- 1Department of Anatomy and Neuroscience, University of Melbourne, Parkville, VIC, Australia
- 2Department of Medicine, University of Melbourne, Parkville, VIC, Australia
- 3Australian Rehabilitation Research Centre, Royal Melbourne Hospital, Parkville, VIC, Australia
- 4Department of Anatomy and Neuroscience, VU Medical Centre, Amsterdam, Netherlands
- 5Florey Institute of Neuroscience and Mental Health, Parkville, VIC, Australia
Subtle gait and balance dysfunction is a precursor to loss of mobility in multiple sclerosis (MS). Biomechanical assessments using advanced gait and balance analysis technologies can identify these subtle changes and could be used to predict mobility loss early in the disease. This update critically evaluates advanced gait and balance analysis technologies and their applicability to identifying early lower limb dysfunction in people with MS. Non-wearable (motion capture systems, force platforms, and sensor-embedded walkways) and wearable (pressure and inertial sensors) biomechanical analysis systems have been developed to provide quantitative gait and balance assessments. Non-wearable systems are highly accurate, reliable and provide detailed outcomes, but require cumbersome and expensive equipment. Wearable systems provide less detail but can be used in community settings and can provide real-time feedback to patients and clinicians. Biomechanical analysis using advanced gait and balance analysis technologies can identify changes in gait and balance in early MS and consequently have the potential to significantly improve monitoring of mobility changes in MS.
Introduction
Mobility loss in people with multiple sclerosis (pwMS) is a major contributor to decreased quality of life, disruption to employment, and increased financial burden (1, 2). Motor weakness, loss of coordination, and spasticity can all manifest canonically as alterations in walking (gait) and balance that ultimately lead to mobility loss. Subtle gait and balance changes are apparent in pwMS even at the earliest disease stages and can be measured using advanced movement analysis techniques (3–5). Given their sensitivity, advanced movement analysis techniques could be used to identify patients at risk of mobility loss (6) or as outcomes in trials of therapies to preserve mobility.
Clinical assessment of gait in pwMS often involves visual evaluation and walking performance, tests of maximum distance walked, or timed walks (7). Both visual and performance tests are relatively reliable over time (8, 9); however, reliability varies with the degree of disability (8–10), and the tests are insensitive to subtle changes early in the disease (3–5, 7, 11).
Over the past two decades, advanced movement analysis technologies have been developed to improve objectivity, accuracy, quantification, and sensitivity to disease-related changes of clinical assessments of gait and balance (12–14). Advanced movement analysis technologies measure aspects of lower limb functions such as positions, angles, velocities, accelerations (kinematics), and forces and moments (kinetics) of limb segments and joints during walking. As such, these technologies can provide more sensitive markers of changes in walking and balance in pwMS than standard clinical assessments.
This review aims to present a synopsis of techniques that we consider to have potential utility for gait and balance assessment in pwMS and a discussion of the techniques when applied to gait/balance assessment in pwMS. We review both non-wearable and wearable gait analysis systems and discuss the variables measured by these systems as well as advantages, disadvantages, sensitivity, and accuracy. This information is also summarized in Table 1 for reference.
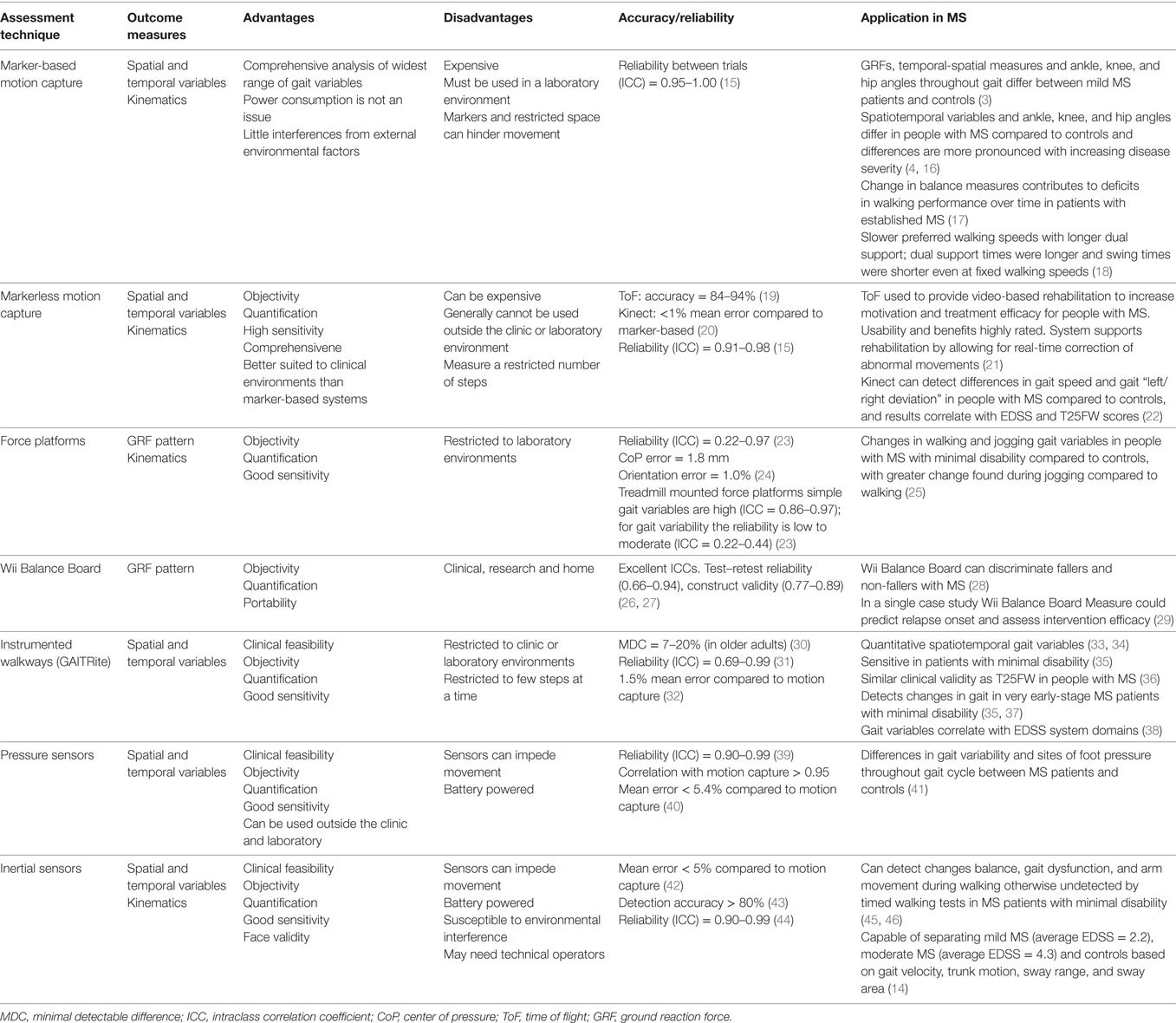
Table 1. Comparison of advanced techniques used for gait assessment in people with multiple sclerosis (MS).
Non-Wearable Gait Analysis Technologies
Non-wearable technologies generally provide the most sensitive and accurate gait data, yet require dedicated laboratory environments and are expensive compared to wearable systems (47). Three main non-wearable technologies are as follows: optical motion capture systems, force platforms/balance boards, and instrumented walkway mats.
Optical Motion Capture
Optical motion capture systems are based on optoelectronic stereophotogrammetry and measure kinematics of gait in three dimensions (47–50). These systems include marker-based and marker-less systems.
Marker-Based Systems
Marker-based systems utilize reflective markers placed on anatomical landmarks (e.g., joints) allowing them to capture motion of body parts. These systems are highly accurate (mean noise estimate = 0.03–0.05%) and reproducible [intraclass correlation coefficient (ICC) > 0.95] (15, 51). These systems can track the whole body, allowing them to record the most extensive range of kinematic variables of any gait assessment technique. These systems can be combined with force plates and/or electromyography (EMG) to collect ground reaction force (GRF) and muscle activation, enabling simultaneous assessments of kinematics and forces. The key limitation of marker-based systems is the need for dedicated spaces and technical operators, making them expensive and of limited clinical utility.
Several studies have used marker-based systems to quantify kinematic changes in gait and balance in pwMS (3, 4, 16, 18, 52). These studies show that, compared to healthy controls, pwMS displays: (1) reduced gait speed and stride length and prolonged double support time, even with fixed walking speed (3, 4, 16, 18), (2) differences in hip, knee, and ankle motion (3, 4, 16), and (3) abnormal timing of tibialis anterior and gastrocnemius activation (3, 4) with the degree of gait impairment associated with disease severity (4, 16). Reduced stride length appeared to be a consequence of reduced hip extension in mid and terminal stance, together with knee extension in late swing and at heel strike (16). Although increased double support time is usually interpreted as a strategy for increasing stability during gait, the opposite is true if destabilizing swing dynamics exist, particularly at non-preferred walking speeds (18). This could in part explain concomitant alterations to head and body centers of mass positions throughout gait that could provide additional stability (18). Indeed, two studies by Peebles and colleagues noted that dynamic stability (measured as the margin of stability which relates to the motion of the center of mass relative to the foot strike) worsened at faster walking speeds in pwMS and clinical gait disturbance (53) and was more severe in patients with a history of falls (54).
Two longitudinal studies have studied changes in gait using marker-based systems (17, 52). Fritz et al. (17) found no significant change in gait velocity over 2–3 years in 57 pwMS, despite an increase in T25FW. However, the authors did not provide a comprehensive assessment of gait function (e.g., timing of gait cycle events or joint motion), potentially limiting their ability to detect subtle changes. Galea et al. (52) noted a range of progressive changes over a brief 12-month period in 38 pwMS and mild diseases (EDSS < 3) including changes in ankle kinematics.
Marker-Less Systems
Although not as accurate and reliable as marker-based optical motion capture, marker-less motion tracking has the advantages of reduced preparation time and no hindrance to movement by body-mounted markers. Two categories of marker-less motion capture systems are available: active and passive vision systems. Active systems emit visible or infrared light using either laser, patterned or modulated light pulses. Passive systems utilize real-time image analysis.
Time of flight (ToF) systems are active marker-less systems that measure the motion of joints and segments across the whole body. ToF systems emit light (often infrared) that is reflected by all objects in the scene. A sensor is used to capture the reflected light and to calculate the distance based on the phase shift between the emitted and reflected light (55). These systems use self-contained light sources and a single camera making them relatively cheap and robust to differences in illumination. Recent advances in ToF systems have increased the accuracy of identification of gait patterns to 84–94% (19); however, the reliability of ToF has not been established. A single pilot study in pwMS employed ToF-based video applications during patient rehabilitation to improve usability and increase motivation (21). The real-time feedback from ToF allowed patients to self-correct abnormal movements, which was seen as a positive feature (21).
Similar to ToF, structured light systems operate by analyzing the deformation of a reflected light beam. The Kinect® sensor developed for video gaming is one of the most commonly used structured light systems due to its low cost (20, 35). Kinect can measure spatiotemporal features of gait such as heel strike and toe off, as well as knee and hip angles (56). Algorithms have been developed to improve the accuracy of gait measurements with Kinect, resulting in mean error estimates of <1% (20, 35, 57). Several studies have demonstrated that Kinect can accurately assess stride dynamics during walking to provide measures of walking speed, stride time, and stride length in healthy subjects (20, 35, 58, 59). Kinect has been used in a single study of MS patients during T25FW (22 MS patients, median EDSS = 3) (22). The investigators found differences in the degree of directional variability of gait, with good test–retest reliability (ICC > 0.9). Gait speed measured with Kinect correlated with T25FW time and EDSS (including brainstem and pyramidal subscores) (22). Further investigations are required to determine the applicability and reliability of Kinect for gait analysis in larger MS cohorts in clinical and home environments.
Force Platforms
Force platforms are steel blocks equipped with strain gauges or piezoelectric transducers measure GRF and can be embedded in a walkway or in treadmills for continuous recordings of multiple gait cycles. The gait cycle results in a repetitive and unique GRF pattern with precisely timed events such as heel-contact and toe-off that can be quantitatively assessed (60). Additionally, center of pressure (CoP) can be measured continuously between the body and ground as an indicator of balance. Force platforms are generally expensive and require dedicated laboratory environments and skilled technical personnel to operate. However, they can be used in conjunction with motion capture and EMG systems to provide joint kinetics (moments, power, and forces applied by each joint when braking or propelling) making them useful for laboratory-based assessments of gait and balance in pwMS. Additionally, graphical representations of gait, known as “butterfly diagrams,” can be produced that represent the 2D envelop of the GRF vectors during a step and could have clinical utility (25).
In-floor force platforms display high test–retest reliability for gait (61) and balance (62–65) variables. The reliability of treadmill-based force platforms for simple gait variables (mean stride frequency, stride width, time and length, and double stance phase) is also high (ICC = 0.86–0.97); however, for more complex measures such as gait variability, the reliability is low to moderate (ICC = 0.22–0.44) (23). Significant differences also exist in the GRF patterns during treadmill walking compared to overground walking, so it is unclear whether treadmills are optimal for identifying pathological gait function in neurological diseases (66–68).
In pwMS, force platforms have been used to study changes in gait initiation, postural stability, and balance associated with therapeutic interventions and disease progression (46, 69–72). Notably, Orsnes et al. (73) examined the timing of heel-contact and toe-off events in pwMS treated with baclofen (an agent used to treat spasticity in pwMS) using treadmill-embedded force platforms. The investigators observed only minimal improvements in gait and balance with treatment. A more recent study employed treadmill platforms to study both walking and jogging in minimally disabled pwMS (mean EDSS = 1.8) (25). Compared to controls, patients displayed greater step time difference between left and right feet and increased step width during both walking and jogging, but with greater change during jogging. The authors also noted that variability in the location of the CoP throughout gait cycle correlated with EDSS cerebellar scores.
Portable balance boards provide an alternative to laboratory-based force platforms. These boards use four force transducers (one on each corner of the platform) from which the CoP position can be calculated using suitable software (26). Nintendo Wii Balance Board (Nintendo, Kyoto, Japan) is the most widely tested balance board due to its low cost, portability (weighing only 3.5 kg), and wide availability. Wii Balance Board is suitable for clinical, laboratory, and home testing and demonstrates good test–retest reliability (ICC = 0.66–0.94) and construct validity when benchmarked against laboratory-grade force platforms (ICC = 0.77–0.89) (26, 27).
Wii Balance Board has been used with custom software to study postural sway in pwMS (28). Compared to laboratory force plates, Wii tended to overestimate postural sway although the test–retest reliability of the Wii has been found to be high (84%) (26–28). Castelli et al. (28) were also able to discriminate pwMS who reported fallers vs non-fallers. A case study employing Wii Balance Board noted changes in balance recorded during an exercise intervention in a single participant who had a relapse in the 6-week intervention period (29). The authors suggested that balance changes could provide a means to predict relapse onset (29). Several trials using Wii Balance Board have been undertaken and have shown potential improvements in mobility balance and QoL in pwMS (74–77), indicating that physical programs using this low cost technology could be useful for patients’ physical therapy. Overall, the cost and weight advantages of Wii, together with its high reliability and validity, make it a useful tool for assessing balance in MS in the clinic and home. Further investigations are required to identify the most useful measures that can be obtained from the device for clinical monitoring.
Instrumented Walkways
Instrumented walkway mats are portable mats a few meters in length with sensors to identify foot contacts. GAITRite is the most commonly used instrumented walkway mat and can determine spatiotemporal measures of gait (including walking speed; step and stride lengths; base of support; step, stride, swing, stance, single support, and double support times; and toe in/out angle) with high sensitivity for detecting pathology-related changes (30, 32). Spatiotemporal outputs from GAITRite do not require skilled personnel for analysis and interpretation (78), facilitating its use in clinical settings. The GAITrite has been validated against highly advanced motion capture systems for spatiotemporal measures (33, 34) and has high test–retest reliability (ICC 0.82–0.98) (79, 80) for most gait variables in young and older healthy adults at preferred and fast walking speeds.
In pwMS, GAITrite measurement of gait variables, including time to complete, velocity, cadence and number of steps, velocity, swing time, and single support, have been shown to be comparable to the T25FW in detecting gait dysfunction (36) and correlate with cerebellar EDSS subscores (38). GAITRite is also sensitive to changes in gait in very early-stage MS in patients with minimal disability (35, 37). A key shortcoming of GAITRite for gait assessment in pwMS is the restriction of data capture to a few steps at a time. Therefore, GAITRite provides no information regarding longer-term variability on any gait measures (81), measures that have been suggested as an indicator of gait dysfunction in pwMS (14, 37).
Advantages and Disadvantages of Non-Wearable Systems
Overall, non-wearable systems provide the most comprehensive measurements of gait kinematics available. These measurements are highly accurate, reliable, and sensitive to pathological changes, even early in the disease when clinical assessments lack sensitivity. However, these systems can be costly and are difficult to deploy in environments where everyday activities are performed (82). Low-cost marker-less optical motion capture systems such as Kinect, and portable balance boards such as Wii Balance Board, could overcome these problems, especially in clinical settings; however, as we discuss in the next section, the development of wearable technology could provide gait assessment in the community over longer time periods.
Gait Analysis Technologies Using Wearable Sensors
Wearable sensors have been developed for detailed measurement of gait kinematics in daily life (47). They can be placed on various parts of the body (e.g., under the foot, ankle, wrist, or waist) depending on requirements (e.g., pressure measurement under foot or center of mass movement at the waist). Two of the most promising new wearable sensors used to study gait dysfunction in pwMS are pressure sensors and inertial sensors.
Pressure Sensors
Pressure sensors are instrumented insoles placed or integrated into the shoe to measure changes in pressure between the foot and the ground. These sensors are comparable to the force platforms as they also measure the force from the ground applied to the foot, but unlike force platforms, they measure the force irrespective of its components in different directions (i.e., x-, y-, and z-axes) (39). Pressure sensors use plantar pressure measurements to calculate spatial-temporal gait variables, including phases of gait (e.g., stance time and swing time), and step time, length, and frequency (39, 83). There are a wide range of systems that use electromechanical sensors for plantar pressure analysis including capacitive, resistive, and piezoresistive sensors (39). When compressed, they calculate variations in applied load measuring proportional change in voltage (capacitive), conductance (resistive), or voltage (piezoresistive) (39). Arrays of sensors in configuration can measure plantar pressure in a matrix along the entire plantar surface.
The accuracy of discrete pressure sensor systems is comparable to optical motion capture (5.4% mean error) (40), external pressure calibration (ICC = 0.99), and when multiple insole pressure sensor systems are compared (ICC > 0.95) (39). In general, discrete and matrix pressure sensor insoles have good to excellent reliability for pressure measurements within and between trials (ICC = 0.80–0.99) (39, 84). However, as gait speed affects plantar pressure, it is recommended that gait speeds are controlled when collecting gait data with pressure sensors (84).
Three studies have used pressure sensor technology to study gait dysfunction in pwMS. One study used discrete pressure sensor insoles combined with mobile technology that included a hand held mobile device, to assess plantar pressure and step timing and observed greater plantar pressure in stance phase and greater variability in step timing in pwMS compared to controls (41). Two related studies assessed gait in early-stage MS (4) and changes in gait over the subsequent 12 months (52). Cross-sectionally, pwMS patients with pyramidal signs displayed increased double limb support and decreased walking speed and stride length compared to those with no pyramidal signs (4). Longitudinally, pwMS exhibited a decline in gait performance over 12 months in the absence of EDSS change (52). These results demonstrate that pressure sensors have the sensitivity to detect gait dysfunction in patients with no or minimal clinical disability.
Inertial Sensors
Inertial sensors measure an object’s acceleration and can also be used to report velocity, orientation, and gravitational forces. Inertial sensors are the most widely used type of wearable systems for gait and balance analysis and have been validated in healthy volunteers and in groups with motor impairment (85–87). The most promising inertial sensors for 3D gait analysis consist of a combination of tri-axial accelerometer, tri-axial gyroscope, and tri-axial magnetometer. Tri-axial sensors can capture spatiotemporal (e.g., swing time and cadence) and 3D kinematic data including joint and segment angles. Similar to the pressure sensors, inertial sensors can be integrated into insoles making them highly suitable for gait analysis. However, they can also be attached to other parts of the body such as on a belt or the wrist as illustrated in Figure 1. Additionally, technology is being developed for inertial sensor data collection, storage and/or transmission with smart devices such as phones and watches (88–90).
Trunk- or shank-placed inertial sensors have been used to study gait dysfunction in pwMS, commonly during the TUG test (termed “instrumented TUG”) (14, 42, 44, 46, 91, 92). Spain et al. (91) reported increased sway acceleration during quiet stance with eyes closed and increased trunk motion during instrumented TUG in pwMS with normal walking speed. In a follow-up longitudinal study (14), the authors assessed changes in gait and balance over 18 months, demonstrating no worsening of balance and objective gait measures (sway and gait velocity, respectively), but differentiation of mild MS (average EDSS = 2.2), moderate MS (average EDSS = 4.3), and control groups based on gait velocity, trunk motion, sway range, and sway area. Variability in sway area, sway range, and trunk motion over time were significantly different between all three groups. Similarly, Solomon et al. (93) found that inertial sensor data differentiated pwMS and no clinical gait dysfunction from controls using measures of postural sway (mediolateral sway path length and mediolateral sway range). Importantly, inertial sensors during TUG appear to be quite reproducible (ICC > 0.85 for all trunk and shank recordings from pwMS tested over two sessions), and some variables (stride velocity, cadence, and cycle time) correlate significantly with EDSS and number of recent falls (92).
Advantages and Disadvantages of Wearable Systems
The great advantage of wearable sensors is the ability to measure gait in an individual patient’s everyday environment for extended periods of time. These systems now employ small wireless sensors that can remotely send signals to the laboratory or clinic. Connectivity between wearable systems and ubiquitous smart phones and watches could further improve the usability of these devices. Importantly, the cost of wearable sensors is generally lower than non-wearable systems making analyses on large numbers of patients feasible. Finally, wearable systems actively engage the patient in both assessment and rehabilitation and could reduce clinic visits by providing more real time information to the patient and treating clinician (94).
Wearable systems also have certain disadvantages. First, wearable sensors can generally measure a smaller number of gait variables than non-wearable laboratory systems. Therefore, early studies of wearables should involve benchmarking and validation against these more comprehensive systems. Second, the placement of the sensors on body parts could hinder daily activities, though this could be improved with integration of sensors into clothing, smartphones, and watches. Third, algorithms used to measure speed and distance with wearable systems can lead to amplification of measurement error (95). Indeed, the algorithms required to calculate gait variables, which in some cases require technical personnel to implement, are currently a barrier to clinical application. However, algorithm development is an active area of research and clinician and patient interfaces continue to improve (96). Finally, the use of wearable sensors by patients themselves in uncontrolled everyday environments can make them more susceptible to signal noise (e.g., magnetic or vibration interference), leading to incorrect data and inadequate durations of recording when out of the clinic (97).
Conclusion and Future Directions
Escalating treatment in response to changing disease state in early MS can substantially affect outcomes, and identifying change in disease state throughout the course of MS is essential for optimal treatment (6). Current clinical and performance tests (EDSS and T25FW) for assessing gait function in pwMS are adequate for identifying advanced gait dysfunction, but fail to detect early subtle gait dysfunction or progression. In contrast, advanced motion analysis using wearable and non-wearable systems can overcome these problems. Laboratory-based systems offer the greatest sensitivity and are reliable over a wide spectrum of measures; however, these are costly, time- and space-intensive, and require technical skills for operation. Portable (i.e., Kinect, Wii Balance Board, and GAITRite) and wearable sensors offer less expensive alternatives for reliably measuring gait and balance variables and can be applied both in and out of the clinic. An example clinical application is balance training interventions for preventing falls (98, 99) that could be deployed and assessed using simple balance board technologies.
Future developments in portable and wearable systems will, in our opinion, allow these technologies to be used for monitoring and predicting disability in real-world environments. The feasibility of using wearable sensors has already been demonstrated for monitoring gait characteristics related to fall risk and symptoms in small groups of older adults (100) and people with Parkinson’s disease (101). Further studies are needed to investigate: (a) the gait characteristics that predict change in symptoms such as falls, relapses, or disability progression, and (b) the feasibility and utility of continuous monitoring of gait and balance in pwMS.
Author Contributions
CS, FB, LL, and SK made substantial contributions to the conception and design of this work, drafting the work and revising it critically for important intellectual content; gave final approval of the version to be published; and agree to be accountable for all aspects of the work in ensuring that questions related to the accuracy or integrity of any part of the work are appropriately investigated and resolved. AW, MG, MS, BM, FK and TK made substantial contributions to the conception and design of this work; revising it critically for important intellectual content; gave final approval of the version to be published; and agree to be accountable for all aspects of the work in ensuring that questions related to the accuracy or integrity of any part of the work are appropriately investigated and resolved.
Conflict of Interest Statement
No author of this manuscript has received financial support or other benefits from commercial sources for the work reported in the manuscript, or has any other financial interests that could create any potential conflict of interest or the appearance of a conflict of interest with regard to the work.
The reviewer WS-M and handling editor declared their shared affiliation.
Funding
This work was supported by National Health and Medical Research Council of Australia (APP1054147).
References
1. Edgley K, Sullican MJ, Dehoux E. A survey of multiple sclerosis: II. Determinants of employment status. Can J Rehabil (1991) 4:127–32.
2. Kobelt G, Berg J, Lindgren P, Fredrikson S, Jonsson B. Costs and quality of life of patients with multiple sclerosis in Europe. J Neurol Neurosurg Psychiatry (2006) 77:918–26. doi:10.1136/jnnp.2006.090365
3. Benedetti MG, Piperno R, Simoncini L, Bonato P, Tonini A, Giannini S. Gait abnormalities in minimally impaired multiple sclerosis patients. Mult Scler (1999) 5:363–8. doi:10.1191/135245899678846393
4. Martin CL, Phillips BA, Kilpatrick TJ, Butzkueven H, Tubridy N, Mcdonald E, et al. Gait and balance impairment in early multiple sclerosis in the absence of clinical disability. Mult Scler (2006) 12:620–8. doi:10.1177/1352458506070658
5. Kalron A, Achiron A, Dvir Z. Muscular and gait abnormalities in persons with early onset multiple sclerosis. J Neurol Phys Ther (2011) 35:164–9. doi:10.1097/NPT.0b013e31823801f4
6. Jokubaitis VG, Spelman T, Kalincik T, Lorscheider J, Havrdova E, Horakova D, et al. Predictors of long-term disability accrual in relapse-onset multiple sclerosis. Ann Neurol (2016) 80:89–100. doi:10.1002/ana.24682
7. Kieseier BC, Pozzilli C. Assessing walking disability in multiple sclerosis. Mult Scler (2012) 18:914–24. doi:10.1177/1352458512444498
8. Nilsagard Y, Lundholm C, Gunnarsson LG, Dcnison E. Clinical relevance using timed walk tests and ‘timed up and go’ testing in persons with multiple sclerosis. Physiother Res Int (2007) 12:105–14. doi:10.1002/pri.358
9. Learmonth YC, Dlugonski DD, Pilutti LA, Sandroff BM, Motl RW. The reliability, precision and clinically meaningful change of walking assessments in multiple sclerosis. Mult Scler (2013) 19:1784–91. doi:10.1177/1352458513483890
10. Bethoux F, Bennett S. Evaluating walking in patients with multiple sclerosis: which assessment tools are useful in clinical practice? Int J MS Care (2011) 13:4–14. doi:10.7224/1537-2073-13.1.4
11. Nieuwenhuis MM, Van Tongeren H, Sorensen PS, Ravnborg M. The six spot step test: a new measurement for walking ability in multiple sclerosis. Mult Scler (2006) 12:495–500. doi:10.1191/1352458506ms1293oa
12. Aly NM, Playfer JR, Smith SL, Halliday DM. A novel computer-based technique for the assessment of tremor in Parkinson’s disease. Age Ageing (2007) 36:395–9. doi:10.1093/ageing/afm061
13. Najafi B, Khan T, Wrobel J. Laboratory in a box: wearable sensors and its advantages for gait analysis. Conf Proc IEEE Eng Med Biol Soc (2011) 2011:6507–10. doi:10.1109/IEMBS.2011.6091605
14. Spain RI, Mancini M, Horak FB, Bourdette D. Body-worn sensors capture variability, but not decline, of gait and balance measures in multiple sclerosis over 18 months. Gait Posture (2014) 39:958–64. doi:10.1016/j.gaitpost.2013.12.010
15. Schmitz A, Ye M, Boggess G, Shapiro R, Yang R, Noehren B. The measurement of in vivo joint angles during a squat using a single camera markerless motion capture system as compared to a marker based system. Gait Posture (2015) 41:694–8. doi:10.1016/j.gaitpost.2015.01.028
16. Kelleher KJ, Spence W, Solomonidis S, Apatsidis D. The characterisation of gait patterns of people with multiple sclerosis. Disabil Rehabil (2010) 32:1242–50. doi:10.3109/09638280903464497
17. Fritz NE, Marasigan RE, Calabresi PA, Newsome SD, Zackowski KM. The impact of dynamic balance measures on walking performance in multiple sclerosis. Neurorehabil Neural Repair (2015) 29:62–9. doi:10.1177/1545968314532835
18. Remelius JG, Jones SL, House JD, Busa MA, Averill JL, Sugumaran K, et al. Gait impairments in persons with multiple sclerosis across preferred and fixed walking speeds. Arch Phys Med Rehabil (2012) 93:1637–42. doi:10.1016/j.apmr.2012.02.019
19. Afendi T, Kurugollu F, Crookes D, Bouridane A. A frontal view gait recognition based on 3D imaging using a time of flight camera. Signal Processing Conference (EUSIPCO), 2014 Proceedings of the 22nd European. Lisbon, Portugal: (2014). p. 2435–9.
20. Clark RA, Pua YH, Bryant AL, Hunt MA. Validity of the Microsoft Kinect for providing lateral trunk lean feedback during gait retraining. Gait Posture (2013) 38:1064–6. doi:10.1016/j.gaitpost.2013.03.029
21. Eguiluz G, Garcia MB. Use of a time-of-flight camera with an Omek Beckon framework to analyze, evaluate and correct in real time the verticality of multiple sclerosis patients during exercise. Int J Environ Res Public Health (2013) 10:5807–29. doi:10.3390/ijerph10115807
22. Behrens J, Pfuller C, Mansow-Model S, Otte K, Paul F, Brandt AU. Using perceptive computing in multiple sclerosis – the short maximum speed walk test. J Neuroeng Rehabil (2014) 11:89. doi:10.1186/1743-0003-11-89
23. Faude O, Donath L, Roth R, Fricker L, Zahner L. Reliability of gait parameters during treadmill walking in community-dwelling healthy seniors. Gait Posture (2012) 36:444–8. doi:10.1016/j.gaitpost.2012.04.003
24. Goldberg SR, Kepple TM, Stanhope SJ. In situ calibration and motion capture transformation optimization improve instrumented treadmill measurements. J Appl Biomech (2009) 25:401–6. doi:10.1123/jab.25.4.401
25. Kalron A, Dvir Z, Givon U, Baransi H, Achiron A. Gait and jogging parameters in people with minimally impaired multiple sclerosis. Gait Posture (2014) 39:297–302. doi:10.1016/j.gaitpost.2013.07.124
26. Clark RA, Bryant AL, Pua Y, Mccrory P, Bennell K, Hunt M. Validity and reliability of the Nintendo Wii Balance Board for assessment of standing balance. Gait Posture (2010) 31:307–10. doi:10.1016/j.gaitpost.2009.11.012
27. Bartlett HL, Ting LH, Bingham JT. Accuracy of force and center of pressure measures of the Wii Balance Board. Gait Posture (2014) 39:224–8. doi:10.1016/j.gaitpost.2013.07.010
28. Castelli L, Stocchi L, Patrignani M, Sellitto G, Giuliani M, Prosperini L. We-measure: toward a low-cost portable posturography for patients with multiple sclerosis using the commercial Wii balance board. J Neurol Sci (2015) 359:440–4. doi:10.1016/j.jns.2015.10.016
29. Goble DJ, Cone BL, Thurman J, Corey-Bloom J. Balance declines may predict relapse onset in multiple sclerosis-a case study. J Dev Phys Disabil (2014) 26:145–50. doi:10.1007/s10882-013-9350-4
30. Youdas JW, Childs KB, Mcneil ML, Mueller AC, Quilter CM, Hollman JH. Responsiveness of 2 procedures for measurement of temporal and spatial gait parameters in older adults. PM R (2010) 2:537–43. doi:10.1016/j.pmrj.2010.02.008
31. Cho KH, Lee HJ, Lee WH. Test-retest reliability of the GAITRite walkway system for the spatio-temporal gait parameters while dual-tasking in post-stroke patients. Disabil Rehabil (2015) 37:512–6. doi:10.3109/09638288.2014.932445
32. Stokic DS, Horn TS, Ramshur JM, Chow JW. Agreement between temporospatial gait parameters of an electronic walkway and a motion capture system in healthy and chronic stroke populations. Am J Phys Med Rehabil (2009) 88:437–44. doi:10.1097/PHM.0b013e3181a5b1ec
33. Bilney B, Morris M, Webster K. Concurrent related validity of the GAITRite walkway system for quantification of the spatial and temporal parameters of gait. Gait Posture (2003) 17:68–74. doi:10.1016/S0966-6362(02)00053-X
34. Webster KE, Wittwer JE, Feller JA. Validity of the GAITRite® walkway system for the measurement of averaged and individual step parameters of gait. Gait Posture (2005) 22:317–21. doi:10.1016/j.gaitpost.2004.10.005
35. Gabel M, Gilad-Bachrach R, Renshaw E, Schuster A. Full body gait analysis with Kinect. Conf Proc IEEE Eng Med Biol Soc (2012) 2012:1964–7. doi:10.1109/EMBC.2012.6346340
36. Hochsprung A, Heredia-Camacho B, Castillo M, Izquierdo G, Escudero-Uribe S. Clinical validity of the quantitative gait variables in patients with multiple sclerosis. A comparison of the Timed 25-foot Walk Test and the GAITRite ® Electronic Walkway system. Rev Neurol (2014) 59:8–12.
37. Sosnoff JJ, Sandroff BM, Motl RW. Quantifying gait abnormalities in persons with multiple sclerosis with minimal disability. Gait Posture (2012) 36:154–6. doi:10.1016/j.gaitpost.2011.11.027
38. Givon U, Zeilig G, Achiron A. Gait analysis in multiple sclerosis: characterization of temporal–spatial parameters using GAITRite functional ambulation system. Gait Posture (2009) 29:138–42. doi:10.1016/j.gaitpost.2008.07.011
39. Castro MP, Meucci M, Soares DP, Fonseca P, Borgonovo-Santos M, Sousa F, et al. Accuracy and repeatability of the gait analysis by the WalkinSense system. Biomed Res Int (2014) 2014:348659. doi:10.1155/2014/348659
40. Howell A, Kobayashi T, Hayes H, Foreman K, Bamberg S. Kinetic gait analysis using a low-cost insole. IEEE Trans Biomed Eng (2013) 60(12):3284–90. doi:10.1109/TBME.2013.2250972
41. Viqueira Villarejo M, Maeso Garcia J, Garcia Zapirain B, Mendez Zorrilla A. Technological solution for determining gait parameters using pressure sensors: a case study of multiple sclerosis patients. Biomed Mater Eng (2014) 24:3511–22. doi:10.3233/BME-141177
42. Salarian A, Burkhard PR, Vingerhoets FJ, Jolles BM, Aminian K. A novel approach to reducing number of sensing units for wearable gait analysis systems. IEEE Trans Biomed Eng (2013) 60:72–7. doi:10.1109/TBME.2012.2223465
43. Williamson R, Andrews BJ. Sensor systems for lower limb functional electrical stimulation (FES) control. Med Eng Phys (2000) 22:313–25. doi:10.1016/S1350-4533(00)00038-2
44. Greene BR, Rutledge S, Mcgurgan I, McGuigan C, OConnell K, Caulfield B, et al. Assessment and classification of early-stage multiple sclerosis with inertial sensors: comparison against clinical measures of disease state. IEEE J Biomed Health Inform (2015) 19:1356–61. doi:10.1109/JBHI.2015.2435057
45. Olazaran J, Hernandez-Tamames JA, Molina E, Garcia-Polo P, Dobato JL, Alvarez-Linera J, et al. Clinical and anatomical correlates of gait dysfunction in Alzheimer’s disease. J Alzheimers Dis (2013) 33:495–505. doi:10.3233/JAD-2012-121207
46. Carpinella I, Cattaneo D, Ferrarin M. Quantitative assessment of upper limb motor function in multiple sclerosis using an instrumented action research arm test. J Neuroeng Rehabil (2014) 11:67. doi:10.1186/1743-0003-11-67
47. Muro-de-la-Herran A, Garcia-Zapirain B, Mendez-Zorrilla A. Gait analysis methods: an overview of wearable and non-wearable systems, highlighting clinical applications. Sensors (Basel) (2014) 14:3362–94. doi:10.3390/s140203362
48. Gomatam ANM, Sasi S. Multimodal gait recognition based on stereo vision and 3D template matching. In: Arabnia HR, editor. CISST. CSREA Press (2004). p. 405–10.
49. Haitao L, Yang C, Zengfu W. Automatic gait recognition from a distance. Control and Decision Conference (CCDC), 2010 Chinese. Xuzhou, China: (2010). p. 2777–82.
50. Kim J, Gravunder A, Stanley CJ, Park HS. Low-cost implementation of a self-paced treadmill by using a commercial depth sensor. Conf Proc IEEE Eng Med Biol Soc (2013) 2013:874–7. doi:10.1109/EMBC.2013.6609640
51. Maletsky LP, Sun J, Morton NA. Accuracy of an optical active-marker system to track the relative motion of rigid bodies. J Biomech (2007) 40:682–5. doi:10.1016/j.jbiomech.2006.01.017
52. Galea MP, Cofre Lizama LE, Butzkueven H, Kilpatrick TJ. Gait and balance deterioration over a 12-month period in multiple sclerosis patients with EDSS scores </= 3.0. NeuroRehabilitation (2017) 40:277–84. doi:10.3233/NRE-161413
53. Peebles AT, Reinholdt A, Bruetsch AP, Lynch SG, Huisinga JM. Dynamic margin of stability during gait is altered in persons with multiple sclerosis. J Biomech (2016) 49:3949–55. doi:10.1016/j.jbiomech.2016.11.009
54. Peebles AT, Bruetsch AP, Lynch SG, Huisinga JM. Dynamic balance in persons with multiple sclerosis who have a falls history is altered compared to non-fallers and to healthy controls. J Biomech (2017) 63:158–63. doi:10.1016/j.jbiomech.2017.08.023
55. Kolb A, Barth E, Koch R, Larsen R. Time-of-flight cameras in computer graphics. Comput Graph Forum (2010) 29:141–59. doi:10.1111/j.1467-8659.2009.01583.x
56. Pfister A, West AM, Bronner S, Noah JA. Comparative abilities of Microsoft Kinect and Vicon 3D motion capture for gait analysis. J Med Eng Technol (2014) 38:274–80. doi:10.3109/03091902.2014.909540
57. Shotton J, Girshick R, Fitzgibbon A, Sharp T, Cook M, Finocchio M, et al. Efficient human pose estimation from single depth images. IEEE Trans Pattern Anal Mach Intell (2013) 35:2821–40. doi:10.1109/TPAMI.2012.241
58. Stone EE, Skubic M. Evaluation of an inexpensive depth camera for passive in-home fall risk assessment. Pervasive Computing Technologies for Healthcare (PervasiveHealth), 2011 5th International Conference on. Dublin, Ireland: (2011). p. 71–7.
59. Auvinet E, Multon F, Aubin CE, Meunier J, Raison M. Detection of gait cycles in treadmill walking using a Kinect. Gait Posture (2015) 41:722–5. doi:10.1016/j.gaitpost.2014.08.006
60. Bae J, Kong K, Byl N, Tomizuka M. A mobile gait monitoring system for abnormal gait diagnosis and rehabilitation: a pilot study for Parkinson disease patients. J Biomech Eng (2011) 133:041005. doi:10.1115/1.4003525
61. Bennell K, Crossley K, Wrigley T, Nitschke J. Test-retest reliability of selected ground reaction force parameters and their symmetry during running. J Appl Biomech (1999) 15:330–6. doi:10.1123/jab.15.3.330
62. Goldie PA, Bach TM, Evans OM. Force platform measures for evaluating postural control: reliability and validity. Arch Phys Med Rehabil (1989) 70:510–7.
63. Bauer C, Gröger I, Rupprecht R, Gaßmann KG. Intrasession Reliability of Force Platform Parameters in Community-Dwelling Older Adults. Arch Phys Med Rehabil (2008) 89:1977–82. doi:10.1016/j.apmr.2008.02.033
64. Pinsault N, Vuillerme N. Test-retest reliability of centre of foot pressure measures to assess postural control during unperturbed stance. Med Eng Phys (2009) 31:276–86. doi:10.1016/j.medengphy.2008.08.003
65. Meshkati Z, Namazizadeh M, Salavati M, Mazaheri M. Reliability of force-platform measures of postural sway and expertise-related differences. J Sport Rehabil (2011) 20:442–56. doi:10.1123/jsr.20.4.442
66. Brouwer B, Parvataneni K, Olney SJ. A comparison of gait biomechanics and metabolic requirements of overground and treadmill walking in people with stroke. Clin Biomech (Bristol, Avon) (2009) 24:729–34. doi:10.1016/j.clinbiomech.2009.07.004
67. Chockalingam N, Chatterley F, Healy AC, Greenhalgh A, Branthwaite HR. Comparison of pelvic complex kinematics during treadmill and overground walking. Arch Phys Med Rehabil (2012) 93:2302–8. doi:10.1016/j.apmr.2011.10.022
68. Nagano H, Begg RK, Sparrow WA, Taylor S. A comparison of treadmill and overground walking effects on step cycle asymmetry in young and older individuals. J Appl Biomech (2013) 29:188–93. doi:10.1123/jab.29.2.188
69. Hatzitaki V, Koudouni A, Orologas A. Learning of a novel visuo-postural co-ordination task in adults with multiple sclerosis. J Rehabil Med (2006) 38:295–301. doi:10.1080/16501970600680247
70. Remelius JG, Hamill J, Kent-Braun J, Van Emmerik RE. Gait initiation in multiple sclerosis. Motor Control (2008) 12:93–108. doi:10.1123/mcj.12.2.93
71. Cattaneo D, Jonsdottir J. Sensory impairments in quiet standing in subjects with multiple sclerosis. Mult Scler (2009) 15:59–67. doi:10.1177/1352458508096874
72. Prosperini L, Fortuna D, Gianni C, Leonardi L, Pozzilli C. The diagnostic accuracy of static posturography in predicting accidental falls in people with multiple sclerosis. Neurorehabil Neural Repair (2013) 27:45–52. doi:10.1177/1545968312445638
73. Orsnes GB, Sorensen PS, Larsen TK, Ravnborg M. Effect of baclofen on gait in spastic MS patients. Acta Neurol Scand (2000) 101:244–8. doi:10.1034/j.1600-0404.2000.101004244x./
74. Kalron A, Frid L. Nintendo Wii virtual reality game improves short term balance capabilities in multiple sclerosis patients: a pilot quasi-experimental study. J Phys Ther (2012) 5:54–62.
75. Brichetto G, Spallarossa P, De Carvalho ML, Battaglia MA. The effect of Nintendo(R) Wii(R) on balance in people with multiple sclerosis: a pilot randomized control study. Mult Scler (2013) 19:1219–21. doi:10.1177/1352458512472747
76. Nilsagard YE, Forsberg AS, Von Koch L. Balance exercise for persons with multiple sclerosis using Wii games: a randomised, controlled multi-centre study. Mult Scler (2013) 19:209–16. doi:10.1177/1352458512450088
77. Prosperini L, Fortuna D, Gianni C, Leonardi L, Marchetti MR, Pozzilli C. Home-based balance training using the Wii balance board: a randomized, crossover pilot study in multiple sclerosis. Neurorehabil Neural Repair (2013) 27:516–25. doi:10.1177/1545968313478484
78. Kressig RW, Beauchet O. Guidelines for clinical applications of spatio-temporal gait analysis in older adults. Aging Clin Exp Res (2006) 18:174–6. doi:10.1007/BF03327437
79. Menz HB, Latt MD, Tiedemann A, Mun San Kwan M, Lord SR. Reliability of the GAITRite® walkway system for the quantification of temporo-spatial parameters of gait in young and older people. Gait Posture (2004) 20:20–5. doi:10.1016/S0966-6362(03)00068-7
80. Van Uden CJT, Besser MP. Test-retest reliability of temporal and spatial gait characteristics measured with an instrumented walkway system (GAITRite). BMC Musculoskelet Disord (2004) 5:13. doi:10.1186/1471-2474-5-13
81. Greene BR, Foran TG, Mcgrath D, Doheny EP, Burns A, Caulfield B. A comparison of algorithms for body-worn sensor-based spatiotemporal gait parameters to the GAITRite electronic walkway. J Appl Biomech (2012) 28:349–55. doi:10.1123/jab.28.3.349
82. Owings TM, Grabiner MD. Measuring step kinematic variability on an instrumented treadmill: how many steps are enough? J Biomech (2003) 36:1215–8. doi:10.1016/S0021-9290(03)00108-8
83. Kong K, Tomizuka M. A gait monitoring system based on air pressure sensors embedded in a shoe. IEEE/ASME Trans Mechatron (2009) 14:358–70. doi:10.1109/TMECH.2008.2008803
84. Kemozek TW, Zimmer KA. Reliability and running speed effects of in-shoe loading measurements during slow treadmill running. Foot Ankle Int (2000) 21:749–52. doi:10.1177/107110070002100906
85. Tien I, Glaser SD, Aminoff MJ. Characterization of gait abnormalities in Parkinson’s disease using a wireless inertial sensor system. Conf Proc IEEE Eng Med Biol Soc (2010) 2010:3353–6. doi:10.1109/IEMBS.2010.5627904
86. Bugane F, Benedetti MG, D’angeli V, Leardini A. Estimation of pelvis kinematics in level walking based on a single inertial sensor positioned close to the sacrum: validation on healthy subjects with stereophotogrammetric system. Biomed Eng Online (2014) 13:146. doi:10.1186/1475-925X-13-146
87. Mason BS, Rhodes JM, Goosey-Tolfrey VL. Validity and reliability of an inertial sensor for wheelchair court sports performance. J Appl Biomech (2014) 30:326–31. doi:10.1123/jab.2013-0148
88. McGuire ML. An overview of gait analysis and step detection in mobile computing devices. Proceedings of the 2012 4th International Conference on Intelligent Networking and Collaborative Systems, (INCoS) 2012. Bucharest, Romania: (2012). p. 648–51.
89. Johnston AH, Weiss GM. Smartwatch-based biometric gait recognition. 2015 IEEE 7th International Conference on Biometrics Theory, Applications and Systems, BTAS 2015. Arlington, VA, USA: (2015).
90. Xu W. Mobile applications based on smart wearable devices. SenSys 2015 – Proceedings of the 13th ACM Conference on Embedded Networked Sensor Systems. New York, NY (2015). p. 505–6.
91. Spain RI, St. George RJ, Salarian A, Mancini M, Wagner JM, Horak FB, et al. Body-worn motion sensors detect balance and gait deficits in people with multiple sclerosis who have normal walking speed. Gait Posture (2012) 35:573–8. doi:10.1016/j.gaitpost.2011.11.026
92. Craig JJ, Bruetsch AP, Lynch SG, Horak FB, Huisinga JM. Instrumented balance and walking assessments in persons with multiple sclerosis show strong test-retest reliability. J Neuroeng Rehabil (2017) 14:43. doi:10.1186/s12984-017-0251-0
93. Solomon AJ, Jacobs JV, Lomond KV, Henry SM. Detection of postural sway abnormalities by wireless inertial sensors in minimally disabled patients with multiple sclerosis: a case-control study. J Neuroeng Rehabil (2015) 12:74. doi:10.1186/s12984-015-0066-9
94. Longtin Y, Sax H, Leape LL, Sheridan SE, Donaldson L, Pittet D. Patient participation: current knowledge and applicability to patient safety. Mayo Clin Proc (2010) 85:53–62. doi:10.4065/mcp.2009.0248
95. Tao W, Liu T, Zheng R, Feng H. Gait analysis using wearable sensors. Sensors (Basel) (2012) 12:2255–83. doi:10.3390/s120202255
96. Ejupi A, Gschwind YJ, Valenzuela T, Lord SR, Delbaere K. A Kinect and inertial sensor-based system for the self-assessment of fall risk: a home-based study in older people. Hum Comput Interact (2015) 31:261–93. doi:10.1080/07370024.2015.1085309
97. Khusainov R, Azzi D, Achumba IE, Bersch SD. Real-time human ambulation, activity, and physiological monitoring: taxonomy of issues, techniques, applications, challenges and limitations. Sensors (Basel) (2013) 13:12852–902. doi:10.3390/s131012852
98. Nilsagard YE, Von Koch LK, Nilsson M, Forsberg AS. Balance exercise program reduced falls in people with multiple sclerosis: a single-group, pretest-posttest trial. Arch Phys Med Rehabil (2014) 95:2428–34. doi:10.1016/j.apmr.2014.06.016
99. Gunn H, Markevics S, Haas B, Marsden J, Freeman J. Systematic review: the effectiveness of interventions to reduce falls and improve balance in adults with multiple sclerosis. Arch Phys Med Rehabil (2015) 96:1898–912. doi:10.1016/j.apmr.2015.05.018
100. Mancini M, Schlueter H, El-Gohary M, Mattek N, Duncan C, Kaye J, et al. Continuous monitoring of turning mobility and its association to falls and cognitive function: a pilot study. J Gerontol A Biol Sci Med Sci (2016) 71:1102–8. doi:10.1093/gerona/glw019
Keywords: multiple sclerosis, mobility loss, gait, balance, biomechanics
Citation: Shanahan CJ, Boonstra FMC, Cofré Lizama LE, Strik M, Moffat BA, Khan F, Kilpatrick TJ, van der Walt A, Galea MP and Kolbe SC (2018) Technologies for Advanced Gait and Balance Assessments in People with Multiple Sclerosis. Front. Neurol. 8:708. doi: 10.3389/fneur.2017.00708
Received: 01 September 2017; Accepted: 07 December 2017;
Published: 02 February 2018
Edited by:
Robert Weissert, University of Regensburg, GermanyReviewed by:
Wilhelm Schulte-Mattler, University of Regensburg, GermanyMatthias Grothe, University of Greifswald, Germany
Copyright: © 2018 Shanahan, Boonstra, Cofré Lizama, Strik, Moffat, Khan, Kilpatrick, van der Walt, Galea and Kolbe. This is an open-access article distributed under the terms of the Creative Commons Attribution License (CC BY). The use, distribution or reproduction in other forums is permitted, provided the original author(s) and the copyright owner are credited and that the original publication in this journal is cited, in accordance with accepted academic practice. No use, distribution or reproduction is permitted which does not comply with these terms.
*Correspondence: Scott C. Kolbe, a29sYmVzQHVuaW1lbGIuZWR1LmF1
†These authors have contributed equally to this work.