- Advanced MRI Section, Laboratory of Functional and Molecular Imaging, National Institute of Neurological Disorders and Stroke, National Institutes of Health, Bethesda, MD, USA
Modern neuroimaging methods may provide unique insights into the mechanism and role of sleep, as well as into particular mechanisms of brain function in general. Many of the recent neuroimaging studies have used concurrent EEG and fMRI, which present unique technical challenges ranging from the difficulty of inducing sleep in the MRI environment to appropriate instrumentation and data processing methods to obtain artifact free data. In addition, the use of EEG-fMRI during sleep leads to unique data interpretation issues, as common approaches developed for the analysis of task-evoked activity do not apply to sleep. Reviewed are a variety of statistical approaches that can be used to characterize brain activity from fMRI data acquired during sleep, with an emphasis on approaches that investigate the presence of correlated activity between brain regions. Each of these approaches has advantages and disadvantages that must be considered in concert with the theoretical questions of interest. Specifically, fundamental theories of sleep control and function should be considered when designing these studies and when choosing the associated statistical approaches. For example, the notion that local brain activity during sleep may be triggered by local, use-dependent activity during wakefulness may be tested by analyzing sleep networks as statistically independent components. Alternatively, the involvement of regions in more global processes such as arousal may be investigated with correlation analysis.
Introduction
Across the sleep-wake cycle, the human brain displays a wide range of behaviors that have long intrigued scientists. Rather than simply being a state of reduced wakefulness, sleep is now understood as a complex state that is “by, for, and of the brain” (Hobson, 2005) and that has a characteristic signature of neuro-electrical and metabolic activity. What does this activity represent, how is it orchestrated, and how does it support the functions of sleep? Answering these questions is not only important for sleep research but may also provide clues about brain function in general.
Over the years, researchers have used a variety of approaches to study the relationship between sleep and brain activity. With time, these approaches have become increasingly sophisticated. Before the invention of electroencephalography (EEG), researchers studied sleep by examining its behavioral characteristics (e.g., arousal threshold). An increased arousal threshold was understood to equate to an increased sleep “depth” and reduced brain activity. However, determining arousal threshold had a significant limitation because it required the disruption of sleep. The use of EEG as a surrogate for the behavioral definition then gained acceptance because of a strong correlation between EEG slow-waves and arousal threshold (Blake and Gerard, 1937). This was very important for sleep researchers because they could study sleep without disrupting it.
Nevertheless, it was soon realized that there were limitations to the characterization of sleep based on arousal threshold and the level of EEG slow-wave activity alone. Importantly, the discovery of REM sleep in the 1950s (Aserinsky and Kleitman, 1953) made it clear that the sleep-wake cycle was more than a gradual variation in global (i.e., whole brain) activity that was indexed by arousal threshold. In addition, it became increasingly apparent that the EEG had significant limitations in capturing changes in spatial activity patterns due to difficulties in interpretation and poor localization.
The ability to spatially localize brain signals reflecting neural activity improved dramatically in the 1990s with the advent of neuroimaging techniques based on single photon emission computed tomography (SPECT; Kuhl et al., 1976), positron emission tomography (PET, Phelps et al., 1981), and Blood Oxygenation Level Dependent functional MRI (BOLD fMRI; Kwong et al., 1992; Ogawa et al., 1992). Early PET studies of sleep have demonstrated regional metabolic variations across the sleep-wake cycle (e.g., Maquet et al., 1996; Braun et al., 1997), confirming early indications from EEG studies that sleep is more than a global change in activity.
BOLD fMRI is the most recent neuroimaging method applied to sleep and it has specific advantages over PET and SPECT because it is entirely non-invasive and has superior spatial and temporal resolution. These characteristics offer the opportunity to localize sleep-specific changes in brain activity and also to examine the network dynamics associated with the functions of sleep (e.g., learning and memory). Thus, combined with EEG, fMRI may be a powerful tool to characterize sleep and study its functions. In the following, methods for concurrent EEG-fMRI will be discussed, focusing on data analysis and highlighting applications to the study of sleep. Emphasis will be on introducing specific methods and exemplary work rather than providing a comprehensive review.
What Do EEG and fMRI Measure?
It is interesting to consider that, although EEG and fMRI have been around for decades, the origin of their signals and relationship to behavior remain poorly understood. One reason for this is the fact that much of the exquisite detail in the spatio-temporal patterns of the brain’s electrical activity is lost when observed through the macroscopic measures of EEG and fMRI. For example, the spatial resolution of these methods is at the millimeters scale, equivalent to assemblies of hundreds of thousands to millions of neurons. Considering the range of behaviors available to simple creatures such as the roundworm and the fruit fly, whose brains have about 300 and 100,000 neurons respectively, it becomes clear that the interpretation of EEG and fMRI is challenging and requires gross simplification of the underlying networks. A popular simplification is to model the brain as a collection of interconnected functional processing modules, each with a distinct function subserved by a large assembly of several classes of neurons.
The macroscopic signals measured with BOLD fMRI are the result of a blood flow response that overcompensates for the increased oxygen consumption required for neural activity. A working hypothesis is that, in most brain regions, the fMRI signal is coupled to the level of excitatory and inhibitory synaptic transmission (Jueptner and Weiller, 1995; Attwell and Laughlin, 2001; Logothetis et al., 2001; Logothetis, 2008) and may reflect a brain region’s level of local processing (Attwell and Iadecola, 2002). Measurements in the motor cortex suggest that the contribution of inhibitory synapses to the neocortical fMRI signal may be small (Waldvogel et al., 2000); however, this finding may not generalize to all brain regions. Thus, in principle, increased fMRI signal resulting from increased synaptic activity in a region may be associated with reduced, constant, or increased spiking activity (e.g., Lauritzen, 2001). Conversely, in regions with ineffective neurovascular control, brain activity may not lead to a significant BOLD signal. Furthermore, depending on the local circuitry, bulk changes in synaptic transmission that lead to fMRI signal changes may represent a variety of processes with differing behavioral relevance, including local processing and more global modulatory effects of attention and arousal.
Although it was originally used to study task-evoked activity, an interesting, somewhat more recent, and rapidly growing application of fMRI is the study of spontaneous brain activity, i.e., activity that is not evoked by explicit tasks (Biswal et al., 1995; for review see: Auer, 2008). Such work has shown that much of the brain is continually active with activity patterns that are highly correlated between regions with known anatomical and implied functional connectivity (e.g., Honey et al., 2009). Analysis of spontaneous activity may reveal the functional connectivity of the brain, and its potential alteration during various cognitive and behavioral states, including sleep. Thus, the study of spontaneous brain activity with fMRI may be a particularly attractive tool for sleep research.
The spatial resolution of fMRI is limited by instrumental and physiological factors. The instrumental factors relate to the intrinsic MRI sensitivity, as increasing MRI resolution results in higher image noise levels. This limits the resolution to about 1.5–2.0 mm on modern 3T systems, and about 1.0 mm on 7T systems (Triantafyllou et al., 2005). The physiological factors relate to the fact that the BOLD fMRI signal is filtered by the vasculature; despite the fact that neurovascular control may be spatially highly specific (e.g., Duong et al., 2001; Iadecola and Nedergaard, 2007), dispersion of the resulting blood oxygenation change across the venous vasculature leads to spatial and temporal blurring (Turner, 2002; de Zwart et al., 2005). This generally limits the spatial and temporal resolution to 1–2 mm (Shmuel et al., 2007) and 3–4 s respectively (Turner, 2002; de Zwart et al., 2005).
A loss in spatial and temporal resolution of neuro-electrical signals also occurs with EEG, although to a different extent and through different mechanisms. Volume conduction and spatial summation result in a loss of most neuro-electrical-based information when measured from the scalp. Scalp signals, therefore, are strongly biased by neuronal architecture and primarily reflect activity from large neuronal populations with a long and highly anisotropic arrangement of dendrites (Hamalainen et al., 1993). For this reason, the dominant signals in the EEG are generally the lower frequency (0–30 Hz) signals, which primarily reflect the modulation of large cortical areas. Typically, EEG spatial and temporal resolutions are limited to 1 cm and 10 ms respectively. As a result, the EEG signal may relate to neuronal activity associated with local cortical processing in a complicated and non-stationary fashion. Higher frequency signals on the other hand may more directly reflect processing in focal regions but are more difficult to detect due to their small amplitude and the extensive averaging required for their measurement. This is particularly the case for sources deeper in the brain (away from the detectors on the scalp), some of which may be invisible to the EEG. Thus, both EEG and fMRI signals are difficult to interpret and their comparison is complicated by the fact that they represent different aspects of neuronal activity.
The EEG-fMRI Experiment
The simultaneous measurement of both EEG and fMRI signals has potential advantages for the study of brain activity because each method contributes unique information (e.g., Ritter and Villringer, 2006). For sleep, this combination allows the characterization of various sleep features and “stages” using classic, EEG-based criteria, as well as correlative analysis between EEG features and the fMRI signal. This potentially provides novel information about sleep that is not available with each method alone. In this section, some of the practical aspects of performing EEG in the fMRI environment will be briefly discussed.
Because of both safety and performance issues, EEG-fMRI requires specialized, MRI-compatible EEG hardware. Specifically, the EEG equipment that goes into the MRI scan room needs to be non-magnetic, and it needs to have features to either avoid significant electric voltages being induced on the EEG leads or minimize their associated currents. The latter may cause harm to the subject, distort the EEG signals, and damage the EEG signal amplifiers. Such voltages could result from the radiofrequency fields or the switching of the gradient fields associated with MRI image acquisition (e.g., Lemieux et al., 1997). Proper geometric arrangement of the electrode leads and the use of adequately resistive electrode material or built-in resistors that limit electrode currents can minimize safety hazards.
The fMRI environment negatively impacts EEG data quality because of gradient switching artifacts and cardio-ballistic artifacts. Both of these artifacts can be dealt with effectively in post-processing, recovering much of the data quality of EEG performed outside the scanner. Successful removal of gradient artifacts requires sufficient dynamic range of the EEG amplifiers and digitizers, and may benefit from precise synchronization of the EEG and MRI digitizers (see below). The latter is achieved by use of a synchronization signal derived from the MRI scan clock. To a lesser extent, the EEG may affect MRI data quality in the form of magnetic susceptibility artifacts resulting from disruptions of the magnetic field by the electrodes. This effect is generally negligible. In summary, modern technology allows concurrent measurement of EEG and fMRI without significant loss in data quality, compared to EEG or fMRI performed in isolation, while offering novel opportunities for the study of sleep.
With regard to the study of sleep, another practical concern with concurrent EEG-MRI, and with MRI in general, is subject discomfort. The MRI environment is not very conducive to sleep because there is substantial acoustic noise and the subject must lay still in a position that generally grows uncomfortable over time. For this reason, the study of extended periods of sleep, and of deep sleep in particular, may require sleep depriving the subjects. Nevertheless, it may be possible to facilitate sleep inside the scanner by techniques that derive from our understanding of sleep physiology. Subjects should be screened for even minor sleep difficulties (e.g., sleep-initiation insomnia). Although subjects may not have a diagnosis of a psychological disorder, they may have a subclinical presentation of the disorder that would prevent them from falling asleep during fMRI. Subjects should follow strict “sleep hygiene” practices in the days or weeks preceding the study. This includes maintaining a regular sleep schedule (a constant bedtime and wake time) and avoiding long naps. Adherence to this schedule can be monitored with actigraphy. These procedures would facilitate the ability of subjects to reach slow-wave sleep and REM sleep without sleep deprivation.
The acoustic noise inherent to MRI scanning can be mitigated in a variety of ways that can be used in combination. First, one can employ barrier protection (e.g., insert earplugs). Second, one can use active noise cancelation (use of an additional noise source that destructively interferes with the scanner noise). Outside the MRI environment, this approach has been used successfully to reduce the acoustic noise associated with transcranial magnetic stimulation (Massimini et al., 2005), and recently, commercial systems for use in the MRI environment have become available (e.g., Chambers et al., 2007). Third, the acoustic noise associated with scanning can be decreased by lowering the spatial resolution and decreasing the gradient switching speed (e.g., Horovitz et al., 2009). However, the trade-off for this approach is generally a decreased spatial resolution. Fourth, some investigators have gone as far as attaching a polyurethane “acoustic hood” to the magnet bore (Fransson et al., 2009).
Analysis of EEG-fMRI Data
The analysis of concurrently acquired EEG and fMRI data can proceed along various ways, depending on the research question at hand. A schematic of the most common processing pathways and major steps is given in the Figure 1. Common to all approaches is pre-processing for artifact removal from both EEG and fMRI data, as will be discussed in the following two sections. The third section in this chapter will discuss the direct comparison of features in the EEG signals with fMRI activity. Further analysis of fMRI data for the study of correlation and independence between brain regions during various stages of sleep will be discussed in the next chapter.
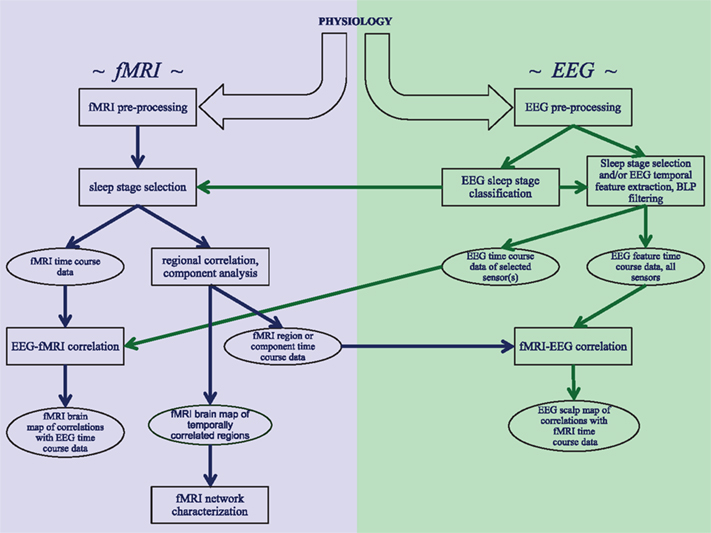
Figure 1. Schematic overview of the analysis strategies and processing steps that are discussed in this review. Three distinct analysis approaches are shown: investigating correlations within fMRI data from sleep stages identified with EEG, correlating EEG features with fMRI data, and correlating fMRI features with EEG data.
EEG Pre-Processing
An important first step in the analysis of concurrently acquired EEG and fMRI data is the removal of artifacts that are inherent to the individual modalities or result from interference caused by their combination. As indicated above, cardio-ballistic and gradient artifacts are among the strongest signals seen in EEG acquired in the MRI environment. They result from electro-motive forces in the conductive EEG leads, which in turn result from MRI gradient switching and from small electrode movements due to cardiac pulsations (Goldman et al., 2000). Fortunately, both can be removed almost entirely by dedicated processing methods (Laufs et al., 2008). For example, gradient artifacts can be effectively removed by template subtraction (Mandelkow et al., 2006; Grouiller et al., 2007; Laufs et al., 2008; Moosmann et al., 2009), provided amplifiers with sufficient dynamic range are used. Cardio-ballistic artifacts, on the other hand, may be better removed by independent component analysis (Liu et al., 2002; Benar et al., 2003; Vanderperren et al., 2010), as they may be too variable for template subtraction methods to be adequately effective. It is possible to do this effectively without significantly affecting the brain signals (e.g., Liu et al., 2002).
After removal of EEG artifacts that are caused or enhanced by the MRI environment, some additional pre-processing may be performed including eye-blink removal, notch filtering to remove line frequency artifacts (at 50 or 60 Hz and their harmonics), and low-pass filtering (below about 100 Hz) to reduce the contribution of thermal noise and instrumental drifts. Generally, EEG data are also high-pass filtered to reduce the effects of instrumental drift and saturation of the amplifiers. This is done either implicitly (by the amplifier electronics) or explicitly (during pre-processing) at 1 Hz or below.
fMRI Pre-Processing
MRI pre-processing includes standard fMRI-specific artifact reduction and corrections for geometric distortion and slice timing differences. Furthermore, head motion correction is performed through image registration procedures. Although these pre-processing steps substantially improve the temporal stability of the fMRI data, they do not fully remove the contributions of non-neuronal signal sources such as head motion, the respiratory and cardiac cycles, and instrumental instabilities that manifest as slow signal drift.
Separating these slow, non-neurogenic signal fluctuations from neurogenic signals can be particularly problematic for the study of spontaneous activity during sleep as one does not have an a priori model for the neuronal activity as is available for studies of task-evoked activity. Additionally, task-evoked studies generally have the flexibility to optimize the task paradigm in order to minimize the contribution from non-neuronal signal sources. For example, a paradigm with rapid alternation of rest and active periods facilitates the distinction between rapid neurogenic signals from slow extraneous signals.
A popular way to eliminate non-neurogenic signals from sleep data is through regression analysis. For this purpose, one or more signals (regressors) are chosen to represent non-neuronal sources, which can then be used with multi-linear regression analysis and projected out of the data (see e.g., Bianciardi et al., 2009a). Regressor signals can be derived from various sources, including separately acquired peripheral signals representing pulmonary and cardiac cycles (e.g., from respiration bellows and pulse oximeters), head motion signals derived from the motion correction pre-processing, and fMRI signals in regions of interest [ROI; e.g., cerebro-spinal fluid (CSF) or white matter] or averaged over the entire brain. To represent instrumental drift one can further include a range of low-order polynomials.
Although regression analysis can aid the separation of non-neurogenic from neurogenic signals, its effectiveness is difficult to quantify and may be quite variable. For example, the regression may inadvertently remove neurogenic signal or incompletely remove non-neurogenic signal. The former is possible when some of the regressors are contaminated with neurogenic signal, for example when the CSF or white matter ROI contain signal from venous blood draining from active regions (e.g., Bianciardi et al., 2011). Another example is the use of the “global” brain signal as a regressor, potentially introducing artifactual correlation in the data (see e.g., Fox et al., 2009). Alternatively, it is possible that some neurogenic signal is captured with the polynomial “drift” regressors. Incomplete removal of non-neurogenic signal can occur when it is not fully represented in the regressors.
Combined Analysis of EEG and fMRI Data
After pre-processing of EEG and fMRI data for artifact removal, combined analysis can be performed by comparing certain EEG features with fMRI data, which effectively combines the superior spatial resolution and coverage of fMRI with the good temporal resolution of EEG. Such an analysis can in principle be done in various ways, and how to precisely do this (which EEG features to select, and how to compare them with fMRI) remains an active area of research. The fact that this issue has not been resolved is largely due to an incomplete understanding of the underlying signal sources and a potentially circuit- and context-specific relationship between the neuro-electric and metabolic signals (e.g., Maier et al., 2008). Nevertheless, the various approaches to comparing EEG and fMRI signals that have been used most frequently will be briefly reviewed.
As the raw EEG signal may be rather noisy, and its absolute level may be prone to artifacts (e.g., motion and slow drifts), most analysis methods perform feature extraction prior to comparison with fMRI. The temporal incidence of such features is then compared to the BOLD fMRI signal on a pixel-by-pixel or region-by-region basis. This is generally done after convolution with a canonical hemodynamic response function (HRF), which represents the BOLD temporal response as an impulse of neuronal activity. In the following, some of the features that can be derived from the EEG signal will be discussed.
A feature often extracted from the EEG signal is band-limited power (BLP; e.g., Goldman et al., 2002; Laufs et al., 2003; Leopold et al., 2003), which reflects instantaneous activity in a specific frequency band. Extraction of the BLP can be done by band-pass filtering the entire EEG recording or by performing time-frequency analysis on relatively short time segments, not exceeding the duration of the hemodynamic response. The rationale for this approach is that EEG BLP should have a neuro-metabolic correlate that would be reflected in the fMRI signal. A caveat with this analysis is not all of the EEG activity is oscillatory, and therefore, some information is lost. Alternatively, and relevant to the study of sleep, it is possible to extract temporal EEG graphoelements, monitor their temporal incidence, and after convolution with the HRF, correlate them with the fMRI signal. This has been done with K-complexes (Czisch et al., 2009), slow-waves (Dang-Vu et al., 2008), infraslow-waves (Picchioni et al., 2011), and sleep spindles (Schabus et al., 2007; Andrade et al., 2011). The study conducted by Andrade and colleagues is particularly unique because it was designed to measure the changes in functional connectivity as a function of spindle activity. Finally, several other physiological phenomena that occur during sleep can be used as the event onset for event-related fMRI analyses such as eye movements during REM sleep (Wehrle et al., 2005; Hong et al., 2009; Miyauchi et al., 2009). In addition, during lucid dreaming, the onset of a predefined dreamed motor movement can be signaled to the investigators with changes in the electro-oculogram, and they can be used as onset markers in the fMRI analysis (Dresler et al., 2011).
Both BLP and temporal EEG graphoelement extraction are usually performed on an electrode-by-electrode basis without taking the scalp distribution of the EEG into account; EEG information is derived from a single spatial location, whereas fMRI information is spatially diverse. This means that correlations between the two are not matched in space. One method to address this disadvantage is to use source localization techniques such as LORETA. Another method to address this disadvantage is to extract so-called “microstates” (Wackermann et al., 1993; Britz et al., 2010; Musso et al., 2010), which are a few dominant scalp patterns that the brain appears to generate consistently. The temporal occurrence of each of these patterns is then correlated (again after convolving with the HRF) with the fMRI signal, revealing reproducible fMRI patterns. Lastly, the reverse is possible as well: a signal time course of a brain region can be extracted from the fMRI data and correlated against EEG features such as BLP or other spectral characteristics. Such fMRI signals can be extracted using, e.g., seed analysis or independent component analysis (e.g., Mantini et al., 2007; Sadaghiani et al., 2010) as will be discussed below. The purpose of this analysis would be to investigate which EEG characteristics underlie the fMRI signal variations.
An advantage of combined analysis over analyzing the fMRI and EEG signals independently would be the potential for an improved understanding of the neuronal processes underlying the generation of the signals in the individual modalities. For example, comparison of the fMRI with the EEG signals aids in separating neurogenic from non-neurogenic contributions to the fMRI data, the latter of which would presumably be absent from the EEG signal. A caveat with this analysis is that EEG may not capture much of the brain activity that is represented in fMRI and vice versa. In fact, reported EEG-fMRI correlations are generally low (correlation values of below about 0.3) suggesting they represent the minority of the variance in the signals.
Exploring Functional Connections with fMRI Data
After pre-processing, the EEG data can be simply used to classify the classical sleep stages (e.g., Rechtschaffen and Kales, 1968), after which stage-specific features in the fMRI data can be analyzed. Such features may include local fMRI activity levels, or fMRI spectral characteristics (see e.g., He et al., 2010; He, 2011). In addition, one can further analyze the fMRI data to identify stage-specific network activity that may originate from distinct sleep processes. The two sections in this chapter will discuss two approaches for further fMRI data analysis: one that aims at identifying functionally connected brain regions, and one that aims at identifying functionally independent networks of brain regions.
Finding Functional Connections with Seed Region Correlation
During sleep, widespread changes in brain activity occur, and many of these changes are coordinated across many cortical and subcortical regions. At the cellular level, most brain regions see decreases in the concentration of neurotransmitters such as serotonin, norepinephrine, and acetylcholine during non-REM sleep, with a restoration of acetylcholine only during REM sleep (Trulson and Jacobs, 1979; Aston-Jones and Bloom, 1981; Marrosu et al., 1995). At the systems level, neocortical activity that supports the conscious processing of stimuli is reduced and is thought to be replaced by spontaneous processes that support the restorative functions of sleep. These restorative functions may be intimately linked to the memory consolidation attributed to sleep (Yoo et al., 2007; van der Werf et al., 2009) and such memory consolidation may coincide with a unique change in network activity. For example, this consolidation may be effectuated by a reversal of the hippocampo-neocortical dialog that preferentially occurs during sleep (Buzsaki, 1998; Hasselmo, 1999). Recent EEG-PET and EEG-fMRI studies have indeed shown a learning-dependent increase in hippocampal (Peigneux et al., 2004) or specific cortical regions (Rasch et al., 2007; Bergmann et al., 2012) during sleep. Therefore, the identification of brain regions that are functionally connected during sleep may help us further understand the process of memory consolidation. Compared to the study of activity levels during sleep with fMRI or PET, studying functional networks during sleep with these techniques is a new area of exploration.
A simple way to investigate functional connectivity in the brain is to determine correlations between signals from various brain regions. Generally, this involves calculating the Pearson product-moment correlation coefficient at zero lag (time-shift) between the signals. Although the study of the lag-dependence of this correlation (so-called cross-correlation analysis) is also possible (see “Granger causality analysis” section below), it is commonly omitted as it generally provides little additional information due to the substantial temporal blurring of the neuronal signals by the sluggish hemodynamic response. Correlation analysis can in principle be done on a voxel- (i.e., single MRI volume element) by-voxel basis, but doing this for all possible voxel combinations would be rather computational intensive and its result would be expansive and require further summarizing and interpretation. For this reason, connectivity analysis is often restricted to the calculation of correlations between one or a few “seed” region(s) and all other voxels in the brain. The seed region can be defined either anatomically or functionally, and this can be done in native space (i.e., before non-linear registration to a standard brain atlas) or in standard space (i.e., after non-linear registration to a standard brain atlas).
Defining a seed region anatomically is performed by tracing it manually or by using standard atlas coordinates. When using standard atlas coordinates, investigators can use the discontinuous clusters that define an anatomical region or a single point in space within that region in combination with geometrically defined shapes (e.g., spheres). Defining a seed region functionally is performed by collecting functional data in a separate session with a task that is known to elicit robust activity in the region of interest and extracting the time course of significantly activated voxels in native space. Defining the seed region anatomically is advantageous because it provides more standardization across studies, whereas defining the seed region functionally is advantageous because the exact brain region that controls a behavior or a cognitive activity often varies between individuals. It is also possible to define the seed region using principal or independent component analysis (see below). It is customary to take the region’s averaged signal to represent the region; an alternative is to extract one or more signals with principal component analysis (e.g., Bianciardi et al., 2009b).
Investigators who analyzed cortico-cortical connectivity during sleep have done so in the context of the default-mode network. The anterior-posterior nodes of the default-mode network continue to be coupled in stage 1 and 2 sleep (Horovitz et al., 2008; Larson-Prior et al., 2009; see Samann et al., 2011 as an exception) but lose much of this coupling in stage 3 and 4 sleep (Horovitz et al., 2009; Samann et al., 2011). The latter result is consistent with data that indicated decreased neocortical connectivity using EEG (Massimini et al., 2005). In REM sleep, the anterior-posterior connectivity is restored with the exception of the dorsomedial prefrontal cortex, which may explain some of the phenomenological characteristics of dreaming (Koike et al., 2011).
The utility of fMRI to study correlated networks during sleep is particularly clear when one is interested in the connectivity of subcortical regions, which are less amenable to connectivity analyses with EEG data. Kaufmann et al. (2006) used a seed region in the hypothalamus, which contains several nuclei that are critical to sleep-wake regulation. For example, the ventrolateral preoptic nucleus is part of the putative bistable sleep switch (McGinty and Szymusiak, 2000; Saper et al., 2001). There was little connectivity with the hypothalamus during wakefulness and only during sleep did connectivity with other regions appear. This was attributed to the emergence of sleep-active neurons in the ventrolateral preoptic nucleus. Andrade et al. (2011) used a seed region in the hippocampus. In stage 2 sleep, hippocampal connectivity with neocortical regions increased compared to wakefulness. The authors suggest that this may reflect an increase in hippocampo-neocortical information transfer and that such information transfer may support the systems consolidation of memories (Frankland and Bontempi, 2005). In stage 3 and 4 sleep, hippocampal connectivity with neocortical regions decreased compared to wakefulness. The authors suggest that this may support other types of memory consolidation because the processing of memories that are stored in these regions could take place in an isolated manner.
Many of these previous studies have implications for sleep-dependent learning (e.g., the potential importance of cortical isolation for memory processing); however, only one study was designed to measure learning and correlated it with connectivity changes during sleep (van Dongen et al., 2011). The investigators used a face-location associative memory task that is known to depend on the fusiform gyrus and measured connectivity of the fusiform gyrus during light sleep. It was discovered that neocortical connectivity increased during sleep and these increases were correlated with performance on the task after sleep. These investigators have fundamentally advanced the study of sleep networks because they combined (1) the use of an established cognitive task, (2) the administration of that task before and after sleep, (3) the identification of a brain region on which that task is known to depend, and (4) the measurement of changes in connectivity with that region during an intervening period of sleep. This is a novel approach in the study of sleep networks with fMRI.
An advantage of the regional correlation approach is its mathematical and practical simplicity. On the other hand the interpretation of correlation values may be confounded by the presence of multiple signal sources within a signal region, including neuronal and non-neuronal sources. For example, incomplete removal of global (e.g., instrumental drift related) signals during pre-processing will bias the correlation values. Component analysis, which will be discussed in the following section, may overcome this problem.
Finding Independent Networks with Component Analysis
Sleep can also be considered a local brain phenomenon. One theory of sleep function suggests that neocortical brain activity during sleep is triggered by local, use-dependent activity during wakefulness (Krueger et al., 2008). Two main pieces of evidence support this theory. First, hemispheric asymmetries in neocortical sleep EEG oscillations can be induced by the local application of sleep regulators (e.g., Yoshida et al., 2004). Second, if one preferentially uses a neocortical region during wakefulness, then the sleep EEG oscillations in that region have the highest amplitude compared to other neocortical regions (e.g., Huber et al., 2004). This local activity may reflect the sleep-dependent memory consolidation associated with the task. Of course, in reality, our lives require us to consolidate an enormous variety of tasks. If we assume that – during sleep – learning each task depends on local brain activity in a variety of regions, then it would be important to use statistical techniques such as principal component analysis to analyze this brain activity.
An early application of principal component analysis was in the field of psychology. It is commonly used on questionnaires to group items according to the underlying psychological constructs that they are purported to measure. For example, the Revised NEO Personality Inventory is a 240-item questionnaire that groups items into components such as Extraversion and Conscientiousness. The same basic principle applies to fMRI research. In the simplified example of fMRI data collected for a single subject, questionnaire items are replaced with voxels, questionnaire respondents are replaced with time points, and psychological constructs are replaced with neuroanatomical regions. Techniques such as principal component analysis can be thought of as multivariate data reduction techniques. They allow the user to reduce a large variable set into a series of smaller variable subsets, where the variables within each subset are correlated with each other and the subsets are orthogonal to each other. Although this is the basic technique, there are several choices to make when using this overall data analysis approach.
First, the user must choose the specific technique to be employed: factor analysis, principal component analysis, or independent component analysis. The first two techniques are virtually identical with the exception that factor analysis is designed to assume that the error terms across the components are equal. In both of these techniques, the data are assumed to be Gaussian, whereas this is not the case for independent component analysis. This means that when independent component analysis attempts to fit a component to non-Gaussian data, it is better able to do so. This is because it maximizes the independence between components when fitting them to the data rather than maximizing the amount of variance that each component explains. Second, the user must choose whether to perform an exploratory analysis (i.e., post hoc components) or a confirmatory analysis (i.e., a priori components). Confirmatory factor analysis is often performed within the framework of structural equation modeling, which is also called latent variable modeling. In this technique, the user specifies the list of measured variables that will be grouped under each latent variable and tests the overall model fit as well as the fit between each measured variable with its latent variable. Third, in the context of fMRI research, these techniques are generally used to define components that are independent in the spatial domain, although in principle, it is possible to define components that are independent in the temporal domain. However, one needs at least n2 degrees of freedom (i.e., independent observations or time points) to extract n independent components; for this reason, temporal independent component analysis is generally not feasible with fMRI data (although see Smith et al., 2012).
Independent component analysis has been applied to fMRI data separately as well as to combined EEG-fMRI data (for reviews see Esposito and Goebel, 2011; Eichele et al., 2009 respectively). It has been used to identify brain networks during various behavioral conditions including wake (Smith et al., 2009), relaxation (Demertzi et al., 2011), and sleep (Liu et al., 2008; Samann et al., 2011). During sleep, it has been used to define the default-mode network at the individual level with a template method to measure changes in its connectivity. These changes have been tracked through the dynamic changes of sleep state in one subject in one recording session using recursive independent component analysis (Samann et al., 2011). This is a novel application of independent component analysis that could allow the real-time monitoring of the network changes that occur during sleep.
With a few exceptions (Fukunaga et al., 2007; Fransson et al., 2009; as reviewed in Duyn, 2011), there have been no attempts to characterize in an exploratory manner the unique pattern of components that might be present during sleep. In addition, there have been no attempts to use these techniques to understand how the components that are present during sleep relate to the sleep-dependent improvements in a variety of waking cognitive tasks.
Compared to the regional correlation analysis described in the previous section, component analysis may allow a better distinction between the multiple sources that contribute to a regions’ fMRI signal. As such it may provide a better separation of artifact sources such as motion and drift from neuronal sources. On the other hand, the identification of such artifactual sources may be somewhat subjective. In addition, the result of independent component analysis is to some extent dependent on the number of components one sets out to resolve. This number needs to be estimated from the data; however, overestimating the number of components may lead to an artifactual “splitting” of components and complicate interpretation. These difficulties may be largely overcome by performing the analysis over group data (with group referring to multiple sleep epochs and or multiple subjects), after which individual subject or epochs can be reconstructed through dual-regression or back projection methods (for review see Calhoun et al., 2009).
fMRI Functional Network Characterization
A brain region that is part of a network can have various roles in that network. For example, its activity can drive (or be the result of) the activity in other networks, or alternatively, it can modulate activity in other regions within the network. Discriminating between these roles may help us understand network function. Although the connectivity and component analyses discussed in the previous chapter allows one to identify the brain regions that are part of a network, it does not provide any information about the specific roles of these regions in the network or address the causal relationship between activities in the regions under study. Under certain conditions, however, the role of regions in a network may be elucidated through a technique called “graph analysis” or through further analysis of the regions’ signal time courses with “effective” connectivity techniques such as Granger causality analysis and dynamic causal modeling (Friston, 1994), which address causal relationships. These techniques, which will be discussed in the following section, allow stronger statements regarding causality and some allow both the cognitive and the neural variables to be included in a single causal model. The latter may help us understand the causal relationship between pre-sleep cognitive performance, brain connectivity during sleep in each sleep stage, and post-sleep cognitive performance.
Granger Causality Analysis
One way effective connectivity between regions in a network can be investigated is through granger causality analysis of the fMRI signal (Goebel et al., 2003), which is designed to look for temporal precedence in the regions’ activity time courses. If activity in a region precedes that of another region, it is considered to have a causal effect on it. Granger causality can be determined from lagged correlation analysis, i.e., the activity in one region is correlated with the time-shifted activity of a second region. While lagged correlation analysis has been successfully applied to electrophysiological sleep data (Qin et al., 1997; Wilson and Yan, 2010; Fucke et al., 2011), unfortunately, the application to BOLD fMRI data has proved more challenging. The reason for this is that the temporal information contained in the BOLD fMRI signal is rather coarse as the neuronal signals are heavily filtered by the HRF. In addition, filter characteristics are not known a priori and vary across the brain and across subjects. As a result, much of the fine-scale temporal information contained in neural signals is lost in fMRI signals, severely restricting the ability to identify causality from temporal precedence (Smith et al., 2011). Partly because of this reason, Granger causality methods have found limited application in fMRI studies of brain networks.
Structural Equation Modeling and Dynamic Causal Modeling
Alternative ways to investigate the causal role of regions in a network is through structural equation modeling and dynamic causal modeling. In structural equation modeling, the user specifies both the presence as well as the direction of the paths between the variables (i.e., relationships between brain activity and/or behavior) and tests the fit between the predicted model and the observed model (Loehlin, 2004). Statistical measures of fit can be obtained for the overall model and the individual path coefficients. Dynamic causal modeling (Friston et al., 2005) is somewhat different in that it makes different assumptions regarding the inputs into the model, which are based on the experimental task used in the fMRI experiment. Although there are always unknown inputs into any effective connectivity model, in structural equation modeling, all unmeasured variables are treated as stochastic unknowns. These variables are often called input variables or upstream variables. In dynamic causal modeling, one set of input variables – those based on the experimental task – are explicitly included in the model. However, this is less relevant in fMRI measurements obtained during sleep and other resting states as there is no simultaneous experimental task.
Effective connectivity techniques may be quite useful in determining the causal role of regions in relatively simple networks. However, it is not clear if this condition applies to sleep, during which many brain regions alter their activity and may interact in a complex fashion. A second problem with connectivity techniques in general is our incomplete understanding of the BOLD fMRI signal, which may represent different neural processes in different brain regions. For example, while the fMRI signal is thought to reflect local computation in cortical modules, its meaning in subcortical regions is poorly understood and may not be the same. For example, local synaptic activity that is thought to be responsible for the fMRI signal may be dominated by a region’s input rather than by local connections. Therefore, BOLD fMRI derived connectivity measures need to be interpreted with caution and should not be interpreted as a sometimes implied “path” of “information flow.” It is clear that an improved understanding of the BOLD signal in these regions will be important for the analysis of networks involving these regions.
Graph Theoretical Analysis
A third class of methods that may be used to study network activity during sleep includes graph theoretical analysis, which is a mathematical approach to the study of complex systems. This type of analysis is designed to identify and characterize patterns in the connections between modules in a network, for example by analyzing the matrix of calculated signal correlations between the all module pairs. It has been applied to the human brain based on anatomical and functional connectivity data (e.g., Salvador et al., 2005; Power et al., 2011; for review see Bullmore and Sporns, 2009). One of the findings of this work is that the brain resembles a so-called “small world” network, which minimizes the number of jumps that are necessary to connect any two nodes in the network with an efficient wiring pattern (few long-range connections) and achieves effective long-range connectivity with a minimal number of long-range connections (Watts and Strogatz, 1998). Alternatively, the connection pattern in the brain can also be analyzed by measures such as “regional homogenetity” (Zang et al., 2004) or “eigenvector centrality” (Lohmann et al., 2010), which can be analyzed on a voxel-by-voxel basis.
An important strength of graph theoretical analysis is that it allows the examination of the type of network underlying a system’s function without requiring a full understanding of the individual connections. In other words, there is more emphasis on examining a network’s connectedness rather than on its connectivity. The main disadvantage of this approach is that generally no causal information is derived from the results, similar to the limitation of functional connectivity methods.
In a preliminary application to sleep, graph theoretical analysis has been used to examine fMRI connectivity in a network of 90 anatomically defined brain regions by identifying connections from a functional connectivity analysis (Spoormaker et al., 2010). Above-threshold correlations were taken as the presence of a functional connection (note: the partial correlation analysis as described in Salvador et al., 2005 was omitted). During stage 1 and 2 sleep, the authors found a shift from an ideal small world network to a more “random” network (i.e., more long-range connections). On the other hand, during stage 3 and 4 sleep, a shift was found from an ideal small world network to a more “regular” network (i.e., fewer long-range connections).
Can the Answers to Questions about Sleep Networks Inform Other Areas of Neuroscience?
There are several potential areas of crossover between studying functional brain networks during sleep in normal subjects and studying functional brain networks in other areas in neuroscience. For example, the use of functional neuroimaging techniques in sleep disorders may provide a better understanding of their pathophysiology (Drummond et al., 2004; Nofzinger, 2005). Similarly, EEG-fMRI may be used to study other resting states such as relaxation, pathological conditions such as coma (e.g., Boly et al., 2009), and pharmacologically altered states such as general anesthesia (Boveroux et al., 2008). It is important to mention that natural sleep fulfills a unique set of functions that are not fulfilled during these other alterations in consciousness. However, there still may be lessons to learn by comparing them. For example, both coma and general anesthesia display decreases in thalamocortical connectivity in heteromodal neocortical regions such as the posterior cingulate/precuneus (Noirhomme et al., 2010). Because sleep is an excellent model for natural changes in consciousness, more studies on thalamocortical connectivity during natural sleep should be conducted. It could be predicted that the same changes in thalamocortical connectivity observed in coma and general anesthesia would also be observed in natural sleep. Another example is the comparison of stimulus processing during waking and sleeping conditions (Portas et al., 2000; Born et al., 2002; Czisch et al., 2002, 2009; Wehrle et al., 2007; Dang-Vu et al., 2011). Other than elucidating sleep mechanisms, such studies may shed light on the interplay between spontaneous and evoked activity and inform on the mechanism underlying stimulus processing.
Sleep is associated with major changes in functional brain networks. The fact that these changes occur naturally suggest that if we understand them, we may also better understand a variety of normal and pathological phenomena that are characterized by changes in network activity. As a result, applying careful methods to study functional brain networks in sleep may also help us understand normal waking brain function as well as pathological states such as disorders of consciousness.
Conflict of Interest Statement
The author declares that the research was conducted in the absence of any commercial or financial relationships that could be construed as a potential conflict of interest.
Acknowledgments
This work was supported by the Intramural Program of the National Institute of Neurological Disorders and Stroke, National Institutes of Health. The author would like to thank Dante Picchioni for the substantial number of suggestions that he provided on this manuscript.
References
Andrade, K. C., Spoormaker, V. I., Dresler, M., Wehrle, R., Holsboer, F., Samann, P. G., and Czisch, M. (2011). Sleep spindles and hippocampal functional connectivity in human NREM sleep. J. Neurosci. 31, 10331–10339.
Aserinsky, E., and Kleitman, N. (1953). Regularly occurring periods of eye motility, and concomitant phenomena, during sleep. Science 118, 273–274.
Aston-Jones, G., and Bloom, F. E. (1981). Activity of norepinephrine-containing locus coeruleus neurons in behaving rats anticipates fluctuations in the sleep-wake cycle. J. Neurosci. 1, 876–886.
Attwell, D., and Iadecola, C. (2002). The neural basis of functional brain imaging signals. Trends Neurosci. 25, 621–625.
Attwell, D., and Laughlin, S. B. (2001). An energy budget for signaling in the grey matter of the brain. J. Cereb. Blood Flow Metab. 21, 1133–1145.
Auer, D. P. (2008). Spontaneous low-frequency blood oxygenation level-dependent fluctuations and functional connectivity analysis of the “resting” brain. Magn. Reson. Imaging 26, 1055–1064.
Benar, C., Aghakhani, Y., Wang, Y., Izenberg, A., Al-Asmi, A., Dubeau, F., and Gotman, J. (2003). Quality of EEG in simultaneous EEG-fMRI for epilepsy. Clin. Neurophysiol. 114, 569–580.
Bergmann, T. O., Molle, M., Diedrichs, J., Born, J., and Siebner, H. R. (2012). Sleep spindle-related reactivation of category-specific cortical regions after learning face-scene associations. Neuroimage 59, 2733–2742.
Bianciardi, M., Fukunaga, M., van Gelderen, P., de Zwart, J. A., and Duyn, J. H. (2011). Negative BOLD-fMRI signals in large cerebral veins. J. Cereb. Blood Flow Metab. 31, 401–412.
Bianciardi, M., Fukunaga, M., van Gelderen, P., Horovitz, S. G., de Zwart, J. A., Shmueli, K., and Duyn, J. H. (2009a). Sources of functional magnetic resonance imaging signal fluctuations in the human brain at rest: a 7 T study. Magn. Reson. Imaging 27, 1019–1029.
Bianciardi, M., van Gelderen, P., Duyn, J. H., Fukunaga, M., and de Zwart, J. A. (2009b). Making the most of fMRI at 7 T by suppressing spontaneous signal fluctuations. Neuroimage 44, 448–454.
Biswal, B., Yetkin, F. Z., Haughton, V. M., and Hyde, J. S. (1995). Functional connectivity in the motor cortex of resting human brain using echo-planar MRI. Magn. Reson. Med. 34, 537–541.
Boly, M., Tshibanda, L., Vanhaudenhuyse, A., Noirhomme, Q., Schnakers, C., Ledoux, D., Boveroux, P., Garweg, C., Lambermont, B., Phillips, C., Luxen, A., Moonen, G., Bassetti, C., Maquet, P., and Laureys, S. (2009). Functional connectivity in the default network during resting state is preserved in a vegetative but not in a brain dead patient. Hum. Brain Mapp. 30, 2393–2400.
Born, A. P., Law, I., Lund, T. E., Rostrup, E., Hanson, L. G., Wildschiodtz, G., Lou, H. C., and Paulson, O. B. (2002). Cortical deactivation induced by visual stimulation in human slow-wave sleep. Neuroimage 17, 1325–1335.
Boveroux, P., Bonhomme, V., Boly, M., Vanhaudenhuyse, A., Maquet, P., and Laureys, S. (2008). Brain function in physiologically, pharmacologically, and pathologically altered states of consciousness. Int. Anesthesiol. Clin. 46, 131–146.
Braun, A. R., Balkin, T. J., Wesenten, N. J., Carson, R. E., Varga, M., Baldwin, P., Selbie, S., Belenky, G., and Herscovitch, P. (1997). Regional cerebral blood flow throughout the sleep-wake cycle. An H2[15]O PET study. Brain 120, 1173–1197.
Britz, J., Van De Ville, D., and Michel, C. M. (2010). BOLD correlates of EEG topography reveal rapid resting-state network dynamics. Neuroimage 52, 1162–1170.
Bullmore, E., and Sporns, O. (2009). Complex brain networks: graph theoretical analysis of structural and functional systems. Nat. Rev. Neurosci. 10, 186–198.
Buzsaki, G. (1998). Memory consolidation during sleep: a neurophysiological perspective. J. Sleep Res. 7(Suppl. 1), 17–23.
Calhoun, V. D., Liu, J., and Adali, T. (2009). A review of group ICA for fMRI data and ICA for joint inference of imaging, genetic, and ERP data. Neuroimage 45, S163–S172.
Chambers, J., Bullock, D., Kahana, Y., Kots, A., and Palmer, A. (2007). Developments in active noise control sound systems for magnetic resonance imaging. Appl. Acoust. 68, 281–295.
Czisch, M., Wehrle, R., Stiegler, A., Peters, H., Andrade, K., Holsboer, F., and Samann, P. G. (2009). Acoustic oddball during NREM sleep: a combined EEG/fMRI study. PLoS ONE 4, e6749. doi:10.1371/journal.pone.0006749
Czisch, M., Wetter, T. C., Kaufmann, C., Pollmacher, T., Holsboer, F., and Auer, D. P. (2002). Altered processing of acoustic stimuli during sleep: reduced auditory activation and visual deactivation detected by a combined fMRI/EEG study. Neuroimage 16, 251–258.
Dang-Vu, T. T., Bonjean, M., Schabus, M., Boly, M., Darsaud, A., Desseilles, M., Degueldre, C., Balteau, E., Phillips, C., Luxen, A., Sejnowski, T. J., and Maquet, P. (2011). Interplay between spontaneous and induced brain activity during human non-rapid eye movement sleep. Proc. Natl. Acad. Sci. U.S.A. 108, 15438–15443.
Dang-Vu, T. T., Schabus, M., Desseilles, M., Albouy, G., Boly, M., Darsaud, A., Gais, S., Rauchs, G., Sterpenich, V., Vandewalle, G., Carrier, J., Moonen, G., Balteau, E., Degueldre, C., Luxen, A., Phillips, C., and Maquet, P. (2008). Spontaneous neural activity during human slow wave sleep. Proc. Natl. Acad. Sci. U.S.A. 105, 15160–15165.
de Zwart, J. A., Silva, A. C., van Gelderen, P., Kellman, P., Fukunaga, M., Chu, R., Koretsky, A. P., Frank, J. A., and Duyn, J. H. (2005). Temporal dynamics of the BOLD fMRI impulse response. Neuroimage 24, 667–677.
Demertzi, A., Soddu, A., Faymonville, M. E., Bahri, M. A., Gosseries, O., Vanhaudenhuyse, A., Phillips, C., Maquet, P., Noirhomme, Q., Luxen, A., and Laureys, S. (2011). Hypnotic modulation of resting state fMRI default mode and extrinsic network connectivity. Prog. Brain Res. 193, 309–322.
Dresler, M., Koch, S. P., Wehrle, R., Spoormaker, V. I., Holsboer, F., Steiger, A., Samann, P. G., Obrig, H., and Czisch, M. (2011). Dreamed movement elicits activation in the sensorimotor cortex. Curr. Biol. 21, 1833–1837.
Drummond, S. P., Smith, M. T., Orff, H. J., Chengazi, V., and Perlis, M. L. (2004). Functional imaging of the sleeping brain: review of findings and implications for the study of insomnia. Sleep Med. Rev. 8, 227–242.
Duong, T. Q., Kim, D. S., Ugurbil, K., and Kim, S. G. (2001). Localized cerebral blood flow response at submillimeter columnar resolution. Proc. Natl. Acad. Sci. U.S.A. 98, 10904–10909.
Duyn, J. (2011). Spontaneous fMRI activity during resting wakefulness and sleep. Prog. Brain Res. 193, 295–305.
Eichele, T., Calhoun, V. D., and Debener, S. (2009). Mining EEG-fMRI using independent component analysis. Int. J. Psychophysiol. 73, 53–61.
Esposito, F., and Goebel, R. (2011). Extracting functional networks with spatial independent component analysis: the role of dimensionality, reliability and aggregation scheme. Curr. Opin. Neurol. 24, 378–385.
Fox, M. D., Zhang, D., Snyder, A. Z., and Raichle, M. E. (2009). The global signal and observed anticorrelated resting state brain networks. J. Neurophysiol. 101, 3270–3283.
Frankland, P. W., and Bontempi, B. (2005). The organization of recent and remote memories. Nat. Rev. Neurosci. 6, 119–130.
Fransson, P., Skiold, B., Engstrom, M., Hallberg, B., Mosskin, M., Aden, U., Lagercrantz, H., and Blennow, M. (2009). Spontaneous brain activity in the newborn brain during natural sleep – an fMRI study in infants born at full term. Pediatr. Res. 66, 301–305.
Friston, K. J. (1994). Functional and effective connectivity in neuroimaging: a synthesis. Hum. Brain Mapp. 2, 56–78.
Friston, K. J., Penny, W., and David, O. (2005). Modeling brain responses. Int. Rev. Neurobiol. 66, 89–124.
Fucke, T., Suchanek, D., Nawrot, M. P., Seamari, Y., Heck, D. H., Aertsen, A., and Boucsein, C. (2011). Stereotypical spatiotemporal activity patterns during slow-wave activity in the neocortex. J. Neurophysiol. 106, 3035–3044.
Fukunaga, M., Horovitz, S., Carr, W., Picchioni, D., de Zwart, J., van Gelderen, P., Balkin, T., Braun, A., and Duyn, J. (2007). “Spatially structured BOLD fMRI activity during deep sleep,” in Poster presented at the meeting of the Organization for Human Brain Mapping, Chicago, IL.
Goebel, R., Roebroeck, A., Kim, D. S., and Formisano, E. (2003). Investigating directed cortical interactions in time-resolved fMRI data using vector autoregressive modeling and Granger causality mapping. Magn. Reson. Imaging 21, 1251–1261.
Goldman, R. I., Stern, J. M., Engel, J. Jr., and Cohen, M. S. (2000). Acquiring simultaneous EEG and functional MRI. Clin. Neurophysiol. 111, 1974–1980.
Goldman, R. I., Stern, J. M., Engel, J. Jr., and Cohen, M. S. (2002). Simultaneous EEG and fMRI of the alpha rhythm. Neuroreport 13, 2487–2492.
Grouiller, F., Vercueil, L., Krainik, A., Segebarth, C., Kahane, P., and David, O. (2007). A comparative study of different artefact removal algorithms for EEG signals acquired during functional MRI. Neuroimage 38, 124–137.
Hamalainen, M., Hari, R., Ilmoniemi, R., Knuutila, J., and Lounasmaa, O. V. (1993). Magnetoencephalography – theory, instrumentation, and applications to noninvasive studies of the working human brain. Rev. Mod. Phys. 65, 413–497.
Hasselmo, M. E. (1999). Neuromodulation: acetylcholine and memory consolidation. Trends Cogn. Sci. (Regul. Ed.) 3, 351–359.
He, B. J. (2011). Scale-free properties of the functional magnetic resonance imaging signal during rest and task. J. Neurosci. 31, 13786–13795.
He, B. J., Zempel, J. M., Snyder, A. Z., and Raichle, M. E. (2010). The temporal structures and functional significance of scale-free brain activity. Neuron 66, 353–369.
Honey, C. J., Sporns, O., Cammoun, L., Gigandet, X., Thiran, J. P., Meuli, R., and Hagmann, P. (2009). Predicting human resting-state functional connectivity from structural connectivity. Proc. Natl. Acad. Sci. U.S.A. 106, 2035–2040.
Hong, C. C., Harris, J. C., Pearlson, G. D., Kim, J. S., Calhoun, V. D., Fallon, J. H., Golay, X., Gillen, J. S., Simmonds, D. J., van Zijl, P. C., Zee, D. S., and Pekar, J. J. (2009). fMRI evidence for multisensory recruitment associated with rapid eye movements during sleep. Hum. Brain Mapp. 30, 1705–1722.
Horovitz, S. G., Braun, A. R., Carr, W. S., Picchioni, D., Balkin, T. J., Fukunaga, M., and Duyn, J. H. (2009). Decoupling of the brain’s default mode network during deep sleep. Proc. Natl. Acad. Sci. U.S.A. 106, 11376–11381.
Horovitz, S. G., Fukunaga, M., de Zwart, J. A., van Gelderen, P., Fulton, S. C., Balkin, T. J., and Duyn, J. H. (2008). Low frequency BOLD fluctuations during resting wakefulness and light sleep: a simultaneous EEG-fMRI study. Hum. Brain Mapp. 29, 671–682.
Huber, R., Ghilardi, M. F., Massimini, M., and Tononi, G. (2004). Local sleep and learning. Nature 430, 78–81.
Iadecola, C., and Nedergaard, M. (2007). Glial regulation of the cerebral microvasculature. Nat. Neurosci. 10, 1369–1376.
Jueptner, M., and Weiller, C. (1995). Review: does measurement of regional cerebral blood flow reflect synaptic activity? Implications for PET and fMRI. Neuroimage 2, 148–156.
Kaufmann, C., Wehrle, R., Wetter, T. C., Holsboer, F., Auer, D. P., Pollmacher, T., and Czisch, M. (2006). Brain activation and hypothalamic functional connectivity during human non-rapid eye movement sleep: an EEG/fMRI study. Brain 129, 655–667.
Koike, T., Kan, S., Misaki, M., and Miyauchi, S. (2011). Connectivity pattern changes in default-mode network with deep non-REM and REM sleep. Neurosci. Res. 69, 322–330.
Krueger, J. M., Rector, D. M., Roy, S., Van Dongen, H. P., Belenky, G., and Panksepp, J. (2008). Sleep as a fundamental property of neuronal assemblies. Nat. Rev. Neurosci. 9, 910–919.
Kuhl, D. E., Edwards, R. Q., Ricci, A. R., Yacob, R. J., Mich, T. J., and Alavi, A. (1976). The Mark IV system for radionuclide computed tomography of the brain. Radiology 121, 405–413.
Kwong, K. K., Belliveau, J. W., Chesler, D. A., Goldberg, I. E., Weisskoff, R. M., Poncelet, B. P., Kennedy, D. N., Hoppel, B. E., Cohen, M. S., Turner, R., Cheng, H., Brady, T. J., and Rosen, B. R. (1992). Dynamic magnetic resonance imaging of human brain activity during primary sensory stimulation. Proc. Natl. Acad. Sci. U.S.A. 89, 5675–5679.
Larson-Prior, L. J., Zempel, J. M., Nolan, T. S., Prior, F. W., Snyder, A. Z., and Raichle, M. E. (2009). Cortical network functional connectivity in the descent to sleep. Proc. Natl. Acad. Sci. U.S.A. 106, 4489–4494.
Laufs, H., Daunizeau, J., Carmichael, D. W., and Kleinschmidt, A. (2008). Recent advances in recording electrophysiological data simultaneously with magnetic resonance imaging. Neuroimage 40, 515–528.
Laufs, H., Kleinschmidt, A., Beyerle, A., Eger, E., Salek-Haddadi, A., Preibisch, C., and Krakow, K. (2003). EEG-correlated fMRI of human alpha activity. Neuroimage 19, 1463–1476.
Lauritzen, M. (2001). Relationship of spikes, synaptic activity, and local changes of cerebral blood flow. J. Cereb. Blood Flow Metab. 21, 1367–1383.
Lemieux, L., Allen, P. J., Franconi, F., Symms, M. R., and Fish, D. R. (1997). Recording of EEG during fMRI experiments: patient safety. Magn. Reson. Med. 38, 943–952.
Leopold, D. A., Murayama, Y., and Logothetis, N. K. (2003). Very slow activity fluctuations in monkey visual cortex: implications for functional brain imaging. Cereb. Cortex 13, 422–433.
Liu, W. C., Flax, J. F., Guise, K. G., Sukul, V., and Benasich, A. A. (2008). Functional connectivity of the sensorimotor area in naturally sleeping infants. Brain Res. 1223, 42–49.
Liu, Z., de Zwart, J. A., van Gelderen, P., Kuo, L. W., and Duyn, J. H. (2002). Statistical feature extraction for artifact removal from concurrent fMRI-EEG recordings. Neuroimage 59, 2073–2087.
Loehlin, J. C. (2004). Latent Variable Models: An Introduction to Factor, Path, and Structural Equation Analysis, 4th Edn. Mahwah, NJ: Lawrence Erlbaum Associates.
Logothetis, N. K., Pauls, J., Augath, M., Trinath, T., and Oeltermann, A. (2001). Neurophysiological investigation of the basis of the fMRI signal. Nature 412, 150–157.
Lohmann, G., Margulies, D. S., Horstmann, A., Pleger, B., Lepsien, J., Goldhahn, D., Schloegl, H., Stumvoll, M., Villringer, A., and Turner, R. (2010). Eigenvector centrality mapping for analyzing connectivity patterns in fMRI data of the human brain. PLoS ONE 5, e10232. doi:10.1371/journal.pone.0010232
Maier, A., Wilke, M., Aura, C., Zhu, C., Ye, F. Q., and Leopold, D. A. (2008). Divergence of fMRI and neural signals in V1 during perceptual suppression in the awake monkey. Nat. Neurosci. 11, 1193–1200.
Mandelkow, H., Halder, P., Boesiger, P., and Brandeis, D. (2006). Synchronization facilitates removal of MRI artefacts from concurrent EEG recordings and increases usable bandwidth. Neuroimage 32, 1120–1126.
Mantini, D., Perrucci, M. G., Del Gratta, C., Romani, G. L., and Corbetta, M. (2007). Electrophysiological signatures of resting state networks in the human brain. Proc. Natl. Acad. Sci. U.S.A. 104, 13170–13175.
Maquet, P., Peters, J., Aerts, J., Delfiore, G., Degueldre, C., Luxen, A., and Franck, G. (1996). Functional neuroanatomy of human rapid-eye-movement sleep and dreaming. Nature 383, 163–166.
Marrosu, F., Portas, C., Mascia, M. S., Casu, M. A., Fa, M., Giagheddu, M., Imperato, A., and Gessa, G. L. (1995). Microdialysis measurement of cortical and hippocampal acetylcholine release during sleep-wake cycle in freely moving cats. Brain Res. 671, 329–332.
Massimini, M., Ferrarelli, F., Huber, R., Esser, S. K., Singh, H., and Tononi, G. (2005). Breakdown of cortical effective connectivity during sleep. Science 309, 2228–2232.
McGinty, D., and Szymusiak, R. (2000). The sleep-wake switch: a neuronal alarm clock. Nat. Med. 6, 510–511.
Miyauchi, S., Misaki, M., Kan, S., Fukunaga, T., and Koike, T. (2009). Human brain activity time-locked to rapid eye movements during REM sleep. Exp. Brain Res. 192, 657–667.
Moosmann, M., Schonfelder, V. H., Specht, K., Scheeringa, R., Nordby, H., and Hugdahl, K. (2009). Realignment parameter-informed artefact correction for simultaneous EEG-fMRI recordings. Neuroimage 45, 1144–1150.
Musso, F., Brinkmeyer, J., Mobascher, A., Warbrick, T., and Winterer, G. (2010). Spontaneous brain activity and EEG microstates. A novel EEG/fMRI analysis approach to explore resting-state networks. Neuroimage 52, 1149–1161.
Noirhomme, Q., Soddu, A., Lehembre, R., Vanhaudenhuyse, A., Boveroux, P., Boly, M., and Laureys, S. (2010). Brain connectivity in pathological and pharmacological coma. Front. Syst. Neurosci. 4:160. doi:10.3389/fnsys.2010.00160
Ogawa, S., Tank, D. W., Menon, R., Ellermann, J. M., Kim, S. G., Merkle, H., and Ugurbil, K. (1992). Intrinsic signal changes accompanying sensory stimulation: functional brain mapping with magnetic resonance imaging. Proc. Natl. Acad. Sci. U.S.A. 89, 5951–5955.
Peigneux, P., Laureys, S., Fuchs, S., Collette, F., Perrin, F., Reggers, J., Phillips, C., Degueldre, C., Del Fiore, G., Aerts, J., Luxen, A., and Maquet, P. (2004). Are spatial memories strengthened in the human hippocampus during slow wave sleep? Neuron 44, 535–545.
Phelps, M. E., Kuhl, D. E., and Mazziota, J. C. (1981). Metabolic mapping of the brain’s response to visual stimulation: studies in humans. Science 211, 1445–1448.
Picchioni, D., Horovitz, S. G., Fukunaga, M., Carr, W. S., Meltzer, J. A., Balkin, T. J., Duyn, J. H., and Braun, A. R. (2011). Infraslow EEG oscillations organize large scale cortical-subcortical interactions during sleep: a combined EEG/fMRI study. Brain Res. 1374, 63–72.
Portas, C. M., Krakow, K., Allen, P., Josephs, O., Armony, J. L., and Frith, C. D. (2000). Auditory processing across the sleep-wake cycle: simultaneous EEG and fMRI monitoring in humans. Neuron 28, 991–999.
Power, J. D., Cohen, A. L., Nelson, S. M., Wig, G. S., Barnes, K. A., Church, J. A., Vogel, A. C., Laumann, T. O., Miezin, F. M., Schlaggar, B. L., and Petersen, S. E. (2011). Functional network organization of the human brain. Neuron 72, 665–678.
Qin, Y. L., McNaughton, B. L., Skaggs, W. E., and Barnes, C. A. (1997). Memory reprocessing in corticocortical and hippocampocortical neuronal ensembles. Philos. Trans. R. Soc. Lond. B Biol. Sci. 352, 1525–1533.
Rasch, B., Buchel, C., Gais, S., and Born, J. (2007). Odor cues during slow-wave sleep prompt declarative memory consolidation. Science 315, 1426–1429.
Rechtschaffen, A., and Kales, A. (1968). A Manual of Standardized Terminology, Techniques, and Scoring System for Sleep Stages of Human Subjects. Los Angeles: Brain Research Institute.
Sadaghiani, S., Scheeringa, R., Lehongre, K., Morillon, B., Giraud, A. L., and Kleinschmidt, A. (2010). Intrinsic connectivity networks, alpha oscillations, and tonic alertness: a simultaneous electroencephalography/functional magnetic resonance imaging study. J. Neurosci. 30, 10243–10250.
Salvador, R., Suckling, J., Coleman, M. R., Pickard, J. D., Menon, D., and Bullmore, E. (2005). Neurophysiological architecture of functional magnetic resonance images of human brain. Cereb. Cortex 15, 1332–1342.
Samann, P. G., Wehrle, R., Hoehn, D., Spoormaker, V. I., Peters, H., Tully, C., Holsboer, F., and Czisch, M. (2011). Development of the brain’s default mode network from wakefulness to slow wave sleep. Cereb. Cortex 21, 2082–2093.
Saper, C. B., Chou, T. C., and Scammell, T. E. (2001). The sleep switch: hypothalamic control of sleep and wakefulness. Trends Neurosci. 24, 726–731.
Schabus, M., Dang-Vu, T. T., Albouy, G., Balteau, E., Boly, M., Carrier, J., Darsaud, A., Degueldre, C., Desseilles, M., Gais, S., Phillips, C., Rauchs, G., Schnakers, C., Sterpenich, V., Vandewalle, G., Luxen, A., and Maquet, P. (2007). Hemodynamic cerebral correlates of sleep spindles during human non-rapid eye movement sleep. Proc. Natl. Acad. Sci. U.S.A. 104, 13164–13169.
Shmuel, A., Yacoub, E., Chaimow, D., Logothetis, N. K., and Ugurbil, K. (2007). Spatio-temporal point-spread function of fMRI signal in human gray matter at 7 Tesla. Neuroimage 35, 539–552.
Smith, S. M., Fox, P. T., Miller, K. L., Glahn, D. C., Fox, P. M., MacKay, C. E., Filippini, N., Watkins, K. E., Toro, R., Laird, A. R., and Beckmann, C. F. (2009). Correspondence of the brain’s functional architecture during activation and rest. Proc. Natl. Acad. Sci. U.S.A. 106, 13040–13045.
Smith, S. M., Miller, K. L., Moeller, S., Xu, J., Auerbach, E. J., Woolrich, M. W., Beckmann, C. F., Jenkinson, M., Andersson, J., Glasser, M. F., Van Essen, D. C., Feinberg, D. A., Yacoub, E. S., and Ugurbil, K. (2012).Temporally-independent functional modes of spontaneous brain activity. Proc. Natl. Acad. Sci. U.S.A. 109, 3131–3136.
Smith, S. M., Miller, K. L., Salimi-Khorshidi, G., Webster, M., Beckmann, C. F., Nichols, T. E., Ramsey, J. D., and Woolrich, M. W. (2011). Network modelling methods for fMRI. Neuroimage 54, 875–891.
Spoormaker, V. I., Schroter, M. S., Gleiser, P. M., Andrade, K. C., Dresler, M., Wehrle, R., Samann, P. G., and Czisch, M. (2010). Development of a large-scale functional brain network during human non-rapid eye movement sleep. J. Neurosci. 30, 11379–11387.
Triantafyllou, C., Hoge, R. D., Krueger, G., Wiggins, C. J., Potthast, A., Wiggins, G. C., and Wald, L. L. (2005). Comparison of physiological noise at 1.5 T, 3 T and 7 T and optimization of fMRI acquisition parameters. Neuroimage 26, 243–250.
Trulson, M. E., and Jacobs, B. L. (1979). Raphe unit activity in freely moving cats: correlations with level of behavioral arousal. Brain Res. 163, 135–150.
Turner, R. (2002). How much cortex can a vein drain? Downstream dilution of activation-related cerebral blood oxygenation changes. Neuroimage 16, 1062–1067.
van der Werf, Y. D., Altena, E., Schoonheim, M. M., Sanz-Arigita, E. J., Vis, J. C., De Rijke, W., and van Someren, E. J. (2009). Sleep benefits subsequent hippocampal functioning. Nat. Neurosci. 12, 122–123.
van Dongen, E. V., Takashima, A., Barth, M., and Fernandez, G. (2011). Functional connectivity during light sleep is correlated with memory performance for face-location associations. Neuroimage 57, 262–270.
Vanderperren, K., De Vos, M., Ramautar, J. R., Novitskiy, N., Mennes, M., Assecondi, S., Vanrumste, B., Stiers, P., Van den Bergh, B. R., Wagemans, J., Lagae, L., Sunaert, S., and Van Huffel, S. (2010). Removal of BCG artifacts from EEG recordings inside the MR scanner: a comparison of methodological and validation-related aspects. Neuroimage 50, 920–934.
Wackermann, J., Lehmann, D., Michel, C. M., and Strik, W. K. (1993). Adaptive segmentation of spontaneous EEG map series into spatially defined microstates. Int. J. Psychophysiol. 14, 269–283.
Waldvogel, D., van Gelderen, P., Muellbacher, W., Ziemann, U., Immisch, I., and Hallett, M. (2000). The relative metabolic demand of inhibition and excitation. Nature 406, 995–998.
Watts, D. J., and Strogatz, S. H. (1998). Collective dynamics of “small-world” networks. Nature 393, 440–442.
Wehrle, R., Czisch, M., Kaufmann, C., Wetter, T. C., Holsboer, F., Auer, D. P., and Pollmacher, T. (2005). Rapid eye movement-related brain activation in human sleep: a functional magnetic resonance imaging study. Neuroreport 16, 853–857.
Wehrle, R., Kaufmann, C., Wetter, T. C., Holsboer, F., Auer, D. P., Pollmacher, T., and Czisch, M. (2007). Functional microstates within human REM sleep: first evidence from fMRI of a thalamocortical network specific for phasic REM periods. Eur. J. Neurosci. 25, 863–871.
Wilson, D. A., and Yan, X. (2010). Sleep-like states modulate functional connectivity in the rat olfactory system. J. Neurophysiol. 104, 3231–3239.
Yoo, S. S., Hu, P. T., Gujar, N., Jolesz, F. A., and Walker, M. P. (2007). A deficit in the ability to form new human memories without sleep. Nat. Neurosci. 10, 385–392.
Yoshida, H., Peterfi, Z., García-Garcia, F., Kirkpatrick, R., Yasuda, T., and Krueger, J. M. (2004). State-specific asymmetries in EEG slow wave activity induced by local application of TNFalpha. Brain Res. 1009, 129–136.
Keywords: sleep networks, neuroimaging, fMRI, EEG, methodology, independent component analysis, dynamic causal modeling, graph analysis
Citation: Duyn JH (2012) EEG-fMRI methods for the study of brain networks during sleep. Front. Neur. 3:100. doi: 10.3389/fneur.2012.00100
Received: 14 December 2011; Accepted: 01 June 2012;
Published online: 02 July 2012.
Edited by:
Michael Czisch, Max Planck Institute of Psychiatry, GermanyReviewed by:
Thien Thanh Dang-Vu, University of Montreal, CanadaPhilipp Georg Sämann, Max Planck Institute of Psychiatry, Germany
Copyright: © 2012 Duyn. This is an open-access article distributed under the terms of the Creative Commons Attribution Non Commercial License, which permits non-commercial use, distribution, and reproduction in other forums, provided the original authors and source are credited.
*Correspondence: Jeff H. Duyn, Advanced MRI Section, Laboratory of Functional and Molecular Imaging, National Institute of Neurological Disorders and Stroke, National Institutes of Health, 10 Center Drive, Bethesda, MD 20892, USA. e-mail: jhd@helix.nih.gov