric condition that could interfere with cognitive functioning. In this cross-sectional study, patients were evaluated using clinical and cognitive scales, and they underwent an MRI scan. We collected data regarding MS type, disease duration, current treatment, and global disability, the latter measured using the Expanded Disability Status Scale (EDSS) (18). In addition, a global score of depression and anxiety symptoms was obtained for the patients using the Hospital Anxiety and Depression Scale (HADS) (19). The Ethics Committee at the Hospital Clinic of Barcelona approved the study and all the participants signed an informed consent form prior to their enrollment on the study.
Cognition was assessed using the Brief Repeatable Battery of Neuropsychological tests (BRB-N) (20). This battery includes different tests assessing cognitive domains as follows: (1) verbal learning and memory: Selective Reminding Test (SRT, with two subtests: consistent long-term retrieval as an indicator of consolidation, and delayed retrieval); (2) visuospatial learning and memory: 10/36 Spatial Recall Test (SPART, with two subtests: immediate retrieval and for delayed retrieval); (3) attention, working memory, and information processing speed: Symbol Digit Modalities Test (SDMT) and Paced Auditory Serial Addition Test (PASAT) 3 s per digit version; and (4) verbal fluency and cognitive flexibility: Word List Generation (WLG).
We first calculated z scores for all BRB-N tests, using demographically adjusted (age and education) regression models according to the normative data published in the Spanish population (21), classifying patients as PwMS-CI or PwMS-CP. Patients were classified as PwMS-CI if performance was below z = −1.5 standard deviations (SD) of the norm in at least two cognitive tests of the same or different cognitive domain. In addition, raw values were transformed into z scores (zBRB) by subtracting the mean and dividing by the SD of the whole sample in order to obtain a mean score of cognitive performance, avoiding the educational effect related to CR and the aging effect on cognition.
CR was assessed using the Cognitive Reserve Questionnaire (CRQ) (22), a standardized scale in which higher scores represent higher levels of CR (maximum 25 points). This test is composed of eight items that measure different intellectual enrichment factors, including the individual's education, their parent's education, training courses, occupation, musical training, language studies, reading activity, and intellectual games in which they have participated during their adult lifetime. Items do not contemplate a specific period, thus addressing experiences throughout life (23). This questionnaire was administered by an experienced neuropsychologist before the cognitive assessment. The CRQ has been previously applied to both healthy elderly and diseased populations (24, 25).
MR images were acquired on a 3-Tesla Magnetom Trio (SIEMENS, Erlanger, Germany) scanner using a 32-channel phased-array head coil. The protocol applied involved a 3D-Magnetization Prepared Rapid Acquisition Gradient Echo (MPRAGE), 3D-T2 fluid-attenuated inversion recovery (FLAIR), and DWI sequences (see Supplementary Material for a detailed description of the sequences).
WM lesions were defined semi-automatically on the 3D-MPRAGE sequence using the Jim7 software (http://www.xinapse.com/j-im-7-software/). To improve MS lesion identification, the co-registered 3D-FLAIR image was used as a reference. Thereafter, lesion in-painting was applied to the 3D-MPRAGE image to enhance segmentation and registration in PwMS (26). The FSL and SIENAX tools (27) were used to obtain the normalized Lv and GMv (nLv and nGMv).
Cortical parcellation was performed with the Mindboggle software (28) using a cortical labeling parcellation scheme from FreeSurfer (https://surfer.nmr.mgh.harvard.edu/) that is based on the Desikan-Killiany atlas (29). Subcortical GM structures were segmented by applying the FIRST tool (fsl.fmrib.ox.ac.uk/fsl/fslwiki/FIRST). Thirty-one cortical regions and seven subcortical GM structures per hemisphere were used as nodes of the network.
DWI processing was performed as described previously (5, 30). High Angular Resolution Diffusion Imaging (HARDI) images were denoised and corrected for geometric distortions and head motion (31). The structural connectome was obtained using multi-tissue constrained spherical deconvolution-based tractography, applying the second-order integration over fiber orientation distributions and an anatomically constrained tractography framework (32), available in the MRtrix3 software package (http://www.mrtrix.org/). WM and lesion masks were registered to the undistorted HARDI images by applying boundary-based registration (33). Fiber tracking required a seeding mask that corresponded to the normal-appearing WM and MS lesions, thereby avoiding premature cessation of the reconstruction in areas with a more complex structural architecture and with low fractional anisotropy (FA) (30). Anatomical exclusion criteria were applied to minimize the number of anatomically aberrant connections originated from the tractography procedure (30). Finally, the total 76 segmented cortical and subcortical regions were used to define the nodes of the network, and matrices were generated to represent the mean FA values of the connections.
Graph theory metrics were computed using the Brain Connectivity Toolbox (https://sites.google.com/site/bctnet). Graph metrics were analyzed to express the global connectivity properties of the network, including the nodal strength (the sum of weights connected to the node); measures of segregation, such as the local efficiency (the average of the inverse of the shortest path length in the network computed on node neighborhoods), the clustering coefficient (the fraction of a node's neighbors that are neighbors of each other), and transitivity (the ratio of triangles to triplets in the network); integration, as measured through the global efficiency (the average inverse shortest path length in the whole network); and brain resilience, reflected by assortativity (a correlation coefficient of the degrees of separation of all the nodes at two opposite ends of a link) (34). A representative image of the MRI metrics used in this study is presented in Figure 1.
Figure 1. Structural connectivity framework for the neuroimaging processing of the volumetric (A) and network (B) analysis. FA, fractional anisotropy; nGMv, normalized gray matter volume; nLv, normalized lesion volume.
All demographic, clinical, neuropsychological, MRI markers of brain burden, and connectivity values were described through the mean and SD, and by the absolute numbers and the proportions for quantitative and qualitative data, respectively. The normality of continuous data was checked using histograms and appropriate statistical methods as the Shapiro–Wilks test. We compared the aforementioned characteristics between PwMS-CI and PwMS-CP patients using a Chi-squared test, a Wilcoxon–Mann–Whitney U-test or a Student's t-test, depending on the data distribution.
Age- and gender-adjusted linear regressions were done to analyze the associations between CRQ and cognition, between structural connectivity and cognition, and between CRQ and structural connectivity on the entire cohort and in each group of PwMS separately. To understand the role of CR and structural connectivity on cognitive performance in the context of other demographic and MS-related factors, we fitted a multiple regression model that included relevant demographic and clinical variables. Variables were standardized using the mean and SD: CRQ score, age, gender, EDSS, HADS score, nLv, nGMv, and graph measures of segregation, integration, and brain resilience (nodal strength, global and local efficiency, clustering coefficient, transitivity, and assortativity). The Akaike Information Criterion (AIC) was then used to select the variables that best fit a model based on the whole cohort. As the main objective of the study was to determine the influence of CR and structural connectivity on cognitive performance in patients with different cognitive status, we applied the same multiple regression model separately in PwMS-CI and PwMS-CP, with the variables selected from the AIC. We computed the strength of the associations using Hedges' g effect size.
Furthermore, an age- and gender-adjusted logistic regression analysis was used to estimate the odds ratio (OR) of having an impaired cognitive performance associated with the increase per unit of the CR and MRI connectivity metrics associated to cognition in the multiple regression model.
Statistical analyses were performed with R statistical software (version 3.6.0, www.R-project.org), setting the level of significance at p < 0.05 and correcting multiple comparisons for the false discovery rate (FDR).
This study was carried out on a population of 181 PwMS who were mostly female (71%), middle-aged adults (42.9 ± 10.0 years), and who had a median CRQ score of 16 (interquartile range, IQR: 12–24). In the cohort, 81 patients (45%) were classified as PwMS-CI, and the remaining 100 patients (55%) were considered PwMS-CP. The group of PwMS-CI more frequently presented with a secondary progressive phenotype of the disease, with lower CRQ scores and with higher EDSS scores. They also presented worse volumetric and connectivity measures in images than PwMS-CP, although assortativity was no different (see Table 1 for further details).
We found significant associations between CRQ and zBRB scores in the entire cohort (β = 0.324, 95% confidence interval, CI: 0.24–0.41, p < 0.001), in PwMS-CP (β = 0.253, 95% CI: 0.17–0.34, p < 0.001; Hedges' g: 0.521, 95% CI: 0.22–0.82), and also in PwMS-CI (β = 0.300, 95% CI: 0.19–0.41, p < 0.001; Hedges' g: 0.626, 95% CI: 0.33–0.93). In parallel, significant associations were also found between graph structural connectivity properties and cognitive scores in the entire cohort and in the PwMS-CI group in all studied graph measures except for assortativity (entire PwMS cohort β between 0.215 and 0.285, 95% CI: 0.12–0.38, p < 0.001) (Table 2).
Table 2. Associations between graph structural connectivity properties and cognition in both PwMS groups.
Regarding the relationship between the CRQ and structural connectivity, we found a very low effect size association in PwMS-CP and a low-to-moderate correlation in PwMS-CI. However, after multiple comparisons, those associations did not reach statistical significance (p < 0.05). Nodal strength, transitivity, and global efficiency were the metrics showing a moderate effect size in this group (Table 3).
Based on the AIC, the final multiple linear regression model included CRQ score, age, gender, the HADS score, global and local efficiency, transitivity, and nLv as variables associated with cognitive performance. This model was applied to each group of patients separately. In PwMS-CP, 34% of the cognitive performance (mean zBRB score) was explained by the model (adj R2 = 0.34, p < 0.001). In this group, a one-point increase in the CRQ score was associated with a 0.26-point increase in the zBRB (the only significant variable in the model) (β = 0.259, 95% CI: 0.17–0.35, p < 0.001). The CRQ showed a moderate association with cognitive performance (Hedges' g = 0.534, 95% CI: 0.24–0.83). In the PwMS-CI, 55% of the variability in the zBRB was explained by the model (adj R2 = 0.55, p < 0.001). In these patients, a one-point increase in the CRQ score was associated with a 0.18-point increase in the zBRB (β = 0.179, 95% CI: 0.07–0.29, p = 0.012). Moreover, in this model age (β = −0.119, 95% CI: −0.21 to −0.02, p = 0.041) and global efficiency (β = 0.504, 95% CI: 0.18–0.83, p = 0.012) were significantly associated negatively and positively with the zBRB, respectively (Table 4 and Figure 2). Transitivity and global efficiency were the variables showing a moderate and high association with cognition (transitivity Hedges' g = −0.590, 95% CI: −0.89 to −0.29 and global efficiency Hedges' g = 1.162, 95% CI: 0.85–1.48; Table 4).
Table 4. Associations between clinical and MRI variables and cognitive performance in both PwMS groups.
Figure 2. Prediction value in the cognitive explanatory model. Marginal effects of the Cognitive Reserve Questionnaire (CRQ) score (A), age (B), and global efficiency (C) are shown. The PwMS-CP group is colored green and the PwMS-CI is represented in red.
We evaluated the predictive value of CRQ and global efficiency on cognitive status. The age- and gender-adjusted association between CRQ score and global efficiency on having an impaired cognitive status was −0.338 (OR: 0.71, 95% CI: 0.52–0.97, p = 0.036) for CRQ score and −0.531 (OR: 0.59, 95% CI: 0.41–0.82, p = 0.002) for global efficiency.
In this study, we set out to understand the protective effect of CR on structural network integrity and their impact on cognition in PwMS in relation to other important demographic and MS-related factors. As such, we explored the relationship between CR and structural brain connectivity and analyzed different determinants of neuropsychological performance, including clinical information and some metrics of brain burden, in the presence or absence of CI. Although we found a marginal association between CR and structural connectivity integrity, it is only after brain damage reaches a significant level and CI is present that we found a moderate association between these measures. While the CR is the only variable associated with cognition in patients with good cognitive performance, when CI flourishes, structural brain damage, and aging are also related to this parameter. Indeed, in PwMS-CI, the impact of network integrity dysfunction is stronger than the effect of lifelong intellectual enhancement. The observed benefit of CR on cognitive performance has practical implications, including the implementation of strategies for intellectual life enrichment in addition to conventional therapies to palliate the effect of brain damage.
The relationship between CR and cognition has been studied in several neurological diseases, including MS (7). It has been suggested that more intellectual enrichment potentially protects PwMS from cognitive decline (7, 14, 35). Indeed, we found that higher scores in the CRQ scale were associated with better cognitive performance, meaning that CR could help to preserve the cognitive function. However, to the best of our knowledge, the effect that CR may have on structural connectivity networks remains unknown. In this regard, we observed a relationship between structural brain connectivity dysfunction and CRQ scores with low-to-moderate effect sizes. Specifically, in the PwMS-CI group, lower scores of CRQ were associated with decreased nodal strength, transitivity, and global efficiency. Thus, CR might have a positive effect on the integration mechanisms that support long-range connections (36) and on network segregation, reflecting compensatory mechanisms against cerebral damage. Other studies focusing on functional networks found links between CR and network efficiency in healthy elderly individuals (15) and in PwMS (12), which makes the protective role of CR more plausible on functional than on structural connectivity. Considered together, a higher CR tends to ameliorate the negative impact of MS on brain connectivity and seems to protect against cognitive decline.
Investigating the interaction between CR and structural connectivity on cognitive performance, we demonstrate the protective effect of intellectual life enrichment assessed with the CRQ on cognition in PwMS, with and without CI, irrespective of age, mood disorders, and brain burden. More specifically, we studied CR and structural connectivity integrity along with clinical and more conventional MRI parameters of brain damage in a model that explains cognitive performance in patients with different cognitive status. In the PwMS-CP group, CR explains the 34% of the variance in neuropsychological performance, whereas in the PwMS-CI group, CR together with age and global efficiency explain the 55% of the variance in cognitive performance. In this latter group, the association between CR and cognition was weaker than in the PwMS-CP cohort. The link between aging and structural brain connectivity with cognition in PwMS-CI was expected, since, as patients get older and brain damage due to pathological events accumulates, its impact on cognition augments. Aging is known to promote alterations in neuronal structure, loss of synapses, and dysfunction of neuronal networks (37). Also, previous studies have described a decreased global efficiency on PwMS compared to healthy volunteers, suggesting a disrupted topological organization of the WM networks due to impaired structural connections (38). Besides, abnormalities of global efficiency have been associated with negative consequences on cognition impacting different cognitive domains such as memory and attention performance (39–41). As the compensation and adaptation of brain mechanisms probably deteriorate with age and with brain damage, it would appear that brain network dysfunction leads to CI (16). Overall, our results reinforce the protective capacity of CR at any stage of the disease, including in PwMS that suffer cognitive decline.
Our findings entail relevant clinical repercussions as they emphasize the use of the CRQ scale in routine clinical practice to achieve a comprehensive assessment of PwMS and to identify at-risk individuals of cognitive decline. Neurologists should recommend that PwMS participate in early interventions to maximize their brain resources, such as intellectual enhancement or neuropsychological programs.
Our study is not absent of limitations, particularly as our cohort was composed predominantly of relapsing-remitting MS patients, and thus, it limits the capacity to generalize these findings to more advanced phenotypes. However, this is the most common phenotype encountered in the clinic in the current treatment era, with lower rates of worsening and evolution to SPMS in patients compared to earlier natural history cohorts (42). Furthermore, despite the fact that CRQ scores were different in the PwMS-CP and PwMS-CI groups, results remained unchanged when we balanced CRQ scores (data not shown). In addition, CR cannot be measured directly and there is still no consensus as to what is the best proxy for CR (43). Nevertheless, the CRQ measures different intellectual enrichment factors addressing experiences throughout life and is easily applicable in the clinical field due to its brevity and the absence of open responses (22). We do not have longitudinal data on cognitive performance so we were unable to establish a causal effect, yet our results are promising and in accordance with the existing literature. Finally, the inclusion of fMRI in future studies might be useful to further explore compensatory and plasticity mechanisms driven by intellectual enrichment in MS.
In conclusion, CR could have a positive effect on the connectivity of the brain network, which can be observed in patients with more severe clinical impairment. The results presented here highlight the important protective value of CR on cognitive performance, regardless of cognitive status. However, once CI has flourished, over and above the effect of CR, cognition is also influenced by the presence of structural brain damage and aging. This study draws attention to the benefits of promoting an intellectually rich lifestyle in PwMS, as it may have an important impact on their future cognitive status through all stages of the disease.
The raw data supporting the conclusions of this article will be made available by the authors, without undue reservation.
The studies involving human participants were reviewed and approved by Ethical Committee at the Hospital Clinic Barcelona. The patients/participants provided their written informed consent to participate in this study.
All authors listed have made a substantial, direct and intellectual contribution to the work, and approved it for publication.
This work was funded by a Proyecto de Investigación en Salud (FIS 2015. PI15/00587, SL, AS, FIS 2018 PI18/01030, SL, AS), integrated into the Plan Estatal de Investigación Científica y Técnica de Innovación I+D+I, and co-funded by the Instituto de Salud Carlos III-Subdirección General de Evaluación and the Fondo Europeo de Desarrollo Regional (FEDER, Otra manera de hacer Europa), the Red Española de Esclerosis Múltiple (REEM: RD16/0015/0002, RD16/0015/0003, RD12/0032/0002, RD12/0060/01-02), Ayuda Merck de Investigación 2017, the Premi Fundació Societat Catalana de Neurologia 2017, TEVA SLU, and the Fundació Cellex. EL-S holds a predoctoral grant from the University of Barcelona (APIF). AP-U was supported by the Medical Research Council (Grant no. MR/K501256/1 and MR/N013468/1) and the Fundación Alfonso Martin Escudero.
NS-V received compensation for consulting services and speaker honoraria from Genzyme-Sanofi, Almirall, Novartis, Merck, and Biogen. MS received speaker honoraria from Genzyme, Novartis, and Biogen. IP-V holds a patent for an affordable eye tracking system to measure eye movement in neurological diseases and holds stock options in Aura Innovative Robotics. YB received speaking honoraria from Biogen, Novartis, and Genzyme. EM-L received grants for the institution for research and educational purposes from Sanofi-Genzyme and Novartis. She received travel support for international and national meetings over the last 3 years from Roche and Sanofi-Genzyme. She has received honoraria for consultancies from Roche and Sanofi. She has been a member of the working committee of International Multiple Sclerosis Visual System (IMSVISUAL) Consortium. From 16 April 2019, she works in the European Medicine Agency (Division E Evaluation); AS received compensation for consulting services and speaker honoraria from Bayer-Schering, Merck-Serono, Biogen-Idec, Sanofi-Aventis, TEVA, Novartis, and Roche. SL received compensation for consulting services and speaker honoraria from Biogen Idec, Novartis, TEVA, Genzyme, Sanofi, and Merck. MA holds equities in Bionure and Goodgut.
The remaining authors declare that the research was conducted in the absence of any commercial or financial relationships that could be construed as a potential conflict of interest.
The Supplementary Material for this article can be found online at: https://www.frontiersin.org/articles/10.3389/fneur.2020.581700/full#supplementary-material
1. Chiaravalloti ND, DeLuca J. Cognitive impairment in multiple sclerosis. Lancet Neurol. (2008) 7:1139–51. doi: 10.1016/S1474-4422(08)70259-X
2. Højsgaard Chow H, Schreiber K, Magyari M, Ammitzbøll C, Börnsen L, Romme Christensen J, et al. Progressive multiple sclerosis, cognitive function, and quality of life. Brain Behav. (2018) 8:e00875. doi: 10.1002/brb3.875
3. Di Filippo M, Portaccio E, Mancini A, Calabresi P. Multiple sclerosis and cognition: synaptic failure and network dysfunction. Nat Rev Neurosci. (2018) 19:599–609. doi: 10.1038/s41583-018-0053-9
4. Hulst HE, Steenwijk MD, Versteeg A, Pouwels PJW, Vrenken H, Uitdehaag BMJ, et al. Cognitive impairment in MS: impact of white matter integrity, gray matter volume, and lesions. Neurology. (2013) 80:1025–32. doi: 10.1212/WNL.0b013e31828726cc
5. Llufriu S, Martinez-Heras E, Solana E, Sola-Valls N, Sepulveda M, Blanco Y, et al. Structural networks involved in attention and executive functions in multiple sclerosis. Neuroimage Clin. (2017) 13:288–96. doi: 10.1016/j.nicl.2016.11.026
6. Sporns O. Network attributes for segregation and integration in the human brain. Curr Opin Neurobiol. (2013) 23:162–71. doi: 10.1016/j.conb.2012.11.015
7. Santangelo G, Altieri M, Gallo A, Trojano L. Does cognitive reserve play any role in multiple sclerosis? A meta-analytic study. Mult Scler Relat Disord. (2019) 30:265–76. doi: 10.1016/j.msard.2019.02.017
8. Sumowski JF, Leavitt VM. Cognitive reserve in multiple sclerosis. Mult Scler. (2013) 19:1122–7. doi: 10.1177/1352458513498834
9. Stern Y. What is cognitive reserve? Theory and research application of the reserve concept. J Int Neuropsychol Soc. (2002) 8:448–60. doi: 10.1017/S1355617702813248
10. Modica CM, Bergsland N, Dwyer MG, Ramasamy DP, Carl E, Zivadinov R, et al. Cognitive reserve moderates the impact of subcortical gray matter atrophy on neuropsychological status in multiple sclerosis. Mult Scler. (2016) 22:36–42. doi: 10.1177/1352458515579443
11. Sumowski JF, Rocca MA, Leavitt VM, Dackovic J, Mesaros S, Drulovic J, et al. Brain reserve and cognitive reserve protect against cognitive decline over 4.5 years in MS. Neurology. (2014) 82:1776–83. doi: 10.1212/WNL.0000000000000433
12. Fuchs TA, Benedict RHB, Bartnik A, Choudhery S, Li X, Mallory M, et al. Preserved network functional connectivity underlies cognitive reserve in multiple sclerosis. Hum Brain Mapp. (2019) 40:5231–41. doi: 10.1002/hbm.24768
13. Amato MP, Razzolini L, Goretti B, Stromillo ML, Rossi F, Giorgio A, et al. Cognitive reserve and cortical atrophy in multiple sclerosis: a longitudinal study. Neurology. (2013) 80:1728–33. doi: 10.1212/WNL.0b013e3182918c6f
14. Rocca MA, Riccitelli GC, Meani A, Pagani E, Del Sette P, Martinelli V, et al. Cognitive reserve, cognition, and regional brain damage in MS: a 2 -year longitudinal study. Mult Scler. (2019) 25:372–81. doi: 10.1177/1352458517750767
15. Marques P, Moreira P, Magalhães R, Costa P, Santos N, Zihl J, et al. The functional connectome of cognitive reserve. Hum Brain Mapp. (2016) 37:3310–22. doi: 10.1002/hbm.23242
16. Schoonheim MM, Meijer KA, Geurts JJG. Network collapse and cognitive impairment in multiple sclerosis. Front Neurol. (2015) 6:82. doi: 10.3389/fneur.2015.00082
17. Polman CH, Reingold SC, Banwell B, Clanet M, Cohen JA, Filippi M, et al. Diagnostic criteria for multiple sclerosis: 2010 revisions to the McDonald criteria. Ann Neurol. (2011) 69:292–302. doi: 10.1002/ana.22366
18. Kurtzke JF. Rating neurologic impairment in multiple sclerosis: an expanded disability status scale (EDSS). Neurology. (1983) 33:1444–52. doi: 10.1212/WNL.33.11.1444
19. Zigmond AS, Snaith RP. The hospital anxiety and depression scale. Acta Psychiatr Scand. (1983) 67:361–70. doi: 10.1111/j.1600-0447.1983.tb09716.x
20. Rao SM, Leo GJ, Bernardin L, Unverzagt F. Cognitive dysfunction in multiple sclerosis. I. Frequency, patterns, and prediction. Neurology. (1991) 41:685–91. doi: 10.1212/WNL.41.5.685
21. Sepulcre J, Vanotti S, Hernández R, Sandoval G, Cáceres F, Garcea O, et al. Cognitive impairment in patients with multiple sclerosis using the brief repeatable battery-neuropsychology test. Mult Scler. (2006) 12:187–95. doi: 10.1191/1352458506ms1258oa
22. Rami L, Valls-Pedret C, Bartres-Faz D, Caprile C, Solé-Padullés C, Castellvi M, et al. Cognitive reserve questionnaire. Scores obtained in a healthy elderly population and in one with Alzheimer's disease. Rev Neurol. (2011) 52:195–201. doi: 10.33588/rn.5204.2010478
23. Landenberger T, de O., Cardoso N, de Oliveira CR, de L., Argimon II. Instruments for measuring cognitive reserve: a systematic review. Psicologia Teoria Prática. (2019) 21:58–74. doi: 10.5935/1980-6906/psicologia.v21n2p58-74
24. Kartschmit N, Mikolajczyk R, Schubert T, Lacruz ME. Measuring cognitive reserve (CR) – a systematic review of measurement properties of CR questionnaires for the adult population. PLoS ONE. (2019) 14:e0219851. doi: 10.1371/journal.pone.0219851
25. Sola-Valls N, Ariño H, Escudero D, Solana E, Lladó A, Sánchez-Valle R, et al. Telemedicine assessment of long-term cognitive and functional status in anti-leucine-rich, glioma-inactivated 1 encephalitis. Neurol Neuroimmunol Neuroinflamm. (2020) 7:e652. doi: 10.1212/NXI.0000000000000652
26. Battaglini M, Jenkinson M, de Stefano N. Evaluating and reducing the impact of white matter lesions on brain volume measurements. Hum Brain Mapp. (2012) 33:2062–71. doi: 10.1002/hbm.21344
27. Smith SM, Jenkinson M, Woolrich MW, Beckmann CF, Behrens TEJ, Johansen-Berg H, et al. Advances in functional and structural MR image analysis and implementation as FSL. Neuroimage. (2004) 23(Suppl. 1):S208–19. doi: 10.1016/j.neuroimage.2004.07.051
28. Klein A, Ghosh SS, Bao FS, Giard J, Häme Y, Stavsky E, et al. Mindboggling morphometry of human brains. PLoS Comput Biol. (2017) 13:e1005350. doi: 10.1371/journal.pcbi.1005350
29. Desikan RS, Ségonne F, Fischl B, Quinn BT, Dickerson BC, Blacker D, et al. An automated labeling system for subdividing the human cerebral cortex on MRI scans into gyral based regions of interest. Neuroimage. (2006) 31:968–80. doi: 10.1016/j.neuroimage.2006.01.021
30. Martínez-Heras E, Varriano F, Prčkovska V, Laredo C, Andorrà M, Martínez-Lapiscina EH, et al. Improved framework for tractography reconstruction of the optic radiation. PLoS ONE. (2015) 10:e0137064. doi: 10.1371/journal.pone.0137064
31. Veraart J, Fieremans E, Novikov DS. Diffusion MRI noise mapping using random matrix theory. Magn Reson Med. (2016) 76:1582–93. doi: 10.1002/mrm.26059
32. Smith RE, Tournier JD, Calamante F, Connelly A. Anatomically-constrained tractography: improved diffusion MRI streamlines tractography through effective use of anatomical information. Neuroimage. (2012) 62:1924–38. doi: 10.1016/j.neuroimage.2012.06.005
33. Greve DN, Fischl B. Accurate and robust brain image alignment using boundary-based registration. Neuroimage. (2009) 48:63–72. doi: 10.1016/j.neuroimage.2009.06.060
34. Rubinov M, Sporns O. Complex network measures of brain connectivity: uses and interpretations. Neuroimage. (2010) 52:1059–69. doi: 10.1016/j.neuroimage.2009.10.003
35. Artemiadis A, Bakirtzis C, Ifantopoulou P, Zis P, Bargiotas P, Grigoriadis N, et al. The role of cognitive reserve in multiple sclerosis: a cross-sectional study in 526 patients. Mult Scler Relat Disord. (2020) 41:102047. doi: 10.1016/j.msard.2020.102047
36. Fornito A, Zalesky A, Breakspear M. The connectomics of brain disorders. Nat Rev Neurosci. (2015) 16:159–72. doi: 10.1038/nrn3901
37. Petralia RS, Mattson MP, Yao PJ. Communication breakdown: the impact of ageing on synapse structure. Ageing Res Rev. (2014) 14:31–42. doi: 10.1016/j.arr.2014.01.003
38. Shu N, Liu Y, Li K, Duan Y, Wang J, Yu C, et al. Diffusion tensor tractography reveals disrupted topological efficiency in white matter structural networks in multiple sclerosis. Cereb Cortex. (2011) 21:2565–77. doi: 10.1093/cercor/bhr039
39. Llufriu S, Rocca MA, Pagani E, Riccitelli GC, Solana E, Colombo B, et al. Hippocampal-related memory network in multiple sclerosis: a structural connectivity analysis. Mult Scler. (2019) 25:801–10. doi: 10.1177/1352458518771838
40. Solana E, Martinez-Heras E, Martinez-Lapiscina EH, Sepulveda M, Sola-Valls N, Bargalló N, et al. Magnetic resonance markers of tissue damage related to connectivity disruption in multiple sclerosis. Neuroimage Clin. (2018) 20:161–8. doi: 10.1016/j.nicl.2018.07.012
41. Hawkins R, Shatil AS, Lee L, Sengupta A, Zhang L, Morrow S, et al. Reduced global efficiency and random network features in patients with relapsing-remitting multiple sclerosis with cognitive impairment. AJNR Am J Neuroradiol. (2020) 41:449–55. doi: 10.3174/ajnr.A6435
42. University of California San Francisco MS-EPIC Team Cree BAC, Gourraud P-A, Oksenberg JR, Bevan C. Long-term evolution of multiple sclerosis disability in the treatment era. Ann Neurol. (2016) 80:499–510. doi: 10.1002/ana.24747
Keywords: cognitive reserve, structural connectivity, graph theory, cognition, multiple sclerosis
Citation: Lopez-Soley E, Solana E, Martínez-Heras E, Andorra M, Radua J, Prats-Uribe A, Montejo C, Sola-Valls N, Sepulveda M, Pulido-Valdeolivas I, Blanco Y, Martinez-Lapiscina EH, Saiz A and Llufriu S (2020) Impact of Cognitive Reserve and Structural Connectivity on Cognitive Performance in Multiple Sclerosis. Front. Neurol. 11:581700. doi: 10.3389/fneur.2020.581700
Received: 09 July 2020; Accepted: 30 September 2020;
Published: 30 October 2020.
Edited by:
Rosa Cortese, University College London, United KingdomReviewed by:
Antonio Giorgio, University of Siena, ItalyCopyright © 2020 Lopez-Soley, Solana, Martínez-Heras, Andorra, Radua, Prats-Uribe, Montejo, Sola-Valls, Sepulveda, Pulido-Valdeolivas, Blanco, Martinez-Lapiscina, Saiz and Llufriu. This is an open-access article distributed under the terms of the Creative Commons Attribution License (CC BY). The use, distribution or reproduction in other forums is permitted, provided the original author(s) and the copyright owner(s) are credited and that the original publication in this journal is cited, in accordance with accepted academic practice. No use, distribution or reproduction is permitted which does not comply with these terms.
*Correspondence: Elisabeth Solana, ZWxpc2FiZXRoLnNvbGFuYUBpZGliYXBzLm9yZw==; Sara Llufriu, c2xsdWZyaXVAY2xpbmljLmNhdA==
†These authors have contributed equally to this work and share first authorship
Disclaimer: All claims expressed in this article are solely those of the authors and do not necessarily represent those of their affiliated organizations, or those of the publisher, the editors and the reviewers. Any product that may be evaluated in this article or claim that may be made by its manufacturer is not guaranteed or endorsed by the publisher.
Research integrity at Frontiers
Learn more about the work of our research integrity team to safeguard the quality of each article we publish.