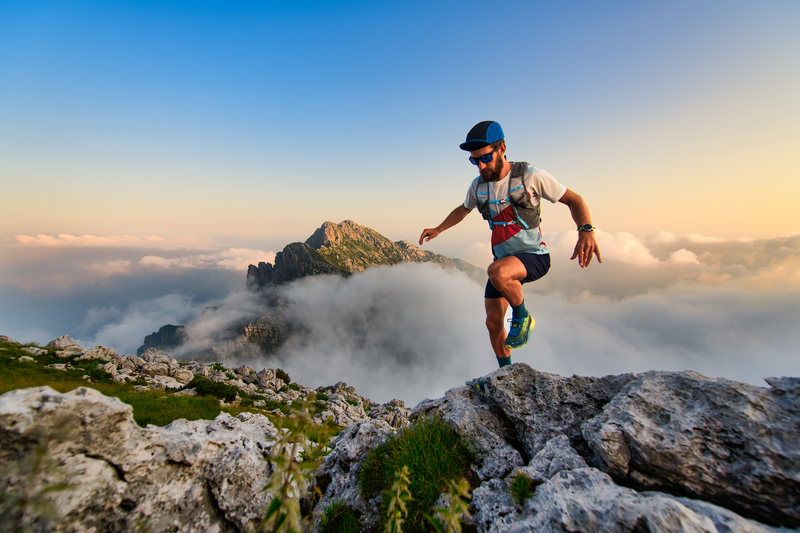
95% of researchers rate our articles as excellent or good
Learn more about the work of our research integrity team to safeguard the quality of each article we publish.
Find out more
REVIEW article
Front. Neurol. , 14 May 2012
Sec. Sleep Disorders
Volume 3 - 2012 | https://doi.org/10.3389/fneur.2012.00077
This article is part of the Research Topic Functional brain network changes in human sleep in health and disease: the role of neuroimaging View all 11 articles
High density EEG (hdEEG) during sleep combines the superior temporal resolution of EEG recordings with high spatial resolution. Thus, this method allows a topographical analysis of sleep EEG activity and thereby fosters the shift from a global view of sleep to a local one. HdEEG allowed to investigate sleep rhythms in terms of their characteristic behavior (e.g., the traveling of slow waves) and in terms of their relationship to cortical functioning (e.g., consciousness and cognitive abilities). Moreover, recent studies successfully demonstrated that hdEEG can be used to study brain functioning in neurological and neuro-developmental disorders, and to evaluate therapeutic approaches. This review highlights the potential, the problems, and future perspective of hdEEG in sleep research.
The study of spontaneous neural activity during sleep offers some important advantages for investigating brain function. Sleep recordings minimize possible confounding factors related to waking activities, including changes in the level of attention and distractibility, and issues of motivation or cognitive capacity. Such a reduction in confounding factors might be especially relevant for studies investigating changes in brain activity in children and patients with cognitive and/or behavioral impairments. When we go to sleep our brain activity changes dramatically. Since Hans Berger has established the human EEG in 1929 we can visualize this changed brain activity, which enables quantification and qualification of sleep. Specific sleep EEG rhythms were shown. The most prominent ones are slow waves, large amplitude waves below 4.5 Hz, and spindles, waxing, and waning oscillations between 12 and 15 Hz. Numerous studies, using intracellular recordings in animals, have provided detailed insights into the ionic and synaptic mechanisms that are responsible for generating these sleep rhythms (Steriade et al., 1993b). Slow waves are generated by corticocortical and thalamocortical circuits. However, such slow waves can be generated and sustained in the neocortex alone (e.g., Steriade et al., 1993a; Amzica and Steriade, 1995; Shu et al., 2003) since these waves were shown to be still present after thalamectomy and decortication (Steriade, 2003). The activity of slow waves (slow wave activity, SWA, EEG power between 0.75 and 4.5 Hz) represents a well established electrophysiological measure of sleep homeostasis (Achermann and Borbély, 2011). The homeostatic regulation of sleep reflects the time course of sleep pressure, which increases during wakefulness and wanes during non-rapid eye movement (NREM) sleep (Achermann and Borbély, 2011). Numerous experiments have shown that SWA during NREM sleep closely mirrors this time course of sleep pressure. Sleep deprivation (SD) results in an increase of sleep pressure. Correspondingly, sleep after SD shows increased levels of SWA compared to a baseline night. On the other hand, a daytime nap reduces sleep pressure and results in a decrease of SWA in the following sleep period. What functions this precise regulation of SWA subserves is still a matter of debate, but includes cortical plasticity processes (Diekelmann and Born, 2010).
Sleep spindles are initiated by a deep brain structure, the thalamic reticular nucleus (Steriade, 2000), in connection with principal thalamic nuclei, and are synchronized by corticocortical, corticothalamic, and thalamocortical loops (Kandel and Buzsaki, 1997). Numerous studies investigated their relationship to memory, intellectual ability, and sleep maintenance. Thus, several authors suggest a beneficial effect of sleep spindles for sleep-dependent memory formation (Gais et al., 2002; Schabus et al., 2006; Diekelmann and Born, 2010). Sleep spindle activity was also shown to be elevated in subjects with higher intellectual abilities (Bodizs et al., 2005; Schabus et al., 2006; Fogel et al., 2007; Geiger et al., 2011). Finally, sleep spindles have been related to sleep maintenance by protecting the cortex from external influences (Steriade et al., 1969; Elton et al., 1997; Dang-Vu et al., 2010).
In recent years novel EEG amplifiers for high density EEG (hdEEG) with up to 256 electrodes became available (Figure 1). This method allows overcoming the disadvantageous low spatial resolution of standard EEG recordings. Therefore, new analysis tools have become available for the investigation of trait- and state-like activity in the sleep EEG topography. Thus, hdEEG fosters the shift from a global view of sleep to a local one, allowing to investigate localized changes in the main sleep rhythms and thereby revealing possible abnormalities of brain functioning and maturation. So far, hdEEG studies during sleep exclusively focused on NREM sleep, since the major characteristics of NREM sleep, spindles, and slow waves, are believed to be locally regulated. Therefore, REM sleep is mentioned only marginally in this review.
Figure 1. The photo shows an 11-year-old subject wearing a high density EEG net with 128 electrodes (“dense net array” of Electrical Geodesics Inc.).
In the next chapters we will highlight the potential of hdEEG by showing the analysis of the sleep EEG with high spatial resolution in healthy and clinical populations. We will also discuss current problems of this method. Finally, the review provides future perspectives of hdEEG.
Spectral analysis of the sleep EEG allows a quantification of the activity in specific frequency bands for single electrodes. The high number of electrodes in hdEEG allows a precise mapping of the spectral power distribution across the scalp. This enables the investigation of topographical power differences in specific frequency bands (e.g., slow waves, <4.5 Hz) within and between healthy subjects (see Figure 2) and in clinical populations.
Figure 2. Topographical distribution of slow wave activity (EEG power between 0.75 and 4.5 Hz) during the first hour of NREM sleep for individuals within defined age-groups. Black dots represent all 109 EEG electrodes included in this analysis. Values are color coded (red: maxima, blue: minima). Numbers plotted on the right side of each map depict the maximal (red) and minimal (blue) value within each map. Topographical maps are proportionally scaled and missing electrode values were interpolated. Representative subjects up to 24 years were selected from the study by Kurth et al. (2010). Topographical plots of healthy control subjects older than 60 years from Neumann et al. (unpublished data) (A) Maturation of slow wave topography illustrated for 4- to >60-year-old subjects. Each plot includes data of one representative subject. (B) Each column represents the SWA maps of two different sleep sessions (at least 1 week apart) for one subject. The two maps of each subject illustrate the topographical “fingerprint” of the power distribution in the SWA range.
That sleep is not only a global brain process but shows also local aspects has already been suggested in early studies using classical EEG recordings with few electrodes. These studies found electrode specific differences of the sleep EEG (Findji et al., 1981; Hori, 1985; Werth et al., 1996, 1997a; Werth et al., 1997b; Cajochen et al., 1999). For example, including up to 27 electrodes, studies showed that the topographical distributions of the activity of sleep EEG rhythms (Buchsbaum et al., 1982; Zeitlhofer et al., 1993; Finelli et al., 2001b) are like individual “fingerprints.” Therefore, such topographical fingerprints are supposed to reflect trait-like activity (Finelli et al., 2001a). Figure 2B illustrates these topographical fingerprints in the SWA range for individuals recorded with hdEEG during sleep. Most stable fingerprints, which persists under experimental perturbations, as for instance by SD (De Gennaro et al., 2005), are found for the frequency band between 8 and 16 Hz. Moreover, a twin study revealed a high heritability of such topographic “fingerprints” (De Gennaro et al., 2008b).
Besides this trait-like activity also state-like activity has been found during sleep. For example, using extensive hand sensory stimulation during waking and EEG recordings (eight electrodes) during sleep, Kattler et al. (1994) showed that waking plasticity results in a local increase of SWA during subsequent sleep. An interesting paradigm to relate waking activity and sleep is the investigation of learning task induced synaptic plasticity. HdEEG recordings made it possible to discover such local changes in the sleep EEG.
Various studies support a role of sleep for learning and memory (for a review see Diekelmann and Born, 2010). Especially, sleep spindles and slow waves seem to be related to these beneficial effects (Gais et al., 2002; Huber et al., 2004; Huber et al., 2006; Schabus et al., 2006; Hill et al., 2008). Recent studies using hdEEG were able to show that sleep SWA on a local level can benefit learning (e.g., Huber et al., 2004; Landsness et al., 2009, 2011b; Maatta et al., 2010). These studies showed that SWA was locally increased after a learning task involving a circumscribed brain region. In other words, the plastic changes induced in a right parietal region by visuomotor adaptation learning was reflected in increased SWA during subsequent sleep in this region. Moreover, this local increase of SWA correlated with the sleep-dependent performance improvement in this learning task. These examples illustrate the importance of hdEEG, because a re-analysis of this data showed that a sufficient number of electrodes is needed to detect this local increase of SWA after learning (see Figure 3). Hence, these hdEEG scalp recordings were important to show that SWA is locally regulated. However, what electrode number is needed to discover local changes in brain activity depends on the size of the target area and the individual variability of the expected effect.
Figure 3. Effect of electrode down sampling on the detection of local changes in SWA during sleep. (A) Topographical maps illustrate the size of the local SWA increase after the rotation learning compared to the control condition (Huber et al., 2004) for different electrode numbers. EffMax defines the maximal effect (ratio learning/control) present in each topographical map. (B) Size of the effect plotted as a function of the electrode number. An asymptotic level of the effect is reached around 100 electrodes. When the electrode number is reduced below 100 there is an exponential decrease in the size of the effect. This pronounced decrease in the effect decreases the probability to detect a local change in SWA in a significant cluster of electrodes.
Several studies support the relationship between plastic changes and the local regulation of SWA. Thus, a local depression of cortical circuits through arm immobilization was followed by a local decrease of SWA over the relevant cortical areas (Huber et al., 2006). A next study provided evidence that it is indeed the potentiation of local cortical circuits, which leads to a local increase of SWA. Direct cortical potentiation by means of transcranial magnetic stimulation (TMS) resulted in a local increase of SWA during subsequent sleep (Huber et al., 2007). Another method to directly manipulate synaptic strength of cortical circuits is the paired associative stimulation (PAS) protocol, in which electrical peripheral somatosensory stimuli are followed at different intervals by TMS (Abbott and Nelson, 2000; Classen et al., 2004). Depending on the inter stimulus interval and the subject, this stimulation leads to long term potentiation (LTP) or long term depression (LTD), as measured by somatosensory and motor evoked potentials (Abbott and Nelson, 2000; Stefan et al., 2000; Classen et al., 2004). Using this paradigm combined with hdEEG during sleep, Huber et al. (2008) was able to show that PAS induced LTD was followed by a local decrease of sleep SWA and PAS induced LTP by a local increase of SWA. It is important to note that PAS induced LTD and PAS induced LTP involved the exact same amount of stimuli. Moreover, the PAS induced change in synaptic strength during waking, assessed by TMS-evoked potentials, was positively correlated with the changes in SWA during sleep and localized to similar cortical regions (Huber et al., 2008). Other studies supported this local relationship between PAS induced plastic changes and sleep EEG activity in the slow wave, but also the slow spindle frequency range (Bergmann et al., 2008; De Gennaro et al., 2008a). In summary, these studies suggest a close relationship between the homeostatic regulation of sleep and changes in synaptic strength.
Several parameters of sleep slow waves, e.g., their slopes, seem to be sensitive markers for changes in synaptic strength (Riedner et al., 2007). These findings are also supported by studies investigating such a relationship on a cellular level (Vyazovskiy et al., 2008, 2009, 2011) and with large-scale computer models of the thalamocortical system (Hill and Tononi, 2005; Esser et al., 2007; Olcese et al., 2010). For instance, a recent study in rats provides both molecular and electrophysiological evidence for a close relationship between the changes in synaptic strength and the homeostatic regulation of sleep (Vyazovskiy et al., 2008). This study demonstrated that synaptic potentiation preferentially occurs during wakefulness whereas synaptic depression takes place mainly during sleep and thereby ensuring a balance of synaptic strength across 24 h (Vyazovskiy et al., 2008). In the synaptic homeostasis hypothesis proposed by Tononi and Cirelli (2006), the reduction of synaptic strength during sleep is termed synaptic downscaling, reflecting a generalized decrease of synaptic strength. Furthermore, this synaptic plasticity, best measured by the slope of cortical evoked responses, was related to changes in SWA. More specifically, synaptic potentiation during wakefulness was associated with the level of SWA at sleep onset whereas synaptic depression in the course of sleep correlated with the decline of SWA in the course of a sleep period (Vyazovskiy et al., 2008).
As highlighted above, learning before sleep is reflected in a local increase of SWA and spindle power that may benefit post-sleep learning task performance (Gais et al., 2002; Huber et al., 2004, 2006; Schabus et al., 2006; Hill et al., 2008; Landsness et al., 2009). However, active encoding of learning a task is assumed to be restricted to waking (Aarons, 1976; Hasselmo, 1999). This assumption may not always be true: Even though newborns are the majority of the time asleep, they need to adapt to the postnatal environment by learning associations, e.g., conditioned head rotation reflexes (Papousek, 1961). Interestingly, a hdEEG study during sleep in newborn infants demonstrated that they are capable of learning associations (conditioned eye movement response) while asleep (Fifer et al., 2010). Moreover, they found a localized change in cortical activation over frontal areas as measured by event-related scalp potentials. This change is thought to reflect the updating of incomplete memories (Pascalis et al., 1998; Fifer et al., 2006).
Sleep deprivation leads to global activity changes during sleep as can be measured by an overall increase in slow frequency NREM sleep EEG power (Borbély et al., 1981). A recent investigation aimed at comparing and separating such global effects in EEG power after a SD from local effects achieved by specific behavioral manipulations during SD (Sarasso et al., 2011). To do so, 16 subjects underwent two 24-h SD protocols. In one protocol subjects were engaged with extensive audiobook listening, known to activate left fronto-temporal networks. In the other SD protocol subjects extensively played a driving simulator game, which is known to activate mainly occipito-parietal cortices. Recovery and baseline nights were recorded with a 256-channel hdEEG. Global effects were seen by a power increase in frequencies below 12 Hz after both manipulations. Notably, when comparing the two manipulations, significant SWA and spindle power increases were found for the regions known to be task-specifically activated during waking. Thus, SD reveals global and local effects (Sarasso et al., 2011).
It is needless to say that the most dramatic remodeling of neural connections takes place during childhood, which is accompanied by increased sleep need (Iglowstein et al., 2003) and by increased levels of SWA (Jenni and Carskadon, 2004). Studies introduced in previous paragraphs propose that hdEEG during sleep provides information about cortical plasticity on a local level. Therefore, hdEEG might be a promising non-invasive method to address the question whether a functional relationship between sleep and brain maturation exists. To address this question we obtained high density sleep EEG and structural magnetic resonance images (MRI) in children and adolescents. Indeed, we found a tight relationship between sleep SWA and several MR derived markers of brain maturation, e.g., gray matter volume (Buchmann et al., 2011). More specifically, this relationship between the decrease in gray matter volume and SWA was most pronounced in areas maturing during adolescence–parts of the prefrontal cortex and the medial temporal lobe. In another study we used hdEEG to map cortical activity during sleep from early childhood to late adolescence (Kurth et al., 2010). This analysis showed that the maximal SWA undergoes a shift from posterior to anterior regions across the first two decades of life. Figure 2 gives an impression of this shift from posterior to anterior regions for single individuals. Notably, the maximal SWA decreases with age. In conclusion, SWA parallels the time course of cortical maturation (Shaw et al., 2008) and might reflect cortical plasticity during development (Kurth et al., 2010).
Slow waves and spindles, the two main sleep rhythms, mirror the functioning and integrity of the thalamocortical system and corticocortical connections (Steriade and Timofeev, 2003). Moreover, slow waves seem to reflect cortical maturation (Kurth et al., 2010; Buchmann et al., 2011). Thus, alterations in these rhythms might be used as an indicator for thalamocortical or corticocortical dysfunctioning found in neuro-degenerative and neuro-developmental disorders. Therefore, hdEEG is a well-suited tool to investigate localized changes during sleep in these disorders.
Attention-deficit hyperactivity disorder (ADHD) is the most common psychiatric disorder in childhood (Olfson, 1992). Several studies provide evidence that the underlying cause of ADHD is a maturational delay (e.g., Kinsbourne, 1973; Shaw et al., 2007). Considering that slow waves, and their topographical distribution, reflect cortical maturation (Kurth et al., 2010; Buchmann et al., 2011), it seems worthwhile to investigate the high density sleep EEG in ADHD children. Preliminary results from our group provide evidence that there are topographical differences in the SWA distribution over central regions between ADHD children and age-matched healthy controls (Ringli et al., under revision). Moreover, in healthy children we found a positive correlation between daytime motor activity, as assessed by actigraphy, and SWA over motor cortical areas (Ringli et al., under revision). This local SWA increase may reflect the use dependent plasticity of the motor cortex. However, this correlation was not found in ADHD patients. Thus, it seems that hyperactivity or increased stimulation of the motor cortex in ADHD can not explain the increase in SWA over motor areas, supporting the conclusion that differences in SWA topography found in ADHD children reflects a maturational delay (Ringli et al., under revision).
Another example showing the fruitfulness of applying hdEEG sleep recordings in clinical populations are studies in schizophrenic subjects by Ferrarelli et al. (2007, 2010b). These studies uncovered topographical differences in sleep spindle activity of schizophrenic subjects. The reduced sleep spindle activity over centroparietal areas might reflect a dysfunction of thalamic reticular and thalamocortical mechanisms and could represent a biological marker of illness. In healthy subjects the topographical distribution of sleep spindle activity is characterized by a frontal maximum in the slow (<13 Hz) spindle frequency range and a centroparietal maximum in the fast (>13 Hz) spindle frequency range (for a review see De Gennaro and Ferrara, 2003). In schizophrenic patients the centroparietal reduction of sleep spindle activity was restricted to the fast spindle frequency range (Ferrarelli et al., 2007). A subsequent analysis including whole-night hdEEG during sleep in schizophrenic patients showed that the activity in the spindle frequency range is affected throughout the night (Ferrarelli et al., 2010b). This difference in spindle activity is unlikely a result of antipsychotics as a third group of non-schizophrenic patients treated with the same medication showed no such differences in spindle activity (Ferrarelli et al., 2010b). The diminished sleep spindle activity points to a dysfunction of the thalamocortical system since sleep spindles are generated by the thalamocortical system. A recent study of the same group investigated the properties of the thalamocortical circuits in schizophrenic patients and healthy control subjects using a combination of TMS and hdEEG during wakefulness (Ferrarelli et al., 2008). Comparing the induced gamma-oscillation by TMS between schizophrenics and healthy controls, they found a pronounced decrease in this evoked oscillation especially in a fronto-central region in schizophrenic patients. The authors concluded that patients with schizophrenia might show a dysfunction in frontal thalamocortical circuits (Ferrarelli et al., 2008).
Interestingly, spindle activity is also associated with general intellectual abilities (Bodizs et al., 2005; Schabus et al., 2006; Fogel et al., 2007; Geiger et al., 2011). A recent study investigated the association between regional aspects of the sleep EEG especially in the spindle frequency range and different measures of intellectual ability (e.g., fluid intelligence) in children (Geiger et al., 2012). Notably, for frequencies in the slow and fast spindle frequency range, the correlations with intellectual ability was over central and parietal areas (Geiger et al., 2012). Many schizophrenic patients suffer from cognitive impairments (for a review see O’Carroll, 2000) that might be reflected in spindle deficits over the centroparietal cortex (Ferrarelli et al., 2007, 2010b). The study by Geiger et al. (2012) cannot rule out that spindle activity over the frontal cortex relates to intellectual abilities since only children were included in the analysis. The relationship between sleep spindles and intelligence might change in the course of cortical maturation.
Slow wave sleep abnormalities are prominent in depression (Borbély and Wirz-Justice, 1982; Benca et al., 1992). SD provides therapeutic benefits in depressed patients (for a review see Hemmeter et al., 2010). It has been proposed that these benefits of SD relate to renormalizing the abnormal slow wave homeostasis (Borbély, 1987). Using hdEEG a recent study directly investigated the role of slow wave homeostasis in the antidepressant action of SD by using a selective slow wave deprivation (SWD) technique (Landsness et al., 2011c). SWD of slow waves in fronto-central regions was associated with a decrease in depressive symptom ratings suggesting a localized effect of SWD. A previous study using source localization of hdEEG data provide evidence that the anterior cingulate cortex, a critical brain structure in major depression, is indeed linked to generation of sleep slow waves (Murphy et al., 2009). In addition to the investigation of spontaneous brain activity, the analysis of evoked brain responses (e.g., auditory evoked potentials, AEP) are used to measure changes in cortical excitability, which likely reflect changes in synaptic strength. A recent investigation demonstrated that the homeostatic decrease of SWA in the course of a sleep period is paralleled by a significant reduction of the AEP amplitude from pre- to post-sleep (Hulse et al., 2011). This reduction of AEP amplitude was most prominent over fronto-central cortical regions. Goldstein et al. (2011) have shown that this reduction in AEP amplitude is absent in patients suffering from major depressive disorder (Goldstein et al., 2011). This finding supports the proposal of a diminished sleep homeostasis in depressed patients.
Several results show regional differences, e.g., the local differences in the amplitude of AEPs. These local differences might be overseen with an insufficient number of electrodes. Collectively, these studies demonstrate that hdEEG is a powerful tool to study brain functioning in psychiatric disorders and to evaluate therapeutic approaches.
Preliminary hdEEG data during sleep provides evidence that patients with a hemispheric stroke exhibit a local fronto-central reduction of the slope of slow waves in the affected compared to the unaffected hemisphere and also compared to healthy controls (Neumann et al., unpublished data). Since the slope of slow waves presumably reflects synaptic strength (Esser et al., 2007), this finding indicates a reduction of synaptic strength and a corresponding reduction in neuronal synchronization over regions roughly matching the location of the ischemic damage (Neumann et al., unpublished data). Rehabilitative therapy after stroke aims to foster recovery of the brain by taking advantage of the brain’s increased potential for synaptic plasticity and reorganization after stroke (Brown et al., 2007; Murphy and Corbett, 2009; Kalra, 2010). As mentioned before, SWA seems to be a sensitive marker for local synaptic changes. Therefore, hdEEG during sleep might be a good method to assess local cortical reorganization after stroke therapy and consequently evaluate therapeutic effects. Sarasso et al. (2010) investigated the neural reorganization after speech therapy in one representative patient with left hemisphere stroke lesions. As a post-stroke aphasia rehabilitation they used a computer based speech therapy, which is suggested to reorganize a language based core network (IMITATE; Lee et al., 2010). Preliminary results of this subject showed that after one session of IMITATE training, SWA was mainly increased in regions activated during execution of IMITATE. Therefore, these local changes of SWA might reflect the effectiveness of the therapy (Sarasso et al., 2010).
Slow oscillations are the fundamental phenomenon that organizes other sleep rhythms as spindles and slow waves (Steriade et al., 1993c). Using hdEEG, Massimini et al. (2004) explored the spatiotemporal dynamics of sleep slow oscillations in eight adults. To do so, they used a 256-channel hdEEG and co-registered the electrodes to individual MRI. According to this study, slow oscillations periodically sweep the cerebral cortex with a definite site of origin and specific pattern of propagation (Massimini et al., 2004). This data showed that slow oscillations behave like traveling waves. More specifically, the timing of the negative peak of slow waves, a clear-cut feature of slow waves, varies systematically across electrodes. The origin of slow waves is defined as the location of the electrode showing the earliest negative peak. Thereafter, the location of the negative peak rapidly spreads to neighboring regions (Massimini et al., 2004). Though slow waves could originate from practically every cortical site, they preferentially originated from anterior cortical regions and propagated in an antero-posterior direction (Massimini et al., 2004). Following the pattern of origin and propagation of hundreds of sleep slow oscillations provides a blueprint of cortical connectivity. Why there is a predominant origin of slow waves over frontal areas is not known. It might be speculated that this frontal predominance is due to the increased excitability seen in frontal regions of adults (Horne, 1993; Couyoumdjian et al., 2010), which is also reflected in the frontal predominance of SWA (Werth et al., 1997a; Cajochen et al., 1999; Finelli et al., 2001b; Massimini et al., 2004; Kurth et al., 2010). Since there is a shift of maximal SWA from occipital to frontal regions (Kurth et al., 2010), it might be interesting to explore the traveling behavior of slow waves in children.
High density EEG allows to map the distribution of EEG activity. However, under certain conditions, it might be difficult to relate scalp potentials to the activity of the underlying cortex because local potentials can be generated by sources that are distant from the recording sites (Wendel et al., 2009). Source modeling of EEG activity overcomes this problem (Wendel et al., 2009). Several sleep studies performed LORETA (low resolution brain electromagnetic tomography) source analysis of sleep spindles using 19 electrodes (Anderer et al., 2001; Mander et al., 2011; Saletin et al., 2011). Depending on the sleep spindle frequency they showed different cortical contributions (Anderer et al., 2001). Moreover, cortical sources seem to change across the spindle time series (Mander et al., 2011; Saletin et al., 2011). For an accurate source modeling of EEG activity a large number of electrodes is required (Michel et al., 2004). However, up to now, only few hdEEG studies during sleep performed source localization. The mapping of SWA using hdEEG suggests that regions of the cortex are differentially involved and that slow waves behave like traveling waves (Werth et al., 1997a; Cajochen et al., 1999; Finelli et al., 2001b; Massimini et al., 2004; Kurth et al., 2010). Using source modeling of sleep slow waves, Murphy et al. (2009) explored cortical sources that are involved in the origin and propagation of slow waves. Even though each spontaneous slow wave has its unique pattern, the probability that a slow wave originates from a certain area was highest in the left hemisphere, in the insula and cingulate gyrus. Moreover, slow waves preferentially propagated via the cingulate gyrus. These analysis also showed that slow waves are not always largest where they originate from (Murphy et al., 2009) as observed with topographical hdEEG analysis (Massimini et al., 2004). Murphy et al. (2009) performed source modeling using three different source localization methods: LORETA, local autoregressive average (LAURA) and the Bayesian minimum norm. All three methods revealed similar results. Nevertheless, Murphy et al. (2009) preferred LAURA since the Bayesian minimum norm was difficult to implement for large amounts of data, as is typically the case in sleep EEG studies. Furthermore, the LORETA constraints rely on a biological assumption that neighboring areas have similar activations whereas LAURA constraints derive from known properties of the decay of current from the source (Murphy et al., 2009). Murphy et al. (2011) also used LAURA to localize the source of the plasticity related induction of SWA over specific cortical areas, since they found this method to be the most effective one to analyze slow waves (Murphy et al., 2009). They showed that source localization might not be needed to assess the local regulation of SWA during sleep. Indeed, Murphy et al. (2011) confirmed the link between waking cortical plasticity and sleep SWA, and revealed the same regions that were previously found in topographical hdEEG analysis (Huber et al., 2004, 2006, 2007).
In cats it was shown that spike-wave seizures can emerge from cortical slow oscillations during sleep (Steriade and Amzica, 1994). Thus, source localization of hdEEG data might be helpful for the identification of epileptic sources during sleep. This finding implies that spike-wave seizures may originate from cortical sources (Tucker et al., 2009) and that they are not generated by thalamocortical circuits as previously believed (Gloor, 1978). Moreover, hdEEG studies during wakefulness revealed that spike-wave discharges are not generalized, as believed so far, but are rather localized to frontal areas (Holmes et al., 2004; Tucker et al., 2007). A recent study applied hdEEG in a patient suffering from spike-wave seizures during sleep (Tucker et al., 2009). They found that each seizure was preceded by a series of cortical slow oscillations. Moreover, source localization analysis using the LORETA and LAURA approach showed that the seizures and the cortical slow oscillation originated from the same frontopolar region (Tucker et al., 2009). These results suggest that cortical slow oscillations may facilitate the pathological synchronization of the seizure (Vanhatalo et al., 2004; Tucker et al., 2009). On a cellular level cortical slow oscillations are characterized by an alternation of down- and up-states (Steriade et al., 1993a; Amzica and Steriade, 1998; Destexhe et al., 1999; Steriade et al., 2001; Vyazovskiy et al., 2009). The down-state reflects hyperpolarization of cortical neurons. This hyperpolarization suppresses spiking activity of the cortical neurons. The up-state mirrors depolarization of these neurons. The duration of these up and down states is a good marker for cortical synchronization and excitability (Vyazovskiy et al., 2009). The comparison of the sleep slow oscillations of epileptic with non-epileptic subjects using source localization of hdEEG data during sleep provides preliminary evidence that the suppression of neuronal spiking activity during the down states of epileptic cortical slow oscillations is reduced compared to non-epileptic subjects (Gilbert et al., 2011). Thus, the modulation of cortical excitability may be the pathological basis of this type of seizures (Gilbert et al., 2011).
Even though our brain is active during NREM sleep, consciousness fades during this vigilance state. The fading of consciousness might be related to an impairment of effective cortical connectivity reflecting the causal interactions of different systems (Gerstein and Perkel, 1969; Lee et al., 2003). Some studies investigated this prediction using hdEEG and navigated TMS (Massimini et al., 2005; Ferrarelli et al., 2010a). The TMS/hdEEG combination allows to assess the ability of cortical areas to interact. Thus, this method can be used to track the propagation of an induced activity from one part of the brain to the rest of the brain and compare this propagation between sleep and wakefulness (Massimini et al., 2005). The study showed that during wakefulness the TMS activation propagated across the entire cortex along known pathways. However, during deep NREM sleep, the response to TMS did not propagate beyond the stimulation site (Massimini et al., 2005). This finding suggests a breakdown of long-range and transcallosal effective connectivity during deep NREM sleep. To further proof the concept that the fading of consciousness is reflected in a breakdown of cortical effective connectivity, Ferrarelli et al. (2010a) induced a pharmacological loss of consciousness by means of midazolam. After injection of the anesthetic TMS-evoked brain responses were changed compared to wakefulness showing striking similarity to the ones obtained during deep NREM sleep (Ferrarelli et al., 2010a). Thus, the breakdown of effective cortical connectivity as assessed by the combination of TMS and hdEEG might be a promising indicator of unconsciousness (Massimini et al., 2005).
An interesting clinical population to further investigate consciousness are patients with altered states of consciousness after severe brain damage, as for example patients in vegetative state or minimal conscious state. Unlike coma patients, vegetative state or minimal conscious state patients are awake (Kaplan and Bauer, 2011). Patients in the minimal conscious state show, in contrast to patients in the vegetative state, minimal signs of awareness and attention, but they are unable to communicate effectively (Giacino et al., 2002). However, the differential diagnosis between these two states is rather difficult and often associated with misdiagnosis (Schnakers et al., 2009). Recent studies report differences in brain functioning (Boly et al., 2008; Vanhaudenhuyse et al., 2010; Landsness et al., 2011a) and prognosis (Laureys and Boly, 2007; Luaute et al., 2010) between these patient groups. Minimally conscious patients are thought to exhibit conscious cognitive processing (Schiff, 2007; Kaplan and Bauer, 2011) whereas vegetative state patients do not. Thus, the measuring effective cortical connectivity may allow to differentiate these two groups of subjects. Using TMS/hdEEG Rosanova et al. (2012) demonstrated a breakdown of effective cortical connectivity in vegetative state patients as seen during NREM sleep in healthy subjects. In contrast, minimally conscious patients showed a widespread TMS-evoked response similar to locked-in, conscious patients. Interestingly, longitudinal data in patients, who gradually recovered consciousness, showed that this change in the breakdown of effective cortical connectivity precedes significant modification of the spontaneous EEG (Rosanova et al., 2012). Moreover this clear-cut modification of effective connectivity was detected before the patient recovered the ability of functional communication (Rosanova et al., 2012). Thus, TMS/hdEEG might be used as an indicator for consciousness independent of the patients ability to communicate and may serve as a diagnostic tool for the differentiation between the vegetative and the minimal conscious state at the individual patient’s level (Rosanova et al., 2012).
Volume conduction is defined as the process of current flow through the tissues between the electrical generator and the recording electrode (Olejniczak, 2006). This process is inherent to any measure of scalp EEG. Volume conductance might be problematic for hdEEG since it may lead to blurring of the topography of a brain’s electrical fields (Gevins et al., 1994). Such a blurring may question the ability of hdEEG to detect localized brain activity. However, recent studies provide good evidence that hdEEG can be used to localize changes in EEG activity from small target areas (Huber et al., 2004, 2006, 2007, 2008; Maatta et al., 2010; Landsness et al., 2011b). Moreover, hdEEG allows to follow the slow waves while they travel across the cortex in the millisecond range (Massimini et al., 2004).
An increasing density of electrodes, as used in hdEEG, raises the possibility for electrolyte spreading and bridging (Greischar et al., 2004). There is recent evidence that hdEEG shows a lower signal-to-noise ratio compared to low-density EEG (Kayser et al., 2000; Greischar et al., 2004). Moreover, there exists a significant relationship between this power decrease and electrolyte spreading/bridging (Greischar et al., 2004). Therefore, electrolyte bridges should be prevented. Two factors may help to reduce such electrolyte bridges. First, electrolyte spreading is less common in the electrode housings used in a cap system compared to a sponge electrodes (Greischar et al., 2004). Second, electrolyte bridges can be detected and removed using an on-line bridge detection software before the recording (e.g., Electrical Geodesics Inc., 2003).
All-night sleep EEG with up to 256 electrodes produces huge amounts of data (in the Terabyte range for an experiment). Therefore, high-capacity workstations are needed to process, store, and backup such data. The question arises whether it is worth the effort and whether the superior spatial resolution is really needed. As has been shown in Figure 3, localized changes of SWA following visuomotor adaptation learning were only observed when using a high number of electrodes. The number of electrodes needed for a reliable EEG measure, for example to perform source localization or to reveal topographical differences, is a crucial question. Theoretically, more electrodes lead to a better spatial resolution. The number of electrodes needed to show a local increase depends on the size of the target area and the magnitude of change. Thus, very local changes in small target areas might be neglected with an insufficient number of electrodes. To date, electrode nets with 128 and 256 electrodes are most often used in sleep research. We believe that 256 electrodes will not relevantly enhance spatial resolution compared to 128 electrodes. But it is worth to mention that 256-electrode caps have other advantages compared to 128 electrodes. First, it is important to uniformly and accurately cover the scalp surface. During each experiment, some electrodes need to be excluded due to artifacts. It is a less pronounced problem to exclude them with the 256-electrode caps, since there is a spatial over sampling and therefore a uniform and accurate covering is guaranteed.
Second, more electrodes provide a better distribution of the pressure, when the subject lies on the electrodes. Thus, 256-electrode caps are more comfortable to wear than 128-electrode nets. This point is especially important in sleep research to provide good sleep quality. Disadvantages are that 256-electrode nets are more expensive than 128-electrode nets and that they need more time for preparation (approximately 45 vs. 30 min). Furthermore, twice as much data is generated. Thus, the decision to use 128-electrode or 256-electrode caps depends on cost–benefit calculation.
EEG studies with a high number of electrodes require a statistic that accounts for multiple comparisons. As for other neuroimaging techniques (e.g., functional MRI, fMRI) statistical non-parametric mapping (SnPM; Nichols and Holmes, 2002) can be used to control for multiple testing. In these analyses, a permutation test is used to determine the significances of clusters of supra-threshold electrodes and to explore the size of the cluster whose incidence probability is lower than a certain threshold (e.g., 5%).
High density EEG is a non-invasive, low-cost method that has several advantages to investigate sleep on the cortical level. This method overcomes the poor spatial resolution of EEG montages with only few electrodes and allows the investigation of regional aspects of sleep. Even though fMRI has a better spatial resolution and additionally allows the examination of subcortical structures, the rather poor time resolution is insufficient to track changes in cortical activity related to sleep characteristics as slow waves or sleep spindles (Volkow et al., 1997; Dang-Vu et al., 2008; Murphy et al., 2009). Intracranial recordings are important to investigate cellular mechanisms of sleep, but are limited to animal studies or clinical populations. Furthermore, they are limited to small cortical areas and neglect the large cortical areas that are for example involved in the generation and propagation of slow waves (Murphy et al., 2009). Numerous studies prove the potential of hdEEG to precisely investigate sleep. Beyond tracking sleep in healthy subjects, this method can be used to study brain functioning in neurological and neuro-developmental disorders, and to evaluate therapeutic approaches. Future studies should provide further proof and enhance the ability of hdEEG during sleep as a diagnostic tool in disorders with brain dysfunction, e.g., schizophrenia. Beside the clinical relevance of hdEEG, this method might be helpful for the understanding of basic mechanisms of sleep. For instance, process S, the homeostatic process of sleep regulation potentially reflecting synaptic downscaling was long believed to be a global process. However, local differences in SWA raised the question of whether process S is also locally regulated. So far, one study investigated the regional aspects of process S by investigating topographical aspects of the dynamics of this process (Rusterholz and Achermann, 2011). Their analysis showed clear regional differences in the dynamics of the homeostatic process. For example, the increase and decline of process S were slowest in fronto-central areas. A good explanation for these regional differences in the dynamics of process S is still missing. However, these differences might be related to region-specific plastic brain processes occurring during wakefulness and sleep (Rusterholz and Achermann, 2011). HdEEG may shed further light on this issue. An interesting possibility might be investigating the dynamics of the global and local aspects of the homeostatic process using specific manipulations during SD in combination with hdEEG, as done in the study by Sarasso et al. (2011).
Present studies about sleep and hdEEG focused on NREM sleep. A possible explanation for this is the assumption that slow waves and spindles are locally regulated. So far, there is little evidence that REM sleep is locally regulated on a cortical level. However, van der Helm et al. (2011) showed a negative correlation between the overnight decrease in emotion reactivity (emotion regulation) and gamma activity during REM sleep. A topographical analysis using 19 electrodes indicated that this association is restricted to prefrontal areas. This region is also known to play an established role in emotion regulation (e.g., Quirk and Beer, 2006). Therefore, in future studies it might be interesting to study the distribution and changes of cortical activity during REM sleep with hdEEG.
The authors declare that the research was conducted in the absence of any commercial or financial relationships that could be construed as a potential conflict of interest.
We thank Rositsa Neumann, Claudio Bassetti, Maya Ringli, Salomé Kurth, and Monique LeBourgeois for providing unpublished data. This work was supported by the Swiss National Science Foundation (P00A-114923 to Reto Huber).
Abbott, L. F., and Nelson, S. B. (2000). Synaptic plasticity: taming the beast. Nat. Neurosci. 3(Suppl.), 1178–1183.
Achermann, P., and Borbély, A. A. (2011). “Sleep homeostasis and models of sleep regulation,” in Principles and Practice of Sleep Medicine, 5th Edn, eds M. H. Kryger, T. Roth, and W. C. Dement (Philadelphia: Elsevier Saunders), 405–417.
Amzica, F., and Steriade, M. (1995). Disconnection of intracortical synaptic linkages disrupts synchronization of a slow oscillation. J. Neurosci. 15, 4658–4677.
Amzica, F., and Steriade, M. (1998). Electrophysiological correlates of sleep delta waves. Electroencephalogr. Clin. Neurophysiol. 107, 69–83.
Anderer, P., Klosch, G., Gruber, G., Trenker, E., Pascual-Marqui, R. D., Zeitlhofer, J., Barbanoj, M. J., Rappelsberger, P., and Saletu, B. (2001). Low-resolution brain electromagnetic tomography revealed simultaneously active frontal and parietal sleep spindle sources in the human cortex. Neuroscience 103, 581–592.
Benca, R. M., Obermeyer, W. H., Thisted, R. A., and Gillin, J. C. (1992). Sleep and psychiatric disorders. A meta-analysis. Arch. Gen. Psychiatry 49, 651–668; discussion 669–670.
Bergmann, T. O., Molle, M., Marshall, L., Kaya-Yildiz, L., Born, J., and Roman Siebner, H. (2008). A local signature of LTP- and LTD-like plasticity in human NREM sleep. Eur. J. Neurosci. 27, 2241–2249.
Bodizs, R., Kis, T., Lazar, A. S., Havran, L., Rigo, P., Clemens, Z., and Halasz, P. (2005). Prediction of general mental ability based on neural oscillation measures of sleep. J. Sleep Res. 14, 285–292.
Boly, M., Faymonville, M. E., Schnakers, C., Peigneux, P., Lambermont, B., Phillips, C., Lancellotti, P., Luxen, A., Lamy, M., Moonen, G., Maquet, P., and Laureys, S. (2008). Perception of pain in the minimally conscious state with PET activation: an observational study. Lancet Neurol. 7, 1013–1020.
Borbély, A. A. (1987). The S-deficiency hypothesis of depression and the two-process model of sleep regulation. Pharmacopsychiatry 20, 23–29.
Borbély, A. A., Baumann, F., Brandeis, D., Strauch, I., and Lehmann, D. (1981). Sleep deprivation: effect on sleep stages and EEG power density in man. Electroencephalogr. Clin. Neurophysiol. 51, 483–493.
Borbély, A. A., and Wirz-Justice, A. (1982). Sleep, sleep deprivation and depression. A hypothesis derived from a model of sleep regulation. Hum. Neurobiol. 1, 205–210.
Brown, C. E., Li, P., Boyd, J. D., Delaney, K. R., and Murphy, T. H. (2007). Extensive turnover of dendritic spines and vascular remodeling in cortical tissues recovering from stroke. J. Neurosci. 27, 4101–4109.
Buchmann, A., Ringli, M., Kurth, S., Schaerer, M., Geiger, A., Jenni, O. G., and Huber, R. (2011). EEG sleep slow-wave activity as a mirror of cortical maturation. Cereb. Cortex 21, 607–615.
Buchsbaum, M. S., Mendelson, W. B., Duncan, W. C., Coppola, R., Kelsoe, J., and Gillin, J. C. (1982). Topographic cortical mapping of EEG sleep stages during daytime naps in normal subjects. Sleep 5, 248–255.
Cajochen, C., Foy, R., and Dijk, D. J. (1999). Frontal predominance of a relative increase in sleep delta and theta EEG activity after sleep loss in humans. Sleep Res. Online 2, 65–69.
Classen, J., Wolters, A., Stefan, K., Wycislo, M., Sandbrink, F., Schmidt, A., and Kunesch, E. (2004). Paired associative stimulation. Suppl. Clin. Neurophysiol. 57, 563–569.
Couyoumdjian, A., Sdoia, S., Tempesta, D., Curcio, G., Rastellini, E., L, D. E. G., and Ferrara, M. (2010). The effects of sleep and sleep deprivation on task-switching performance. J. Sleep Res. 19, 64–70.
Dang-Vu, T. T., Mckinney, S. M., Buxton, O. M., Solet, J. M., and Ellenbogen, J. M. (2010). Spontaneous brain rhythms predict sleep stability in the face of noise. Curr. Biol. 20, R626–R627.
Dang-Vu, T. T., Schabus, M., Desseilles, M., Albouy, G., Boly, M., Darsaud, A., Gais, S., Rauchs, G., Sterpenich, V., Vandewalle, G., Carrier, J., Moonen, G., Balteau, E., Degueldre, C., Luxen, A., Phillips, C., and Maquet, P. (2008). Spontaneous neural activity during human slow wave sleep. Proc. Natl. Acad. Sci. U.S.A. 105, 15160–15165.
De Gennaro, L., Ferrara, M., Vecchio, F., Curcio, G., and Bertini, M. (2005). An electroencephalographic fingerprint of human sleep. Neuroimage 26, 114–122.
De Gennaro, L., Fratello, F., Marzano, C., Moroni, F., Curcio, G., Tempesta, D., Pellicciari, M. C., Pirulli, C., Ferrara, M., and Rossini, P. M. (2008a). Cortical plasticity induced by transcranial magnetic stimulation during wakefulness affects electroencephalogram activity during sleep. PLoS ONE 3, e2483. doi: 10.1371/journal.pone.0002483
De Gennaro, L., Marzano, C., Fratello, F., Moroni, F., Pellicciari, M. C., Ferlazzo, F., Costa, S., Couyoumdjian, A., Curcio, G., Sforza, E., Malafosse, A., Finelli, L. A., Pasqualetti, P., Ferrara, M., Bertini, M., and Rossini, P. M. (2008b). The electroencephalographic fingerprint of sleep is genetically determined: a twin study. Ann. Neurol. 64, 455–460.
Destexhe, A., Contreras, D., and Steriade, M. (1999). Spatiotemporal analysis of local field potentials and unit discharges in cat cerebral cortex during natural wake and sleep states. J. Neurosci. 19, 4595–4608.
Electrical Geodesics Inc. (2003). On-line detection of electrolyte bridges. EGI. Newsletter 7, 1, Eugene, OR.
Elton, M., Winter, O., Heslenfeld, D., Loewy, D., Campbell, K., and Kok, A. (1997). Event-related potentials to tones in the absence and presence of sleep spindles. J. Sleep Res. 6, 78–83.
Esser, S. K., Hill, S. L., and Tononi, G. (2007). Sleep homeostasis and cortical synchronization: I. Modeling the effects of synaptic strength on sleep slow waves. Sleep 30, 1617–1630.
Ferrarelli, F., Huber, R., Peterson, M. J., Massimini, M., Murphy, M., Riedner, B. A., Watson, A., Bria, P., and Tononi, G. (2007). Reduced sleep spindle activity in schizophrenia patients. Am. J. Psychiatry 164, 483–492.
Ferrarelli, F., Massimini, M., Peterson, M. J., Riedner, B. A., Lazar, M., Murphy, M. J., Huber, R., Rosanova, M., Alexander, A. L., Kalin, N., and Tononi, G. (2008). Reduced evoked gamma oscillations in the frontal cortex in schizophrenia patients: a TMS/EEG study. Am. J. Psychiatry 165, 996–1005.
Ferrarelli, F., Massimini, M., Sarasso, S., Casali, A., Riedner, B. A., Angelini, G., Tononi, G., and Pearce, R. A. (2010a). Breakdown in cortical effective connectivity during midazolam-induced loss of consciousness. Proc. Natl. Acad. Sci. U.S.A. 107, 2681–2686.
Ferrarelli, F., Peterson, M. J., Sarasso, S., Riedner, B. A., Murphy, M. J., Benca, R. M., Bria, P., Kalin, N. H., and Tononi, G. (2010b). Thalamic dysfunction in schizophrenia suggested by whole-night deficits in slow and fast spindles. Am. J. Psychiatry 167, 1339–1348.
Fifer, W. P., Byrd, D. L., Kaku, M., Eigsti, I. M., Isler, J. R., Grose-Fifer, J., Tarullo, A. R., and Balsam, P. D. (2010). Newborn infants learn during sleep. Proc. Natl. Acad. Sci. U.S.A. 107, 10320–10323.
Fifer, W. P., Grieve, P. G., Grose-Fifer, J., Isler, J. R., and Byrd, D. (2006). High-density electroencephalogram monitoring in the neonate. Clin. Perinatol. 33, 679–691.
Findji, F., Catani, P., and Liard, C. (1981). Topographical distribution of delta rhythms during sleep: evolution with age. Electroencephalogr. Clin. Neurophysiol. 51, 659–665.
Finelli, L. A., Achermann, P., and Borbely, A. A. (2001a). Individual ‘fingerprints’ in human sleep EEG topography. Neuropsychopharmacology 25, S57–62.
Finelli, L. A., Borbély, A. A., and Achermann, P. (2001b). Functional topography of the human nonREM sleep electroencephalogram. Eur. J. Neurosci. 13, 2282–2290.
Fogel, S. M., Nader, R., Cote, K. A., and Smith, C. T. (2007). Sleep spindles and learning potential. Behav. Neurosci. 121, 1–10.
Gais, S., Molle, M., Helms, K., and Born, J. (2002). Learning-dependent increases in sleep spindle density. J. Neurosci. 22, 6830–6834.
Geiger, A., Huber, R., Kurth, S., Ringli, M., Achermann, P., and Jenni, O. G. (2012). Sleep electroencephalography topography and children’s intellectual ability. Neuroreport 23, 93–97.
Geiger, A., Huber, R., Kurth, S., Ringli, M., Jenni, O. G., and Achermann, P. (2011). The sleep EEG as a marker of intellectual ability in school age children. Sleep 34, 181–189.
Gerstein, G. L., and Perkel, D. H. (1969). Simultaneously recorded trains of action potentials: analysis and functional interpretation. Science 164, 828–830.
Gevins, A., Le, J., Martin, N. K., Brickett, P., Desmond, J., and Reutter, B. (1994). High resolution EEG: 124-channel recording, spatial deblurring and MRI integration methods. Electroencephalogr. Clin. Neurophysiol. 90, 337–358.
Giacino, J. T., Ashwal, S., Childs, N., Cranford, R., Jennett, B., Katz, D. I., Kelly, J. P., Rosenberg, J. H., Whyte, J., Zafonte, R. D., and Zasler, N. D. (2002). The minimally conscious state: definition and diagnostic criteria. Neurology 58, 349–353.
Gilbert, T. T., Luu, P., Holmes, M., and Tucker, D. (2011). “An investigation of spontaneous cortical slow oscillations during NREM sleep using 256-channel EEG in non-epileptic and epileptic subjects,” in 25th Annual Meeting of the Associated Professional Sleep Societies (APSS) : Sleep, Abstract Supplement vol. 34, Minneapolis, :218.
Gloor, P. (1978). Generalized epilepsy with bilateral synchronous spike and wave discharge. New findings concerning its physiological mechanisms. Electroencephalogr. Clin. Neurophysiol. Suppl. 34, 245–249.
Goldstein, M. R., Plante, D. T., Hulse, B. K., Sarasso, S., Landsness, E. C., Tononi, G., and Benca, R. M. (2011). Overnight changes in waking auditory evoked potential amplitude reflect altered sleep homeostasis in major depression. Acta Psychiatr. Scand. 1–10.
Greischar, L. L., Burghy, C. A., Van Reekum, C. M., Jackson, D. C., Pizzagalli, D. A., Mueller, C., and Davidson, R. J. (2004). Effects of electrode density and electrolyte spreading in dense array electroencephalographic recording. Clin. Neurophysiol. 115, 710–720.
Hasselmo, M. E. (1999). Neuromodulation: acetylcholine and memory consolidation. Trends Cogn. Sci. (Regul. Ed.) 3, 351–359.
Hemmeter, U. M., Hemmeter-Spernal, J., and Krieg, J. C. (2010). Sleep deprivation in depression. Expert Rev. Neurother. 10, 1101–1115.
Hill, S., and Tononi, G. (2005). Modeling sleep and wakefulness in the thalamocortical system. J. Neurophysiol. 93, 1671–1698.
Hill, S., Tononi, G., and Ghilardi, M. F. (2008). Sleep improves the variability of motor performance. Brain Res. Bull. 76, 605–611.
Holmes, M. D., Brown, M., and Tucker, D. M. (2004). Are “generalized” seizures truly generalized? Evidence of localized mesial frontal and frontopolar discharges in absence. Epilepsia 45, 1568–1579.
Hori, T. (1985). Spatiotemporal changes of EEG activity during waking-sleeping transition period. Int. J. Neurosci. 27, 101–114.
Horne, J. A. (1993). Human sleep, sleep loss and behaviour. Implications for the prefrontal cortex and psychiatric disorder. Br J Psychiatry 162, 413–419.
Huber, R., Esser, S. K., Ferrarelli, F., Massimini, M., Peterson, M. J., and Tononi, G. (2007). TMS-induced cortical potentiation during wakefulness locally increases slow wave activity during sleep. PLoS ONE 2, e276. doi: 10.1371/journal.pone.0000276
Huber, R., Ghilardi, M. F., Massimini, M., Ferrarelli, F., Riedner, B. A., Peterson, M. J., and Tononi, G. (2006). Arm immobilization causes cortical plastic changes and locally decreases sleep slow wave activity. Nat. Neurosci. 9, 1169–1176.
Huber, R., Ghilardi, M. F., Massimini, M., and Tononi, G. (2004). Local sleep and learning. Nature 430, 78–81.
Huber, R., Maatta, S., Esser, S. K., Sarasso, S., Ferrarelli, F., Watson, A., Ferreri, F., Peterson, M. J., and Tononi, G. (2008). Measures of cortical plasticity after transcranial paired associative stimulation predict changes in electroencephalogram slow-wave activity during subsequent sleep. J. Neurosci. 28, 7911–7918.
Hulse, B. K., Landsness, E. C., Sarasso, S., Ferrarelli, F., Guokas, J. J., Wanger, T., and Tononi, G. (2011). A postsleep decline in auditory evoked potential amplitude reflects sleep homeostasis. Clin. Neurophysiol. 122, 1549–1555.
Iglowstein, I., Jenni, O. G., Molinari, L., and Largo, R. H. (2003). Sleep duration from infancy to adolescence: reference values and generational trends. Pediatrics 111, 302–307.
Jenni, O. G., and Carskadon, M. A. (2004). Spectral analysis of the sleep electroencephalogram during adolescence. Sleep 27, 774–783.
Kandel, A., and Buzsaki, G. (1997). Cellular-synaptic generation of sleep spindles, spike-and-wave discharges, and evoked thalamocortical responses in the neocortex of the rat. J. Neurosci. 17, 6783–6797.
Kaplan, P. W., and Bauer, G. (2011). “Anoxia, coma, and brain death,” in Niedermeyer’s Electroencephalography: Basic Principles, Clinical Applications, and Related Fields, 6th Edn, eds D. L. Schomer, and F. H. Lopes Da Silva (Philadelphia, PA: Lippincott Williams & Wilkins), 435–456.
Kattler, H., Dijk, D. J., and Borbély, A. A. (1994). Effect of unilateral somatosensory stimulation prior to sleep on the sleep EEG in humans. J. Sleep Res. 3, 159–164.
Kayser, J., Tenke, C. E., Bhattacharya, N., Stuart, B. K., and Bruder, G. E. (2000). A direct comparison of Geodesic Sensor Net (128-channel) and conventional (30-channel) ERPs in tonal and phonetic odball tasks. Psychophysiology 37, S17.
Kinsbourne, M. (1973). Minimal brain dysfunction as a neurodevelopmental lag. Ann. N. Y. Acad. Sci. 205, 268–273.
Kurth, S., Ringli, M., Geiger, A., Lebourgeois, M., Jenni, O. G., and Huber, R. (2010). Mapping of cortical activity in the first two decades of life: a high-density sleep electroencephalogram study. J. Neurosci. 30, 13211–13219.
Landsness, E., Bruno, M. A., Noirhomme, Q., Riedner, B., Gosseries, O., Schnakers, C., Massimini, M., Laureys, S., Tononi, G., and Boly, M. (2011a). Electrophysiological correlates of behavioural changes in vigilance in vegetative state and minimally conscious state. Brain 134, 2222–2232.
Landsness, E. C., Ferrarelli, F., Sarasso, S., Goldstein, M. R., Riedner, B. A., Cirelli, C., Perfetti, B., Moisello, C., Ghilardi, M. F., and Tononi, G. (2011b). Electrophysiological traces of visuomotor learning and their renormalization after sleep. Clin. Neurophysiol. 122, 2418–2425.
Landsness, E. C., Goldstein, M. R., Peterson, M. J., Tononi, G., and Benca, R. M. (2011c). Antidepressant effects of selective slow wave sleep deprivation in major depression: a high-density EEG investigation. J. Psychiatr. Res. 45, 1019–1026.
Landsness, E. C., Crupi, D., Hulse, B. K., Peterson, M. J., Huber, R., Ansari, H., Coen, M., Cirelli, C., Benca, R. M., Ghilardi, M. F., and Tononi, G. (2009). Sleep-dependent improvement in visuomotor learning: a causal role for slow waves. Sleep 32, 1273–1284.
Laureys, S., and Boly, M. (2007). What is it like to be vegetative or minimally conscious? Curr. Opin. Neurol. 20, 609–613.
Lee, J., Fowler, R., Rodney, D., Cherney, L., and Small, S. L. (2010). IMITATE: An intensive computer-based treatment for aphasia based on action observation and imitation. Aphasiology 24, 449–465.
Lee, L., Harrison, L. M., and Mechelli, A. (2003). A report of the functional connectivity workshop, Dusseldorf 2002. Neuroimage 19, 457–465.
Luaute, J., Maucort-Boulch, D., Tell, L., Quelard, F., Sarraf, T., Iwaz, J., Boisson, D., and Fischer, C. (2010). Long-term outcomes of chronic minimally conscious and vegetative states. Neurology 75, 246–252.
Maatta, S., Landsness, E., Sarasso, S., Ferrarelli, F., Ferreri, F., Ghilardi, M. F., and Tononi, G. (2010). The effects of morning training on night sleep: a behavioral and EEG study. Brain Res. Bull. 82, 118–123.
Mander, B. A., Santhanam, S., Saletin, J. M., and Walker, M. P. (2011). Wake deterioration and sleep restoration of human learning. Curr. Biol. 21, R183–184.
Massimini, M., Ferrarelli, F., Huber, R., Esser, S. K., Singh, H., and Tononi, G. (2005). Breakdown of cortical effective connectivity during sleep. Science 309, 2228–2232.
Massimini, M., Huber, R., Ferrarelli, F., Hill, S., and Tononi, G. (2004). The sleep slow oscillation as a traveling wave. J. Neurosci. 24, 6862–6870.
Michel, C. M., Murray, M. M., Lantz, G., Gonzalez, S., Spinelli, L., and Grave De Peralta, R. (2004). EEG source imaging. Clin. Neurophysiol. 115, 2195–2222.
Murphy, M., Huber, R., Esser, S., Riedner, B. A., Massimini, M., Ferrarelli, F., Ghilardi, M. F., and Tononi, G. (2011). The cortical topography of local sleep. Curr. Top. Med. Chem. 11, 2438–2446.
Murphy, M., Riedner, B. A., Huber, R., Massimini, M., Ferrarelli, F., and Tononi, G. (2009). Source modeling sleep slow waves. Proc. Natl. Acad. Sci. U.S.A. 106, 1608–1613.
Murphy, T. H., and Corbett, D. (2009). Plasticity during stroke recovery: from synapse to behaviour. Nat. Rev. Neurosci. 10, 861–872.
Nichols, T. E., and Holmes, A. P. (2002). Nonparametric permutation tests for functional neuroimaging: a primer with examples. Hum. Brain Mapp. 15, 1–25.
Olcese, U., Esser, S. K., and Tononi, G. (2010). Sleep and synaptic renormalization: a computational study. J. Neurophysiol. 104, 3476–3493.
Olfson, M. (1992). Diagnosing mental disorders in office-based pediatric practice. J. Dev. Behav. Pediatr. 13, 363–365.
Papousek, H. (1961). Conditioned head rotation reflexes in infants in the first months of life. Acta Paediatr. 50, 565–576.
Pascalis, O., De Haan, M., Nelson, C. A., and De Schonen, S. (1998). Long-term recognition memory for faces assessed by visual paired comparison in 3- and 6-month-old infants. J. Exp. Psychol. Learn Mem. Cogn. 24, 249–260.
Quirk, G. J., and Beer, J. S. (2006). Prefrontal involvement in the regulation of emotion: convergence of rat and human studies. Curr. Opin. Neurobiol. 16, 723–727.
Riedner, B. A., Vyazovskiy, V. V., Huber, R., Massimini, M., Esser, S., Murphy, M., and Tononi, G. (2007). Sleep homeostasis and cortical synchronization: III. A high-density EEG study of sleep slow waves in humans. Sleep 30, 1643–1657.
Rosanova, M., Gosseries, O., Casarotto, S., Boly, M., Casali, A. G., Bruno, M. A., Mariotti, M., Boveroux, P., Tononi, G., Laureys, S., and Massimini, M. (2012). Recovery of cortical effective connectivity and recovery of consciousness in vegetative patients. Brain 1–13.
Rusterholz, T., and Achermann, P. (2011). Topographical aspects in the dynamics of sleep homeostasis in young men: individual patterns. BMC Neurosci. 12, 84. doi: 10.1186/1471-2202-12-84
Saletin, J. M., Goldstein, A. N., and Walker, M. P. (2011). The role of sleep in directed forgetting and remembering of human memories. Cereb. Cortex 21, 2534–2541.
Sarasso, S., Hung, C., Ferrarelli, F., Riedner, B. A., Cirelli, C., and Tononi, G. (2011). “Local, use-dependent changes in the sleep EEG after prolonged wakefulness,” in 25th Annual Meeting of the Associated Professional Sleep Societies (APSS): Sleep, Abstract Supplement vol. 34, Minneapolis, :104.
Sarasso, S., Santhanam, P., Maatta, S., Poryazova, R., Ferrarelli, F., Tononi, G., and Small, S. L. (2010). Non-fluent aphasia and neural reorganization after speech therapy: insights from human sleep electrophysiology and functional magnetic resonance imaging. Arch. Ital. Biol. 148, 271–278.
Schabus, M., Hodlmoser, K., Gruber, G., Sauter, C., Anderer, P., Klosch, G., Parapatics, S., Saletu, B., Klimesch, W., and Zeitlhofer, J. (2006). Sleep spindle-related activity in the human EEG and its relation to general cognitive and learning abilities. Eur. J. Neurosci. 23, 1738–1746.
Schiff, N. D. (2007). Bringing neuroimaging tools closer to diagnostic use in the severely injured brain. Brain 130, 2482–2483.
Schnakers, C., Vanhaudenhuyse, A., Giacino, J., Ventura, M., Boly, M., Majerus, S., Moonen, G., and Laureys, S. (2009). Diagnostic accuracy of the vegetative and minimally conscious state: clinical consensus versus standardized neurobehavioral assessment. BMC Neurol. 9, 35. doi: 10.1186/1471-2377-9-35
Shaw, P., Eckstrand, K., Sharp, W., Blumenthal, J., Lerch, J. P., Greenstein, D., Clasen, L., Evans, A., Giedd, J., and Rapoport, J. L. (2007). Attention-deficit/hyperactivity disorder is characterized by a delay in cortical maturation. Proc. Natl. Acad. Sci. U.S.A. 104, 19649–19654.
Shaw, P., Kabani, N. J., Lerch, J. P., Eckstrand, K., Lenroot, R., Gogtay, N., Greenstein, D., Clasen, L., Evans, A., Rapoport, J. L., Giedd, J. N., and Wise, S. P. (2008). Neurodevelopmental trajectories of the human cerebral cortex. J. Neurosci. 28, 3586–3594.
Shu, Y., Hasenstaub, A., and Mccormick, D. A. (2003). Turning on and off recurrent balanced cortical activity. Nature 423, 288–293.
Stefan, K., Kunesch, E., Cohen, L. G., Benecke, R., and Classen, J. (2000). Induction of plasticity in the human motor cortex by paired associative stimulation. Brain 123 Pt 3, 572–584.
Steriade, M. (2000). Corticothalamic resonance, states of vigilance and mentation. Neuroscience 101, 243–276.
Steriade, M., and Amzica, F. (1994). Dynamic coupling among neocortical neurons during evoked and spontaneous spike-wave seizure activity. J. Neurophysiol. 72, 2051–2069.
Steriade, M., Contreras, D., Curro Dossi, R., and Nunez, A. (1993a). The slow ( <1 Hz) oscillation in reticular thalamic and thalamocortical neurons: scenario of sleep rhythm generation in interacting thalamic and neocortical networks. J. Neurosci. 13, 3284–3299.
Steriade, M., Mccormick, D. A., and Sejnowski, T. J. (1993b). Thalamocortical oscillations in the sleeping and aroused brain. Science 262, 679–685.
Steriade, M., Nunez, A., and Amzica, F. (1993c). Intracellular analysis of relations between the slow ( <1 Hz) neocortical oscillation and other sleep rhythms of the electroencephalogram. J. Neurosci. 13, 3266–3283.
Steriade, M., Iosif, G., and Apostol, V. (1969). Responsiveness of thalamic and cortical motor relays during arousal and various stages of sleep. J. Neurophysiol. 32, 251–265.
Steriade, M., and Timofeev, I. (2003). Neuronal plasticity in thalamocortical networks during sleep and waking oscillations. Neuron 37, 563–576.
Steriade, M., Timofeev, I., and Grenier, F. (2001). Natural waking and sleep states: a view from inside neocortical neurons. J. Neurophysiol. 85, 1969–1985.
Tononi, G., and Cirelli, C. (2006). Sleep function and synaptic homeostasis. Sleep Med. Rev. 10, 49–62.
Tucker, D. M., Brown, M., Luu, P., and Holmes, M. D. (2007). Discharges in ventromedial frontal cortex during absence spells. Epilepsy Behav. 11, 546–557.
Tucker, D. M., Waters, A. C., and Holmes, M. D. (2009). Transition from cortical slow oscillations of sleep to spike-wave seizures. Clin. Neurophysiol. 120, 2055–2062.
van der Helm, E., Yao, J., Dutt, S., Rao, V., Saletin, J. M., and Walker, M. P. (2011). REM sleep depotentiates amygdala activity to previous emotional experiences. Curr. Biol. 21, 2029–2032.
Vanhatalo, S., Palva, J. M., Holmes, M. D., Miller, J. W., Voipio, J., and Kaila, K. (2004). Infraslow oscillations modulate excitability and interictal epileptic activity in the human cortex during sleep. Proc. Natl. Acad. Sci. U.S.A. 101, 5053–5057.
Vanhaudenhuyse, A., Noirhomme, Q., Tshibanda, L. J., Bruno, M. A., Boveroux, P., Schnakers, C., Soddu, A., Perlbarg, V., Ledoux, D., Brichant, J. F., Moonen, G., Maquet, P., Greicius, M. D., Laureys, S., and Boly, M. (2010). Default network connectivity reflects the level of consciousness in non-communicative brain-damaged patients. Brain 133, 161–171.
Volkow, N. D., Rosen, B., and Farde, L. (1997). Imaging the living human brain: magnetic resonance imaging and positron emission tomography. Proc. Natl. Acad. Sci. U.S.A. 94, 2787–2788.
Vyazovskiy, V. V., Cirelli, C., Pfister-Genskow, M., Faraguna, U., and Tononi, G. (2008). Molecular and electrophysiological evidence for net synaptic potentiation in wake and depression in sleep. Nat. Neurosci. 11, 200–208.
Vyazovskiy, V. V., Cirelli, C., and Tononi, G. (2011). Electrophysiological correlates of sleep homeostasis in freely behaving rats. Prog. Brain Res. 193, 17–38.
Vyazovskiy, V. V., Olcese, U., Lazimy, Y. M., Faraguna, U., Esser, S. K., Williams, J. C., Cirelli, C., and Tononi, G. (2009). Cortical firing and sleep homeostasis. Neuron 63, 865–878.
Wendel, K., Vaisanen, O., Malmivuo, J., Gencer, N. G., Vanrumste, B., Durka, P., Magjarevic, R., Supek, S., Pascu, M. L., Fontenelle, H., and Grave De Peralta Menendez, R. (2009). EEG/MEG source imaging: methods, challenges, and open issues. Comput. Intell Neurosci. 656092.
Werth, E., Achermann, P., and Borbély, A. A. (1996). Brain topography of the human sleep EEG: antero-posterior shifts of spectral power. Neuroreport 8, 123–127.
Werth, E., Achermann, P., and Borbély, A. A. (1997a). Fronto-occipital EEG power gradients in human sleep. J. Sleep Res. 6, 102–112.
Werth, E., Achermann, P., Dijk, D. J., and Borbély, A. A. (1997b). Spindle frequency activity in the sleep EEG: individual differences and topographic distribution. Electroencephalogr. Clin. Neurophysiol. 103, 535–542.
Keywords: high density EEG, sleep slow waves, source localization, synaptic homeostasis, traveling waves, cortical maturation, attention-deficit hyperactivity disorder, stroke
Citation: Lustenberger C and Huber R (2012) High density electroencephalography in sleep research: potential, problems, future perspective. Front. Neur. 3:77. doi: 10.3389/fneur.2012.00077
Received: 20 January 2012; Paper pending published: 03 February 2012;
Accepted: 20 April 2012; Published online: 14 May 2012.
Edited by:
Linda J. Larson-Prior, Washington University School of Medicine in St. Louis, USAReviewed by:
Sara J. Aton, University of Pennsylvania, USACopyright: © 2012 Lustenberger and Huber. This is an open-access article distributed under the terms of the Creative Commons Attribution Non Commercial License, which permits non-commercial use, distribution, and reproduction in other forums, provided the original authors and source are credited.
*Correspondence: Reto Huber, Child Development Center, Children’s Hospital Zurich, Steinwiesstrasse 75, 8032 Zurich, Switzerland. e-mail:cmV0by5odWJlckBraXNwaS51emguY2g=
Disclaimer: All claims expressed in this article are solely those of the authors and do not necessarily represent those of their affiliated organizations, or those of the publisher, the editors and the reviewers. Any product that may be evaluated in this article or claim that may be made by its manufacturer is not guaranteed or endorsed by the publisher.
Research integrity at Frontiers
Learn more about the work of our research integrity team to safeguard the quality of each article we publish.