- 1Laboratory for Synthetic Biology, RIKEN Quantitative Biology Center, Suita, Osaka, Japan
- 2Department of Systems Pharmacology, Graduate School of Medicine, The University of Tokyo, Tokyo, Japan
A systems approach to studying biology uses a variety of mathematical, computational, and engineering tools to holistically understand and model properties of cells, tissues, and organisms. Building from early biochemical, genetic, and physiological studies, systems biology became established through the development of genome-wide methods, high-throughput procedures, modern computational processing power, and bioinformatics. Here, we highlight a variety of systems approaches to the study of biological rhythms that occur with a 24-h period—circadian rhythms. We review how systems methods have helped to elucidate complex behaviors of the circadian clock including temperature compensation, rhythmicity, and robustness. Finally, we explain the contribution of systems biology to the transcription–translation feedback loop and posttranslational oscillator models of circadian rhythms and describe new technologies and “–omics” approaches to understand circadian timekeeping and neurophysiology.
Systems Biology—A Brief History
In contrast to a reductionist approach, systems biology emphasizes the interaction of components rather than the components themselves: to see the forest for the trees. This holistic approach is not a modern idea, but can be traced as far back as the Greek Aristotle “…the totality is not, as it were, a mere heap, but the whole is something besides the parts…” In the modern era, Karl Ludwig von Bertalanffy is generally credited as one of the founders of general systems theory with his model of individual cell growth in the early 20th century (1). Later, the Dutch physicist Balthasar van der Pol working with electric circuits developed his eponymous equation to describe relaxation oscillations (2), which was used for theoretical models of neuronal systems (3, 4). In the 1950s, Alan Hodgkin and Andrew Huxley described the first mathematical model of an action potential propagating along a neuron, which famously predicted the existence of ion channels before their experimental discovery (5), and Alan Turing proposed a reaction–diffusion system in “The Chemical Basis of Morphogenesis” to explain how an initially homogenous system—the embryo—forms patterns through the action of morphogens (6).
These early systems models of cellular behavior were overshadowed by the excitement of the molecular biology revolution. Geneticists and biochemists learned to devise assays to measure the impact of single genes and single enzymes. In the 1970s, Ronald Konopka in Seymour Benzer’s lab used chemical mutagenesis to screen fruit flies for defects in their rhythmic emergence from the pupae state. He discovered three alleles of the Period gene, which is one of the earliest examples of a gene determining behavior in an organism (7). For the next 30 years, circadian biologists mostly pursued reductionist approaches similar to Konopka’s strategy to examine circadian behaviors in different organisms by knocking out single genes or isolating individual tissues.
The era of functional genomics and next-generation sequencing has begun to shift the balance back toward systems biology. In the following sections, we review the contributions of mathematical models, microarray technology, RNA sequencing, proteomics, and neurophysiological approaches to systematically dissect circadian behavior and uncover new modes of regulation (for an overview, see Figure 1).
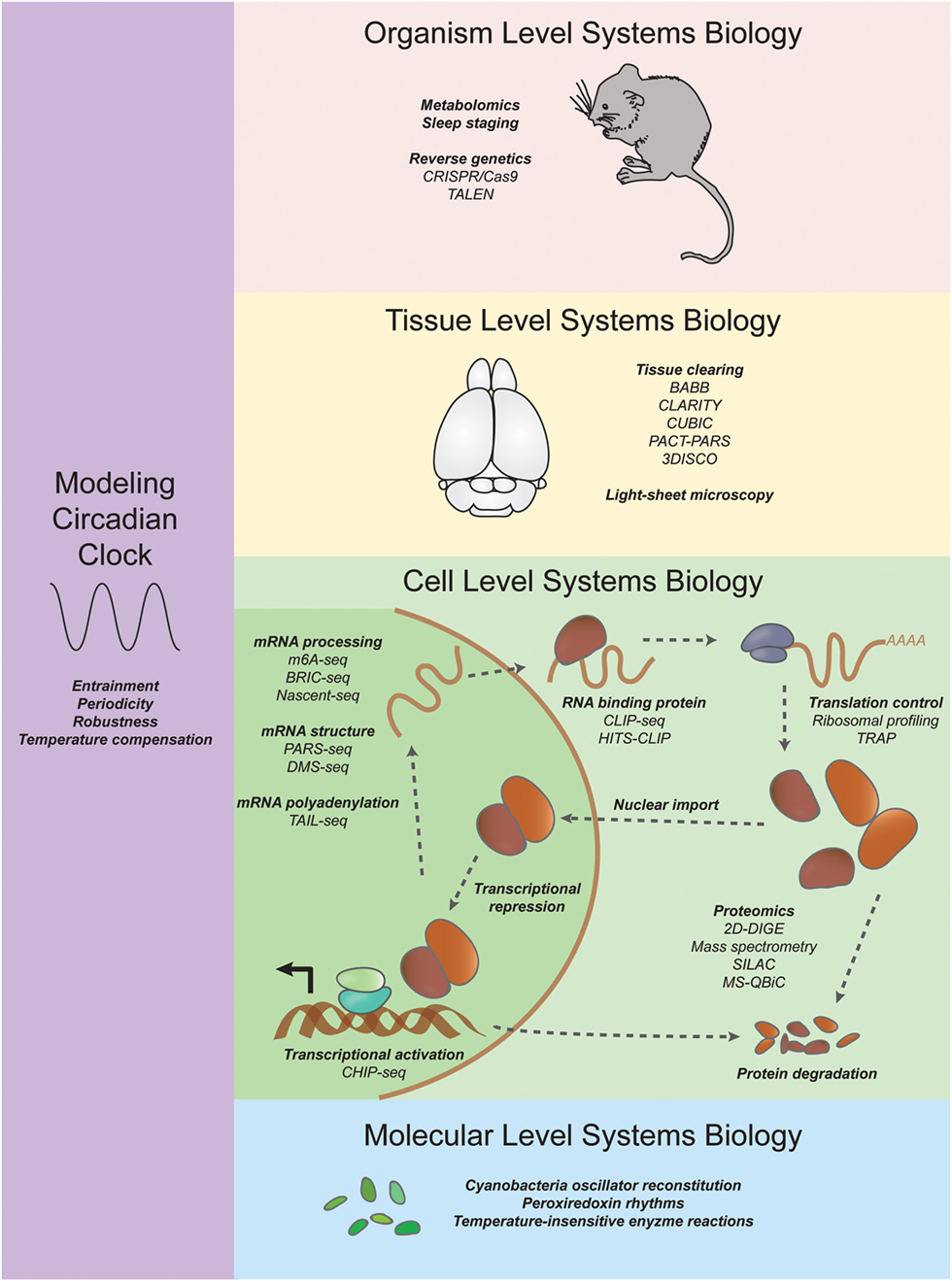
Figure 1. Systems approaches to studying circadian rhythms. On an organism level, researchers are using CRISPR/Cas9 and TALEN coupled with new sleep staging techniques to uncover mutations in genes that increase or decrease sleep. On a tissue level, new tissue clearing techniques such as CLARITY and CUBIC are enabling researchers to investigate the neuroanatomical basis of behavior (see Systems Neurophysiology). On a cell level, systems transcriptomics experiments have revealed not only rhythmic mRNA levels through microarrays and RNA sequencing but also other molecular details such as chromatin state, mRNA structure and modification, ribosome binding, and rhythmic protein abundance (see Systems Transcriptomics, Systems Proteomics and Metabolomics, and Systems Approaches to Study Translation Regulation in Circadian Rhythms). On a molecular level, reconstitution of a cyanobacteria posttranslational oscillator and the discovery of transcription/translation independent peroxiredoxin rhythms have expanded our understanding of circadian oscillations (see Periodicity and the Rise of the Posttranslation Circadian Oscillator). Systems modelers have discovered insights into constraints and parameters necessary for unique features of the circadian clock such as entrainment, periodicity, robustness, and temperature compensation (see Modeling the Systems Properties of Circadian Rhythms).
Modeling the Systems Properties of Circadian Rhythms
The circadian clock is an interconnected network—a network of small molecules and metabolites, a network of genes and proteins, and a network of cells, neurons, and tissues. At each level, the interacting network of components can create complex behaviors. These systems-level properties include three defining characteristics of circadian rhythms: (1) periodicity—rhythms are autonomous with a period that matches the daily 24-h rotation of the Earth, (2) entrainment—rhythms can be reset by environmental cues such as light, temperature, or food intake, and (3) temperature compensation—periodicity of rhythms persistent despite fluctuations over physiologically relevant temperature ranges.
Before genetics led to the identification of molecular components governing a transcription and translation feedback loop that underlies the mechanism of circadian oscillation in many organisms, theoretical studies sought to model how oscillation, periodicity, entrainment, and temperature compensation could arise. The first was Goodwin’s model of a molecular oscillator using negative feedback (8–10). Understanding the different types of behavior in networks have enabled mathematical biologists to make predictions about which biological processes affect circadian rhythm behavior such as period length and temperature compensation. For example, in a hypothetical biochemical network with negative feedback, there are necessary constraints on reaction rates for the generation of instability at steady state (11). Using this constraint and other ideas from signal processing in the Goodwin model for circadian oscillation, it could be shown that transcription and translation rate are not important for setting period length, but instead a critical feature is the degradation rate of the repressor (12). These studies highlight the fundamental contributions of systems modelers even without knowledge of the molecular network underpinning circadian rhythms.
Identification of the molecular components of circadian rhythms led to an explosion of models incorporating these proteins and functions. Goldbeter’s model used non-linearity of Hill-type equations in the Goodwin model when he reported the first model of circadian rhythms based on observations of PERIOD phosphorylation and degradation in Drosophila (13). Non-linearity in feedback repression could occur through cooperative binding of multiple repressors to a promoter or via repressive multisite phosphorylation of a transcriptional activator. Derivations of this type of model have been used to examine Drosophila (13–19), Neurospora (14, 16, 20, 21), and mammalian circadian rhythms (22–30). In the next subsections, we discuss how these and other models contributed to our understanding of the systems properties of circadian rhythms.
Periodicity and Design of the Transcription–Translation Feedback Loop
The period of a biological rhythm is tied to the 24-h rotational movement of the Earth. Organisms across different domains of life evolved timing mechanisms called biological clocks to coordinate function and behavior to specific times of the day (31). Each day environmental cues such as light and temperature reset your biological clock in a process called entrainment (32). Food can also entrain biological rhythms by affecting clock machinery in the liver (33, 34). Entrainment allows us to recover from the jet lag inducing effects of airplane travel by either advancing or delaying the phase of the circadian clock. Response to external cues is not instantaneous—timekeeping of the circadian clock persists, which is why we feel jet lagged in the first place.
Flexibility in period length was apparent from the earliest studies of mutant organisms (7, 35, 36). Systematic screening of chemical libraries also revealed chemical compounds that could alter period length by targeting specific clock proteins (24, 37–44). Pharmacological and/or genetic perturbation could extend the range of periods in the fibroblast from 27 to 54 h (41) and suprachiasmatic nucleus (SCN) from 17 to 42 h (45). Investigating why some mutant organisms have short or long periods revealed the molecular mechanisms of circadian rhythms and researchers could begin to test models by designing and manipulating components in the circuit. They were perhaps inspired by synthetic bacteria genetic circuits that recapitulate transcriptional oscillations (46) and bistable switches (47). For circadian rhythms, mathematical modeling guided construction of a synthetic 26-h oscillator based on siRNA-based silencing of a tetracycline-dependent transactivator (48). Construction of a mammalian promoter/enhancer database allowed researchers to identify high-scoring or low-scoring cis-elements and validate high- or low-amplitude expression, respectively, in cells (49), which enabled synthetic reconstruction of different circadian phases in cells by mixing combinations of promoter elements (50, 51). Researchers have also implemented artificial photic input pathways to clock cells to investigate singularity behavior, in which the circadian clock is reset after perturbations of different strengths and timing (52). More recently, researchers have succeeded in replacing the endogenous repressor in mice with a tunable one (53) and artificially manipulating the molecular circuitry of pacemaker cells in the brain (54, 55) to alter period length. These synthetic biology reconstruction experiments probe the sufficiency of circadian networks to generate oscillations and oscillations of different periods as well as test ideas about how network components interact and function within cells.
Periodicity and the Rise of the Posttranslation Circadian Oscillator
Scientists originally thought that a transcription–translation feedback network was required for 24-h rhythms. But then, a remarkable study was published. Working in cyanobacteria, Kondo and colleagues mixed a small number of cyanobacterial proteins KaiA, KaiB, and KaiC, and ATP in a test tube to produce rhythmic 24-h oscillations in KaiC protein phosphorylation (56). In a manner similar to simple chemical reaction–diffusion systems creating Turing patterns, 24-h periodicity could be established in the absence of a transcription–translation negative feedback loop architecture.
A few years later, it was discovered that an antioxidant enzyme called peroxiredoxin in cultured human red blood cells undergoes temperature-independent circadian cycles of hyperoxidation. Because red blood cells lack a nucleus and peroxiredoxin rhythms persist in the presence of transcription and translation inhibitors, these rhythms prove the existence of a non-transcriptional-based circadian oscillator in mammals (57) and was later found to be conserved in a wide range of species (58). In mice, rhythmic peroxiredoxin oxidation is thought to occur through hemoglobin-dependent H2O2 generation and proteasome degradation (59), but it remains unclear how rhythmic oxygen delivery occurs in isolated cells and how the rhythms of peroxiredoxin oxidation are temperature compensated. In the future, a more detailed understanding of the relationship between rhythmic peroxiredoxin oxidation and canonical circadian clocks is needed.
The reconstitution of a phosphorylation oscillator in cyanobacteria (56) prompted modelers and synthetic biologists to question what the minimal components are for a circadian oscillator. In cyanobacteria, biochemical studies have driven our understanding of the mechanism of the oscillator. KaiC was discovered to be both a kinase and a phosphatase (60–62). KaiC autophosphorylation is triggered by allosteric activation by KaiA (63, 64) and regulated through feedback inhibition by KaiB (60, 65). Importantly, a sequential ordering of phosphorylation at two sites on KaiC is necessary for oscillation (66, 67) and remarkably, when Kai protein complexes from different starting phases are mixed, the phosphorylation state of the population remains in synchrony (68).
Several models have been proposed to explain the mechanism of oscillation (69–71) and synchrony of the cyanobacteria oscillator on a population level (67, 72, 73). A central idea is that there is monomer shuffling between KaiC hexamers, which was proposed in mathematical models (72, 74) and by experiments from the Kondo laboratory (65, 68), and confirmed elsewhere by FRET experiments (75). Other models do not explicitly rely on monomer exchange for synchrony (67, 73), but rather synchrony arises as an emergent property of the system based on KaiA’s affinity for different phosphorylated forms of KaiC. Of course, concepts such as differential affinity and monomer exchange have been incorporated together into more sophisticated models of cyanobacteria rhythms (76, 77).
Studies in cyanobacteria provide a foundation to understand the requirements (ordered phosphorylation, synchrony, etc.) for a generic phosphorylation oscillator. Most models of non-circadian phosphorylation oscillators require additional mechanisms for rhythmicity such as protein synthesis and degradation (78) or allosteric feedback from substrate (79, 80). However, a theoretical study demonstrated that autonomous circadian oscillations are possible with a single substrate reversibly phosphorylated at only two sites (81) and suggested that a well-defined ordering of phosphorylation states and sequestering checkpoints for enzyme activity could be design principles for single-molecule oscillators for the circadian clock and potentially other cellular oscillators. The Jolley model (81) results in a substrate with four possible modification states similar to MAPK (82) and cyanobacteria models (67). While a general phosphorylation oscillator has not yet been built based on these models, the reconstruction of temporal (56) and spatial (83) oscillators from purified components provide inspiration for future work. Furthermore, the recently reported success in transplanting the circadian clock from cyanobacteria into the non-circadian bacterium Escherichia coli (84) implies some amount generality for the network and design principles upon which circadian rhythms lie.
Temperature Compensation
Insensitivity to temperature was originally identified as an essential characteristic of biological time-measuring systems in bees, flies, and marine organisms (85–88) and references therein. In particular, it was postulated that temperature independence was the result of a temperature compensation mechanism involving the opposing effects of enzyme activities in response to changes in temperature (87). Researchers began to identify genetic mutants with defects in temperature compensation in Neurospora (89, 90) and Drosophila (91, 92). In flies, repressor dimerization was thought to be involved in temperature compensation because loss of the repressor’s dimerization domain caused the period to strongly depend on temperature (91). Researchers incorporated these ideas into models of circadian rhythms by suggesting that nuclear import of the repressor decreases with temperature and repressor dimerization increases with temperature (93, 94). Other models emphasized the importance of degradation of the repressor (95, 96) and other parameters needed for temperature compensation (97). The conceptual point of these models is that for circadian rhythms to be temperature compensated, some biochemical reactions accelerate circadian oscillations, while other biochemical reactions decelerate circadian oscillations. The balance model supposes that the former acceleration reactions are less sensitive to temperature, whereas the latter deceleration reactions are more sensitive to temperature. A molecular basis for this type of temperature compensation was proposed in plants (98) and also formulated mathematically as a balance equation (99) to explain how Neurospora repressor stability decreases with an increase in temperature (95, 100), which is ultimately caused by phosphorylation-dependent degradation from a kinase (101).
In 1968, Pittendrigh and colleagues argued against a balancing model in which temperature shortens a reaction in the first half of a circadian cycle while simultaneously lengthening a reaction in the second half of the cycle in their experiments with Drosophila (102). They used short light pulses to shift the phase of Drosophila pupae at different temperatures and showed that the period and wave form of the phase response curve changes only a little bit with temperature. They proposed a model where circadian output from a temperature-dependent oscillation is subjected to feedback inhibition from another temperature-dependent reaction (102, 103). These early studies suggested a model in which the enzymatic reactions that comprise the clock are temperature compensated. However, the idea of a temperature-compensated enzyme is counterintuitive because most chemical processes are temperature dependent. In cyanobacteria, the kinetic profile of the phosphorylation to dephosphorylation ratio is temperature compensated in vitro (56, 104). This was the first indication that temperature compensation could occur through the enzymes themselves as opposed to compensation that occurs through competing biochemical reactions.
The canonical transcription–translation feedback loop underlying circadian rhythms in eukaryotes may also be affected by temperature-insensitive enzymatic reactions. In eukaryotes, it was first discovered in mammals that the phosphorylation-dependent degradation rate of the repressor is temperature insensitive in cells, and temperature-insensitive phosphorylation is preserved in vitro (41). This suggests that temperature-insensitive enzymatic reactions can influence the circadian transcription–translation network. In addition to component-level temperature compensation (41), detailed examination of the degradation of the repressor revealed three distinct stages of degradation that depend on when during the circadian cycle protein translation is arrested (105). The authors in this study suggested that temperature-insensitive and -sensitive phosphorylation at different sites of the repressor are responsible for temperature compensation. In the future, it will be particularly interesting to uncover the mechanisms and structural basis of temperature compensation in these individual reactions and to synthetically engineer temperature compensation in circadian clocks similar to synthetically temperature-compensated genetic networks in bacteria (106).
Robustness to Gene Dosage
Circadian rhythms are surprisingly robust to changes in gene dosage—there has been much discussion about why knockout of core clock genes only results in subtle period lengthening or shortening (107). There have been efforts to understand networks effects by systematically altering individual gene levels (108) or by globally altering transcription levels with drugs (109). Resistance to internal noise from the stochastic nature of biochemical networks in the cell is an essential property for a robust circadian clock network (110). Theoretical models suggested that intercellular coupling between individual oscillator cells is necessary for synchrony and noise resistance (111). Indeed, dissociated SCN neurons and isolated cells from tissues such as lung and liver are arrhythmic compared to intact tissues with altered rhythmicity (112, 113). Robustness is also ensured by interlocking-feedback loops at the genetic circuit level, for review, see Ref. (114), and has been featured in models of circadian rhythms from different organisms (17, 115–118). In mammals, genetic (119–122) and pharmacological (38, 44) perturbation of the secondary feedback loop showed that it primarily served as a stabilizing mechanism.
Modeling approaches have revealed that activator and repressor complex formation are necessary for noise resistance (123) and that a 1:1 stoichiometric balance of repressors binding activators rather than binding DNA is important for robust circadian timekeeping (124). Experiments in mammals seem to support these models because rhythm generation in mouse embryonic fibroblasts can be abolished by constitutive expression of the mammalian repressor (125) or by artificially altering the stoichiometry between activators and repressors (126). Indeed, the natural stoichiometry between activators and repressors in a mouse liver is close to 1:1 as measured by western blotting (127) and mass spectrometry (128).
The difference in repression mechanisms—Hill-type non-linearity from models based on the Goodwin oscillator or protein-based sequestration leads to subtle differences in the activity of the activator in circadian models as the concentration of the repressor increases. For Hill-type models, there is an all-or-none switch that occurs when multisite phosphorylation or cooperative binding reaches some critical level. The activator is like a light bulb that is on until it suddenly gets switched off. For protein-sequestration models, the activity of the activator linearly decreases as a function of the molar ratio between activator and repressor, which is like a light bulb slowly turned down by a dimmer. These differences can affect the synchronized period between coupled heterogeneous oscillators compared to the mean period of uncoupled oscillators (129). Importantly, understanding the differences in repression mechanisms for coupled oscillators can lead to testable predictions on how clock components interact with other proteins, such as regulation of the tumor antigen p53 (130).
Systems Transcriptomics
Identification of the Components of the Circadian Clock
On a tissue level, the central clock in mammals is located in a structure of the brain called the SCN. Ganglion cells in the retina detect light signals through a photopigment called melanopsin and relay this information to the SCN. SCN neurons project to different regions of the brain and synchronize biological clocks in peripheral tissues by secretion of hormones as previously reviewed (131, 132). However, most tissues in an organism have the core transcriptional architecture for circadian rhythmicity including liver, lung, and muscles (133) as well as cultured cells (134–136).
The genetic network for circadian rhythms is based on delayed feedback repression of transcription. Briefly, a CLOCK:BMAL1 heterodimer activates transcription at promoter elements called E-boxes. A protein called PERIOD (PER) heterodimerizes with another protein CRYPTOCHROME (CRY) and translocates to the nucleus where it represses transcription of the Period gene and other genes that activate Period transcription, reviewed elsewhere extensively (137–140). Several components of the core transcriptional network were identified in forward-genetics screens (i.e., random mutation of an organism’s genome and searching for mutants with abnormal rhythms) including Period (7) and Timeless (141) in Drosophila, Frequency (35) in Neurospora, and Clock (142, 143) in mice.
Systems approaches have been successful in identifying other core clock components such as Bmal, which was identified using an iterative search for other bHLH proteins (144, 145). Genomics-based strategies helped to identify activators of Bmal such as Rora (122) and Nr1d1 (121), and functional genomics strategies in Drosophila revealed Clockwork Orange (146–148) as a homolog of the mammalian Dec1 and Dec2 (149).
Systems Experiments to Study the Transcriptome
Some of the earliest systems approaches to study circadian rhythms were to simply analyze all the mRNA in a tissue or organism to determine which mRNAs had cyclic expression. These studies used microarrays to identify cycling mRNAs in Drosophila (150, 151), in the mouse liver, heart, and SCN (152–155), rat pineal gland (156), isolated fibroblasts (157, 158), and in plants (159). There was considerable tissue specificity in rhythmic genes because only approximately 10% of cycling genes were common to at least one other tissue (160). Additionally, there are approximately 100-fold fewer cycling transcripts in NIH3T3 and U2OS cell culture models compared to mice tissue (161). This study also revealed 12-h oscillatory transcripts in liver, heart, lungs, and other tissues, but not in cultured cells (161). These “harmonic” rhythms are perturbed by a disrupted circadian clock in the SCN (162). Rhythmicity of the core clock component PER2 in these tissues could be confirmed with luminescent reporter mice (163).
Recent studies have begun to use RNA sequencing to measure steady-state mRNA expression in tissues such as the mouse liver (164–166) or to identify transcription factor-binding sites using chromatin-immunoprecipitation coupled with RNA-sequencing (CHIP-seq) (164–170). Comparative genomic approaches revealed the importance of E-boxes, D-boxes (171), and RREs (155, 171) in timing circadian mRNA expression, which have allowed ensemble-based predictions of phase response from combinations of these elements (25).
Systems Experiments Analyzing Chromatin State
Next-generation sequencing experiments revealed both circadian initiation and recruitment of RNA polymerase II (RNAPII) to circadian promoters (164, 168) and concomitant circadian changes in chromatin state (164, 166, 168). In particular, H3K4me3 histone methylation have circadian oscillations that slightly lag RNAPII occupancy (168). Circadian regulation of chromatin state was first observed in an increase in phosphorylation of histone H3S10 in the SCN in response to light (172). Additionally, rhythmic acetylation of histone 3 was observed in the promoters of Per1, Per2, and Cry1 in mouse liver (173, 174). CLOCK itself has intrinsic histone acetylase activity (175) and is rhythmically recruited to circadian promoters (174, 176). CLOCK can acetylate other non-histone proteins including BMAL1, which promotes recruitment of CRY1 and thus BMAL1–CLOCK inactivation (177). SIRT1, a sirtuin histone deacetylase whose activity depends on the coenzyme nicotinamide adenine dinucleotide (NAD+), interacts with CLOCK and can deacetylate BMAL1 (174) and PER2 (178). SIRT1 also controls H3K4me3 methylation through circadian deadenylation of the histone methyltransferase mixed-lineage leukemia 1 (179). Circadian regulation results in cycles of NAD + biosynthesis (180), NAD + recycling (181), alters Clock and Bmal1 binding (182), and NAD redox rhythms have been observed directly in cells (183). Together, these studies suggest a direct link between metabolism and epigenetic regulation of circadian rhythms.
MicroRNAs (miRNAs) in Circadian Rhythms
In addition to discovering cycling transcripts, systems transcriptomics experiments have uncovered other cycling RNAs such as long non-coding RNAs (lncRNAs) and miRNAs. For example, CHIP-seq experiments revealed clock proteins such as Clock, Bmal1, and Nr1d1 binding at sites outside of canonical gene promoters (166, 167, 169, 170, 184, 185), which suggested circadian regulation of non-protein-coding transcripts. MiRNAs bind target mRNAs typically in 3′ untranslated regions (3′ UTRs) to inhibit translation and destabilize the mRNA, for review see Ref. (186–189). Microarray studies uncovered miRNA expression inversely correlated with circadian activators Clock and Bmal1 and positively correlated with circadian suppressors Per, Cry1, and Nr1d1 (190), and other miRNAs that have diurnal expression patterns (191). MiRNAs are regulated by circadian proteins such as CLOCK (170, 192, 193) and NR1D1 (194) and modulate the expression of circadian genes such as Bmal1 (195–198), Clock (193, 199, 200), the circadian polyA deadenylase Nocturnin (201), Per1 and Per2 (202–204), Clockwork Orange (205), Timeless in Drosophila (206), and Cry1 (207). Knockout of the core miRNA-processing machinery in mouse liver revealed that ~30% of the rhythmic transcriptome is posttranscriptionally modulated by miRNAs (208).
lncRNAs in Circadian Rhythms
In addition to miRNAs, next-generation sequencing experiments have revealed extensive transcription of lncRNAs (209, 210) and circadian expression of lncRNAs (166, 211, 212). An in depth study revealed differential expression of 112 lncRNAs in the rat pineal gland, and light expression at night could modulate the level of some of these lncRNAs (213). A study of mouse liver revealed 19 out of 123 lncRNAs detected with robust oscillations and detected antisense transcripts associated with Per2 (166). Antisense transcription of Per2 in mice liver has been reported by others (164, 165) and originally in the silk moth (214), but it remains unclear what the function of antisense Per2 is for circadian rhythms. In Neurospora, the antisense transcript of frequency (called Qrf—Frq, spelled backward) is important for entrainment to light, oscillates in a reciprocal pattern to Frq, and promotes Frq gene silencing via heterochromatin formation (215–217). Deletion of a lncRNA associated with Prader–Willi syndrome in mice results in increased energy expenditure and altered expression of circadian genes such as Clock, Cry, and Per (218). Additionally, a lncRNA highly upregulated in liver perturbs the expression levels of Clock, Cry, and Per in hepatoma cells (219). Together, these studies suggest a role for non-protein-coding transcripts in the regulation of circadian rhythms.
Posttranscriptional Regulation of Circadian Rhythms
Next-generation sequencing studies have also examined to what extent rhythmic steady-state mRNA transcripts result from de novo rhythmic transcription versus rhythms via posttranscriptional regulation. By analyzing expression of introns as an indicator of pre-mRNA levels, a study by Koike et al. determined that the majority circadian mRNAs do not undergo rhythmic transcription (164). Another method to directly assess de novo transcription called Nascent-seq confirmed this result and further showed that many mRNAs with de novo rhythmic transcription do not have rhythms in steady levels of mRNA (165). A similar nascent-seq study in Drosophila also revealed a considerable posttranscriptional contribution to cycling mRNA amplitudes (220).
There are a variety of mechanisms for posttranscriptional regulation of circadian rhythms including splicing, mRNA export, polyadenylation, mRNA stability, methylation, and regulated translation—for review, see Ref. (221). The first indication of posttranscriptional regulation of circadian rhythms was that stability of Drosophila Per mRNA oscillates (222), which was also later observed in mammals (223). Posttranscriptional regulators such as LARK bind to the 3′ UTR of Per1 mRNA to enhance PER1 translation (224, 225). LARK also promotes alternative translation of the casein kinase homolog Doubletime in Drosophila (226). Researchers have uncovered other proteins that regulate translation of clock components. For example, the heterogenous nuclear ribonucleoprotein Q (hnRNP Q) modulates translation of Nr1d1, Per1, Per3, Cry1, and the rate-limiting enzyme in melatonin synthesis AANAT (227–232). Cry1 mRNA stability is also regulated by AU-rich element RNA-binding protein (AUF1) also known as hnRNP D (233, 234), and Per2 mRNA stability was found to be modulated by polypyrimidine tract-binding protein also known as hnRNP I (235).
mRNA PolyA Tail Length and Circadian Rhythms
Other mRNA processing mechanims may also posttranscriptionally regulate circadian rhythms. The 3′ end of newly transcribed pre-mRNA in the nucleus is cleaved and a polyA tail is added at one of the several possible sites (236). Deadenylation of this polyA tail in the cytoplasm by enzymes such as the poly(A)-specific ribonuclease and the Ccr4-Not complex can shorten tail length and accelerate mRNA degradation (237, 238). Daily variation in polyA tail length was first observed for vasopressin mRNA in the SCN (239). In Xenopus, another deadenylase called Nocturnin was discovered in a screen to detect rhythmically expressed mRNAs in retinal photoreceptors (240, 241) and was later shown to be expressed in multiple mouse tissues (242). Nocturnin is one of the few mRNAs that remain rhythmic after the liver clock is conditionally inactivated by drug-mediated Bmal1 expression (243) and can be posttranscriptionally regulated by miR-122 (201). Mice lacking Nocturnin do not have any obvious circadian behavior deficiencies, but are resistant to diet-induced obesity (244). However, in Drosophila, loss of Nocturnin results in abnormal behavior rhythms in constant light (245). A microarray method to measure polyA tail length suggested that rhythmic nuclear adenylation is coupled to rhythmic transcription and that rhythmicity in polyA tail length is related to rhythmic protein expression (246). These studies suggest that posttranscriptional regulation by deadenylation may be important for proper circadian rhythms and that next-generation sequencing techniques such as polyA tail profiling (247, 248) will be critical for fully understanding the contribution of polyA tail length to circadian rhythms.
Systems Experiments to Measure mRNA Modification, Structure, and RNA-Binding Proteins
Besides polyadenylation, mRNA processing by other mechanisms may contribute to circadian rhythms. A recent study showed that reduction of Mettl3, an m6A mRNA methylase involved in mRNA processing and nuclear export, reduces m6A methylation of circadian transcripts and extends period (249). Next-generation sequencing studies of m6A methylation may reveal other contexts in which methylation of mRNA is important for circadian rhythms (250). In addition, other RNA-sequencing techniques to probe RNA secondary structure such as dimethyl sulfate sequencing—DMS-seq and parallel analysis of RNA structure—PARS-seq (251, 252), BRIC-seq for mRNA stability (253, 254), and various methods to analyze RNA-binding sites of specific RNA-binding proteins such as CLIP, CLIP-seq, HITS-CLIP, iCLIP, and PAR-CLIP (255–260) will be critical for understanding how mRNA processing is involved in circadian rhythms. For example, CLIP-seq of mRNAs bound to cold-inducible binding protein, which is required for high-amplitude circadian gene expression, revealed binding to Clock and other circadian transcripts (261).
Systems Proteomics and Metabolomics
Circadian Proteomics
Researchers are beginning to use systems approaches to study the circadian proteome and metabolome. Using two-dimensional difference gel electrophoresis (2D-DIGE), Reddy and colleagues revealed that approximately 20% of the soluble proteins in the mouse liver oscillate. Surprisingly, for many rhythmic proteins, the corresponding mRNA was not rhythmic, which suggests translational and posttranslational control of protein rhythms (262). 2D-DIGE has also been used to investigate circadian differences in the mouse retina (263) and day and night differences in the mouse heart (264). In addition to mice, 2D gel-based mass spectrometry has been used to investigate chronological changes in eukaryotic algae (265, 266) and in plants (267, 268).
Other groups have employed stable isotope labeling by amino acids in cell culture (SILAC) to compare two groups of samples—one mixed with “heavy” amino acids and one mixed with “light” amino acids based on the composition of different element isotopes (269). SILAC-based quantitative mass spectrometry has been used to uncover cycling proteins in the mouse liver (270, 271) and SCN (272). Traditional SILAC approaches use chemical synthesis of peptides with isotopically labeled amino acids (269, 273) or gene expression systems in E. coli (274, 275). However, cell-free protein synthesis systems are potentially a more cost-effect tool to express isotope-labeled peptides because the volume of the reaction is much lower and purification is easier because there is no need for culturing, harvesting, and disrupting cells (275–278). Recently, a cell-free protein synthesis system called the PURE system (279) coupled with high-resolution mass spectrometry in a workflow called MS-QBiC was used to quantify 20 selected circadian clock proteins over a 24-h time series (128). This study estimated the absolute number of protein molecules for core clock components per cell and the delay between steady-state levels of mRNA (measured by qPCR) and protein copy number (128).
In addition to SILAC, label-free approaches such as MaxLFQ (280) have been used to quantify proteins in mouse skeletal muscle (281). Mass spectrometry has been used to examine the global proteome in cyanobacteria (282). Mass spectrometry has also been used to analyze the global phosphoproteome and revealed ~5,000 phosphosites that significantly oscillate in the mouse liver (283) and ~3,000 phosphosites in Arabidopsis (284). Given the widespread discrepancies between transcript and protein rhythmicity in a number of organisms, in the future, it will be useful to understand the role of translation and posttranslational regulation as well as cycling protein modification states (e.g., phosphorylation) to circadian networks.
Circadian Metabolomics
Researchers have looked at rhythmic metabolites in humans (285–288) and in mice (289–292) and have shown that circadian proteins directly regulate metabolism (44, 184). Researchers have also used comprehensive metabolite profiling to analyze diet effects in mice (293–295) and the effects of sleep loss in humans (296–299). Computational databases have been developed to compare published transcriptomes, proteomes, and metabolomes (292). Metabolic profiling is still quite noisy compared to transcriptome data at least for identifying tissue-specific signatures (300), and many challenges remain including identification of unknown metabolites, standardization of data repositories and reporting methods, and integration with other types of data. Researchers are beginning to use metabolic profiling over larger time courses and with higher resolution in cell culture lines (301). In the future, coupling these methods with gene knockout or knockdown of core clock components will enable researchers to identify connections between circadian rhythms and metabolism. For example, are there harmonics in metabolite rhythms (i.e., multiples of a 24-h rhythm like 8- and 12-h rhythms) similar to the harmonics of mRNA rhythms (161, 162), and would these rhythms be influenced by circadian genes?
One benefit of systems studies is the development of a molecular timetable to detect an individual’s body time based on a single time point assay. Molecular timetables have been developed with mice transcriptome data (212, 302) and applied to mice (291) and human (288) metabolite data, proteomic data (128), and even human breath (303). In theory, metabolite timetables could enable researchers to hone chronotherapeutic strategies for clinical conditions. However, despite the strong evidence that circadian timing effects xenobiotic metabolism, bioavailability, and drug efficacy and that many of the most successful drugs in the United States target proteins with circadian rhythm components (212), ongoing clinical trials rarely exploit time-of-day-dependent drug delivery (304).
Systems Approaches to Study Translation Regulation in Circadian Rhythms
Although 10% of genes are rhythmic in the liver (152), de novo transcription is only responsible for a small fraction of this rhythmicity (164). Thus, gene expression studies using microarrays and RNA-sequencing may not correlate with translation of the corresponding mRNA nor with protein abundance (305). In the mouse liver, systems studies of the proteome are unable to detect low-abundant components of the core circadian circuit (270, 271), unless special care is taken to examine a particular protein on a case-by-case basis (128). Thus, researchers have begun to use next-generation sequencing techniques of mRNA attached to mRNA in monosomes and polysomes (306, 307) and with affinity purification (308–310) as a proxy for protein abundance and to understand how translation regulation affects protein abundance.
It has been known for more than 50 years that perturbation of translation disrupts circadian rhythms (311). Until recently, there has been a shortage of good tools to measure translation directly. In 2009, Nicholas Ingolia in Jonathan Weissman’s lab developed a technique called ribosomal profiling, which uses RNA sequencing of ribosome-bound mRNA protected from RNAse degradation, to determine the location and abundance of ribosomes in the yeast transcriptome (312). Researchers have begun to use this method to study circadian rhythms in ribosomal occupancy (313, 314). These studies discovered a class of rhthmically translated mRNAs without corresponding steady-state mRNA rhythms (313, 314), which in the case of mouse liver may be a result of rhythmic ribosomal biogenesis (315). Researchers have previously observed that global translation is rhythmic in the mouse liver (316, 317), which is probably a result of activation of the TORC1 pathway (315, 318–320). Interestingly, diurnally regulated translation in the mouse liver is only moderately affected by knockout of the core clock component Bmal1 and many genes that contained 5′-terminal oligo pyrimidine tract or translation initiator of short 5′ untranslated region (5′-UTR) sequence have rhythms in ribosomal occupancy independent of trancriptional rhythms (321). These studies in addition to previous research (322–326) suggest that feeding rhythms can synchronize the liver in the absence of cues from neuronal pacemaker cells in the SCN.
The Janich and Jang studies (313, 314) also revealed widespread circadian translation of upstream open reading frames (uORFs) in 5′ UTRs. Translation of uORFs globally represses translation efficiency—a measure of the ratio of ribosomal occupancy, determined by ribosomal profiling, to steady-state mRNA, measured by RNA-sequencing (314). Interesting, many circadian mRNAs also have uORFs in their 5′ UTRs (Table 1), which may disrupt translation of the downstream coding sequence by ribosomal pausing on the mRNA, alternative translation, or other mechanisms (327). Ribosome pausing on uORFs may be alleviated by the action of the non-canonical initiation factors density regulated protein (DENR) and multiple copies in T-cell lymphoma (MCT-1), which act to promote translation reinitiation downstream of uORFs (328, 329). Depletion of DENR by shRNAs in NIH3T3 cells shortens the period by 1.5 h, which suggests that uORFs may be relevant for circadian function (314). In other biological contexts, repression of translation by uORFs can be regulated by trans-acting factors. For example, in Drosphila, the master switch gene Sex-lethal (Sxl) is important for sex, for review see Ref. (330–332). SXL-binding downstream of a short uORF on male-specific lethal (msl)-2 enhances translation repression by the uORF on downstream reading frame translation (333). During mitosis, one of the most translationally repressed mRNAs is early mitotic inhibitor 1 (Emi1) that inhibits the activity of the anaphase-promoting complex (334). Emi1 has multiple transcript isoforms and the isoform with several uORFs in the 5′ UTR is severely crippled for translation initiation in single-molecule reporter experiments (335). These studies suggest that uORF-mediated translational repression is important in a variety of biological functions and may have an unexplored role in circadian rhythms.
What is the consequence of disrupted translation for circadian rhythms? One clue came when researchers showed that codon usage affects circadian function in cyanobacteria (336), Neurospora (337), and Drosophila (338). While cyanobacteria with codon-optimized Kai genes have enhanced circadian rhythmicty at cooler temperatures, this modification impairs cell growth, which suggests that non-optimal translation could provide an adaptive response to changes in the environment (336). In Neurospora, codon optimization of Frq alters FRQ protein structure, which impairs circadian rhythms (337). Similarly, in Drosophila, codon optimization results in conformational changes of the Drosophila PER protein altering PER phosphorylation, stability, and impairs behavioral rhythms (338). Additionally, it is becoming clear that translation control is interlinked with both circadian rhythms and sleep disorders. For example, Ataxin2 functions as a critical translation activator of Per2 in flies (339, 340), and individuals with disease mutations in human Ataxin2 have disturbed rapid eye movement sleep (341, 342).
Systems Neurophysiology
Systems neurophysiologists are beginning to connect the circadian circuit to more complex outputs from the clock such as activity rhythms. Forward genetics in mice have already uncovered core components in the circadian network (142), and researchers have begun to use forward genetics for complex behavior such as sleep (343). On the other hand, the development of TALEN (344), Zinc-Finger Nucleases (345), and CRISPR/Cas9 (346, 347) gene knockout systems have accelerated the pace at which researchers can pursue reverse genetics in mice. In particular, CRISPR/Cas9 systems have been extensively modified to improve targeting efficiency and specificity (346–353). However, the need for invasive techniques such as electroencephalography and electromyography to characterize sleep hampers high-throughput phenotyping. To facilitate rapid phenotyping, researchers have developed a respiration-based, sleep staging system in combination with redundant CRISPR targeting to reveal new genes important for sleep regulation (354, 355). In particular, researchers generated and analyzed more than 21 different KO mice and discovered different ion channels that could increase or decrease sleep duration (355). These studies have revealed the genetic bases for behaviors such as sleep, but do not show how neural networks and structures in the brain are wired to carry out such behavior. In the past, researchers have used conventional histology and immunohistochemistry of sliced brain sections to reveal the when and where of gene function, but recent advances in tissue clearing have begun to enable direct imaging of intact organs (356).
Optical sectioning using light-sheet microscopy in combination with recently developed tissue-clearing techniques is a potent strategy to begin to explore the neuroanatomical basis of behavior (357–362). Image analysis algorithms, automated comparative analysis, and feature extraction will enable researchers to quickly test and analyze neural activity in different parts of the brain with different mutant mice and under a variety of experimental conditions. These approaches will be useful to determine what areas of the brain are affected by sleep/wake pharmaceutical reagents such as methamphetamine and to develop a whole-brain anatomical atlas to catalog and characterize every individual cell in the brain.
Conclusion
Systems experiments from modeling to metabolomics have significantly increased our understanding of circadian rhythms, but many challenges remain. For modeling, we still do not have a comprehensive understanding of temperature compensation nor the role individual enzymes have in temperature-independent and -dependent reactions. We do not understand the contribution of temperature-compensating reactions at the molecular, cell, tissue or organism level and how these temperature-compensating systems interface with one another. At an enzymatic level, we could learn much by designing and building de novo temperature-compensated reactions or by converting temperature-sensitive enzymes into temperature-compensating ones. There is also a need for understanding how recently discovered posttranslational oscillators such as the peroxiredoxin system interface with the genetic circadian circuit, and for an evolutionary investigation into how and why these distinct circadian timekeeping systems arose. Modeling is needed to make connections between different timekeeping systems, different organization levels of timekeeping from molecule to tissue, and between circadian rhythms and other rhythms such as the cell cycle.
For systems “–omics” researchers, there is a large variation in the rhythmicity of transcripts, metabolites, and proteomes detected even with similarly defined experimental systems. This may be in part due to how different algorithms detect rhythmicity (153, 363, 364), differences in sampling intervals (every 2, 3, 4, or 6 h), sampling duration, environmental conditions, and biological variability (365). As surveys of the circadian proteome increase, there has been an increasing realization of the widespread gap between transcript rhythms and protein levels. Posttranscriptional and posttranslational studies that examine mRNA structure and processing, translation, and protein modification will enhance our understanding of how transcriptional rhythms become protein rhythms, and how rhythms could evolve without genetic underpinnings.
For systems neurophysiologists, there is a pressing need to develop fast and reproducible assays that connect behavioral phenotypes to particular features and neurons in the brain and other tissues. Developments in computational processing power, data storage, and deep learning approaches will aid researchers in handling and analyzing the overwhelming amount of data generated by systems studies. Nevertheless, it will be important to validate findings with molecular techniques, case studies, and synthetic biology approaches to reconstitute behavior. Finally, can we translate this knowledge base to relevance in the clinic? It will be important to develop new assays and algorithms for body time estimation from samples at one or two time points. A combination of transcriptome, metabolome, and proteome timetables may further reduce the need for additional samples and increase accuracy of body time estimation. Integration of chronotherapeutics to clinical trial design and dosing protocols may enhance the success of drug candidates and perhaps lead to a reevaluation of the timing of drug delivery to achieve the greatest benefit to patients.
Author Contributions
Discussed and conceived of the direction of the review: AM and HU. Illustrated the table and figure and wrote the paper: AM.
Conflict of Interest Statement
The authors declare that the research was conducted in the absence of any commercial or financial relationships that could be construed as a potential conflict of interest.
Acknowledgments
We thank all lab members at The University of Tokyo and RIKEN QBiC, in particular, Koji Ode for critical reading and advice. This work was supported by a RIKEN Foreign Postdoctoral Fellowship (AM).
Funding
This work was supported by a grant from AMED-CREST (HU), Brain/MINDS (HU), Basic Science and Platform Technology Program for Innovative Biological Medicine (HU), a KAKENHI Grant-in-Aid from JSPS (Scientific Research S, 25221004, HU), an intramural Grant-in-Aid from RIKEN QBiC (HU), and a grant from the Takeda Science Foundation (HU).
References
1. Drack M, Apfalter W, Pouvreau D. On the making of a system theory of life: Paul A Weiss and Ludwig von Bertalanffy’s conceptual connection. Q Rev Biol (2007) 82:349–73. doi:10.1086/522810
2. van der Pol B. LXXXVIII. On “relaxation-oscillations”. Philos Mag (1926) 2:978–92. doi:10.1080/14786442608564127
3. Fitzhugh R. Impulses and physiological states in theoretical models of nerve membrane. Biophys J (1961) 1:445–66. doi:10.1016/S0006-3495(61)86902-6
4. Nagumo J, Arimoto S, Yoshizawa S. An active pulse transmission line simulating nerve axon. Proc IEEE Inst Electr Electron Eng (1962) 50:2061–70. doi:10.1109/JRPROC.1962.288235
5. Le Novère N. The long journey to a Systems Biology of neuronal function. BMC Syst Biol (2007) 1:28. doi:10.1186/1752-0509-1-28
6. Turing AM. The chemical basis of morphogenesis. Philos Trans R Soc Lond B Biol Sci (1952) 237:37–72. doi:10.1098/rstb.1952.0012
7. Konopka RJ, Benzer S. Clock mutants of Drosophila melanogaster. Proc Natl Acad Sci U S A (1971) 68:2112–6. doi:10.1073/pnas.68.9.2112
8. Gonze D, Abou-Jaoudé W. The Goodwin model: behind the Hill function. PLoS One (2013) 8:e69573. doi:10.1371/journal.pone.0069573
9. Goodwin BC. Oscillatory behavior in enzymatic control processes. Adv Enzyme Regul (1965) 3:425–38. doi:10.1016/0065-2571(65)90067-1
10. Woller A, Gonze D, Erneux T. The Goodwin model revisited: Hopf bifurcation, limit-cycle, and periodic entrainment. Phys Biol (2014) 11:045002. doi:10.1088/1478-3975/11/4/045002
11. Thron CD. The secant condition for instability in biochemical feedback control – I. The role of cooperativity and saturability. Bull Math Biol (1991) 53:383–401. doi:10.1016/S0092-8240(05)80394-5
12. Forger DB. Signal processing in cellular clocks. Proc Natl Acad Sci U S A (2011) 108:4281–5. doi:10.1073/pnas.1004720108
13. Goldbeter A. A model for circadian oscillations in the Drosophila period protein (PER). Proc Biol Sci (1995) 261:319–24. doi:10.1098/rspb.1995.0153
14. Gonze D, Leloup JC, Goldbeter A. Theoretical models for circadian rhythms in Neurospora and Drosophila. C R Acad Sci III (2000) 323:57–67. doi:10.1016/S0764-4469(00)00111-6
15. Leloup JC, Goldbeter A. A model for circadian rhythms in Drosophila incorporating the formation of a complex between the PER and TIM proteins. J Biol Rhythms (1998) 13:70–87. doi:10.1177/074873098128999934
16. Leloup JC, Gonze D, Goldbeter A. Limit cycle models for circadian rhythms based on transcriptional regulation in Drosophila and Neurospora. J Biol Rhythms (1999) 14:433–48. doi:10.1177/074873099129000948
17. Ruoff P, Christensen MK, Sharma VK. PER/TIM-mediated amplification, gene dosage effects and temperature compensation in an interlocking-feedback loop model of the Drosophila circadian clock. J Theor Biol (2005) 237:41–57. doi:10.1016/j.jtbi.2005.03.030
18. Ueda HR, Hagiwara M, Kitano H. Robust oscillations within the interlocked feedback model of Drosophila circadian rhythm. J Theor Biol (2001) 210:401–6. doi:10.1006/jtbi.2000.2226
19. Wang J, Zhou T. A computational model clarifies the roles of positive and negative feedback loops in the Drosophila circadian clock. Phys Lett A (2010) 374:2743–9. doi:10.1016/j.physleta.2010.04.059
20. Ruoff P, Vinsjevik M, Monnerjahn C, Rensing L. The Goodwin model: simulating the effect of light pulses on the circadian sporulation rhythm of Neurospora crassa. J Theor Biol (2001) 209:29–42. doi:10.1006/jtbi.2000.2239
21. Tseng Y-Y, Hunt SM, Heintzen C, Crosthwaite SK, Schwartz J-M. Comprehensive modelling of the Neurospora circadian clock and its temperature compensation. PLoS Comput Biol (2012) 8:e1002437. doi:10.1371/journal.pcbi.1002437
22. Becker-Weimann S, Wolf J, Herzel H, Kramer A. Modeling feedback loops of the Mammalian circadian oscillator. Biophys J (2004) 87:3023–34. doi:10.1529/biophysj.104.040824
23. Forger DB, Peskin CS. A detailed predictive model of the mammalian circadian clock. Proc Natl Acad Sci U S A (2003) 100:14806–11. doi:10.1073/pnas.2036281100
24. Hirota T, Lee JW, St John PC, Sawa M, Iwaisako K, Noguchi T, et al. Identification of small molecule activators of cryptochrome. Science (2012) 337:1094–7. doi:10.1126/science.1223710
25. Jolley CC, Ukai-Tadenuma M, Perrin D, Ueda HR. A mammalian circadian clock model incorporating daytime expression elements. Biophys J (2014) 107:1462–73. doi:10.1016/j.bpj.2014.07.022
26. Leloup J-C, Goldbeter A. Toward a detailed computational model for the mammalian circadian clock. Proc Natl Acad Sci U S A (2003) 100:7051–6. doi:10.1073/pnas.1132112100
27. Leloup J-C, Goldbeter A. Modelling the dual role of Per phosphorylation and its effect on the period and phase of the mammalian circadian clock. IET Syst Biol (2011) 5:44. doi:10.1049/iet-syb.2009.0068
28. Mirsky HP, Liu AC, Welsh DK, Kay SA, Doyle FJ. A model of the cell-autonomous mammalian circadian clock. Proc Natl Acad Sci U S A (2009) 106:11107–12. doi:10.1073/pnas.0904837106
29. Relógio A, Westermark PO, Wallach T, Schellenberg K, Kramer A, Herzel H. Tuning the mammalian circadian clock: robust synergy of two loops. PLoS Comput Biol (2011) 7:e1002309. doi:10.1371/journal.pcbi.1002309
30. Yan J, Shi G, Zhang Z, Wu X, Liu Z, Xing L, et al. An intensity ratio of interlocking loops determines circadian period length. Nucleic Acids Res (2014) 42:10278–87. doi:10.1093/nar/gku701
31. Dunlap JC. Molecular bases for circadian clocks. Cell (1999) 96:271–90. doi:10.1016/S0092-8674(00)80566-8
32. Roenneberg T, Daan S, Merrow M. The art of entrainment. J Biol Rhythms (2003) 18:183–94. doi:10.1177/0748730403018003001
33. Froy O. The relationship between nutrition and circadian rhythms in mammals. Front Neuroendocrinol (2007) 28:61–71. doi:10.1016/j.yfrne.2007.03.001
34. Panda S. Circadian physiology of metabolism. Science (2016) 354:1008–15. doi:10.1126/science.aah4967
35. Feldman JF, Hoyle MN. Isolation of circadian clock mutants of Neurospora crassa. Genetics (1973) 75:605–13.
36. Ralph MR, Menaker M. A mutation of the circadian system in golden hamsters. Science (1988) 241:1225–7. doi:10.1126/science.3413487
37. Chen Z, Yoo S-H, Park Y-S, Kim K-H, Wei S, Buhr E, et al. Identification of diverse modulators of central and peripheral circadian clocks by high-throughput chemical screening. Proc Natl Acad Sci U S A (2012) 109:101–6. doi:10.1073/pnas.1118034108
38. He B, Nohara K, Park N, Park Y-S, Guillory B, Zhao Z, et al. The small molecule nobiletin targets the molecular oscillator to enhance circadian rhythms and protect against metabolic syndrome. Cell Metab (2016) 23:610–21. doi:10.1016/j.cmet.2016.03.007
39. Hirota T, Lewis WG, Liu AC, Lee JW, Schultz PG, Kay SA. A chemical biology approach reveals period shortening of the mammalian circadian clock by specific inhibition of GSK-3beta. Proc Natl Acad Sci U S A (2008) 105:20746–51. doi:10.1073/pnas.0811410106
40. Hirota T, Lee JW, Lewis WG, Zhang EE, Breton G, Liu X, et al. High-throughput chemical screen identifies a novel potent modulator of cellular circadian rhythms and reveals CKIα as a clock regulatory kinase. PLoS Biol (2010) 8:e1000559. doi:10.1371/journal.pbio.1000559
41. Isojima Y, Nakajima M, Ukai H, Fujishima H, Yamada RG, Masumoto K, et al. CKIε/δ-dependent phosphorylation is a temperature-insensitive, period-determining process in the mammalian circadian clock. Proc Natl Acad Sci U S A (2009) 106(37):15744–9. doi:10.1073/pnas.0908733106
42. Lee JW, Hirota T, Peters EC, Garcia M, Gonzalez R, Cho CY, et al. A small molecule modulates circadian rhythms through phosphorylation of the period protein. Angew Chem Int Ed Engl (2011) 50:10608–11. doi:10.1002/anie.201103915
43. Meng Q-J, Maywood ES, Bechtold DA, Lu W-Q, Li J, Gibbs JE, et al. Entrainment of disrupted circadian behavior through inhibition of casein kinase 1 (CK1) enzymes. Proc Natl Acad Sci U S A (2010) 107:15240–5. doi:10.1073/pnas.1005101107
44. Solt LA, Wang Y, Banerjee S, Hughes T, Kojetin DJ, Lundasen T, et al. Regulation of circadian behaviour and metabolism by synthetic REV-ERB agonists. Nature (2012) 485:62–8. doi:10.1038/nature11030
45. Patton AP, Chesham JE, Hastings MH. Combined pharmacological and genetic manipulations unlock unprecedented temporal elasticity and reveal phase-specific modulation of the molecular circadian clock of the mouse suprachiasmatic nucleus. J Neurosci (2016) 36:9326–41. doi:10.1523/JNEUROSCI.0958-16.2016
46. Elowitz MB, Leibler S. A synthetic oscillatory network of transcriptional regulators. Nature (2000) 403:335–8. doi:10.1038/35002125
47. Gardner TS, Cantor CR, Collins JJ. Construction of a genetic toggle switch in Escherichia coli. Nature (2000) 403:339–42. doi:10.1038/35002131
48. Tigges M, Dénervaud N, Greber D, Stelling J, Fussenegger M. A synthetic low-frequency mammalian oscillator. Nucleic Acids Res (2010) 38:2702–11. doi:10.1093/nar/gkq121
49. Kumaki Y, Ukai-Tadenuma M, Uno KD, Nishio J, Masumoto K, Nagano M, et al. Analysis and synthesis of high-amplitude Cis-elements in the mammalian circadian clock. Proc Natl Acad Sci U S A (2008) 105:14946–51. doi:10.1073/pnas.0802636105
50. Ukai-Tadenuma M, Kasukawa T, Ueda HR. Proof-by-synthesis of the transcriptional logic of mammalian circadian clocks. Nat Cell Biol (2008) 10:1154–63. doi:10.1038/ncb1775
51. Ukai-Tadenuma M, Yamada RG, Xu H, Ripperger JA, Liu AC, Ueda HR. Delay in feedback repression by cryptochrome 1 is required for circadian clock function. Cell (2011) 144:268–81. doi:10.1016/j.cell.2010.12.019
52. Ukai H, Kobayashi TJ, Nagano M, Masumoto K, Sujino M, Kondo T, et al. Melanopsin-dependent photo-perturbation reveals desynchronization underlying the singularity of mammalian circadian clocks. Nat Cell Biol (2007) 9:1327–34. doi:10.1038/ncb1653
53. D’Alessandro M, Beesley S, Kim JK, Chen R, Abich E, Cheng W, et al. A tunable artificial circadian clock in clock-defective mice. Nat Commun (2015) 6:8587. doi:10.1038/ncomms9587
54. Edwards MD, Brancaccio M, Chesham JE, Maywood ES, Hastings MH. Rhythmic expression of cryptochrome induces the circadian clock of arrhythmic suprachiasmatic nuclei through arginine vasopressin signaling. Proc Natl Acad Sci U S A (2016) 113:2732–7. doi:10.1073/pnas.1519044113
55. Smyllie NJ, Chesham JE, Hamnett R, Maywood ES, Hastings MH. Temporally chimeric mice reveal flexibility of circadian period-setting in the suprachiasmatic nucleus. Proc Natl Acad Sci U S A (2016) 113:3657–62. doi:10.1073/pnas.1511351113
56. Nakajima M, Imai K, Ito H, Nishiwaki T, Murayama Y, Iwasaki H, et al. Reconstitution of circadian oscillation of cyanobacterial KaiC phosphorylation in vitro. Science (2005) 308:414–5. doi:10.1126/science.1108451
57. O’Neill JS, Reddy AB. Circadian clocks in human red blood cells. Nature (2011) 469:498–503. doi:10.1038/nature09702
58. Edgar RS, Green EW, Zhao Y, van Ooijen G, Olmedo M, Qin X, et al. Peroxiredoxins are conserved markers of circadian rhythms. Nature (2012) 485:459–64. doi:10.1038/nature11088
59. Cho C-S, Yoon HJ, Kim JY, Woo HA, Rhee SG. Circadian rhythm of hyperoxidized peroxiredoxin II is determined by hemoglobin autoxidation and the 20S proteasome in red blood cells. Proc Natl Acad Sci U S A (2014) 111:12043–8. doi:10.1073/pnas.1401100111
60. Kitayama Y, Iwasaki H, Nishiwaki T, Kondo T. KaiB functions as an attenuator of KaiC phosphorylation in the cyanobacterial circadian clock system. EMBO J (2003) 22:2127–34. doi:10.1093/emboj/cdg212
61. Nishiwaki T, Iwasaki H, Ishiura M, Kondo T. Nucleotide binding and autophosphorylation of the clock protein KaiC as a circadian timing process of cyanobacteria. Proc Natl Acad Sci U S A (2000) 97:495–9. doi:10.1073/pnas.97.1.495
62. Xu Y, Mori T, Johnson CH. Cyanobacterial circadian clockwork: roles of KaiA, KaiB and the KaiBC promoter in regulating KaiC. EMBO J (2003) 22:2117–26. doi:10.1093/emboj/cdg168
63. Nishiwaki T, Satomi Y, Nakajima M, Lee C, Kiyohara R, Kageyama H, et al. Role of KaiC phosphorylation in the circadian clock system of Synechococcus elongatus PCC 7942. Proc Natl Acad Sci U S A (2004) 101:13927–32. doi:10.1073/pnas.0403906101
64. Xu Y, Mori T, Pattanayek R, Pattanayek S, Egli M, Johnson CH. Identification of key phosphorylation sites in the circadian clock protein KaiC by crystallographic and mutagenetic analyses. Proc Natl Acad Sci U S A (2004) 101:13933–8. doi:10.1073/pnas.0404768101
65. Kageyama H, Nishiwaki T, Nakajima M, Iwasaki H, Oyama T, Kondo T. Cyanobacterial circadian pacemaker: Kai protein complex dynamics in the KaiC phosphorylation cycle in vitro. Mol Cell (2006) 23:161–71. doi:10.1016/j.molcel.2006.05.039
66. Nishiwaki T, Satomi Y, Kitayama Y, Terauchi K, Kiyohara R, Takao T, et al. A sequential program of dual phosphorylation of KaiC as a basis for circadian rhythm in cyanobacteria. EMBO J (2007) 26:4029–37. doi:10.1038/sj.emboj.7601832
67. Rust MJ, Markson JS, Lane WS, Fisher DS, O’Shea EK. Ordered phosphorylation governs oscillation of a three-protein circadian clock. Science (2007) 318:809–12. doi:10.1126/science.1148596
68. Ito H, Kageyama H, Mutsuda M, Nakajima M, Oyama T, Kondo T. Autonomous synchronization of the circadian KaiC phosphorylation rhythm. Nat Struct Mol Biol (2007) 14:1084–8. doi:10.1038/nsmb1312
69. Emberly E, Wingreen NS. Hourglass model for a protein-based circadian oscillator. Phys Rev Lett (2006) 96:038303. doi:10.1103/PhysRevLett.96.038303
70. Mehra A, Hong CI, Shi M, Loros JJ, Dunlap JC, Ruoff P. Circadian rhythmicity by autocatalysis. PLoS Comput Biol (2006) 2:e96. doi:10.1371/journal.pcbi.0020096
71. Takigawa-Imamura H, Mochizuki A. Predicting regulation of the phosphorylation cycle of KaiC clock protein using mathematical analysis. J Biol Rhythms (2006) 21:405–16. doi:10.1177/0748730406291329
72. Clodong S, Dühring U, Kronk L, Wilde A, Axmann I, Herzel H, et al. Functioning and robustness of a bacterial circadian clock. Mol Syst Biol (2007) 3:90. doi:10.1038/msb4100128
73. van Zon JS, Lubensky DK, Altena PRH, ten Wolde PR. An allosteric model of circadian KaiC phosphorylation. Proc Natl Acad Sci U S A (2007) 104:7420–5. doi:10.1073/pnas.0608665104
74. Yoda M, Eguchi K, Terada TP, Sasai M. Monomer-shuffling and allosteric transition in KaiC circadian oscillation. PLoS One (2007) 2:e408. doi:10.1371/journal.pone.0000408
75. Mori T, Williams DR, Byrne MO, Qin X, Egli M, Mchaourab HS, et al. Elucidating the ticking of an in vitro circadian clockwork. PLoS Biol (2007) 5:e93. doi:10.1371/journal.pbio.0050093
76. Eguchi K, Yoda M, Terada TP, Sasai M. Mechanism of robust circadian oscillation of KaiC phosphorylation in vitro. Biophys J (2008) 95:1773–84. doi:10.1529/biophysj.107.127555
77. Nagai T, Terada TP, Sasai M. Synchronization of circadian oscillation of phosphorylation level of KaiC in vitro. Biophys J (2010) 98:2469–77. doi:10.1016/j.bpj.2010.02.036
78. Liu P, Kevrekidis IG, Shvartsman SY. Substrate-dependent control of ERK phosphorylation can lead to oscillations. Biophys J (2011) 101:2572–81. doi:10.1016/j.bpj.2011.10.025
79. Chickarmane V, Kholodenko BN, Sauro HM. Oscillatory dynamics arising from competitive inhibition and multisite phosphorylation. J Theor Biol (2007) 244:68–76. doi:10.1016/j.jtbi.2006.05.013
80. Shankaran H, Ippolito DL, Chrisler WB, Resat H, Bollinger N, Opresko LK, et al. Rapid and sustained nuclear-cytoplasmic ERK oscillations induced by epidermal growth factor. Mol Syst Biol (2009) 5:332. doi:10.1038/msb.2009.90
81. Jolley CC, Ode KL, Ueda HR. A design principle for a posttranslational biochemical oscillator. Cell Rep (2012) 2:938–50. doi:10.1016/j.celrep.2012.09.006
82. Markevich NI, Hoek JB, Kholodenko BN. Signaling switches and bistability arising from multisite phosphorylation in protein kinase cascades. J Cell Biol (2004) 164:353–9. doi:10.1083/jcb.200308060
83. Loose M, Fischer-Friedrich E, Ries J, Kruse K, Schwille P. Spatial regulators for bacterial cell division self-organize into surface waves in vitro. Science (2008) 320:789–92. doi:10.1126/science.1154413
84. Chen AH, Lubkowicz D, Yeong V, Chang RL, Silver PA. Transplantability of a circadian clock to a noncircadian organism. Sci Adv (2015) 1:e1500358. doi:10.1126/sciadv.1500358
85. Bruce VG, Pittendrigh CS. Temperature independence in a unicellular “clock”. Proc Natl Acad Sci U S A (1956) 42:676–82. doi:10.1073/pnas.42.9.676
86. Enright JT. Temperature compensation in short-duration time-measurement by an intertidal amphipod. Science (1967) 156:1510–2. doi:10.1126/science.156.3781.1510
87. Hastings JW, Sweeney BM. On the mechanism of temperature independence in a biological clock. Proc Natl Acad Sci U S A (1957) 43:804–11. doi:10.1073/pnas.43.9.804
88. Pittendrigh CS. On temperature independence in the clock system controlling emergence time in Drosophila. Proc Natl Acad Sci U S A (1954) 40:1018–29. doi:10.1073/pnas.40.10.1018
89. Gardner GF, Feldman JF. Temperature compensation of circadian period length in clock mutants of Neurospora crassa. Plant Physiol (1981) 68:1244–8. doi:10.1104/pp.68.6.1244
90. Loros JJ, Feldman JF. Loss of temperature compensation of circadian period length in the frq-9 mutant of Neurospora crassa. J Biol Rhythms (1986) 1:187–98. doi:10.1177/074873048600100302
91. Huang ZJ, Curtin KD, Rosbash M. PER protein interactions and temperature compensation of a circadian clock in Drosophila. Science (1995) 267:1169–72. doi:10.1126/science.7855598
92. Sawyer LA, Hennessy JM, Peixoto AA, Rosato E, Parkinson H, Costa R, et al. Natural variation in a Drosophila clock gene and temperature compensation. Science (1997) 278:2117–20. doi:10.1126/science.278.5346.2117
93. Hong CI, Tyson JJ. A proposal for temperature compensation of the circadian rhythm in Drosophila based on dimerization of the per protein. Chronobiol Int (1997) 14:521–9. doi:10.3109/07420529709001473
94. Leloup JC, Goldbeter A. Temperature compensation of circadian rhythms: control of the period in a model for circadian oscillations of the per protein in Drosophila. Chronobiol Int (1997) 14:511–20. doi:10.3109/07420529709001472
95. Ruoff P, Vinsjevik M, Monnerjahn C, Rensing L. The Goodwin oscillator: on the importance of degradation reactions in the circadian clock. J Biol Rhythms (1999) 14:469–79. doi:10.1177/074873099129001037
96. Tyson JJ, Hong CI, Thron CD, Novak B. A simple model of circadian rhythms based on dimerization and proteolysis of PER and TIM. Biophys J (1999) 77:2411–7. doi:10.1016/S0006-3495(99)77078-5
97. Takeuchi T, Hinohara T, Kurosawa G, Uchida K. A temperature-compensated model for circadian rhythms that can be entrained by temperature cycles. J Theor Biol (2007) 246:195–204. doi:10.1016/j.jtbi.2006.12.028
98. Gould PD, Locke JCW, Larue C, Southern MM, Davis SJ, Hanano S, et al. The molecular basis of temperature compensation in the Arabidopsis circadian clock. Plant Cell (2006) 18:1177–87. doi:10.1105/tpc.105.039990
99. Ruoff P, Rensing L, Kommedal R, Mohsenzadeh S. Modeling temperature compensation in chemical and biological oscillators. Chronobiol Int (1997) 14:499–510. doi:10.3109/07420529709001471
100. Ruoff P, Loros JJ, Dunlap JC. The relationship between FRQ-protein stability and temperature compensation in the Neurospora circadian clock. Proc Natl Acad Sci U S A (2005) 102:17681–6. doi:10.1073/pnas.0505137102
101. Mehra A, Shi M, Baker CL, Colot HV, Loros JJ, Dunlap JC. A role for casein kinase 2 in the mechanism underlying circadian temperature compensation. Cell (2009) 137:749–60. doi:10.1016/j.cell.2009.03.019
102. Zimmerman WF, Pittendrigh CS, Pavlidis T. Temperature compensation of the circadian oscillation in Drosophila pseudoobscura and its entrainment by temperature cycles. J Insect Physiol (1968) 14:669–84. doi:10.1016/0022-1910(68)90226-6
103. Pavlidis T, Zimmerman WF, Osborn J. [A mathematical model for the temperature effects on circadian rhythms]. J Theor Biol (1968) 18:210–21. doi:10.1016/0022-5193(68)90162-8
104. Tomita J, Nakajima M, Kondo T, Iwasaki H. No transcription-translation feedback in circadian rhythm of KaiC phosphorylation. Science (2005) 307:251–4. doi:10.1126/science.1102540
105. Zhou M, Kim JK, Eng GWL, Forger DB, Virshup DM. A Period2 phosphoswitch regulates and temperature compensates circadian period. Mol Cell (2015) 60:77–88. doi:10.1016/j.molcel.2015.08.022
106. Hussain F, Gupta C, Hirning AJ, Ott W, Matthews KS, Josic K, et al. Engineered temperature compensation in a synthetic genetic clock. Proc Natl Acad Sci U S A (2014) 111:972–7. doi:10.1073/pnas.1316298111
107. Hogenesch JB, Ueda HR. Understanding systems-level properties: timely stories from the study of clocks. Nat Rev Genet (2011) 12:407–16. doi:10.1038/nrg2972
108. Baggs JE, Price TS, DiTacchio L, Panda S, Fitzgerald GA, Hogenesch JB. Network features of the mammalian circadian clock. PLoS Biol (2009) 7:e52. doi:10.1371/journal.pbio.1000052
109. Dibner C, Sage D, Unser M, Bauer C, d’Eysmond T, Naef F, et al. Circadian gene expression is resilient to large fluctuations in overall transcription rates. EMBO J (2009) 28:123–34. doi:10.1038/emboj.2008.262
110. Barkai N, Leibler S. Circadian clocks limited by noise. Nature (2000) 403:267–8. doi:10.1038/35002255
111. Ueda HR, Hirose K, Iino M. Intercellular coupling mechanism for synchronized and noise-resistant circadian oscillators. J Theor Biol (2002) 216:501–12. doi:10.1006/jtbi.2002.3000
112. DeBruyne JP, Weaver DR, Reppert SM. Peripheral circadian oscillators require CLOCK. Curr Biol (2007) 17:R538–9. doi:10.1016/j.cub.2007.05.067
113. Liu AC, Welsh DK, Ko CH, Tran HG, Zhang EE, Priest AA, et al. Intercellular coupling confers robustness against mutations in the SCN circadian clock network. Cell (2007) 129:605–16. doi:10.1016/j.cell.2007.02.047
114. Zhang EE, Kay SA. Clocks not winding down: unravelling circadian networks. Nat Rev Mol Cell Biol (2010) 11:764–76. doi:10.1038/nrm2995
115. Akman OE, Rand DA, Brown PE, Millar AJ. Robustness from flexibility in the fungal circadian clock. BMC Syst Biol (2010) 4:88. doi:10.1186/1752-0509-4-88
116. Saithong T, Painter KJ, Millar AJ. The contributions of interlocking loops and extensive nonlinearity to the properties of circadian clock models. PLoS One (2010) 5:e13867. doi:10.1371/journal.pone.0013867
117. Smolen P, Baxter DA, Byrne JH. Modeling circadian oscillations with interlocking positive and negative feedback loops. J Neurosci (2001) 21:6644–56.
118. Sriram K, Gopinathan MS. A two variable delay model for the circadian rhythm of Neurospora crassa. J Theor Biol (2004) 231:23–38. doi:10.1016/j.jtbi.2004.04.006
119. André E, Conquet F, Steinmayr M, Stratton SC, Porciatti V, Becker-André M. Disruption of retinoid-related orphan receptor beta changes circadian behavior, causes retinal degeneration and leads to vacillans phenotype in mice. EMBO J (1998) 17:3867–77. doi:10.1093/emboj/17.14.3867
120. Liu AC, Tran HG, Zhang EE, Priest AA, Welsh DK, Kay SA. Redundant function of REV-ERBalpha and beta and non-essential role for Bmal1 cycling in transcriptional regulation of intracellular circadian rhythms. PLoS Genet (2008) 4:e1000023. doi:10.1371/journal.pgen.1000023
121. Preitner N, Damiola F, Lopez-Molina L, Zakany J, Duboule D, Albrecht U, et al. The orphan nuclear receptor REV-ERBα controls circadian transcription within the positive limb of the mammalian circadian oscillator. Cell (2002) 110:251–60. doi:10.1016/S0092-8674(02)00825-5
122. Sato TK, Panda S, Miraglia LJ, Reyes TM, Rudic RD, McNamara P, et al. A functional genomics strategy reveals Rora as a component of the mammalian circadian clock. Neuron (2004) 43:527–37. doi:10.1016/j.neuron.2004.07.018
123. Vilar JMG, Kueh HY, Barkai N, Leibler S. Mechanisms of noise-resistance in genetic oscillators. Proc Natl Acad Sci U S A (2002) 99:5988–92. doi:10.1073/pnas.092133899
124. Kim JK, Forger DB. A mechanism for robust circadian timekeeping via stoichiometric balance. Mol Syst Biol (2012) 8:630. doi:10.1038/msb.2012.62
125. Chen R, Schirmer A, Lee Y, Lee H, Kumar V, Yoo S-H, et al. Rhythmic PER abundance defines a critical nodal point for negative feedback within the circadian clock mechanism. Mol Cell (2009) 36:417–30. doi:10.1016/j.molcel.2009.10.012
126. Lee Y, Chen R, Lee H, Lee C. Stoichiometric relationship among clock proteins determines robustness of circadian rhythms. J Biol Chem (2011) 286:7033–42. doi:10.1074/jbc.M110.207217
127. Lee C, Etchegaray JP, Cagampang FR, Loudon AS, Reppert SM. Posttranslational mechanisms regulate the mammalian circadian clock. Cell (2001) 107:855–67. doi:10.1016/S0092-8674(01)00610-9
128. Narumi R, Shimizu Y, Ukai-Tadenuma M, Ode KL, Kanda GN, Shinohara Y, et al. Mass spectrometry-based absolute quantification reveals rhythmic variation of mouse circadian clock proteins. Proc Natl Acad Sci U S A (2016) 113:E3461–7. doi:10.1073/pnas.1603799113
129. Kim JK. Protein sequestration versus Hill-type repression in circadian clock models. IET Syst Biol (2016) 10:125–35. doi:10.1049/iet-syb.2015.0090
130. Gotoh T, Kim JK, Liu J, Vila-Caballer M, Stauffer PE, Tyson JJ, et al. Model-driven experimental approach reveals the complex regulatory distribution of p53 by the circadian factor Period 2. Proc Natl Acad Sci U S A (2016) 113(47):13516–21. doi:10.1073/pnas.1607984113
131. Saper CB, Lu J, Chou TC, Gooley J. The hypothalamic integrator for circadian rhythms. Trends Neurosci (2005) 28:152–7. doi:10.1016/j.tins.2004.12.009
132. Turek FW. Circadian neural rhythms in mammals. Annu Rev Physiol (1985) 47:49–64. doi:10.1146/annurev.ph.47.030185.000405
133. Yamazaki S, Numano R, Abe M, Hida A, Takahashi R, Ueda M, et al. Resetting central and peripheral circadian oscillators in transgenic rats. Science (2000) 288:682–5. doi:10.1126/science.288.5466.682
134. Balsalobre A, Damiola F, Schibler U. A serum shock induces circadian gene expression in mammalian tissue culture cells. Cell (1998) 93:929–37. doi:10.1016/S0092-8674(00)81199-X
135. Yagita K, Okamura H. Forskolin induces circadian gene expression of rPer1, rPer2 and dbp in mammalian rat-1 fibroblasts. FEBS Lett (2000) 465:79–82. doi:10.1016/S0014-5793(99)01724-X
136. Yagita K, Tamanini F, van Der Horst GT, Okamura H. Molecular mechanisms of the biological clock in cultured fibroblasts. Science (2001) 292:278–81. doi:10.1126/science.1059542
137. Bell-Pedersen D, Cassone VM, Earnest DJ, Golden SS, Hardin PE, Thomas TL, et al. Circadian rhythms from multiple oscillators: lessons from diverse organisms. Nat Rev Genet (2005) 6:544–56. doi:10.1038/nrg1633
138. Mohawk JA, Green CB, Takahashi JS. Central and peripheral circadian clocks in mammals. Annu Rev Neurosci (2012) 35:445–62. doi:10.1146/annurev-neuro-060909-153128
139. Partch CL, Green CB, Takahashi JS. Molecular architecture of the mammalian circadian clock. Trends Cell Biol (2014) 24:90–9. doi:10.1016/j.tcb.2013.07.002
140. Reppert SM, Weaver DR. Molecular analysis of mammalian circadian rhythms. Annu Rev Physiol (2001) 63:647–76. doi:10.1146/annurev.physiol.63.1.647
141. Sehgal A, Price JL, Man B, Young MW. Loss of circadian behavioral rhythms and per RNA oscillations in the Drosophila mutant timeless. Science (1994) 263:1603–6. doi:10.1126/science.8128246
142. King DP, Zhao Y, Sangoram AM, Wilsbacher LD, Tanaka M, Antoch MP, et al. Positional cloning of the mouse circadian clock gene. Cell (1997) 89:641–53. doi:10.1016/S0092-8674(00)80245-7
143. Vitaterna MH, King DP, Chang AM, Kornhauser JM, Lowrey PL, McDonald JD, et al. Mutagenesis and mapping of a mouse gene, Clock, essential for circadian behavior. Science (1994) 264:719–25. doi:10.1126/science.8171325
144. Hogenesch JB, Chan WK, Jackiw VH, Brown RC, Gu YZ, Pray-Grant M, et al. Characterization of a subset of the basic-helix-loop-helix-PAS superfamily that interacts with components of the dioxin signaling pathway. J Biol Chem (1997) 272:8581–93. doi:10.1074/jbc.272.13.8581
145. Ikeda M, Nomura M. cDNA cloning and tissue-specific expression of a novel basic helix-loop-helix/PAS protein (BMAL1) and identification of alternatively spliced variants with alternative translation initiation site usage. Biochem Biophys Res Commun (1997) 233:258–64. doi:10.1006/bbrc.1997.6371
146. Kadener S, Stoleru D, McDonald M, Nawathean P, Rosbash M. Clockwork Orange is a transcriptional repressor and a new Drosophila circadian pacemaker component. Genes Dev (2007) 21:1675–86. doi:10.1101/gad.1552607
147. Lim C, Chung BY, Pitman JL, McGill JJ, Pradhan S, Lee J, et al. Clockwork orange encodes a transcriptional repressor important for circadian-clock amplitude in Drosophila. Curr Biol (2007) 17:1082–9. doi:10.1016/j.cub.2007.05.039
148. Matsumoto A, Ukai-Tadenuma M, Yamada RG, Houl J, Uno KD, Kasukawa T, et al. A functional genomics strategy reveals clockwork orange as a transcriptional regulator in the Drosophila circadian clock. Genes Dev (2007) 21:1687–700. doi:10.1101/gad.1552207
149. Honma S, Kawamoto T, Takagi Y, Fujimoto K, Sato F, Noshiro M, et al. Dec1 and Dec2 are regulators of the mammalian molecular clock. Nature (2002) 419:841–4. doi:10.1038/nature01123
150. McDonald MJ, Rosbash M. Microarray analysis and organization of circadian gene expression in Drosophila. Cell (2001) 107:567–78. doi:10.1016/S0092-8674(01)00545-1
151. Ueda HR, Matsumoto A, Kawamura M, Iino M, Tanimura T, Hashimoto S. Genome-wide transcriptional orchestration of circadian rhythms in Drosophila. J Biol Chem (2002) 277:14048–52. doi:10.1074/jbc.C100765200
152. Akhtar RA, Reddy AB, Maywood ES, Clayton JD, King VM, Smith AG, et al. Circadian cycling of the mouse liver transcriptome, as revealed by cDNA microarray, is driven by the suprachiasmatic nucleus. Curr Biol (2002) 12:540–50. doi:10.1016/S0960-9822(02)00759-5
153. Panda S, Antoch MP, Miller BH, Su AI, Schook AB, Straume M, et al. Coordinated transcription of key pathways in the mouse by the circadian clock. Cell (2002) 109:307–20. doi:10.1016/S0092-8674(02)00722-5
154. Storch K-F, Lipan O, Leykin I, Viswanathan N, Davis FC, Wong WH, et al. Extensive and divergent circadian gene expression in liver and heart. Nature (2002) 417:78–83. doi:10.1038/nature744
155. Ueda HR, Chen W, Adachi A, Wakamatsu H, Hayashi S, Takasugi T, et al. A transcription factor response element for gene expression during circadian night. Nature (2002) 418:534–9. doi:10.1038/nature00906
156. Humphries A, Klein D, Baler R, Carter DA. cDNA array analysis of pineal gene expression reveals circadian rhythmicity of the dominant negative helix-loop-helix protein-encoding gene, Id-1. J Neuroendocrinol (2002) 14:101–8. doi:10.1046/j.0007-1331.2001.00738.x
157. Duffield GE, Best JD, Meurers BH, Bittner A, Loros JJ, Dunlap JC. Circadian programs of transcriptional activation, signaling, and protein turnover revealed by microarray analysis of mammalian cells. Curr Biol (2002) 12:551–7. doi:10.1016/S0960-9822(02)00765-0
158. Grundschober C, Delaunay F, Pühlhofer A, Triqueneaux G, Laudet V, Bartfai T, et al. Circadian regulation of diverse gene products revealed by mRNA expression profiling of synchronized fibroblasts. J Biol Chem (2001) 276:46751–8. doi:10.1074/jbc.M107499200
159. Schaffer R, Landgraf J, Accerbi M, Simon V, Larson M, Wisman E. Microarray analysis of diurnal and circadian-regulated genes in Arabidopsis. Plant Cell (2001) 13:113–23. doi:10.1105/tpc.13.1.113
160. Duffield GE. DNA microarray analyses of circadian timing: the genomic basis of biological time. J Neuroendocrinol (2003) 15:991–1002. doi:10.1046/j.1365-2826.2003.01082.x
161. Hughes ME, DiTacchio L, Hayes KR, Vollmers C, Pulivarthy S, Baggs JE, et al. Harmonics of circadian gene transcription in mammals. PLoS Genet (2009) 5:e1000442. doi:10.1371/journal.pgen.1000442
162. Hughes ME, Hong H-K, Chong JL, Indacochea AA, Lee SS, Han M, et al. Brain-specific rescue of Clock reveals system-driven transcriptional rhythms in peripheral tissue. PLoS Genet (2012) 8:e1002835. doi:10.1371/journal.pgen.1002835
163. Yoo S-H, Yamazaki S, Lowrey PL, Shimomura K, Ko CH, Buhr ED, et al. PERIOD2:LUCIFERASE real-time reporting of circadian dynamics reveals persistent circadian oscillations in mouse peripheral tissues. Proc Natl Acad Sci U S A (2004) 101:5339–46. doi:10.1073/pnas.0308709101
164. Koike N, Yoo S-H, Huang H-C, Kumar V, Lee C, Kim T-K, et al. Transcriptional architecture and chromatin landscape of the core circadian clock in mammals. Science (2012) 338:349–54. doi:10.1126/science.1226339
165. Menet JS, Rodriguez J, Abruzzi KC, Rosbash M. Nascent-Seq reveals novel features of mouse circadian transcriptional regulation. Elife (2012) 1:e00011. doi:10.7554/eLife.00011
166. Vollmers C, Schmitz RJ, Nathanson J, Yeo G, Ecker JR, Panda S. Circadian oscillations of protein-coding and regulatory RNAs in a highly dynamic mammalian liver epigenome. Cell Metab (2012) 16:833–45. doi:10.1016/j.cmet.2012.11.004
167. Hatanaka F, Matsubara C, Myung J, Yoritaka T, Kamimura N, Tsutsumi S, et al. Genome-wide profiling of the core clock protein BMAL1 targets reveals a strict relationship with metabolism. Mol Cell Biol (2010) 30:5636–48. doi:10.1128/MCB.00781-10
168. Le Martelot G, Canella D, Symul L, Migliavacca E, Gilardi F, Liechti R, et al. Genome-wide RNA polymerase II profiles and RNA accumulation reveal kinetics of transcription and associated epigenetic changes during diurnal cycles. PLoS Biol (2012) 10:e1001442. doi:10.1371/journal.pbio.1001442
169. Rey G, Cesbron F, Rougemont J, Reinke H, Brunner M, Naef F. Genome-wide and phase-specific DNA-binding rhythms of BMAL1 control circadian output functions in mouse liver. PLoS Biol (2011) 9:e1000595. doi:10.1371/journal.pbio.1000595
170. Yoshitane H, Ozaki H, Terajima H, Du N-H, Suzuki Y, Fujimori T, et al. CLOCK-controlled polyphonic regulation of circadian rhythms through canonical and noncanonical e-boxes. Mol Cell Biol (2014) 34:1776–87. doi:10.1128/MCB.01465-13
171. Ueda HR, Hayashi S, Chen W, Sano M, Machida M, Shigeyoshi Y, et al. System-level identification of transcriptional circuits underlying mammalian circadian clocks. Nat Genet (2005) 37:187–92. doi:10.1038/ng1504
172. Crosio C, Cermakian N, Allis CD, Sassone-Corsi P. Light induces chromatin modification in cells of the mammalian circadian clock. Nat Neurosci (2000) 3:1241–7. doi:10.1038/81767
173. Etchegaray J-P, Lee C, Wade PA, Reppert SM. Rhythmic histone acetylation underlies transcription in the mammalian circadian clock. Nature (2003) 421:177–82. doi:10.1038/nature01314
174. Nakahata Y, Kaluzova M, Grimaldi B, Sahar S, Hirayama J, Chen D, et al. The NAD+-dependent deacetylase SIRT1 modulates CLOCK-mediated chromatin remodeling and circadian control. Cell (2008) 134:329–40. doi:10.1016/j.cell.2008.07.002
175. Doi M, Hirayama J, Sassone-Corsi P. Circadian regulator CLOCK is a histone acetyltransferase. Cell (2006) 125:497–508. doi:10.1016/j.cell.2006.03.033
176. Ripperger JA, Schibler U. Rhythmic CLOCK-BMAL1 binding to multiple E-box motifs drives circadian Dbp transcription and chromatin transitions. Nat Genet (2006) 38:369–74. doi:10.1038/ng1738
177. Hirayama J, Sahar S, Grimaldi B, Tamaru T, Takamatsu K, Nakahata Y, et al. CLOCK-mediated acetylation of BMAL1 controls circadian function. Nature (2007) 450:1086–90. doi:10.1038/nature06394
178. Asher G, Gatfield D, Stratmann M, Reinke H, Dibner C, Kreppel F, et al. SIRT1 regulates circadian clock gene expression through PER2 deacetylation. Cell (2008) 134:317–28. doi:10.1016/j.cell.2008.06.050
179. Aguilar-Arnal L, Katada S, Orozco-Solis R, Sassone-Corsi P. NAD(+)-SIRT1 control of H3K4 trimethylation through circadian deacetylation of MLL1. Nat Struct Mol Biol (2015) 22:312–8. doi:10.1038/nsmb.2990
180. Peek CB, Affinati AH, Ramsey KM, Kuo H-Y, Yu W, Sena LA, et al. Circadian clock NAD+ cycle drives mitochondrial oxidative metabolism in mice. Science (2013) 342:1243417. doi:10.1126/science.1243417
181. Nakahata Y, Sahar S, Astarita G, Kaluzova M, Sassone-Corsi P. Circadian control of the NAD+ salvage pathway by CLOCK-SIRT1. Science (2009) 324:654–7. doi:10.1126/science.1170803
182. Rutter J, Reick M, Wu LC, McKnight SL. Regulation of clock and NPAS2 DNA binding by the redox state of NAD cofactors. Science (2001) 293:510–4. doi:10.1126/science.1060698
183. Huang G, Zhang Y, Shan Y, Yang S, Chelliah Y, Wang H, et al. Circadian oscillations of NADH redox state using a heterologous metabolic sensor in mammalian cells. J Biol Chem (2016) 291:23906–14. doi:10.1074/jbc.M116.728774
184. Cho H, Zhao X, Hatori M, Yu RT, Barish GD, Lam MT, et al. Regulation of circadian behaviour and metabolism by REV-ERB-α and REV-ERB-β. Nature (2012) 485:123–7. doi:10.1038/nature11048
185. Feng D, Liu T, Sun Z, Bugge A, Mullican SE, Alenghat T, et al. A circadian rhythm orchestrated by histone deacetylase 3 controls hepatic lipid metabolism. Science (2011) 331:1315–9. doi:10.1126/science.1198125
186. Bartok O, Kyriacou CP, Levine J, Sehgal A, Kadener S. Adaptation of molecular circadian clockwork to environmental changes: a role for alternative splicing and miRNAs. Proc Biol Sci (2013) 280:20130011. doi:10.1098/rspb.2013.0011
187. Krol J, Loedige I, Filipowicz W. The widespread regulation of microRNA biogenesis, function and decay. Nat Rev Genet (2010) 11:597–610. doi:10.1038/nrg2843
188. Mehta N, Cheng H-YM. Micro-managing the circadian clock: the role of microRNAs in biological timekeeping. J Mol Biol (2013) 425:3609–24. doi:10.1016/j.jmb.2012.10.022
189. Yates LA, Norbury CJ, Gilbert RJC. The long and short of microRNA. Cell (2013) 153:516–9. doi:10.1016/j.cell.2013.04.003
190. Na YJ, Sung JH, Lee SC, Lee YJ, Choi YJ, Park WY, et al. Comprehensive analysis of microRNA-mRNA co-expression in circadian rhythm. Exp Mol Med (2009) 41:638–47. doi:10.3858/emm.2009.41.9.070
191. Xu S, Witmer PD, Lumayag S, Kovacs B, Valle D. MicroRNA (miRNA) transcriptome of mouse retina and identification of a sensory organ-specific miRNA cluster. J Biol Chem (2007) 282:25053–66. doi:10.1074/jbc.M700501200
192. Cheng H-YM, Papp JW, Varlamova O, Dziema H, Russell B, Curfman JP, et al. microRNA modulation of circadian-clock period and entrainment. Neuron (2007) 54:813–29. doi:10.1016/j.neuron.2007.05.017
193. Gao Q, Zhou L, Yang S-Y, Cao J-M. A novel role of microRNA 17-5p in the modulation of circadian rhythm. Sci Rep (2016) 6:30070. doi:10.1038/srep30070
194. Gatfield D, Le Martelot G, Vejnar CE, Gerlach D, Schaad O, Fleury-Olela F, et al. Integration of microRNA miR-122 in hepatic circadian gene expression. Genes Dev (2009) 23:1313–26. doi:10.1101/gad.1781009
195. Curtis AM, Fagundes CT, Yang G, Palsson-McDermott EM, Wochal P, McGettrick AF, et al. Circadian control of innate immunity in macrophages by miR-155 targeting Bmal1. Proc Natl Acad Sci U S A (2015) 112:7231–6. doi:10.1073/pnas.1501327112
196. Shende VR, Neuendorff N, Earnest DJ. Role of miR-142-3p in the post-transcriptional regulation of the clock gene Bmal1 in the mouse SCN. PLoS One (2013) 8:e65300. doi:10.1371/journal.pone.0065300
197. Tan X, Zhang P, Zhou L, Yin B, Pan H, Peng X. Clock-controlled mir-142-3p can target its activator, Bmal1. BMC Mol Biol (2012) 13:27. doi:10.1186/1471-2199-13-27
198. Zhang W, Wang P, Chen S, Zhang Z, Liang T, Liu C. Rhythmic expression of miR-27b-3p targets the clock gene Bmal1 at the posttranscriptional level in the mouse liver. FASEB J (2016) 30:2151–60. doi:10.1096/fj.201500120
199. Ding X, Sun B, Huang J, Xu L, Pan J, Fang C, et al. The role of miR-182 in regulating pineal CLOCK expression after hypoxia-ischemia brain injury in neonatal rats. Neurosci Lett (2015) 591:75–80. doi:10.1016/j.neulet.2015.02.026
200. Lerner I, Bartok O, Wolfson V, Menet JS, Weissbein U, Afik S, et al. Clk post-transcriptional control denoises circadian transcription both temporally and spatially. Nat Commun (2015) 6:7056. doi:10.1038/ncomms8056
201. Kojima S, Gatfield D, Esau CC, Green CB. MicroRNA-122 modulates the rhythmic expression profile of the circadian deadenylase Nocturnin in mouse liver. PLoS One (2010) 5:e11264. doi:10.1371/journal.pone.0011264
202. Chen R, D’Alessandro M, Lee C. miRNAs are required for generating a time delay critical for the circadian oscillator. Curr Biol (2013) 23:1959–68. doi:10.1016/j.cub.2013.08.005
203. Nagel R, Clijsters L, Agami R. The miRNA-192/194 cluster regulates the Period gene family and the circadian clock. FEBS J (2009) 276:5447–55. doi:10.1111/j.1742-4658.2009.07229.x
204. Zhao X, Zhu X, Cheng S, Xie Y, Wang Z, Liu Y, et al. MiR-29a/b/c regulate human circadian gene hPER1 expression by targeting its 3’UTR. Acta Biochim Biophys Sin (2014) 46:313–7. doi:10.1093/abbs/gmu007
205. Chen W, Liu Z, Li T, Zhang R, Xue Y, Zhong Y, et al. Regulation of Drosophila circadian rhythms by miRNA let-7 is mediated by a regulatory cycle. Nat Commun (2014) 5:5549. doi:10.1038/ncomms6549
206. Chen X, Rosbash M. mir-276a strengthens Drosophila circadian rhythms by regulating timeless expression. Proc Natl Acad Sci U S A (2016) 113:E2965–72. doi:10.1073/pnas.1605837113
207. Lee K-H, Kim S-H, Lee H-R, Kim W, Kim D-Y, Shin J-C, et al. MicroRNA-185 oscillation controls circadian amplitude of mouse Cryptochrome 1 via translational regulation. Mol Biol Cell (2013) 24:2248–55. doi:10.1091/mbc.E12-12-0849
208. Du N-H, Arpat AB, De Matos M, Gatfield D. MicroRNAs shape circadian hepatic gene expression on a transcriptome-wide scale. Elife (2014) 3:e02510. doi:10.7554/eLife.02510
209. Derrien T, Johnson R, Bussotti G, Tanzer A, Djebali S, Tilgner H, et al. The GENCODE v7 catalog of human long noncoding RNAs: analysis of their gene structure, evolution, and expression. Genome Res (2012) 22:1775–89. doi:10.1101/gr.132159.111
210. Hangauer MJ, Vaughn IW, McManus MT. Pervasive transcription of the human genome produces thousands of previously unidentified long intergenic noncoding RNAs. PLoS Genet (2013) 9:e1003569. doi:10.1371/journal.pgen.1003569
211. Schick S, Becker K, Thakurela S, Fournier D, Hampel MH, Legewie S, et al. Identifying novel transcriptional regulators with circadian expression. Mol Cell Biol (2015) 36:545–58. doi:10.1128/MCB.00701-15
212. Zhang R, Lahens NF, Ballance HI, Hughes ME, Hogenesch JB. A circadian gene expression atlas in mammals: implications for biology and medicine. Proc Natl Acad Sci U S A (2014) 111:16219–24. doi:10.1073/pnas.1408886111
213. Coon SL, Munson PJ, Cherukuri PF, Sugden D, Rath MF, Møller M, et al. Circadian changes in long noncoding RNAs in the pineal gland. Proc Natl Acad Sci U S A (2012) 109:13319–24. doi:10.1073/pnas.1207748109
214. Sauman I, Reppert SM. Circadian clock neurons in the silkmoth Antheraea pernyi: novel mechanisms of Period protein regulation. Neuron (1996) 17:889–900. doi:10.1016/S0896-6273(00)80220-2
215. Kramer C, Loros JJ, Dunlap JC, Crosthwaite SK. Role for antisense RNA in regulating circadian clock function in Neurospora crassa. Nature (2003) 421:948–52. doi:10.1038/nature01427
216. Li N, Joska TM, Ruesch CE, Coster SJ, Belden WJ. The frequency natural antisense transcript first promotes, then represses, frequency gene expression via facultative heterochromatin. Proc Natl Acad Sci U S A (2015) 112:4357–62. doi:10.1073/pnas.1406130112
217. Xue Z, Ye Q, Anson SR, Yang J, Xiao G, Kowbel D, et al. Transcriptional interference by antisense RNA is required for circadian clock function. Nature (2014) 514:650–3. doi:10.1038/nature13671
218. Powell WT, Coulson RL, Crary FK, Wong SS, Ach RA, Tsang P, et al. A Prader-Willi locus lncRNA cloud modulates diurnal genes and energy expenditure. Hum Mol Genet (2013) 22:4318–28. doi:10.1093/hmg/ddt281
219. Cui M, Zheng M, Sun B, Wang Y, Ye L, Zhang X. A long noncoding RNA perturbs the circadian rhythm of hepatoma cells to facilitate hepatocarcinogenesis. Neoplasia (2015) 17:79–88. doi:10.1016/j.neo.2014.11.004
220. Rodriguez J, Tang C-HA, Khodor YL, Vodala S, Menet JS, Rosbash M. Nascent-Seq analysis of Drosophila cycling gene expression. Proc Natl Acad Sci U S A (2013) 110:E275–84. doi:10.1073/pnas.1219969110
221. Lim C, Allada R. Emerging roles for post-transcriptional regulation in circadian clocks. Nat Neurosci (2013) 16:1544–50. doi:10.1038/nn.3543
222. So WV, Rosbash M. Post-transcriptional regulation contributes to Drosophila clock gene mRNA cycling. EMBO J (1997) 16:7146–55. doi:10.1093/emboj/16.23.7146
223. Wilsbacher LD, Yamazaki S, Herzog ED, Song E-J, Radcliffe LA, Abe M, et al. Photic and circadian expression of luciferase in mPeriod1-luc transgenic mice invivo. Proc Natl Acad Sci U S A (2002) 99:489–94. doi:10.1073/pnas.012248599
224. Kojima S, Hirose M, Tokunaga K, Sakaki Y, Tei H. Structural and functional analysis of 3’ untranslated region of mouse Period1 mRNA. Biochem Biophys Res Commun (2003) 301:1–7. doi:10.1016/S0006-291X(02)02938-8
225. Kojima S, Matsumoto K, Hirose M, Shimada M, Nagano M, Shigeyoshi Y, et al. LARK activates posttranscriptional expression of an essential mammalian clock protein, PERIOD1. Proc Natl Acad Sci U S A (2007) 104:1859–64. doi:10.1073/pnas.0607567104
226. Huang Y, McNeil GP, Jackson FR. Translational regulation of the DOUBLETIME/CKIδ/ε kinase by LARK contributes to circadian period modulation. PLoS Genet (2014) 10:e1004536. doi:10.1371/journal.pgen.1004536
227. Kim D-Y, Woo K-C, Lee K-H, Kim T-D, Kim K-T. hnRNP Q and PTB modulate the circadian oscillation of mouse Rev-erb alpha via IRES-mediated translation. Nucleic Acids Res (2010) 38:7068–78. doi:10.1093/nar/gkq569
228. Kim D-Y, Kwak E, Kim S-H, Lee K-H, Woo K-C, Kim K-T. hnRNP Q mediates a phase-dependent translation-coupled mRNA decay of mouse Period3. Nucleic Acids Res (2011) 39:8901–14. doi:10.1093/nar/gkr605
229. Kim T-D, Woo K-C, Cho S, Ha D-C, Jang SK, Kim K-T. Rhythmic control of AANAT translation by hnRNP Q in circadian melatonin production. Genes Dev (2007) 21:797–810. doi:10.1101/gad.1519507
230. Kwak E, Kim T-D, Kim K-T. Essential role of 3’-untranslated region-mediated mRNA decay in circadian oscillations of mouse Period3 mRNA. J Biol Chem (2006) 281:19100–6. doi:10.1074/jbc.M511927200
231. Lee K-H, Woo K-C, Kim D-Y, Kim T-D, Shin J, Park SM, et al. Rhythmic interaction between Period1 mRNA and hnRNP Q leads to circadian time-dependent translation. Mol Cell Biol (2012) 32:717–28. doi:10.1128/MCB.06177-11
232. Lim I, Jung Y, Kim D-Y, Kim K-T. HnRNP Q has a suppressive role in the translation of mouse Cryptochrome1. PLoS One (2016) 11:e0159018. doi:10.1371/journal.pone.0159018
233. Lee K-H, Kim S-H, Kim H-J, Kim W, Lee H-R, Jung Y, et al. AUF1 contributes to Cryptochrome1 mRNA degradation and rhythmic translation. Nucleic Acids Res (2014) 42:3590–606. doi:10.1093/nar/gkt1379
234. Woo K-C, Ha D-C, Lee K-H, Kim D-Y, Kim T-D, Kim K-T. Circadian amplitude of cryptochrome 1 is modulated by mRNA stability regulation via cytoplasmic hnRNP D oscillation. Mol Cell Biol (2010) 30:197–205. doi:10.1128/MCB.01154-09
235. Woo K-C, Kim T-D, Lee K-H, Kim D-Y, Kim W, Lee K-Y, et al. Mouse period 2 mRNA circadian oscillation is modulated by PTB-mediated rhythmic mRNA degradation. Nucleic Acids Res (2009) 37:26–37. doi:10.1093/nar/gkn893
236. Proudfoot NJ, Furger A, Dye MJ. Integrating mRNA processing with transcription. Cell (2002) 108:501–12. doi:10.1016/S0092-8674(02)00617-7
237. Doidge R, Mittal S, Aslam A, Winkler GS. Deadenylation of cytoplasmic mRNA by the mammalian Ccr4-Not complex. Biochem Soc Trans (2012) 40:896–901. doi:10.1042/BST20120074
238. Virtanen A, Henriksson N, Nilsson P, Nissbeck M. Poly(A)-specific ribonuclease (PARN): an allosterically regulated, processive and mRNA cap-interacting deadenylase. Crit Rev Biochem Mol Biol (2013) 48:192–209. doi:10.3109/10409238.2013.771132
239. Robinson BG, Frim DM, Schwartz WJ, Majzoub JA. Vasopressin mRNA in the suprachiasmatic nuclei: daily regulation of polyadenylate tail length. Science (1988) 241:342–4. doi:10.1126/science.3388044
240. Green CB, Besharse JC. Identification of a novel vertebrate circadian clock-regulated gene encoding the protein nocturnin. Proc Natl Acad Sci U S A (1996) 93:14884–8. doi:10.1073/pnas.93.25.14884
241. Green CB, Besharse JC. Use of a high stringency differential display screen for identification of retinal mRNAs that are regulated by a circadian clock. Brain Res Mol Brain Res (1996) 37:157–65. doi:10.1016/0169-328X(95)00307-E
242. Wang Y, Osterbur DL, Megaw PL, Tosini G, Fukuhara C, Green CB, et al. Rhythmic expression of Nocturnin mRNA in multiple tissues of the mouse. BMC Dev Biol (2001) 1:9. doi:10.1186/1471-213X-1-9
243. Kornmann B, Schaad O, Bujard H, Takahashi JS, Schibler U. System-driven and oscillator-dependent circadian transcription in mice with a conditionally active liver clock. PLoS Biol (2007) 5:e34. doi:10.1371/journal.pbio.0050034
244. Green CB, Douris N, Kojima S, Strayer CA, Fogerty J, Lourim D, et al. Loss of Nocturnin, a circadian deadenylase, confers resistance to hepatic steatosis and diet-induced obesity. Proc Natl Acad Sci U S A (2007) 104:9888–93. doi:10.1073/pnas.0702448104
245. Nagoshi E, Sugino K, Kula E, Okazaki E, Tachibana T, Nelson S, et al. Dissecting differential gene expression within the circadian neuronal circuit of Drosophila. Nat Neurosci (2010) 13:60–8. doi:10.1038/nn.2451
246. Kojima S, Sher-Chen EL, Green CB. Circadian control of mRNA polyadenylation dynamics regulates rhythmic protein expression. Genes Dev (2012) 26:2724–36. doi:10.1101/gad.208306.112
247. Chang H, Lim J, Ha M, Kim VN. TAIL-seq: genome-wide determination of poly(A) tail length and 3’ end modifications. Mol Cell (2014) 53:1044–52. doi:10.1016/j.molcel.2014.02.007
248. Subtelny AO, Eichhorn SW, Chen GR, Sive H, Bartel DP. Poly(A)-tail profiling reveals an embryonic switch in translational control. Nature (2014) 508:66–71. doi:10.1038/nature13007
249. Fustin J-M, Doi M, Yamaguchi Y, Hida H, Nishimura S, Yoshida M, et al. RNA-methylation-dependent RNA processing controls the speed of the circadian clock. Cell (2013) 155:793–806. doi:10.1016/j.cell.2013.10.026
250. Wang X, Lu Z, Gomez A, Hon GC, Yue Y, Han D, et al. N6-methyladenosine-dependent regulation of messenger RNA stability. Nature (2014) 505:117–20. doi:10.1038/nature12730
251. Rouskin S, Zubradt M, Washietl S, Kellis M, Weissman JS. Genome-wide probing of RNA structure reveals active unfolding of mRNA structures in vivo. Nature (2014) 505:701–5. doi:10.1038/nature12894
252. Wan Y, Qu K, Zhang QC, Flynn RA, Manor O, Ouyang Z, et al. Landscape and variation of RNA secondary structure across the human transcriptome. Nature (2014) 505:706–9. doi:10.1038/nature12946
253. Imamachi N, Tani H, Mizutani R, Imamura K, Irie T, Suzuki Y, et al. BRIC-seq: a genome-wide approach for determining RNA stability in mammalian cells. Methods (2014) 67:55–63. doi:10.1016/j.ymeth.2013.07.014
254. Tani H, Akimitsu N. Genome-wide technology for determining RNA stability in mammalian cells: historical perspective and recent advantages based on modified nucleotide labeling. RNA Biol (2012) 9:1233–8. doi:10.4161/rna.22036
255. Castello A, Fischer B, Eichelbaum K, Horos R, Beckmann BM, Strein C, et al. Insights into RNA biology from an atlas of mammalian mRNA-binding proteins. Cell (2012) 149:1393–406. doi:10.1016/j.cell.2012.04.031
256. Hafner M, Landthaler M, Burger L, Khorshid M, Hausser J, Berninger P, et al. Transcriptome-wide identification of RNA-binding protein and microRNA target sites by PAR-CLIP. Cell (2010) 141:129–41. doi:10.1016/j.cell.2010.03.009
257. Jensen KB, Darnell RB. CLIP: crosslinking and immunoprecipitation of in vivo RNA targets of RNA-binding proteins. Methods Mol Biol (2008) 488:85–98. doi:10.1007/978-1-60327-475-3_6
258. König J, Zarnack K, Rot G, Curk T, Kayikci M, Zupan B, et al. iCLIP reveals the function of hnRNP particles in splicing at individual nucleotide resolution. Nat Struct Mol Biol (2010) 17:909–15. doi:10.1038/nsmb.1838
259. Licatalosi DD, Mele A, Fak JJ, Ule J, Kayikci M, Chi SW, et al. HITS-CLIP yields genome-wide insights into brain alternative RNA processing. Nature (2008) 456:464–9. doi:10.1038/nature07488
260. Ule J, Jensen KB, Ruggiu M, Mele A, Ule A, Darnell RB. CLIP identifies Nova-regulated RNA networks in the brain. Science (2003) 302:1212–5. doi:10.1126/science.1090095
261. Morf J, Rey G, Schneider K, Stratmann M, Fujita J, Naef F, et al. Cold-inducible RNA-binding protein modulates circadian gene expression posttranscriptionally. Science (2012) 338:379–83. doi:10.1126/science.1217726
262. Reddy AB, Karp NA, Maywood ES, Sage EA, Deery M, O’Neill JS, et al. Circadian orchestration of the hepatic proteome. Curr Biol (2006) 16:1107–15. doi:10.1016/j.cub.2006.04.026
263. Tsuji T, Hirota T, Takemori N, Komori N, Yoshitane H, Fukuda M, et al. Circadian proteomics of the mouse retina. Proteomics (2007) 7:3500–8. doi:10.1002/pmic.200700272
264. Podobed P, Pyle WG, Ackloo S, Alibhai FJ, Tsimakouridze EV, Ratcliffe WF, et al. The day/night proteome in the murine heart. Am J Physiol Regul Integr Comp Physiol (2014) 307:R121–37. doi:10.1152/ajpregu.00011.2014
265. Akimoto H, Wu C, Kinumi T, Ohmiya Y. Biological rhythmicity in expressed proteins of the marine dinoflagellate Lingulodinium polyedrum demonstrated by chronological proteomics. Biochem Biophys Res Commun (2004) 315:306–12. doi:10.1016/j.bbrc.2004.01.054
266. Wagner V, Fiedler M, Markert C, Hippler M, Mittag M. Functional proteomics of circadian expressed proteins from Chlamydomonas reinhardtii. FEBS Lett (2004) 559:129–35. doi:10.1016/S0014-5793(04)00051-1
267. Choudhary MK, Nomura Y, Shi H, Nakagami H, Somers DE. Circadian profiling of the arabidopsis proteome using 2D-DIGE. Front Plant Sci (2016) 7:1007. doi:10.3389/fpls.2016.01007
268. Hwang H, Cho M-H, Hahn B-S, Lim H, Kwon Y-K, Hahn T-R, et al. Proteomic identification of rhythmic proteins in rice seedlings. Biochim Biophys Acta (2011) 1814:470–9. doi:10.1016/j.bbapap.2011.01.010
269. Brun V, Masselon C, Garin J, Dupuis A. Isotope dilution strategies for absolute quantitative proteomics. J Proteomics (2009) 72:740–9. doi:10.1016/j.jprot.2009.03.007
270. Mauvoisin D, Wang J, Jouffe C, Martin E, Atger F, Waridel P, et al. Circadian clock-dependent and -independent rhythmic proteomes implement distinct diurnal functions in mouse liver. Proc Natl Acad Sci U S A (2014) 111:167–72. doi:10.1073/pnas.1314066111
271. Robles MS, Cox J, Mann M. In-vivo quantitative proteomics reveals a key contribution of post-transcriptional mechanisms to the circadian regulation of liver metabolism. PLoS Genet (2014) 10:e1004047. doi:10.1371/journal.pgen.1004047
272. Chiang C-K, Mehta N, Patel A, Zhang P, Ning Z, Mayne J, et al. The proteomic landscape of the suprachiasmatic nucleus clock reveals large-scale coordination of key biological processes. PLoS Genet (2014) 10:e1004695. doi:10.1371/journal.pgen.1004695
273. Gerber SA, Rush J, Stemman O, Kirschner MW, Gygi SP. Absolute quantification of proteins and phosphoproteins from cell lysates by tandem MS. Proc Natl Acad Sci U S A (2003) 100:6940–5. doi:10.1073/pnas.0832254100
274. Hanke S, Besir H, Oesterhelt D, Mann M. Absolute SILAC for accurate quantitation of proteins in complex mixtures down to the attomole level. J Proteome Res (2008) 7:1118–30. doi:10.1021/pr7007175
275. Simicevic J, Schmid AW, Gilardoni PA, Zoller B, Raghav SK, Krier I, et al. Absolute quantification of transcription factors during cellular differentiation using multiplexed targeted proteomics. Nat Methods (2013) 10:570–6. doi:10.1038/nmeth.2441
276. Beynon RJ, Doherty MK, Pratt JM, Gaskell SJ. Multiplexed absolute quantification in proteomics using artificial QCAT proteins of concatenated signature peptides. Nat Methods (2005) 2:587–9. doi:10.1038/nmeth774
277. Mirzaei H, McBee JK, Watts J, Aebersold R. Comparative evaluation of current peptide production platforms used in absolute quantification in proteomics. Mol Cell Proteomics (2008) 7:813–23. doi:10.1074/mcp.M700495-MCP200
278. Stergachis AB, MacLean B, Lee K, Stamatoyannopoulos JA, MacCoss MJ. Rapid empirical discovery of optimal peptides for targeted proteomics. Nat Methods (2011) 8:1041–3. doi:10.1038/nmeth.1770
279. Shimizu Y, Inoue A, Tomari Y, Suzuki T, Yokogawa T, Nishikawa K, et al. Cell-free translation reconstituted with purified components. Nat Biotechnol (2001) 19:751–5. doi:10.1038/90802
280. Cox J, Hein MY, Luber CA, Paron I, Nagaraj N, Mann M. Accurate proteome-wide label-free quantification by delayed normalization and maximal peptide ratio extraction, termed MaxLFQ. Mol Cell Proteomics (2014) 13:2513–26. doi:10.1074/mcp.M113.031591
281. Deshmukh AS, Murgia M, Nagaraj N, Treebak JT, Cox J, Mann M. Deep proteomics of mouse skeletal muscle enables quantitation of protein isoforms, metabolic pathways, and transcription factors. Mol Cell Proteomics (2015) 14:841–53. doi:10.1074/mcp.M114.044222
282. Guerreiro ACL, Benevento M, Lehmann R, van Breukelen B, Post H, Giansanti P, et al. Daily rhythms in the cyanobacterium Synechococcus elongatus probed by high-resolution mass spectrometry-based proteomics reveals a small defined set of cyclic proteins. Mol Cell Proteomics (2014) 13:2042–55. doi:10.1074/mcp.M113.035840
283. Robles MS, Humphrey SJ, Mann M. Phosphorylation is a central mechanism for circadian control of metabolism and physiology. Cell Metab (2017) 25:118–27. doi:10.1016/j.cmet.2016.10.004
284. Reiland S, Messerli G, Baerenfaller K, Gerrits B, Endler A, Grossmann J, et al. Large-scale Arabidopsis phosphoproteome profiling reveals novel chloroplast kinase substrates and phosphorylation networks. Plant Physiol (2009) 150:889–903. doi:10.1104/pp.109.138677
285. Ang JE, Revell V, Mann A, Mäntele S, Otway DT, Johnston JD, et al. Identification of human plasma metabolites exhibiting time-of-day variation using an untargeted liquid chromatography-mass spectrometry metabolomic approach. Chronobiol Int (2012) 29:868–81. doi:10.3109/07420528.2012.699122
286. Chua EC-P, Shui G, Lee IT-G, Lau P, Tan L-C, Yeo S-C, et al. Extensive diversity in circadian regulation of plasma lipids and evidence for different circadian metabolic phenotypes in humans. Proc Natl Acad Sci U S A (2013) 110:14468–73. doi:10.1073/pnas.1222647110
287. Dallmann R, Viola AU, Tarokh L, Cajochen C, Brown SA. The human circadian metabolome. Proc Natl Acad Sci U S A (2012) 109:2625–9. doi:10.1073/pnas.1114410109
288. Kasukawa T, Sugimoto M, Hida A, Minami Y, Mori M, Honma S, et al. Human blood metabolite timetable indicates internal body time. Proc Natl Acad Sci U S A (2012) 109:15036–41. doi:10.1073/pnas.1207768109
289. Eckel-Mahan KL, Patel VR, Mohney RP, Vignola KS, Baldi P, Sassone-Corsi P. Coordination of the transcriptome and metabolome by the circadian clock. Proc Natl Acad Sci U S A (2012) 109:5541–6. doi:10.1073/pnas.1118726109
290. Fustin J-M, Doi M, Yamada H, Komatsu R, Shimba S, Okamura H. Rhythmic nucleotide synthesis in the liver: temporal segregation of metabolites. Cell Rep (2012) 1:341–9. doi:10.1016/j.celrep.2012.03.001
291. Minami Y, Kasukawa T, Kakazu Y, Iigo M, Sugimoto M, Ikeda S, et al. Measurement of internal body time by blood metabolomics. Proc Natl Acad Sci U S A (2009) 106:9890–5. doi:10.1073/pnas.0900617106
292. Patel VR, Eckel-Mahan K, Sassone-Corsi P, Baldi P. CircadiOmics: integrating circadian genomics, transcriptomics, proteomics and metabolomics. Nat Methods (2012) 9:772–3. doi:10.1038/nmeth.2111
293. Abbondante S, Eckel-Mahan KL, Ceglia NJ, Baldi P, Sassone-Corsi P. Comparative circadian metabolomics reveal differential effects of nutritional challenge in the serum and liver. J Biol Chem (2016) 291:2812–28. doi:10.1074/jbc.M115.681130
294. Eckel-Mahan KL, Patel VR, de Mateo S, Orozco-Solis R, Ceglia NJ, Sahar S, et al. Reprogramming of the circadian clock by nutritional challenge. Cell (2013) 155:1464–78. doi:10.1016/j.cell.2013.11.034
295. Hatori M, Vollmers C, Zarrinpar A, DiTacchio L, Bushong EA, Gill S, et al. Time-restricted feeding without reducing caloric intake prevents metabolic diseases in mice fed a high-fat diet. Cell Metab (2012) 15:848–60. doi:10.1016/j.cmet.2012.04.019
296. Chua EC-P, Shui G, Cazenave-Gassiot A, Wenk MR, Gooley JJ. Changes in plasma lipids during exposure to total sleep deprivation. Sleep (2015) 38:1683–91. doi:10.5665/sleep.5142
297. Davies SK, Ang JE, Revell VL, Holmes B, Mann A, Robertson FP, et al. Effect of sleep deprivation on the human metabolome. Proc Natl Acad Sci U S A (2014) 111:10761–6. doi:10.1073/pnas.1402663111
298. Giskeødegård GF, Davies SK, Revell VL, Keun H, Skene DJ. Diurnal rhythms in the human urine metabolome during sleep and total sleep deprivation. Sci Rep (2015) 5:14843. doi:10.1038/srep14843
299. Weljie AM, Meerlo P, Goel N, Sengupta A, Kayser MS, Abel T, et al. Oxalic acid and diacylglycerol 36:3 are cross-species markers of sleep debt. Proc Natl Acad Sci U S A (2015) 112:2569–74. doi:10.1073/pnas.1417432112
300. Su G, Burant CF, Beecher CW, Athey BD, Meng F. Integrated metabolome and transcriptome analysis of the NCI60 dataset. BMC Bioinformatics (2011) 12:S36. doi:10.1186/1471-2105-12-S1-S36
301. Sengupta A, Krishnaiah SY, Rhoades S, Growe J, Slaff B, Venkataraman A, et al. Deciphering the duality of clock and growth metabolism in a cell autonomous system using NMR profiling of the secretome. Metabolites (2016) 6:23. doi:10.3390/metabo6030023
302. Ueda HR, Chen W, Minami Y, Honma S, Honma K, Iino M, et al. Molecular-timetable methods for detection of body time and rhythm disorders from single-time-point genome-wide expression profiles. Proc Natl Acad Sci U S A (2004) 101:11227–32. doi:10.1073/pnas.0401882101
303. Martinez-Lozano Sinues P, Tarokh L, Li X, Kohler M, Brown SA, Zenobi R, et al. Circadian variation of the human metabolome captured by real-time breath analysis. PLoS One (2014) 9:e114422. doi:10.1371/journal.pone.0114422
304. Selfridge JM, Gotoh T, Schiffhauer S, Liu J, Stauffer PE, Li A, et al. Chronotherapy: intuitive, sound, founded…but not broadly applied. Drugs (2016) 76:1507–21. doi:10.1007/s40265-016-0646-4
305. Gygi SP, Rochon Y, Franza BR, Aebersold R. Correlation between protein and mRNA abundance in yeast. Mol Cell Biol (1999) 19:1720–30. doi:10.1128/MCB.19.3.1720
306. Sivan G, Kedersha N, Elroy-Stein O. Ribosomal slowdown mediates translational arrest during cellular division. Mol Cell Biol (2007) 27:6639–46. doi:10.1128/MCB.00798-07
307. Zong Q, Schummer M, Hood L, Morris DR. Messenger RNA translation state: the second dimension of high-throughput expression screening. Proc Natl Acad Sci U S A (1999) 96:10632–6. doi:10.1073/pnas.96.19.10632
308. Doyle JP, Dougherty JD, Heiman M, Schmidt EF, Stevens TR, Ma G, et al. Application of a translational profiling approach for the comparative analysis of CNS cell types. Cell (2008) 135:749–62. doi:10.1016/j.cell.2008.10.029
309. Heiman M, Schaefer A, Gong S, Peterson JD, Day M, Ramsey KE, et al. A translational profiling approach for the molecular characterization of CNS cell types. Cell (2008) 135:738–48. doi:10.1016/j.cell.2008.10.028
310. Sanz E, Yang L, Su T, Morris DR, McKnight GS, Amieux PS. Cell-type-specific isolation of ribosome-associated mRNA from complex tissues. Proc Natl Acad Sci U S A (2009) 106:13939–44. doi:10.1073/pnas.0907143106
311. Feldman JF. Lengthening the period of a biological clock in Euglena by cycloheximide, an inhibitor of protein synthesis. Proc Natl Acad Sci U S A (1967) 57:1080–7. doi:10.1073/pnas.57.4.1080
312. Ingolia NT, Ghaemmaghami S, Newman JRS, Weissman JS. Genome-wide analysis in vivo of translation with nucleotide resolution using ribosome profiling. Science (2009) 324:218–23. doi:10.1126/science.1168978
313. Jang C, Lahens NF, Hogenesch JB, Sehgal A. Ribosome profiling reveals an important role for translational control in circadian gene expression. Genome Res (2015) 25:1836–47. doi:10.1101/gr.191296.115
314. Janich P, Arpat AB, Castelo-Szekely V, Lopes M, Gatfield D. Ribosome profiling reveals the rhythmic liver translatome and circadian clock regulation by upstream open reading frames. Genome Res (2015) 25:1848–59. doi:10.1101/gr.195404.115
315. Jouffe C, Cretenet G, Symul L, Martin E, Atger F, Naef F, et al. The circadian clock coordinates ribosome biogenesis. PLoS Biol (2013) 11:e1001455. doi:10.1371/journal.pbio.1001455
316. Fishman B, Wurtman RJ, Munro HN. Daily rhythms in hepatic polysome profiles and tyrosine transaminase activity: role of dietary protein. Proc Natl Acad Sci U S A (1969) 64:677–82. doi:10.1073/pnas.64.2.677
317. Uchiyama Y, Asari A. A morphometric study of the variations in subcellular structures of rat hepatocytes during 24 hours. Cell Tissue Res (1984) 236:305–15. doi:10.1007/BF00214231
318. Cao R, Li A, Cho H, Lee B, Obrietan K. Mammalian target of rapamycin signaling modulates photic entrainment of the suprachiasmatic circadian clock. J Neurosci (2010) 30:6302–14. doi:10.1523/JNEUROSCI.5482-09.2010
319. Cao R, Robinson B, Xu H, Gkogkas C, Khoutorsky A, Alain T, et al. Translational control of entrainment and synchrony of the suprachiasmatic circadian clock by mTOR/4E-BP1 signaling. Neuron (2013) 79:712–24. doi:10.1016/j.neuron.2013.06.026
320. Cornu M, Oppliger W, Albert V, Robitaille AM, Trapani F, Quagliata L, et al. Hepatic mTORC1 controls locomotor activity, body temperature, and lipid metabolism through FGF21. Proc Natl Acad Sci U S A (2014) 111:11592–9. doi:10.1073/pnas.1412047111
321. Atger F, Gobet C, Marquis J, Martin E, Wang J, Weger B, et al. Circadian and feeding rhythms differentially affect rhythmic mRNA transcription and translation in mouse liver. Proc Natl Acad Sci U S A (2015) 112:E6579–88. doi:10.1073/pnas.1515308112
322. Damiola F, Le Minh N, Preitner N, Kornmann B, Fleury-Olela F, Schibler U. Restricted feeding uncouples circadian oscillators in peripheral tissues from the central pacemaker in the suprachiasmatic nucleus. Genes Dev (2000) 14:2950–61. doi:10.1101/gad.183500
323. Hara R, Wan K, Wakamatsu H, Aida R, Moriya T, Akiyama M, et al. Restricted feeding entrains liver clock without participation of the suprachiasmatic nucleus. Genes Cells (2001) 6:269–78. doi:10.1046/j.1365-2443.2001.00419.x
324. Izumo M, Pejchal M, Schook AC, Lange RP, Walisser JA, Sato TR, et al. Differential effects of light and feeding on circadian organization of peripheral clocks in a forebrain Bmal1 mutant. Elife (2014) 3. doi:10.7554/eLife.04617
325. Sabath E, Salgado-Delgado R, Guerrero-Vargas NN, Guzman-Ruiz MA, del Carmen Basualdo M, Escobar C, et al. Food entrains clock genes but not metabolic genes in the liver of suprachiasmatic nucleus lesioned rats. FEBS Lett (2014) 588:3104–10. doi:10.1016/j.febslet.2014.06.045
326. Stokkan KA, Yamazaki S, Tei H, Sakaki Y, Menaker M. Entrainment of the circadian clock in the liver by feeding. Science (2001) 291:490–3. doi:10.1126/science.291.5503.490
327. Barbosa C, Peixeiro I, Romão L. Gene expression regulation by upstream open reading frames and human disease. PLoS Genet (2013) 9:e1003529. doi:10.1371/journal.pgen.1003529
328. Schleich S, Strassburger K, Janiesch PC, Koledachkina T, Miller KK, Haneke K, et al. DENR-MCT-1 promotes translation re-initiation downstream of uORFs to control tissue growth. Nature (2014) 512:208–12. doi:10.1038/nature13401
329. Skabkin MA, Skabkina OV, Hellen CUT, Pestova TV. Reinitiation and other unconventional post-termination events during eukaryotic translation. Mol Cell (2013) 51:249–64. doi:10.1016/j.molcel.2013.05.026
330. Harrison DA. Sex determination: controlling the master. Curr Biol (2007) 17:R328–30. doi:10.1016/j.cub.2007.03.012
331. Salz HK, Erickson JW. Sex determination in Drosophila: the view from the top. Fly (Austin) (2010) 4:60–70. doi:10.4161/fly.4.1.11277
332. Wilhelm JE, Smibert CA. Mechanisms of translational regulation in Drosophila. Biol Cell (2005) 97:235–52. doi:10.1042/BC20040097
333. Medenbach J, Seiler M, Hentze MW. Translational control via protein-regulated upstream open reading frames. Cell (2011) 145:902–13. doi:10.1016/j.cell.2011.05.005
334. Tanenbaum ME, Stern-Ginossar N, Weissman JS, Vale RD. Regulation of mRNA translation during mitosis. Elife (2015) 4. doi:10.7554/eLife.07957
335. Yan X, Hoek TA, Vale RD, Tanenbaum ME. Dynamics of translation of single mRNA molecules in vivo. Cell (2016) 165:976–89. doi:10.1016/j.cell.2016.04.034
336. Xu Y, Ma P, Shah P, Rokas A, Liu Y, Johnson CH. Non-optimal codon usage is a mechanism to achieve circadian clock conditionality. Nature (2013) 495:116–20. doi:10.1038/nature11942
337. Zhou M, Guo J, Cha J, Chae M, Chen S, Barral JM, et al. Non-optimal codon usage affects expression, structure and function of clock protein FRQ. Nature (2013) 495:111–5. doi:10.1038/nature11833
338. Fu J, Murphy KA, Zhou M, Li YH, Lam VH, Tabuloc CA, et al. Codon usage affects the structure and function of the Drosophila circadian clock protein PERIOD. Genes Dev (2016) 30:1761–75. doi:10.1101/gad.281030.116
339. Lim C, Allada R. ATAXIN-2 activates PERIOD translation to sustain circadian rhythms in Drosophila. Science (2013) 340:875–9. doi:10.1126/science.1234785
340. Zhang Y, Ling J, Yuan C, Dubruille R, Emery P. A role for Drosophila ATX2 in activation of PER translation and circadian behavior. Science (2013) 340:879–82. doi:10.1126/science.1234746
341. Tuin I, Voss U, Kang J-S, Kessler K, Rüb U, Nolte D, et al. Stages of sleep pathology in spinocerebellar ataxia type 2 (SCA2). Neurology (2006) 67:1966–72. doi:10.1212/01.wnl.0000247054.90322.14
342. Velázquez-Pérez L, Voss U, Rodríguez-Labrada R, Auburger G, Canales Ochoa N, Sánchez Cruz G, et al. Sleep disorders in spinocerebellar ataxia type 2 patients. Neurodegener Dis (2011) 8:447–54. doi:10.1159/000324374
343. Funato H, Miyoshi C, Fujiyama T, Kanda T, Sato M, Wang Z, et al. Forward-genetics analysis of sleep in randomly mutagenized mice. Nature (2016) 539(7629):378–83. doi:10.1038/nature20142
344. Sung YH, Baek I-J, Kim DH, Jeon J, Lee J, Lee K, et al. Knockout mice created by TALEN-mediated gene targeting. Nat Biotechnol (2013) 31:23–4. doi:10.1038/nbt.2477
345. Carbery ID, Ji D, Harrington A, Brown V, Weinstein EJ, Liaw L, et al. Targeted genome modification in mice using zinc-finger nucleases. Genetics (2010) 186:451–9. doi:10.1534/genetics.110.117002
346. Hsu PD, Lander ES, Zhang F. Development and applications of CRISPR-Cas9 for genome engineering. Cell (2014) 157:1262–78. doi:10.1016/j.cell.2014.05.010
347. Wang H, Yang H, Shivalila CS, Dawlaty MM, Cheng AW, Zhang F, et al. One-step generation of mice carrying mutations in multiple genes by CRISPR/Cas-mediated genome engineering. Cell (2013) 153:910–8. doi:10.1016/j.cell.2013.04.025
348. East-Seletsky A, O’Connell MR, Knight SC, Burstein D, Cate JHD, Tjian R, et al. Two distinct RNase activities of CRISPR-C2c2 enable guide-RNA processing and RNA detection. Nature (2016) 538:270–3. doi:10.1038/nature19802
349. Fonfara I, Richter H, Bratovič M, Le Rhun A, Charpentier E. The CRISPR-associated DNA-cleaving enzyme Cpf1 also processes precursor CRISPR RNA. Nature (2016) 532:517–21. doi:10.1038/nature17945
350. Fu Y, Sander JD, Reyon D, Cascio VM, Joung JK. Improving CRISPR-Cas nuclease specificity using truncated guide RNAs. Nat Biotechnol (2014) 32:279–84. doi:10.1038/nbt.2808
351. Ran FA, Hsu PD, Wright J, Agarwala V, Scott DA, Zhang F. Genome engineering using the CRISPR-Cas9 system. Nat Protoc (2013) 8:2281–308. doi:10.1038/nprot.2013.143
352. Ranganathan V, Wahlin K, Maruotti J, Zack DJ. Expansion of the CRISPR-Cas9 genome targeting space through the use of H1 promoter-expressed guide RNAs. Nat Commun (2014) 5:4516. doi:10.1038/ncomms5516
353. Zetsche B, Gootenberg JS, Abudayyeh OO, Slaymaker IM, Makarova KS, Essletzbichler P, et al. Cpf1 is a single RNA-guided endonuclease of a class 2 CRISPR-Cas system. Cell (2015) 163:759–71. doi:10.1016/j.cell.2015.09.038
354. Sunagawa GA, Sumiyama K, Ukai-Tadenuma M, Perrin D, Fujishima H, Ukai H, et al. Mammalian reverse genetics without crossing reveals Nr3a as a short-sleeper gene. Cell Rep (2016) 14:662–77. doi:10.1016/j.celrep.2015.12.052
355. Tatsuki F, Sunagawa GA, Shi S, Susaki EA, Yukinaga H, Perrin D, et al. Involvement of Ca(2+)-dependent hyperpolarization in sleep duration in mammals. Neuron (2016) 90:70–85. doi:10.1016/j.neuron.2016.02.032
356. Tainaka K, Kuno A, Kubota SI, Murakami T, Ueda HR. Chemical principles in tissue clearing and staining protocols for whole-body cell profiling. Annu Rev Cell Dev Biol (2016) 32:713–41. doi:10.1146/annurev-cellbio-111315-125001
357. Chung K, Deisseroth K. CLARITY for mapping the nervous system. Nat Methods (2013) 10:508–13. doi:10.1038/nmeth.2481
358. Dodt H-U, Leischner U, Schierloh A, Jährling N, Mauch CP, Deininger K, et al. Ultramicroscopy: three-dimensional visualization of neuronal networks in the whole mouse brain. Nat Methods (2007) 4:331–6. doi:10.1038/nmeth1036
359. Ertürk A, Becker K, Jährling N, Mauch CP, Hojer CD, Egen JG, et al. Three-dimensional imaging of solvent-cleared organs using 3DISCO. Nat Protoc (2012) 7:1983–95. doi:10.1038/nprot.2012.119
360. Pan C, Cai R, Quacquarelli FP, Ghasemigharagoz A, Lourbopoulos A, Matryba P, et al. Shrinkage-mediated imaging of entire organs and organisms using uDISCO. Nat Methods (2016) 13:859–67. doi:10.1038/nmeth.3964
361. Susaki EA, Tainaka K, Perrin D, Kishino F, Tawara T, Watanabe TM, et al. Whole-brain imaging with single-cell resolution using chemical cocktails and computational analysis. Cell (2014) 157:726–39. doi:10.1016/j.cell.2014.03.042
362. Yang B, Treweek JB, Kulkarni RP, Deverman BE, Chen C-K, Lubeck E, et al. Single-cell phenotyping within transparent intact tissue through whole-body clearing. Cell (2014) 158:945–58. doi:10.1016/j.cell.2014.07.017
363. Hughes ME, Hogenesch JB, Kornacker K. JTK_CYCLE: an efficient nonparametric algorithm for detecting rhythmic components in genome-scale data sets. J Biol Rhythms (2010) 25:372–80. doi:10.1177/0748730410379711
364. Yang R, Su Z. Analyzing circadian expression data by harmonic regression based on autoregressive spectral estimation. Bioinformatics (2010) 26:i168–74. doi:10.1093/bioinformatics/btq189
Keywords: systems biology, models, theory, RNA sequencing, neurophysiology, circadian rhythm, ribosome profiling
Citation: Millius A and Ueda HR (2017) Systems Biology-Derived Discoveries of Intrinsic Clocks. Front. Neurol. 8:25. doi: 10.3389/fneur.2017.00025
Received: 25 November 2016; Accepted: 17 January 2017;
Published: 06 February 2017
Edited by:
Timo Partonen, National Institute for Health and Welfare, FinlandReviewed by:
Zheng (Jake) Chen, University of Texas Health Science Center at Houston, USAAngela Moreira Borralho Relógio, Charité Medical University of Berlin, Germany
Copyright: © 2017 Millius and Ueda. This is an open-access article distributed under the terms of the Creative Commons Attribution License (CC BY). The use, distribution or reproduction in other forums is permitted, provided the original author(s) or licensor are credited and that the original publication in this journal is cited, in accordance with accepted academic practice. No use, distribution or reproduction is permitted which does not comply with these terms.
*Correspondence: Hiroki R. Ueda, uedah-tky@umin.ac.jp