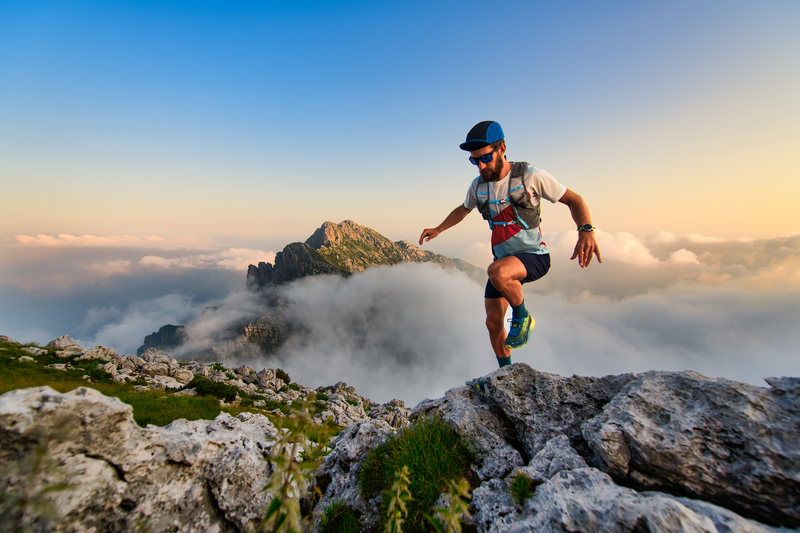
94% of researchers rate our articles as excellent or good
Learn more about the work of our research integrity team to safeguard the quality of each article we publish.
Find out more
MINI REVIEW article
Front. Neurol. , 12 April 2019
Sec. Neurodegeneration
Volume 10 - 2019 | https://doi.org/10.3389/fneur.2019.00350
This article is part of the Research Topic Biomarkers and Clinical Indicators in Motor Neuron Disease View all 38 articles
Amyotrophic lateral sclerosis (ALS) is the most common adult onset motor neuron disease with no effective disease modifying therapies at present. Spinal cord degeneration is a hallmark feature of ALS, highlighted in the earliest descriptions of the disease by Lockhart Clarke and Jean-Martin Charcot. The anterior horns and corticospinal tracts are invariably affected in ALS, but up to recently it has been notoriously challenging to detect and characterize spinal pathology in vivo. With recent technological advances, spinal imaging now offers unique opportunities to appraise lower motor neuron degeneration, sensory involvement, metabolic alterations, and interneuron pathology in ALS. Quantitative spinal imaging in ALS has now been used in cross-sectional and longitudinal study designs, applied to presymptomatic mutation carriers, and utilized in machine learning applications. Despite its enormous clinical and academic potential, a number of physiological, technological, and methodological challenges limit the routine use of computational spinal imaging in ALS. In this review, we provide a comprehensive overview of emerging spinal cord imaging methods and discuss their advantages, drawbacks, and biomarker potential in clinical applications, clinical trial settings, monitoring, and prognostic roles.
Amyotrophic lateral sclerosis (ALS) is a relentlessly progressive neurodegenerative disorder. Anterior horn pathology and corticospinal tract degeneration has been identified as a core feature of ALS since the earliest descriptions of the condition (1, 2). Despite repeated attempts to detect and characterize spinal cord pathology in vivo (3), technological constraints have traditionally precluded reliable quantitative spinal imaging in ALS. Due to the plethora of methodological challenges, such as the small cross-sectional area of the human spinal cord, respiratory, and cardiac movement effects, the overwhelming majority of imaging studies have focused on cerebral alterations in ALS (4).
The diagnosis of ALS is primarily clinical and requires the careful exclusion of ALS-mimics (5). Given the heterogeneity of clinical presentations and the prevalence of atypical phenotypes, diagnostic delay in ALS is not uncommon, and the average period between symptom onset and definite diagnosis is ~12 months worldwide (6). The median survival from symptom onset ranges from 20 to 48 months (7–9). Progression rates in ALS show considerable variation, and prognosis depends on age at onset, region of onset, co-morbid cognitive impairment, nutritional status, and certain genotypes are associated with faster progression (10–16). Given the considerable clinical, cognitive, and genetic heterogeneity of ALS, there is an unmet need for early diagnostic biomarkers to aid patient stratification into specific phenotypes (17). Clinical trials of ALS continue to rely on survival, functional scores and respiratory measures as outcome measures despite the potential of candidate imaging markers (18).
Magnetic resonance imaging (MRI) not only contributed to the characterization of ALS-associated cerebral changes, it has also contributed important pathophysiological insights, such as the role of inflammation (19), patterns of spread (20, 21), inhibitory dysfunction (22, 23), and network-wise propagation (24, 25). In addition to describing unifying disease-associated signatures, imaging studies of ALS have gradually characterized the features of specific genotypes (26, 27), phenotypes (28, 29), the substrate of cognitive and extra-pyramidal impairments (30), as well as presymptomatic (31) and longitudinal changes (32). Despite the momentous advances however, the overwhelming majority of imaging studies in ALS remain cerebral, overlooking a disease-defining site of ALS pathology; the spinal cord (3).
One of the key challenges of spinal cord imaging stems from its elongated dimensions, small cross-sectional area in the axial plane coupled with long sagittal and coronal expansion (33). Furthermore, the cord is surrounded by tissues that have very different magnetic susceptibility profiles and is it subject to both direct (cardiac and respiratory) and fluid-mediated [cerebrospinal fluid (CSF)] movement effects. The main challenges of quantitative spinal cord imaging include (i) partial volume effects, (ii) an inhomogeneous magnetic field environment, and (iii) physiological and patient motion (34).
Partial volume refers to scenarios where different tissues contribute to the same voxel. In spinal cord imaging this occurs when a voxel is at the CSF/white matter, white matter/gray matter, CSF/vascular, white matter/vascular interfaces. Signals from different tissue densities with different amounts of spins contribute to the total MR signal in these voxels, which results in indistinct tissue-boundaries. Partial volume effects can be reduced by increasing the spatial resolution, but this in turn results in lower signal-to-noise (SNR) and contrast-to-noise ratios (CNR). Magnetic fields strengths of three or seven Tesla compared to conventional 1.5 Tesla platforms (35–38), higher number of phased-array coils with parallel imaging (35, 38, 39), and corrections for physiological motion improves spatial resolution, SNR, and CNR (35, 38, 39).
Due to its proximity to the lungs and the heart, almost the entire spinal cord undergoes repetitive displacement due to respiration, CSF, and cardiac pulsation (40–43). The movement of the human spinal cord linearly increases caudally with distance from the head. The available literature suggest that physiological anterior-posterior (A-P) cord movement (0.60 ± 0.34 mm) exceeds those observed in superior-inferior (SI) (0.4 ± 0.1 mm) and right-left (RL) direction (0.17 ± 0.09 mm) (44, 45). Spinal imaging is also susceptible to movement artifacts from swallowing and patient movements during long MR acquisitions which can create ghosting artifacts (42, 46). By “gating” the acquisition, i.e., synchronizing with the respiratory or cardiac cycles, the effect of periodical movements can be significantly reduced (38, 39, 47). Motion artifacts can also be reduced using “saturation bands” that cover the esophagus, chest, and abdomen, by attenuating signals from moving structures so that it does not corrupt the signal from the spinal cord itself. Velocity compensating gradient sequences and signal averaging across multiple phases of motion can also be applied to minimize motion artifacts. Reducing acquisition time by using fast sequences, i.e., fast-spin-echo, parallel imaging that increases acquisition speed by factors from 1.5 to 3, i.e., SENSitivity Encoding/GeneRalized Autocalibration Partial Parallel Acquisition-type reconstructions, partial Fourier imaging, reducing the size of the phase-encoded direction, and decreasing the k-space matrix size effectively reduce both physiological and subject motion effects (48–53). MRI compatible cervical collars, which minimize involuntary neck movements, may also reduce movement artifacts (46). Co-registration of all data when dealing with multiple series acquisition, e.g., diffusion tensor imaging (DTI) and functional MRI (fMRI), can also be performed to limit the inconsistency in derived maps (54, 55).
The spinal canal is surrounded by bones, ligaments, disks, arteries, and venous plexi. Its proximity to the esophagus, mediastinum, and the lungs, each containing various amounts of air, create a challenging scanning environment. Adipose tissue, bone, and air have different magnetic susceptibility profiles, and respiration-induced B0 field fluctuations (43) also contribute to the inhomogeneity of the magnetic field around the spinal cord, resulting in geometric distortions and signal intensity loss (56). To some extent, these artifacts can be counteracted with “shimming.” Shimming aims at compensating for field inhomogeneities by creating an auxiliary magnetic field via shim coils (57). While shimming improves overall field homogeneity, it is limited to smooth variations across larger regions and cannot fully compensate for small, and localized field variations, such as those observed at cartilaginous discs between the vertebral bodies. Echo planar imaging sequences, such as DTI, are particularly sensitive to geometric distortions around vertebral disks. In addition to shimming, parallel imaging, and careful slices positioning may reduce magnetic field inhomogeneity, i.e., slices centered in the middle of each vertebral body and perpendicular to the spinal cord (38, 47, 58). The specific geometry of the magnetic field inhomogeneities should be considered in order to correct for its effect (59–61).
The role of conventional spinal MRI in ALS is to rule of alternative structural, inflammatory or neoplastic pathologies which may result in a combination of upper and lower motor neuron involvement mimicking ALS (62). Compressive myelopathies and radiculopathies are relatively common and early, predominantly lower limb presentations of ALS are sometimes attributed to these radiological findings resulting in laminectomies and other invasive procedures (63, 64). Conventional, clinical spinal sequences are typically only qualitatively interpreted without specific measurements. The majority of clinical spinal scans in ALS are reported as normal, but non-specific signs such as high signal along the corticospinal tracts are occasionally observed on T2-weighted imaging (65–67).
In sharp contrast with clinical sequences, advanced quantitative spinal protocols allow for the detailed and quantitative characterization of spinal gray and white matter integrity (38, 47, 58, 68). These protocols provide high resolution, high SNR, and high CNR images compared to standard clinical sequences. Furthermore, purpose-designed spinal protocols are based on mathematical MR signal modeling (e.g., diffusion-based methods, quantitative magnetization transfer, and MR spectroscopy) and the derived outputs can be quantitatively interpreted to provide accurate, motion-corrected white, and gray matter metrics.
Gross axonal and gray matter loss have traditionally been estimated by measuring spinal cord cross-sectional areas at specific levels and interpreted as a proxy of atrophy in the context of reference normative values (69–72). The “cross-sectional approach” consists of estimating a mean cord cross-sectional area over a representative number of slices at a given vertebral level (70, 71, 73, 74), which can be relatively easily calculated from conventional MR sequences such as T1- or T2-weighted images. A variety of indexes, such as A-P dimension, L-R width, and radial distance can be derived from the cross-sectional area (CSA) approach. These measures reflect on different aspects of pathology, such as global vs. regional, lateral vs. anterior tissue loss, and are often interpreted as predominantly motor or sensory involvement (70, 75). More specific gray and white matter measures can be derived from higher resolution images followed by tissue-type segmentation methods (72, 76, 77). Novel quantitative approaches, such as tensor based morphometry and surface based-morphometry permit a more fine-grained characterization of cord topography and the definition of disease-associated signatures (74, 78). Recent studies demonstrated that spinal cord atrophy, especially gray matter atrophy, correlates with disability and disease progression and may be predictive of respiratory failure and of survival in ALS (58, 70, 72, 73, 79). The main findings of structural spinal cord studies are summarized in Table 1.
Table 1. Quantitative spinal imaging studies in ALS, ALS, amyotrophic lateral sclerosis; ALSFRS-r, the revised ALS functional scale; FA, fractional anisotropy; CSA, cross-sectional area; CST, corticospinal tract; FVC, force vital capacity; ihMT, inhomogeneous magnetization transfer; ihMTR, inhomogeneous magnetization transfer ratio; MD, mean diffusivity; MT, magnetization transfer; MTR, magnetization transfer ratio; MMT, manual muscle testing; SC, spinal cord; SOD1, superoxide dismutase 1 gene.
Diffusion weighted imaging (DWI) relies on the evaluation of water diffusion in CNS tissues and is primarily used to characterize white matter integrity (90, 91). DWI-derived metrics, such as axial diffusivity (AD), mean diffusivity (MD), fractional anisotropy (FA), radial diffusivity (RD) enable the quantitative characterization of white matter integrity. Novel high-directional approaches, such as high-angular resolution diffusion imaging (92), q-ball imaging (93), diffusion kurtosis imaging (94), diffusion basis spectrum imaging (DBSI) (95) are particularly well-suited to assess the integrity of crossing-fibers (96, 97). Emerging diffusion techniques such as neurite orientation dispersion and density imaging (NODDI) (98) help to estimate the microstructural attributes of dendrites and axons (99). While in ALS NODDI has been primarily used in cerebral studies in ALS (100, 101), it also has been also piloted in spinal applications (90, 102). Specific DTI indices (AD, RD) have been associated with specific pathological processes, such as axonal (103, 104) vs. myelin-related (105, 106) degeneration, but this interpretation is likely to be simplistic, as DTI measures are affected by axonal density, axonal diameter, myelin thickness and fiber orientation, fiber coherence, and acquisition parameters. DTI has been extensively used to study cerebral changes in ALS and describe phenotype-associated (107), genotype-specific (27), presymptomatic (32), and longitudinal white matter changes in the brain (81). In contrast to the plethora of cerebral DTI studies, relatively few spinal DTI studies have been published in ALS to date (58, 69, 73, 80–82, 85). These have consistently highlighted both motor and sensory tract alterations (Table 1).
Hydrogen nuclei linked to macromolecules such as the proteins and lipids of the myelin sheet have an extremely short T2 signal. While these macromolecules are not directly detectable by standard MRI sequences, magnetization transfer (MT) imaging enables the characterization of these structures. Macromolecular spins can be saturated using an off-resonance RF pulse, then the magnetization transfer between bound and free pools can be measured (108). Magnetization transfer occurs by means of cross relaxation processes, such as dipole-dipole interactions and chemical exchange. Magnetization transfer ratio (MTR) is calculated as the percentage difference of MT images with macromolecules signal saturation and one without. MTR enables inferences on myelin content, axonal count, and density as shown by three MS histological studies, and has been used extensively to assess demyelination, remyelination, and degeneration in MS (109–111). Conversely, relatively few studies have used cerebral MT imaging in ALS, and the majority of these focused on corticospinal tract alterations (112–115). Relatively few studies evaluated spinal MT changes in ALS, but they have shown progressive reduction overt time and correlation with muscle weakness (58, 69, 73). The key findings of spinal MT imaging studies in ALS and associated technical challenges are summarized in Tables 1, 2.
Table 2. The advantages and methodological challenges associated with specific spinal imaging techniques.
Inhomogeneous magnetization transfer (ihMT) imaging is a novel method (116, 117), which allows the unprecedented characterization of myelin integrity, by isolating key myelin components from the broader macromolecular pool. ihMT shows unparalleled potential to detect and quantify demyelination (118) and may be adapted to spinal applications. ihMT imaging has already been applied to ALS cohorts and demonstrated significant correlation with muscle strength and disability profiles (58).
Magnetic resonance spectroscopy (MRS) is well-established, non-invasive imaging tool which provides neurochemical insights based on the concentration and relaxation profile of specific metabolites in cerebral and spinal tissues. MRS has been extensively used in cerebral studies of ALS (119), used to assess the therapeutic effect of Riluzole (120, 121), and also used to study brainstem metabolic alterations (122). Cross-sectional and longitudinal (123), single voxel and whole brain multi-voxel studies have both contributed to our understanding of ALS pathophysiology (124). The main targets of proton spectroscopy (1H-MRS) include the following metabolites; N-Acetyl Aspartate (NAA), creatine (Cr), choline (Cho), and myo-Inositol (Myo). These metabolites are typically associated with neuronal integrity/viability (NAA), tissue energy metabolism (Cr), membrane integrity (Cho), and glial function (Myo). (125). Relatively few studies have used 1H-MRS to characterize metabolic changes at the spinal level, and the majority of these studies focused on multiple sclerosis (126, 127) MRS however seems particularly applicable to ALS cohorts, where it promises the characterization of presymptomatic changes and by including both the upper and lower motor components of the motor system, it has led to particularly significant clinico-radiological correlations (31, 83, 84). For the contribution of MRS studies to ALS research and specific methodological considerations please see Tables 1, 2.
Functional MRI (fMRI) detects local variations in blood oxygenation level-dependent MR signal at rest and during activation paradigms (128). FMRI has been extensively applied to ALS cohorts to describe network changes and assess altered activation patterns when performing motor or cognitive tasks (129–131). Following decades of successful cerebral studies, the first spinal fMRI studies have now been published (55, 132). Emerging spinal cord fMRI studies in healthy controls provide proof of feasibility and the first studies using spinal fMRI in neurological conditions are underway (133).
Quantitative spinal MRI studies in ALS have consistently detected corticospinal tract and anterior horns degeneration and changes correlated with functional disability (36, 58, 80, 82, 85). Segmental spinal cord atrophy was not only linked to muscle weakness (58, 70, 88), but also to electrophysiological markers such as transracial magnetic stimulation (TMS) and motor evoked potentials (69). Two studies have demonstrated that both white and gray matter atrophy contributes to global cord atrophy in ALS (58, 72), but a recent study indicates that cord atrophy in ALS may be predominantly driven by anterior horn degeneration (72), confirming the role of spinal MRI as a putative LMN marker. DTI and MTR indices of the corticospinal tract (CST) correlated with TMS facilitation motor thresholds, a functional parameter that reflects pyramidal tract integrity.
In contrast to the plethora of longitudinal cerebral studies in ALS (21), relatively few longitudinal spinal studies are available to demonstrate that spinal MRI metrics can track subtle progressive changes over time (73, 81, 87, 89). These longitudinal studies captured decreasing CST MTR and progressive cord atrophy (73, 87) While some longitudinal studies also captured progressive DTI alterations (81), other studies did not (73). Some studies suggest that CSA estimates may be more reliable markers of longitudinal cord pathology than MTR or DTI metrics (73, 87). Progressive cord atrophy not only mirrors clinical progression, but early cervical cord atrophy is thought to predict respiratory dysfunction in ALS (89, 134). Furthermore, spinal MRI metrics may be superior predictive indicators of survival than clinical measures (79). Given the scarcity of longitudinal spinal imaging studies in ALS, it remains to be established which imaging metrics capture early ALS-associated changes, therefore may be used in diagnostic applications, and which metrics can track changes in the later stages making them suitable as monitoring markers.
Several spinal MRI studies (58, 69) have captured dorsal column degeneration using DTI, MT, and ihMT imaging, and one study demonstrated progressive sensory tract degeneration over time (135). Dorsal column pathology can be detected relatively soon after symptoms onset, which suggests that sensory involvement is a core and relatively early feature of ALS. Combined spinal DTI and neurophysiology studies have also confirmed considerable sensory pathway degeneration in ALS patients without sensory symptoms (86). The combined MRI-neurophysiology approach revealed sub-clinical sensory deficits in 85% of ALS patients. These findings suggest that sensory dysfunction may have been underestimated by previous studies and that sensory afferent pathways may be affected early in the course of ALS and are important facets of ALS pathogenesis (69, 86). In contrast to longitudinal cerebral studies (4, 32), longitudinal spinal studies suggest that dorsal column metrics (73), and CST DTI indices (87) may be relatively constant (135).
1H-MRS studies in ALS have shown reduced NAA/Cr and NAA/Myo ratios at the C2 vertebral level (31, 83, 84). One spinal MRS study captured reduced NAA/Myo and NAA/Cr ratios in presymptomatic superoxide dismutase 1 gene (SOD1+) carriers (31). In addition to group-level differences in symptomatic and presymptomatic ALS cohorts, NAA/Myo and NAA/Cho reductions correlate with force vital capacity (FVC) and revised ALS functional scale (ALSFRS-r) and inversely correlated to the rates of decline (31, 83, 84).
Existing spinal studies in ALS indicate that it is possible to detect disease-specific imaging signatures at a group level, and emerging machine-learning studies (76) have demonstrated that it may be possible to classify individual scans into “ALS” and “Healthy” groups. Despite the pioneering studies however, it is clear that spinal imaging lags behind cerebral imaging. Cerebral imaging has shown that phenotype and genotype specific patterns can be detected, multi-time point longitudinal studies have shown divergent rates of gray and white matter degeneration, studies have been validated by post mortem examination and robust multi-site studies have also been published (136). It is likely that improved coil designs with higher number of phased-array elements, new generation scanners with higher gradients optimized for advanced diffusion-weighted imaging, ultra-high filed platforms with superior spatial resolution, and SNR, spinal imaging will contribute unprecedented insights in ALS. It is conceivable that spinal imaging will contribute to the longstanding debate about dying back and dying forward, and ALS being a primarily spinal vs. cerebral disease. Spinal imaging provides a unique opportunity to appraise both lower and upper motor neuron degeneration. It is also likely that imaging sequences currently primarily used in cerebral imaging in ALS such as resting state fMRI, task-based fMRI, quantitative susceptibility weighted imaging, presymptomatic imaging, texture analyses, and post mortem imaging will filter down to spinal applications. Data-sharing initiatives, cross-platform harmonization, inclusion of upper motor neuron (UMN) and lower motor neuron (LMN) predominant ALS cohorts, correlations with advanced neurophysiological techniques are trends of ALS imaging which is likely to be adopted in spinal studies. One of the key ambitions of multiparametric spinal imaging is to overcome the methodological challenges of thoracic and lumbar imaging.
The momentous advances in spinal imaging in ALS suggest the spinal metrics may soon be used as validated diagnostic, monitoring, and prognostic markers, contributing both to individualized patient care and pharmacological trials.
ME, GQ, PB, and P-FP contributed equally to the conceptualization, drafting, and revision of the manuscript.
Peter Bede is supported by the Health Research Board (HRB—Ireland; HRB EIA-2017-019), the Iris O'Brien Foundation, the Irish Institute of Clinical Neuroscience IICN—Novartis Ireland Research Grant, and the Research Motor Neuron (RMN-Ireland) Foundation. P-FP is supported by the French Association for Myopathies (AFM-Telethon), the Institute for Research in Brain and Spinal Cord (IRME) the French Association for Research in ALS (ARSLA) and the Target ALS Foundation.
The authors declare that the research was conducted in the absence of any commercial or financial relationships that could be construed as a potential conflict of interest.
1H-MRS, proton spectroscopy; A-P, anterior-posterior; AD, axial diffusivity; ALS, Amyotrophic lateral sclerosis; ALSFRS-R, revised ALS functional scale; Cho, choline; CNR, contrast-to-noise ratio; Cr, creatine; CSA, cross-sectional area; CSF, cerebrospinal flood; CST, corticospinal tract; DTI, diffusion tensor imaging; FA, fractional anisotropy; fMRI, functional MRI; ihMT, Inhomogeneous magnetization transfer; LMN, lower motor neuron; MD, mean diffusivity; MRI, Magnetic resonance imaging; MRS, Magnetic resonance spectroscopy; MT, Magnetization transfer; MTR, Magnetization transfer ratio; Myo, myo-Inositol; NAA, N-Acetyl Aspartate; NODDI, neurite orientation dispersion and density imaging; RD, radial diffusivity; RL, right-left; SNR, signal-to-noise ratio; TMS, transracial magnetic stimulation; SOD1, superoxide dismutase 1 gene; SOD1+, presymptomatic superoxide dismutase 1 gene.
1. Clarke JL, Jackson JH. On a case of muscular atrophy, with disease of the spinal cord and medulla oblongata. Med Chir Trans. (1867) 50:489–98. doi: 10.1177/095952876705000122
2. Charcot J, Joffroy A. Deux cas d'atrophie musculaire progressive avec lésions de la substance grise et de faisceaux antérolatéraux de la moelle épinière. Arch Physiol Norm Pathol. (1869) 1:354–7.
3. Bede P, Bokde AL, Byrne S, Elamin M, Fagan AJ, Hardiman O. Spinal cord markers in ALS: diagnostic and biomarker considerations. Amyotroph Lateral Scler. (2012) 13:407–15. doi: 10.3109/17482968.2011.649760
4. Bede P, Querin G, Pradat PF. The changing landscape of motor neuron disease imaging: the transition from descriptive studies to precision clinical tools. Curr Opin Neurol. (2018) 31:431–8. doi: 10.1097/WCO.0000000000000569
5. Andersen PM, Abrahams S, Borasio GD, de Carvalho M, Chio A, Van Damme P, et al. EFNS guidelines on the clinical management of amyotrophic lateral sclerosis (MALS)–revised report of an EFNS task force. Eur J Neurol. (2012) 19:360–75. doi: 10.1111/j.1468-1331.2011.03501.x
6. Mitchell JD, Callagher P, Gardham J, Mitchell C, Dixon M, Addison-Jones R, et al. Timelines in the diagnostic evaluation of people with suspected amyotrophic lateral sclerosis (ALS)/motor neuron disease (MND)–a 20-year review: can we do better? Amyotroph Lateral Scler. (2010) 11:537–41. doi: 10.3109/17482968.2010.495158
7. Marin B, Boumediene F, Logroscino G, Couratier P, Babron MC, Leutenegger AL, et al. Variation in worldwide incidence of amyotrophic lateral sclerosis: a meta-analysis. Int J Epidemiol. (2017) 46:57–74. doi: 10.1093/ije/dyw061
8. Chio A, Logroscino G, Traynor BJ, Collins J, Simeone JC, Goldstein LA, et al. Global epidemiology of amyotrophic lateral sclerosis: a systematic review of the published literature. Neuroepidemiology. (2013) 41:118–30. doi: 10.1159/000351153
9. Gordon PH, Salachas F, Bruneteau G, Pradat PF, Lacomblez L, Gonzalez-Bermejo J, et al. Improving survival in a large French ALS center cohort. J Neurol. (2012) 259:1788–92. doi: 10.1007/s00415-011-6403-4
10. Elamin M, Phukan J, Bede P, Jordan N, Byrne S, Pender N, et al. Executive dysfunction is a negative prognostic indicator in patients with ALS without dementia. Neurology. (2011) 76:1263–9. doi: 10.1212/WNL.0b013e318214359f
11. Byrne S, Elamin M, Bede P, Shatunov A, Walsh C, Corr B, et al. Cognitive and clinical characteristics of patients with amyotrophic lateral sclerosis carrying a C9orf72 repeat expansion: a population-based cohort study. Lancet Neurol. (2012) 11:232–40. doi: 10.1007/978-0-387-88555-1
12. Westeneng HJ, Debray TPA, Visser AE, van Eijk RPA, Rooney JPK, Calvo A, et al. Prognosis for patients with amyotrophic lateral sclerosis: development and validation of a personalised prediction model. Lancet Neurol. (2018) 17:423–33. doi: 10.1016/S1474-4422(18)30089-9
13. Chio A, Logroscino G, Hardiman O, Swingler R, Mitchell D, Beghi E, et al. Prognostic factors in ALS: a critical review. Amyotroph Lateral Scler. (2009) 10:310–23. doi: 10.3109/17482960802566824
14. Pupillo E, Messina P, Logroscino G, Beghi E. Long-term survival in amyotrophic lateral sclerosis: a population-based study. Ann Neurol. (2014) 75:287–97. doi: 10.1002/ana.24096
15. Yunusova Y, Plowman EK, Green JR, Barnett C, Bede P. Clinical measures of bulbar dysfunction in ALS. Front Neurol. (2019) 10:106. doi: 10.3389/fneur.2019.00106
16. Christidi F, Karavasilis E, Rentzos M, Kelekis N, Evdokimidis I, Bede P. Clinical and radiological markers of extra-motor deficits in amyotrophic lateral sclerosis. Front Neurol. (2018) 9:1005. doi: 10.3389/fneur.2018.01005
17. Pradat PF, Dib M. Biomarkers in amyotrophic lateral sclerosis: facts and future horizons. Mol Diagn Ther. (2009) 13:115–25. doi: 10.1007/BF03256320
18. Mitsumoto H, Brooks BR, Silani V. Clinical trials in amyotrophic lateral sclerosis: why so many negative trials and how can trials be improved? Lancet Neurol. (2014) 13:1127–38. doi: 10.1016/S1474-4422(14)70129-2
19. Evans MC, Couch Y, Sibson N, Turner MR. Inflammation and neurovascular changes in amyotrophic lateral sclerosis. Mol Cell Neurosci. (2013) 53:34–41. doi: 10.1016/j.mcn.2012.10.008
20. Bede P, Hardiman O. Longitudinal structural changes in ALS: a three time-point imaging study of white and gray matter degeneration. Amyotroph Lateral Scler Frontotemporal Degener. (2018) 19:232–41. doi: 10.1080/21678421.2017.1407795
21. Menke RAL, Proudfoot M, Talbot K, Turner MR. The two-year progression of structural and functional cerebral MRI in amyotrophic lateral sclerosis. Neuroimage Clin. (2018) 17:953–61. doi: 10.1016/j.nicl.2017.12.025
22. Goodin DS, Rowley HA, Olney RK. Magnetic resonance imaging in amyotrophic lateral sclerosis. Ann Neurol. (1988) 23:418–20. doi: 10.1002/ana.410230424
23. Bede P, Hardiman O. Lessons of ALS imaging: pitfalls and future directions - a critical review. Neuroimage Clin. (2014) 4:436–43. doi: 10.1016/j.nicl.2014.02.011
24. Verstraete E, van den Heuvel MP, Veldink JH, Blanken N, Mandl RC, Hulshoff Pol HE, et al. Motor network degeneration in amyotrophic lateral sclerosis: a structural and functional connectivity study. PLoS ONE. (2010) 5:e13664. doi: 10.1371/journal.pone.0013664
25. Bede P, Omer T, Finegan E, Chipika RH, Iyer PM, Doherty MA, et al. Connectivity-based characterisation of subcortical grey matter pathology in frontotemporal dementia and ALS: a multimodal neuroimaging study. Brain Imaging Behav. (2018) 12:1696–707. doi: 10.1007/s11682-018-9837-9
26. Turner MR, Hammers A, Al-Chalabi A, Shaw CE, Andersen PM, Brooks DJ, et al. Distinct cerebral lesions in sporadic and 'D90A' SOD1 ALS: studies with [11C]flumazenil PET. Brain. (2005) 128:1323–9. doi: 10.1093/brain/awh509
27. Bede P, Bokde ALW, Byrne S, Elamin M, McLaughlin RL, Kenna K, et al. Multiparametric MRI study of ALS stratified for the C9orf72 genotype. Neurology. (2013) 81:361–9. doi: 10.1093/med/9780199696277.003.0005
28. Lomen-Hoerth C. Clinical phenomenology and neuroimaging correlates in ALS-FTD. J Mol Neurosci. (2011) 45:656–62. doi: 10.1007/s12031-011-9636-x
29. Bede P, Bokde A, Elamin M, Byrne S, McLaughlin RL, Jordan N, et al. Grey matter correlates of clinical variables in amyotrophic lateral sclerosis (ALS): a neuroimaging study of ALS motor phenotype heterogeneity and cortical focality. J Neurol Neurosurg Psychiatry. (2013) 84:766–73. doi: 10.1136/jnnp-2012-302674
30. Feron M, Couillandre A, Mseddi E, Termoz N, Abidi M, Bardinet E, et al. Extrapyramidal deficits in ALS: a combined biomechanical and neuroimaging study. J Neurol. (2018) 265:2125–36. doi: 10.1007/s00415-018-8964-y
31. Carew JD, Nair G, Andersen PM, Wuu J, Gronka S, Hu X, et al. Presymptomatic spinal cord neurometabolic findings in SOD1-positive people at risk for familial ALS. Neurology. (2011) 77:1370–5. doi: 10.1212/WNL.0b013e318231526a
32. Schuster C, Elamin M, Hardiman O, Bede P. Presymptomatic and longitudinal neuroimaging in neurodegeneration–from snapshots to motion picture: a systematic review. J Neurol Neurosurg Psychiatry. (2015) 86:1089–96. doi: 10.1136/jnnp-2014-309888
33. Fradet L, Arnoux PJ, Ranjeva JP, Petit Y, Callot V. Morphometrics of the entire human spinal cord and spinal canal measured from in vivo high-resolution anatomical magnetic resonance imaging. Spine. (2014) 39:E262–9. doi: 10.1097/BRS.0000000000000125
34. Stroman PW, Wheeler-Kingshott C, Bacon M, Schwab JM, Bosma R, Brooks J, et al. The current state-of-the-art of spinal cord imaging: methods. Neuroimage. (2014) 84:1070–81. doi: 10.1016/j.neuroimage.2013.04.124
35. Zhao W, Cohen-Adad J, Polimeni JR, Keil B, Guerin B, Setsompop K, et al. Nineteen-channel receive array and four-channel transmit array coil for cervical spinal cord imaging at 7T. Magn Reson Med. (2014) 72:291–300. doi: 10.1002/mrm.24911
36. Cohen-Adad J, Zhao W, Keil B, Ratai EM, Triantafyllou C, Lawson R, et al. 7-T MRI of the spinal cord can detect lateral corticospinal tract abnormality in amyotrophic lateral sclerosis. Muscle Nerve. (2013) 47:760–2. doi: 10.1002/mus.23720
37. Atassi N, Xu M, Triantafyllou C, Keil B, Lawson R, Cernasov P, et al. Ultra high-field (7tesla) magnetic resonance spectroscopy in amyotrophic lateral sclerosis. PLoS ONE. (2017) 12:e0177680. doi: 10.1371/journal.pone.0177680
38. Massire A, Rasoanandrianina H, Taso M, Guye M, Ranjeva JP, Feiweier T, et al. Feasibility of single-shot multi-level multi-angle diffusion tensor imaging of the human cervical spinal cord at 7T. Magn Reson Med. (2018) 80:947–57. doi: 10.1002/mrm.27087
39. Taso M, Le Troter A, Sdika M, Ranjeva JP, Guye M, Bernard M, et al. Construction of an in vivo human spinal cord atlas based on high-resolution MR images at cervical and thoracic levels: preliminary results. MAGMA. (2014) 27:257–67. doi: 10.1007/s10334-013-0403-6
40. Kharbanda HS, Alsop DC, Anderson AW, Filardo G, Hackney DB. Effects of cord motion on diffusion imaging of the spinal cord. Magn Reson Med. (2006) 56:334–9. doi: 10.1002/mrm.20959
41. Clark CA, Werring DJ, Miller DH. Diffusion imaging of the spinal cord in vivo: estimation of the principal diffusivities and application to multiple sclerosis. Magn Reson Med. (2000) 43:133–8. doi: 10.1002/(SICI)1522-2594(200001)43:1<133::AID-MRM16>3.0.CO;2-X
42. Verma T, Cohen-Adad J. Effect of respiration on the B0 field in the human spinal cord at 3T. Magn Reson Med. (2014) 72:1629–36. doi: 10.1002/mrm.25075
43. Vannesjo SJ, Miller KL, Clare S, Tracey I. Spatiotemporal characterization of breathing-induced B0 field fluctuations in the cervical spinal cord at 7T. Neuroimage. (2018) 167:191–202. doi: 10.1016/j.neuroimage.2017.11.031
44. Figley CR, Stroman PW. Investigation of human cervical and upper thoracic spinal cord motion: implications for imaging spinal cord structure and function. Magn Reson Med. (2007) 58:185–9. doi: 10.1002/mrm.21260
45. Mikulis DJ, Wood ML, Zerdoner OA, Poncelet BP. Oscillatory motion of the normal cervical spinal cord. Radiology. (1994) 192:117–21. doi: 10.1148/radiology.192.1.8208922
46. Yiannakas MC, Kearney H, Samson RS, Chard DT, Ciccarelli O, Miller DH, et al. Feasibility of grey matter and white matter segmentation of the upper cervical cord in vivo: a pilot study with application to magnetisation transfer measurements. Neuroimage. (2012) 63:1054–9. doi: 10.1016/j.neuroimage.2012.07.048
47. Cohen-Adad J, El Mendili MM, Lehericy S, Pradat PF, Blancho S, Rossignol S, et al. Demyelination and degeneration in the injured human spinal cord detected with diffusion and magnetization transfer MRI. Neuroimage. (2011) 55:1024–33. doi: 10.1016/j.neuroimage.2010.11.089
48. Jaermann T, Crelier G, Pruessmann KP, Golay X, Netsch T, van Muiswinkel AM, et al. SENSE-DTI at 3 T. Magn Reson Med. (2004) 51:230–6. doi: 10.1002/mrm.10707
49. Glockner JF, Hu HH, Stanley DW, Angelos L, King K. Parallel MR imaging: a user's guide. Radiographics. (2005) 25:1279–97. doi: 10.1148/rg.255045202
50. Noebauer-Huhmann IM, Glaser C, Dietrich O, Wallner CP, Klinger W, Imhof H, et al. MR imaging of the cervical spine: assessment of image quality with parallel imaging compared to non-accelerated MR measurements. Eur Radiol. (2007) 17:1147–55. doi: 10.1007/s00330-006-0411-2
51. Fruehwald-Pallamar J, Szomolanyi P, Fakhrai N, Lunzer A, Weber M, Thurnher MM, et al. Parallel imaging of the cervical spine at 3T: optimized trade-off between speed and image quality. AJNR Am J Neuroradiol. (2012) 33:1867–74. doi: 10.3174/ajnr.A3101
52. Samson RS, Levy S, Schneider T, Smith AK, Smith SA, Cohen-Adad J, et al. ZOOM or Non-ZOOM? assessing spinal cord diffusion tensor imaging protocols for multi-centre studies. PLoS ONE. (2016) 11:e0155557. doi: 10.1371/journal.pone.0155557
53. Yokohama T, Iwasaki M, Oura D, Furuya S, Okuaki T. The reliability of reduced field-of-view DTI for highly accurate quantitative assessment of cervical spinal cord tracts. Magn Reson Med Sci. (2018) 18:36–43. doi: 10.2463/mrms.mp.2017-0078
54. Mohammadi S, Freund P, Feiweier T, Curt A, Weiskopf N. The impact of post-processing on spinal cord diffusion tensor imaging. Neuroimage. (2013) 70:377–85. doi: 10.1016/j.neuroimage.2012.12.058
55. Vahdat S, Lungu O, Cohen-Adad J, Marchand-Pauvert V, Benali H, Doyon J. Simultaneous brain-cervical cord fMRI reveals intrinsic spinal cord plasticity during motor sequence learning. PLoS Biol. (2015) 13:e1002186. doi: 10.1371/journal.pbio.1002186
56. Andre JB, Bammer R. Advanced diffusion-weighted magnetic resonance imaging techniques of the human spinal cord. Top Magn Reson Imaging. (2010) 21:367–78. doi: 10.1097/RMR.0b013e31823e65a1
57. Romeo F, Hoult DI. Magnet field profiling: analysis and correcting coil design. Magn Reson Med. (1984) 1:44–65. doi: 10.1002/mrm.1910010107
58. Rasoanandrianina H, Grapperon AM, Taso M, Girard OM, Duhamel G, Guye M, et al. Region-specific impairment of the cervical spinal cord (SC) in amyotrophic lateral sclerosis: a preliminary study using SC templates and quantitative MRI (diffusion tensor imaging/inhomogeneous magnetization transfer). NMR Biomed. (2017) 30:e3801. doi: 10.1002/nbm.3801
59. Zeng H, Constable RT. Image distortion correction in EPI: comparison of field mapping with point spread function mapping. Magn Reson Med. (2002) 48:137–46. doi: 10.1002/mrm.10200
60. Samson RS, Ciccarelli O, Kachramanoglou C, Brightman L, Lutti A, Thomas DL, et al. Tissue- and column-specific measurements from multi-parameter mapping of the human cervical spinal cord at 3 T. NMR Biomed. (2013) 26:1823–30. doi: 10.1002/nbm.3022
61. Papinutto N, Bakshi R, Bischof A, Calabresi PA, Caverzasi E, Constable RT, et al. Gradient nonlinearity effects on upper cervical spinal cord area measurement from 3D T1 -weighted brain MRI acquisitions. Magn Reson Med. (2018) 79:1595–601. doi: 10.1002/mrm.26776
62. Bede P, Bokde AL, Byrne SC, Elamin M, Walsh RJ, Hardiman O. Waterskier's Hirayama syndrome. J Neurol. (2011) 258:2078–9. doi: 10.1007/s00415-011-6046-5
63. Belsh JM, Schiffman PL. The Amyotrophic Lateral Sclerosis (ALS) patient perspective on misdiagnosis and its repercussions. J Neurol Sci. (1996) 139:110–6. doi: 10.1016/0022-510X(96)00088-3
64. Traynor BJ, Codd MB, Corr B, Forde C, Frost E, Hardiman O. amyotrophic lateral sclerosis mimic syndromes: a population-based study. Arch Neurol. (2000) 57:109–13. doi: 10.1001/archneur.57.1.109
65. Rocha AJ, Maia Junior AC. Is magnetic resonance imaging a plausible biomarker for upper motor neuron degeneration in amyotrophic lateral sclerosis/primary lateral sclerosis or merely a useful paraclinical tool to exclude mimic syndromes? a critical review of imaging applicability in clinical routine. Arq Neuropsiquiatr. (2012) 70:532–9. doi: 10.1590/S0004-282X2012000700012
66. Lebouteux MV, Franques J, Guillevin R, Delmont E, Lenglet T, Bede P, et al. Revisiting the spectrum of lower motor neuron diseases with snake eyes appearance on magnetic resonance imaging. Eur J Neurol. (2014) 21:1233–41. doi: 10.1111/ene.12465
67. Turner MR, Talbot K. Mimics and chameleons in motor neurone disease. Pract Neurol. (2013) 13:153–64. doi: 10.1136/practneurol-2013-000557
68. Taso M, Girard OM, Duhamel G, Le Troter A, Feiweier T, Guye M, et al. Tract-specific and age-related variations of the spinal cord microstructure: a multi-parametric MRI study using diffusion tensor imaging (DTI) and inhomogeneous magnetization transfer (ihMT). NMR Biomed. (2016) 29:817–32. doi: 10.1002/nbm.3530
69. Cohen-Adad J, El Mendili MM, Morizot-Koutlidis R, Lehericy S, Meininger V, Blancho S, et al. Involvement of spinal sensory pathway in ALS and specificity of cord atrophy to lower motor neuron degeneration. Amyotroph Lateral Scler Frontotemporal Degener. (2013) 14:30–8. doi: 10.3109/17482968.2012.701308
70. Branco LM, De Albuquerque M, De Andrade HM, Bergo FP, Nucci A, Franca MC Jr. Spinal cord atrophy correlates with disease duration and severity in amyotrophic lateral sclerosis. Amyotroph Lateral Scler Frontotemporal Degener. (2014) 15:93–7. doi: 10.3109/21678421.2013.852589
71. El Mendili MM, Chen R, Tiret B, Villard N, Trunet S, Pelegrini-Issac M, et al. Fast and accurate semi-automated segmentation method of spinal cord MR images at 3T applied to the construction of a cervical spinal cord template. PLoS ONE. (2015) 10:e0122224. doi: 10.1371/journal.pone.0122224
72. Paquin ME, El Mendili MM, Gros C, Dupont SM, Cohen-Adad J, Pradat PF. Spinal cord gray matter atrophy in amyotrophic lateral sclerosis. AJNR Am J Neuroradiol. (2018) 39:184–92. doi: 10.3174/ajnr.A5427
73. El Mendili MM, Cohen-Adad J, Pelegrini-Issac M, Rossignol S, Morizot-Koutlidis R, Marchand-Pauvert V, et al. Multi-parametric spinal cord MRI as potential progression marker in amyotrophic lateral sclerosis. PLoS ONE. (2014) 9:e95516. doi: 10.1371/journal.pone.0095516
74. El Mendili MM, Lenglet T, Stojkovic T, Behin A, Guimaraes-Costa R, Salachas F, et al. Cervical spinal cord atrophy profile in adult SMN1-linked SMA. PLoS ONE. (2016) 11:e0152439. doi: 10.1371/journal.pone.0152439
75. Lundell H, Barthelemy D, Skimminge A, Dyrby TB, Biering-Sorensen F, Nielsen JB. Independent spinal cord atrophy measures correlate to motor and sensory deficits in individuals with spinal cord injury. Spinal Cord. (2011) 49:70–5. doi: 10.1038/sc.2010.87
76. Querin G, El Mendili MM, Bede P, Delphine S, Lenglet T, Marchand-Pauvert V, et al. Multimodal spinal cord MRI offers accurate diagnostic classification in ALS. J Neurol Neurosurg Psychiatry. (2018) 89:1220–1221. doi: 10.1136/jnnp-2017-317214
77. De Leener B, Levy S, Dupont SM, Fonov VS, Stikov N, Louis Collins D, et al. SCT: Spinal Cord Toolbox, an open-source software for processing spinal cord MRI data. Neuroimage. (2017) 145:24–43. doi: 10.1016/j.neuroimage.2016.10.009
78. Taso M, Le Troter A, Sdika M, Cohen-Adad J, Arnoux PJ, Guye M, et al. A reliable spatially normalized template of the human spinal cord–Applications to automated white matter/gray matter segmentation and tensor-based morphometry (TBM) mapping of gray matter alterations occurring with age. Neuroimage. (2015) 117:20–8. doi: 10.1016/j.neuroimage.2015.05.034
79. Querin G, El Mendili MM, Lenglet T, Delphine S, Marchand-Pauvert V, Benali H, et al. Spinal cord multi-parametric magnetic resonance imaging for survival prediction in amyotrophic lateral sclerosis. Eur J Neurol. (2017) 24:1040–6. doi: 10.1111/ene.13329
80. Valsasina P, Agosta F, Benedetti B, Caputo D, Perini M, Salvi F, et al. Diffusion anisotropy of the cervical cord is strictly associated with disability in amyotrophic lateral sclerosis. J Neurol Neurosurg Psychiatry. (2007) 78:480–4. doi: 10.1136/jnnp.2006.100032
81. Agosta F, Rocca MA, Valsasina P, Sala S, Caputo D, Perini M, et al. A longitudinal diffusion tensor MRI study of the cervical cord and brain in amyotrophic lateral sclerosis patients. J Neurol Neurosurg Psychiatry. (2009) 80:53–5. doi: 10.1136/jnnp.2008.154252
82. Nair G, Carew JD, Usher S, Lu D, Hu XP, Benatar M. Diffusion tensor imaging reveals regional differences in the cervical spinal cord in amyotrophic lateral sclerosis. Neuroimage. (2010) 53:576–83. doi: 10.1016/j.neuroimage.2010.06.060
83. Carew JD, Nair G, Pineda-Alonso N, Usher S, Hu X, Benatar M. Magnetic resonance spectroscopy of the cervical cord in amyotrophic lateral sclerosis. Amyotroph Lateral Scler. (2011) 12:185–91. doi: 10.3109/17482968.2010.515223
84. Ikeda K, Murata K, Kawase Y, Kawabe K, Kano O, Yoshii Y, et al. Relationship between cervical cord 1H-magnetic resonance spectroscopy and clinoco-electromyographic profile in amyotrophic lateral sclerosis. Muscle Nerve. (2013) 47:61–7. doi: 10.1002/mus.23467
85. Wang Y, Liu L, Ma L, Huang X, Lou X, Wang Y, et al. Preliminary study on cervical spinal cord in patients with amyotrophic lateral sclerosis using MR diffusion tensor imaging. Acad Radiol. (2014) 21:590–6. doi: 10.1016/j.acra.2014.01.014
86. Iglesias C, Sangari S, El Mendili MM, Benali H, Marchand-Pauvert V, Pradat PF. Electrophysiological and spinal imaging evidences for sensory dysfunction in amyotrophic lateral sclerosis. BMJ Open. (2015) 5:e007659. doi: 10.1136/bmjopen-2015-007659
87. de Albuquerque M, Branco LM, Rezende TJ, de Andrade HM, Nucci A, Franca MC Jr. Longitudinal evaluation of cerebral and spinal cord damage in amyotrophic lateral sclerosis. Neuroimage Clin. (2017) 14:269–76. doi: 10.1016/j.nicl.2017.01.024
88. Piaggio N, Pardini M, Roccatagliata L, Scialo C, Cabona C, Bonzano L, et al. Cord cross-sectional area at foramen magnum as a correlate of disability in amyotrophic lateral sclerosis. Eur Radiol Exp. (2018) 2:13. doi: 10.1186/s41747-018-0045-6
89. Grolez G, Kyheng M, Lopes R, Moreau C, Timmerman K, Auger F, et al. MRI of the cervical spinal cord predicts respiratory dysfunction in ALS. Sci Rep. (2018) 8:1828. doi: 10.1038/s41598-018-19938-2
90. Cohen Y, Anaby D, Morozov D. Diffusion MRI of the spinal cord: from structural studies to pathology. NMR Biomed. (2017) 30:e3592. doi: 10.1002/nbm.3592
91. Novikov DS, Fieremans E, Jespersen SN, Kiselev VG. Quantifying brain microstructure with diffusion MRI: theory and parameter estimation. NMR Biomed. 2018:e3998. doi: 10.1002/nbm.3998
92. Tuch DS, Reese TG, Wiegell MR, Makris N, Belliveau JW, Wedeen VJ. High angular resolution diffusion imaging reveals intravoxel white matter fiber heterogeneity. Magn Reson Med. (2002) 48:577–82. doi: 10.1002/mrm.10268
93. Tuch DS, Reese TG, Wiegell MR, Wedeen VJ. Diffusion MRI of complex neural architecture. Neuron. (2003) 40:885–95. doi: 10.1016/S0896-6273(03)00758-X
94. Jensen JH, Helpern JA, Ramani A, Lu H, Kaczynski K. Diffusional kurtosis imaging: the quantification of non-gaussian water diffusion by means of magnetic resonance imaging. Magn Reson Med. (2005) 53:1432–40. doi: 10.1002/mrm.20508
95. Wang Y, Wang Q, Haldar JP, Yeh FC, Xie M, Sun P, et al. Quantification of increased cellularity during inflammatory demyelination. Brain. (2011) 134:3590–601. doi: 10.1093/brain/awr307
96. Cohen-Adad J, Descoteaux M, Rossignol S, Hoge RD, Deriche R, Benali H. Detection of multiple pathways in the spinal cord using q-ball imaging. Neuroimage. (2008) 42:739–49. doi: 10.1016/j.neuroimage.2008.04.243
97. Trojsi F, Caiazzo G, Di Nardo F, Fratello M, Santangelo G, Siciliano M, et al. High angular resolution diffusion imaging abnormalities in the early stages of amyotrophic lateral sclerosis. J Neurol Sci. (2017) 380:215–22. doi: 10.1016/j.jns.2017.07.039
98. Zhang H, Schneider T, Wheeler-Kingshott CA, Alexander DC. NODDI: practical in vivo neurite orientation dispersion and density imaging of the human brain. Neuroimage. (2012) 61:1000–16. doi: 10.1016/j.neuroimage.2012.03.072
99. Barritt AW, Gabel MC, Cercignani M, Leigh PN. Emerging magnetic resonance imaging techniques and analysis methods in amyotrophic lateral sclerosis. Front Neurol. (2018) 9:1065. doi: 10.3389/fneur.2018.01065
100. Broad RJ, Gabel MC, Dowell NG, Schwartzman DJ, Seth AK, Zhang H, et al. Neurite orientation and dispersion density imaging (NODDI) detects cortical and corticospinal tract degeneration in ALS. J Neurol Neurosurg Psychiatry. (2018) doi: 10.1136/jnnp-2018-318830. [Epub ahead of print].
101. Wen J, Zhang H, Alexander DC, Durrleman S, Routier A, Rinaldi D, et al. Neurite density is reduced in the presymptomatic phase of C9orf72 disease. J Neurol Neurosurg Psychiatry. (2018). doi: 10.1136/jnnp-2018-318994. [Epub ahead of print].
102. By S, Xu J, Box BA, Bagnato FR, Smith SA. Application and evaluation of NODDI in the cervical spinal cord of multiple sclerosis patients. Neuroimage Clin. (2017) 15:333–42. doi: 10.1016/j.nicl.2017.05.010
103. Budde MD, Xie M, Cross AH, Song SK. Axial diffusivity is the primary correlate of axonal injury in the experimental autoimmune encephalomyelitis spinal cord: a quantitative pixelwise analysis. J Neurosci. (2009) 29:2805–13. doi: 10.1523/JNEUROSCI.4605-08.2009
104. Sun SW, Liang HF, Trinkaus K, Cross AH, Armstrong RC, Song SK. Noninvasive detection of cuprizone induced axonal damage and demyelination in the mouse corpus callosum. Magn Reson Med. (2006) 55:302–8. doi: 10.1002/mrm.20774
105. Song SK, Sun SW, Ramsbottom MJ, Chang C, Russell J, Cross AH. Dysmyelination revealed through MRI as increased radial (but unchanged axial) diffusion of water. Neuroimage. (2002) 17:1429–36. doi: 10.1006/nimg.2002.1267
106. Song SK, Yoshino J, Le TQ, Lin SJ, Sun SW, Cross AH, et al. Demyelination increases radial diffusivity in corpus callosum of mouse brain. Neuroimage. (2005) 26:132–40. doi: 10.1016/j.neuroimage.2005.01.028
107. Bede P, Elamin M, Byrne S, McLaughlin RL, Kenna K, Vajda A, et al. Patterns of cerebral and cerebellar white matter degeneration in ALS. J Neurol Neurosurg Psychiatry. (2015) 86:468–70. doi: 10.1136/jnnp-2014-308172
108. Sled JG. Modelling and interpretation of magnetization transfer imaging in the brain. Neuroimage. (2018) 182:128–35. doi: 10.1016/j.neuroimage.2017.11.065
109. Schmierer K, Scaravilli F, Altmann DR, Barker GJ, Miller DH. Magnetization transfer ratio and myelin in postmortem multiple sclerosis brain. Ann Neurol. (2004) 56:407–15. doi: 10.1002/ana.20202
110. Schmierer K, Tozer DJ, Scaravilli F, Altmann DR, Barker GJ, Tofts PS, et al. Quantitative magnetization transfer imaging in postmortem multiple sclerosis brain. J Magn Reson Imaging. (2007) 26:41–51. doi: 10.1002/jmri.20984
111. Mottershead JP, Schmierer K, Clemence M, Thornton JS, Scaravilli F, Barker GJ, et al. High field MRI correlates of myelin content and axonal density in multiple sclerosis–a post-mortem study of the spinal cord. J Neurol. (2003) 250:1293–301. doi: 10.1007/s00415-003-0192-3
112. Borsodi F, Culea V, Langkammer C, Khalil M, Pirpamer L, Quasthoff S, et al. Multimodal assessment of white matter tracts in amyotrophic lateral sclerosis. PLoS ONE. (2017) 12:e0178371. doi: 10.1371/journal.pone.0178371
113. Carrara G, Carapelli C, Venturi F, Ferraris MM, Lequio L, Chio A, et al. A distinct MR imaging phenotype in amyotrophic lateral sclerosis: correlation between T1 magnetization transfer contrast hyperintensity along the corticospinal tract and diffusion tensor imaging analysis. AJNR Am J Neuroradiol. (2012) 33:733–9. doi: 10.3174/ajnr.A2855
114. Tanabe JL, Vermathen M, Miller R, Gelinas D, Weiner MW, Rooney WD. Reduced MTR in the corticospinal tract and normal T2 in amyotrophic lateral sclerosis. Magn Reson Imaging. (1998) 16:1163–9. doi: 10.1016/S0730-725X(98)00129-5
115. Kato Y, Matsumura K, Kinosada Y, Narita Y, Kuzuhara S, Nakagawa T. Detection of pyramidal tract lesions in amyotrophic lateral sclerosis with magnetization-transfer measurements. AJNR Am J Neuroradiol. (1997) 18:1541–7.
116. Girard OM, Callot V, Prevost VH, Robert B, Taso M, Ribeiro G, et al. Magnetization transfer from inhomogeneously broadened lines (ihMT): improved imaging strategy for spinal cord applications. Magn Reson Med. (2017) 77:581–91. doi: 10.1002/mrm.26134
117. Varma G, Girard OM, Prevost VH, Grant AK, Duhamel G, Alsop DC. Interpretation of magnetization transfer from inhomogeneously broadened lines (ihMT) in tissues as a dipolar order effect within motion restricted molecules. J Magn Reson. (2015) 260:67–76. doi: 10.1016/j.jmr.2015.08.024
118. Van Obberghen E, McHinda S, le Troter A, Prevost VH, Viout P, Guye M, et al. Evaluation of the sensitivity of Inhomogeneous Magnetization Transfer (ihMT) MRI for multiple sclerosis. AJNR Am J Neuroradiol. (2018) 39:634–41. doi: 10.3174/ajnr.A5563
119. Pioro EP, Antel JP, Cashman NR, Arnold DL. Detection of cortical neuron loss in motor neuron disease by proton magnetic resonance spectroscopic imaging in vivo. Neurology. (1994) 44:1933–8. doi: 10.1212/WNL.44.10.1933
120. Kalra S, Cashman NR, Genge A, Arnold DL. Recovery of N-acetylaspartate in corticomotor neurons of patients with ALS after riluzole therapy. Neuroreport. (1998) 9:1757–61. doi: 10.1097/00001756-199806010-00016
121. Kalra S, Tai P, Genge A, Arnold DL. Rapid improvement in cortical neuronal integrity in amyotrophic lateral sclerosis detected by proton magnetic resonance spectroscopic imaging. J Neurol. (2006) 253:1060–3. doi: 10.1007/s00415-006-0162-7
122. Pioro EP, Majors AW, Mitsumoto H, Nelson DR, Ng TC. 1H-MRS evidence of neurodegeneration and excess glutamate + glutamine in ALS medulla. Neurology. (1999) 53:71–9. doi: 10.1212/WNL.53.1.71
123. Rule RR, Suhy J, Schuff N, Gelinas DF, Miller RG, Weiner MW. Reduced NAA in motor and non-motor brain regions in amyotrophic lateral sclerosis: a cross-sectional and longitudinal study. Amyotroph Lateral Scler Other Motor Neuron Disord. (2004) 5:141–9. doi: 10.1080/14660820410017109
124. Verma G, Woo JH, Chawla S, Wang S, Sheriff S, Elman LB, et al. Whole-brain analysis of amyotrophic lateral sclerosis by using echo-planar spectroscopic imaging. Radiology. (2013) 267:851–7. doi: 10.1148/radiol.13121148
125. Hock A, Henning A, Boesiger P, Kollias SS. (1)H-MR spectroscopy in the human spinal cord. AJNR Am J Neuroradiol. (2013) 34:1682–9. doi: 10.3174/ajnr.A3342
126. Marliani AF, Clementi V, Albini Riccioli L, Agati R, Carpenzano M, Salvi F, et al. Quantitative cervical spinal cord 3T proton MR spectroscopy in multiple sclerosis. AJNR Am J Neuroradiol. (2010) 31:180–4. doi: 10.3174/ajnr.A1738
127. Wyss PO, Hock A, Kollias S. The application of human spinal cord magnetic resonance spectroscopy to clinical studies: a review. Semin Ultrasound CT MR. (2017) 38:153–62. doi: 10.1053/j.sult.2016.07.005
128. Proudfoot M, Bede P, Turner MR. Imaging cerebral activity in amyotrophic lateral sclerosis. Front Neurol. (2018) 9:1148. doi: 10.3389/fneur.2018.01148
129. Abrahams S, Goldstein LH, Simmons A, Brammer M, Williams SCR, Giampietro V, et al. Word retrieval in amyotrophic lateral sclerosis: a functional magnetic resonance imaging study. Brain. (2004) 127:1507–17. doi: 10.1093/brain/awh170
130. Agosta F, Valsasina P, Absinta M, Riva N, Sala S, Prelle A, et al. Sensorimotor functional connectivity changes in amyotrophic lateral sclerosis. Cereb Cortex. (2011) 21:2291–8. doi: 10.1093/cercor/bhr002
131. Kollewe K, Munte TF, Samii A, Dengler R, Petri S, Mohammadi B. Patterns of cortical activity differ in ALS patients with limb and/or bulbar involvement depending on motor tasks. J Neurol. (2011) 258:804–10. doi: 10.1007/s00415-010-5842-7
132. Kong Y, Eippert F, Beckmann CF, Andersson J, Finsterbusch J, Buchel C, et al. Intrinsically organized resting state networks in the human spinal cord. Proc Natl Acad Sci USA. (2014) 111:18067–72. doi: 10.1073/pnas.1414293111
133. Powers JM, Ioachim G, Stroman PW. Ten key insights into the use of spinal cord fMRI. Brain Sci. (2018) 8:E173. doi: 10.3390/brainsci8090173
134. Gil J, Funalot B, Verschueren A, Danel-Brunaud V, Camu W, Vandenberghe N, et al. Causes of death amongst French patients with amyotrophic lateral sclerosis: a prospective study. Eur J Neurol. (2008) 15:1245–51. doi: 10.1111/j.1468-1331.2008.02307.x
135. El Mendili MM. Analysis of the Structural Integrity of the Spinal Cord in Motor Neuron Diseases Using a Multi-Parametric MRI Approach. Doctoral dissertation, Université Pierre et Marie Curie-Paris VI (2016).
Keywords: ALS (Amyotrophic lateral sclerosis), MRI—magnetic resonance imaging, MND, spinal cord, neuroimaging
Citation: El Mendili MM, Querin G, Bede P and Pradat P-F (2019) Spinal Cord Imaging in Amyotrophic Lateral Sclerosis: Historical Concepts—Novel Techniques. Front. Neurol. 10:350. doi: 10.3389/fneur.2019.00350
Received: 30 December 2018; Accepted: 21 March 2019;
Published: 12 April 2019.
Edited by:
Justin John Yerbury, University of Wollongong, AustraliaReviewed by:
Virginie Callot, Centre National de la Recherche Scientifique (CNRS), FranceCopyright © 2019 El Mendili, Querin, Bede and Pradat. This is an open-access article distributed under the terms of the Creative Commons Attribution License (CC BY). The use, distribution or reproduction in other forums is permitted, provided the original author(s) and the copyright owner(s) are credited and that the original publication in this journal is cited, in accordance with accepted academic practice. No use, distribution or reproduction is permitted which does not comply with these terms.
*Correspondence: Pierre-François Pradat, cGllcnJlLWZyYW5jb2lzLnByYWRhdEBwc2wuYXBocC5mcg==
†These authors have contributed equally as co-senior authors
Disclaimer: All claims expressed in this article are solely those of the authors and do not necessarily represent those of their affiliated organizations, or those of the publisher, the editors and the reviewers. Any product that may be evaluated in this article or claim that may be made by its manufacturer is not guaranteed or endorsed by the publisher.
Research integrity at Frontiers
Learn more about the work of our research integrity team to safeguard the quality of each article we publish.