- 1Institut du Cerveau et de la Moelle épinière–ICM, INSERM U 1127, CNRS UMR 7225, Sorbonne Université, UPMC Univ Paris 06, UMRS 1127, CNRS UMR 7225, Paris, France
- 2ICM, “Movement Investigations and Therapeutics” Team (MOV'IT), Paris, France
- 3ICM, Centre de NeuroImagerie de Recherche–CENIR, Paris, France
- 4Service de Neuroradiologie, Hôpital Pitié-Salpêtrière, APHP, Paris, France
- 5Dynamics and Pathophysiology of Neuronal Networks Team, Center for Interdisciplinary Research in Biology, Collège de France, CNRS UMR7241/INSERM U1050, MemoLife Labex, Paris, France
- 6Department of Neurology, Avicenne University Hospital, Sorbonne Paris Nord University, Bobigny, France
- 7Département des Maladies du Système Nerveux, Hôpital Pitié-Salpêtrière, APHP, Paris, France
The diagnosis of Parkinson's disease and atypical Parkinsonism remains clinically difficult, especially at the early stage of the disease, since there is a significant overlap of symptoms. Multimodal MRI has significantly improved diagnostic accuracy and understanding of the pathophysiology of Parkinsonian disorders. Structural and quantitative MRI sequences provide biomarkers sensitive to different tissue properties that detect abnormalities specific to each disease and contribute to the diagnosis. Machine learning techniques using these MRI biomarkers can effectively differentiate atypical Parkinsonian syndromes. Such approaches could be implemented in a clinical environment and improve the management of Parkinsonian patients. This review presents different structural and quantitative MRI techniques, their contribution to the differential diagnosis of atypical Parkinsonian disorders and their interest for individual-level diagnosis.
Introduction
Parkinsonism is defined by the presence of resting tremor, rigidity, bradykinesia, and postural instability. Parkinson's disease (PD) is the most common neurodegenerative cause of Parkinsonism. Atypical Parkinsonism refers to other neurodegenerative disorders that commonly include progressive supranuclear palsy (PSP), corticobasal degeneration, multiple system atrophy (MSA), with its cerebellar (MSA-C) and Parkinsonian (MSA-P) variants, and dementia with Lewy body (1–6). Degeneration of the substantia nigra (SN) is the pathological hallmark of neurodegenerative Parkinsonian disorders (1, 3–7). Neuroimaging plays a major role in the diagnosis of Parkinsonian disorders and the differentiation of PD from atypical Parkinsonism. Magnetic resonance imaging (MRI) improves diagnostic accuracy, reduces the rate of misdiagnosis, facilitates early diagnosis, and may be useful for the follow-up of disease progression (8–11). Usually, the diagnosis of sporadic PD does not require an MRI examination when the clinical presentation is typical. In contrast, MRI is needed when the clinical presentation is atypical, that is, in the presence of symptoms called “red flags,” such as rapid progression of gait impairment, early and recurrent falls and impaired balance, early bulbar or inspiratory respiratory dysfunction, and early and severe autonomic dysfunction (12). In this case, MRI shows a number of features that can help the clinician to distinguish PD from atypical Parkinsonism. This review details the main MRI characteristics of PD and the two main causes of atypical Parkinsonism, PSP and MSA, focusing on the features that can be used in clinical practice.
MRI Techniques and Biomarkers
MRI provides in vivo biomarkers that inform about the underlying neurodegenerative processes. Regional brain atrophy is detected using T1-weighted three-dimensional (3D) sequences and reflects neuronal loss. Diffusion anomalies reflect the presence of microstructural alterations in the tissues, while iron-sensitive imaging detects the presence of iron deposits. Multimodal MRI is defined as the combination of information provided by these different sequences. Brain abnormalities can be assessed in several ways: (i) qualitatively, by visual inspection of regional brain atrophy and signal changes using conventional structural MRI, or (ii) quantitatively, by measurements of changes in volumes, diffusion metrics and iron-related signals (8–10, 13, 14). The main MRI techniques and their respective contributions are summarized in Table 1. The usefulness of neuroimaging biomarkers can be assessed by a five-level scale, as recently proposed for PSP (9). Level 1 defines biomarkers useful at the group level when comparing a specific disease with healthy subjects or other clinically overlapping diseases. Level 2 defines a biomarker useful at the individual level because it reaches a sensitivity and specificity >80% for the clinical diagnosis of a given patient. Level 3 indicates that the biomarker is effective for early clinical diagnosis, when patients present with mild or non-specific symptoms but do not yet meet the clinical criteria for the disease. Level 4 biomarkers are strongly correlated with pathology and could be used as surrogate criteria for pathological diagnosis. Level 5 biomarkers provide a direct measure of the underlying neuropathological changes (9). Here, we detail the different biomarkers in the three diseases in light of this scale.
Imaging Findings in Parkinsonian Disorders
Parkinson's Disease
Parkinson's disease is an alpha-synucleinopathy, the main neuropathological characteristic of which is the neurodegeneration of the dopaminergic neurons of the substantia nigra pars compacta (SNpc) (1–3, 15, 16). In addition to SN degeneration, several other nuclei in the brainstem, basal forebrain and cortex are affected in PD in later stages, which helps to explain the presence of non-motor symptoms (15, 16). However, conventional MRI most often does not reveal specific abnormalities in PD outside the SN, and the basal ganglia are normal or only show subtle changes in terms of volume, diffusion measurements or iron deposition (8, 17–19). Lesions of the small brainstem nuclei, basal forebrain and cortex are mostly detected using specific quantitative approaches that are not used in clinical practice except for the locus coeruleus (18).
Conventional and Structural Imaging
Substantia nigra
In clinical practice, degeneration of the SNpc can be detected by visual inspection of the images using neuromelanin-sensitive and iron-sensitive imaging. These nigral changes differentiate neurodegenerative Parkinsonism from essential tremor and other non-degenerative Parkinsonian syndromes (20). Neuromelanin-sensitive imaging relies on the T1 shortening effect of neuromelanin, a pigment contained in the SNpc, that has paramagnetic properties when it is bound to iron (17, 21, 22). A direct correlation between the signal intensity of post-mortem samples of SNpc on neuromelanin-sensitive sequences and the density of neuromelanin-containing neurons has been demonstrated (23). Using neuromelanin-sensitive imaging, the SNpc appears as an area of high signal intensity in healthy subjects. Patients with degenerative Parkinsonian syndromes show a reduction in the size and signal intensity of the SNpc, reflecting the loss of dopaminergic neurons (11, 24, 25) (Figure 1). The sensitivity and specificity of this technique are above 80%, and the performances between the visual assessment of trained radiologists and the quantification of volume and signal intensity are similar (26). In line with neuropathological findings (7), greater involvement of the posterolateral part of the SNpc with relative preservation of the medial part has been shown in patients with PD (27). A neuromelanin signal decrease has also been observed in patients with idiopathic rapid eye movement sleep behavior disorder (RBD) (28). RBD is a frequent non-motor feature of PD characterized by abnormal behavior and increased muscle tone during rapid eye movement sleep (29, 30). Patients with RBD have a high risk of developing Parkinsonism, including PD, dementia with Lewy bodies and, more rarely, MSA, with a rate of conversion of 33.5% within 5 years and 82.4% at 10 years, as reported by a recent meta-analysis (31). Idiopathic RBD is therefore considered a prodromal phase of Parkinsonism and PD, with an estimated period of 10–15 years of progressive neuronal loss before the onset of the main motor symptoms (31–33).
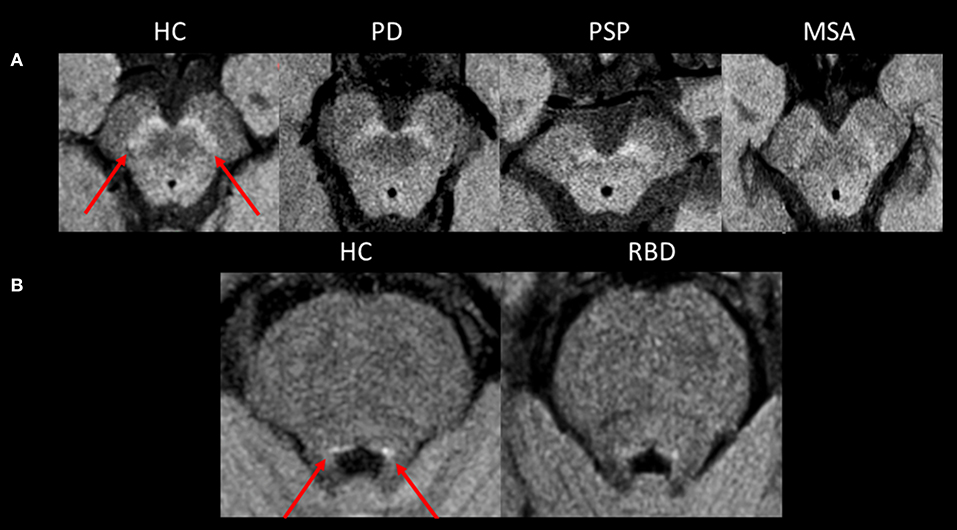
Figure 1. Neuromelanin imaging. (A) Axial neuromelanin-sensitive images at 3 Tesla passing through the midbrain at the level of the substantia nigra pars compacta in a healthy control and patients with PD, PSP, and MSA. The substantia nigra pars compacta is seen as an area of high signal in a healthy control (arrows). Its size and signal are decreased in neurodegenerative Parkinsonian syndromes. (B) Axial neuromelanin-sensitive images at 3 Tesla passing through the midbrain at the level of the coeruleus/subcoeruleus complex. The signal within the coeruleus/subcoeruleus complex is decreased in the patient with RBD compared to the healthy control. HC, healthy control; MSA, multiple system atrophy; PD, Parkinson's disease; PSP, progressive supranuclear palsy; RBD, rapid-eye movement sleep behavior disorder.
Using susceptibility-weighted imaging (SWI), healthy subjects show an area of normal high signal intensity located at the dorsolateral part of the SNpc, a feature also known as the “swallow tail sign” or the “dorsolateral nigral hyperintensity” (DNH) sign (34). Histopathological correlations have shown that the DNH corresponds to nigrosome 1, a region where dopaminergic neurons are affected early and severely (35) (Figure 2). The loss of this hyperintensity in Parkinsonian subjects is probably due to the presence of iron deposits in Parkinsonian subjects (17, 22). The absence of DNH was shown to be predictive for ipsilateral dopamine transporter (DAT) deficiency on radiotracer imaging, with high sensitivity and specificity (87.5 and 83.6%), supporting its potential as a marker of SN pathology (36). This DNH sign has a sensitivity between 79 and 100% and a specificity between 85 and 100% for the differentiation of degenerative Parkinsonian syndromes from healthy subjects (34, 36, 37). The DNH sign is also an early marker observed in two-thirds of idiopathic RBD patients (38).
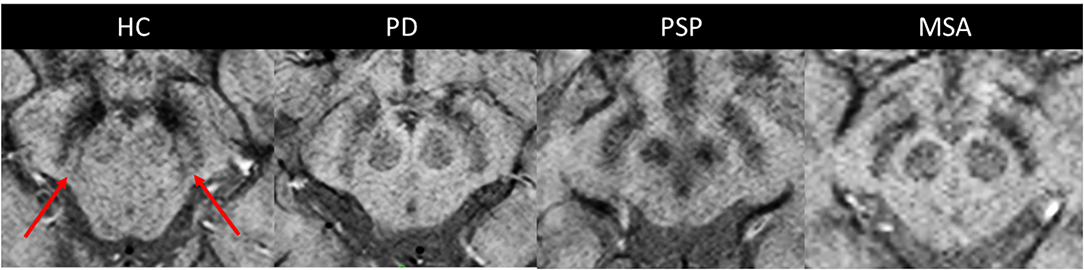
Figure 2. The dorsolateral nigral hyperintensity. Axial susceptibility-weighted images at 3 Tesla passing through the midbrain at the level of the substantia nigra pars compacta in a healthy control and patients with PD, PSP, and MSA. The dorsolateral nigral hyperintensity is well-depicted at the dorsolateral part of the substantia nigra pars compacta in a healthy control (arrows). It is lost in neurodegenerative parkinsonian syndromes. HC, healthy control; MSA, multiple system atrophy; PD, Parkinson's disease; PSP, progressive supranuclear palsy.
Locus coeruleus/subcoeruleus complex
The locus coeruleus/subcoeruleus complex is composed of catecholaminergic neurons containing neuromelanin and is affected in approximately two-thirds of PD patients (39, 40). Using neuromelanin-sensitive imaging, the signal is decreased within the coeruleus/subcoeruleus complex of these patients. The involvement of this nucleus has been associated with the presence of RBD in PD patients and with idiopathic RBD (40, 41). The relationship between RBD and signal changes in this complex suggests that RBD in PD may be related to damage of the subcoeruleus part of the complex that is involved in the control of movement during REM sleep (29, 30, 40).
Brainstem
Structural brain imaging using conventional MRI with visual assessment of T2- and T1-weighted sequences is usually normal in patients with PD. Its main role is to detect or exclude secondary causes of Parkinsonism (such as vascular encephalopathy, demyelinating lesions, tumors or normal pressure hydrocephalus) and to look for signs of atypical Parkinsonism (10, 13, 19, 42). Manual morphometric measurements of the brainstem, including the anteroposterior midbrain diameter (43, 44), the midsagittal midbrain area, the midbrain to pons area ratio (43, 45) and the magnetic resonance Parkinsonism index (MRPI), are normal in PD (45, 46). Atrophy rates of the brainstem are not different from those of healthy subjects (47).
Other areas
Overall, except for the SN, the basal ganglia are mostly spared in PD patients (48, 49). In the cortex, no or mild changes have been reported in early PD (50). Conversely, impaired cognition and dementia in PD have been associated with marked cortical atrophy in many brain regions, including the frontal, parietal, and temporal areas, and the substantia innominata (50–52). Cortical atrophy accelerates with disease progression and worsening of cognitive decline (50). Longitudinal MRI studies have reported a higher rate of cortical thinning in PD patients with mild cognitive impairment than in PD patients with normal cognition and healthy subjects, which is correlated with cognitive decline (50, 51).
Diffusion Imaging
In the SN, changes in diffusion metrics have been reported at the group level. Using diffusion tensor imaging (DTI), reduced fractional anisotropy (FA) (26, 53) and increased diffusivity (26) values were evidenced in the SN in PD patients. However, there is a large variability of results across studies, and these measures do not appear useful at the individual level (54). Using DTI, two meta-analyses reported either frontal predominance of extranigral FA changes (55) or more widespread FA and mean diffusivity (MD) changes involving the corpus callosum and cingulate and temporal cortices in PD patients (56). Most studies did not detect any significant changes using global region-of-interest analyses in the putamen (57–61), midbrain (62, 63), pons (57–61, 64) and cerebellum (65). Free water is a more advanced diffusion metric derived from a bi-tensor diffusion model that shows better sensitivity and reliability for the discrimination of PD from healthy subjects. Increased free water values have only been observed in the SN in PD (66, 67), while no changes were found in other brain regions (66). In contrast, using region-of-interest measurements, diffusivity changes were measured in specific regions that are affected in PD, including the pedunculopontine nucleus in relation to gait disorders (68), the locus coeruleus for RBD (40), the medulla oblongata for autonomic dysfunction (69), and basal forebrain for cognitive decline (70).
Iron Imaging
Using R2* relaxometry (71, 72) or quantitative susceptibility mapping (QSM) (71, 73, 74), an increase in iron deposition was detected in the SN, with QSM showing greater accuracy than R2* relaxometry (71, 75, 76). Outside the SN, R2*, and susceptibility values were mostly normal in the putamen (61, 73, 74, 77). However, an increase in susceptibility values was reported in the red nucleus and globus pallidus of patients with late-stage PD (73).
Summary
The neuromelanin signal decrease within the SNpc is an early marker of Parkinsonism, useful at the individual level, meeting the criteria for a level 3 biomarker. Neuromelanin signal also correlates with the reduction of dopaminergic neurons in the SN and may be a surrogate marker of dopaminergic neuron degeneration (level 4). The DNH sign is also a level 3 biomarker and may hold potential for being a level 4 biomarker, although a direct correlation with the SN degeneration has not yet been evidenced. Neuromelanin signal decrease within the coeruleus/subcoeruleus complex may also be used as an early biomarker of Parkinsonism at the individual level (level 3). Free water changes appear useful at the individual level (level 2) although their correlation with neurodegenerative changes remains to be determined. Their use in clinical practice would require normative values that are currently not available in Radiology departments. Other findings were mostly shown at the group-level (level 1), with no demonstrable clinical relevance to date.
Progressive Supranuclear Palsy
Progressive supranuclear palsy (PSP) is a tauopathy characterized by the presence of intracerebral depositions of Tau protein and neurodegeneration predominant in the brainstem, basal ganglia and cerebellar nuclei (3, 5, 6, 78, 79). PSP has a heterogeneous clinical expression. The most common clinical presentation of PSP is Richardson's syndrome (PSP-RS), which is characterized by early axial rigidity, postural instability, falls and vertical supranuclear gaze palsy (5, 79, 80). There are a number of other clinical phenotypes of PSP, including PSP with predominant Parkinsonism (PSP-P), progressive gait freezing (PSP-PGF), postural instability (PSP-PI), frontal presentation (PSP-F), speech/language disorder (PSP-SL), ocular motor dysfunction and corticobasal syndrome (PSP-CBS) (5, 79, 80). Imaging patterns in PSP variants are not clearly understood since most studies have focused on PSP-RS and/or did not differentiate PSP variants.
Conventional and Structural Imaging
Substantia nigra
As in PD, PSP patients also show a reduction in the neuromelanin signal and volume of the SNpc (27, 81, 82) and a loss of the DNH sign (37). Neuropathological studies have reported a different pattern of nigral degeneration from PD without predilection for the lateral part (7). Only one study using neuromelanin-sensitive imaging reported a difference between signal changes in PD and PSP (27), but this requires further investigation.
Locus coeruleus/subcoeruleus complex
The coeruleus/subcoeruleus complex is also involved in PSP (3, 5, 6). In line with histological studies, decreased neuromelanin signal of the coeruleus/subcoeruleus complex has been reported in PSP (18), but other studies did not find such changes (27).
Brainstem
Patients with PSP-RS typically exhibit marked midbrain atrophy. The “hummingbird” sign (83), less frequently called the “penguin” sign (84), describes the flat or concave aspect of the midbrain tegmentum visible on midline T1-weighted sagittal sections (Figures 3, 4). The “morning glory” sign corresponds to the concave aspect of the lateral margin of the midbrain tegmentum on axial slices, which is also observed in PSP-RS (86). Although these features are suggestive of PSP-RS with a specificity of 99.5% for the “hummingbird sign” and 97.7% for the “morning glory sign,” they show low sensitivity (51.6 and 36.8%, respectively) (87) (Table 2).
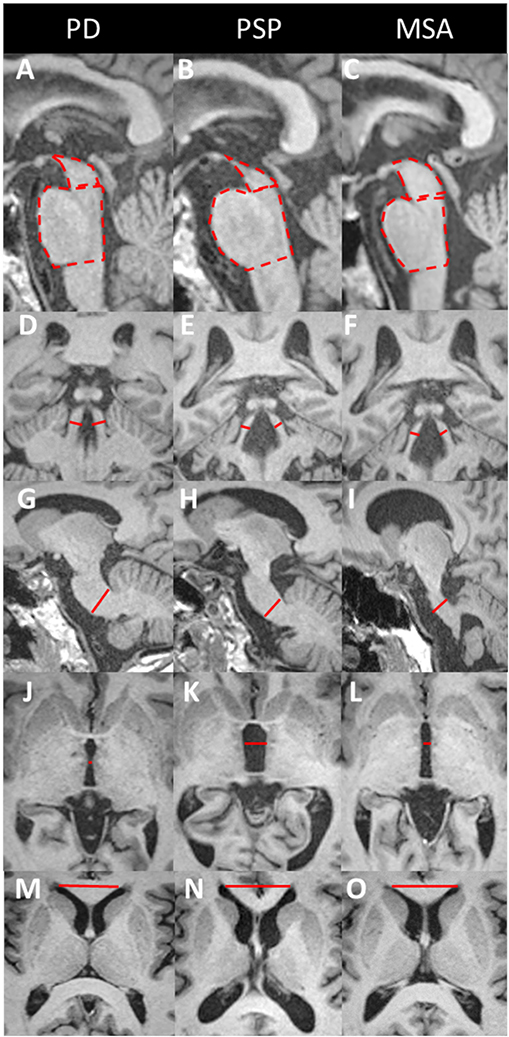
Figure 3. Midbrain to pons area ratio, Magnetic resonance Parkinsonism index (MRPI) and MRPI 2.0. Three-dimensional T1-weighted images in patients with PD (left), PSP (middle), and MSA (right). The MRPI combines measurements of the midsagittal surfaces of the midbrain (dashed line) and pons (dashed line) (A–C) and widths of the superior (red lines, D–F) and middle (red line, G–I) cerebellar peduncles [MRPI = (pons/midbrain) × (middle cerebellar peduncle/superior cerebellar peduncle)]. The limit between the midbrain and pons is defined by the line passing through the inferior border of the inferior colliculus and the pontomesencephalic sulcus. The inferior border of the pons is parallel to the previous one. The widths of the superior (D–F) and middle cerebellar peduncles (G–I) are measured in the parasagittal and coronal planes that best show the peduncles, respectively. The MRPI 2.0 is the product of the MRPI by the ratio of the width of the third ventricle (red line, J–L) to the largest left-to right width of the frontal horns of the lateral ventricles (red line, M–O) on axial views. For further details about the measurement technique [please refer to Quattrone et al. (46, 85)]. PSP patients show atrophy of the midbrain (B) and superior cerebellar peduncles (E) and enlargement of the third ventricle (K). In MSA patients, the atrophy is greater in the pons (C) and middle cerebellar peduncles (I), with an enlarged fourth ventricle. PD patients do not show any significant brainstem atrophy or MRPI changes. MSA, multiple system atrophy; PD, Parkinson's disease; PSP, progressive supranuclear palsy.
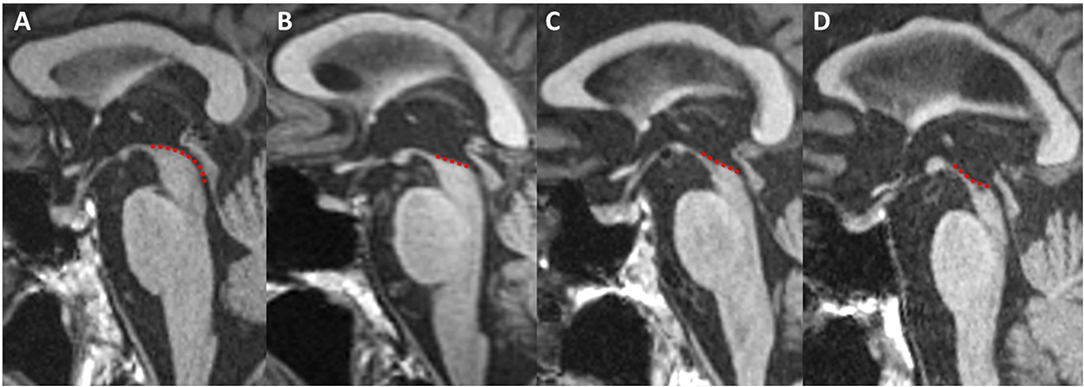
Figure 4. Midbrain atrophy in PSP patients. Midsagittal T1-weighted images in a healthy control (A) and PSP patients with increasing midbrain atrophy (B–D). Healthy subjects and PD patients show a normal convex profile of the midbrain superior surface. PSP patients present a flat (mild) or concave (moderate to severe) aspect (dashed line). This shape is known as the “penguin” or “hummingbird” sign. HC, healthy control; PD, Parkinson's disease; PSP, progressive supranuclear palsy.
Several manual morphometric indexes have been proposed as clinical biomarkers usable in individual patients. The anteroposterior midbrain diameter has limited discriminating power with a significant overlap of individual values (9, 44). The midbrain midsagittal area discriminate PSP-RS from healthy subjects and PD patients (<122 mm2) with sensitivity and specificity above 80% and from MSA patients (<114 mm2) with a lower sensitivity (45). The midbrain to pons midsagittal surface ratio (<0.18) has a sensitivity and specificity >90% in differentiating PSP-RS from healthy subjects, PD and MSA-P patients (43, 46), although some studies have found lower accuracies (45).
The superior cerebellar peduncles (SCPs) are atrophic in PSP, while the middle cerebellar peduncles (MCPs) are relatively spared, resulting in an increase in the MRPI in PSP. Compared to the midbrain to pons ratio, the MRPI showed better performance for the differentiation of PSP-RS from PD (when > 13.6) and from MSA-P (when > 12.9), with very good (43) to excellent sensitivity and specificity (46, 95, 96) (Figure 3, Table 2). Manual and automated approaches have shown similar performance for measuring the MRPI (96). A recent study has validated the use of a web platform providing an automated calculation of the MRPI, suggesting that the approach could be applicable in clinical practice (97). Automated MRPI values showed high performance in differentiating PSP-RS and PSP-P patients from non-PSP participants (93.6 and 86.5% accuracy, respectively). Differentiation was also good at the early stage of the disease (90.1 and 85.9%, respectively) (97). More recently, the MRPI 2.0 has been introduced to improve the discrimination of PSP-P from PD over that of the MRPI (sensitivity: 100% for the MRPI 2.0 vs. 73.5% for the MRPI) (85). Abnormal MRPI and MRPI 2.0 values also appeared to be early features of PSP-P (98) (Figure 3, Table 2).
In line with studies based on morphometric measurements, group-level studies using voxel-by-voxel analyses (49, 94, 99) or automated segmentation software, such as Freesurfer (48, 100) have reported volume reductions in the brainstem, including the midbrain and superior cerebellar peduncles, in PSP-RS.
Longitudinal studies have shown that the rates of midbrain atrophy in PSP (2.2 ± 1.5% per year) were seven times greater than those in healthy subjects (47). Furthermore, motor deficit severity correlates with midbrain atrophy (47). Similarly, the rate of progression of brainstem atrophy measured by the MRPI and MRPI 2.0 over 1- and 2-years intervals was higher in PSP patients than in PD patients (101). The progression rate was greater in PSP-RS patients than in PSP-P patients (101).
There is evidence that midbrain atrophy is a marker of PSP-RS phenotype rather than PSP pathology, as suggested by the absence of midbrain atrophy in patients with autopsy-proven PSP without clinical presentation of PSP-RS (9, 102). Other studies have also found midbrain atrophy in PSP variants, including PSP-P (9, 46, 98, 103), PSP-SL (102, 104, 105), and PSP-F (9, 102, 106), although it is typically less severe in these variants than in PSP-RS (9, 103, 107).
Other regions
In PSP-RS, among the basal ganglia, the volumes of the putamen and globus pallidus are smaller than those in PD, and the volume of the thalamus is smaller than that in MSA-P (8, 9, 48, 62). The usefulness of these findings at the individual level remains to be determined (9). Cortical atrophy with preferential frontal involvement has been demonstrated in PSP-RS patients but not in healthy subjects, PD and MSA patients at the group level (18, 93, 94). Frontal atrophy also occurs in PSP variants, particularly in PSP-F (9, 106), PSP-SL (9, 108), and PSP-CBS (9, 109), and to a greater extent than that of PSP-RS, probably reflecting different pathological loads between the brainstem and the cortex (9). The rate of frontal atrophy in PSP was shown to be three times that of healthy subjects and twice that of PD (47). Executive dysfunction correlated with increased rates of frontal atrophy in PSP (47).
Diffusion Imaging
In the SN, decreased FA (18) and increased free water (66) have been reported at the group level in PSP. Diffusion-weighted imaging (DWI) studies using manual measurements of apparent diffusion coefficient (ADC) and DTI studies in PSP-RS patients have demonstrated increased diffusivity values in the putamen, the caudate nucleus and the globus pallidus compared with those in PD patients and healthy subjects (58, 63, 92, 110, 111), as well as in the thalamus (58), the midbrain (18, 63, 110), the SCP (90, 110, 112), and the precentral and prefrontal white matter (58, 110) compared with those in PD, MSA and healthy subjects (58, 110, 111). In the SCP, ADC values were higher in PSP-RS than in healthy subjects and PD patients with a sensitivity of 90–100% and a specificity of 85–100% (90, 111) and in MSA-P patients with a sensitivity of 96.4% and a specificity of 93.3% (90), supporting the use of this measurement as a level 2 biomarker (9). Putamen diffusivity values were higher in PSP than in PD but overlapped between PSP and MSA-P (57, 90, 92, 113). Diffusivity in the thalamus was greater in PSP patients than in MSA patients (62) (Table 2). Reduced FA has been reported in the midbrain, pons, SCP, thalamus, cerebellar white and gray matter, dentate nucleus, corpus callosum, the precentral, superior frontal and parieto-occipital gray matter and the precentral white matter of PSP patients compared to healthy subjects and PD patients (18). In line with these results, free-water measurements were increased in the basal ganglia, midbrain, thalamus, dentate nucleus, cerebellar peduncles, cerebellar vermis and lobules V and VI, and corpus callosum in PSP patients compared with those in PD patients and healthy subjects (66). Free water-corrected fractional anisotropy values were increased in the putamen, caudate, thalamus, and vermis and decreased in the superior cerebellar peduncle and corpus callosum (66).
Tractography studies have shown more specific involvement of the SCP, corpus callosum and superior longitudinal fasciculus in PSP than in PD and MSA-P (9).
Iron-Sensitive Imaging
PSP patients show increased iron deposition in many regions. R2* relaxation rates were increased in the putamen, caudate nucleus and globus pallidus compared to those in PD patients and healthy subjects (77, 114). R2* and QSM values in the putamen overlapped between PSP and MSA (74, 113). Using QSM, PSP patients had higher susceptibilities in the basal ganglia and thalamus than healthy subjects and early-stage PD patients (115) and in the red nucleus than in MSA patients (74) (Table 2).
Other Biomarkers
Other techniques have been investigated in a research setting, with differences only being observed at the group level. Compared to that in healthy subjects, the magnetization transfer ratio in PSP patients was reduced in the globus pallidus, putamen, caudate nucleus, SN, and white matter, possibly reflecting changes in the degree of myelination or axonal density in the affected structures (116). Using spectroscopy, metabolic changes were observed in the basal ganglia and the frontal areas. Reduced N-acetylcholine (NAA)/creatine (Cr) or NAA/choline (Cho) ratios were reported in the lentiform nucleus (117–121), and a reduced NAA/Cr ratio was reported in the frontal cortex (117). Using resting-state fMRI, PSP patients have shown connectivity disruptions between the dorsal midbrain tegmentum and subcortical and cortical networks, including those involving the cerebellar, diencephalic, basal ganglia, and cortical regions (122), and between the thalamus and the striatum, supplementary motor area and cerebellum (123). These findings help to understand the pathophysiology of Parkinsonism but are of little use in discriminating diseases.
Summary
Measurements of midbrain atrophy including the midbrain to pons ratio, the MRPI and the MRPI 2.0 are the most robust biomarkers for clinical diagnosis of PSP-RS at the individual level (level 2). Frontal atrophy in addition to midbrain atrophy has also been reported as a level 2 biomarker although the degree of evidence appears lower. Further, the midbrain to pons ratio, the MRPI and the MRPI 2.0 have proven to be useful for the early diagnosis of PSP-RS (level 3), but their relevance at the prodromal stage of the disease remains unclear. Increased MRPI and the MRPI 2.0 also appear to be early features in PSP-P (98). Relevant biomarkers have not yet been validated for other PSP variants. Diffusivity in the SCP has shown accuracies above 90% for the differentiation of PSP-RS from PD and MSA, suggesting its clinical utility as a level 2 biomarker. However, normative values in healthy subjects and cut-off values still need to be defined.
Multiple System Atrophy
Multiple system atrophy (MSA) is a synucleinopathy characterized by the accumulation of alpha-synuclein within glial cytoplasmic inclusions (3, 4, 78). MSA patients typically present with autonomic dysfunction with a variable degree of Parkinsonism, more predominantly in the Parkinsonian variant (MSA-P), and with cerebellar signs, more predominantly in the cerebellar variant (MSA-C). MSA-P is mainly associated with changes in the putamen, whereas MSA-C shows prominent involvement of the cerebellum, pons and MCP (3, 4, 8, 78). Striatonigral degeneration and olivopontocerebellar atrophy often overlap in MSA patients (3, 4), and a clinical and brain imaging continuum exists between both variants as the disease evolves (3, 4, 78, 124).
Conventional and Structural Imaging
Substantia nigra
Nigral abnormalities can be depicted using neuromelanin-sensitive imaging (27, 81, 82) and SWI (37). Neuropathological descriptions and neuromelanin-based imaging studies have shown a similar pattern of nigral degeneration between MSA-P and PD, with a greater involvement of the lateral part of the SNpc (7, 27).
Locus coeruleus/subcoeruleus complex
The locus coeruleus/subcoeruleus complex may be involved in MSA (3), resulting in signal loss on neuromelanin-sensitive imaging (27) that is less severe than that in PD patients (3, 27).
Basal ganglia, brainstem and cerebellum
In MSA-P, the putamen is atrophic, with a characteristic flattening of its lateral border, hypointense on T1-weighted gradient echo images, and hypointense in SWI due to the presence of iron deposits (125) (Figure 5). This hypointensity is surrounded by a rim of hyperintensity at its dorsolateral margin on proton density- and T2-weighted images (126) (Figure 5). In line with the underlying neuropathology (3), these signal changes are typically more prominent on the posterior part of the putamen (125). A hyperintense putaminal rim may occasionally be seen in PD patients and healthy subjects (3, 87, 95, 97). Signal abnormalities are influenced by the sequence parameters and the magnetic field strength of the MRI scanner (112). Hypointensity in the putamen have been shown to be greater using SWI than T2*- and T2-weighted images and increased with field strength (127). Therefore, overall sensitivity values are extremely variable across studies, ranging from 38 to 100% for T2 hypointensity in the putamen and from 56 to 90% for the hyperintense dorsolateral rim, whereas specificity values varied between 87 and 100% in distinguishing MSA-P patients from PD or healthy subjects (125) (Table 3).
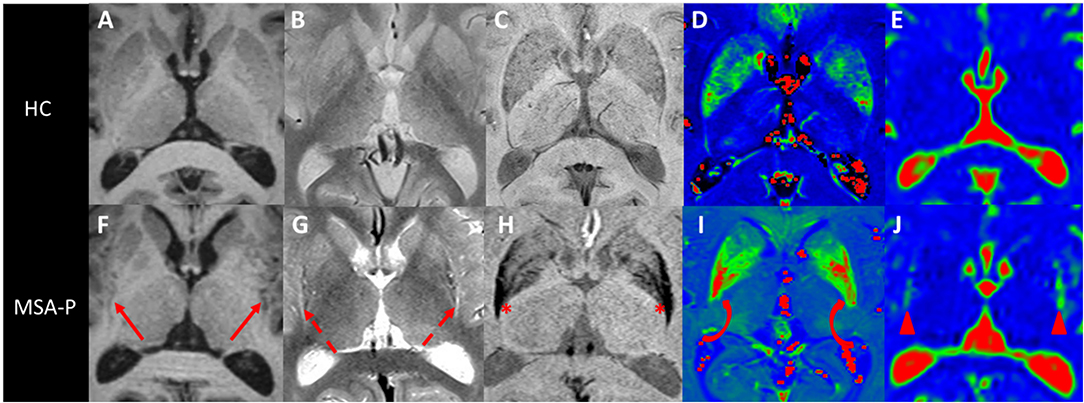
Figure 5. Putaminal abnormalities in MSA-P. Axial T1 (A,F), proton density (B,G), and susceptibility (C,H) weighted images and R2* (D,I) and ADC (E,J) maps passing through the putamen in a healthy control (upper row) and a patient with MSA-P (lower row). The posterior putamen are atrophic (F, arrows), showing a lateral hyperintense rim (G, dashed arrows), a hypointensity on susceptibility-weighted images (H, *) associated with increased R2* values (I, curved arrows), reflecting elevated iron depositions, and increased ADC values (J, arrowheads). ADC, apparent diffusion coefficient; HC, healthy control; MSA-P, Parkinsonian variant of multiple system atrophy; R2*, T2* relaxation rate.
In MSA-C, the pons is atrophic, showing areas of increased signal intensity on proton density-weighted images affecting the transverse pontine fibers with the shape of a cross, well-known as the “hot cross bun” sign (126) (Figure 6). This sign has limited specificity, as it can be encountered in other pathologies, such as spinocerebellar ataxia (128). The MCPs present with atrophy and T2 hyperintensity, and the cerebellum is atrophic (8) (Table 3).
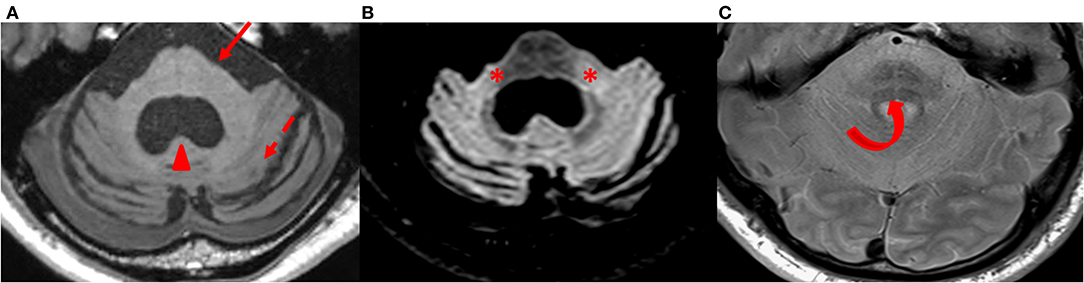
Figure 6. Findings in MSA-C patients. Axial T1-weighted (A), FLAIR (B), and proton density-weighted (C) images at the level of the pons and cerebellum in a patient with MSA-C. The pons (arrow) and the cerebellum (dashed arrow) are atrophic with an enlarged fourth ventricle (arrowhead) (A). Middle cerebellar peduncles are hyperintense on FLAIR images (*) (B). A “hot cross bun sign” is well visible in the pons (curved arrow) (C). MSA-C, cerebellar variant of multiple system atrophy.
Regional brain atrophy can be quantified. While PSP patients have greater midbrain and SCP atrophy, MSA-C patients have predominant atrophy in the pons and MCPs, resulting in increased midbrain to pons ratio and decreased MRPI, with sensitivity and specificity equal to 95% and 100% for the categorization of MSA-P and PSP patients, respectively (46). Therefore, the midbrain to pons ratio and the MRPI could be suggested as level 2 biomarkers.
In MSA-P, atrophy rates have been shown to be greatest in the pons, equal to 4.5 ± 3.2% per year, over 20 times that in healthy subjects and three times the rate of pontine atrophy in PSP. Atrophy rates in the cerebellum (3.2 ± 1.9% per year) were more than ten times higher than those of healthy subjects and twice that of PSP (47). The severity of motor deficit correlated with ponto-cerebellar atrophy in MSA-P (47).
Diffusion
In MSA-P, diffusivity of the posterior putamen is increased, which is visible by simple inspection of ADC maps and can also be quantified (Figure 5). DWI studies have consistently reported an increase in ADC and Trace(D) values in the putamen in MSA-P compared to healthy subjects and PD patients (57–61, 90, 92, 129, 130), with an overall sensitivity of 90% and specificity of 93% in discriminating MSA-P from PD patients in a recent meta-analysis (57). These diffusion abnormalities were greater in the posterior putamen (59, 63, 129). UPDRS motor scores correlated positively with Trace(D) values in both the entire and posterior putamen in MSA-P patients (60). However, the ability of diffusivity measurements in the putamen to distinguish MSA and PSP is debated. Some studies have reported reliably higher ADC values in MSA-P than PSP (58, 111), while others have described a significant overlap of values, which would limit the interest for discriminating individual patients (57, 58, 129).
Other diffusion abnormalities in MSA-P involve the MCPs, the pons and the cerebellum (58–61, 64, 90–92, 130), reflecting a certain degree of olivopontocerebellar changes in addition to striatonigral degeneration (58). Increased ADC values in the MCPs allow complete differentiation of MSA-P from PD and PSP patients with 100% sensitivity and specificity (58). FA values are decreased in the MCP and pons (64). Diffusivity in the putamen and the MCP could therefore improve the categorization between MSA-P and MP on an individual basis. Increased diffusivity was also observed in the thalamus of MSA-P patients (58–61, 90–92) but to a lesser extent compared to PSP patients (62) (Table 3).
In MSA-C, the MCPs and cerebellum are more affected and the putamen is less affected than in MSA-P (8, 131, 132). Mean diffusivity in the cerebellum seems to be a robust discriminating marker, allowing the differentiation of MSA-P and MSA-C from PD and PSP-RS patients with a 100% positive predictive value (65) (Table 3).
Increased disease duration correlated with increased Trace(D) values in the pons of MSA-P patients and in the cerebellum and MCPs of MSA-C patients (64, 91).
Iron-Sensitive Imaging
MSA patients have greater iron deposits in the putamen than healthy subjects and PD patients (115), with greater involvement of the posterolateral part of the putamen (115, 133) (Figure 5). Increased R2* values in the putamen showed 78% sensitivity and 100% specificity for the discrimination of MSA-P patients from healthy subjects and PD patients (61, 77, 113). R2* values correlated positively with atrophy of the putamen (77). Similar differences are observed between MSA-C and PD (61). Using QSM, susceptibility values were also shown to be increased in MSA compared to PD (74). The combination of mean diffusivity and R2* measurements in the putamen improved the distinction between MSA-P and PD patients, resulting in an accuracy of 96% (61). Although the signal decrease due to the presence of iron deposits observed using SWI in the putamen is visually greater in MSA-P than PSP, there is a significant overlap of R2* and susceptibility values in the putamen between the two diseases (74, 113) (Table 3).
Other Biomarkers
The magnetization transfer ratio was reduced in the putamen of MSA patients (116). Using spectroscopy, decreased NAA/Cr and Cho/Cr ratios were reported in the lenticular nucleus in MSA-P compared to those in healthy subjects. The NAA/Cr ratio in the lenticular nucleus (118) and NAA/Cr in the cerebellum were decreased in MSA-C (134). The NAA/Cr ratio was decreased in the pons in both MSA types (135). Resting-state fMRI studies have shown connectivity abnormalities in the primary sensorimotor and premotor cortex and prefrontal, inferior parietal and occipital areas in MSA-C and MSA-P patients. Unlike in patients with PD, decreased or increased connectivity in different regions of the visual associative cortices and decreased connectivity in the right cerebellum were observed in MSA patients (136).
Summary
Although morphometric measurements, such as the midbrain to pons ratio and the MRPI were initially designed for PSP, these indices also allow differentiation MSA from PSP and PD. Thus, they could be used as clinical biomarkers for MSA (level 2). Other level 2 biomarkers include the characteristic shape of the putamen, T2* and SWI signal decrease and ADC increase in the posterior putamen in MSA-P, and atrophy of the pons, cerebellar peduncles and cerebellum with the cross-bun sign in MSA-C. The potential utility of these measurements for the early diagnosis has to be investigated. Putamen abnormalities assessed by quantitative MRI techniques contribute to the diagnosis of MSA-P as level 2 biomarkers, although there is a significant overlap of diffusivity and R2* values between PSP and MSA-P. Other potential clinical biomarkers include diffusivity in the MCP and the cerebellum.
Machine-Learning-Based Differentiation of Parkinsonian Disorders
Very promising results have been obtained by combining fully automated quantitative MRI analysis with machine learning approaches to discriminate between Parkinsonian syndromes (94, 100, 137). Machine learning algorithms first learn to classify individual patients into different diagnostic categories using training data sets and then are applied to a new test data set. The algorithm then classifies each new subject in one of the groups of patients (138). Diagnostic precision is usually assessed with accuracy or balanced accuracy, which takes into account the differences in the number of subjects between the groups (94). A large multicenter study (n = 464) including healthy subjects (n = 73) and patients with PD (204), PSP-RS (109), MSA-P (20), and MSA-C (59) using a support vector machine (SVM) trained with MRI morphometric data reported balanced accuracies above 80% for between-group classification (94). The volumes of the midbrain, basal ganglia, and cerebellar peduncles had the largest contribution to group differentiation (94). Another volumetric study including 110 Parkinsonian patients in the early to moderate stages of the disease (40 PD, 40 MSA, 30 PSP) using measurements in 22 subcortical regions showed an accuracy of 97.4% for the discrimination of PD from to MSA and PSP (100). In contrast, the diagnostic accuracy using validated clinical consensus criteria obtained at the time of MRI acquisition was only 62.9%. The midbrain, putamen and cerebellar gray matter volumes were the most significant brain regions involved in this classification (100).
Combining volumetric measures with diffusion or iron measures could improve categorization. In one study, the combination of volumetry and DTI metrics allowed 100% accuracy in the discrimination of PD and PSP patients using SVM (99). Adding R2* values to volumetry and DTI metrics resulted in 95% accuracy for the classification of PD, MSA-C and MSA-P patients in another study (139). Machine learning approaches appeared also applicable to data from different clinical departments and MRI scanners. A recent multicenter study used free water and free water-corrected fractional anisotropy measurements as input for a linear SVM algorithm to differentiate between Parkinsonian syndromes (140). This automated pipeline was referred to as automated imaging differentiation in Parkinsonism (AID-P). The study included the largest cohort of subjects to date (n = 1,002) collected from 17 international sites using MRI scanners from different vendors. Three models were tested, which included diffusion imaging measurements from several brain regions, scores from the Movement Disorders Society Unified Parkinson's Disease Rating Scale part III (MDS-UPDRS III), and both diffusion imaging and MDS-UPDRS III. In the differentiation of PD from atypical Parkinsonism, diffusion imaging plus MDS-UPDRS III showed a significantly higher AUC (0.962) than the MDS-UPDRS III score alone (0.775), similar to that of diffusion imaging alone (0.955). Similarly, diffusion imaging plus MDS-UPDRS III (AUC 0·897) and diffusion imaging alone (AUC 0.926) significantly outperformed MDS-UPDRS III alone (AUC 0.582) in the differentiation of MSA from PSP. Harmonization of diffusion imaging data did not significantly improve the performance of machine learning models. These fully automated approaches promise to be highly generalizable (140). Further studies including PSP variants are needed, however. In addition, validation as part of the diagnostic assessment of clinical populations would make it possible to implement machine learning approaches in clinical practice.
Conclusion
Research using multimodal MRI in Parkinsonian syndromes has enabled the development of several in vivo biomarkers, some of which have demonstrated individual-level diagnostic utility. SNpc neurodegeneration in Parkinsonism is evidenced by a reduction in neuromelanin-sensitive signal and a loss of DNH in SWI. Similarly, the decrease in neuromelanin-sensitive signal in the coeruleus/subcoeruleus complex, which is associated with RBD, is an early marker of PD and MSA, and may be considered a surrogate biomarker for the degeneration of catecholaminergic neurons in this complex. However, to date, there is no definitive MRI biomarker of Parkinsonism. Overall, apart from SN changes and from the quantitative changes in specific regions of interest reported in group studies, conventional MRI used in clinical practice is generally normal in early PD patients. The midbrain to pons ratio and the MRPI are robust clinical biomarkers of PSP-RS, while abnormalities in the putamen (atrophy, flattening of the lateral border, T2, T2* and SWI signal changes, and increased diffusivity) and in the pons and cerebellum (atrophy and signal changes) strongly suggest the diagnosis of MSA-P and MSA-C, respectively. Diffusion imaging and R2* relaxometry allow accurate differentiation of groups, but the lack of normative and cut-off values, which vary with scanners, still hampers their use in clinical routine. New consensus criteria for the diagnosis of Parkinsonian syndromes incorporating MRI biomarkers should be considered in the future. Diagnostic accuracy could also benefit from machine learning approaches at a time when artificial intelligence promises to play a growing role in medicine.
Author Contributions
LC drafted the manuscript. NP, BD, and DG revised the manuscript. SL drafted and revised the manuscript.
Conflict of Interest
BD received research support grants from Fondation de France, Inserm, ANR; speech honoraria from Ipsen, Merz Pharma, Orkyn; and received travel funding from Merz Pharma, Elivie, Orkyn. SL received grants from Investissements d'avenir [grant numbers ANR-10-IAIHU-06 and ANR-11-INBS-0006] and Biogen Inc.
The remaining authors declare that the research was conducted in the absence of any commercial or financial relationships that could be construed as a potential conflict of interest.
Supplementary Material
The Supplementary Material for this article can be found online at: https://www.frontiersin.org/articles/10.3389/fneur.2020.00665/full#supplementary-material
References
1. Dickson DW. Neuropathology of Parkinson disease. Parkinsonism Relat Disord. (2018) 46:S30–3. doi: 10.1016/j.parkreldis.2017.07.033
2. Kalia LV, Lang AE. Parkinson's disease. Lancet. (2015) 386:896–912. doi: 10.1016/S0140-6736(14)61393-3
3. Dickson DW. Parkinson's disease and Parkinsonism: neuropathology. Cold Spring Harb Perspect Med. (2012) 2:a009258. doi: 10.1101/cshperspect.a009258
4. Castellani R. Multiple system atrophy. Am J Pathol. (1998) 153:671–6. doi: 10.1016/S0002-9440(10)65608-8
5. Steele JC, Richardson JC, Olszewski J. Progressive supranuclear palsy: a heterogeneous degeneration involving the brain stem, Basal Ganglia and cerebellum with vertical gaze and pseudobulbar palsy, nuchal dystonia and dementia. Semin Neurol. (2014) 34:129–50. doi: 10.1055/s-0034-1377058
6. Dickson DW, Rademakers R, Hutton ML. Progressive supranuclear palsy: pathology and genetics. Brain Pathol. (2007) 17:74–82. doi: 10.1111/j.1750-3639.2007.00054.x
7. Fearnley JM, Lees AJ. Ageing and Parkinson's disease: substantia nigra regional selectivity. Brain. (1991) 114 (Pt 5):2283–301. doi: 10.1093/brain/114.5.2283
8. Heim B, Krismer F, Seppi K. Structural imaging in Atypical Parkinsonism. Int Rev Neurobiol. (2018) 142:67–148. doi: 10.1016/bs.irn.2018.08.010
9. Whitwell JL, Höglinger GU, Antonini A, Bordelon Y, Boxer AL, Colosimo C, et al. Radiological biomarkers for diagnosis in PSP: where are we and where do we need to be? Mov Disord. (2017) 32:955–71. doi: 10.1002/mds.27038
10. Kassubek J. MRI-based neuroimaging: atypical Parkinsonisms and other movement disorders. Curr Opin Neurol. (2018) 31:425–30. doi: 10.1097/WCO.0000000000000578
11. Lehericy S, Vaillancourt DE, Seppi K, Monchi O, Rektorova I, Antonini A, et al. The role of high-field magnetic resonance imaging in Parkinsonian disorders: pushing the boundaries forward. Mov Disord. (2017) 32:510–25. doi: 10.1002/mds.26968
12. Postuma RB, Berg D, Stern M, Poewe W, Olanow CW, Oertel W, et al. MDS clinical diagnostic criteria for Parkinson's disease. Mov Disord. (2015) 30:1591–601. doi: 10.1002/mds.26424
13. Rizzo G, Zanigni S, De Blasi R, Grasso D, Martino D, Savica R, et al. Brain MR contribution to the differential diagnosis of Parkinsonian syndromes: an update. Parkinsons Dis. (2016) 2016:2983638. doi: 10.1155/2016/2983638
14. Saeed U, Compagnone J, Aviv RI, Strafella AP, Black SE, Lang AE, et al. Imaging biomarkers in Parkinson's disease and Parkinsonian syndromes: current and emerging concepts. Transl Neurodegener. (2017) 6:8. doi: 10.1186/s40035-017-0076-6
15. Braak H, Del Tredici K, Rüb U, de Vos RAI, Jansen Steur ENH, Braak E. Staging of brain pathology related to sporadic Parkinson's disease. Neurobiol Aging. (2003) 24:197–11. doi: 10.1016/S0197-4580(02)00065-9
16. Del Tredici K, Braak H. Review: sporadic Parkinson's disease: development and distribution of α-synuclein pathology. Neuropathol Appl Neurobiol. (2016) 42:33–50. doi: 10.1111/nan.12298
17. Lehéricy S, Bardinet E, Poupon C, Vidailhet M, François C. 7 Tesla magnetic resonance imaging: a closer look at substantia nigra anatomy in Parkinson's disease. Mov Disord. (2014) 29:1574–81. doi: 10.1002/mds.26043
18. Pyatigorskaya N, Yahia-Cherif L, Gaurav R, Ewenczyk C, Gallea C, Valabregue R, et al. Multimodal magnetic resonance imaging quantification of brain changes in progressive Supranuclear palsy. Mov Disord. (2020) 35:161–70. doi: 10.1002/mds.27877
19. Heim B, Krismer F, de Marzi R, Seppi K. Magnetic resonance imaging for the diagnosis of Parkinson's disease. J Neural Transm (Vienna). (2017) 124:915–64. doi: 10.1007/s00702-017-1717-8
20. Reimão S, Pita Lobo P, Neutel D, Guedes LC, Coelho M, Rosa MM, et al. Substantia nigra neuromelanin-MR imaging differentiates essential tremor from Parkinson's disease. Mov Disord. (2015) 30:953–9. doi: 10.1002/mds.26182
21. Cassidy CM, Zucca FA, Girgis RR, Baker SC, Weinstein JJ, Sharp ME, et al. Neuromelanin-sensitive MRI as a noninvasive proxy measure of dopamine function in the human brain. Proc Natl Acad Sci USA. (2019) 116:5108–17. doi: 10.1073/pnas.1807983116
22. Lehéricy S, Sharman MA, Dos Santos CL, Paquin R, Gallea C. Magnetic resonance imaging of the substantia nigra in Parkinson's disease. Mov Disord. (2012) 27:822–30. doi: 10.1002/mds.25015
23. Kitao S, Matsusue E, Fujii S, Miyoshi F, Kaminou T, Kato S, et al. Correlation between pathology and neuromelanin MR imaging in Parkinson's disease and dementia with Lewy bodies. Neuroradiology. (2013) 55:947–53. doi: 10.1007/s00234-013-1199-9
24. Sulzer D, Cassidy C, Horga G, Kang UJ, Fahn S, Casella L, et al. Neuromelanin detection by magnetic resonance imaging (MRI) and its promise as a biomarker for Parkinson's disease. NPJ Parkinson's Dis. (2018) 4:11. doi: 10.1038/s41531-018-0047-3
25. Sasaki M, Shibata E, Tohyama K, Takahashi J, Otsuka K, Tsuchiya K, et al. Neuromelanin magnetic resonance imaging of locus ceruleus and substantia nigra in Parkinson's disease. Neuroreport. (2006) 17:1215–8. doi: 10.1097/01.wnr.0000227984.84927.a7
26. Pyatigorskaya N, Magnin B, Mongin M, Yahia-Cherif L, Valabregue R, Arnaldi D, et al. comparative study of MRI biomarkers in the substantia nigra to discriminate idiopathic Parkinson disease. Am J Neuroradiol. (2018) 39:1460–7. doi: 10.3174/ajnr.A5702
27. Ohtsuka C, Sasaki M, Konno K, Kato K, Takahashi J, Yamashita F, et al. Differentiation of early-stage Parkinsonisms using neuromelanin-sensitive magnetic resonance imaging. Parkinsonism Relat Disord. (2014) 20:755–60. doi: 10.1016/j.parkreldis.2014.04.005
28. Pyatigorskaya N, Gaurav R, Arnaldi D, Leu-Semenescu S, Yahia-Cherif L, Valabregue R, et al. Magnetic resonance imaging biomarkers to assess substantia nigra damage in idiopathic rapid eye movement sleep behavior disorder. Sleep. (2017) 40: doi: 10.1093/sleep/zsx149
29. Arnulf I, Neutel D, Herlin B, Golmard J-L, Leu-Semenescu S, Cochen de Cock V, et al. Sleepiness in idiopathic REM sleep behavior disorder and Parkinson disease. Sleep. (2015) 38:1529–35. doi: 10.5665/sleep.5040
30. Boeve BF, Silber MH, Saper CB, Ferman TJ, Dickson DW, Parisi JE, et al. Pathophysiology of REM sleep behaviour disorder and relevance to neurodegenerative disease. Brain. (2007) 130:2770–88. doi: 10.1093/brain/awm056
31. Galbiati A, Verga L, Giora E, Zucconi M, Ferini-Strambi L. The risk of neurodegeneration in REM sleep behavior disorder: a systematic review and meta-analysis of longitudinal studies. Sleep Med Rev. (2019) 43:37–46. doi: 10.1016/j.smrv.2018.09.008
32. Postuma RB, Gagnon JF, Vendette M, Fantini ML, Massicotte-Marquez J, Montplaisir J. Quantifying the risk of neurodegenerative disease in idiopathic REM sleep behavior disorder. Neurology. (2009) 72:1296–300. doi: 10.1212/01.wnl.0000340980.19702.6e
33. Iranzo A, Tolosa E, Gelpi E, Molinuevo JL, Valldeoriola F, Serradell M, et al. Neurodegenerative disease status and post-mortem pathology in idiopathic rapid-eye-movement sleep behaviour disorder: an observational cohort study. Lancet Neurol. (2013) 12:443–53. doi: 10.1016/S1474-4422(13)70056-5
34. Schwarz ST, Afzal M, Morgan PS, Bajaj N, Gowland PA, Auer DP. The “swallow tail” appearance of the healthy nigrosome–a new accurate test of Parkinson's disease: a case-control and retrospective cross-sectional MRI study at 3T. PLoS ONE. (2014) 9:e93814. doi: 10.1371/journal.pone.0093814
35. Damier P, Hirsch EC, Agid Y, Graybiel AM. The substantia nigra of the human brain. I. Nigrosomes and the nigral matrix, a compartmental organization based on calbindin D(28K) immunohistochemistry. Brain. (1999) 122 (Pt 8):1421–36. doi: 10.1093/brain/122.8.1421
36. Mahlknecht P, Krismer F, Poewe W, Seppi K. Meta-analysis of dorsolateral nigral hyperintensity on magnetic resonance imaging as a marker for Parkinson's disease. Mov Disord. (2017) 32:619–23. doi: 10.1002/mds.26932
37. Reiter E, Mueller C, Pinter B, Krismer F, Scherfler C, Esterhammer R, et al. Dorsolateral nigral hyperintensity on 3.0T susceptibility-weighted imaging in neurodegenerative Parkinsonism. Mov Disord. (2015) 30:1068–76. doi: 10.1002/mds.26171
38. De Marzi R, Seppi K, Högl B, Müller C, Scherfler C, Stefani A, et al. Loss of dorsolateral nigral hyperintensity on 3.0 tesla susceptibility-weighted imaging in idiopathic rapid eye movement sleep behavior disorder. Ann Neurol. (2016) 79:1026–30. doi: 10.1002/ana.24646
39. Ehrminger M, Latimier A, Pyatigorskaya N, Garcia-Lorenzo D, Leu-Semenescu S, Vidailhet M, et al. The coeruleus/subcoeruleus complex in idiopathic rapid eye movement sleep behaviour disorder. Brain. (2016) 139:1180–8. doi: 10.1093/brain/aww006
40. García-Lorenzo D, Longo-Dos Santos C, Ewenczyk C, Leu-Semenescu S, Gallea C, Quattrocchi G, et al. The coeruleus/subcoeruleus complex in rapid eye movement sleep behaviour disorders in Parkinson's disease. Brain. (2013) 136:2120–9. doi: 10.1093/brain/awt152
41. Knudsen K, Fedorova TD, Hansen AK, Sommerauer M, Otto M, Svendsen KB, et al. In-vivo staging of pathology in REM sleep behaviour disorder: a multimodality imaging case-control study. Lancet Neurol. (2018) 17:618–28. doi: 10.1016/S1474-4422(18)30162-5
42. Rizzo G, Copetti M, Arcuti S, Martino D, Fontana A, Logroscino G. Accuracy of clinical diagnosis of Parkinson disease: a systematic review and meta-analysis. Neurology. (2016) 86:566–76. doi: 10.1212/WNL.0000000000002350
43. Mangesius S, Hussl A, Krismer F, Mahlknecht P, Reiter E, Tagwercher S, et al. MR planimetry in neurodegenerative Parkinsonism yields high diagnostic accuracy for PSP. Parkinsonism Relat Disord. (2018) 46:47–55. doi: 10.1016/j.parkreldis.2017.10.020
44. Righini A, Antonini A, De Notaris R, Bianchini E, Meucci N, Sacilotto G, et al. MR imaging of the superior profile of the midbrain: differential diagnosis between progressive supranuclear palsy and Parkinson disease. AJNR Am J Neuroradiol. (2004) 25:927–32.
45. Möller L, Kassubek J, Südmeyer M, Hilker R, Hattingen E, Egger K, et al. Manual MRI morphometry in Parkinsonian syndromes. Mov Disord. (2017) 32:778–82. doi: 10.1002/mds.26921
46. Quattrone A, Nicoletti G, Messina D, Fera F, Condino F, Pugliese P, et al. MR imaging index for differentiation of progressive supranuclear palsy from Parkinson disease and the Parkinson variant of multiple system atrophy. Radiology. (2008) 246:214–21. doi: 10.1148/radiol.2453061703
47. Paviour DC, Price SL, Jahanshahi M, Lees AJ, Fox NC. Longitudinal MRI in progressive supranuclear palsy and multiple system atrophy: rates and regions of atrophy. Brain. (2006) 129:1040–9. doi: 10.1093/brain/awl021
48. Messina D, Cerasa A, Condino F, Arabia G, Novellino F, Nicoletti G, et al. Patterns of brain atrophy in Parkinson's disease, progressive supranuclear palsy and multiple system atrophy. Parkinsonism Relat Disord. (2011) 17:172–6. doi: 10.1016/j.parkreldis.2010.12.010
49. Yu F, Barron DS, Tantiwongkosi B, Fox P. Patterns of gray matter atrophy in atypical Parkinsonism syndromes: a VBM meta-analysis. Brain Behav. (2015) 5:e00329. doi: 10.1002/brb3.329
50. Compta Y, Pereira JB, Ríos J, Ibarretxe-Bilbao N, Junqué C, Bargalló N, et al. Combined dementia-risk biomarkers in Parkinson's disease: a prospective longitudinal study. Parkinsonism Relat Disord. (2013) 19:717–24. doi: 10.1016/j.parkreldis.2013.03.009
51. Delgado-Alvarado M, Gago B, Navalpotro-Gomez I, Jiménez-Urbieta H, Rodriguez-Oroz MC. Biomarkers for dementia and mild cognitive impairment in Parkinson's disease. Mov Disord. (2016) 31:861–81. doi: 10.1002/mds.26662
52. Gratwicke J, Jahanshahi M, Foltynie T. Parkinson's disease dementia: a neural networks perspective. Brain. (2015) 138:1454–76. doi: 10.1093/brain/awv104
53. Vaillancourt DE, Spraker MB, Prodoehl J, Abraham I, Corcos DM, Zhou XJ, et al. High-resolution diffusion tensor imaging in the substantia nigra of de novo Parkinson disease. Neurology. (2009) 72:1378–84. doi: 10.1212/01.wnl.0000340982.01727.6e
54. Schwarz ST, Abaei M, Gontu V, Morgan PS, Bajaj N, Auer DP. Diffusion tensor imaging of nigral degeneration in Parkinson's disease: a region-of-interest and voxel-based study at 3 T and systematic review with meta-analysis. Neuroimage Clin. (2013) 3:481–8. doi: 10.1016/j.nicl.2013.10.006
55. Cochrane CJ, Ebmeier KP. Diffusion tensor imaging in Parkinsonian syndromes: a systematic review and meta-analysis. Neurology. (2013) 80:857–64. doi: 10.1212/WNL.0b013e318284070c
56. Atkinson-Clement C, Pinto S, Eusebio A, Coulon O. Diffusion tensor imaging in Parkinson's disease: review and meta-analysis. Neuroimage Clin. (2017) 16:98–110. doi: 10.1016/j.nicl.2017.07.011
57. Bajaj S, Krismer F, Palma J-A, Wenning GK, Kaufmann H, Poewe W, et al. Diffusion-weighted MRI distinguishes Parkinson disease from the Parkinsonian variant of multiple system atrophy: a systematic review and meta-analysis. PLoS ONE. (2017) 12:e0189897. doi: 10.1371/journal.pone.0189897
58. Nicoletti G, Lodi R, Condino F, Tonon C, Fera F, Malucelli E, et al. Apparent diffusion coefficient measurements of the middle cerebellar peduncle differentiate the Parkinson variant of MSA from Parkinson's disease and progressive supranuclear palsy. Brain. (2006) 129:2679–87. doi: 10.1093/brain/awl166
59. Pellecchia MT, Barone P, Mollica C, Salvatore E, Ianniciello M, Longo K, et al. Diffusion-weighted imaging in multiple system atrophy: a comparison between clinical subtypes. Mov Disord. (2009) 24:689–96. doi: 10.1002/mds.22440
60. Pellecchia MT, Barone P, Vicidomini C, Mollica C, Salvatore E, Ianniciello M, et al. Progression of striatal and extrastriatal degeneration in multiple system atrophy: a longitudinal diffusion-weighted MR study. Mov Disord. (2011) 26:1303–9. doi: 10.1002/mds.23601
61. Barbagallo G, Sierra-Peña M, Nemmi F, Traon AP-L, Meissner WG, Rascol O, et al. Multimodal MRI assessment of nigro-striatal pathway in multiple system atrophy and Parkinson disease. Mov Disord. (2016) 31:325–34. doi: 10.1002/mds.26471
62. Surova Y, Nilsson M, Lätt J, Lampinen B, Lindberg O, Hall S, et al. Disease-specific structural changes in thalamus and dentatorubrothalamic tract in progressive supranuclear palsy. Neuroradiology. (2015) 57:1079–91. doi: 10.1007/s00234-015-1563-z
63. Tsukamoto K, Matsusue E, Kanasaki Y, Kakite S, Fujii S, Kaminou T, et al. Significance of apparent diffusion coefficient measurement for the differential diagnosis of multiple system atrophy, progressive supranuclear palsy, and Parkinson's disease: evaluation by 3.0-T MR imaging. Neuroradiology. (2012) 54:947–55. doi: 10.1007/s00234-012-1009-9
64. Chen B, Fan G, Sun W, Shang X, Shi S, Wang S, et al. Usefulness of diffusion-tensor MRI in the diagnosis of Parkinson variant of multiple system atrophy and Parkinson's disease: a valuable tool to differentiate between them? Clin Radiol. (2017) 72:610.e9–15. doi: 10.1016/j.crad.2017.02.005
65. Nicoletti G, Rizzo G, Barbagallo G, Tonon C, Condino F, Manners D, et al. Diffusivity of cerebellar hemispheres enables discrimination of cerebellar or Parkinsonian multiple system atrophy from progressive supranuclear palsy-Richardson syndrome and Parkinson disease. Radiology. (2013) 267:843–50. doi: 10.1148/radiol.12120364
66. Planetta PJ, Ofori E, Pasternak O, Burciu RG, Shukla P, DeSimone JC, et al. Free-water imaging in Parkinson's disease and atypical Parkinsonism. Brain. (2016) 139:495–508. doi: 10.1093/brain/awv361
67. Ofori E, Pasternak O, Planetta PJ, Li H, Burciu RG, Snyder AF, et al. Longitudinal changes in free-water within the substantia nigra of Parkinson's disease. Brain. (2015) 138:2322–31. doi: 10.1093/brain/awv136
68. Gallea C, Ewenczyk C, Degos B, Welter M-L, Grabli D, Leu-Semenescu S, et al. Pedunculopontine network dysfunction in Parkinson's disease with postural control and sleep disorders. Mov Disord. (2017) 32:693–704. doi: 10.1002/mds.26923
69. Pyatigorskaya N, Mongin M, Valabregue R, Yahia-Cherif L, Ewenczyk C, Poupon C, et al. Medulla oblongata damage and cardiac autonomic dysfunction in Parkinson disease. Neurology. (2016) 87:2540–5. doi: 10.1212/WNL.0000000000003426
70. Schulz J, Pagano G, Fernández Bonfante JA, Wilson H, Politis M. Nucleus basalis of Meynert degeneration precedes and predicts cognitive impairment in Parkinson's disease. Brain. (2018) 141:1501–16. doi: 10.1093/brain/awy072
71. Barbosa JHO, Santos AC, Tumas V, Liu M, Zheng W, Haacke EM, et al. Quantifying brain iron deposition in patients with Parkinson's disease using quantitative susceptibility mapping, R2 and R2. Magn Reson Imaging. (2015) 33:559–65. doi: 10.1016/j.mri.2015.02.021
72. Du G, Lewis MM, Kanekar S, Sterling NW, He L, Kong L, et al. Combined diffusion tensor imaging and apparent transverse relaxation rate differentiate Parkinson disease and Atypical Parkinsonism. AJNR Am J Neuroradiol. (2017) 38:966–72. doi: 10.3174/ajnr.A5136
73. Guan X, Xuan M, Gu Q, Huang P, Liu C, Wang N, et al. Regionally progressive accumulation of iron in Parkinson's disease as measured by quantitative susceptibility mapping. NMR Biomed. (2017) 30:3489. doi: 10.1002/nbm.3489
74. Sjöström H, Granberg T, Westman E, Svenningsson P. Quantitative susceptibility mapping differentiates between Parkinsonian disorders. Parkinsonism Relat Disord. (2017) 44:51–7. doi: 10.1016/j.parkreldis.2017.08.029
75. Deistung A, Schäfer A, Schweser F, Biedermann U, Turner R, Reichenbach JR. Toward in vivo histology: a comparison of quantitative susceptibility mapping (QSM) with magnitude-, phase-, and R2*-imaging at ultra-high magnetic field strength. Neuroimage. (2013) 65:299–314. doi: 10.1016/j.neuroimage.2012.09.055
76. Deistung A, Schweser F, Reichenbach JR. Overview of quantitative susceptibility mapping. NMR Biomed. (2017) 30:3569. doi: 10.1002/nbm.3569
77. Lee J-H, Han Y-H, Kang B-M, Mun C-W, Lee S-J, Baik S-K. Quantitative assessment of subcortical atrophy and iron content in progressive supranuclear palsy and Parkinsonian variant of multiple system atrophy. J Neurol. (2013) 260:2094–101. doi: 10.1007/s00415-013-6951-x
78. Levin J, Kurz A, Arzberger T, Giese A, Höglinger GU. The differential diagnosis and treatment of Atypical Parkinsonism. Dtsch Arztebl Int. (2016) 113:61–9. doi: 10.3238/arztebl.2016.0061
79. Höglinger GU, Respondek G, Stamelou M, Kurz C, Josephs KA, Lang AE, et al. Clinical diagnosis of progressive supranuclear palsy: the movement disorder society criteria. Mov Disord. (2017) 32:853–64. doi: 10.1002/mds.26987.
80. Litvan I, Agid Y, Calne D, Campbell G, Dubois B, Duvoisin RC, et al. Clinical research criteria for the diagnosis of progressive supranuclear palsy (Steele-Richardson-Olszewski syndrome): report of the NINDS-SPSP international workshop. Neurology. (1996) 47:1–9. doi: 10.1212/WNL.47.1.1
81. Kashihara K, Shinya T, Higaki F. Reduction of neuromelanin-positive nigral volume in patients with MSA, PSP and CBD. Intern Med. (2011) 50:1683–7. doi: 10.2169/internalmedicine.50.5101
82. Martin-Bastida A, Pietracupa S, Piccini P. Neuromelanin in Parkinsonian disorders: an update. Int J Neurosci. (2017) 127:1116–23. doi: 10.1080/00207454.2017.1325883
83. Oba H, Yagishita A, Terada H, Barkovich AJ, Kutomi K, Yamauchi T, et al. New and reliable MRI diagnosis for progressive supranuclear palsy. Neurology. (2005) 64:2050–5. doi: 10.1212/01.WNL.0000165960.04422.D0
84. Kato N, Arai K, Hattori T. Study of the rostral midbrain atrophy in progressive supranuclear palsy. J Neurol Sci. (2003) 210:57–60. doi: 10.1016/S0022-510X(03)00014-5
85. Quattrone A, Morelli M, Nigro S, Quattrone A, Vescio B, Arabia G, et al. A new MR imaging index for differentiation of progressive supranuclear palsy-Parkinsonism from Parkinson's disease. Parkinsonism Relat Disord. (2018) 54:3–8. doi: 10.1016/j.parkreldis.2018.07.016
86. Adachi M, Kawanami T, Ohshima H, Sugai Y, Hosoya T. Morning glory sign: a particular MR finding in progressive supranuclear palsy. Magn Reson Med Sci. (2004) 3:125–32. doi: 10.2463/mrms.3.125
87. Mueller C, Hussl A, Krismer F, Heim B, Mahlknecht P, Nocker M, et al. The diagnostic accuracy of the hummingbird and morning glory sign in patients with neurodegenerative Parkinsonism. Parkinsonism Relat Disord. (2018) 54:90–4. doi: 10.1016/j.parkreldis.2018.04.005
88. Paviour DC, Price SL, Stevens JM, Lees AJ, Fox NC. Quantitative MRI measurement of superior cerebellar peduncle in progressive supranuclear palsy. Neurology. (2005) 64:675–9. doi: 10.1212/01.WNL.0000151854.85743.C7
89. Kataoka H, Nishimori Y, Kiriyama T, Nanaura H, Izumi T, Eura N, et al. Increased signal in the superior cerebellar peduncle of patients with progressive Supranuclear Palsy. J Mov Disord. (2019) 12:166–71. doi: 10.14802/jmd.19002
90. Nicoletti G, Tonon C, Lodi R, Condino F, Manners D, Malucelli E, et al. Apparent diffusion coefficient of the superior cerebellar peduncle differentiates progressive supranuclear palsy from Parkinson's disease. Mov Disord. (2008) 23:2370–6. doi: 10.1002/mds.22279
91. Schocke MFH, Seppi K, Esterhammer R, Kremser C, Mair KJ, Czermak BV, et al. Trace of diffusion tensor differentiates the Parkinson variant of multiple system atrophy and Parkinson's disease. Neuroimage. (2004) 21:1443–51. doi: 10.1016/j.neuroimage.2003.12.005
92. Seppi K, Schocke MFH, Esterhammer R, Kremser C, Brenneis C, Mueller J, et al. Diffusion-weighted imaging discriminates progressive supranuclear palsy from PD, but not from the Parkinson variant of multiple system atrophy. Neurology. (2003) 60:922–7. doi: 10.1212/01.WNL.0000049911.91657.9D
93. Worker A, Blain C, Jarosz J, Chaudhuri KR, Barker GJ, Williams SCR, et al. Cortical thickness, surface area and volume measures in Parkinson's disease, multiple system atrophy and progressive supranuclear palsy. PLoS ONE. (2014) 9:e114167. doi: 10.1371/journal.pone.0114167
94. Huppertz H-J, Möller L, Südmeyer M, Hilker R, Hattingen E, Egger K, et al. Differentiation of neurodegenerative Parkinsonian syndromes by volumetric magnetic resonance imaging analysis and support vector machine classification. Mov Disord. (2016) 31:1506–17. doi: 10.1002/mds.26715
95. Morelli M, Arabia G, Novellino F, Salsone M, Giofrè L, Condino F, et al. MRI measurements predict PSP in unclassifiable Parkinsonisms: a cohort study. Neurology. (2011) 77:1042–7. doi: 10.1212/WNL.0b013e31822e55d0
96. Nigro S, Arabia G, Antonini A, Weis L, Marcante A, Tessitore A, et al. Magnetic resonance Parkinsonism index: diagnostic accuracy of a fully automated algorithm in comparison with the manual measurement in a large Italian multicentre study in patients with progressive supranuclear palsy. Eur Radiol. (2017) 27:2665–75. doi: 10.1007/s00330-016-4622-x
97. Nigro S, Antonini A, Vaillancourt DE, Seppi K, Ceravolo R, Strafella AP, et al. Automated MRI classification in progressive Supranuclear palsy: a large International Cohort study. Mov Disord. (2020) 35:976–83. doi: 10.1002/mds.28007
98. Quattrone A, Morelli M, Williams DR, Vescio B, Arabia G, Nigro S, et al. MR Parkinsonism index predicts vertical supranuclear gaze palsy in patients with PSP-Parkinsonism. Neurology. (2016) 87:1266–73. doi: 10.1212/WNL.0000000000003125
99. Cherubini A, Morelli M, Nisticó R, Salsone M, Arabia G, Vasta R, et al. Magnetic resonance support vector machine discriminates between Parkinson disease and progressive supranuclear palsy. Mov Disord. (2014) 29:266–9. doi: 10.1002/mds.25737
100. Scherfler C, Göbel G, Müller C, Nocker M, Wenning GK, Schocke M, et al. Diagnostic potential of automated subcortical volume segmentation in atypical Parkinsonism. Neurology. (2016) 86:1242–9. doi: 10.1212/WNL.0000000000002518
101. Quattrone A, Morelli M, Quattrone A, Vescio B, Nigro S, Arabia G, et al. Magnetic resonance Parkinsonism index for evaluating disease progression rate in progressive supranuclear palsy: a longitudinal 2-year study. Parkinsonism Relat Disord. (2020) 72:1–6. doi: 10.1016/j.parkreldis.2020.01.019
102. Whitwell JL, Jack CR, Parisi JE, Gunter JL, Weigand SD, Boeve BF, et al. Midbrain atrophy is not a biomarker of progressive supranuclear palsy pathology. Eur J Neurol. (2013) 20:1417–22. doi: 10.1111/ene.12212
103. Agosta F, Kostić VS, Galantucci S, Mesaros S, Svetel M, Pagani E, et al. The in vivo distribution of brain tissue loss in Richardson's syndrome and PSP-Parkinsonism: a VBM-DARTEL study. Eur J Neurosci. (2010) 32:640–7. doi: 10.1111/j.1460-9568.2010.07304.x
104. Whitwell JL, Duffy JR, Strand EA, Machulda MM, Senjem ML, Gunter JL, et al. Neuroimaging comparison of primary progressive apraxia of speech and progressive supranuclear palsy. Eur J Neurol. (2013) 20:629–37. doi: 10.1111/ene.12004
105. Josephs KA, Duffy JR, Strand EA, Machulda MM, Senjem ML, Master AV, et al. Characterizing a neurodegenerative syndrome: primary progressive apraxia of speech. Brain. (2012) 135:1522–36. doi: 10.1093/brain/aws032
106. Hassan A, Parisi JE, Josephs KA. Autopsy-proven progressive supranuclear palsy presenting as behavioral variant frontotemporal dementia. Neurocase. (2012) 18:478–88. doi: 10.1080/13554794.2011.627345
107. Longoni G, Agosta F, Kostić VS, Stojković T, Pagani E, Stošić-Opinćal T, et al. MRI measurements of brainstem structures in patients with Richardson's syndrome, progressive supranuclear palsy-Parkinsonism, and Parkinson's disease. Mov Disord. (2011) 26:247–55. doi: 10.1002/mds.23293
108. Santos-Santos MA, Mandelli ML, Binney RJ, Ogar J, Wilson SM, Henry ML, et al. Features of patients with Nonfluent/Agrammatic primary progressive aphasia with underlying progressive supranuclear palsy pathology or corticobasal degeneration. JAMA Neurol. (2016) 73:733–42. doi: 10.1001/jamaneurol.2016.0412
109. Whitwell JL, Jack CR, Boeve BF, Parisi JE, Ahlskog JE, Drubach DA, et al. Imaging correlates of pathology in corticobasal syndrome. Neurology. (2010) 75:1879–87. doi: 10.1212/WNL.0b013e3181feb2e8
110. Lehéricy S, Hartmann A, Lannuzel A, Galanaud D, Delmaire C, Bienaimée M-J, et al. Magnetic resonance imaging lesion pattern in Guadeloupean Parkinsonism is distinct from progressive supranuclear palsy. Brain. (2010) 133:2410–25. doi: 10.1093/brain/awq162
111. Rizzo G, Martinelli P, Manners D, Scaglione C, Tonon C, Cortelli P, et al. Diffusion-weighted brain imaging study of patients with clinical diagnosis of corticobasal degeneration, progressive supranuclear palsy and Parkinson's disease. Brain. (2008) 131:2690–700. doi: 10.1093/brain/awn195
112. Mahlknecht P, Hotter A, Hussl A, Esterhammer R, Schocke M, Seppi K. Significance of MRI in diagnosis and differential diagnosis of Parkinson's disease. Neurodegener Dis. (2010) 7:300–18. doi: 10.1159/000314495
113. Focke NK, Helms G, Pantel PM, Scheewe S, Knauth M, Bachmann CG, et al. Differentiation of typical and atypical Parkinson syndromes by quantitative MR imaging. Am J Neuroradiol. (2011) 32:2087–92. doi: 10.3174/ajnr.A2865
114. Boelmans K, Holst B, Hackius M, Finsterbusch J, Gerloff C, Fiehler J, et al. Brain iron deposition fingerprints in Parkinson's disease and progressive supranuclear palsy. Mov Disord. (2012) 27:421–7. doi: 10.1002/mds.24926
115. Han Y-H, Lee J-H, Kang B-M, Mun C-W, Baik S-K, Shin Y-I, et al. Topographical differences of brain iron deposition between progressive supranuclear palsy and Parkinsonian variant multiple system atrophy. J Neurol Sci. (2013) 325:29–35. doi: 10.1016/j.jns.2012.11.009
116. Eckert T, Sailer M, Kaufmann J, Schrader C, Peschel T, Bodammer N, et al. Differentiation of idiopathic Parkinson's disease, multiple system atrophy, progressive supranuclear palsy, and healthy controls using magnetization transfer imaging. Neuroimage. (2004) 21:229–35. doi: 10.1016/j.neuroimage.2003.08.028
117. Abe K, Terakawa H, Takanashi M, Watanabe Y, Tanaka H, Fujita N, et al. Proton magnetic resonance spectroscopy of patients with Parkinsonism. Brain Res Bull. (2000) 52:589–95. doi: 10.1016/S0361-9230(00)00321-X
118. Davie CA, Barker GJ, Machado C, Miller DH, Lees AJ. Proton magnetic resonance spectroscopy in Steele-Richardson-Olszewski syndrome. Mov Disord. (1997) 12:767–71. doi: 10.1002/mds.870120525
119. Federico F, Simone IL, Lucivero V, de Mari M, Giannini P, Iliceto G, et al. Proton magnetic resonance spectroscopy in Parkinson's disease and progressive supranuclear palsy. J Neurol Neurosurg Psychiatry. (1997) 62:239–42. doi: 10.1136/jnnp.62.3.239
120. Guevara CA, Blain CR, Stahl D, Lythgoe DJ, Leigh PN, Barker GJ. Quantitative magnetic resonance spectroscopic imaging in Parkinson's disease, progressive supranuclear palsy and multiple system atrophy. Eur J Neurol. (2010) 17:1193–202. doi: 10.1111/j.1468-1331.2010.03010.x
121. Tedeschi G, Litvan I, Bonavita S, Bertolino A, Lundbom N, Patronas NJ, et al. Proton magnetic resonance spectroscopic imaging in progressive supranuclear palsy, Parkinson's disease and corticobasal degeneration. Brain. (1997) 120 (Pt 9):1541–52. doi: 10.1093/brain/120.9.1541
122. Gardner RC, Boxer AL, Trujillo A, Mirsky JB, Guo CC, Gennatas ED, et al. Intrinsic connectivity network disruption in progressive supranuclear palsy. Ann Neurol. (2013) 73:603–16. doi: 10.1002/ana.23844
123. Whitwell JL, Avula R, Master A, Vemuri P, Senjem ML, Jones DT, et al. Disrupted thalamocortical connectivity in PSP: a resting-state fMRI, DTI, and VBM study. Parkinsonism Relat Disord. (2011) 17:599–605. doi: 10.1016/j.parkreldis.2011.05.013
124. Bhattacharya K, Saadia D, Eisenkraft B, Yahr M, Olanow W, Drayer B, et al. Brain magnetic resonance imaging in multiple-system atrophy and Parkinson disease: a diagnostic algorithm. Arch Neurol. (2002) 59:835–42. doi: 10.1001/archneur.59.5.835
125. Lehericy S, Bensimon G, Vidailhet M. Parkinsonian syndromes. In: Toga AW editor. Brain Mapping (Waltham: Academic Press), 769–85. doi: 10.1016/B978-0-12-397025-1.00088-9
126. Watanabe H, Saito Y, Terao S, Ando T, Kachi T, Mukai E, et al. Progression and prognosis in multiple system atrophy: an analysis of 230 Japanese patients. Brain. (2002) 125:1070–83. doi: 10.1093/brain/awf117
127. Watanabe H, Ito M, Fukatsu H, Senda J, Atsuta N, Kaga T, et al. Putaminal magnetic resonance imaging features at various magnetic field strengths in multiple system atrophy. Mov Disord. (2010) 25:1916–23. doi: 10.1002/mds.23196
128. Way C, Pettersson D, Hiller A. The “hot cross bun” sign is not always multiple system Atrophy: etiologies of 11 cases. J Mov Disord. (2019) 12:27–30. doi: 10.14802/jmd.18
129. Seppi K, Schocke MFH, Prennschuetz-Schuetzenau K, Mair KJ, Esterhammer R, Kremser C, et al. Topography of putaminal degeneration in multiple system atrophy: a diffusion magnetic resonance study. Mov Disord. (2006) 21:847–52. doi: 10.1002/mds.20843
130. Seppi K, Schocke MFH, Mair KJ, Esterhammer R, Scherfler C, Geser F, et al. Progression of putaminal degeneration in multiple system atrophy: a serial diffusion MR study. Neuroimage. (2006) 31:240–5. doi: 10.1016/j.neuroimage.2005.12.006
131. Prakash N, Hageman N, Hua X, Toga AW, Perlman SL, Salamon N. Patterns of fractional anisotropy changes in white matter of cerebellar peduncles distinguish spinocerebellar ataxia-1 from multiple system atrophy and other ataxia syndromes. Neuroimage. (2009) 47:T72–81. doi: 10.1016/j.neuroimage.2009.05.013
132. Oishi K, Konishi J, Mori S, Ishihara H, Kawamitsu H, Fujii M, et al. Reduced fractional anisotropy in early-stage cerebellar variant of multiple system atrophy. J Neuroimaging. (2009) 19:127–31. doi: 10.1111/j.1552-6569.2008.00262.x
133. Yoon RG, Kim SJ, Kim HS, Choi CG, Kim JS, Oh J, et al. The utility of susceptibility-weighted imaging for differentiating Parkinsonism-predominant multiple system atrophy from Parkinson's disease: correlation with 18F-flurodeoxyglucose positron-emission tomography. Neurosci Lett. (2015) 584:296–301. doi: 10.1016/j.neulet.2014.10.046
134. Zanigni S, Evangelisti S, Testa C, Manners DN, Calandra-Buonaura G, Guarino M, et al. White matter and cortical changes in atypical Parkinsonisms: a multimodal quantitative MR study. Parkinsonism Relat Disord. (2017) 39:44–51. doi: 10.1016/j.parkreldis.2017.03.001
135. Watanabe H, Fukatsu H, Katsuno M, Sugiura M, Hamada K, Okada Y, et al. Multiple regional 1H-MR spectroscopy in multiple system atrophy: NAA/Cr reduction in pontine base as a valuable diagnostic marker. J Neurol Neurosurg Psychiatry. (2004) 75:103–9.
136. Agosta F, Sarasso E, Filippi M. Functional MRI in Atypical Parkinsonisms. Int Rev Neurobiol. (2018) 142:149–73. doi: 10.1016/bs.irn.2018.09.002
137. Salvatore C, Cerasa A, Castiglioni I, Gallivanone F, Augimeri A, Lopez M, et al. Machine learning on brain MRI data for differential diagnosis of Parkinson's disease and progressive Supranuclear palsy. J Neurosci Methods. (2014) 222:230–7. doi: 10.1016/j.jneumeth.2013.11.016
138. Orrù G, Pettersson-Yeo W, Marquand AF, Sartori G, Mechelli A. Using support vector machine to identify imaging biomarkers of neurological and psychiatric disease: a critical review. Neurosci Biobehav Rev. (2012) 36:1140–52. doi: 10.1016/j.neubiorev.2012.01.004
139. Péran P, Barbagallo G, Nemmi F, Sierra M, Galitzky M, Traon AP-L, et al. MRI supervised and unsupervised classification of Parkinson's disease and multiple system atrophy. Mov Disord. (2018) 33:600–8. doi: 10.1002/mds.27307
140. Archer DB, Bricker JT, Chu WT, Burciu RG, McCracken JL. Development and Validation of the Automated Imaging Differentiation in Parkinsonism (AID-P): A Multicentre Machine Learning Study–The Lancet Digital Health. Available online at: https://www.thelancet.com/journals/landig/article/PIIS2589-7500(19)30105-0/fulltext (accessed December 13, 2019).
Keywords: parkinson's disease, progressive supranuclear palsy, multiple system atrophy, multimodal magnetic resonance imaging, diagnosis, machine learning
Citation: Chougar L, Pyatigorskaya N, Degos B, Grabli D and Lehéricy S (2020) The Role of Magnetic Resonance Imaging for the Diagnosis of Atypical Parkinsonism. Front. Neurol. 11:665. doi: 10.3389/fneur.2020.00665
Received: 01 March 2020; Accepted: 03 June 2020;
Published: 17 July 2020.
Edited by:
Yu Zhang, VA Palo Alto Health Care System, United StatesReviewed by:
Nikolaus R. McFarland, University of Florida, United StatesHong Li, Medical University of South Carolina, United States
Mechelle M. Lewis, Pennsylvania State University (PSU), United States
Copyright © 2020 Chougar, Pyatigorskaya, Degos, Grabli and Lehéricy. This is an open-access article distributed under the terms of the Creative Commons Attribution License (CC BY). The use, distribution or reproduction in other forums is permitted, provided the original author(s) and the copyright owner(s) are credited and that the original publication in this journal is cited, in accordance with accepted academic practice. No use, distribution or reproduction is permitted which does not comply with these terms.
*Correspondence: Lydia Chougar, chougar.lydia@gmail.com