Corrigendum: Neuroinflammation and Cytokines in Myalgic Encephalomyelitis/Chronic Fatigue Syndrome (ME/CFS): A Critical Review of Research Methods
- Division of Neurotherapeutics, Massachusetts General Hospital, Harvard Medical School, Boston, MA, United States
Myalgic encephalomyelitis/chronic fatigue syndrome (ME/CFS) is the label given to a syndrome that can include long-term flu-like symptoms, profound fatigue, trouble concentrating, and autonomic problems, all of which worsen after exertion. It is unclear how many individuals with this diagnosis are suffering from the same condition or have the same underlying pathophysiology, and the discovery of biomarkers would be clarifying. The name “myalgic encephalomyelitis” essentially means “muscle pain related to central nervous system inflammation” and many efforts to find diagnostic biomarkers have focused on one or more aspects of neuroinflammation, from periphery to brain. As the field uncovers the relationship between the symptoms of this condition and neuroinflammation, attention must be paid to the biological mechanisms of neuroinflammation and issues with its potential measurement. The current review focuses on three methods used to study putative neuroinflammation in ME/CFS: (1) positron emission tomography (PET) neuroimaging using translocator protein (TSPO) binding radioligand (2) magnetic resonance spectroscopy (MRS) neuroimaging and (3) assays of cytokines circulating in blood and cerebrospinal fluid. PET scanning using TSPO-binding radioligand is a promising option for studies of neuroinflammation. However, methodological difficulties that exist both in this particular technique and across the ME/CFS neuroimaging literature must be addressed for any results to be interpretable. We argue that the vast majority of ME/CFS neuroimaging has failed to use optimal techniques for studying brainstem, despite its probable centrality to any neuroinflammatory causes or autonomic effects. MRS is discussed as a less informative but more widely available, less invasive, and less expensive option for imaging neuroinflammation, and existing studies using MRS neuroimaging are reviewed. Studies seeking to find a peripheral circulating cytokine “profile” for ME/CFS are reviewed, with attention paid to the biological and methodological reasons for lack of replication among these studies. We argue that both the biological mechanisms of cytokines and the innumerable sources of potential variance in their measurement make it unlikely that a consistent and replicable diagnostic cytokine profile will ever be discovered.
Introduction
Chronic fatigue syndrome (CFS) is an often-debilitating illness that can feel like an ongoing flu that lasts for years. Symptoms include reduced energy production, body aches, non-refreshing sleep, and difficulty recovering from both physical and mental exertion. Among many patients and some scientists, the preferred name for chronic fatigue syndrome is myalgic encephalomyelitis (ME), leading this condition to frequently be referred to as ME/CFS (among some scientists, the preferred name is “systemic exercise intolerance syndrome” [SEID; (1)] but use of this term remains rare). While it is more commonly used in Europe, the term “myalgic encephalomyelitis” is almost unheard of in the United States outside of experts and advocates, and “chronic fatigue syndrome” is generally used instead. The current review is largely centered on some of the research methods necessary for justifying the term “myalgic encephalomyelitis,” which essentially means “muscle pain (myalgia) related to central nervous system inflammation (encephalomyelitis).”
For this condition to warrant the name ME, “encephalomyelitis” should be a consistent finding reported by multiple groups using multiple methods. To move past a defensive posture of “this is a real condition with biological differences from healthy controls” toward diagnostic biomarkers and effective treatment options, the field's neuroimmunology research must be able to answer:
• How would a measured component of neuroinflammation lead to symptoms?
• How do we accurately measure that component of neuroinflammation?
• What can and cannot be concluded from the chosen method?
In this review, we focus on three specific methods that have been used to study the neuroimmunology of ME/CFS:
• positron emission tomography (PET) using translocator protein (TSPO) binding radioligand,
• magnetic resonance spectroscopy (MRS), and
• assays measuring cytokines in blood and cerebrospinal fluid
We offer a particular focus on what can and cannot be concluded by studies using these methods.
We review the above three methods because:
1) we believe that PET scanning using TSPO-binding radioligand is the best-available and most direct option for studies of neuroinflammation but that methods must be optimized,
2) MRS is much more widely available than PET with TSPO-binding radioligand and has good potential for a less expensive and invasive option for indirectly imaging neuroinflammation, and
3) studies commonly seek to find a distinct peripheral circulating cytokine “profile” in ME/CFS, and we offer critiques of current approaches.
“Encephalomyelitis”
There have been scores of historical outbreaks of viral-like illnesses that lead to profound and lasting fatigue, perhaps most famously in Los Angeles (1934), Iceland (1948), London (1955), and Nevada (1984) (2–5). In 1955, an Icelandic doctor suggested the name “benign myalgic encephalomyelitis” after noting some similarities in cerebrospinal fluid abnormalities between patients from the London Royal Free Hospital outbreak and other putatively similar outbreaks, including a 1948 outbreak in Akureyri, Iceland (Sigurdsson May 26, 1956, in The Lancet). A lack of consistent methods and cerebrospinal fluid sample sizes precluded strong conclusions about similarities, or lack thereof, across the outbreaks. Sigurdsson (2) described “symptoms and signs of damage to the brain and spinal cord, in a greater or lesser degree” and “protracted muscle pain with paresis and cramp” in explaining his choice of the term “benign myalgic encephalomyelitis.” The term “benign” was included not because the symptoms were mild, but rather for discriminant validity because this “new clinical entity” was believed by Sigurdsson to have a “relatively benign outcome” (including lack of fatalities), relative to possibly similar conditions such as poliomyelitis. Another seemingly similar outbreak occurred in 1984–5 in Incline Village, Nevada. If there existed any connection to previous outbreaks that connection was not made, and a new term, “chronic fatigue syndrome,” was coined. This has contributed to confusion over whether “chronic fatigue syndrome” and “myalgic encephalomyelitis” are the same entity. The causes of and connections among outbreaks remain incompletely understood.
Despite the issues with name and diagnosis, there may be a core/root condition “ME/CFS” that involves inflammation of the central nervous system. Many studies, including those reviewed below, have reported results consistent with a neuroinflammatory process [e.g., (6–9)]. However, despite some cases of direct evidence and a fair amount of indirect evidence from case-control studies, consistent and well-replicated direct evidence for nervous system inflammation is still somewhat limited, relative to what one would expect for a condition named after a mechanistic trait.
Inflammation Neurocircuitry
Many patients with ME/CFS report having experienced a viral or bacterial infection directly prior to the onset of their illness [e.g., (10–14)]. This has led researchers to investigate the hypothesis that resulting inflammation may be a mechanism by which this syndrome occurs [e.g., (9); (6)]. Given the putative centrality of neuroinflammation in ME/CFS, dysregulation in peripheral immune system to nervous system inflammation pathways should be a target for hypotheses and research [e.g., (15)].
When an inflammatory response occurs in the periphery, the brain is alerted to the presence of inflammation-associated molecules such as proinflammatory cytokines circulating in blood. While new potential neuroimmune pathways are still being discovered [e.g., (16)], we know of three ways in which this alert can occur. Immune proteins such as cytokines will:
1) be actively transported across the blood-brain barrier (BBB),
2) passively diffuse through the BBB via circumventricular organs if present in high enough concentrations, or
3) be detected by chemoreceptors in the afferent (sensory) vagus nerve, which synapses in the nucleus of the solitary tract (NTS) of dorsal brainstem (17–21).
The process of afferent neuroimmune signaling triggers the sickness response (sometimes called sickness behaviors), a general innate immune system reaction [e.g., (22)] that includes many symptoms that overlap with ME/CFS symptoms [e.g., (15)].
Cytokine signaling from the peripheral side of the BBB triggers a “mirror response” of glial activation and cytokine release on the brain side of the BBB (18). Glia are a class of cells that function at the intersection of the nervous and immune systems; the primary glia of the central nervous systems are microglia, tissue-resident macrophages that are capable of detecting danger-associated molecules such as alarmins and mitochondrial DNA, or immune signaling molecules such as chemokines and proinflammatory cytokines (23). When this detection occurs, microglia and other glial cell types enter a functional and morphological state of activation, and in turn produce their own chemokines and proinflammatory cytokines that can cause the activation and proliferation of nearby glia. Importantly, a relatively large brain-side “mirror response” of glial activation and cytokine release can be triggered by a small quantity of proinflammatory cytokine, if that small quantity of cytokine has been detected by the chemoreceptors of the afferent vagus nerve. Mirror responses may follow specific neural circuits (discussed below), as glia are most dense along white matter tracts (24, 25). This explains why, from the above-described three mechanisms of cytokine-to-brain communication, neuroimmune signaling continues along specific brain pathways. Basic neuroimmunology research has begun to elucidate these pathways, which should be the focus of ME/CFS neuroimaging studies. Kraynak et al. (19) conducted a useful meta-analysis of this basic neuroimmunology research. They synthesized results from studies that performed neuroimaging during peripheral immune activation by either an immune stimulating antigen (e.g., lipopolysaccharide [LPS]) or proinflammatory cytokines (e.g., interferon alpha [IFN-α]). Such challenges consistently activated known intrinsic brain networks and specific structures. Consistent activation occurred in basal ganglia (bilateral striatum), limbic structures (right amygdala, bilateral hippocampus, and hypothalamus), brainstem/pons, and neocortex (right anterior insular cortex, right temporal and left parahippocampal gyri, subgenual and dorsal anterior cingulate cortex [sgACC and dACC], and dorsomedial and ventromedial prefrontal cortex [dmPFC and vmPFC]). The meta-analysis also investigated functional connectivity patterns among the above structures, finding especially strong connectivity between brainstem and right anterior insula, anterior insula, and amygdala/parahippocampal gyrus, and between brainstem and sgACC/vmPFC.
Though not as robust, right temporal and left parahippocampal gyri also showed significant functional connectivity with the above structures. Therefore, these could be considered a priori functional circuits of interest in studies of putative neuroinflammatory conditions such as ME/CFS. The dACC (which would be considered anterior midcingulate cortex [aMCC] by some anatomists) did not show functional connectivity with the above circuits but was consistently activated and therefore could also be considered an a priori region of interest in neuroinflammation studies. Given the role of dACC in attention and cognitive control, we suggest that its function in ME/CFS could be considered particularly important for “brain fog” symptoms. Furthermore, Kraynak et al. (19) reported that the thalamus was also consistently detected across multiple study designs, but not in a way that demonstrated functional connectivity. However, we consider thalamus an important region of interest in ME/CFS given its detection by Nakatomi et al. (8) and given the role of thalamus in sensory filtering, a likely mechanism for the common symptom of sensory sensitivity (discussed further in section MRS studies in ME/CFS).
In brainstem/pons, the meta-analysis did find functional connectivity but failed to find consistent activation across studies in the area of nucleus of the solitary tract (NTS) and area postrema. This might be considered unexpected because these neighboring structures are central to two of the three cytokine-to-brain pathways described in section Inflammation neurocircuitry: the NTS is where vagus nerve enters the brainstem, and area postrema is a key circumventricular organ. We suspect that the area of NTS and area postrema was not consistently activated in all studies of this meta-analysis because most neuroimaging studies do not use brainstem-specific spatial registration techniques (discussed in more detail below in section Brainstem-specific analyses and techniques). We therefore strongly recommend that neuroimaging studies of ME/CFS consider this area (at the dorsal surface of brainstem just inferior to pons) as an a priori region of interest. In addition to its role in afferent cytokine-to-brain signaling, this area of brainstem may hold particular importance for ME/CFS symptoms. In the afferent direction, area postrema is dense with mast cells (26), which is perhaps important for some ME/CFS patients, given comorbidity between ME/CFS and mast cell activation disorder. In the efferent direction, this area includes the dorsal motor nucleus of the vagus nerve (DMV), which is potentially important given its role in autonomic functions [e.g., (27)] that are dysfunctional in ME/CFS, such as appropriate heart rate adjustments to postural changes and exertion. Furthermore, an efferent signal from DMV should trigger an anti-inflammatory reflex, which serves to limit the inflammatory response (28). Functional analysis of this area critically relies upon brainstem-specific techniques (see Figure 1) in order for signal to be detected (27, 29).
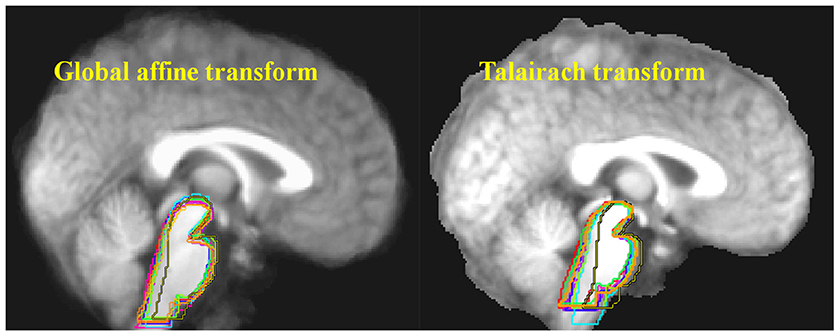
Figure 1. Ten structural MRI scans were aligned using two different standard neocortex-based spatial registration techniques. The brainstem of each individual brain was then traced to demonstrate how poorly they are aligned by these methods. In functional neuroimaging, detection of activation in a given brain structure is completely dependent upon the alignment of that structure across all subjects. No signal will be detected if the region of interest is not aligned. Reprinted from Napadow et al. (29) with permission from Elsevier.
Because neuroinflammation can affect normal function and structure, even methods that do not directly measure neuroinflammation (e.g., fMRI and structural MR) can be clarifying if their focus is on neuroinflammation-relevant brain circuits and structures. However, there are neuroimaging techniques that can more directly measure neuroinflammation, such as PET and MRS. The current gold standard for in vivo imaging of neuroinflammation is PET scanning using a translocator protein-binding radioligand.
Measuring Microglial Activation: PET and The Translocator Protein
Positron emission tomography (PET) is a neuroimaging method that involves the injection of a radioactive tracer (radiotracer). The radiotracer is biologically relevant in some manner; for example it may mimic endogenous glucose or an endogenous neurotransmitter, or it may bind to a receptor or other molecule of interest. Radiotracers typically use a small amount of rapidly-decaying radiation, and as its radiation decays its location within the body or brain is calculated by the PET scanner. This allows neuroscientists to determine where the biological process of interest is occurring. Several radiotracers have been developed to detect and localize microglial activation by binding to the translocator protein [usually referred to as TSPO but also sometimes referred to as TP-18; reviewed in (30–32)].
First known as the peripheral benzodiazepine receptor (PBR), what is now called the 18kD translocator protein (TSPO), is part of a larger protein complex known as mitochondrial permeability transition pore (MPTP). TSPO is expressed by non-neuronal cells of the central nervous system, and is mostly localized to the outer mitochondrial membrane. TSPO is of interest in the functional imaging of neuroinflammation because it is produced when microglia become activated, and microglial activation is a key component of classically-defined neuroinflammation. Importantly for its use as a proxy for neuroimmune functional state, TSPO is not highly expressed by microglia at a constitutive level but is upregulated upon microglial activation.
Some researchers argue that microglial activation is not a perfect synonym for neuroinflammation and that classically-defined inflammation is when circulating immune cells penetrate into tissue [e.g., (21)]. However, microglial activation would be a predictable correlate to classically-defined neuroinflammation, which would be defined as the infiltration into brain parenchyma of peripheral immune cells such as T cells, dendritic cells, and peripheral mast cells (18). Microglial activation is central to the increased permeability of the BBB that is necessary for this process, and therefore the binding of radiotracer to TSPO is an expected state during classically-defined neuroinflammation, and an absence of such binding would be fairly good evidence for a lack of classically-defined neuroinflammation. Other expected changes during classically-defined neuroinflammation would include activation of other resident immunocompetent cells in addition to microglia (such as astrocytes), disruption of BBB, penetration of peripheral immune cells to the brain side of the BBB, and additional possible pathological consequences such as cell loss, iron accumulation, and edema. Each of these expected changes can be measured with neuroimaging [for review of methods see (33)] and such studies would provide concurrent validity for TSPO-binding radioligand studies. Here we describe one PET TSPO study of healthy individuals, and one of individuals with ME/CFS.
PET Scanning Using TSPO-Binding Radioligand in Healthy Humans
Sandiego et al. (34) used PET scanning with TSPO-binding radioligand to understand the effects of a peripheral immune challenge on the brains of healthy humans. They used lipopolysaccharide (LPS, sometimes called endotoxin), a molecule found in the outer membrane of gram-negative bacteria, which triggers an immune response via TLR4 signaling. LPS is a commonly-used experimental immune challenge, but the effect of peripheral LPS injection on immune response in the brain had previously only been studied in animal models.
Using a within-subjects design, Sandiego et al. (34) reported significantly increased PBR28 signal in many brain structures following LPS-injection, including bilateral caudate nucleus and putamen of the basal ganglia, large areas of the neocortex, amygdala, hippocampus, and thalamus. They also found a significant increase in several peripherally-circulating cytokines; however, these circulating cytokine levels correlated with neither PBR28 signal nor subjective sickness symptoms such as fatigue. This is an important concept found repeatedly in the neuroinflammation literature and discussed further below: circulating cytokine levels are often a poor measure for subjective symptoms and often do not reflect what is happening on the brain side of the BBB. For example, in a study of ME/CFS, Nakatomi et al. (8) reported a lack of correlation between circulating cytokine levels and TSPO-binding radioligand signal in ME/CFS patients' brains, along with a lack of correlation between circulating cytokine levels and their subjective symptoms.
PET Scanning Using TSPO-Binding Radioligand in ME/CFS
Nakatomi et al. (8) conducted the first case-control study using PET to measure TSPO expression in the central nervous system of ME/CFS patients vs. healthy controls. They found significantly increased PET signal, especially in a region between mid-pons and thalamus, in patients vs. controls. Based on how the “mirror response” of peripheral-to-central nervous system immune signaling works, this is the general pattern one would expect based on a paper from our group, which hypothesizes that some cases of ME/CFS could be explained by exaggerated afferent neuroimmune signaling entering the central nervous system at the nucleus of the solitary tract (NTS) in dorsal brainstem (15). Nakatomi et al. (8) remains an important, groundbreaking study that should be replicated with complementary methods. Here, we describe several specific ways to complement and improve upon future studies using the same general method of PET scanning using TSPO-binding radioligand.
Methods to Address Potential Confounds in PET Studies Using TSPO-Binding Radioligand
There are several potential ways to interpret differences in TSPO-binding radioligand signal in patients vs. controls. Isolating and addressing potential confounding variables will make interpretation easier but also adds difficulty and considerable cost to a study. Type 1 or type 2 errors in studies of TSPO-binding PET radioligand uptake in brain could potentially be explained by the following methodological confounds:
• Standard neuroimaging techniques were not designed for brainstem study
• The first-generation radioligand PK11195 has high non-specific binding and low signal-to-background ratio
• PET signal calculated with an anatomical reference brain region relies on equal radioligand uptake in that region across cases and controls
• Radioligand access to brain is modified by general metabolism, which can differ across cases and controls
• Activated peripheral immune cells bind radioligand and can differ in quantity across cases and controls
• A single nucleotide polymorphism (SNP) in the TSPO gene causes differential radioligand binding
• Use of healthy controls harms discriminant validity
Here, we will address each of these issues and describe solutions.
Brainstem-Specific Analyses and Techniques
Standard neuroimaging techniques were not designed for brainstem study
One can almost consider the structural and functional neuroimaging analysis of brainstem to be a separate technique from the analysis of neocortex because brainstem analysis has its own issues that must be resolved for the data to be interpretable [e.g., (27, 29, 35)]. The vast majority of neuroimaging studies do not use brainstem-appropriate techniques. Two prominent issues are (1) the need for independent spatial registration of brainstem, and (2) the unique susceptibility of brainstem to physiologically-based movement artifact.
Standard MRI and fMRI analysis software platforms use the neocortex for spatial registration. In neuroimaging, spatial registration is the process of lining up all participant brains so their anatomy overlaps, allowing structural differences or functional activations to be meaningfully compared. Nakatomi et al. (8) used Statistical Parametric Mapping 5 software (SPM5; Wellcome Department of Cognitive Neurology), which is a well-validated and widely-accepted technique in neuroimaging. However, like most standard techniques, the brainstem is not the focus of standard SPM5 spatial registration. Instead, the neocortex of each individual brain in a study is lined up with the neocortex of a canonical brain (see Figure 1). This is because the vast majority of functional neuroimaging studies examine the types of “higher” cognitive and emotional processes that are associated with the neocortex, as opposed to studying the types of “lower” processes that are associated with the brainstem (e.g., autonomic, arousal, pain, neuroimmune communication). Given the anatomical reality that the brainstem comprises many densely-packed but functionally-heterogeneous nuclei, any small errors in spatial registration caused by failure to use brainstem-specific registration are highly likely to lead to decreased sensitivity in signal and type 2 errors (29). It is likely a testament to the strength of the PET signal in Nakatomi et al. (8) that their results remained statistically significant despite the fact that brainstem-specific analysis techniques were not used, however it is also likely that the lack of dorsal signal is explained by this confound.
Furthermore, the brainstem is especially prone to physiologically-driven movement artifact given that it pulses with every heartbeat [e.g., (36, 37)]. This is especially important for fMRI studies as opposed to PET, but this artifact is rarely considered in studies using either method. This can be corrected by recording physiological measures during acquisition to use as a movement artifact regressor during functional analysis. It is important to note that failure to control for systematic differences in movement between patients and controls has caused significant confusion in some clinical neuroimaging fields [e.g., (38)].
A large majority of neuroimaging studies in ME/CFS have not used brainstem-specific spatial co-registration, normalization, or physiologically-derived movement artifact regression techniques. In a disorder defined by symptoms related to fatigue, autonomic nervous system problems [e.g., (39–46)], and putative neuroimmune signaling [e.g., (6, 9, 15)], brainstem is an obvious region of interest. Standard analysis techniques would surely fail to coregister the very small nuclei that may be related to key ME/CFS symptoms (e.g., nucleus of the solitary tract, area postrema, dorsal motor nucleus of the vagus nerve, periaqueductal gray, reticularis gigantocellularis, and others). It is therefore quite likely that functional brainstem abnormalities in this condition, if any, have been missed by those studies that reported the results of standard techniques. It is noteworthy that several studies that did deliberately focus on brainstem have found abnormalities. For example, Costa et al. (47) reported brainstem hypoperfusion in ME/CFS patients vs. depressive and healthy controls. Barnden et al. (48) reported differential regression values of seated pulse pressure (systolic–diastolic) against brainstem total gray matter volume (measured by voxel-based morphometry and centered on tegmental area) in ME/CFS patients vs. healthy controls. Similarly, Barnden et al. (39) reported an abnormal association between indicators of autonomic function volumetric measures in the area of the vasomotor center in the brainstem's medulla oblongata, which (along with glossopharangeal nerve) is innervated by the neuroimmune and autonomic parasympathetic vagus nerve. Barnden et al. (49) reported abnormal T1-weighted spin echo MRI signal in brainstem of Fukuda criteria ME/CFS patients.
By using brainstem-specific spatial registration in addition to standard neocortex spatial registration, neuroimaging studies of ME/CFS are much more likely to detect any functional and structural abnormalities that may be driving autonomic and neuroimmune-related symptoms. We believe it likely that failure to use brainstem-specific techniques has resulted in type 2 errors in the ME/CFS neuroimaging field, in PET studies as well as other modalities like MRI and fMRI.
PBR28 or Other Second-Generation Radioligands Instead of PK11195
The first-generation radioligand PK11195 has high non-specific binding and low signal-to-background ratio
Nakatomi et al. (8) used the first-generation TSPO-binding radioligand, [11C]-(R)-PK11195 (referred to hereafter as PK11195). The development of PK11195 in the 1980s led to advances in the understanding of brain diseases with an inflammatory component such as multiple sclerosis, Rasmussen's encephalitis, Huntington and Alzheimer's diseases, and others (31). However, PK11195 has fairly low brain penetrance and also high non-specific binding in that it binds to other types of immune cells and proteins, including those in the general blood circulation [e.g., (50)]. If there are systematic differences in BBB permeability or in the quality and quantity of PK11195-binding antigens between cases and controls, this can lead to type 1 or type 2 error. Since PK11195's development, a newer, second-generation family of TSPO-binding radioligands has been created (33). Second-generation TSPO-binding radioligands, including PBR28, FEPPA, and DPA-714 [reviewed in (51)], feature a much higher signal-to-background ratio than PK11195.
Arterial Line (A-Line) Sampling During PET Neuroimaging Allows Data Interpretation
PET signal calculated with an anatomical reference region relies on equal radioligand uptake in that region across cases and controls
Nakatomi et al. (8) used a cerebellar reference region to calculate non-displaceable binding potential: in order to compare patients to controls, each individual study participant had the amount of PET signal in brain regions of interest compared to the amount in the cerebellum. In other words, each person's cerebellum was used as their own “baseline” comparator to decide if other regions were showing evidence of radioligand uptake and therefore microglial activation. This is a standard and widely-accepted technique for PET study analyses, however it is not a quantitative analysis technique: the “signal” reported in such studies is a relative signal and not a quantitative one. This may be particularly important for studies of a poorly-understood condition like ME/CFS because we cannot be certain that the cerebella of patients are not affected by their condition. For example, cerebellar folia (gyri) contain several large blood vessels which could contain different amounts of TSPO-expressing circulating immune cells in patients vs. controls. Furthermore, a recent report found increased HHV-6 infection of cerebellum Purkinje cells in mood disorders vs. controls (52); such an infection would be likely to increase TSPO expression and render invalid the cerebellum as a “baseline” reference region. The gold standard for quantitative data would be arterial line (A-line) sampling for kinetic modeling of TSPO, which counters other potential confounds as well. Throughout the scan, blood samples are extracted from the radial artery at regular timepoints. Sample analysis allows determination of the exact quantity of free radioligand available to enter the brain, which is used to interpret brain signal.
Radioligand access to brain is modified by general metabolism, which can differ across cases and controls
One common theory of ME/CFS is that it is, at root, a disorder of mitochondrial dysfunction and reduced metabolism [e.g., (53, 54)]. This creates a possible alternative explanation for the increased PK11195 uptake demonstrated in Nakatomi et al. (8). If metabolism is reduced in ME/CFS patients relative to healthy controls, the radioligand would be metabolized more slowly in patients. This means that more radioligand would reach the brain for the simple reason that more remains circulating from the original injection. This problem is made worse by low-brain-penetrance radioligand such as PK11195 as opposed to second-generation radioligands such as PBR28. The use of A-line sampling during scanning can provide an ongoing measure of arterial radioligand availability, allowing any individual differences in radiotracer metabolism to be taken into account.
Activated peripheral immune cells bind radioligand and can differ in quantity across cases and controls
While PBR28 has improved non-specific binding, the antigen that it binds to can occur in non-target tissues and in blood. Neurologists, neuroimmunologists, and neuroscientists use PET radioligands that bind to TSPO because TSPO is produced by activated microglia, the resident tissue macrophages of the central nervous system. However, there are many different kinds of tissue macrophages as well as macrophages in general circulation, and these cells also produce TSPO. Many medical conditions are associated with changes in TSPO expression within different peripheral organs [e.g., (55–58)]. Use of an A-line protects against the possibility that group differences in circulating cells, molecules, and tissue macrophages (possibly due to comorbid conditions) cause differences in peripheral TSPO binding, thereby leaving less TSPO-binding radioligand capable of reaching the brain.
Genetic Analysis of the TSPO Gene
A single nucleotide polymorphism (SNP) in the TSPO gene causes differential radioligand binding
In vitro studies demonstrate that PK11195 and second-generation TSPO-binding radioligands have different binding sites on the TSPO protein (59). The gene for TSPO (Ala147Thr) can have different polymorphisms, including the rs6971 SNP which significantly explains the binding affinity of second-generation TSPO PET radioligands (60, 61). The literature has therefore described a trimodal binding affinity distribution in terms of high-affinity binder (HAB), low-affinity binder (LAB), and mixed affinity binder (MAB) subjects. Because they used PK11195, which binds to a different site on TSPO, Nakatomi et al. (8) did not need to report genetic analysis of the rs6971 SNP. Practically speaking, it is unlikely that replication efforts would be confounded due to the accidental recruitment of all HAB patients and all LAB controls but this is a potential confound that must be ruled out. Therefore, all efforts to replicate and expand upon the pioneering work of Nakatomi et al. (8) should report genetic analyses.
Control Group Selection and Discriminant Validity
An important goal for the ME/CFS field is to find objective biomarkers for both symptom severity and diagnosis; TSPO as measured by PET radioligand binding is one such potential biomarker. Diagnostic biomarkers must show discriminant validity, that is (assuming for a moment that ME/CFS is one entity), they must be able to differentiate ME/CFS from other medical conditions. Nakatomi et al. (8) reported increased PK11195 signal in ME/CFS patients relative to healthy controls, as opposed to mechanistically relevant disease conditions or sedentary controls. An important consideration is that PET studies have shown increased TSPO radioligand uptake in many different neurological and psychiatric conditions, such as autism, traumatic brain injury, major depression, bipolar disorder, Parkinson's disease, chronic pain, multiple sclerosis, and schizophrenia [e.g., (62–66)]. This represents another reason to include non-healthy control groups in studies of putative ME/CFS biomarkers. Furthermore, there is some evidence from a rodent model that translocator protein radioligand uptake may be influenced by exercise (67), which is another argument for the importance of including sedentary controls in studies of ME/CFS. The use of sedentary-matched controls is an important consideration in all studies for which it is possible, not just PET scan studies.
Magnetic resonance spectroscopy (MRS) in neuroinflammation
MRS Can Complement PET for Studying Neuroinflammation
PET is a highly sensitive neuroimaging method, capable of detecting very subtle biological changes that would be missed by other imaging modalities. PET is also capable of quantifying specific neuroinflammation-relevant biological targets such as TSPO. However, there are also multiple downsides to this method, some of which are not present with magnetic resonance spectroscopy (MRS), a neuroimaging technique that uses the MRI modality. Like PET, MRS is capable of measuring the concentration of specific biochemicals. We discuss the mechanisms of MRS here, followed by some of the relative advantages and disadvantages of MRS vs. PET in the study of neuroinflammation.
MRS can measure the relative concentrations of a variety of biochemicals, often referred to in the MRS literature as “metabolites.” This can be accomplished with a powerful magnet because chemicals vary in the density of electrons surrounding their nuclei. Therefore, a strong magnetic field “bounces” back from each metabolite in a signature way, and this can be measured by the MR computer: differences in the reflected magnetic fields can be converted into a readable output spectrum. MRS methods are currently capable of detecting a few dozen metabolites with known spectral properties, and MRS researchers choose from this list of metabolites when designing their analyses. After an a priori decision to focus on a particular part of the spectrum, metabolites are generally reported as a ratio (one metabolite vs. another reference metabolite) as opposed to an absolute concentration. These are among the reasons that MRS is not nearly as sensitive or specific as quantitative measurement of PET radioligand uptake, but there are also some ways in which MRS has advantages over or can complement PET when they are acquired together.
PET is somewhat invasive because PET radioligands must be injected; patient discomfort can increase if an arterial line is used for quantitative measurement. MRS, on the other hand, requires neither an injection nor radiation. Largely because PET radioligands have a short radioactivity half-life, they must be made on-site or near imaging facilities. This is a limiting factor especially for radioligands that are not yet approved for clinical use, because most hospitals with PET scanners would not have access to experimental radioligands. These are among the reasons PET studies are generally more than twice as expensive as studies using MRI-based methods such as MRS. Furthermore, due to the radiation involved in PET procedures, only a limited number of research scans per year are allowed for each participant, whereas there is no such limitation for MRI or MRS scanning. Relatedly, study recruitment can be more difficult when a protocol calls for an injection of radioligand or an A-line. A small number of facilities have access to dual MR-PET scanners, which can combine modalities in a single scanning session (68, 69). This can allow the discovery of MRS correlates to sensitive PET signal. As an example relevant to ME/CFS, in a neuroinflammatory process, one would expect both microglia and astrocytes to become activated. TSPO is produced by activated microglia but most evidence shows that it is not as strongly produced by astrocytes. MRS is capable of measuring inflammation-associated chemical changes beyond only microglial activation, including in astrocytes. With a dual MR-PET scanner, signal from MRS and TSPO-binding radioligand can be measured in the same patient at the same time, helping to better clarify the relationship between their respective neuroimaging signals.
Importance of a Priori Decisionmaking in MRS Studies
Similarly to how different colors occupy a different place along the visible light spectrum, MRS-detectable metabolites each occupy a different place along the magnetic resonance spectrum. However, unlike the human eye's ability to detect the entire visible light spectrum at once, MRS must be somewhat targeted to a limited window within the whole spectrum. If study participants were capable of spending unlimited time in a scanner, all metabolites could theoretically be measured in the entire brain but in reality, researchers must make thoughtful hypothesis-driven decisions about what spectra to measure and in which specific brain regions. If researchers are interested in testing the hypothesis of neuroinflammation in ME/CFS, these decisions should be based in the human neuroinflammation literature.
In some cases two metabolites almost overlap on the spectrum, while in other cases a given metabolite is quite distant from the others. Each of these scenarios presents a unique problem that must be considered before data acquisition begins. Two relatively “distant” metabolites like lactate and NAA cannot be captured with good resolution in the same scan sequence. On the other hand, glutamine, glutamate, and gamma-aminobutyric acid (GABA) are so close together that they can appear as a single peak in the MRS output unless that region of the spectrum is deliberately targeted. If a researcher is interested in understanding the relative contributions of glutamine, glutamate, and GABA, she must make that decision before the experiment begins and focus acquisition directly on the area of the spectrum where these metabolites exist. Furthermore, a priori decisions about which brain structure to measure are also important.
MRS spectra can be recorded from a “slice” of brain or from a single voxel (the 3-dimensional MRI analog to a “pixel”), each of which takes about 15–25 min to acquire. Slices cover more anatomy but have the disadvantage of including several different types of tissue within the same slice (i.e., white matter, gray matter, blood vessels, and ventricles/cerebrospinal fluid). This is a problem because the spectral signal represents an average over the measured area, and different types of tissue have different metabolite concentrations. Therefore, if multiple tissue types are in the same region, interpretation becomes difficult. With thoughtful placement, single voxel MRS has the ability to include only one tissue type, but only from a very tiny section of anatomy (e.g., 1mm3). The spectra recorded from slice or single voxel MRS is usually reported as a ratio of one metabolite relative to another, which can then be compared across different brain regions or in patients vs. controls.
MRS Studies in ME/CFS
Several MRS-detectable metabolites are fairly well validated proxies for inflammation, metabolism, and brain health, and are therefore of particular potential interest for studying neuroinflammation in ME/CFS. A few studies have used MRS imaging in ME/CFS (see Table 1). These studies have looked in a wide variety of brain regions, measuring a wide variety of metabolites (70, 72–79, 81). Brief descriptions of measured metabolites are listed here.
Choline is important in the maintenance of membrane health, and therefore is a potential marker of BBB status (82). It is considered a marker for neuroinflammation because of its relationship to glial activation and BBB permeability (33).
Creatine is a critical regulator of energy homeostasis in the brain [e.g., (83)]. It is believed to have static levels throughout the brain of healthy individuals and is therefore often used as the standard to which other metabolites are normalized (33). Creatine and phosphocreatine are close enough on the spectrum that they are usually pooled.
Gamma-amino butyric acid (GABA) is an inhibitory neurotransmitter and has been linked to reduced cognitive ability (84).
Glutamate is the primary excitatory amino acid in the nervous system, and is produced by activated glial cells. Glutamate levels vary with a number of neurological disorders (85).
Glutathione is involved in the oxidative and nitrosative stress pathways as an antioxidant (86). Oxidative damage and inflammation are generally associated with low glutathione.
Lactate is an end-product of oxidative metabolism and is therefore a potentially interesting biomarker for a metabolism-associated illness such as ME/CFS. Lactate levels in healthy brain tissue are so low as to be almost undetectable by conventional MRS at 1.5T or 3T magnet strength, but when measured in ventricular cerebrospinal fluid, elevated lactate is associated with neuroinflammation (33, 87–92).
Myo-inositol is a carbocyclic sugar residing largely in astrocytes, and is upregulated during astrocyte activation (33). This makes myo-inositol a potentially interesting complement to PET scan studies that use TSPO-binding radioligand to measure microglial activation. Myo-inositol also upregulates during myelin decay (93).
N-acetyl acetate (NAA) production occurs in the mitochondria. Because this metabolite is found in the cytoplasm of neurons, it is considered a marker of neuronal density and therefore often used as a rough marker of brain health (82). However, NAA's normal metabolic and neurochemical functions remain incompletely understood and therefore its relationship to different disease states is controversial and complicated (94).
As evident in Table 1, there is not a clear and consistent characterization of metabolite alterations in ME/CFS. This is not because of failed replication attempts, but rather due to wide a variety of experimental designs, diagnostic criteria selection, subject populations (e.g., juvenile vs. adult), comparison control groups (e.g., healthy, fibromyalgia, or anxiety disorder), brain regions examined, and metabolites targeted.
Regarding MRS metabolite targeting: change in a given metabolite is usually reported as a ratio, relative to a chosen reference baseline. For meaningful interpretation, this requires the reference metabolite (i.e., the ratio denominator) to be stable. Due to its stability in healthy individuals, creatine is the most commonly used ratio reference metabolite, and it is the most commonly used ratio reference in studies of ME/CFS (see Table 1). However, creatine may not be ideal to use in an undercharacterized condition such as ME/CFS. Use of creatine as a ratio reference in case control studies is based on the assumption that its levels will not differ between cases and controls (i.e., interpretation of numerator changes relies upon confidence that the denominator is constant). However, creatine alterations have been reported in autism (95), a condition that may have some mechanistic (and therefore metabolite) similarities with ME/CFS. Autism, like ME/CFS, is a neuroinflammation-associated condition with large sex differences in prevalence, and sensory overload symptoms. Interestingly, an MRS study found sensory sensitivity symptoms in autism to correlate with phosphocreatine abnormalities in thalamus, a brain structure central to sensory filtering and processing (96).
Thalamus is one example of a neuroinflammation-associated a priori region of interest (19) that remains relatively understudied in ME/CFS. The choices of brain regions listed in Table 1 generally do not appear to be based in neuroinflammation-specific hypotheses. Given the fact that early MRS studies of ME/CFS have largely been exploratory, this is somewhat understandable. However, given the putative importance of neuroinflammation in this condition, we believe that the ME/CFS neuroimaging field could benefit from basing a priori targeting of brain regions of interest in the newly emerging human neuroinflammation literature recently meta-analyzed by Kraynak et al. (19), and described above. One MRS study from the human neuroinflammation literature could be a particularly important guide for a priori decisions regarding target brain regions, given the field's focus on the possible importance of peripheral proinflammatory cytokine signaling.
Lessons for MRS Studies of ME/CFS FROM a Study of Inflammatory Challenge in Healthy Humans
One study reviewed by Kraynak et al. (19) was Haroon et al. (97), which investigated the brain response, as measured by MRS, to injection of peripheral proinflammatory cytokine. This type of translational research seems particularly relevant to the ME/CFS field, which has long pursued evidence of neuroinflammation driven by circulating proinflammatory cytokines.
Validating a large animal literature [e.g., (98)], newer human studies have demonstrated that exogenous proinflammatory cytokines (e.g., injected IFN-α) or other immune challenges (e.g., injected typhoid vaccine) can influence behavior and fMRI brain activity in otherwise healthy humans [e.g., (99–102)]. These papers each reported increased BOLD (blood oxygen level-dependent) response in basal ganglia and dACC after challenge. However, the specific biological basis of these BOLD response alterations was not known. A clarifying question would be if brain metabolites, as measured by MRS, were also altered by exogenous proinflammatory cytokine injection or peripheral immune challenge.
IFN-α is frequently used as a treatment for hepatitis-C, and has a fairly common side effect of inducing depressive episodes or a possibly ME/CFS-relevant neurovegetative syndrome including profound fatigue (103). In order to better understand the mechanisms behind this cytokine-induced side effect, Haroon et al. (97) used MRS to investigate the effect of IFN-α injection on basal ganglia and dACC. They recruited 31 hepatitis-C virus positive individuals, who were separated into two groups: IFN-α injection vs. no injection. Both groups were assessed at baseline and again after a month. Relative to the control group, the injection group experienced increases in subjective depression and fatigue, peripheral blood inflammatory cytokines TNF and sTNFR2, and increased MRS signal for glutamate in the dACC and the left basal ganglia. No statistically significant correlations were found between brain MRS signal and inflammatory cytokines circulating in blood. Unfortunately, the authors did not report brainstem results and did not conduct brainstem-specific analysis. Based on this study and the Kraynak et al. (19) meta-analysis, basal ganglia and dACC are attractive a priori regions of interest in brain scan studies interested in using MRS scans to examine neuroinflammation-related changes in ME/CFS patients vs. matched controls.
Peripheral cytokines in ME/CFS
Brain scans are expensive and require many hours of analysis before they are interpretable. Therefore, the discovery of a cheap, easy-to-obtain biomarker from peripheral blood would be an attractive alternative. One common blood measure in ME/CFS studies are cytokines, a broad class of inflammation-related signaling molecules comprising interferon (IFN), tumor necrosis factors (TNF), chemokines, lymphokines, and, most commonly, interleukins (IL). The ME/CFS field has pursued cytokine research in the hopes of finding a blood test that is capable of diagnosing or measuring symptom severity. Blundell et al. (104) recently reviewed this cytokine literature and explained their motivation: “Here we focus on circulating cytokines and we seek to determine whether a pro-inflammatory circulating cytokine profile exists in patients with CFS in comparison to controls and how this cytokine profile differs from controls following stimulation such as exercise.” Thus, a consistent and replicable “cytokine profile” would be a diagnostic biomarker, and further, would be evidence for an inflammatory process at the root of ME/CFS pathophysiology. However, at the conclusion of their literature review, the authors reported that they did not find a consistent “cytokine profile” in ME/CFS. In this section, we will make the argument that a lack of consistent “cytokine profile” is an inevitable result of 1) the way that cytokines actually function biologically and 2) the methods used to measure cytokines. We end with recommendations that will hopefully allow more meaningful comparisons in the future.
Biological Mechanisms Limit the Value of Peripheral Blood Cytokines as a Stable Biomarker
Cytokines are a communication factor released by activated innate immune cells such as macrophages and mast cells in the periphery, as well as glia and endothelial cells on the brain side of the BBB. This cytokine signaling is a key component of the sickness response (22, 98), which has symptoms that overlap with key ME/CFS symptoms (15). Relatedly, cytokines are a key component of neuroinflammation; one of the key ways peripheral inflammation triggers neuroinflammation is when the vagus nerve detects peripheral cytokines [e.g., (105, 106)]. Thus, cytokines are a class of molecule that, at first blush, seem to hold promise as a potential peripheral biomarker for neuroinflammation in ME/CFS. However, the way that cytokines actually function mechanistically tarnishes some of this promise.
The core problem with looking for cytokines in peripheral blood is that cytokines generally do not function as endocrine signalers, but are rather normally autocrine and paracrine signalers (see Box 1). In other words, cytokines do not function by flowing through blood (where many studies hope to measure them due to easy access) but rather by acting locally, directly in the vicinity of infection or injury. Cytokines do not need to function as circulating endocrine molecules to drive subjective sickness symptoms because they can be detected by the sensitive and highly branched afferent vagus nerve, which communicates their presence to the brain via brainstem and triggers neuroinflammation and sickness responses (15, 105, 106). A large neuroimmunology literature consistently concludes that cytokines do not have to be detectable in the periphery in order to have an effect on sickness-related symptoms. For example, Campisi et al. (107) stated, “Elevated levels of circulating cytokines and endotoxin are not necessary for the activation of the sickness or corticosterone response.” Another fact of cytokine biology that makes a stable, predictable blood profile difficult is that cytokine-cytokine interactions are in constant dynamic flux and are exquisitely complicated (108), and their levels can be affected by a huge number of variables (reviewed below). Furthermore, as relatively large, lipophobic, polypeptide protein molecules, cytokines generally do not easily diffuse across an intact BBB and thus, circulating levels do not accurately reflect brain cytokine levels. Therefore, a peripheral cytokine profile may not be meaningful in informing any existing central nervous system cytokine profile. This general point is made by many papers in the cytokine methods literature: there is limited ability for a putative “cytokine profile” to inform underlying disease processes.
Box 1.
Despite the limited value of measuring blood cytokine levels in understanding pathophysiology and neuroinflammation, blood cytokine levels are used as a dependent variable in many ME/CFS studies, probably due to ease of collection. The cytokine methods literature emphasizes the need for optimization and standardization of collection, storage, and assay methods, but these factors have varied widely in ME/CFS cytokine studies. For this reason, previous studies of peripheral cytokines in ME/CFS cannot be meaningfully compared as Blundell et al. (104) set out to do.
Cytokine Studies in ME/CFS as Reviewed by Blundell et al. 2015 (104)
The limitations of ME/CFS cytokine studies can be seen in the recent literature review by Blundell et al. (104), which aimed to “determine if a pro-inflammatory circulating cytokine profile exists in ME/CFS patients relative to controls.” Here we give a brief overview of the Blundell et al. review, and then we detail the assay methodology used in the ME/CFS cytokine literature, using the studies from the Blundell et al. review and studies published since then (see Table A1).
Blundell et al. (104) published a systematic review but were not able to conduct a conventional meta-analysis due to dissimilarities among reviewed studies. The authors began with a quality assessment, finding that 14 out of 38 reviewed studies were of poor quality due to failure to control for one or more items on a list of confounding factors that can influence cytokine levels: age, subject activity level, BMI, gender, menstrual cycle stage, comorbid diseases, antidepressant use, or diurnal variation. However, beyond those confounds, the study designs differ so much that any comparison may not be meaningful (e.g., comparing sleeping patients to exercising patients).
Despite the lack of consistent study design, the authors concluded that there is “little or no evidence to support the hypothesis that proinflammatory circulating cytokines are raised in CFS” (104). They reasoned that a failure to find consistent results across studies could be due to heterogeneity in the ME/CFS population, or due to the local rather than systemic role of cytokines in ME/CFS. While these are reasonable explanations, we would argue that the reviewed studies show such inconsistencies in cytokine measurement methods that consistent findings would be impossible even if they shared comparable research designs.
Blundell et al. (104) briefly noted the different assay types (i.e., bioassay vs. immunoassay) and sample matrices (i.e., serum vs. plasma) across studies. However, beyond these two measurement issues (and the short list of potential confounds mentioned above), a large cytokine methods literature demonstrates a staggering number of potential confounds in the measure of cytokines, with potential problems arising at every step of the way. Here, we describe the importance of additional factors in the collection, handling and processing, storage, and assaying of cytokines (detailed in Table A1).
Methodological Confounds That Must Be Considered Before Comparing Cytokine Studies
The biological mechanisms of cytokines make a consistent and stable circulating profile unlikely, which limits the ability of peripheral cytokines to provide insight into underlying pathophysiology in ME/CFS. Therefore, a peripheral cytokine profile is unlikely to be a feasible and useful biomarker. In addition to these biological factors, there are many methodological problems.
• Even if cytokines were meaningful peripheral biomarkers: Blood cannot be compared to cerebrospinal fluid
• Even within blood sampling: Venous and arterial blood samples cannot be compared
• Even if blood sampling methods were equal: Plasma, serum, and PBMC sample matrices cannot be compared
• Even if ME/CFS researchers use a consistent sample matrix: Bioassay, ELISA, and multiplex assay results cannot be compared, even across kits of the same assay type
• Even if ME/CFS researchers standardize their methods: The same exact lab, personnel, and protocol will likely get different results from the same manufacturer's kit
The relevant details of methods used in previous studies of cytokines in ME/CFS are listed in Table A1. Importantly, this table adds to the number of factors that were listed by Blundell et al. (104) to clearly display the widespread variance of cytokine methodology in the ME/CFS literature. The intention of this section is to show that (1) currently-existing studies in the ME/CFS cytokine literature cannot be meaningfully compared due to differences in methods and (2) the ME/CFS field must consider the mechanisms of cytokines and establish some consistency in methods for any role of cytokines in ME/CFS to be elucidated.
Blood Cannot Be Compared to Cerebrospinal Fluid
Choosing between blood and cerebrospinal fluid is the first point of potential variability in attempts to identify a cytokine profile, as the concentrations of various cytokines are not necessarily equivalent across body fluid sample types. Most ME/CFS studies have analyzed cytokines from peripheral blood samples (see Table A1). Less frequently, others have analyzed cytokines from cerebrospinal fluid (119, 129, 177, 178). In addition to many examples of this phenomenon in the rodent literature [e.g., (179–181)], human studies have also demonstrated that cytokine concentration in cerebrospinal fluid vs. blood can differ, with some examples showing a positive correlation, some showing lack of correlation, and some showing anticorrelation [e.g., (182–186)]. Because the presence of cytokines usually reflects local rather than systemic conditions (see Box 1), measuring cytokines from the cerebrospinal fluid is a more direct representation of the central nervous system environment than from peripheral blood. Therefore, for studies interested in ME/CFS neuroinflammation, cerebrospinal fluid sampling is more likely to be useful. However, because cytokines are locally-acting paracrine and autocrine factors, one cannot assume that a sample of cerebrospinal fluid taken during a lumbar puncture spinal tap accurately reflects the entirety of the central nervous system cerebrospinal fluid. For example, Milligan et al. (179) reported IL-1 detection in cerebrospinal fluid samples taken from the lumbosacral region but not from the cervical region.
Venous and Arterial Blood Samples Cannot Be Compared
Because they are produced and removed in local tissues, cytokines differ in concentration between venous blood samples (which have been filtered through organs and tissues) and arterial blood samples [taken before that filtration; (187)]. Additionally, there is a difference between blood samples taken from an indwelling cannula and a single needle stick. An indwelling cannula causes an immune response that can alter local cytokine production, and thus the resulting cytokine measurements may reflect local artifact rather than systemic change in concentrations (188). These are important factors to consider when designing and interpreting cytokine studies.
Plasma, Serum, and PBMC Sample Matrices Cannot Be Compared
When using peripheral blood samples, assays can be conducted on different sample matrices: whole blood, plasma, peripheral blood mononuclear cell (PBMC) isolate, or serum.
Whole blood can be:
• Collected into a tube with anticoagulants and then centrifuged. The resulting layers allow separation of plasma and of PBMCs.
• Collected into a tube without any additives and then centrifuged. After clotting factors are removed, the resulting liquid is serum.
During the processes required to make plasma or serum from blood, cells in the blood secrete inflammatory mediators that can alter cytokine measurements. For example, plasma preparation involves the removal of many proteins (e.g., fibrinogen), including the direct removal of circulating cytokines, obviously altering sample cytokine levels (189). During the coagulation process necessary for serum isolation, platelets release vascular endothelial growth factor, which can significantly alter cytokine levels (190). These are among the reasons that any attempt to compare cytokine levels across studies must take type of sample matrix into account.
Many other variables during sample handling and processing can affect cytokine levels in the sample matrix, including glass vs. plastic vials, type of anticoagulant (e.g., heparin, citrate, or EDTA), and centrifugation speed (190). Many studies in the ME/CFS cytokine literature differ in these details, limiting their comparability. Perhaps the most important methodological details involve time and temperature. Because both rapid degradation and de novo production of cytokines and other proteins occur inside of sample tubes (187, 191), without fast and careful processing, cytokine measurements may reflect processes that happened inside of a sample tube and not what happened in the bodies of study participants. While there is no way to completely avoid these confounds (192), these processes are greatly curtailed at −80°C but not at −20°C, meaning handling speed and storage temperature are crucial. The studies reviewed in Blundell et al. (104) ranged from immediate to 4 h between collection and plasma/serum separation, with many not reporting timing. Furthermore, 25 out of 57 studies in the ME/CFS cytokine literature either stored samples at −20°C or failed to report storage temperature at all (see Table A1). Thus, methodological details such as tubes, anticoagulants, centrifugation, and delays in processing are likely sources of type 2 error in the ME/CFS cytokine literature and limit the comparability across studies.
Bioassay, ELISA, and Multiplex Assay Results Cannot Be Compared, Even Across Kits of the Same Assay Type
After cytokine study samples have been collected, processed, and stored, they must be assayed. The assay methods for cytokine measurement have evolved over the past decades, and that evolution explains some current priorities in ME/CFS research. Bioassays are a form of assay that utilizes the biological activity of its target analyte to measure its concentration, while both enzyme-linked-immunosorbent assay (ELISA) and multiplex are immunoassays that usually use tagged antibodies. ELISA is the most commonly used method in ME/CFS (in the ME/CFS literature, all cytokine studies before 2007 were performed using bioassay or ELISA methods), but multiplex are becoming more common. ELISA formats are singleplex, meaning they characterize a single analyte (i.e., a single cytokine) while newer multiplex assays can measure many at the same time. Historically, ELISA is considered the gold standard because each kit can optimize sensitivity and specificity for the single specific cytokine being measured, and optimize for an expected concentration range (187).
Multiplex immunoassay methods are a more recent development, allowing for a larger number of cytokines (i.e., from 2 to 100+) to be characterized in the same assay. This is a seemingly-appealing option with the potential for identifying a putative cytokine profile in a complex multivariable disease, such as ME/CFS, that likely cannot be characterized by a single cytokine or other analyte. The ME/CFS literature has followed the advancing technology, generally shifting to multiplex. However, multiplex sacrifices quality for quantity. Because all cytokines are measured in the same multiplex kit well, there is inevitably cross-reactivity among the antibodies, and non-specificity with other non-cytokine proteins in the sample. Each manufacturer could theoretically optimize a select number of cytokines, but not all of them (e.g., the most sensitive and specific antibody for a given cytokine would have to be replaced by another antibody that is less cross-reactive). Companies also continuously develop new, revamped kits that cannot necessarily be compared to previous versions manufactured by the same company. In other words, one manufacturer's newest multiplex kit model may be particularly good at measuring IL-1β and bad at measuring TNF-α, while the inverse is true for that manufacturer's previous model, or another manufacturer's newest kit model. Furthermore, there can be a large range of concentrations among various cytokines in a given sample, and multiplex kits are unable to maximize sensitivity across that range. Therefore, a given kit may be relatively good at measuring high concentrations of IL-1β but lack sensitivity at lower levels. These forms of variance are true across the scores of cytokines each manufacturer advertises an ability to measure.
Currently, there are no standardized regulatory guidelines for the quality and validity of multiplex assays (193). Concordance between ELISA and multiplex varies widely and is especially poor if plasma or serum is used (189, 194); these are the most common sample types in ME/CFS, meaning ME/CFS studies using ELISA cannot be meaningfully compared to those using multiplex. Until multiplex methods are standardized, the best-case (but impractical) scenario for a researcher interested in 20 specific cytokines would be using 20 separate ELISA kits as opposed to using a 20-cytokine multiplex kit. However, absolute cytokine concentrations would not be comparable across studies if different researchers were to use kits from different manufacturers (195, 196). This is exactly what has happened in the ME/CFS literature, where many different kit manufacturers have been used (see Table A1). Cross-manufacturer differences in reported absolute values of cytokines occur because they are completely dependent on the standard curves from each kit, and studies have shown significant variation in standard curves across different manufacturers (195, 196). Taking all variables into account, it is unsurprising that many studies have found profound differences in absolute cytokine levels across manufacturers and kits, even when compared on the same sample (196–201). This clearly limits the ability for different studies in the ME/CFS cytokine literature to be compared.
Table A1 lists the various manufacturers and kit models used in the ME/CFS cytokine literature. Blundell et al. (104) correctly identified the importance of bioassay vs. immunoassay for a single cytokine (TGF-β), but this distinction was not made for any other cytokine. Furthermore, the distinction was not made between ELISA and multiplex immunoassays, nor was manufacturer or kit model taken into account for any cytokine. These details introduce enough variance as to make any attempted comparison of absolute cytokine concentrations in the ME/CFS literature indecipherable. A seemingly reasonable solution would be for all research groups to use the same assay kit model from the same manufacturer. However, we believe that peripheral cytokines are a fundamentally noisy variable and that this fact must be taken into account when considering the implications of any cytokine study.
The Same Exact Lab, Personnel, and Protocol Will Likely Get Different Results From the Same Manufacturer's Kit
Assuming that there actually is a predictable, consistent peripheral “cytokine profile” in a complex illness such as ME/CFS, one potential solution to some of the above-described issues is if a single lab were to use the exact same techniques, equipment, and procedures across multiple studies, or if different labs standardized these procedures. However, empirical evidence shows that this is not the case. An experienced immunology lab, led by a PI with decades of experience and over 100 publications, conducted a within- and between-lab comparison study. Breen et al. (198) compared the ability of four multiplex kits to detect 13 cytokines in human plasma and serum. The four kits were tested on the same sample across six different laboratories and across multiple lots of the same kit. Their results showed a large amount of variance both within the same lab and across multiple labs. While all 13 cytokines were detected by at least one kit, none of the kits were able to detect all 13 cytokines. Additionally, their results alarmingly indicate that each cytokine within each multiplex kit had at least one significant lab and/or lot effect. In other words, measuring the same sample twice with the same kit in the same laboratory following the same strict protocol yielded significant differences in absolute cytokine values (Figure 2).
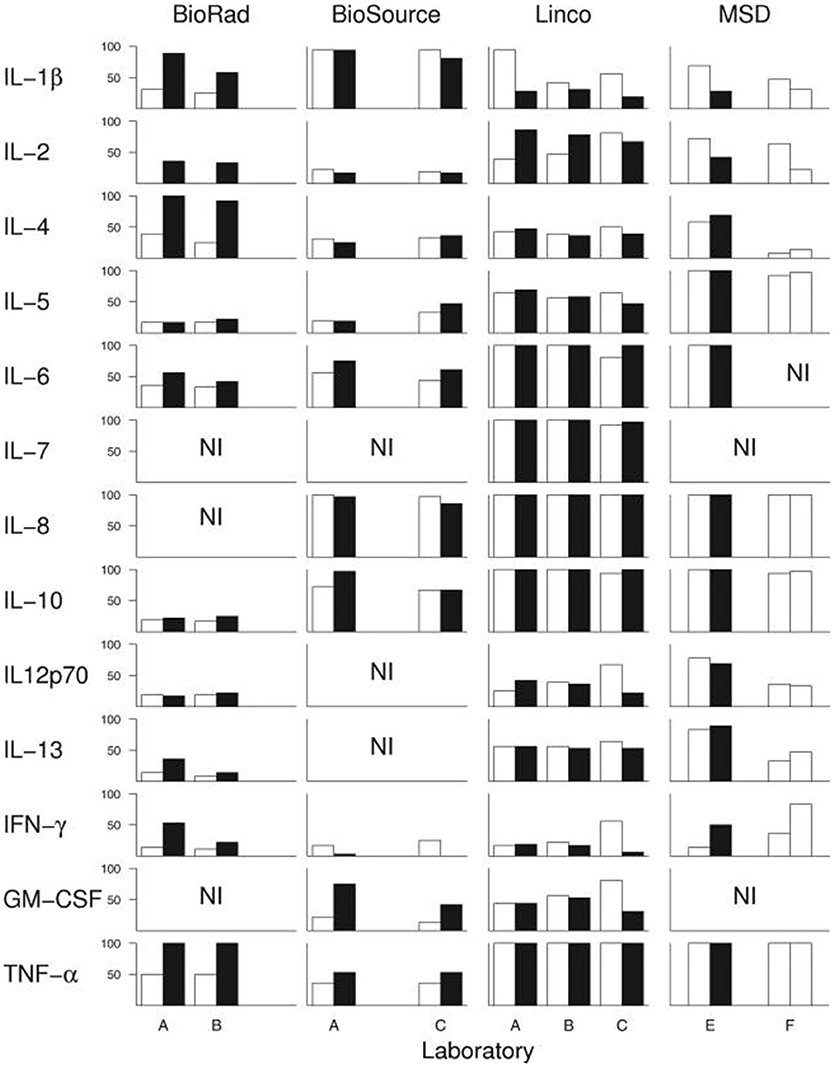
Figure 2. Breen et al. (198) conducted an experiment to test whether widely-used cytokine assays yield consistent results for 13 different cytokines. The same laboratories ran four different multiplex cytokine assay kits more than once on the same serum samples. Black and white bars represent assay kit data from different lots. Bars indicate percentage of serum samples (n = 36) with detectable levels of the indicated cytokine. A-F denote the six different labs in which the assays were conducted. NI: cytokines not included in each kit. Figure reproduced from Breen et al. (198). Reproduced with permission from American Society for Microbiology.
However, the results of the comparison demonstrated that while each of the kits varied in their sensitivity to detect the absolute concentration of cytokines, the kits detected similar cytokine patterns (relative concentrations, as opposed to absolute concentration). These findings contribute to our recommendation that cytokine assays are best suited to measuring relative changes in cytokine concentrations in a within-subject study design, rather than comparing absolute concentrations across groups (described below).
Cytokines Can Be Highly Influenced by Individual Behavior
A final note of warning against overinterpreting studies of peripheral cytokines is that study participants can contribute noise in myriad ways. Factors that can significantly affect circulating cytokine levels within an individual include: time of day (202–204), status of alcohol, nicotine, or other drug use (205–211), quality and amount of sleep (212), acute and chronic stress (213), acute and chronic fitness habits specific to type of exercise (214–216), sex (217, 218), phase of menstrual cycle (219, 220), age (221), chronic dietary patterns (222), and acute differences immediately following a meal (223, 224). Thus, even eating a spicy burrito with extra guacamole the day of sample collection will result in a different cytokine profile than eating Indian food or a slice of chocolate cake. A research participant adding sour cream to the mashed potatoes they had for lunch will alter their cytokine profile. Capsaicin, the main source of heat in hot peppers, alters levels of IL-6, IL-10, TNFα, NOx, and MDA (225), and the natural sugars in avocado alter gene expression of IL-1α, IL-6, and IL-8 (227, 228). The bacteria used in dairy (i.e., the sour cream on the mashed potatoes) increase IL-1β, TNFα, and IFNγ (229, 230). Cumin, a spice commonly used in Indian cuisine, reduces expression of inflammatory cytokines CXL-1 and−2, TNFα, IL-1β, IL-6, and IL-18 (231, 232). Chocolate increases IL-10 and IL-1β (233). Clearly, cytokines can be affected by a huge number of variables unrelated to disease.
This type of variance, driven by individual behaviors, could be reasonably well explained in a single study using a within-subjects design. However, it can prevent comparability across studies that use different designs. For example, a study that collects blood samples during fasting cannot be compared to studies of non-fasting individuals undergoing exercise challenge. This type of variability in study design is widespread in the ME/CFS cytokine literature (see Table A1).
Are Peripheral Circulating Cytokines Useful at All?
Given how cytokines work biologically, we do not believe that a consistent and stable proinflammatory circulating cytokine profile exists in patients with ME/CFS in comparison to controls, nor do we believe that finding such a profile is a realistic goal. Cytokines do not normally function as circulating endocrine molecules, and their presence in the periphery mostly represents spillover from their actual site of action. This biology also limits the value of any peripheral cytokine profile in elucidating the underlying pathophysiology of ME/CFS or any other chronic inflammatory condition. Cytokine measurement in the periphery is beset by innumerable confounds: biological, methodological, and behavioral. Detailed reporting of methods will help inform comparability across studies, while study designs with within-subjects measurements across multiple timepoints can help explain some of the behavioral variance.
We would argue that the most effective way to use peripheral cytokines in the characterization of ME/CFS patients is through within-subject or mixed-model challenge study designs (e.g., measuring before and after an exercise challenge, with BMI- and daily activity-matched controls). In such a study, cytokine levels would be most meaningful as a complementary measure, as opposed to a primary outcome measure. For example, cerebrospinal fluid could be sampled at both timepoints in a study measuring cognitive performance at baseline and during post-exercise symptom provocation. In such an example, if cognitive performance negatively correlates with a general increase in proinflammatory cytokines, this is indirect evidence that neuroinflammation is part of “brain fog.” This approach moves the focus of cytokine studies away from whether a distinct cytokine profile exists in ME/CFS patients, and toward the use of cytokines for understanding the mechanisms of key symptoms.
Conclusion
The above review focused on neuroinflammation and the methods used to measure it. We argued for the importance of anchoring methodological details in known biological mechanisms and existing research literature.
The ME/CFS research field has been stuck in a somewhat defensive posture, with a focus on demonstrating “this is a real condition” by showing significant biological differences between patients and controls. We believe this has led to a situation in which too much is made of the specifics reported by descriptive studies (such as the average “cytokine profile” present in cases vs. controls at the moment of assay) and not enough emphasis has been placed on potential mechanisms driving symptoms. The field is ready to move past proving “this is a real condition” and to start elucidating the specific relationship of ME/CFS symptoms to neuroinflammation.
Moving past a defensive posture and toward understanding pathophysiology requires careful focus on research methods. In designing a study, a goal of ME/CFS researchers should be to determine if a significant result can actually inform disease mechanisms, or if it is simply a reportable difference between patients and controls. For example, a PET study of TSPO binding may find differences between patients and controls when using a cerebellum reference, and this holds some value for the “this is a real condition” argument. But because of the difficulty in interpretation, such a study is less valuable for discerning actual pathophysiology.
In consideration of neuroinflammation-related mechanisms and research methods, the following recommendations emerge:
• The relationship of ME/CFS to neuroinflammation is a fundamental question that needs to be directly addressed from multiple research angles.
• The existing neuroinflammation basic science literature should serve as a guide for choosing ROIs in ME/CFS brain scan studies.
• ME/CFS causes changes to patients' lives that could accidentally be explaining some study results (i.e., sedentary lifestyle or diet can affect cytokines). This makes careful selection of control groups particularly important.
• Cytokines seem attractive because they are easy to collect and measure, but are a very noisy variable and the specific findings of any given study should not be overinterpreted.
• Some methodological details are so fundamental (e.g., brainstem registration, or selection of a “baseline” reference brain region or metabolite, or choosing between blood serum and cerebrospinal fluid) that they can be completely responsible for a study's results or lack thereof.
Author Contributions
All authors listed have made a substantial, direct and intellectual contribution to the work, and approved it for publication.
Conflict of Interest
The authors declare that the research was conducted in the absence of any commercial or financial relationships that could be construed as a potential conflict of interest.
Acknowledgments
We would like to thank the generous donors that have supported our work, especially Mona Eliassen and an anonymous family. Special thanks for the support of Lisa Shin Ph.D., Darin Dougherty MD, and the Martinos Center for Biomedical Imaging.
References
1. Cohen J. Goodbye Chronic Fatigue Syndrome, Hello SEID. Science. (2015) Available online at: http://www.sciencemag.org/news/2015/02/goodbye-chronic-fatigue-syndrome-hello-seid (Accessed February 10, 2015).
2. Sigurdsson B. A new clinical entity? Lancet (1956) 267:789–790. doi: 10.1016/S0140-6736(56)91252-1
3. Boffey P. Fatigue ‘Virus' Has Experts More Baffled and Skeptical Than Ever. New York, NY: New York Times (1987) Available online at: www.nytimes.com/1987/07/28/science/fatigue-virus-has-experts-more-baffled-and-skeptical-than-ever.html (accessed July 28, 1987).
4. Steinbrook R. 160 Victims at Lake Tahoe: Chronic Flu-Like Illness a Medical Mystery Story. Los Angeles, CA: Los Angeles Times. (1986) Available online at: http://articles.latimes.com/1986-06-07/news/mn-9956_1_lake-tahoe (Accessed June 7, 1986).
5. Hyde B. The Clinical and Scientific Basis of ME/CFS. Ottawa, ON: Nightingale Research Foundation (1992).
6. Mensah FKF, Bansal AS, Ford B, Cambridge G. Chronic fatigue syndrome and the immune system: where are we now? Neurophysiol Clin. (2017) 47:131–8.
7. Cader S, O'Donovan DG, Shepherd C, Chaudhuri A. Neuropathology of post-infectious chronic fatigue syndrome. J Neurol Sci. (2009) 285:S60–1. doi: 10.1016/S0022-510X(09)70274-6
8. Nakatomi Y, Mizuno K, Ishii A, Wada Y, Tanaka M, Tazawa S, et al. Neuroinflammation in patients with chronic fatigue syndrome/myalgic encephalomyelitis: an 11C-(R)-PK11195 PET study. J Nucl Med. (2014) 55:945–50. doi: 10.2967/jnumed.113.131045
9. Komaroff AL, Cho TA. Role of infection and neurologic dysfunction in chronic fatigue syndrome. Semin Neurol. (2011) 31:325–37. doi: 10.1055/s-0031-1287654
10. Blomberg J, Gottfries CG, Elfaitouri A, Rizwan M, Rosén A. Infection elicited autoimmunity and myalgic encephalomyelitis/chronic fatigue syndrome: an explanatory model. Front Immunol. (2018) 9:229. doi: 10.3389/fimmu.2018.00229
11. Magnus P, Gunnes N, Tveito K, Bakken IJ, Ghaderi S, Stoltenberg C, et al. chronic fatigue syndrome/myalgic encephalomyelitis (CFS/ME) is associated with pandemic influenza infection, but not with an adjuvanted pandemic influenza vaccine. Vaccine (2015) 33:6173–7. doi: 10.1016/j.vaccine.2015.10.018
12. Underhill RA. Myalgic encephalomyelitis, chronic fatigue syndrome: an infectious disease. Med Hypotheses (2015) 85:765–73. doi: 10.1016/j.mehy.2015.10.011
13. Hickie I, Davenport T, Wakefield D, Vollmer-Conna U, Cameron B, Vernon SD, et al. Infection outcomes study group. Post-infective and chronic fatigue syndromes precipitated by viral and non-viral pathogens: prospective cohort study. BMJ (2006) 333:575. doi: 10.1136/bmj.38933.585764.AE
14. Tsai SY, Yang TY, Chen HJ, Chen CS, Lin WM, Shen WC, et al. Increased risk of chronic fatigue syndrome following herpes zoster: a population-based study. Eur J Clin Microbiol Infect Dis. (2014) 33:1653–9. doi: 10.1007/s10096-014-2095-x
15. VanElzakker MB. Chronic fatigue syndrome from vagus nerve infection: a psychoneuroimmunological hypothesis. Med Hypotheses (2013) 81:414–23. doi: 10.1016/j.mehy.2013.05.034
16. Herisson F, Frodermann V, Courties G, Rohde D, Sun Y, Vandoorne K, et al. Direct vascular channels connect skull bone marrow and the brain surface enabling myeloid cell migration. Nat Neurosci. (2018) 21:1209–17. doi: 10.1038/s41593-018-0213-2
17. Goehler LE, Park SM, Opitz N, Lyte M, Gaykema RPA. Campylobacter jejuni infection increases anxiety-like behavior in the holeboard: possible anatomical substrates for viscerosensory modulation of exploratory behavior. Brain Behav Immun. (2008) 22:354–66. doi: 10.1016/j.bbi.2007.08.009
18. Kennedy RH, Silver R. Neuroimmune Signaling: Cytokines and the Central Nervous System. In: Volkow ND, Pfaff DW, editors. Neuroscience in the 21st Century. New York, NY: Springer (2016). p. 601–41.
19. Kraynak TE, Marsland AL, Wager TD, Gianaros PJ. Functional neuroanatomy of peripheral inflammatory physiology: a meta-analysis of human neuroimaging studies. Neurosci Biobehav Rev. (2018) 94:76–92. doi: 10.1016/j.neubiorev.2018.07.013
20. McCusker RH, Kelley KW. Immune-neural connections: how the immune system's response to infectious agents influences behavior. J Exp Biol. (2013) 216: 84–98. doi: 10.1242/jeb.073411
21. Graeber MB, Li W, Rodriguez ML. Role of microglia in CNS inflammation. FEBS Lett. (2011) 585:3798–805. doi: 10.1016/j.febslet.2011.08.033
22. Kelley KW, Bluthé RM, Dantzer R, Zhou J, Shen W, Johnson RW, et al. Cytokine-induced sickness behavior. Brain Behav Immun. (2003) 17 (Suppl 1): S112–8. doi: 10.1016/S0889-1591(02)00077-6
23. Prinz M, Priller J. Microglia and brain macrophages in the molecular age: from origin to neuropsychiatric disease. Nat Rev Neurosci. (2014) 15:300–12. doi: 10.1038/nrn3722
24. Sheng JG, Mrak RE, Griffin WS. Enlarged and phagocytic, but not primed, interleukin-1 alpha-immunoreactive microglia increase with age in normal human brain. Acta Neuropathol. (1998) 95:229–34.
25. Hart AD, Wyttenbach A, Perry VH, Teeling JL. Age related changes in microglial phenotype vary between cns regions: grey versus white matter differences. Brain Behav Immun. (2012) 26:754–65. doi: 10.1016/j.bbi.2011.11.006
26. Hendriksen E, van Bergeijk D, Oosting RS, Redegeld FA. Mast cells in neuroinflammation and brain disorders. Neurosci Biobehav Rev. (2017) 79:119–33. doi: 10.1016/j.neubiorev.2017.05.001
27. Sclocco R, Beissner F, Bianciardi M, Polimeni J, Napadow V. Challenges and opportunities for brainstem neuroimaging with ultrahigh field MRI. Neuroimage (2017) 168:412–26. doi: 10.1016/j.neuroimage.2017.02.052
28. Tracey KJ. Reflex control of immunity. Nature Rev Immunol. (2009) 9:418–28. doi: 10.1038/nri2566
29. Napadow V, Dhond R, Kennedy D, Hui KKS, Makris N. Automated brainstem co-registration (ABC) for MRI. Neuroimage (2006) 32:1113–9. doi: 10.1016/j.neuroimage.2006.05.050
30. Choi J, Ifuku M, Noda M, Guilarte T. Translocator protein (18kDa) (TSPO)/peripheral benzodiazepine receptor (PBR) specific ligands induce microglia functions consistent with an activated state. Glia (2011) 59:219–30. doi: 10.1002/glia.21091
31. Cagnin A, Kassiou M, Meikle SR, Banati RB. Positron emission tomography imaging of neuroinflammation. Neurotherapeutics (2007) 4:443–52. doi: 10.1016/j.nurt.2007.04.006
32. Jaremko M, Jaremko T, Jaipuria G, Becker S, Zweckstetter M. Structure of the mammalian TSPO/PBR protein. Biochem Soc Trans. (2015) 43:566–71. doi: 10.1042/BST20150029
33. Albrecht DS, Granziera C, Hooker JM, Loggia ML. In vivo imaging of human neuroinflammation. ACS Chem Neurosci. (2016) 7:470–83. doi: 10.1021/acschemneuro.6b00056
34. Sandiego CM, Gallezot J, Pittman B, Nabulsi N, Lim K, Lin S, et al. Imaging robust microglial activation after lipopolysaccharide administration in humans with PET. Proc Natl Acad Sci USA. (2015) 112:12468–73. doi: 10.1073/pnas.1511003112
35. Beissner F. Functional MRI of the brainstem: common problems and their solutions. Clin Neuroradiol. (2015) 25:251–7. doi: 10.1007/s00062-015-0404-0
36. Terem I, Ni W, Goubran M, Salmani Rahimi M, Zaharchuk G, Yeom K, et al. Revealing sub-voxel motions of brain tissue using phase-based amplified MRI (aMRI). Magn Reson Med. (2018) 80:2549–59. doi: 10.1002/mrm.27236
37. Brooks JC, Faull OK, Pattinson KT, Jenkinson M. Physiological noise in brainstem MRI. Front Human Neurosci. (2013) 7:623. doi: 10.3389/fnhum.2013.00623
38. Yendiki A, Koldewyn K, Kakunoori S, Kanwisher N, Fischl B. Spurious group differences due to head motion in a diffusion MRI study. Neuroimage (2014) 88:79–90. doi: 10.1016/j.neuroimage.2013.11.027
39. Barnden LR, Kwiatek R, Crouch B, Burnet R, Del Fante P. Autonomic correlations with MRI are abnormal in the brainstem vasomotor centre in chronic fatigue syndrome. Neuroimage Clin. (2016) 11:530–7. doi: 10.1016/j.nicl.2016.03.017
40. Gerrity TR, Bates J, Bell DS, Chrousos G, Furst G, Hedrick T, et al. Chronic fatigue syndrome: what role does the autonomic nervous system play in the pathophysiology of this complex illness? Neuroimmunomodulation (2002) 10:134–41. doi: 10.1159/000067176
41. Van Cauwenbergh D, Nijs J, Kos D, Van Weijnen L, Struyf F, Meeus M. Malfunctioning of the autonomic nervous system in patients with chronic fatigue syndrome: a systematic literature review. Eur J Clin Invest. (2014) 44:516–26. doi: 10.1111/eci.12256
42. Beaumont A, Burton AR, Lemon J, Bennett BK, Lloyd A, Vollmer-Conna U. Reduced cardiac vagal modulation impacts on cognitive performance in chronic fatigue syndrome. PLoS ONE (2012) 7:e49518. doi: 10.1371/journal.pone.0049518
43. Burton A, Rahman K, Kadota Y, Lloyd A, Vollmer-Conna U. Reduced heart rate variability predicts poor sleep quality in a case-control study of chronic fatigue syndrome. Exp Brain Res. (2010) 204:71–8. doi: 10.1007/s00221-010-2296-1
44. Newton J, Okonkwo O, Sutcliffe K, Seth A, Shin J, Jones E. Symptoms of autonomic dysfunction in chronic fatigue syndrome. QJM (2007) 100:519–26. doi: 10.1093/qjmed/hcm057
45. Boneva R, Decker M, Maloney E, Lin JM, Jones J, Helgason H, et al. Higher heart rate and reduced heart rate variability persist during sleep in chronic fatigue syndrome: a population-based study. Auton Neurosci. (2007) 137:94–101. doi: 10.1016/j.autneu.2007.08.002
46. He J, Hollingsworth K, Newton J, Blamire A. Cerebral vascular control is associated with skeletal muscle pH in chronic fatigue syndrome patients both at rest and during dynamic stimulation. Neuroimage Clin. (2013) 2:168–73. doi: 10.1016/j.nicl.2012.12.006
47. Costa DC, Tannock C, Brostoff J. Brainstem perfusion is impaired in chronic fatigue syndrome. QJM (1995) 88:767–73.
48. Barnden LR, Crouch B, Kwiatek R, Burnet R, Mernone A, Chryssidis S, et al. A brain MRI study of chronic fatigue syndrome: evidence of brainstem dysfunction and altered homeostasis. NMR Biomed. (2011) 24:1302–12. doi: 10.1002/nbm.1692
49. Barnden L, Zack S, Donald S, Marshall-Gradisnik S, Finegan K, Ireland T, et al. Hyperintense sensorimotor T1 spin echo MRI is associated with brainstem abnormality in chronic fatigue syndrome. Neuroimage Clin. (2018) 20:102–9. doi: 10.1016/j.nicl.2018.07.011
50. Lockhart DA, Matthews B, Rahmoune JC, Hog H, Gee G, Earnshaw A, et al. The peripheral benzodiazepine receptor ligand PK11195 binds with high affinity to the acute phase reactant alpha1-acid glycoprotein: implications for the use of the ligand as a CNS inflammatory marker. Nucl Med Biol. (2003) 30:199–206. doi: 10.1016/S0969-8051(02)00410-9
51. Herrera-Rivero M, Heneka M, Papadopoulos V. Translocator protein and new targets for neuroinflammation. Clin Transl Imaging (2015) 3:391–402. doi: 10.1007/s40336-015-0151-x
52. Prusty BK, Gulve N, Govind S, Krueger GRF, Feichtinger J, Larcombe RL, et al. Active HHV-6 infection of cerebellar purkinje cells in mood disorders. Front Microbiol. (2018) 9:1955. doi: 10.3389/fmicb.2018.01955
53. Naviaux RK, Naviaux JC, Li K, Bright AT, Alaynick WA, Wang L, et al. Metabolic features of chronic fatigue syndrome. Proc Natl Acad Sci USA. (2016) 113:E5472–80. doi: 10.1042/CS20080444
54. Tomas C, Newton J. Metabolic abnormalities in chronic fatigue syndrome/myalgic encephalomyelitis: a mini-review. Biochem Soc Trans. (2018) 46:547–53. doi: 10.1042/BST20170503
55. Narayan N, Owen DR, Mandhair H, Smyth E, Carlucci F, Saleem A, et al. Translocator protein as an imaging marker of macrophage and stromal activation in rheumatoid arthritis pannus. J Nucl Med. (2018) 59:1125–32. doi: 10.2967/jnumed.117.202200
56. Hernstadt H, Wang S, Lim G, Mao J. Spinal translocator protein (TSPO) modulates pain behavior in rats with CFA-induced monoarthritis. Brain Res. (2009) 1286:42–52. doi: 10.1016/j.brainres.2009.06.043
57. Bird J, Izquierdo-Garcia D, Davies J, Rudd J, Probst K, Figg N, et al. Evaluation of translocator protein quantification as a tool for characterising macrophage burden in human carotid atherosclerosis. Atherosclerosis (2010) 210:388–91. doi: 10.1016/j.atherosclerosis.2009.11.047
58. Narayan N, Carlucci F, Taylor PC. An investigation of translocator protein as a tissue and peripheral blood biomarker of inflammation in rheumatoid arthritis. Arthritis Rheum. (2016). 68 (Suppl. 10).
59. Owen DR, Howell OW, Tang SP, Wells LA, Bennacef I, Bergstrom M, et al. Two binding sites for [3H]PBR28 in human brain: implications for TSPO PET imaging of neuroinflammation. J Cereb Blood Flow Metab. (2010) 30:1608–18. doi: 10.1038/jcbfm.2010.63
60. Mizrahi R, Rusjan PM, Kennedy J, Pollock B, Mulsant B, Suridjan I, et al. Translocator protein (18 kDa) polymorphism (rs6971) explains in-vivo brain binding affinity of the PET radioligand [(18)F]-FEPPA. J Cereb Blood Flow Metab. (2012) 32:968–72. doi: 10.1038/jcbfm.2012.46
61. Zanotti-Fregonara P, Zhang Y, Jenko K, Gladding R, Zoghbi S, Fujita M, et al. Synthesis and evaluation of translocator 18 kDa protein (TSPO) positron emission tomography (PET) radioligands with low binding sensitivity to human single nucleotide polymorphism rs6971. ACS Chem Neurosci. (2014) 5:963–71. doi: 10.1021/cn500138n
62. Loggia ML, Chonde DB, Akeju O, Arabasz G, Catana C, Edwards RR, et al. Evidence for brain glial activation in chronic pain patients. Brain (2015) 138:604–15. doi: 10.1093/brain/awu377
63. Turkheimer F, Rizzo G, Bloomfield P, Howes O, Zanotti-Fregonara P, Bertoldo A, et al. The methodology of TSPO imaging with positron emission tomography. Biochem Soc Trans. (2015) 43:586–92. doi: 10.1042/BST20150058
64. Jacobs AH, Tavitian B, INMiND consortium. Noninvasive molecular imaging of neuroinflammation J Cereb Blood Flow Metab. (2012) 32:1393–415. doi: 10.1038/jcbfm.2012.53
65. Politis M, Su P, Piccini P. Imaging of microglia in patients with neurodegenerative disorders. Front Pharmacol. (2012) 3:96. doi: 10.3389/fphar.2012.00096
66. Notter T, Coughlin J, Sawa A, Meyer U. Reconceptualization of Translocator protein as a biomarker of neuroinflammation in psychiatry. Mol Psychiatry (2018) 23:36–47. doi: 10.1038/mp.2017.232
67. Real C, Doorduin J, Feltes PK, Garcia DV, de Paula Faria D, Britto L, et al. Evaluation of exercise-induced modulation of glial activation and dopaminergic damage in a rat model of parkinson's disease using [11C]PBR28 and [18F]FDOPA PET. J Cereb Blood Flow Metab. (2017). doi: 10.1177/0271678X17750351. [Epub ahead of print].
68. Catana C, Procissi D, Wu Y, Judenhofer MS, Qi J, Pichler BJ, et al. Simultaneous in vivo positron emission tomography and magnetic resonance imaging. Proc Natl Acad Sci USA. (2008) 105:3705–10. doi: 10.1073/pnas.0711622105
69. Catana C, Guimaraes AR, Rosen BR. PET and MR imaging: the odd couple or a match made in heaven? J Nucl Med. (2013) 54:815–24. doi: 10.2967/jnumed.112.112771
70. Natelson BH, Vu D, Coplan JD, Mao X, Blate M, Kang G, et al. Elevations of ventricular lactate levels occur in both chronic fatigue syndrome and fibromyalgia. Fatigue Biomed Health Behav. (2017) 5:15–20. doi: 10.1080/21641846.2017.1280114
71. Fukuda K, Straus SE, Hickie I, Sharpe MC, Dobbins JG, Komaroff A. The chronic fatigue syndrome: a comprehensive approach to its definition and study. Ann Internal Med. (1994) 121:953–9. doi: 10.7326/0003-4819-121-12-199412150-00009
72. Van der Schaaf ME, De Lange FP, Schmits IC, Geurts DEM, Roelofs K, van der Meer JWM, et al. Prefrontal structure varies as a function of pain symptoms in chronic fatigue syndrome. Biol Psychiatry (2017) 81:358–65. doi: 10.1016/j.biopsych.2016.07.016
73. Shungu DC, Weiduschat N, Murrough JW, Mao X, Pillemer S, Dyke JP, et al. Increased ventricular lactate in chronic fatigue syndrome. III relationships to cortical glutathione and clinical symptoms implicate oxidative stress in disorder pathophysiology NMR Biomed. (2012) 25:1073–87. doi: 10.1002/nbm.2772
74. Murrough JW, Mao X, Collins KA, Kelly C, Andrade G, Nestadt P, et al. Increased ventricular lactate in chronic fatigue syndrome measured by 1H MRS imaging at 3.0 T. II: comparison with major depressive disorder. NMR Biomed. (2010) 23:643–50. doi: 10.1002/nbm.1512
75. Puri BK, Agour M, Gunatilake KDR, Fernando KAC, Gurusinghe AI, Treasaden IH. An in vivo proton neurospectroscopy study of cerebral oxidative stress in myalgic encephalomyelitis (Chronic Fatigue Syndrome). Prostaglandins Leukot Essent Fatty Acids. (2009) 81:303–5. doi: 10.1016/j.plefa.2009.10.002
76. Mathew SJ, Mao X, Keegan KA, Levine SM, Smith ELP, Heier LA, et al. Ventricular cerebrospinal fluid lactate is increased in chronic fatigue syndrome compared with generalized anxiety disorder: an in vivo 3.0 T (1)H MRS imaging study. NMR Biomed. (2008) 22:251–8. doi: 10.1002/nbm.1315
77. Chaudhuri A, Condon BR, Gow JW, Brennan D, Hadley DM. Proton magnetic resonance spectroscopy of basal ganglia in chronic fatigue syndrome. Neuroreport (2003) 14:225–8. doi: 10.1097/01.wnr.0000054960.21656.64
78. Puri BK, Counsell SJ, Zaman R, Main J, Collins AG, Hajnal JV, et al. Relative increase in choline in the occipital cortex in chronic fatigue syndrome. Acta Psychiatrica Scandinavica (2002) 106:224–6. doi: 10.1034/j.1600-0447.2002.01300.x
79. Brooks JC, Roberts N, Whitehouse G, Majeed T. Proton magnetic resonance spectroscopy and morphometry of the hippocampus in chronic fatigue syndrome. Br J Radiol. (2000) 73:1206–8. doi: 10.1259/bjr.73.875.11144799
80. Holmes GP, Kaplan JE, Gantz NM, Komaroff AL, Schonberger LB, Straus SE, et al. Chronic fatigue syndrome: a working case definition. Ann Internal Med. (1988) 108:387–9. doi: 10.7326/0003-4819-108-3-387
81. Tomoda A, Miike T, Yamada E, Honda H, Moroi T, Ogawa M. Chronic fatigue syndrome in childhood. Brain Dev. (2000) 22:60–4. doi: 10.1016/S0387-7604(99)00111-4
82. Soares DP, Law M. Magnetic resonance spectroscopy of the brain: review of metabolites and clinical applications. Clin Radiol. (2009) 64:12–21. doi: 10.1016/j.crad.2008.07.002
83. Allen AD. Is RA27 3 rubella immunization a cause of chronic fatigue? Med Hypoth. (1988) 27:217–20.
84. Stagg CJ, Bachtiar V, Johansen-Berg H. What are we measuring with GABA magnetic resonance spectroscopy? Commun Integr Biol. (2011) 4:573–5. doi: 10.4161/cib.16213
85. Ramadan S, Lin A, Stanwell P. Glutamate and glutamine: a review of in vivo MRS in the human brain. NMR Biomed. (2013) 26:1630–46. doi: 10.1002/nbm.3045
86. Lapidus KAB, Gabbay V, Mao X, Johnson A, Murrough JW, Mathew SJ, et al. In vivo 1H MRS study of potential associations between glutathione, oxidative stress and anhedonia in major depressive disorder. Neurosci Lett. (2014) 569:74–9. doi: 10.1016/j.neulet.2014.03.056
87. Pérez-Cerdá F, Sánchez-Gómez MV, Matute C. The link of inflammation and neurodegeneration in progressive multiple sclerosis. Multiple Scler Demyel Disord. (2016) 1:1. doi: 10.1186/s40893-016-0012-0
88. Urrila AS, Hakkarainen A, Heikkinen S, Vuori K, Stenberg D, Häkkinen, et al. Metabolic imaging of human cognition: an FMRI/1H-MRS study of brain lactate response to silent word generation. J Cereb Blood Flow Metab. (2003) 23:942–8. doi: 10.1097/01.wcb.0000080652.64357.1d
89. Kozić D, Bjelan M, Boban J, Ostojić J, Turkulov V, Todorović A, et al. A prominent lactate peak as a potential key magnetic resonance spectroscopy (MRS) feature of progressive multifocal leukoencephalopathy (PML): spectrum pattern observed in three patients. Bosnian J Bas Med Sci. (2017) 17:349–54. doi: 10.17305/bjbms.2017.2092
90. Chow SL. The significance of elevated CSF lactate. Arch Dis Childh. (2005) 90:1188–9. doi: 10.1136/adc.2005.075317
91. Nagae-Poetscher LM, Mcmahon M, Braverman N, Lawrie WT, Fatemi A, Degaonkar M, et al. Metabolites in ventricular cerebrospinal fluid detected by proton magnetic resonance spectroscopic imaging. J Magn Reson Imag. (2004) 20:496–500. doi: 10.1002/jmri.20128
92. Kaddah RO, Khalil ME. MR spectroscopy evaluation of white matter signal abnormalities of different non-neoplastic brain lesions. Egypt J Radiol Nucl Med. (2016) 47:233–42. doi: 10.1016/j.ejrnm.2015.10.010
93. Häussinger D, Laubenberger J, vom Dahl S, Ernst T, Bayer S, Langer M, et al. Proton magnetic resonance spectroscopy studies on human brain myo-inositol in hypo-osmolarity and hepatic encephalopathy. Gastroenterology (1994) 107:1475–80.
94. Moffett JR, Ross B, Arun P, Madhavarao CN, Namboodiri MAA. N-acetylaspartate in the CNS: from neurodiagnostics to neurobiology. Prog Neurobiol. (2007) 81:89–131. doi: 10.1016/j.pneurobio.2006.12.003
95. Carvalho Pereira A, Violante IR, Mouga S, Oliveira G, Castelo-Branco M. Medial frontal lobe neurochemistry in autism spectrum disorder is marked by reduced N-acetylaspartate and unchanged gamma-aminobutyric acid and glutamate + glutamine levels. J Auti Dev Disord. (2018) 48:1467–82. doi: 10.1007/s10803-017-3406-8
96. Hardan AY, Minshew NJ, Melhem NM, Srihari S, Jo B, Bansal R, et al. An MRI, and proton spectroscopy study of the thalamus in children with autism. Psychiatry Res. (2008) 163:97–105. doi: 10.1016/j.pscychresns.2007.12.002
97. Haroon E, Woolwine BJ, Chen X, Pace TW, Parekh S, Spivey JR, et al. IFN-α-induced cortical and subcortical glutamate changes assessed by magnetic resonance spectroscopy. Neuropsychopharmacology (2014) 39:1777–85. doi: 10.1038/npp.2014.25
98. Dantzer R, Kelley KW. Twenty years of research on cytokine-induced sickness behavior. Brain BehavImmunity (2007) 21:153–60. doi: 10.1016/j.bbi.2006.09.006
99. Capuron L, Pagnoni G, Demetrashvili M, Woolwine BJ, Nemeroff CB, Berns GS, et al. Anterior cingulate activation and error processing during interferon-alpha treatment. Biol Psychiatry (2005) 58:190–6. doi: 10.1016/j.biopsych.2005.03.033
100. Capuron L, Pagnoni G, Drake DF, Woolwine BJ, Spivey JR, Crowe RJ, et al. Dopaminergic mechanisms of reduced basal ganglia responses to hedonic reward during interferon alfa administration. Arch Gen Psychiatry (2012) 69:1044–53. doi: 10.1001/archgenpsychiatry.2011.2094
101. Brydon L, Neil A. NA, Walker C, Steptoe S, Critchley HD. Peripheral inflammation is associated with altered substantia nigra activity and psychomotor slowing in humans. Biol Psychiatry (2008) 63:1022–9. doi: 10.1016/j.biopsych.2007.12.007
102. Harrison NA, Brydon L, Walker C, Gray MA, Steptoe A, Dolan RJ, et al. Neural origins of human sickness in interoceptive responses to inflammation. Biol Psychiatry (2009) 66:415–22. doi: 10.1016/j.biopsych.2009.03.007
103. Capuron L, Miller AH. Cytokines and psychopathology: lessons from interferon-alpha. Biol Psychiatry (2004) 56:819–24. doi: 10.1016/j.biopsych.2004.02.009
104. Blundell S, Ray KK, Buckland M, White PD. Chronic fatigue syndrome and circulating cytokines: a systematic review. Brain Behav Immunity (2015) 50:186–95. doi: 10.1016/j.bbi.2015.07.004
105. Watkins LR, Maier SF, Goehler LE. Cytokine-to-brain communication: a review & analysis of alternative mechanisms. Life Sciences (1995) 57:1011–26.
106. Goehler LE, Gaykema RP, Hansen MK, Anderson K, Maier SF, Watkins LR. Vagal immune-to-brain communication: a visceral chemosensory pathway. Auton Neurosci. (2000) 85:49–59. doi: 10.1016/S1566-0702(00)00219-8
107. Campisi J, Hansen MK, O'Connor JA, Biedenkapp JC, Watkins LR, Maier SF, et al. Circulating cytokines and endotoxin are not necessarily for the activation of the sickness or corticosterone response produced by peripheral, E. coli challenge. J Appl Physiol. (2003) 95:1873–82. doi: 10.1152/japplphysiol.00371.2003
108. Turrin NP, Plata-Salamán CR. Cytokine-cytokine interactions and the brain. Brain Res Bull. (2000) 51:3–9. doi: 10.1016/S0361-9230(99)00203-8
109. Lynn M, Maclachlan L, Finkelmeyer A, Clark J, Locke L, Todryk, et al. Reduction of glucocorticoid receptor function in chronic fatigue syndrome. Med Inflamm. (2018) 2018:3972104. doi: 10.1155/2018/3972104
110. Richardson AM, Lewis DP, Kita B, Ludlow H, Groome NP, Hedger MP, et al. Weighting of orthostatic intolerance time measurements with standing difficulty score stratifies ME/CFS symptom severity and analyte detection. J Transl Med. (2018) 16:97. doi: 10.1186/s12967-018-1473-z
111. Oka T, Tanahashi T, Sudo N, Lkhagvasuren B, Yamada YU. Changes in fatigue, autonomic functions, and blood biomarkers due to sitting isometric yoga in patients with chronic fatigue syndrome. Biopsychos Med. (2018) 12:3. doi: 10.1186/s13030-018-0123-2
112. SEID. Committee on the Diagnostic Criteria for Myalgic Encephalomyelitis/Chronic Fatigue Syndrome, Board on the Health of Select Populations, and Institute of Medicine. Beyond Myalgic Encephalomyelitis/Chronic Fatigue Syndrome: Redefining an Illness. The National Academies Collection: Reports Funded by National Institutes of Health. Washington (DC): National Academies Press (US) (2015).
113. Moneghetti KJ, Skhiri M, Contrepois K, Kobayashi Y, Maecker H, Davis M, et al. Value of circulating cytokine profiling during submaximal exercise testing in myalgic encephalomyelitis/chronic fatigue syndrome. Sci Rep. (2018) 8:2779. doi: 10.1038/s41598-018-20941-w
114. Wyller VB, Nguyen CB, Ludviksen JA, Mollnes TE. Transforming Growth Factor Beta (TGF-β) in adolescent chronic fatigue syndrome. J Transl Med. (2017) 15:245. doi: 10.1186/s12967-017-1350-1
115. Roerink ME, van der Schaaf ME, Dinarello CA, Knoop H, van der Meer JWM. Interleukin-1 as a mediator of fatigue in disease: a narrative review. J Neuroinflamm. (2017) 14:6. doi: 10.1186/s12974-017-0796-7
116. Milrad SF, Hall DL, Jutagir DR, Lattie EG, Czaja SJ, Perdomo DM, et al. Depression, evening salivary cortisol and inflammation in chronic fatigue syndrome: a psychoneuroendocrinological structural regression model. Int J Psychophysiol. (2018) 131:124–30. doi: 10.1016/j.ijpsycho.2017.09.009
117. Montoya JG, Holmes TH, Anderson JN, Maecker HT, Rosenberg-Hasson Y, Valencia IA, et al. Cytokine signature associated with disease severity in chronic fatigue syndrome patients. Proc Natl Acad Sci USA. (2017) 114:E7150–8. doi: 10.1073/pnas.1710519114
118. Nagy-Szakal D, Williams BL, Mishra N, Che X, Lee B, Bateman L, et al. Fecal metagenomic profiles in subgroups of patients with myalgic encephalomyelitis/chronic fatigue syndrome. Microbiome (2017) 5:44. doi: 10.1186/s40168-017-0261-y
119. Hornig M, Gottschalk CG, Eddy ML, Che X, Ukaigwe JE, Peterson DL, et al. Immune network analysis of cerebrospinal fluid in myalgic encephalomyelitis/chronic fatigue syndrome with atypical and classical presentations. Transl Psychiatry (2017) 7:e1080. doi: 10.1038/tp.2017.44
120. Hanevik K, Kristoffersen E, Mørch K, Rye KP, Sørnes S, Svärd S, et al. Giardia-specific cellular immune responses in post-giardiasis chronic fatigue syndrome. BMC Immunol. (2017) 18:5. doi: 10.1186/s12865-017-0190-3
121. Lidbury BA, Kita B, Lewis DP, Hayward S, Ludlow H, Hedger MP, et al. Activin B is a novel biomarker for chronic fatigue syndrome/myalgic encephalomyelitis (CFS/ME) diagnosis: a cross sectional study. J Transl Med. (2017) 15:60. doi: 10.1186/s12967-017-1161-4
122. Milrad SF, Hall DL, Jutagir DR, Lattie EG, Ironson GH, Wohlgemuth W, et al. Poor sleep quality is associated with greater circulating pro-inflammatory cytokines and severity and frequency of chronic fatigue syndrome/myalgic encephalomyelitis (CFS/ME) symptoms in women. J Neuroimmunol. (2017) 303:43–50. doi: 10.1016/j.jneuroim.2016.12.008
123. Lunde S, Kristoffersen EK, Sapkota D, Risa K, Dahl O, Bruland O, et al. Serum BAFF and APRIL Levels, T-lymphocyte subsets, and immunoglobulins after B-cell depletion using the monoclonal anti-CD20 antibody rituximab in myalgic encephalopathy/chronic fatigue syndrome. PLoS ONE (2016) 11:e0161226. doi: 10.1371/journal.pone.0161226
124. Huth TK, Staines D, Marshall-Gradisnik S. ERK1/2, MEK1/2 and P38 downstream signalling molecules impaired in CD56 Dim CD16+ and CD56 Bright CD16 dim/- natural killer cells in chronic fatigue syndrome/myalgic encephalomyelitis patients. J Transl Med. (2016) 14:97. doi: 10.1186/s12967-016-0859-z
125. Russell L, Broderick G, Taylor R, Fernandes H, Harvey J, Barnes Z, et al. Illness progression in chronic fatigue syndrome: a shifting immune baseline. BMC Immunol. (2016) 17:3. doi: 10.1186/s12865-016-0142-3
126. Reeves WC, Wagner D, Nisenbaum R, Jones JF, Gurbaxani B, Solomon L, et al. Chronic fatigue syndrome - a clinically empirical approach to its definition and study. BMC Med. (2005) 3:19. doi: 10.1186/1741-7015-3-19
127. Landi A, Broadhurst D, Vernon SD, Tyrrell DLJ, Houghton M. Reductions in circulating levels of IL-16, IL-7 and VEGF-A in myalgic encephalomyelitis/chronic fatigue syndrome. Cytokine (2016) 78:27–36. doi: 10.1016/j.cyto.2015.11.018
128. Hardcastle SL, Brenu EW, Johnston S, Nguyen T, Huth T, Ramos S, et al. Serum immune proteins in moderate and severe chronic fatigue syndrome/myalgic encephalomyelitis patients. Int J Med Sci. (2015) 12:764–72. doi: 10.7150/ijms.12399
129. Peterson D, Brenu EW, Gottschalk G, Ramos S, Nguyen T, Staines D, et al. Cytokines in the cerebrospinal fluids of patients with chronic fatigue syndrome/myalgic encephalomyelitis. Mediat Inflamm. (2015) 2015:929720. doi: 10.1155/2015/929720
130. Khaiboullina SF, DeMeirleir KL, Rawat S, Berk GS, Gaynor-Berk RS, Mijatovic T, et al. Cytokine expression provides clues to the pathophysiology of gulf war illness and myalgic encephalomyelitis. Cytokine (2015) 72:1–8. doi: 10.1016/j.cyto.2014.11.019
131. Carruthers BM, Jain AK, De Meirleir KL, Peterson DL, Klimas NG, Lerner AM, et al. Myalgic encephalomyelitis/chronic fatigue syndrome. J Chron Fatig Syndr. (2003) 11:7–115. doi: 10.1300/J092v11n01_02
132. Carruthers BM, van de Sande MI, De Meirleir KL, Klimas NG, Broderick G, Mitchell T, et al. Myalgic encephalomyelitis: international consensus criteria. J Internal Med. (2011) 270:327–38. doi: 10.1111/j.1365-2796.2011.02428.x
133. Wyller VB, Sørensen Ø, Sulheim D, Fagermoen E, Ueland T, Mollnes TE. Plasma cytokine expression in adolescent chronic fatigue syndrome. Brain Behav Immun. (2015) 46:80–6. doi: 10.1016/j.bbi.2014.12.025
134. Royal College Pediatrics & Child Health. Evidence Based Guideline for the Management of CFS/ME in Children and Young People (2004).
135. National Institutes of Health and Clinical Excellence. Chronic Fatigue Syndrome/Myalgic Encephalomyelitis (or Encephalopathy): Diagnosis and Management. Clinical guideline [CG53] (2007). Available online at: https://www.nice.org.uk/guidance/cg53
136. Hornig M, Montoya JG, Klimas NG, Levine S, Felsenstein D, Bateman L, et al. Distinct plasma immune signatures in ME/CFS are present early in the course of illness. Sci. Adv. (2015) 1:1400121. doi: 10.1126/sciadv.1400121
137. Jason LA, Evans M, Porter N, Brown M, Brown A, Hunnell J, et al. The development of a revised Canadian myalgic encephalomyelitis chronic fatigue syndrome case definition. Am J Biochem Biotechnol. (2010) 6:120–35. doi: 10.3844/AJBBSP.2010.120.135
138. Neu D, Mairesse O, Montana X, Gilson M, Corazza F, Lefevre N, et al. Dimensions of pure chronic fatigue: psychophysical, cognitive and biological correlates in the chronic fatigue syndrome. Eur J Appl Physiol. (2014) 114:1841–51. doi: 10.1007/s00421-014-2910-1
139. Garcia MN, Hause AM, Walker CM, Orange JS, Hasbun R, Murray KO. Evaluation of prolonged fatigue post-West Nile virus infection and association of fatigue with elevated antiviral and proinflammatory cytokines. Viral Immunol. (2014) 27:327–33. doi: 10.1089/vim.2014.0035
140. Nakamura T, Schwander S, Donnelly R, Cook DB, Ortega F, Togo F, et al. Exercise and sleep deprivation do not change cytokine expression levels in patients with chronic fatigue syndrome. Clin Vacc Immunol. (2013) 20:1736–42. doi: 10.1128/CVI.00527-13
141. Maes M, Ringel K, Kubera M, Anderson G, Morris G, Galecki P, et al. In myalgic encephalomyelitis/chronic fatigue syndrome, increased autoimmune activity against 5-HT is associated with immuno-inflammatory pathways and bacterial translocation. J Affect Disord. (2013) 150:223–30. doi: 10.1016/j.jad.2013.03.029
142. Stringer EA, Baker KS, Carroll IR, Montoya JG, Chu L, Maecker HT, et al. Daily cytokine fluctuations, driven by leptin, are associated with fatigue severity in chronic fatigue syndrome: evidence of inflammatory pathology. J Transl Med. (2013)11:93. doi: 10.1186/1479-5876-11-93
143. Lattie EG, Antoni MH, Fletcher MA, Penedo F, Czaja S, Lopez C, et al. Stress management skills, neuroimmune processes and fatigue levels in persons with chronic fatigue syndrome. Brain Behav Immun. (2012) 26:849–58. doi: 10.1016/j.bbi.2012.02.008
144. Smylie AL, Broderick G, Fernandes H, Razdan S, Barnes Z, Collado F, et al. A comparison of sex-specific immune signatures in Gulf War illness and chronic fatigue syndrome. BMC Immunol. (2013) 14:29. doi: 10.1186/1471-2172-14-29
145. Broderick G, Katz BZ, Fernandes H, Fletcher MA, Klimas N, Smith FA, et al. Cytokine expression profiles of immune imbalance in post-mononucleosis chronic fatigue. J Transl Med. (2012) 10:191. doi: 10.1186/1479-5876-10-191
146. Jason LA, Jordan K, Miike T, Bell DS, Lapp C, Torres-Harding S, et al. A pediatric case definition for myalgic encephalomyelitis and chronic fatigue syndrome. J Chron Fatig Syndr. (2006) 13:1–44. doi: 10.1300/J092v13n02_01
147. Maes M, Twisk FNM, Kubera M, Ringel K. Evidence for inflammation and activation of cell-mediated immunity in myalgic encephalomyelitis/chronic fatigue syndrome (ME/CFS): increased interleukin-1, tumor necrosis factor-α, PMN-elastase, lysozyme and neopterin. J Affect Disord. (2012) 136:933–9. doi: 10.1016/j.jad.2011.09.004
148. Nas K, Cevik R, Batum S, Sarac AJ, Acar S, Kalkanli S. Immunologic and psychosocial status in chronic fatigue syndrome. Bratisl Med J. (2011) 112:208–12.
149. White AT, Light AR, Hughen RW, Bateman L, Martins TB, Hill HR, et al. Severity of symptom flare after moderate exercise is linked to cytokine activity in chronic fatigue syndrome. Psychophysiology (2010) 47:615–24. doi: 10.1111/j.1469-8986.2010.00978.x
150. Nakamura T, Schwander S, Donnelly R, Cook DB, Ortega F, Togo F, et al. Cytokines across the night in chronic fatigue syndrome with and without fibromyalgia. Clin Vacc Immunol. (2010) 17:582–7. doi: 10.1128/CVI.00379-09
151. Nijs J, Van Oosterwijck J, Meeus M, Lambrecht L, Metzger K, Frémont M, et al. Unravelling the nature of postexertional malaise in myalgic encephalomyelitis/chronic fatigue syndrome: the role of elastase, complement C4a and interleukin-1beta. J Intern Med. (2010) 267:418–35. doi: 10.1111/j.1365-2796.2009.02178.x
152. Robinson M, Gray SR, Watson MS, Kennedy G, Hill A, Belch JJF, et al. Plasma IL-6, its soluble receptors and F2-isoprostanes at rest and during exercise in chronic fatigue syndrome. Scand J Med Sci Sports (2010) 20:282–90. doi: 10.1111/j.1600-0838.2009.00895.x
153. Scully P, McKernan DP, Keohane J, Groeger D, Shanahan F, Dinan TG, et al. Plasma cytokine profiles in females with irritable bowel syndrome and extra-intestinal co-morbidity. Am J Gastroenterol. (2010) 105:2235–43. doi: 10.1038/ajg.2010.159
154. Fletcher MA, Rong Zeng X, Barnes Z, Levis S, Klimas NG. Plasma cytokines in women with chronic fatigue syndrome. J Transl Med. (2009) 7:96. doi: 10.1186/1479-5876-7-96
155. Jammes Y, Steinberg JG, Delliaux S, Brégeon F. Chronic fatigue syndrome combines increased exercise-induced oxidative stress and reduced cytokine and Hsp responses. J Intern Med. (2009) 266:196–206. doi: 10.1111/j.1365-2796.2009.02079.x
156. Nater UM, Youngblood LS, Jones JF, Unger ER, Miller AH, Reeves WC, et al. Alterations in diurnal salivary cortisol rhythm in a population-based sample of cases with chronic fatigue syndrome. Psychosom Med. (2008) 70:298–305. doi: 10.1097/PSY.0b013e3181651025
157. Spence VA, Kennedy G, Belch JJF, Hill A, Khan F. Low-grade inflammation and arterial wave reflection in patients with chronic fatigue syndrome. Clin Sci. (2008) 114:561–6. doi: 10.1042/CS20070274
158. Vollmer-Conna U, Cameron B, Hadzi-Pavlovic D, Singletary K, Davenport T, Vernon S, et al. Postinfective fatigue syndrome is not associated with altered cytokine production. Clin Infect Dis. (2007) 45:732–5. doi: 10.1086/520990
159. Kennedy G, Spence V, Underwood C, Belch JJF. Increased neutrophil apoptosis in chronic fatigue syndrome. J Clin Pathol. (2004) 57:891–3. doi: 10.1136/jcp.2003.015511
160. White PD, Nye KE, Pinching AJ, Yap TM, Power N, Vleck V, et al. Immunological changes after both exercise and activity in chronic fatigue syndrome. J Chronic Fatigue Syndr. (2004) 12:51–66. doi: 10.1300/J092v12n02_06
161. Visser J, Graffelman W, Blauw B, Haspels I, Lentjes E, de Kloet ER, et al. LPS-induced IL-10 production in whole blood cultures from chronic fatigue syndrome patients is increased but supersensitive to inhibition by dexamethasone. J Neuroimmunol. (2001) 119:343–9. doi: 10.1016/S0165-5728(01)00400-3
162. Cheney PR. Interleukin-2 and the chronic fatigue syndrome. Ann Intern Med. (1989) 110:321. doi: 10.7326/0003-4819-110-4-321_1
163. Cannon JG, Angel JB, Ball RW, Abad LW, Fagioli L, Komaroff AL. Acute phase responses and cytokine secretion in chronic fatigue syndrome. J Clin Immunol. (1999) 19:414–21. doi: 10.1023/A:1020558917955
164. Buchwald D, Wener MH, Pearlman T, Kith P. Markers of inflammation and immune activation in chronic fatigue and chronic fatigue syndrome. J Rheumatol. (1997) 24:372–6.
165. Bennett AL, Chao CC, Hu S, Buchwald D, Fagioli LR, Schur PH, et al. Elevation of bioactive transforming growth factor-beta in serum from patients with chronic fatigue syndrome. J. Clin. Immunol. (1997) 17:160–6.
166. MacDonald KL, Osterholm MT, LeDell KH, White KE, Schenck CH, Chao CC, et al. A case-control study to assess possible triggers and cofactors in chronic fatigue syndrome. Am J Med. (1996) 100:548–54.
167. Chao CC, Janoff EN, Hu SX, Thomas K, Gallagher M, Tsang M, et al. Altered cytokine release in peripheral blood mononuclear cell cultures from patients with the chronic fatigue syndrome. Cytokine (1991) 3:292–8.
168. Swanink CM, Vercoulen JH, Galama JM, Roos MT, Meyaard L, van der Ven-Jongekrijg J, et al. Lymphocyte subsets, apoptosis, and cytokines in patients with chronic fatigue syndrome. J. Infect. Dis. (1996) 173:460–3.
169. Sharpe MC, Archard LC, Banatvala JE, Borysiewicz LK, Clare AW, David A, et al. A report–chronic fatigue syndrome: guidelines for research. J R Soc Med. (1991) 84:118–21.
170. Drenth JP, Van Uum SH, Van Deuren M, Pesman GJ, Van der Ven-Jongekrijg J, Van der Meer JW. Endurance run increases circulating IL-6 and IL-1ra but downregulates ex vivo TNF-Alpha and IL-1 beta production. J Appl Physiol. (1995) 79:1497–503. doi: 10.1152/jappl.1995.79.5.1497
171. Peterson PK, Sirr SA, Grammith FC, Schenck CH, Pheley AM, Hu S, et al. Effects of mild exercise on cytokines and cerebral blood flow in chronic fatigue syndrome patients. Clin Diagn Lab Immunol. (1994) 1:222–6.
172. Schluederberg A, Straus SE, Peterson P, Blumentha S, Komaroff AL, Spring SB, et al. Chronic fatigue syndrome research: definition and medical outcome assessment. Ann Intern Med. (1992) 117:325–31. doi: 10.7326/0003-4819-117-4-325
173. Patarca R, Klimas NG, Lugtendorf S, Antoni M, Fletcher MA. Dysregulated expression of tumor necrosis factor in chronic fatigue syndrome: interrelations with cellular sources and patterns of soluble immune mediator expression. Clin Infect Dis. (1994) 18 (Suppl. 1): S147–53. doi: 10.1093/clinids/18.Supplement_1.S147
174. Lloyd A, Gandevia S, Brockman A, Hales J, Wakefield D. Cytokine production and fatigue in patients with chronic fatigue syndrome and healthy control subjects in response to exercise. Clin. Infect. Dis. (1994) 18 (Suppl. 1):S142–46. doi: 10.1093/clinids/18.Supplement
175. Linde A, Andersson B, Svenson SB, Ahrne H, Carlsson M, Forsberg P, et al. Serum levels of lymphokines and soluble cellular receptors in primary epstein-barr virus infection and in patients with chronic fatigue syndrome. J Infect Dis. (1992) 165:994–1000. doi: 10.1093/infdis/165.6.994
176. Straus SE, Dale JK, Peter JB, Dinarello CA. Circulating lymphokine levels in the chronic fatigue syndrome. J Infect Dis. (1989) 160:1085–6. doi: 10.1093/infdis/160.6.1085
177. Lloyd A, Hickie I, Brockman A, Dwyer J, Wakefield D. Cytokine levels in serum and cerebrospinal fluid in patients with chronic fatigue syndrome and control subjects. J. Infect. Dis. (1991) 164:1023–4.
178. Natelson BH, Weaver SA, Tseng C, Ottenweller JE. Spinal fluid abnormalities in patients with chronic fatigue syndrome. Clin Diagn Lab Immunol. (2005) 12:52–5. doi: 10.1128/CDLI.12.1.52-55.2005
179. Milligan ED, O'Connor KA, Nguyen KT, Armstrong CB, Twining C, Gaykema RP, et al. Intrathecal HIV-1 envelope glycoprotein Gp120 induces enhanced pain states mediated by spinal cord proinflammatory cytokines. J Neurosci. (2001) 21:2808–19. doi: 10.1523/JNEUROSCI.21-08-02808.2001
180. Conn CA, McClellan JL, Maassab HF, Smitka CW, Majde JA, Kluger MJ. Cytokines and the acute phase response to influenza virus in mice. Am J Physiol. (1995) 268:R78–84. doi: 10.1152/ajpregu.1995.268.1.R78
181. Chatzipanteli K, Vitarbo E, Alonso OF, Bramlett HM, Dietrich WD. Temporal profile of cerebrospinal fluid, plasma, and brain interleukin-6 after normothermic fluid-percussion brain injury: effect of secondary hypoxia. Ther Hypothermia Temperature Manag. (2012) 2:167–75. doi: 10.1089/ther.2012.0016
182. Backonja MM, Coe CL, Muller DA, Schell K. Altered cytokine levels in the blood and cerebrospinal fluid of chronic pain patients. J Neuroimmunol. (2008) 195:157–63. doi: 10.1016/j.jneuroim.2008.01.005
183. Zimmerman AW, Jyonouchi H, Comi AM, Connors SL, Milstien S, Varsou A, et al. Cerebrospinal fluid and serum markers of inflammation in autism. Pediatr Neurol. (2005) 33:195–201. doi: 10.1016/j.pediatrneurol.2005.03.014
184. Hestad KA, Engedal K, Whist JE, Aukrust P, Farup PG, Mollnes TE, et al. Patients with depression display cytokine levels in serum and cerebrospinal fluid similar to patients with diffuse neurological symptoms without a defined diagnosis. Neuropsych Dis Treat. (2016) 12:817–22. doi: 10.2147/NDT.S101925
185. Bromander S, Anckarsäter R, Kristiansson M, Blennow K, Zetterberg H, Anckarsäter H, et al. Changes in serum and cerebrospinal fluid cytokines in response to non-neurological surgery: an observational study. J Neuroinflamm. (2012) 9:242. doi: 10.1186/1742-2094-9-242
186. Šumanović-Glamuzina D, Culo F, Culo MI, Konjevoda P, Marjana Jerković-RaguŽ. A comparison of blood and cerebrospinal fluid cytokines (IL-1β, IL-6, IL-18, TNF-α) in neonates with perinatal hypoxia. Bosn J Basic Med Sci. (2017) 17:203–10. doi: 10.17305/bjbms.2017.1381
187. Zhou X, Fragala MS, McElhaney JE, Kuchel GA. Conceptual and methodological issues relevant to cytokine and inflammatory marker measurements in clinical research. Curr Opin Clin Nutr Metab Care (2010) 13:541–7. doi: 10.1097/MCO.0b013e32833cf3bc
188. Seiler W, Müller H, Hiemke C. Interleukin-6 in plasma collected with an indwelling cannula reflects local, not systemic, concentrations. Clin Chem. (1994) 40:1778–9.
189. Leng SX, McElhaney JE, Walston JE, Xie D, Fedarko NS, Kuchel GA. ELISA and multiplex technologies for cytokine measurement in inflammation and aging research. J Gerontol. (2008) 63:879–84. doi: 10.1093/gerona/63.8.879
190. Sapan CV, Lundblad RL. Considerations regarding the use of blood samples in the proteomic identification of biomarkers for cancer diagnosis. Cancer Genomics (2006) 3:227–30.
191. Banks RE. Measurement of cytokines in clinical samples using immunoassays: problems and pitfalls. Crit Rev Clin Lab Sci. (2000) 37:131–82. doi: 10.1080/10408360091174187
192. Tighe PJ, Ryder RR, Todd I, Fairclough LC. ELISA in the multiplex era: potentials and pitfalls. Prot Clin Appl. (2015) 9:406–22. doi: 10.1002/prca.201400130
193. Boja ES, Jortani SA, Ritchie J, Hoofnagle AN, Težak Z, Mansfield E, et al. The journey to regulation of protein-based multiplex quantitative assays. Clin Chem. (2011) 57:560–7. doi: 10.1373/clinchem.2010.156034
194. Prabhakar U, Eirikis E, Reddy M, Silvestro E, Spitz S, Pendley C, et al. Validation and comparative analysis of a multiplexed assay for the simultaneous quantitative measurement of Th1/Th2 cytokines in human serum and human peripheral blood mononuclear cell culture supernatants. J Immunol Methods (2004) 291:27–38. doi: 10.1016/j.jim.2004.04.018
195. Nechansky A, Grunt S, Roitt IM, Kircheis R. Comparison of the calibration standards of three commercially available multiplex kits for human cytokine measurement to WHO standards reveals striking differences. Biomarker Insights (2008) 3:227–35.
196. Richens JL, Urbanowicz RA, Metcalf R, Corne J, O'Shea P, Fairclough L. Quantitative validation and comparison of multiplex cytokine kits. J Biomol Screen. (2010) 15:562–8. doi: 10.1177/1087057110362099
197. Khan SS, Smith MS, Reda D, Suffredini AF, McCoy JP. Multiplex bead array assays for detection of soluble cytokines: comparisons of sensitivity and quantitative values among kits from multiple manufacturers. Cytometry (2004) 61B:35–9. doi: 10.1002/cyto.b.20021
198. Breen EC, Reynolds SM, Cox C, Jacobson LP, Magpantay L, Mulder CB, et al. Multisite comparison of high-sensitivity multiplex cytokine assays. Clin Vacc Immunol. (2011) 18:1229–42. doi: 10.1128/CVI.05032-11
199. Siawaya JF, Djoba TR, Babb C, Black G, Golakai HJ, Stanley K, et al. An evaluation of commercial fluorescent bead-based luminex cytokine assays. PLOS ONE (2008) 3:e2535. doi: 10.1371/journal.pone.0002535
200. Chowdhury F, Williams A, Johnson P. Validation and comparison of two multiplex technologies, luminex and mesoscale discovery, for human cytokine profiling. J Immunol Methods (2009) 340:55–64. doi: 10.1016/j.jim.2008.10.002
201. Liu MY, Xydakis AM, Hoogeveen RC, Jones PH, Smith EO, Nelson KW, et al. Multiplexed analysis of biomarkers related to obesity and the metabolic syndrome in human plasma, using the Luminex-100 system. Clin Chem. (2005) 51:1102–9. doi: 10.1373/clinchem.2004.047084
202. Altara R, Manca M, Hermans KCM, Daskalopoulos EP, Brunner-La Rocca HP, Hermans RJJ, et al. Diurnal rhythms of serum and plasma cytokine profiles in healthy elderly individuals assessed using membrane based multiplexed immunoassay. J Transl Med. (2015) 13:129. doi: 10.1186/s12967-015-0477-1
203. Haack M, Pollmächer T, Mullington JM. Diurnal and sleep-wake dependent variations of soluble TNF- and IL-2 receptors in healthy volunteers. Brain Behav Immunity (2004) 18:361–7. doi: 10.1016/j.bbi.2003.12.009
204. Scheff JD, Calvano SE, Lowry SF, Androulakis IP. Modeling the influence of circadian rhythms on the acute inflammatory response. J Theor Biol. (2010) 264:1068–76. doi: 10.1016/j.jtbi.2010.03.026
205. Neupane SP, Skulberg A, Skulberg KR, Aass HCD, Bramness JG. Cytokine changes following acute ethanol intoxication in healthy men: a crossover study. Med Inflamm. (2016) 2016:3758590. doi: 10.1155/2016/3758590
206. Achur RN, Freeman WM, Vrana KE. Circulating cytokines as biomarkers of alcohol abuse and alcoholism. J Neuroimm Pharmacol. (2010) 5:83–91. doi: 10.1007/s11481-009-9185-z
207. Madretsma S, Wolters LM, van Dijk JP, Tak CJ, Feyerabend C, Wilson JH, et al. In-vivo effect of nicotine on cytokine production by human non-adherent mononuclear cells. Eur J Gastroenterol Hepatol. (1996) 8:1017–20.
208. Bao J, Liu Y, Yang J, Gao Q, Shi SQ, Garfield RE, et al. Nicotine inhibits LPS-induced cytokine production and leukocyte infiltration in rat placenta. Placenta (2016) 39:77–83. doi: 10.1016/j.placenta.2016.01.015
209. Revathikumar P, Bergqvist F, Gopalakrishnan S, Korotkova M, Jakobsson P, Lampa J, et al. Immunomodulatory effects of nicotine on interleukin 1β activated human astrocytes and the role of cyclooxygenase 2 in the underlying mechanism. J Neuroinflamm. (2016) 13:256. doi: 10.1186/s12974-016-0725-1
210. Nagarkatti P, Pandey R, Rieder SA, Hegde VL, Nagarkatti M. Cannabinoids as novel anti-inflammatory drugs. Future Med Chem. (2009) 1:1333–49. doi: 10.4155/fmc.09.93
211. Endres S, Whitaker RE, Ghorbani R, Meydani SN, Dinarello CA. Oral aspirin and ibuprofen increase cytokine-induced synthesis of IL-1 beta and of tumour necrosis factor-alpha ex vivo. Immunology (1996) 87:264–70.
212. Vgontzas AN, Zoumakis E, Bixler EO, Lin HM, Follett H, Kales A, et al. Adverse effects of modest sleep restriction on sleepiness, performance, and inflammatory cytokines. J Clin Endocrinol Metab. (2004) 89:2119–26. doi: 10.1210/jc.2003-031562
213. Kiecolt-Glaser JK, Preacher KJ, MacCallum RC, Atkinson C, Malarkey WB, Glaser R. Chronic stress and age-related increases in the proinflammatory cytokine IL-6. Proc Natl Acad Sci USA. (2003) 100:9090–5. doi: 10.1073/pnas.1531903100
214. Ostrowski K, Rohde T, Asp S, Schjerling P, Pedersen BK. Pro- and Anti-inflammatory cytokine balance in strenuous exercise in humans. J Physiol. (1999) 515(Pt 1):287–91.
215. Ostrowski K, Rohde T, Zacho M, Asp S, Pedersen BK. Evidence that interleukin-6 is produced in human skeletal muscle during prolonged running. J Physiol. (1998) 508(Pt 3):949–53.
216. Steensberg A, Keller C, Starkie RL, Osada T, Febbraio MA, Pedersen BK. IL-6 and TNF-alpha expression in, and release from, contracting human skeletal muscle. Am J Physiol Endocrinol Metab. (2002) 283:E1272–8. doi: 10.1152/ajpendo.00255.2002
217. Aulock SV, Deininger S, Draing C, Gueinzius K, Dehus O, Hermann K. Gender difference in cytokine secretion on immune stimulation with LPS and LTA. J Interf Cytok Res. (2006) 26:887–92. doi: 10.1089/jir.2006.26.887
218. Pellegrini P, Contasta I, Del Beato T, Ciccone F, Berghella AM. Gender-specific cytokine pathways, targets, and biomarkers for the switch from health to adenoma and colorectal cancer. Res Art. (2011) 2011:819724. doi: 10.1155/2011/819724
219. Khosravisamani M, Maliji G, Seyfi S, Azadmehr A, Abd Nikfarjam B, Madadi S, et al. Effect of the menstrual cycle on inflammatory cytokines in the periodontium. J Period Res. (2014) 49:770–6. doi: 10.1111/jre.12161
220. Hayashida H, Shimura M, Sugama K, Kazue K, Suzuki K. Effects of the menstrual cycle and acute aerobic exercise on cytokine levels. J Sports Med Doping Stud. (2015) 6:1–5. doi: 10.4172/2161-0673.1000173
221. Barrientos RM, Frank MG, Watkins LR, Maier SF. Memory impairments in healthy aging: role of aging-induced microglial sensitization. Aging Dis. (2010) 1:212–31.
222. Dai G, McMurray DN. Altered cytokine production and impaired antimycobacterial immunity in protein-malnourished guinea pigs. Infect Immun. (1998) 66:3562–8.
223. Grimble RF, Tappia PS. Modulation of pro-inflammatory cytokine biology by unsaturated fatty acids. Zeitschrift Fur Ernahrungswissenschaft (1998) 37(Suppl 1):57–65.
224. Payette C, Blackburn P, Lamarche B, Tremblay A, Bergeron J, Lemieux I, et al. Sex differences in postprandial plasma tumor necrosis factor-alpha, interleukin-6, and C-reactive protein concentrations. Metabolism (2009) 58:1593–601. doi: 10.1016/j.metabol.2009.05.011
225. Demirbilek S, Ozcan Ersoy M, Demirbilek S, Karaman A, Gurbuz N, Bayraktar N, et al. Small-dose capsaicin reduces systemic inflammatory responses in septic rats. Anesth Analg. (2004) 1501–7. doi: 10.1213/01.ANE.0000132975.02854.65
226. Dantzer R, Heijnen CJ, Kavelaars A, Laye S, Capuron L. The neuroimmune basis of fatigue. Trends Neuroscie. (2014) 37:39–46. doi: 10.1016/j.tins.2013.10.003
227. Donnarumma G, Buommino E, Baroni A, Auricchio L, De Filippis A, Cozza V, et al. Effects of AV119, a natural sugar from avocado, on Malassezia furfur invasiveness and on the expression of HBD-2 and cytokines in human keratinocytes. Exp Dermatol. (2007) 16:912–9. doi: 10.1111/j.1600-0625.2007.00613.x
228. Henrotin YE, Labasse AH, Jaspar JM, De Groote DD, Zheng SX, Guillou GB, et al. Effects of three avocado/soybean unsaponifiable mixtures on metalloproteinases, cytokines and prostaglandin E2 production by human articular chondrocytes. Clin Rheumatol. (1998) 17:31–9.
229. Solis Pereyra B, Lemonnier D. Induction of human cytokines by bacteria used in dairy foods. Nutr Res. (1993) 13:1127–40. doi: 10.1016/S0271-5317(05)80737-7
230. Manning PJ, Sutherland WHF, McGrath MM, de Jong SA, Walker RJ, Williams MJA. Postprandial cytokine concentrations and meal composition in obese and lean women. Obesity (2008) 16:2046–52. doi: 10.1038/oby.2008.334
231. Bachmeier BE, Mohrenz IV, Mirisola V, Schleicher E, Romeo F, Höhneke, et al. Curcumin downregulates the inflammatory cytokines CXCL1 and−2 in breast cancer cells via NFκB. Carcinogenesis (2008) 29:779–89. doi: 10.1093/carcin/bgm248
232. Silva LS, da Catalão CHR, Felippotti TT, de Oliveira-Pelegrin GR, Petenusci S, de Freitas LAP, et al. Curcumin suppresses inflammatory cytokines and heat shock protein 70 release and improves metabolic parameters during experimental sepsis. Pharmaceut Biol. (2017) 55:269–276. doi: 10.1080/13880209.2016.1260598
233. Netea SA, Janssen SA, Jaeger M, Jansen T, Jacobs L, Miller-Tomaszewska G, et al. Chocolate consumption modulates cytokine production in healthy individuals. Cytokine (2013) 62:40–3. doi: 10.1016/j.cyto.2013.02.003
Keywords: myalgic encephalomyelitis, neuroimaging, glia, microglia, PBR28, cytokines, translocator protein, positron emission tomography
Citation: VanElzakker MB, Brumfield SA and Lara Mejia PS (2019) Neuroinflammation and Cytokines in Myalgic Encephalomyelitis/Chronic Fatigue Syndrome (ME/CFS): A Critical Review of Research Methods. Front. Neurol. 9:1033. doi: 10.3389/fneur.2018.01033
Received: 26 August 2018; Accepted: 16 November 2018;
Published: 10 January 2019.
Edited by:
Kenneth Joseph Friedman, Medical School, Rutgers, The State University of New Jersey, United StatesReviewed by:
Dinesh Upadhya, Manipal Academy of Higher Education, IndiaAlberto Verrotti, University of L'Aquila, Italy
Copyright © 2019 VanElzakker, Brumfield and Lara Mejia. This is an open-access article distributed under the terms of the Creative Commons Attribution License (CC BY). The use, distribution or reproduction in other forums is permitted, provided the original author(s) and the copyright owner(s) are credited and that the original publication in this journal is cited, in accordance with accepted academic practice. No use, distribution or reproduction is permitted which does not comply with these terms.
*Correspondence: Michael B. VanElzakker, dmFuZWx6YWtAbm1yLm1naC5oYXJ2YXJkLmVkdQ==