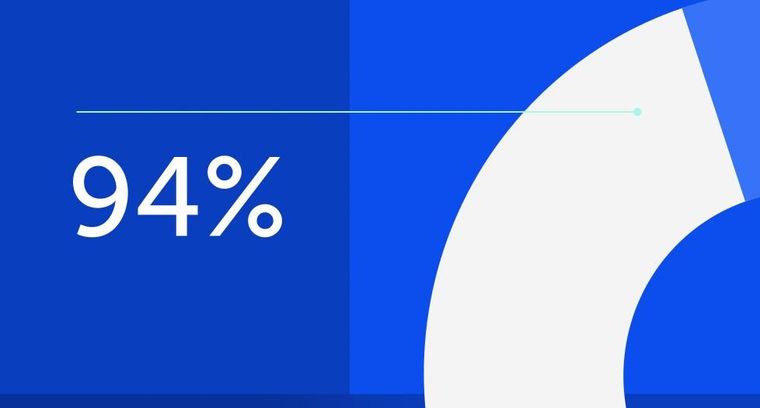
94% of researchers rate our articles as excellent or good
Learn more about the work of our research integrity team to safeguard the quality of each article we publish.
Find out more
ORIGINAL RESEARCH article
Front. Neurol., 30 June 2020
Sec. Sleep Disorders
Volume 11 - 2020 | https://doi.org/10.3389/fneur.2020.00609
The physiological mechanism underlying primary insomnia (PI) is poorly understood. Resting-state functional magnetic resonance imaging (fMRI) has emerged as a powerful tool to explore PI. However, previous studies ignore the dynamics of the brain activity. In the current study, we aimed to explore altered dynamic intrinsic brain activity in PI. Fifty-nine patients with PI and 47 matched healthy controls (HCs) were recruited and underwent resting-state fMRI. The variance of dynamic amplitude of low frequency fluctuation (dALFF) maps across time was calculated to measure the temporal variability of intrinsic brain activity and then compared between patients with PI and HCs. As a result, patients with PI presented increased variance of dALFF in the bilateral hippocampus extending to the parahippocampus, the right putamen and the right anterior insula cortex. In addition, the variance of dALFF in the right putamen was positively correlated with Self-rating Anxiety Scale (SAS) score in PI. Our results revealed increased instability of intrinsic activity in PI.
As the most common sleep disorder, primary insomnia (PI) affects ~30–35% people all around the world (1). The typical characteristic of PI is difficulty falling asleep and feelings of non-restorative sleep (2), this situation usually lasts more than 1 month (3). Associated symptoms with PI such as mood disruption, daytime fatigue and cognitive impairments seriously affect subjects' quality of life (4). However, the physiological mechanism underlying PI is poorly understood.
The pathophysiology of PI has consistently been hypothesized to be related to the hyperarousal in insomniacs (5–8). In recent years, resting-state functional magnetic resonance imaging (fMRI) emerges as a powerful tool to explore the neuromechanism of PI (9). As a novel non-invasive imaging technique for measuring spontaneous brain activity (10), fMRI has been used to find new evidences supporting the hyperarousal hypothesis (11). For example, Chen et al. found greater involvement of anterior insula cortex (AIC) with salience network as well as insula-associated high-frequency gamma power during rest in patients with insomnia (12). Under normal conditions, regions such as the posterior cingulate cortex, the parahippocampal gyrus, and the medial prefrontal cortex in the default mode network (DMN) decrease their activity from wakefulness to slow wave sleep (13). However, activity in the DMN is found altered in sleep deprivation in non-clinical populations (14). The arousal in the DMN is significantly higher in patients with PI even during the day (15, 16). Although these studies deepen our understanding of PI, they ignore the dynamics of the brain activity.
An increasing number of evidences prove that activity and function of the brain is dynamic even during resting state (17). Many methods are proposed to explore the dynamics of the brain (18, 19). One newly developed method named dynamic ALFF (dALFF) is designed to quantify the temporal variability of amplitude of intrinsic brain activity (18). Different from traditional ALFF assuming that brain activity is stationarity, dALFF pays attention on the temporal information of the brain (20). The dALFF depends on simultaneous EEG power fluctuations and can be modulated by non-invasive brain stimulation (21). Exploring the dynamics of the brain activity provides distinct insight into the physiological mechanism of the brain (19, 22, 23). For example, Liao et al. find that combining static and dynamic functional connectomics can differentiate between patients with depression with and without suicidal ideation (24). To our knowledge, few studies have explored altered dynamic intrinsic brain activity in PI.
In the current study, we aimed to explore altered dynamic intrinsic brain activity in PI. Fifty-nine patients with PI and 47 healthy controls (HCs) were recruited and underwent resting-state fMRI. Dynamic ALFF maps of each subject were calculated then the variance of these maps across time was calculated to measure the temporal variability of intrinsic brain activity. In line with previous findings, regions in the DMN and the SN which are consistently found altered might also present altered dynamic intrinsic brain activity in PI.
Patients with PI were recruited from the Department of Neurology at Guangdong Second Provincial General Hospital, Guangzhou, China. Patients were diagnosed according to Diagnostic and Statistical Manual of Mental Disorders, Fourth Edition (DSM-IV) for diagnosis of PI. The inclusion criteria for patients were (a) patients had been complaining of difficulty falling asleep, maintaining sleep, or early awakening for at least 1 month; (b) patients had no other sleep disorders such as hypersomnia, parasomnia, sleep related movement disorder or other psychiatric disorders; (c) patients were younger than 60 years old; (d) free of any psychoactive medication at least 2 weeks prior to and during the study; (e) women who were not pregnant, nursing, or menstruating; (f) the insomnia disorder was not caused by organic disease or severe mental disease such as secondary to depression or generalized anxiety.
Healthy controls (HCs) were recruited from the local community by using advertisements. HCs must met the following criterion: (a) Insomnia Severity Index(ISI) score was <7; (b) no history of swing shifts, shift work, or sleep complaints; (c) no medication or substance abuse (including caffeine, nicotine and alcohol); (d) no brain lesions or prior substantial head trauma; (e) no history of phychiatric or neurological diseases.
Finally, 59 patients with PI and 47 HCs (matched for age, gender, and education) were included in the current study. The Insomnia Severity Index(ISI) (25), Pittsburgh Sleep Quality Index (PSQI) (25, 26) and Epworth Sleepiness Scale (ESS) (27) were used to measure the sleep quality of subjects. The Self-rating Anxiety Scale (SAS) (28) and the Self-rating Depression Scale (SDS) (29) scores were also gotten from each subject. All scales used in the current study were validated Mandarin version.
The current study was approved by the ethics committee of the Guangdong Second Provincial General Hospital. Before the study, written informed consents were obtained from all subjects.
Functional images were preprocessed using Data Processing Assistant for Resting-State fMRI package (DPARSFA, http://www.restfmri.net). The first 10 volumes were removed and followed by slice timing and realignment. The mean frame-wise displacement (FD) was calculated for each subject (30, 31). Subjects were excluded if the translational and rotational displacement exceeded 3.0 mm or 3.0°. No subjects were excluded in this step. The functional images were normalized to the standard EPI template (resampled into 3 × 3 × 3 mm3) and smoothed with 6 × 6 × 6 mm3 full width at half maximum (FWHM) Gaussian kernel. Detrended to reduce low-frequency drift. Then nuisance covariates including Friston 24 motion parameters (32), white matter signal and cerebrospinal fluid signal were regressed out. Then temporal band-pass filter (0.01–0.1 Hz) was performed. Finally, to remove the effect of head motion and ensure the contiguous time points, scrubbing with cubic spline interpolation was used. We also calculated the mean frame-wise displacement (FD) for each subject (30). There was no significant difference of mean FD between patients with PI and HCs (p = 0.161 for two sample t-test).
The dynamic ALFF was calculated with a sliding window method equipped in DynamicBC (v1.1, www.restfmri.net/forum/DynamicBC) (33). The window length was set to 22 TRs (44 s) with 0.6 overlap (34, 35). The ALFF map was calculated divided by the global mean ALFF value for each window. Thus, we got a series dynamic ALFF maps for each subject. Finally, the variance of dALFF maps across time was calculated to measure the temporal variability of intrinsic brain activity (Figure 1). To exclude effect of parameters selection, we validated our results with different window length (50 TRs, 0.6 overlap).
Figure 1. The flow chart of our method. The full-length BOLD fMRI series was segmented into numbers of sliding windows which was represented in different colors. For each sliding window, the ALFF was calculated for each voxel. Last, the variance of dALFF maps across the sliding windows was computed.
Two sample t-test was conducted to determine altered dynamic ALFF in patients with PI compared with HCs. In this procedure, age, sex, and years of education were treated as nuisance covariates. Results were corrected with the Gaussian random field (GRF) where threshold of voxel-wise p < 0.005 and cluster-level p < 0.05.
To investigate the correlation between the altered dALFF and clinical symptoms, linear regression between the altered dALFF and clinical symptoms scores (including ISI, PSQI, SAS, SDS) was built. The altered dALFF was defined as the mean values of peak coordinates with a spherical radius of 6 mm (the MNI coordinates were shown in Table S1).
To exclude the effect of head motion on our results, we calculated the correlation between altered dynamic ALFF and mean FD in patients with PI.
We also validated our results with different parameters selection (window length = 50 TRs, 0.6 overlap).
There was no significant difference between patients with PI and HCs in terms of sociodemographic characteristics, such as age, gender, and year of education. Most of HCs presented normal SAS and SDS scores (SAS or SDS scores <50), except four (or five) had mild anxiety (or depression). Patients with PI presented higher ISI, PSQI, SAS, and SDS scores than those of HCs (all p < 0.001) (Table 1).
Compared with HCs, patients with PI presented significantly increased variance of dALFF in subcortical regions including the bilateral hippocampus extending to the parahippocampus, the right putamen and the right anterior insula cortex (Figure 2, Table 2).
Moreover, we found the variance of dALFF values were associated with SAS score in patients with PI. The peak coordinates of the increased dALFF was in Table S1. The variance of dALFF of the right putamen (R2 = 0.079, p = 0.032 uncorrected, F = 4.813) was significantly associated with SAS score (Figure 3).
To exclude the effect of head motion, we also calculated the correlation between the altered variance of dALFF and mean FD. There was no significant correlation between the variance of dALFF and mean FD (all p > 0.05). In addition, there was no significant difference of mean FD between patients with PI and HCs (p = 0.161).
Our main results could be validated with different parameter selection (the details were shown in Table S1).
In the current study, it was the first time to explore altered dynamic intrinsic brain activity in PI using dALFF. Patients with PI presented increased variance of dALFF in the bilateral hippocampus extending to the parahippocampus, the right putamen and the right anterior insula cortex. In addition, the variance of dALFF in the right putamen was correlated with SAS score in PI. In line with the hyperarousal hypothesis, we found that regions in the DMN presented increased variance of dALFF in patients with PI. Our results revealed increased instability of intrinsic activity in PI.
Dynamic intrinsic brain activity of regions in the DMN was more unstable in patients with PI compared with HCs. Previous studies consistently found structural and functional aberrance in the DMN. For example, as a key region of the DMN, structural and functional aberrance of the bilateral hippocampus was consistently found (11). Evidences from animals (36) and human (37) supported that this aberrance was resulted from sleep restriction or deprivation (36, 37). The attenuated neurogenesis in the hippocampus resulted in a deterioration of neurocognitive performance by disrupting the hippocampus-dependent memory consolidation in PI (38), even in normal sleepers with prolonged sleep deprivation (39) and chronic insomnia (40). In addition to structural aberrance in the hippocampus, altered activity in the DMN including the hippocampus is also found in sleep deprivation (14). The sleep-wake differences in cerebral metabolic rate for glucose of the hippocampus in PI is smaller than that in HCs (41). Functional connectivity of the hippocampus connected to the middle frontal gyrus was found altered and this altered link was correlated with insomnia severity (42). Decreased functional connectivity of the parahippocampal gyrus connected to the posterior DMN node was also found in PI (13). However, few studies focused on altered dynamic intrinsic brain activity in PI. In addition to these findings, we found the temporal intrinsic brain activity of the hippocampus and the parahippocampal gyrus was more unstable in patients with PI compared with HCs. Consist with previous studies, the increased variance of dALFF might be related with rumination in PI (15).
In addition, the increased variance of dALFF of the right AIC was also found in patients with PI. The AIC was posited to be a source of the slow waves that characterized deeper stages of sleep (43). Its involvement with the SN was consistently found greater in patients with PI (12) and in insomnia in rodents (44). The correlation between the AIC BOLD and EEG gamma frequency power was also greater reflecting negative affect in PI (12). While, the effective connectivity of the AIC was found decreased in PI (45). Combing with these evidences, the increased variance of dALFF might reflect overactive but ineffective activity of the AIC in PI.
We also excluded the effect of head motion and parameter selection on our results. There was no significant correlation between mean FD and altered dALFF in PI and no significant difference of the mean FD between PI and HCs. Yan et al. (46) also did not find any meaningful relationships between dALFF with physiological signals. All these results suggested the head motion did not contribute observed results in the current study to some extent. In addition, we validated our results with different parameters selection suggesting the increased dALFF in patients in PI was reliable.
There were many limitations must be considered. First, results obtained come from a single dataset with limited sample size. Future study could use another independent sample set to validate our results. Second, even though abnormal emotional function was a predisposing factor to PI but not the consequence of sleep less (47, 48), we could not exclude the possibility that the increased dALFF found in our results was more relative to the abnormal emotional function in PI. More work had to be done to elaborate the role of altered dALFF in the neuromechanism of PI. Finally, lacking of polysomnography of patients with PI was another weakness.
In the current study, we explored altered dynamic intrinsic brain activity in PI using dALFF. We found that patients with PI presented increased variance of dALFF in the bilateral hippocampus extending to the parahippocampus, the right putamen and the right anterior insula cortex. In addition, the increased variance of dALFF values in the right putamen was correlated with SAS score in PI. Our results revealed increased instability of intrinsic activity in PI.
The datasets generated for this study are available on request to the corresponding author.
The studies involving human participants were reviewed and approved by The ethics committee of Guangdong Second Provincial General Hospital. The patients/participants provided their written informed consent to participate in the research.
XM, JZ, and YW: mainly write the paper. YL and YY: are charge of designing the method. KH and SF: are in charge of data acquisition. GJ: design all study. All authors revised the manuscript and final version of manuscript.
This study has received funding by the National Natural Science Foundation of China (grant no.: 81771807).
No author has financial or other contractual agreements that might cause conflicts of interest.
The Supplementary Material for this article can be found online at: https://www.frontiersin.org/articles/10.3389/fneur.2020.00609/full#supplementary-material
1. Morin CM, Drake CL, Harvey AG, Krystal AD, Manber R, Riemann D, et al. Insomnia disorder. Nat Rev Dis Prim. (2015) 1:15026. doi: 10.1038/nrdp.2015.26
2. Morin CM, Leblanc M, Daley M, Gregoire JP, Mérette C. Epidemiology of insomnia: Prevalence, self-help treatments, consultations, and determinants of help-seeking behaviors. Sleep Med. (2006) 7:123–30. doi: 10.1016/j.sleep.2005.08.008
3. Na N. Diagnostic and statistical manual of mental disorders, 4th edition. Alzheimer Dis Assoc Disord. (1996) 10:20–2.
4. Durmer JS, Dinges DF. Neurocognitive consequences of sleep deprivation. Semin Neurol. (2005) 25:117–29. doi: 10.1055/s-2005-867080
5. Daniel K, Daniel B. Hyperarousal and beyond: new insights to the pathophysiology of insomnia disorder through functional neuroimaging studies. Brain Sci. (2017) 7:23. doi: 10.3390/brainsci7030023
6. Monroe LJ. Psychological and physiological differences between good and poor sleepers. J Abnorm Psychol. (1967) 72:255–64. doi: 10.1037/h0024563
7. Freedman RR, Sattler HL. Physiological and psychological factors in sleep-onset insomnia. J Abnorm Psychol. (1982) 91:380. doi: 10.1037/0021-843X.91.5.380
8. Perlis ML, Giles DE, Mendelson WB, Bootzin RR, Wyatt JK. Psychophysiological insomnia: the behavioural model and a neurocognitive perspective. J Sleep Res. (2010) 6:179–88. doi: 10.1046/j.1365-2869.1997.00045.x
9. Nie X, Shao Y, Liu SY, Li HJ, Wan AL, Nie S, et al. Functional connectivity of paired default mode network subregions in primary insomnia. J Clin Radiol. (2015) 11:3085–93. doi: 10.2147/NDT.S95224
10. Biswal B, Yetkin FZ, Haughton VM, Hyde JS. Functional connectivity in the motor cortex of resting human brain using echo-planar MRI. Mag Reson Med. (2010) 34:537–41. doi: 10.1002/mrm.1910340409
11. O'Byrne JN, Rosa M, Berman J-P, Dang-Vu TT. Neuroimaging findings in primary insomnia. Pathol Biol. (2014) 62:262–9. doi: 10.1016/j.patbio.2014.05.013
12. Chen MC, Chang C, Glover GH, Gotlib IH. Increased insula coactivation with salience networks in insomnia. Biol Psychol. (2014) 97:1–8. doi: 10.1016/j.biopsycho.2013.12.016
13. Sämann PG, Wehrle R, Hoehn D, Spoormaker VI, Peters H, Tully C, et al. Development of the brain's default mode network from wakefulness to slow wave sleep. Cereb Cortex. (2011) 21:2082–93. doi: 10.1093/cercor/bhq295
14. Gujar N, Yoo SS, Hu P, Walker MP. The unrested resting brain: sleep deprivation alters activity within the default-mode network. J Cogn Neurosci. (2014) 22:1637–48. doi: 10.1162/jocn.2009.21331
15. Riemann D, Spiegelhalder K, Feige B, Voderholzer U, Berger M, Perlis M, et al. The hyperarousal model of insomnia: a review of the concept and its evidence. Sleep Med Rev. (2010) 14:19–31. doi: 10.1016/j.smrv.2009.04.002
17. Hellyer PJ, Shanahan M, Scott G, Wise RJS, Sharp DJ, Leech R. The control of global brain dynamics: opposing actions of frontoparietal control and default mode networks on attention. J Neurosci. (2014) 34:451–61. doi: 10.1523/JNEUROSCI.1853-13.2014
18. Tagliazucchi E, Carhartharris R, Leech R, Nutt D, Chialvo DR. Enhanced repertoire of brain dynamical states during the psychedelic experience. Hum Brain Mapp. (2015) 35:5442–56. doi: 10.1002/hbm.22562
19. Han S, Wang X, He Z, Sheng W, Zou Q, Li L, et al. Decreased static and increased dynamic global signal topography in major depressive disorder. Progr Neuro-Psychopharmacol Biol Psychiatr. (2019) 94:109665. doi: 10.1016/j.pnpbp.2019.109665
20. Zang YF, He Y, Zhu CZ, Cao QJ, Sui MQ, Liang M, et al. Altered baseline brain activity in children with ADHD revealed by resting-state functional MRI. Brain Dev. (2007) 29:83–91. doi: 10.1016/j.braindev.2006.07.002
21. Liao W, Li J, Ji GJ, Wu GR, Chen H. Endless fluctuations: temporal dynamics of the amplitude of low frequency fluctuations. IEEE Trans Med Imag. (2019) 38:2523–32. doi: 10.1109/TMI.2019.2904555
22. Kaiser RH, Whitfield-Gabrieli S, Dillon DG, Goer F, Beltzer M, Minkel J, et al. Dynamic resting-state functional connectivity in major depression. Neuropsychopharmacology. (2015) 41:1822–30. doi: 10.1038/npp.2015.352
23. Han S, Cui Q, Wang X, Li L, Li D, He Z, et al. Resting state functional network switching rate is differently altered in bipolar disorder and major depressive disorder. Hum Brain Mapp. (2020). doi: 10.1002/hbm.25017. [Epub ahead of print].
24. Wei L, Jiao L, Duan X, Qian C, Chen H, Chen H. Static and dynamic connectomics differentiate between depressed patients with and without suicidal ideation. Hum Brain Mapp. (2018) 39:4105–18. doi: 10.1002/hbm.24235
25. Bastien CH, Vallières A, Morin CM. Validation of the insomnia severity index as an outcome measure for insomnia research. Sleep Med. (2001) 2:297–307. doi: 10.1016/S1389-9457(00)00065-4
26. Buysse DJ, Reynolds CF III, Monk TH, Berman SR, Kupfer DJ. The pittsburgh sleep quality index: a new instrument for psychiatric practice and research. Psychiatr Res. (1989) 28:193–213. doi: 10.1016/0165-1781(89)90047-4
27. Johns MW. A new method for measuring daytime sleepiness: the epworth sleepiness scale. Sleep. (1991) 14:540–5. doi: 10.1093/sleep/14.6.540
28. Zung WW. A rating instrument for anxiety disorders. Psychosomatics. (1971) 12:371–9. doi: 10.1016/S0033-3182(71)71479-0
29. Zung WW. A self-rating depression scale. Arch Gen Psychiatr. (1965) 12:63–70. doi: 10.1001/archpsyc.1965.01720310065008
30. Han S, Zong X, Hu M, Yu Y, Wang X, Long Z, et al. Frequency-selective alteration in the resting-state corticostriatal-thalamo-cortical circuit correlates with symptoms severity in first-episode drug-naive patients with schizophrenia. Schizophr Res. (2017). 189:175–80. doi: 10.1016/j.schres.2017.02.019
31. Power JD, Barnes KA, Snyder AZ, Schlaggar BL, Petersen SE. Spurious but systematic correlations in functional connectivity MRI networks arise from subject motion. Neuroimage. (2012) 59:2142–54. doi: 10.1016/j.neuroimage.2011.10.018
32. Satterthwaite TD, Wolf DH, Loughead J, Ruparel K, Elliott MA, Hakonarson H, et al. Impact of in-scanner head motion on multiple measures of functional connectivity: relevance for studies of neurodevelopment in youth. NeuroImage. (2012) 60:623–32. doi: 10.1016/j.neuroimage.2011.12.063
33. Wei L, Guo-Rong W, Qiang X, Gong-Jun J, Zhiqiang Z, Yu-Feng Z, et al. DynamicBC: a MATLAB toolbox for dynamic brain connectome analysis. Brain Connect. (2014) 4:780–90. doi: 10.1089/brain.2014.0253
34. Li J, Duan X, Cui Q, Chen H, Liao W. More than just statics: temporal dynamics of intrinsic brain activity predicts the suicidal ideation in depressed patients. Psychol Med. (2018) 49:852–860. doi: 10.1017/S0033291718001502
35. Allen EA, Eswar D, Plis SM, Erhardt EB, Tom E, Calhoun VD. Tracking whole-brain connectivity dynamics in the resting state. Cereb Cortex. (2014) 24:663–76. doi: 10.1093/cercor/bhs352
36. Ruben GM, Natalia S, Stewart DR, Hui G, Ronald S, Dennis MG. Sleep deprivation reduces proliferation of cells in the dentate gyrus of the hippocampus in rats. J Physiol. (2010) 549:563–71. doi: 10.1113/jphysiol.2003.041665
37. Christian M, Peters JD, Liron N, Elizabeth G. Sleep deprivation inhibits adult neurogenesis in the hippocampus by elevating glucocorticoids. Proc Natl Acad Sci USA. (2006) 103:19170–5. doi: 10.1073/pnas.0608644103
38. Fulda S, Schulz H. Cognitive dysfunction in sleep disorders. Sleep Med Rev. (2001) 5:423–45. doi: 10.1053/smrv.2001.0157
39. Noh HJ, Joo EY, Kim ST, Yoon SM, Koo DL, Kim D, et al. The relationship between hippocampal volume and cognition in patients with chronic primary insomnia. J Clin Neurol. (2012) 8:130–8. doi: 10.3988/jcn.2012.8.2.130
40. Van PC. Relationship between hippocampal volume and memory ability in healthy individuals across the lifespan: review and meta-analysis. Neuropsychologia. (2004) 42:1394–13. doi: 10.1016/j.neuropsychologia.2004.04.006
41. Eric Nofzinger A, Daniel Buysse J, Anne Germain D, Jean Miewald M, David Kupfer J. Functional neuroimaging evidence for hyperarousal in insomnia. Am J Psychiatr. (2004) 161:2126–8. doi: 10.1176/appi.ajp.161.11.2126
42. Leerssen J, Wassing R, Ramautar JR, Stoffers D, Lakbila-Kamal O, Perrier J, et al. Increased hippocampal-prefrontal functional connectivity in insomnia. Neurobiol Learn Mem. (2018). 160:144–50. doi: 10.1016/j.nlm.2018.02.006
43. Murphy M, Riedner BA, Huber R, Massimini M, Ferrarelli F, Tononi G. Source modeling sleep slow waves. Proc Natl Acad Sci USA. (2009) 106:1608–13. doi: 10.1073/pnas.0807933106
44. Cano G, Mochizuki T, Saper CB. Neural circuitry of stress-induced insomnia in rats. J Neurosci. (2008) 28:10167–84. doi: 10.1523/JNEUROSCI.1809-08.2008
45. Li C, Dong M, Yin Y, Hua K, Fu S, Jiang G. Aberrant effective connectivity of the right anterior insula in primary insomnia. Front Neurol. (2018) 9:317. doi: 10.3389/fneur.2018.00317
46. Chao-Gan Y, Zhen Y, Colcombe SJ, Xi-Nian Z, Milham MP. Concordance among indices of intrinsic brain function: Insights from inter-individual variation and temporal dynamics. Sci Bull. (2017) 62:1572–84. doi: 10.1016/j.scib.2017.09.015
47. Fernándezmendoza J, Velabueno A, Vgontzas AN, Ramosplatón MJ, Olavarrietabernardino S, Bixler EO, et al. Cognitive-emotional hyperarousal as a premorbid characteristic of individuals vulnerable to insomnia. Psychosom Med. (2010) 72:397–403. doi: 10.1097/PSY.0b013e3181d75319
Keywords: primary insomnia, functional magnetic resonance imaging, intrinsic brain activity, temporal variability, dynamic amplitude of low frequency fluctuation
Citation: Meng X, Zheng J, Liu Y, Yin Y, Hua K, Fu S, Wu Y and Jiang G (2020) Increased Dynamic Amplitude of Low Frequency Fluctuation in Primary Insomnia. Front. Neurol. 11:609. doi: 10.3389/fneur.2020.00609
Received: 17 March 2020; Accepted: 25 May 2020;
Published: 30 June 2020.
Edited by:
Ingo Fietze, Charité – Universitätsmedizin Berlin, GermanyReviewed by:
Arturo Garay, Centro de Educación Médica e Investigaciones Clínicas Norberto Quirno (CEMIC), ArgentinaCopyright © 2020 Meng, Zheng, Liu, Yin, Hua, Fu, Wu and Jiang. This is an open-access article distributed under the terms of the Creative Commons Attribution License (CC BY). The use, distribution or reproduction in other forums is permitted, provided the original author(s) and the copyright owner(s) are credited and that the original publication in this journal is cited, in accordance with accepted academic practice. No use, distribution or reproduction is permitted which does not comply with these terms.
*Correspondence: Guihua Jiang, Z2guamlhbmcyMDAyQDE2My5jb20=
Disclaimer: All claims expressed in this article are solely those of the authors and do not necessarily represent those of their affiliated organizations, or those of the publisher, the editors and the reviewers. Any product that may be evaluated in this article or claim that may be made by its manufacturer is not guaranteed or endorsed by the publisher.
Research integrity at Frontiers
Learn more about the work of our research integrity team to safeguard the quality of each article we publish.