- 1Clinical Department of Medical Psychology, Medical University of Innsbruck, Innsbruck, Austria
- 2Department of Psychology, University Innsbruck, Innsbruck, Austria
- 3Department of Rehabilitation Psychology and Psychotherapy, University of Freiburg, Freiburg, Germany
- 4Department of Psychosomatic Medicine and Psychotherapy, University Hospital Freiburg, Freiburg, Germany
- 5Department of Statistics and Operations Research, University of Granada, Granada, Spain
- 6Division of Biological Chemistry, Biocenter, Medical University of Innsbruck, Innsbruck, Austria
This study on a breast cancer survivor suffering from cancer-related fatigue (CaRF) and depression investigated the bidirectional relationship between cellular immune activity and subjective sleep. The 49-year-old patient (breast cancer diagnosis 5 years before the study, currently in remission) collected her full urine output for 28 days in 12-h intervals (8:00 p.m. to 8:00 a.m. and 8:00 a.m. to 8:00 p.m.). These urine samples were used to determine urinary neopterin (cellular immune activation marker) and creatinine concentrations via high-pressure liquid chromatography (HPLC). Each morning, the patient answered questions on five sleep variables: sleep quality (SQ), sleep recreational value (SRV), total sleep time (TST), total wake time (TWT), and awakenings during sleep period (ADS). For the purpose of this study, the time series of the nighttime urinary neopterin levels and the five sleep variables were determined. Using centered moving average (CMA) smoothing and cross-correlational analysis, this study showed that increases in the positive sleep variables SQ and SRV were followed by urinary neopterin concentration decreases after 96–120 h (SQ, lag 4: r = −0.411; p = 0.044; SRV: lag 4: r = −0.472; p = 0.021) and 120–144 h (SRV, lag 5: r = −0.464; p = 0.026). Increases in the negative sleep variable TWT, by contrast, were followed by increases in urinary neopterin concentrations 72–96 h later (lag 3: r = 0.522; p = 0.009). No systematic effects in the other direction, i.e., from urinary neopterin levels to sleep, were observed in this study. Although preliminary, the findings of this study highlight the benefit of carefully investigating temporal delays and directions of effects when studying the dynamic relationship between sleep and immune variables in the natural context of everyday life.
Introduction
In this study, we investigated the bidirectional relationship between cellular immune activity and subjective sleep in a breast cancer survivor suffering from cancer-related fatigue (CaRF) and depression.
The patient under study, a 49-year-old Caucasian woman with the German Abitur qualification (high school diploma with university entrance examination), is married and has three children. She has suffered chronically from dysthymia (F34.1). About 5 years before this study began, the patient was diagnosed with a ductal mamma carcinoma (C50.4) of her right breast [pT2, pN1biv (6 of 13), cM0, G3, R0, ER 10%, PR 70–80%, HER2+/neu+, score = 3]. For primary cancer therapy, the patient underwent surgery, radiotherapy and antiestrogen therapy (tamoxifen). Antiestrogen therapy ended 6 months before study start. At study start, the patient was clinically free of evidence of metastatic or recurrent lesions. Since cancer diagnosis and therapy, the patient has been suffering from severe CaRF. CaRF symptoms persisted until study start and beyond. Furthermore, chronic depressive disorder symptoms (dysthymia, F34.1) increased since cancer diagnosis and therapy, leading to an additional clinical diagnosis of adjustment disorder with depressed mood (F43.21) at the start of the study. Aside from these health issues, the patient has no history of immunological or endocrinological disease or health conditions that could affect the immune and endocrine systems.
As to the patient’s subjective assessment of her sleep before study start, the Center for Epidemiologic Studies Depression Scale (in German) revealed that her sleep was restless on 5–7 days during the preceding week (score 4 on a 4-point Likert scale). For the same week, the EORTC QLQ-C30 (version 3, in German) showed that the patient had moderate sleep difficulties (score 3 on a 4-point Likert scale).
Background
Long-term behavioral symptoms are prominent in cancer patients (1). They can persist many years after primary treatment, entail various psychological and medical consequences, and lead to a reduction in quality of life (1, 2). They may also facilitate cancer recurrence (1, 2).
One of these behavioral symptoms is sleep disturbance, i.e., symptoms of “perceived or actual alterations in night sleep with resultant daytime impairment” (3, 4). Sleep disturbances affect about 50% of breast cancer survivors (5). In this article, the term “cancer survivor” refers to patients who have finished primary cancer treatment (6).
Emerging evidence suggests that cancer-related sleep disturbances are associated with biological changes, such as immunological dysregulation (2). In healthy individuals, data indicate a connection between sleep disturbances and enhanced systemic and cellular inflammation, including increased pro-inflammatory transcription in circulating leukocytes (2, 7, 8). In cancer patients, studies on the connection between immune activity and sleep disturbances are rare (2) and have produced heterogeneous results (9–11). For example, a study on women suffering from early-stage breast cancer who had recently finished primary treatment revealed that neither subjective sleep quality (SQ) nor sleep disturbances were significantly associated with serum levels of soluble tumor necrosis factor-α receptor II, interleukin-1 receptor antagonist, or C-reactive protein (10).
Heterogeneous findings in the research area of sleep–immune interactions in cancer may be related to the diversity of the studied cancer types and/or treatment-related characteristics (e.g., cancer stage) (12). However, inconsistent findings may also be attributable to the neglect of dynamic aspects related to the topic under study (13). Sleep–immune mechanisms are tightly bound to the circadian system that is composed of a network of transcriptional and/or posttranslational feedback loops (14, 15). This system, in turn, closely interwoven with cellular functions, affects both adaptive and innate immune function (16). Immunological processes, on the other hand, affect sleep factors via cytokines that act on neurons in sleep-involved brain regions (7, 17). Thus, the topic of sleep–immune interrelationships refers to a highly complex phenomenon. Approaching such complexity with inappropriate research designs and methods may result in heterogeneous findings (18).
To investigate the complex psychobiological aspects of sleep–immune interrelationships, e.g., temporal delays, response patterns, and directions of effects between variables, we suggest an idiographic and ecologically valid research approach based on qualitative methods and time-series analysis (18). In general, idiographic research approaches explore data at the individual level and thus overcome methodological problems related to intraindividual variability (e.g., day-to-day fluctuations) and interindividual heterogeneity (e.g., interindividual differences in stress-related cortisol responses) (19, 20). Indeed, when studying sleep–immune processes at the aggregating-nomothetic (group) level, these problems are inherent. Time-series analysis is a statistical tool that is often applied in idiographic research (19) and is capable of detecting temporal and directional relationships in complex processes (21). Approaches applying time-series analysis differ from conventional nomothetic approaches, e.g., by allowing the filtering of serial dependencies, which are prominent in circadian rhythms (21). Serial dependencies are a significant source of variance and may lead to false-positive or false-negative correlations when not statistically accounted for (21, 22).
There are various approaches in time-series analysis to assess and incorporate such autocorrelative effects within statistical models (22). The technique used in this study, centered moving average (CMA) smoothing, strips away random fluctuations and highlights the underlying trend in a time series (23). It can be applied to short time series (24).
Time-series analysis is part of our “integrative single-case design,” a study design that investigates stress system dynamics in a single individual under highly preserved everyday-life conditions (13, 18, 25, 26). In a previous article on the integrative single-case study presented here (same patient), we provided first evidence of real-life bidirectional relations between fatigue, mood, and urinary neopterin concentrations (27). Statistical analysis of 55 consecutive 12-h measurements (28 days) revealed that increases in urinary neopterin levels significantly preceded increases in fatigue intensity with a temporal delay of 60–72 h. Furthermore, increases in positive mood co-occurred with neopterin level increases and preceded decreases in neopterin concentrations with a delay of 132–144 h. Neopterin is released by macrophages upon interferon gamma (IFN-γ) activation and indicates cellular immune system activation and oxidative stress (28).
This article now focuses on the sleep-related time series and investigates the reciprocal relationship between subjective sleep and neopterin release. Subjective sleep estimates appear to be highly relevant to immunological changes (29, 30).
Materials and Methods
Study Design
This integrative single-case study is part of a larger project on the influence of emotionally meaningful incidents on stress system dynamics in breast cancer survivors. The applied study design is described in detail elsewhere (13, 18, 25, 26). Shortly before the study began, the patient was examined medically and psychologically to check for inclusion and exclusion criteria (27). During the study period of 28 days (from July 13th to August 9th, 2006), the patient collected her full urine output in 12-h intervals (from approximately 8:00 p.m. to 8:00 a.m. and from approximately 8:00 a.m. to 8:00 p.m.) to determine urinary neopterin and creatinine levels (total: 55 measurements). Moreover, the patient filled out various questionnaires at approximately 8:00 a.m. and again at approximately 8:00 p.m. to measure psycho-behavioral everyday-life variables such as emotional states, daily routine, and illness-related issues. The sleep questionnaire was answered by the patient every morning at approximately 8:00 a.m. and dealt with her sleep the preceding night. Once a week, the patient brought the frozen (−20°C) urine sample aliquots to the laboratory where they were stored at −70°C until analysis. During these clinical appointments, the general health status of the patient was monitored, and the patient was interviewed regarding psychosocial incidents of the previous week. For the purpose of this study, nighttime neopterin measurements and subjective sleep variables were used, each resulting in time series of 28 measurements.
The patient gave written informed consent to her participation and to the publication of data in accordance with the Declaration of Helsinki, and the Institutional Review Board of the Freiburg University approved the design.
Measurement of Continuous Sleep Variables
Continuous day-to-day sleep assessment was based on subjective sleep ratings. Specifically, each morning at approximately 8:00 a.m., the patient answered questions about her prior night’s sleep. These questions were based on the Deutsche Gesellschaft für Schlafforschung und Schlafmedizin (DGSM) morning protocols (31) and correspond to items from Anglophone sleep diaries, such as the Karolinska Sleep Diary (32) and the Consensus Sleep Diary (33). The open response questions of this sleep questionnaire were as follows:
1. When did you turn out the light last night?
2. How long did it take you to fall asleep (min)?
3. How many times did you wake up during the night?
4. How long, in total, were you awake during the night?
5. How many hours did you sleep last night?
6. When did you wake up this morning?
The sleep questionnaire, furthermore, included two items answered on a 5-point-Likert-scale:
7. Compared with your average quality of sleep, how did you sleep last night?
8. How restful and refreshing was your night’s sleep?
From this sleep questionnaire, the time-series variables “sleep quality” (SQ), “sleep recreational value” (SRV), “total sleep time” (TST), “total wake time” (TWT), and “awakenings during sleep period” (ADS) were formulated.
Measurement of Neopterin per Creatinine
The consecutive urinary neopterin and creatinine levels were determined using high-pressure liquid chromatography (HPLC) (Model ProStar 210 Solvent Delivery Modul; Varian Associates, Palo Alto, CA, USA), as previously described (34). All 55 12-h urinary aliquots were measured in one single run. For the purpose of this study, we only used those 28 urinary aliquots that corresponded to the 12-h intervals from approximately 8:00 p.m. to approximately 8:00 a.m. (nighttime samples). Urinary neopterin concentrations were expressed in micromolars per molar creatinine to compensate for variations in urine density. To check for possible measurement errors, urinary neopterin and creatinine concentrations were analyzed in five independent runs, using a new aliquot for each determination. These five independent urinary neopterin time series were averaged.
Statistical Analyses
Time series (n = 28) were analyzed using IBM SPSS Statistics 23. To determine if a change in one variable significantly preceded a change in another variable, and to identify temporal delays and directions of effects between urinary neopterin and sleep variables, cross-correlation function (CCF) was performed at lag 0 and at higher lags up to ±7. CCF was considered statistically significant when the p < 0.05 criterion was met.
Beforehand, time series were stationarized through differencing and logarithmizing whenever necessary. Whenever autocorrelations were significant, time series were pre-whitened by CMA smoothing to deal with serial dependencies. An initial span of four values was specified for computing averages. To produce non-missing values at the endpoints of the original time series, a second span of two values was defined (35). CMA smoothing residuals were identified by subtracting the smoothed series from the original series (35, 36). These residuals were then used for CCF calculations (37).
Results
Time-series data were based on 28 measurements (n = 28, no missing data). No signs of infection were observed during the study period. The patient under study took aspirin® (acetylsalicylic acid, two tablets, 500 mg each) once during this study’s observation period, in night 14.
When averaged over the whole study period, the patient’s SQ had a mean value of 2.1 ± 1.5 (range: 0–4) and SRV showed a mean level of 1.2 ± 1 (range: 0–3). TST averaged out at 7.4 ± 1.6 (range: 5.1–11.2) hours per night. The mean value of TWT accounted for 21.1 ± 18.4 (range: 0–80) minutes per night. On average, the patient woke up 1.4 ± 0.8 (range: 0–3) times during the night (ADS). Urinary neopterin showed a mean concentration of 172.9 ± 25.7 (range: 131.6–226) μmol/mol creatinine (Table 1).
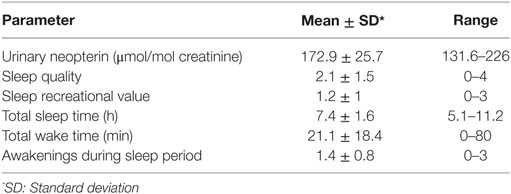
Table 1. Descriptive statistics of urinary neopterin concentrations and sleep variables (n = 28 consecutive measurements).
Figure 1 shows the time series of urinary neopterin per creatinine concentrations, which was stationary and needed no pre-whitening before cross-correlation with sleep variables. The raw time series of the five sleep variables under study are presented in Figures 2A–E. The time series of SQ, SRV, TST, and ADS were stationary. The time series of TWT was not stationary and thus had to be differenced. The time series of SQ, SRV, and TST showed no significant autocorrelations. Due to significant autocorrelation functions, TWT and ADS needed to be pre-whitened via CMA smoothing.
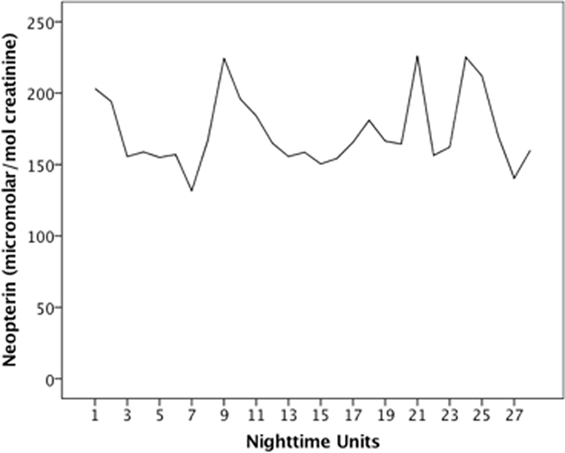
Figure 1. Time series of urinary neopterin. Time series covers night samples (8:00 p.m. to 8:00 a.m.) of a 28-day period during which the patient collected her full urine output in 12-h intervals.
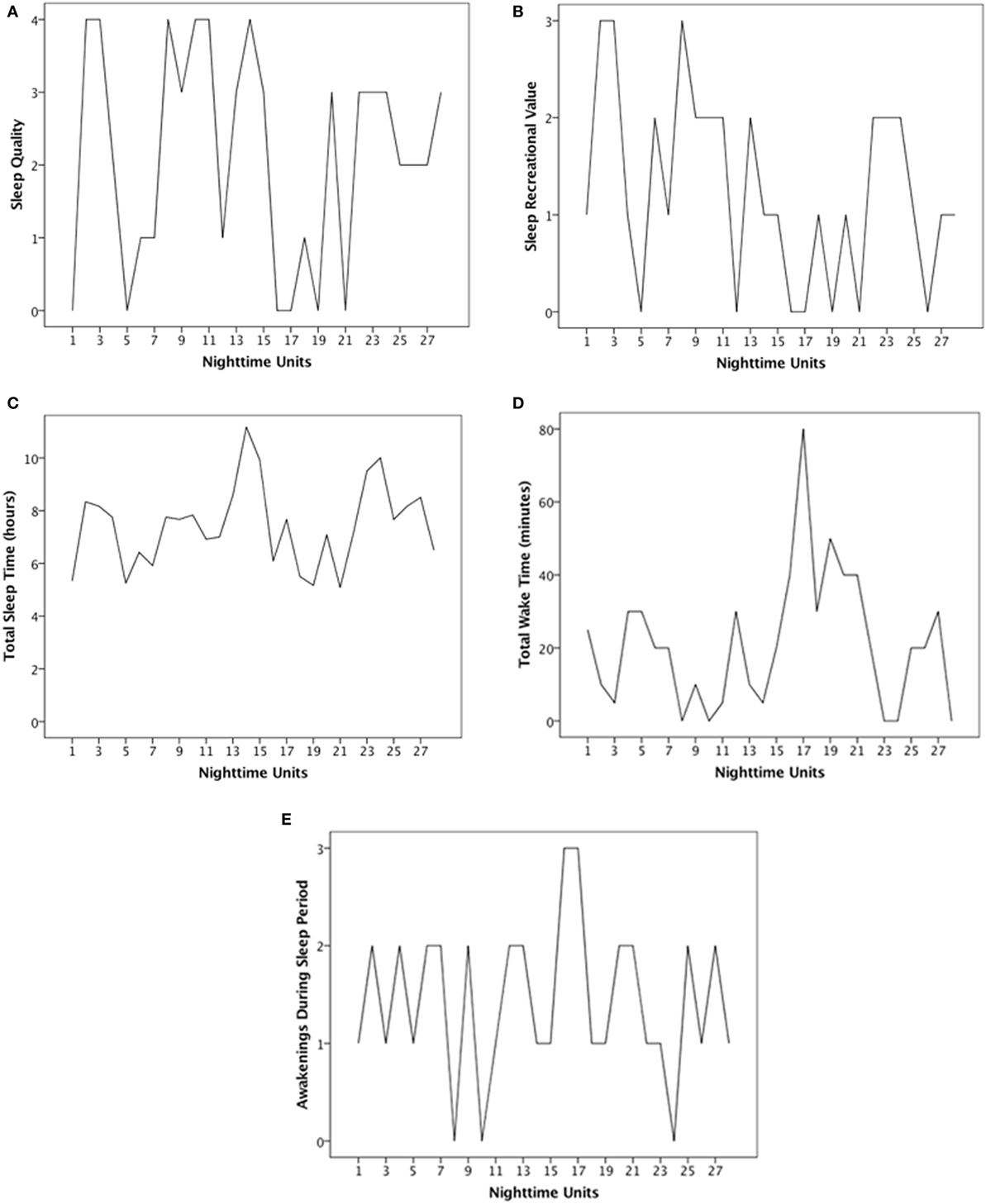
Figure 2. Time series of sleep variables. Time series display subjective ratings of (A) sleep quality (SQ), (B) sleep recreational value (SRV), (C) total sleep time (TST), (D) total wake time (TWT), and (E) awakenings during sleep period (ADS). Each time series covers a period of 28 days during which the patient answered questions about her prior night’s sleep each morning at approximately 8:00 a.m.
The CCF in Figure 3A shows that increased SQ preceded significant decreases in urinary neopterin concentrations by 96–120 h (lag 4: r = −0.411; p = 0.044). Cross-correlational analysis further revealed that increased SRV significantly preceded decreases in urinary neopterin levels with temporal delays of 96–120 h (lag 4: r = −0.472; p = 0.021) and 120–144 h (lag 5: r = −0.464; p = 0.026) (see Figure 3B). There was no statistically significant connection between TST and urinary neopterin levels (see Figure 3C). As presented in Figure 3D, increases in TWT significantly preceded increases in urinary neopterin concentrations with a temporal delay of 72–96 h (lag 3: r = 0.522; p = 0.009). No statistically significant association between ADS and urinary neopterin levels was found (see Figure 3E).
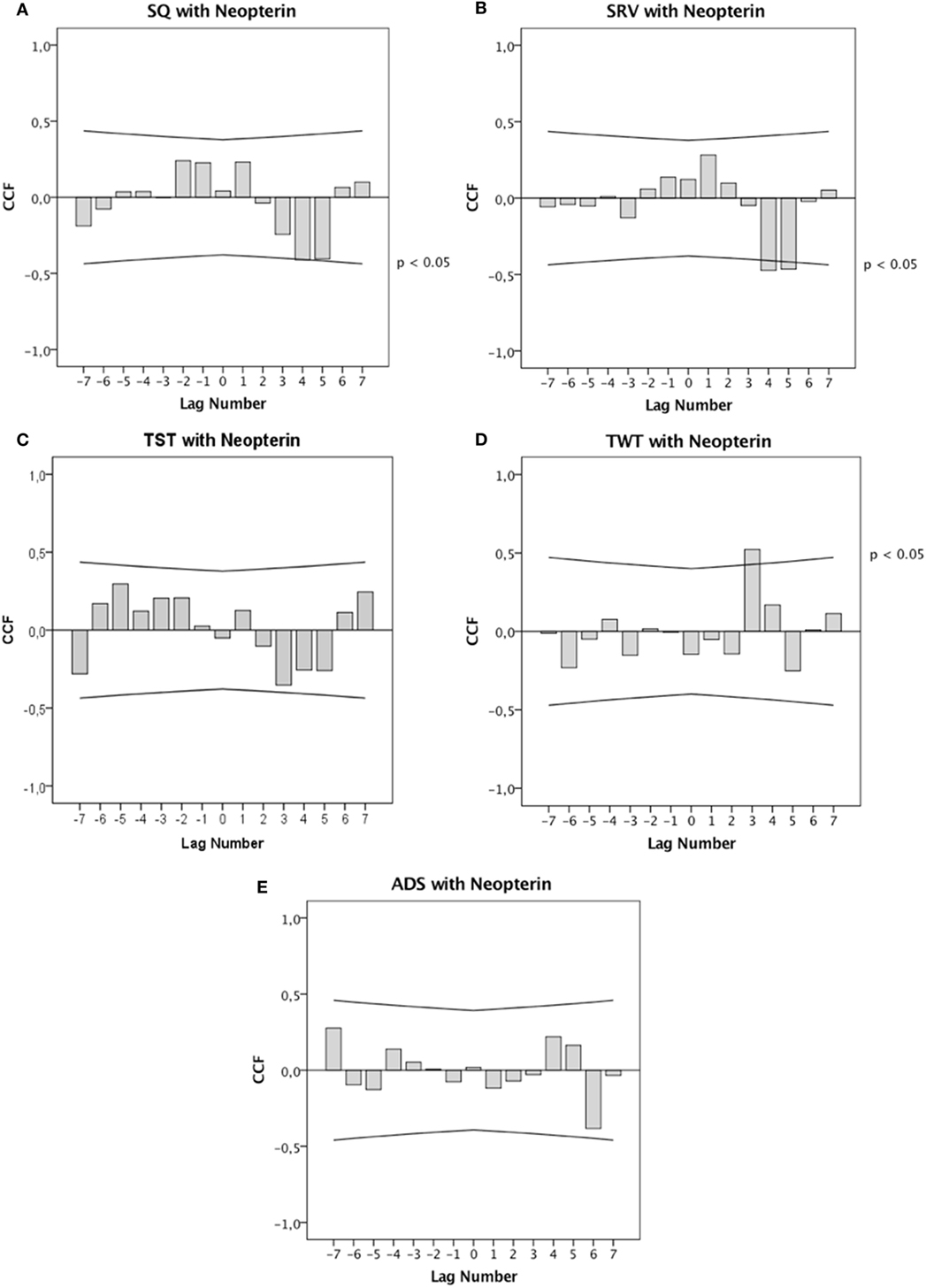
Figure 3. Cross-correlation functions (CCF). CCF between (A) sleep quality (SQ), (B) sleep recreational value (SRV), (C) total sleep time (TST), (D) total wake time (TWT), (E) awakenings during sleep period (ADS), and urinary neopterin levels. Each lag represents a time interval of 24 h. Cross-correlation coefficients (bars) reaching the upper or lower confidence limits (lines) are significant at p < 0.05.
Discussion
This integrative single-case study on a breast cancer survivor suffering from CaRF and depression shows that subjective sleep factors preceded cellular immune activity changes with temporal delays far exceeding time intervals known from the current sleep–immune literature. More specifically, elevations in SQ and SRV were followed by decreases in urinary neopterin concentrations after 96–120 and 120–144 h. Furthermore, elevations in TWT preceded increases in urinary neopterin concentrations after 72–96 h. Taken together, this study shows that decreases in urinary neopterin concentration occur whenever the patient describes her sleep as qualitatively good, restful, and refreshing, whereas urinary neopterin concentration increases were related to longer wake times, a negative sleep factor.
Neopterin is a marker of enhanced cellular immune activation and oxidative stress that has been associated with poorer health outcomes and lower survival rates in disorders such as breast cancer (28, 38). We thus suggest that urinary neopterin concentration decreases in the patient under study are related to beneficial health consequences, whereas neopterin increases represent deteriorating health effects.
In line with this suggestion is the finding that these effects of positive and negative sleep factors on urinary neopterin levels are comparable to the stress effects identified in a prior study on a patient suffering from systemic lupus erythematosus (SLE) (25, 26), a chronic inflammatory disease (39). Specifically, in that patient with SLE, the occurrence of positive, emotionally fulfilling incidents preceded ultimate urinary neopterin concentration decreases after 84 h (26), whereas the occurrence of negative, emotionally painful incidents preceded ultimate increases in urinary neopterin levels 60 h later (25).
By applying time-series analysis on high-frequent measurements within a time frame of 28 days, this study reveals that significant sleep-to-immune changes occurred after 72–144 h, with the majority of significant changes evolving after 96–120 h. At first sight, these time intervals are quite long when compared with clearly shorter temporal delays reported in laboratory studies [e.g., 1–9 h in Ref. (40)]. However, laboratory studies, as a matter of principle, cannot reveal such long time intervals due to an artificial predetermination of restricted time frames in experimental settings. The design of the current exploratory study, by contrast, allows the sleep–immune interplay, which depends on strong external zeitgebers (14) (e.g., light, social interactions, and physical activity) and higher order psychophysiological processing, to unfold dynamically under realistic everyday-life conditions (41). We suggest that such complex sleep–immune aspects are responsible for the long time intervals identified in this study.
As with experimental designs, quasi-experimental aggregating-nomothetic research approaches also fall short of the complexity of the topic under study. Assessment of single blood samples (29), data collection procedures with poor intra- and/or interindividual time coordination (42) and disregard of serial dependencies all exemplify reductionist tendencies characteristic of common data-averaging approaches (21). Another critical issue related to such approaches is that they do not allow testing without a priori methodological restriction (e.g., pre–post design) whether immunological processes affect sleep factors, whether sleep factors affect immunological processes, and whether reciprocal relationships exist (21). Indeed, directions of effects need to be seen as key aspects within the sleep–immune framework (7, 14, 17). Time-series data, on the other hand, are especially well suited to investigate the sequencing of change across time (21). For the patient of this study, the question of the directionality of effects can be answered: sleep typically preceded changes in neopterin.
As to the limitations of this study, first, our data are restricted to subjective sleep ratings, which increase the risk of response bias due to retrospective recall (43). In this regard, objective sleep assessment (e.g., actigraphy) would have revealed a more complete picture. A second limitation of the current investigation is the shortness of time-series data (n = 28), which prevented us from using more powerful modeling approaches such as autoregressive integrated moving average (ARIMA) modeling, where a minimum of 50 measurements is recommended for accurate estimation (44). Third, the findings may not be generalized yet due to the study’s exploratory character and the n = 1 design.
However, case numbers and sample size are not everything. Ecological validity is another important factor when findings from studies on human beings are to be generalized (45). The higher a study’s ecological validity, the higher its potential to generalize findings across settings and conditions (45). Our study, which was developed to achieve conditions as close to the subject’s natural environment as possible, displays particularly high ecological validity (18). In other words: observing “life as it is lived”—naturally, outside of the laboratory setting—is an essential strength of our study design.
Concluding Remarks
This study highlights the advantages of idiographic research approaches, which take a more “microscopic” perspective when studying the complex connections between sleep and immune processes under natural living conditions.
Ethics Statement
This study was carried out in accordance with the recommendations of the Institutional Review Board of the Freiburg University with written informed consent from the subject. The subject gave written informed consent in accordance with the Declaration of Helsinki. The protocol was approved by the Institutional Review Board of the Freiburg University.
Author Contributions
Substantial contributions to the conception or design of the work, the acquisition, analysis, or interpretation of data for the work; final approval of the version to be published; and agreement to be accountable for all aspects of the work in ensuring that questions related to the accuracy or integrity of any part of the work are appropriately investigated and resolved: MS, CB, KF, SP, HB, FO-P, DF, and CS. Drafting the work or revising it critically for important intellectual content: MS, SP, HB, FO-P, DF, and CS.
Conflict of Interest Statement
The authors declare that the research was conducted in the absence of any commercial or financial relationships that could be construed as a potential conflict of interest.
Acknowledgments
The authors are deeply grateful to the patient of this study for her participation.
Funding
The study has no funding. Magdalena Singer holds a PhD research grant from the Leopold-Franzens-Universität Innsbruck, Austria. There are no other relationships that influence the author’s work.
Abbreviations
ADS, awakenings during sleep period; ARIMA, autoregressive integrated moving average; CaRF, cancer-related fatigue; CCF, cross-correlation function; CES-D, Center for Epidemiologic Studies Depression Scale; CMA, centered moving average; CRP, C-reactive protein; DGSM, Deutsche Gesellschaft für Schlafforschung und Schlafmedizin; HPLC, high pressure liquid chromatography; IFN-γ, interferon gamma; IL-1RA, interleukin-1 receptor antagonist; SLE, systemic lupus erythematosus; SQ, sleep quality; SRV, sleep recreational value; sTNF-R, soluble tumor necrosis factor-α receptor; TST, total sleep time; TWT, total wake time.
References
1. Bower JE. Behavioral symptoms in breast cancer patients and survivors: fatigue, insomnia, depression, and cognitive disturbance. J Clin Oncol (2008) 26(5):768–77. doi:10.1200/JCO.2007.14.3248
2. Irwin MR, Olmstead RE, Ganz PA, Haque R. Sleep disturbance, inflammation and depression risk in cancer survivors. Brain Behav Immun (2013) 30:58–67. doi:10.1016/j.bbi.2012.05.002
3. Berger AM. Update on the state of the science: sleep-wake disturbances in adult patients with cancer. Oncol Nurs Forum (2009) 36(4):E165–77. doi:10.1188/09.onf.e165-e177
4. Berger AM, Parker KP, Young-McCaughan S, Mallory GA, Barsevick AM, Beck SL, et al. Sleep wake disturbances in people with cancer and their caregivers: state of the science. Oncol Nurs Forum (2005) 32(6):E98–126. doi:10.1188/05.onf.e98-e126
5. Savard J, Simard S, Blanchet J, Ivers H, Morin CM. Prevalence, clinical characteristics, and risk factors for insomnia in the context of breast cancer. Sleep (2001) 24(5):583–90. doi:10.1093/sleep/24.5.583
6. Mullan F. Seasons of survival: reflections of a physician with cancer. N Engl J Med (1985) 313(4):270–3. doi:10.1056/nejm198507253130421
7. Opp MR, Krueger JM. Sleep and immunity: a growing field with clinical impact. Brain Behav Immun (2015) 47:1–3. doi:10.1016/j.bbi.2015.03.011
8. Irwin MR, Olmstead R, Breen EC, Witarama T, Carrillo C, Sadeghi N, et al. Cognitive behavioral therapy and tai chi reverse cellular and genomic markers of inflammation in late-life insomnia: a randomized controlled trial. Biol Psychiatry (2015) 78(10):721–9. doi:10.1016/j.biopsych.2015.01.010
9. Liu L, Mills PJ, Rissling M, Fiorentino L, Natarajan L, Dimsdale JE, et al. Fatigue and sleep quality are associated with changes in inflammatory markers in breast cancer patients undergoing chemotherapy. Brain Behav Immun (2012) 26(5):706–13. doi:10.1016/j.bbi.2012.02.001
10. Bower JE, Ganz PA, Irwin MR, Kwan L, Breen EC, Cole SW. Inflammation and behavioral symptoms after breast cancer treatment: do fatigue, depression, and sleep disturbance share a common underlying mechanism? J Clin Oncol (2011) 29(26):3517–22. doi:10.1200/JCO.2011.36.1154
11. Sprod LK, Palesh OG, Janelsins MC, Peppone LJ, Heckler CE, Adams MJ, et al. Exercise, sleep quality, and mediators of sleep in breast and prostate cancer patients receiving radiation therapy. Community Oncol (2010) 7(10):463–71. doi:10.1016/S1548-5315(11)70427-2
12. Higgins JPT, Thompson SG, Deeks JJ, Altman DG. Measuring inconsistency in meta-analyses. BMJ (2003) 327(7414):557–60. doi:10.1136/bmj.327.7414.557
13. Schubert C, Haberkorn J, Ocana-Peinado F, König P, Sepp N, Schnapka-Kopf M, et al. Cause-effect relations between 55kD soluble TNF receptor concentrations and specific and unspecific symptoms in a patient with mild SLE disease activity: an exploratory time series analysis study. BMC Res Notes (2015) 8(1):465. doi:10.1186/s13104-015-1398-z
14. Lange T, Dimitrov S, Born J. Effects of sleep and circadian rhythm on the human immune system. Ann N Y Acad Sci (2010) 1193:48–59. doi:10.1111/j.1749-6632.2009.05300.x
15. Reddy AB, O’Neill JS. Healthy clocks, healthy body, healthy mind. Trends Cell Biol (2010) 20(1):36–44. doi:10.1016/j.tcb.2009.10.005
16. Besedovsky L, Lange T, Born J. Sleep and immune function. Pflugers Arch (2012) 463(1):121–37. doi:10.1007/s00424-011-1044-0
17. Bryant PA, Trinder J, Curtis N. Sick and tired: does sleep have a vital role in the immune system? Nat Rev Immunol (2004) 4(6):457–67. doi:10.1038/nri1369
18. Schubert C, Geser W, Noisternig B, Fuchs D, Welzenbach N, König P, et al. Stress system dynamics during “life as it is lived”: an integrative single-case study on a healthy woman. PLoS One (2012) 7:e29415. doi:10.1371/journal.pone.0029415
19. Van Ockenburg SL, Booij SH, Riese H, Rosmalen JG, Janssens KA. How to assess stress biomarkers for idiographic research? Psychoneuroendocrinology (2015) 62:189–99. doi:10.1016/j.psyneuen.2015.08.002
20. Rosmalen JG, Wenting AM, Roest AM, de Jonge P, Bos EH. Revealing causal heterogeneity using time series analysis of ambulatory assessments: application to the association between depression and physical activity after myocardial infarction. Psychosom Med (2012) 74(4):377–86. doi:10.1097/PSY.0b013e3182545d47
21. Borckardt JJ, Nash MR, Murphy MD, Moore M, Shaw D, O’Neil P. Clinical practice as natural laboratory for psychotherapy research: a guide to case-based time-series analysis. Am Psychol (2008) 63(2):77–95. doi:10.1037/0003-066x.63.2.77
22. Jebb AT, Tay L, Wang W, Huang Q. Time series analysis for psychological research: examining and forecasting change. Front Psychol (2015) 6:1–24. doi:10.3389/fpsyg.2015.00727
23. Schubert C, Neises M, Fritzsche K, Burbaum C, Geser W, Ocana-Peiñado FM, et al. Preliminary evidence on the direction of effects between day-to-day changes in cellular immune activation, fatigue and mood in a patient with prior breast cancer: a time-series analysis approach. Pteridines (2007) 18:139–47. doi:10.1515/pteridines.2007.18.1.139
25. Schubert C, Lampe A, Geser W, Noisternig B, Fuchs D, König P, et al. Daily psychosocial stressors and cyclic response patterns in urine cortisol and neopterin in a patient with systemic lupus erythematosus. Psychoneuroendocrinology (2003) 28(3):459–73. doi:10.1016/S0306-4530(02)00034-3
26. Schubert C, Noisternig B, Fuchs D, König P, Chamson E, Mittnik S, et al. Multifaceted effects of positive incidents on urine cortisol and urine neopterin dynamics in a patient with systemic lupus erythematosus. Stress Health (2006) 22:215–27. doi:10.1002/smi.1096
27. Haberkorn J, Burbaum C, Fritzsche K, Geser W, Fuchs D, Ocana-Peinado FM, et al. Day-to-day cause-effect relations between cellular immune activity, fatigue and mood in a patient with prior breast cancer and current cancer-related fatigue and depression. Psychoneuroendocrinology (2013) 38(10):2366–72. doi:10.1016/j.psyneuen.2013.03.001
28. Murr C, Widner B, Wirleitner B, Fuchs D. Neopterin as a marker for immune system activation. Curr Drug Metab (2002) 3(2):175–87. doi:10.2174/1389200024605082
29. Okun ML, Coussons-Read M, Hall M. Disturbed sleep is associated with increased C-reactive protein in young women. Brain Behav Immun (2009) 23(3):351–4. doi:10.1016/j.bbi.2008.10.008
30. Opp MR. Sleep and psychoneuroimmunology. Neurol Clin (2006) 24(3):493–506. doi:10.1016/j.ncl.2006.03.002
31. Hoffmann RM, Müller T, Hajak G, Cassel W. Abend-Morgenprotokolle in Schlafforschung und Schlafmedizin—Ein Standardinstrument für den deutschsprachigen Raum. Somnologie (1997) 1(3):103–9. doi:10.1007/s11818-997-0019-z
32. Akerstedt T, Hume K, Minors D, Waterhouse J. The subjective meaning of good sleep, an intraindividual approach using the Karolinska Sleep Diary. Percept Mot Skills (1994) 79:287–96. doi:10.2466/pms.1994.79.1.287
33. Carney CE, Buysse DJ, Ancoli-Israel S, Edinger JD, Krystal AD, Lichstein KL, et al. The consensus sleep diary: standardizing prospective sleep self-monitoring. Sleep (2012) 35(2):287–302. doi:10.5665/sleep.1642
34. Fuchs D, Weiss G, Reibnegger G, Wachter H. The role of neopterin as a monitor of cellular immune activation in transplantation, inflammatory, infectious, and malignant diseases. Crit Rev Clin Lab Sci (1992) 29(3–4):307–41. doi:10.3109/10408369209114604
36. Velleman P, Hoaglin D. Applications, Basics, and Computing of Exploratory Data Analysis. Boston: Duxbury Press (1981).
37. Box GEP, Jenkins GM. Time-Series Analysis: Forecasting and Control. San Francisco: Holden-Day (1976).
38. Murr C, Bergant A, Widschwendter M, Heim K, Schrocksnadel H, Fuchs D. Neopterin is an independent prognostic variable in females with breast cancer. Clin Chem (1999) 45(11):1998–2004.
39. Heim C, Ehlert U, Hellhammer DH. The potential role of hypocortisolism in the pathophysiology of stress-related bodily disorders. Psychoneuroendocrinology (2000) 25(1):1–35. doi:10.1016/S0306-4530(99)00035-9
40. Irwin MR, Wang M, Ribeiro D, Cho HJ, Olmstead R, Breen EC, et al. Sleep loss activates cellular inflammatory signaling. Biol Psychiatry (2008) 64(6):538–40. doi:10.1016/j.biopsych.2008.05.004
41. Brosschot JF, Gerin W, Thayer JF. The perseverative cognition hypothesis: a review of worry, prolonged stress-related physiological activation, and health. J Psychosom Res (2006) 60(2):113–24. doi:10.1016/j.jpsychores.2005.06.074
42. Friedman EM, Hayney MS, Love GD, Urry HL, Rosenkranz MA, Davidson RJ, et al. Social relationships, sleep quality, and interleukin-6 in aging women. Proc Natl Acad Sci U S A (2005) 102(51):18757–62. doi:10.1073/pnas.0509281102
43. Bolger N, Davis A, Rafaeli E. Diary methods: capturing life as it is lived. Annu Rev Psychol (2003) 54:579–616. doi:10.1146/annurev.psych.54.101601.145030
44. McCleary R, Hay RA, Meidinger EE, McDowall D. Applied Time Series Analysis for the Social Sciences. Beverly Hills, CA: SAGE Publications (1980).
Keywords: cancer, sleep, neopterin, time-series analysis, single-case design
Citation: Singer M, Burbaum C, Fritzsche K, Peterlini S, Bliem HR, Ocaña-Peinado FM, Fuchs D and Schubert C (2018) Subjective Positive and Negative Sleep Variables Differentially Affect Cellular Immune Activity in a Breast Cancer Survivor: A Time-series Analysis Approach. Front. Neurol. 8:693. doi: 10.3389/fneur.2017.00693
Received: 08 August 2017; Accepted: 04 December 2017;
Published: 09 January 2018
Edited by:
Daniel P. Cardinali, UCA Pontificia Universidad Católica Argentina, ArgentinaReviewed by:
Arturo Garay, Centro de Educación Médica e Investigaciones Clínicas Norberto Quirno, ArgentinaEmilio J. Sanchez-Barcelo, University of Cantabria, Spain
Pablo Torterolo, University of the Republic, Uruguay
Copyright: © 2018 Singer, Burbaum, Fritzsche, Peterlini, Bliem, Ocaña-Peinado, Fuchs and Schubert. This is an open-access article distributed under the terms of the Creative Commons Attribution License (CC BY). The use, distribution or reproduction in other forums is permitted, provided the original author(s) or licensor are credited and that the original publication in this journal is cited, in accordance with accepted academic practice. No use, distribution or reproduction is permitted which does not comply with these terms.
*Correspondence: Christian Schubert, Y2hyaXN0aWFuLnNjaHViZXJ0JiN4MDAwNDA7aS1tZWQuYWMuYXQ=