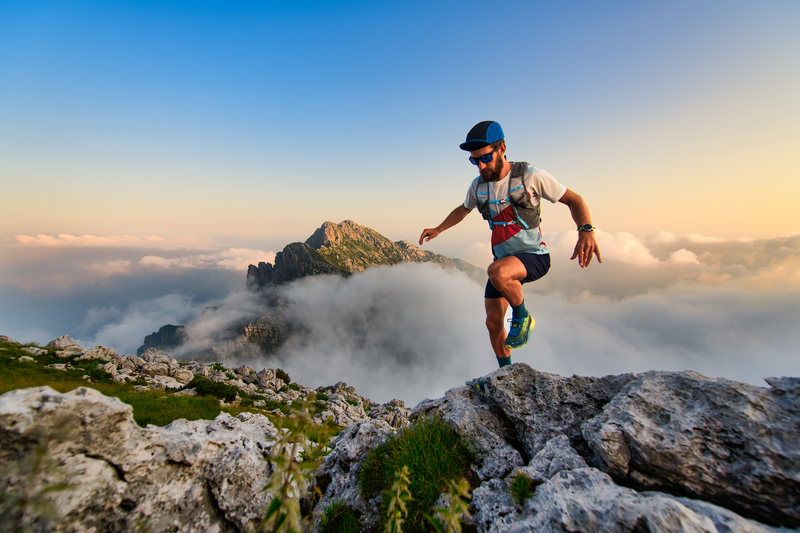
95% of researchers rate our articles as excellent or good
Learn more about the work of our research integrity team to safeguard the quality of each article we publish.
Find out more
PERSPECTIVE article
Front. Neurol. , 16 June 2020
Sec. Neurorehabilitation
Volume 11 - 2020 | https://doi.org/10.3389/fneur.2020.00445
This article is part of the Research Topic Digital Technology in Neurology: From Clinical Assessment to Neurorehabilitation View all 14 articles
Multiple sclerosis (MS) is a frequent chronic inflammatory disease of the central nervous system that affects patients over decades. As the monitoring and treatment of MS become more personalized and complex, the individual assessment and collection of different parameters ranging from clinical assessments via laboratory and imaging data to patient-reported data become increasingly important for innovative patient management in MS. These aspects predestine electronic data processing for use in MS documentation. Such technologies enable the rapid exchange of health information between patients, practitioners, and caregivers, regardless of time and location. In this perspective paper, we present our digital strategy from Dresden, where we are developing the Multiple Sclerosis Documentation System (MSDS) into an eHealth platform that can be used for multiple purposes. Various use cases are presented that implement this software platform and offer an important perspective for the innovative digital patient management in the future. A holistic patient management of the MS, electronically supported by clinical pathways, will have an important impact on other areas of patient care, such as neurorehabilitation.
The low average age at diagnosis and an only slightly reduced life expectancy make multiple sclerosis (MS) a long-term disease that is relevant to patients for decades (1, 2). At the same time, the high inter- and intra-individual variability in the course of the disease constantly leads to new treatment situations (3). As a result, numerous disease data with information about complaints, symptoms, as well as diagnostic and therapeutic measures accumulate within the framework of medical and therapeutic care (4).
Today, certain therapeutic options are linked to the presence of certain disease characteristics (5). When prescribing specific therapies, the effectiveness must be documented individually for each patient. The differentiation between responders and non-responders of immunomodulatory therapies is not conceivable without efficient specific documentation (6, 7). When the documentation of psychological symptoms and other medical disciplines are added, the necessity for a complex course documentation becomes clear (8, 9). In addition, a large number of healthcare institutions depend on a timely and holistic exchange of information between the partners involved (10, 11).
Electronic patient data management represents a suitable implementation for the MS progress documentation of all the points mentioned. Linkable database systems allow individual courses to be displayed in a standardized way over many years, and the data generated can be stored in a readable, transparent, and quickly retrievable form (12, 13). Automated calculations lower the threshold for the systematic application of established scales such as Expanded Disability Status Scale (EDSS) or Multiple Sclerosis Functional Composite (MSFC), which are indispensable for the quantification of neurological deficits (14–19). In addition to the standard instruments, patient-reported outcomes (PROs) increasingly complete the holistic assessment of the disease course (20, 21). The regular use of scales is now a prerequisite in expert recommendations regarding MS therapy. Patient-specific documentation and management is becoming more and more important in the growing field of neurorehabilitation in MS (22). Of particular importance is how complex and individualized neurorehabilitation is designed. Common approaches to neurorehabilitation include the treatment of individual symptomatic impairments, often using motor training approaches (23, 24). Because of the wide range of symptoms and disabilities in MS, single symptomatic interventions can only be seen as part of the rehabilitation program. Comprehensive information and education of patients and relatives and other social and environmental factors are equally important (25). The more stakeholders are involved and the more information is collected and processed, the more complex and costly the processes of neurorehabilitation become, which leads to the necessity of a measurable efficiency of rehabilitation.
Due to the large amount of data to be processed, the large number of communicating persons, and the demands of the healthcare system, all these points predestine electronic data processing for use in the holistic documentation and management of progression in MS (26–28).
The coordinated exchange of health-related information is associated with numerous promising opportunities for daily care in clinics and practices supporting decision-making and the treatment process as a whole (10, 29). Technologies such as an electronic medical record (EMR) enable the rapid exchange of health information between patients, practitioners, and caregivers, regardless of time and location, with the EMR mainly exchanging data between health professionals in single entities of the health system (4, 22, 30–33). Today, every part of the treatment process—from diagnosis, treatment selection, and application to patient education and long-term care, including drug treatment and rehabilitation—can be complemented by a quality-assured implementation of information technologies in healthcare (“eHealth”), which also takes into account data security standards and concerns (4, 34, 35).
Such eHealth services are generally considered useful for physicians and nurses in neurological practices to improve clinical documentation, data collection, and diagnosis of specific MS symptoms, doctor–patient communication, and patient education (33). Practices specialized in MS have an increased need for eHealth services to document interventional and non-interventional drug treatment and rehabilitation studies (36).
Despite the many arguments for detailed electronic documentation of people with MS (pwMS), implementation in clinical practice is difficult and has not yet been standardized. The most significant reason for the lack of acceptance and active use of electronic documentation services is the additional time required. Due to the problematic reimbursement situation for physicians, the additional time for detailed documentation is often lacking, especially since there are no comprehensive initiatives by the funding agencies for this problem, which can be aggravated by non-synergetic double documentation tasks resulting from incompatible data platforms. The interoperability of health data between hospital information systems, documentation systems of physician networks or cooperation projects, study-related platforms, and register databases is often severely limited. Various electronic documentation systems developed by the pharmaceutical industry were not followed up after more or less lengthy pilot phases. Overall, it became apparent how problematic a documentation platform dependent on a single pharmaceutical manufacturer can be.
Cross-project documentation systems or systems that are not limited to a single purpose increase the value and service life of health data. Recent advances in the diagnosis and treatment of MS require far-reaching policy changes in clinical reality in order to develop a holistic and efficient approach to MS management (37). In an ideal scenario of well-connected healthcare providers, the EMR serves as a central source of health information by aggregating multi-modular information from different domains and making it accessible according to the needs of all users, not only in-house healthcare professionals. Due to the heterogeneity of MS, it is of great importance to establish reliable and valid measuring instruments to capture disease-relevant characteristics from the patient's point of view in addition to clinical and imaging procedures (3).
Factors reported by patients themselves such as symptoms, health status, health-related quality of life, but also adherence to and satisfaction with treatment, as well as treatment outcomes, are increasingly becoming the focus of attention. PROs are collected using standardized questionnaires and provide valuable information on the effectiveness of interventions and therapies (20, 38–41). The patients' symptoms and physical impairments remain unexplored by the healthcare providers, especially in the intervals between clinic visits (42–45). In addition, pwMS are often affected by varying degrees of cognitive impairment and may forget what they felt a week or two before planned visits (46–48). One possible solution to this problem is for patients to answer questions about their symptoms electronically, either via Internet or through their app-based electronic devices such as smartphones or tablets (49–51). As we have analyzed, patients are happy to use digital instruments to document their disease status (11, 52). Their responses could then be transferred to the health record and various doctors could receive automated notifications of alarming symptoms, which enables the step from data collection to electronically assisted disease management.
Unlike paper-based documentation with its limitations (missing, ambiguous, or contradictory data), electronic documentation with tablets or smartphones can eliminate these problems (11, 51, 53, 54). This enables a faster and more efficient collection of information, offers high security in data storage, and is environmentally friendly.
In order to enable documentation across cases and institutions, all findings, diagnoses, treatment measures, and reports in the future will be stored in an EMR that must also be accessible and usable by the patient. Such a patient record is the starting point for a digitally supported patient management. It enables the physician to quickly gain an overview of all important data as well as the course of the disease and to offer a personalized treatment to the patient in a process of shared decision-making based on shared information. For example, prescribed medication can be read or a comprehensive clinical picture can be created. The issuing of electronic prescriptions, referrals, or doctor's letters can also contribute to more efficient and cost-effective healthcare. Medical care that is specially tailored to the patient improves the course of the disease by reducing side effects to a minimum. In addition, the acceptance of the medication is increased, which in turn improves the effect of the medication (55–58).
The Multiple Sclerosis Documentation System (MSDS) with its clinical focus was developed in Dresden, Germany. It has established itself as an input platform and is constantly being further developed as a desktop version and for web browsers (24, 59, 60). The first MSDS version (MSDS Clinic) was specially designed for MS outpatient departments at universities in 1999 for the structured collection of clinical data on the pathology of MS as well as for the writing of letters to physicians. For the first time, it allowed several users to access the database at the same time. MSDS found a growing number of users in Germany and was used in the MS Registry pilot project. In its early EMR-like version, MSDS allowed the user to enter patient data, clinical history and clinical examination data as well as results and treatment details. For the first time, it was possible to graphically display the course of an individual patient and create medical reports (60).
MSDS Practice is a modified version of the above mentioned clinical MSDS version designed specifically for neurological outpatient practices. In contrast to MSDS Clinic, MSDS Practice addresses the special requirements of neurological practices through a reduced scope of documentation and a simplified user interface, and it combines a transparent presentation of the course of disease with diagnostic and therapeutic decisions in everyday practice (59).
In view of the increasingly complex therapies, the eHealth project group at Dresden University Hospital developed the multidimensional patient management system MSDS3D in cooperation with MedicalSyn GmbH in 2014. As a further development of the MSDS Clinic, MSDS3D is designed to support physicians in performing more complex processes (e.g., treatment management) and integrates patient, nurse, and physician into these processes. Especially in the case of complex long-term diseases such as MS, those involved in the treatment process want a special, intelligent management system that goes beyond pure documentation (61). In addition, the system can be used not only to enter and interpret patient data, but also as an interactive system to provide information to the patient. Interaction with patients takes place either via multi-touch systems as an interactive patient terminal or via mobile devices such as the patient's smartphone. With the development of MSDS3D, the step from pure patient documentation to an adaptive patient management system for MS was thus completed (4, 24).
MSDS3D can be used to conduct the preliminary and accompanying examinations necessary for the application of complex therapies within a defined clinical pathway, as well as patient surveys on various aspects of their disease. The integrated survey system for questionnaire-based data collection is equipped with a user interface specifically designed for pwMS. Currently, the Early Mobility Impairment Questionnaire (EMIQ) (62), the Multiple Sclerosis Walking Scale (MSWS-12), and Multiple Sclerosis Health Resource Survey (MS-HRS) (63, 64) are integrated in the questionnaire module. The medical staff manages the survey process (e.g., starting the survey) and provides assistance in answering questions. The mobile terminals are controlled by the MSDS3D system located locally in the treatment center via a special server, which also regulates the data flow to and from the patient. Anonymity and data protection are guaranteed in a complex procedure with encrypted transmission. Patient surveys can thus be carried out digitally, as well as cognitive testing (Paced Auditory Serial Addition Test, Symbol Digit Modalities Test) and gait analysis (Timed 25-Foot Walk, 2 Min Walk Test), which have also been integrated into the system (65, 66).
The MSDS3D infrastructure is also used for the European cohort of the Multiple Sclerosis Partners Advancing Technology and Health Solutions (MSPATHS) (67). This Biogen-funded global program for MS centers in Europe and North America successfully integrates digitally collected PROs into routine clinical care. Data collected via tablet includes general information about the person, health insurance, medical history of MS, use of medication and stimulants, laboratory results, vital signs, and MRI results. With the Multiple Sclerosis Performance Test (MSPT) (17, 18) in addition to the anamnestic parameters, all components of the MSFC as well as Neuro-QoL domains are recorded in a standardized manner, which can be visualized back to physician and patient using MSDS3D (17, 18).
Various specific MSDS3D modules allow standardized documentation and visualization of visit schedules and obligatory examinations using a vertical timeline that represents the examination times and horizontally arranged tasks with detailed parameters to be recorded. Administrative functions (e.g., creating a patient, registering a patient for an examination) and evaluation mechanisms are integrated into the patient management system via a toolbar. In diagnostic–therapeutic terms, the implemented instruments are based on the guidelines of the respective professional associations.
Further developments of MSDS3D enable the web-based system-independent use of the platform and the integration of further participants in the treatment process. In addition, image and laboratory data relevant to MS can be captured in the MSDS3D platform so that for the first time they can be systematically investigated combined with clinical data. By implementing lab data into the MSDS3D transferred from the lab server, the analysis of laboratory data from the real world could be performed, easily linking clinical and laboratory data (68, 69).
Particular emphasis was placed on the systematic collection of post-marketing safety data, as randomized controlled trials are not able to identify rare adverse events (70). This was recently shown in a systematic analysis of real-world studies for Fingolimod as an example (71). These post-authorization safety studies (PASS) are used to collect real-world data reflecting the real-life safety profile and utility of drugs, which is supported by MSDS3D (72, 73).
For MSDS3D, drug-specific modules have been developed based on the proposed handling of the specific MS treatment (74, 75). The natalizumab module, specifically adapted for treatment with the monoclonal antibody natalizumab, contains all essential process components from the indication to the infusion procedure and the necessary control tests. The sequence of the visits and the instruments to be filled in are defined in the MSDS3D natalizumab module. Subsequent instruments include disease history, EDSS, and MSFC as well as MRI and para-clinical parameters as lab data.
Specifically, a checklist was integrated that asks for the occurrence of common symptoms associated with progressive multifocal leukoencephalopathy (PML) as a possible side effect of natalizumab therapy and must be answered by each patient alone or in the presence of relatives before each infusion. This is also done via touch screen on the patient terminal or via touch pad. If the checklist contains warnings of a PML, so-called red flags appear, which require an immediate patient consultation with the attending physician. Once all the instruments necessary for the respective visit have been performed, the physician approves the infusion and only then can natalizumab be administered. The infusion itself is documented by the nurse who also arranges the next appointment using the MSDS3D appointment manager. If the patient does not appear at the agreed appointment, the nurse and doctor are reminded by MSDS3D. If all instruments of therapy with natalizumab are marked green, the visit can be verified with the appropriate authorization and transferred to a central register (e.g., MS register or drug-specific register) in a pseudonymized manner. Compliance with the applicable national and European data protection regulations is guaranteed.
The findings from this pilot project are widely applied throughout Germany in the TRUST study initiated by Biogen to accompany patients under treatment with natalizumab (76). In addition, other modules have been developed to collect data of high-efficacy treatments with fingolimod (77, 78) and alemtuzumab (79). For alemtuzumab, MSDS3D provides the necessary regular monitoring to ensure clinical vigilance after completion of the infusion courses over the necessary observation period of 4 years. It enables cross-sectoral standardized management and documentation of patients treated with alemtuzumab and can serve as a data entry system for various databases. We successfully linked clinical and imaging data of individual patients with the promising biomarker serum neurofilament light in Alemtuzumab-treated patients (80). For ocrelizumab, the CONFIDENCE study was integrated into the MSDS3D platform as a large, non-interventional PASS that assesses long-term safety and effectiveness of Ocrelizumab and other MS treatments in comparison (81). Interestingly, these data will be integrated into other studies that have been developed to fulfill international regulatory requirements (EMA, FDA). Cladribine data are collected using the CLARION MSDS3D module in Germany.
Additionally, MSDS3D has found its way into the implementation of various scientific research projects as, for example, the multicenter study “Responsiveness of patient based outcome parameters in MS” (REPABO), in which pwMS were followed over up to 3 years and patients and their study physician rated different scales in parallel each year (82). A new physician tool, MSProDiscuss, was integrated in the PANGAEA module to facilitate physician–patient discussion in evaluating early, subtle signs of disease progression that represent the transition from relapsing–remitting to secondary progressive subtype (83).
In the age of large, complex, digitally available data sets (big data), and the establishment of suitable analytical methods, MS as a widespread chronic disease with various characteristics is predestined for large-scale data research approaches (84, 85). There are many prerequisites for finally investigating origin, progression modifiers, and chances of remission in greater depth with modern analytical methods in larger cohorts: the not yet completely clarified etiology, complex constellations of symptoms, the growing register landscape, as well as newly emerging markers and progression approaches (3, 33, 36). Big data analyses (e.g., data mining and machine learning) will not turn MS into a curable disease, but clear application goals can be derived:
• Comprehensive automated analysis of MRI data.
• Data-driven individualization of therapy recommendations.
• In real-time optimized follow-up by simultaneous consideration of numerous clinical outcomes and PROs.
• Combination of previously isolated domains such as genome, molecular, and epigenetic data.
Typical pitfalls of large complex data sets are potentially poor data quality, data inconsistency, poor data stability, securing patient protection and consent, and other legal barriers (13, 84, 86, 87). In addition, the interpretability of the results must be in the foreground when research moves away from the level of confirmatory hypothesis testing in order not to achieve irrelevant or misleading results. Ultimately, findings from big data have to be elaborated into new testable hypotheses. However, the data-oriented perspective also strengthens the view of the actual effect sizes (clinical important differences), where up to now all significant p-values of certified minimum effects have been too often classified as relevant.
Data from multiple sources such as registries, EMRs, and PASS can be separately analyzed and combined in a meta-analysis or brought together in a single big data source for MS research like the MS Data Alliance (13, 33). As we have described above, MSDS3D has enabled us to free data sources, data collection systems, and study types from their pigeonholes in an integrative manner by building a system that already integrates data from registers, safety studies, and highly specialized EMR processes. Our vision is to provide a platform for holistic management of MS that allows parallel data collection for specific analysis.
Our next steps will be to include neurorehabilitation into this big data approach in MS by creating a neurorehabilitation module in MSDS3D. Here, too, we will follow the approach of making data collected as holistically as possible available to all participants in order to maintain multi-domain patient skills beyond isolated symptomatic approaches. On the professional side, the implementation of clinical pathways for the treatment of symptomatic disabilities will enable data-driven standardized care and make it measurable and verifiable (88). In our system, we have already implemented the necessary data to address, for example, motor deficits and psychosocial problems. We must now take these data and combine it with the efforts of doctors, nurses, and patients who already share and use parts of it. In this way, the electronically supported cycle of data from conception, collection, linking, and utilization can be completed.
TZ, RK, IV, and RH wrote the manuscript. All authors reviewed and approved the final manuscript.
The MSDS3D module development was supported by the Hertie Foundation, Novartis, Teva, Roche, Sanofi, Biogen, and Merck.
TZ, IV, RH declare that the research was conducted in the absence of any commercial or financial relationships that could be construed as a potential conflict of interest. TZ received personal compensation from Biogen, Bayer, Celgene, Novartis, Roche, Sanofi, Teva for the consulting services and additional financial support for the research activities from Bayer, BAT; Biogen, Novartis, Teva, and Sanofi. RK is CEO of MedicalSyn GmbH. RH received personal compensation by Sanofi and travel grants by Celgene and Sanofi.
1. Becker V, Heeschen V, Schuh K, Schieb H, Ziemssen T. Patient satisfaction and healthcare services in specialized multiple sclerosis centres in Germany. Ther Adv Neurol Diso. (2018) 11:1756285617748845. doi: 10.1177/1756285617748845
2. Rensel M. Long-term treatment strategies of pediatric multiple sclerosis, including the use of disease modifying therapies. Children. (2019) 6:73. doi: 10.3390/children6060073
3. Ziemssen T, Kern R, Thomas K. Multiple sclerosis: clinical profiling and data collection as prerequisite for personalized medicine approach. Bmc Neurol. (2016) 16:124. doi: 10.1186/s12883-016-0639-7
4. Kern R, Haase R, Eisele JC, Thomas K, Ziemssen T. Designing an electronic patient management system for multiple sclerosis: building a next generation multiple sclerosis documentation system. Interact J Med Res. (2016) 5:e2. doi: 10.2196/ijmr.4549
5. Inojosa H, Schriefer D, Ziemssen T. Clinical outcome measures in multiple sclerosis: a review. Autoimm Rev. (2020) 19:102512. doi: 10.1016/j.autrev.2020.102512
6. Stangel M, Penner IK, Kallmann BA, Lukas C, Kieseier BC. Towards the implementation of ‘no evidence of disease activity' in multiple sclerosis treatment: the multiple sclerosis decision model. Ther Adv Neurol Diso. (2015) 8:3–13. doi: 10.1177/1756285614560733
7. Stangel M, Penner I, Kallmann B, Lukas C, Kieseier B, Gold R. Multiple sclerosis decision model (MSDM): entwicklung eines mehrfaktorenmodells zur beurteilung des therapie- und krankheitsverlaufs bei schubförmiger multipler sklerose. Aktuel Neurol. (2013) 40:486–93. doi: 10.1055/s-0033-1349643
8. Ziemssen T. Multiple sclerosis beyond EDSS: depression and fatigue. J Neurol Sci. (2009) 277:S37–41. doi: 10.1016/S0022-510X(09)70011-5
9. Kern S, Schrempf W, Schneider H, Schultheiß T, Reichmann H, Ziemssen T. Neurological disability, psychological distress, and health-related quality of life in MS patients within the first three years after diagnosis. Mult Scler. (2009) 15:752–8. doi: 10.1177/1352458509103300
10. Marrie RA, Leung S, Tyry T, Cutter GR, Fox R, Salter A. Use of eHealth and mHealth technology by persons with multiple sclerosis. Mult Scler Relat Disord. (2018) 27:13–19. doi: 10.1016/j.msard.2018.09.036
11. Haase R, Schultheiss T, Kempcke R, Thomas K, Ziemssen T. Use and acceptance of electronic communication by patients with multiple sclerosis: a multicenter questionnaire study. J Med Internet Res. (2012) 14:e135. doi: 10.2196/jmir.2133
12. Persson R, Lee S, Yood MU, Wagner M, Minton N, Niemcryk S, et al. Multi-database study of multiple sclerosis: identification, validation and description of MS patients in two countries. J Neurol. (2019) 266:1095–106. doi: 10.1007/s00415-019-09238-8
13. Glaser A, Stahmann A, Meissner T, Flachenecker P, Horáková D, Zaratin P, et al. Multiple sclerosis registries in Europe – an updated mapping survey. Mult Scler Relat Dis. (2018) 27:171–8. doi: 10.1016/j.msard.2018.09.032
14. D'Souza M, Heikkilä A, Lorscheider J, Haller V, Kravalis K, Gysin S, et al. Electronic neurostatus-EDSS increases the quality of expanded disability status scale assessments: experience from two phase 3 clinical trials. Mult Scler Houndmills Basingstoke Engl. (2019). doi: 10.1177/1352458519845108. [Epub ahead of print].
15. D'Souza M, Steinheimer S, Dorn J, Morrison C, Boisvert J, Kravalis K, et al. Reference videos reduce variability of motor dysfunction assessments in multiple sclerosis. Mult Scler J Exp Transl Clin. (2018) 4:2055217318792399. doi: 10.1177/2055217318792399
16. D'Souza M, Yaldizli Ö, John R, Vogt DR, Papadopoulou A, Lucassen E, et al. Neurostatus e-Scoring improves consistency of expanded disability status scale assessments: a proof of concept study. Mult Scler J. (2016) 23:597–603. doi: 10.1177/1352458516657439
17. Rhodes JK, Schindler D, Rao SM, Venegas F, Bruzik ET, Gabel W, et al. Multiple sclerosis performance test: technical development and usability. Adv Ther. (2019) 36:1741–55. doi: 10.1007/s12325-019-00958-x
18. Rudick RA, Miller D, Bethoux F, Rao SM, Lee J-C, Stough D, et al. The multiple sclerosis performance test (MSPT): an ipad-based disability assessment tool. J Vis Exp Jove. (2014) 88:e51318. doi: 10.3791/51318
19. Kosa P, Barbour C, Wichman A, Sandford M, Greenwood M, Bielekova B. NeurEx: digitalized neurological examination offers a novel high-resolution disability scale. Ann Clin Transl Neur. (2018) 5:1241–9. doi: 10.1002/acn3.640
20. D'Amico E, Haase R, Ziemssen T. Review: patient-reported outcomes in multiple sclerosis care. Mult Scler Relat Disord. (2019) 33:61–6. doi: 10.1016/j.msard.2019.05.019
21. Medina LD, Torres S, Alvarez E, Valdez B, Nair KV. Patient-reported outcomes in multiple sclerosis: validation of the quality of life in neurological disorders (Neuro-QoLTM) short forms. Mult Scler J Exp Transl Clin. (2019) 5:205521731988598. doi: 10.1177/2055217319885986
22. Hendrickson MA, Melton GB, Pitt MB. The review of systems, the electronic health record, and billing. JAMA. (2019) 322:115–16. doi: 10.1001/jama.2019.5667
23. Prosperini L, Filippo MD. Beyond clinical changes: rehabilitation-induced neuroplasticity in MS. Mult Scler J. (2019) 25:1348–62. doi: 10.1177/1352458519846096
24. Ziemssen T, Kempcke R, Eulitz M, Großmann L, Suhrbier A, Thomas K, et al. Multiple sclerosis documentation system (MSDS): moving from documentation to management of MS patients. J Neural Transm. (2013) 120:61–6. doi: 10.1007/s00702-013-1041-x
25. Kesselring J, Beer S. Symptomatic therapy and neurorehabilitation in multiple sclerosis. Lancet Neurol. (2005) 4:643–52. doi: 10.1016/S1474-4422(05)70193-9
26. Berrouiguet S, Perez-Rodriguez MM, Larsen M, Baca-García E, Courtet P, Oquendo M. From eHealth to iHealth: transition to participatory and personalized medicine in mental health. J Med Internet Res. (2018) 20:e2. doi: 10.2196/jmir.7412
27. Egeter J, Hüfner K, Sztankay M, Holzner B, Sperner-Unterweger B. Implementation of an electronic routine outcome monitoring at an inpatient unit for psychosomatic medicine. J Psychosom Res. (2018) 105:64–7. doi: 10.1016/j.jpsychores.2017.12.009
28. Prasser F, Kohlbacher O, Mansmann U, Bauer B, Kuhn KA. Data integration for future medicine (DIFUTURE). Method Inform Med. (2018) 57:e57–65. doi: 10.3414/ME17-02-0022
29. Sola-Valls N, Blanco Y, Sepúlveda M, Martinez-Hernandez E, Saiz A. Telemedicine for monitoring MS activity and progression. Curr Treat Option Neurol. (2015) 17:47. doi: 10.1007/s11940-015-0377-y
30. Meskó B. The real era of the art of medicine begins with artificial intelligence. J Med Internet Res. (2019) 21:e16295. doi: 10.2196/16295
31. Smoller JW. The use of electronic health records for psychiatric phenotyping and genomics. Am J Med Genet B Neuropsychiatr Genet. (2017) 177:601–12. doi: 10.1002/ajmg.b.32548
32. Doyle-Lindrud S. The evolution of the electronic health record. Clin J Oncol Nurs. (2015) 19:153–4. doi: 10.1188/15.CJON.153-154
33. Cohen JA, Trojano M, Mowry EM, Uitdehaag BM, Reingold SC, Marrie RA. Leveraging real-world data to investigate multiple sclerosis disease behavior, prognosis, and treatment. Mult Scler. (2019) 26:23–37. doi: 10.1177/1352458519892555
34. Cimino JJ. Improving the electronic health record—are clinicians getting what they wished for? JAMA. (2013) 309:991–2. doi: 10.1001/jama.2013.890
35. Weathers AL, Esper GJ. How to select and implement an electronic health record in a neurology practice. Neurol Clin Pract. (2013) 3:141–8. doi: 10.1212/CPJ.0b013e31828d9fb7
36. Ziemssen T, Hillert J, Butzkueven H. The importance of collecting structured clinical information on multiple sclerosis. Bmc Med. (2016) 14:81. doi: 10.1186/s12916-016-0627-1
37. Ziemssen T, Derfuss T, Stefano N de, Giovannoni G, Palavra F, Tomic D, et al. Optimizing treatment success in multiple sclerosis. J Neurol. (2016) 263:1053–65. doi: 10.1007/s00415-015-7986-y
38. Wu AW, Kharrazi H, Boulware LE, Snyder CF. Measure once, cut twice—adding patient-reported outcome measures to the electronic health record for comparative effectiveness research. J Clin Epidemiol. (2013) 66:S12–20. doi: 10.1016/j.jclinepi.2013.04.005
39. Banerjee AK, Okun S, Edwards IR, Wicks P, Smith MY, Mayall SJ, et al. Patient-reported outcome measures in safety event reporting: PROSPER consortium guidance. Drug Safety. (2013) 36:1129–49. doi: 10.1007/s40264-013-0113-z
40. Bennett AV, Jensen RE, Basch E. Electronic patient-reported outcome systems in oncology clinical practice. CA Cancer J Clin. (2012) 62:336–47. doi: 10.3322/caac.21150
41. Ammenwerth E, Schnell-Inderst P, Hoerbst A. The impact of electronic patient portals on patient care: a systematic review of controlled trials. J Med Internet Res. (2012) 14:e162. doi: 10.2196/jmir.2238
42. Brichetto G, Pedullà L, Podda J, Tacchino A. Beyond center-based testing: understanding and improving functioning with wearable technology in MS. Mult Scler J. (2019) 25:1402–11. doi: 10.1177/1352458519857075
43. Van Eijk RPA, Bakers JNE, Bunte TM, de Fockert AJ, Eijkemans MJC, van den Berg LH. Accelerometry for remote monitoring of physical activity in amyotrophic lateral sclerosis: a longitudinal cohort study. J Neurol. (2019) 266:2387–95. doi: 10.1007/s00415-019-09427-5
44. Kabelac Z, Tarolli CG, Snyder C, Feldman B, Glidden A, Hsu C-Y, et al. Passive monitoring at home: a pilot study in parkinson disease. Digit Biomark. (2019) 3:22–30. doi: 10.1159/000498922
45. Lipsmeier F, Taylor KI, Kilchenmann T, Wolf D, Scotland A, Schjodt-Eriksen J, et al. Evaluation of smartphone-based testing to generate exploratory outcome measures in a phase 1 parkinson's disease clinical trial. Mov Disord. (2018) 33:1287–97. doi: 10.1002/mds.27376
46. Aminian S, Motl RW, Rowley J, Manns PJ. Management of multiple sclerosis symptoms through reductions in sedentary behaviour: protocol for a feasibility study. BMJ Open. (2019) 9:e026622. doi: 10.1136/bmjopen-2018-026622
47. Koutsouraki E, Kalatha T, Grosi E, Koukoulidis T, Michmizos D. Cognitive decline in multiple sclerosis patients. Hell J Nucl Med. (2019) 22:75–81.
48. Kalb R, Beier M, Benedict RH, Charvet L, Costello K, Feinstein A, et al. Recommendations for cognitive screening and management in multiple sclerosis care. Mult Scler J. (2018) 24:1665–80. doi: 10.1177/1352458518803785
49. Neubert T-A, Dusch M, Karst M, Beissner F. Designing a tablet-based software app for mapping bodily symptoms: usability evaluation and reproducibility analysis. Jmir Mhealth Uhealth. (2018) 6:e127. doi: 10.2196/mhealth.8409
50. Coons SJ, Eremenco S, Lundy JJ, O'Donohoe P, O'Gorman H, Malizia W. Capturing patient-reported outcome (PRO) data electronically: the past, present, and promise of ePRO measurement in clinical trials. Patient. (2015) 8:301–9. doi: 10.1007/s40271-014-0090-z
51. Barentsz MW, Wessels H, van Diest PJ, Pijnappel RM, Haaring C, van der Pol CC, et al. Tablet, web-based, or paper questionnaires for measuring anxiety in patients suspected of breast cancer: patients' preferences and quality of collected data. J Med Internet Res. (2014) 16:e239. doi: 10.2196/jmir.3578
52. Haase R, Schultheiss T, Kempcke R, Thomas K, Ziemssen T. Modern communication technology skills of patients with multiple sclerosis. Mult Scler J. (2013) 19:1240–41. doi: 10.1177/1352458512471882
53. Hassler K, Pearce KJ, Serfass TL. Comparing the efficacy of electronic-tablet to paper-based surveys for on-site survey administration. Int J Soc Res Methodol. (2018) 21:487–97. doi: 10.1080/13645579.2018.1432403
54. Midaglia L, Mulero P, Montalban X, Graves J, Hauser SL, Julian L, et al. Adherence and satisfaction of smartphone- and smartwatch-based remote active testing and passive monitoring in people with multiple sclerosis: nonrandomized interventional feasibility study. J Med Internet Res. (2019) 21:e14863. doi: 10.2196/14863
55. Haase R, Kullmann JS, Ziemssen T. Therapy satisfaction and adherence in patients with relapsing–remitting multiple sclerosis: the THEPA-MS survey. Ther Adv Neurol Diso. (2016) 9:250–63. doi: 10.1177/1756285616634247
56. Prosperini L, Fortuna D, Giannì C, Leonardi L, Marchetti MR, Pozzilli C. Home-based balance training using the wii balance board. Neurorehab Neural Repair. (2013) 27:516–25. doi: 10.1177/1545968313478484
57. Baird JF, Motl RW. Response heterogeneity with exercise training and physical activity interventions among persons with multiple sclerosis. Neurorehab Neural Repair. (2018) 33:3–14. doi: 10.1177/1545968318818904
58. Baird JF, Cederberg KLJ, Sikes EM, Silveira SL, Jeng B, Sasaki JE, et al. Physical activity and walking performance across the lifespan among adults with multiple sclerosis. Mult Scler Relat Disord. (2019) 35:36–41. doi: 10.1016/j.msard.2019.07.003
59. Schultheiß T, Kempcke R, Kratzsch F, Eulitz M, Pette M, Reichmann H, et al. Multiple-sklerose-dokumentationssystem 3D. Der Nervenarzt. (2011) 83:450–7. doi: 10.1007/s00115-011-3376-6
60. Pette M, Eulitz M. Das multiple-sklerose-dokumentationssystem MSDS diskussionsgrundlage für einen dokumentationsstandard multiple sklerose? Der Nervenarzt. (2002) 73:144–8. doi: 10.1007/s00115-001-1220-0
61. Ziemssen T, Stefano ND, Sormani MP, Wijmeersch BV, Wiendl H, Kieseier BC. Optimizing therapy early in multiple sclerosis: an evidence-based view. Mult Scler Relat Dis. (2015) 4:460–9. doi: 10.1016/j.msard.2015.07.007
62. Ziemssen T, Phillips G, Shah R, Mathias A, Foley C, Coon C, et al. Development of the multiple sclerosis (MS) early mobility impairment questionnaire (EMIQ). J Neurol. (2016) 263:1969–83. doi: 10.1007/s00415-016-8210-4
63. Ness NH, Schriefer D, Haase R, Ettle B, Cornelissen C, Ziemssen T. Differentiating societal costs of disability worsening in multiple sclerosis. J Neurol. (2020) 267:1035–42. doi: 10.1007/s00415-019-09676-4
64. Ness N-H, Haase R, Kern R, Schriefer D, Ettle B, Cornelissen C, et al. Multiple sclerosis health resource utilization survey (MS-HRS): development of an adaptive survey on the health economics' perspective on multiple sclerosis (Preprint). J Med Internet Res. (2020) 22:e17921. doi: 10.2196/preprints.17921
65. Rodriguez-Leal F, Haase R, Akgün K, Eisele J, Proschmann U, Schultheiss T, et al. Nonwalking response to fampridine in patients with multiple sclerosis in a real-world setting. Ther Adv Chronic Dis. (2019) 10:204062231983513. doi: 10.1177/2040622319835136
66. Rodriguez-Leal FA, Haase R, Thomas K, Eisele JC, Proschmann U, Schultheiss T, et al. Fampridine response in MS patients with gait impairment in a real-world setting: need for new response criteria? Mult Scler J. (2018) 24:1337–46. doi: 10.1177/1352458517720043
67. Mowry E, Bermel RA, Williams JR, Benzinger TLS, de Moor C, Fisher F, et al. Harnessing real-world data to inform decision-making: Multiple sclerosis partners advancing technology and health solutions (MS PATHS). Front Neurol. (2020) 11:632. doi: 10.3389/fneur.2020.00632
68. Kaufmann M, Haase R, Proschmann U, Ziemssen T, Akgün K. Real world lab data: patterns of lymphocyte counts in fingolimod treated patients. Front Immunol. (2018) 9:2669. doi: 10.3389/fimmu.2018.02669
69. Kaufmann M, Haase R, Proschmann U, Ziemssen T, Akgün K. Real-world lab data in natalizumab treated multiple sclerosis patients up to 6 years long-term follow up. Front Neurol. (2018) 9:1071. doi: 10.3389/fneur.2018.01071
70. Haase R, Wunderlich M, Dillenseger A, Kern R, Akgün K, Ziemssen T. Improving multiple sclerosis management and collecting safety information in the real world: the MSDS3D software approach. Expert Opin Drug Saf . (2018) 17:369–78. doi: 10.1080/14740338.2018.1437144
71. Ziemssen T, Medin J, Couto CA-M, Mitchell CR. Multiple sclerosis in the real world: a systematic review of fingolimod as a case study. Autoimmun Rev. (2017) 16:355–76. doi: 10.1016/j.autrev.2017.02.007
72. Ramirez I. Navigating the maze of requirements for obtaining approval of non-interventional studies (NIS) in the European Union. Gms Ger Medical Sci. (2015) 13:Doc21. doi: 10.3205/000225
73. Kiri VA. A pathway to improved prospective observational post-authorization safety studies. Drug Safety. (2012) 35:711–24. doi: 10.1007/BF03261968
74. Hassoun L, Eisele J, Thomas K, Ziemssen T. Hands on alemtuzumab-experience from clinical practice: whom and how to treat. Mult Scler Demyelinat Disord. (2016) 1:10. doi: 10.1186/s40893-016-0011-1
75. Thomas K, Ziemssen T. Management of fingolimod in clinical practice. Clin Neurol Neurosur. (2013) 115:S60–4. doi: 10.1016/j.clineuro.2013.09.023
76. Ziemssen T, Gass A, Wuerfel J, Bayas A, Tackenberg B, Limmroth V, et al. Design of TRUST, a non-interventional, multicenter, 3-year prospective study investigating an integrated patient management approach in patients with relapsing-remitting multiple sclerosis treated with natalizumab. Bmc Neurol. (2016) 16:98. doi: 10.1186/s12883-016-0625-0
77. Ziemssen T, Kern R, Cornelissen C. Study design of PANGAEA 2.0, a non-interventional study on RRMS patients to be switched to fingolimod. BMC Neurol. (2016) 16:129. doi: 10.1186/s12883-016-0648-6
78. Ziemssen T, Kern R, Cornelissen C. The PANGAEA study design – a prospective, multicenter, non-interventional, long-term study on fingolimod for the treatment of multiple sclerosis in daily practice. BMC Neurol. (1997) 15:93. doi: 10.1186/s12883-015-0342-0
79. Ziemssen T, Engelmann U, Jahn S, Leptich A, Kern R, Hassoun L, et al. Rationale, design, and methods of a non-interventional study to establish safety, effectiveness, quality of life, cognition, health-related and work capacity data on Alemtuzumab in multiple sclerosis patients in Germany (TREAT-MS). BMC Neurol. (2016) 16:109. doi: 10.1186/s12883-016-0629-9
80. Akgün K, Kretschmann N, Haase R, Proschmann U, Kitzler HH, Reichmann H, et al. Profiling individual clinical responses by high-frequency serum neurofilament assessment in MS. Neurol Neuroimmunol Neuroinflamm. (2019) 6:e555. doi: 10.1212/NXI.0000000000000555
81. Dirks P, Zingler V, Leemhuis J, Berthold H, Hieke-Schulz S, Wormser D, et al. Design of a non-interventional post-marketing study to assess the long-term safety and effectiveness of ocrelizumab in German real world multiple sclerosis cohorts – the CONFIDENCE study protocol. BMC Neurol. (2020) 20:95. doi: 10.1186/s12883-020-01667-7
82. Heesen C, Haase R, Melzig S, Poettgen J, Berghoff M, Paul F, et al. Perceptions on the value of bodily functions in multiple sclerosis. Acta Neurol Scand. (2018) 137:356–62. doi: 10.1111/ane.12881
83. Ziemssen T, Piani-Meier D, Bennett B, Johnson C, Tinsley K, Trigg A, et al. A physician-completed digital tool for evaluating disease progression (multiple sclerosis progression discussion tool): validation study. J Med Internet Res. (2020) 22:e16932. doi: 10.2196/16932
84. Warnke C, Hartung H-P. Big data in MS-What can we learn from large international observational studies such as MSBase? Mult Scler. (2019) 26:4–5. doi: 10.1177/1352458519868982
85. Trojano M, Tintore M, Montalban X, Hillert J, Kalincik T, Iaffaldano P, et al. Treatment decisions in multiple sclerosis — insights from real-world observational studies. Nat Rev Neurol. (2017) 13:105–18. doi: 10.1038/nrneurol.2016.188
86. Storelli L, Rocca MA, Pantano P, Pagani E, Stefano ND, Tedeschi G, et al. MRI quality control for the Italian neuroimaging network initiative: moving towards big data in multiple sclerosis. J Neurol. (2019) 266:2848–58. doi: 10.1007/s00415-019-09509-4
87. Ludwin SK, Murray TJ. Dilemmas in medical ethics in the age of big data. Mult Scler J. (2017) 23:1306–8. doi: 10.1177/1352458517722056
Keywords: multiple sclerosis, documentation, digital patient management, post-authorization safety study, MSDS3D
Citation: Ziemssen T, Kern R, Voigt I and Haase R (2020) Data Collection in Multiple Sclerosis: The MSDS Approach. Front. Neurol. 11:445. doi: 10.3389/fneur.2020.00445
Received: 15 December 2019; Accepted: 27 April 2020;
Published: 16 June 2020.
Edited by:
Marcello Moccia, University of Naples Federico II, ItalyReviewed by:
Viktor Von Wyl, University of Zurich, SwitzerlandCopyright © 2020 Ziemssen, Kern, Voigt and Haase. This is an open-access article distributed under the terms of the Creative Commons Attribution License (CC BY). The use, distribution or reproduction in other forums is permitted, provided the original author(s) and the copyright owner(s) are credited and that the original publication in this journal is cited, in accordance with accepted academic practice. No use, distribution or reproduction is permitted which does not comply with these terms.
*Correspondence: Tjalf Ziemssen, dGphbGYuemllbXNzZW5AdW5pa2xpbmlrdW0tZHJlc2Rlbi5kZQ==
Disclaimer: All claims expressed in this article are solely those of the authors and do not necessarily represent those of their affiliated organizations, or those of the publisher, the editors and the reviewers. Any product that may be evaluated in this article or claim that may be made by its manufacturer is not guaranteed or endorsed by the publisher.
Research integrity at Frontiers
Learn more about the work of our research integrity team to safeguard the quality of each article we publish.