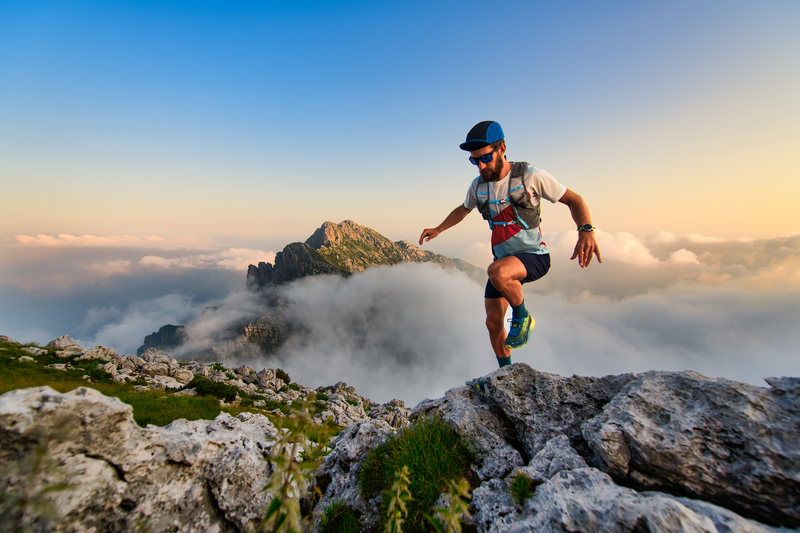
95% of researchers rate our articles as excellent or good
Learn more about the work of our research integrity team to safeguard the quality of each article we publish.
Find out more
ORIGINAL RESEARCH article
Front. Netw. Physiol. , 03 January 2025
Sec. Networks of Dynamical Systems
Volume 4 - 2024 | https://doi.org/10.3389/fnetp.2024.1525135
This article is part of the Research Topic Self-Organization of Complex Physiological Networks: Synergetic Principles and Applications — In Memory of Hermann Haken View all 3 articles
All cells in the human body, including cancer cells, possess specific electrical properties crucial for their functions. These properties are notably different between normal and cancerous cells. Cancer cells are characterized by autonomous oscillations and damped electromagnetic field (EMF) activation. Cancer reduces physiological variability, implying a systemic disconnection that desynchronizes bodily systems and their inherent random processes. The dynamics of heart rate, in this context, could reflect global physiological network instability in the sense of entrainment. Using a medical device that employs an active closed-loop system, such as administering specifically modulated EMF frequencies at targeted intervals and at low energies, we can evaluate the periodic oscillations of the heart. This procedure serves as a closed-loop control mechanism leading to a temporary alteration in plasma membrane ionic flow and the heart’s periodic oscillation dynamics. The understanding of this phenomenon is supported by computer simulations of a mathematical model, which are validated by experimental data. Heart dynamics can be quantified using difference logistic equations, and it correlates with improved overall survival rates in cancer patients.
The human organism functions as an integrated network of organs and systems (Haken, 1977; Haken, 2006; Haken and Portugali, 2016). This hierarchical functional organization of life processes is well described within the formalism of systems biology (Salvador, 2008). Organs, cells, and biomolecules are interacting across different levels to create a dynamic physiological network (Ivanov 2021; Haken, 1977). This system showcases collective behavior emerging from nonlinear and adaptive interactions involving biophysical and biochemical control, along with communication between cells and organs (Schöll, 2022). The human body exhibits a variety of rhythms and oscillations, such as circadian rhythms, cell cycles, and hormone secretion. These oscillators interact within and across tissues and cells, producing a spectrum of behaviors ranging from synchronization to chaos, which are crucial for biological adaptability and evolution. These dynamics, including synchronization phenomena in networks of coupled nonlinear oscillators, are key to understanding biological systems across scales. Such patterns, ranging from cluster synchronization to chimera states with both coherent and incoherent domains, illustrate the universal role of synchronization in both natural and technological contexts (Pikovsky et al., 2001; Rosenblum and Pikovsky, 2004; Boccaletti et al., 2018; Heltberg et al., 2021; Schöll, 2021; Sawicki et al., 2022a; Sawicki et al., 2022b; Berner et al., 2022). Thus, the frequency of oscillation (intrinsic frequency) is the crucial component for understanding the patterns of synchronization, resonance, and chaos. In complex biological systems, endogenous and external electromagnetic fields (EMF) operate as the “fast” primary messenger for physiological and pathological network behavior and control (Costa et al., 2024). Electromagnetic oscillations and synchronization of biomolecules triggered by internal and external pulses are the physical basis of the cellular electromagnetic field (Sun et al., 2022).
Cancer cell behavior has been proposed to be characterized by damped EMF activities (Pokorný et al., 2020). Damped system dynamics in biological systems are influenced by the inherent randomness of biochemical reactions, which disrupt phase coherence and reduce oscillation amplitude. In cancer patients, this leads to an energy deficit in the system, further dampening oscillations and increasing entropy, or disorder. Damping causes reduction in physiological variability that indicates systemic isolation by decoupling systems and their stochastic processes (Pincus, 1994). Physiological system coupling is often measured by variability, especially with heart rate variability serving as a common indicator of physiological variability and overall system stability. Heart rate variability measures the differences between consecutive heartbeats, known as R–R intervals (RRI), over time (Force et al., 1996; D'Angelo et al., 2023; Tiwari et al., 2021).
From the perspective of non-linear dynamics, the human cardiovascular system has a self-oscillatory character at the micro (cellular properties) and macro level. In particular, the heart rate can be synchronized such that the effect of phase locking is observed under the effect of weak external forcing (Anishchenko et al., 2000). The normal sequence and synchronous contraction of heart myocytes (e.g., heart dynamics) results from spontaneous and coordinated rapid flow of ions through ion channels located in the plasma membrane, producing a sequence of action potentials (Grant, 2009). The heart dynamics can be represented by a Van der Pol oscillator (VPO) that is extensively used to model the nonlinear behavior of heartbeats. It is a self-sustained nonlinear dissipative oscillator that exhibits chaotic switching between two types of regular motion, namely, periodic and quasiperiodic oscillations in the principal resonance region under exposure to EMF (Kadji et al., 2007; Van der Pol and Van der Mark, 1927). The driven VPO serves as a paradigmatic model for chaos in low-dimensional systems. When subjected to external forcing (e.g., Lorentz force), VPO can show not only limit cycles (asymptotically stable periodic orbits), but more complex dynamical behavior like strange attractors. Strange attractors, key to nonlinear dynamics and chaotic systems, represent an asymptotic chaotic state with fractal, i.e., non-integer dimension. This results in a system highly sensitive to small changes in initial conditions, leading to practically unpredictable temporal behavior. Cancer cells, in autoregulated damped systems, may be conceived as strange attractors that could interfere directly with the heart dynamics described by a VPO (Uthamacumaran, 2020). This interference leads to phase transition scenarios that determine the system’s initial dynamics prior to EMF exposure (Davies et al., 2011).
A predetermined active closed-loop control system is a type of feedback control system designed to automatically correct any deviations which includes perturbations with feedback dynamics (Gad-el-Hak, 2000). It is more systematically designable, and adaptable to handle noise and uncertainties, making it superior in flexibility and robustness compared to constantly active open-loop control. Closed-loop control uniquely allows for the examination and stabilization of otherwise inaccessible unstable states, offering significant practical and theoretical benefits (Macau and Grebogi, 2007; Schöll et al., 2016; Schöll, 2024). Moreover, it supports the recent advances in controlling low-dimensional chaos in nonlinear systems and its extension to spatiotemporal dynamics. In this study we use a medical device-based methodology to explore the impact of external EMF upon the heart dynamics in cancer patients to assess if heart dynamics could serve as a surrogate for system synchronization, chaos control and ultimate the patient’s initial health status.
We conducted a retrospective analysis of 22 patients submitted to identical exposure procedure at their first exposure to EMF. This corresponded to a subset of 66 adult patients enrolled in the feasibility trial reported by Capareli et al. (2023). Patients were aged 18 or older with advanced, unresectable, or metastatic hepatocellular carcinoma (HCC), confirmed histologically or clinically per American Association for the Study of Liver Diseases guidelines. They were classified as Child–Pugh A or B cirrhosis, Barcelona Clinic Liver Cancer stage B or C, and an Eastern Cooperative Oncology Group performance status of 0–2. There were no restrictions based on disease progression, hematological or organ function, or previous or current therapies. Hospital Sírio Libanês (São Paulo, Brazil) was the sole study site for the feasibility study where all participants provided written informed consent before enrollment. The trial adhered to the Declaration of Helsinki, with protocol approval by the hospital’s institutional review board, ethics committee, and the Comissão Nacional de Ética em Pesquisa. Following full enrollment, the ethics committee approved a compassionate access program under the same protocol but with a revised consent form. The trial was registered at ClinicalTrials.gov (NCT01686412) prior to starting enrollment. More information about the study population can be found elsewhere (Capareli et al., 2023).
All study participants were exposed to low-energy radio frequency EMF using the AutEMdev prototype (Autem Therapeutics NH United States), a high-precision radio frequency emitter device that controls systemic exposure of a dual signal at a carrier frequency of 27.12 MHz and modulates amplitudes from 10 Hz to 20 kHz (Tuszynski and Costa, 2022). Hemodynamic responses were monitored non-invasively and continuously using a high precision beat-to-beat recording device (Task Force Monitor or CNAP500; CNSystems, Graz, Austria), which was synchronized with the AutEMdev in the millisecond (ms). Patients underwent exposure to a pre-programmed fixed range and sequence of modulation frequencies via the AutEMdev during the discovery phase in part 2 as described by Capareli et al. (2023). Thus, each patient underwent hemodynamic monitoring for 15 min immediately prior to EMF exposure, which consisted of a 15-minute session where they were exposed to a pre-programmed fixed sequence of modulation frequencies delivered by the AutEMdev. The carrier wave amplitude-modulated in sinusoidal form with frequencies ranging from 10 to 100Hz, where each frequency increment of 1Hz was exposed sequentially for 10 s from the lowest to the highest frequency.
Digital data were saved and supported real-time cloud computing that represented a closed-loop control system. The EMF generator emitted a fixed sequence of modulation frequencies, each lasting 10 s over a 15-minute session, simultaneously with hemodynamic monitoring that collected metrics every 10 milliseconds. Every participant’s 15-minute tachogram was automatically processed: the autoregressive method was used for detecting outliers and the electro-pressure vectogram gradient phase time plots were used to study 10-second consecutive periodic orbits as described elsewhere (Capareli et al., 2023; García et al., 2023). This analysis allows the identification of instances of outlier heartbeats with significantly longer or shorter durations than expected. Next, the device produces a patient-specific series of modulation frequencies based on computing the feedback responses collected during the exposure to the fixed series of modulation frequencies. Finally, data recorded from 22 cancer patients, selected from their initial exposure to EMF were used in a retrospective correlation with survival. Full description of the exposure procedures can be found elsewhere (Capareli et al., 2023).
There is a significant link between the autonomic nervous system and cardiovascular dynamics resulting from sympathetic activity or vagal activity observed in patients with chronic disease, including cancer. However, in order to study potential direct effects of EMF (e.g., external force on the cardiac myocytes), we developed a computational heart model (e.g., isolated heart) focused on the action potential of the cardiomyocytes. The simulation of action potential in cardiomyocytes considers that the membrane is excited beyond a certain threshold, activating ion channels. This leads to ion currents flowing in or out, changing the cardiomyocytes’ potential and triggering an action potential. After this, the membrane potential resets to its resting state, ready for another excitatory input. During an action potential, the cardiomyocyte enters a refractory phase where it is temporarily unresponsive to external disturbances. The rate at which these cells depolarize varies, affecting how quickly they generate action potential. Thus, the action potential as a function of the time, where the shape of the action potential remains constant while the frequency may be changed in a wide range.
A computational heart model simulated the EMF signal coupling with the cardiomyocytes and their action potentials following Hodgkin-Huxley, FitzHugh-Nagumo, and modified VPO equations. The oscillations, variable excitability, refractoriness, and asymmetric action potentials with distinct depolarization and repolarization rates were validated to reproduce experimental data (García et al., 2023; Berenfeld and Abboud, 1996; Kumai, 2017). In the following we focus on the FitzHugh-Nagumo model, which simplifies the complex dynamics of ionic voltage-gated channels described by the Hodgkin-Huxley equations, by means of an electronic analogue that captures two basic processes (Figure 1). 1) A current
where
The FitzHugh-Nagumo model allows the input of an external current into the system, which in our case is an ionic current in phase to the local electric field delivered in the proximities of the membrane, therefore having the form:
where
In the absence of an external oscillating current I = 0 and with suitable choice of parameters, the solution of Equation 1 for the action potential evolves as an oscillating function
Figure 2. Evolution towards the limit cycle of an unforced solution of the FHN model with parameters
This trajectory is a combination of a slow and a fast dynamic part, as seen in the time series of Figure 2A and in the phase portrait of Figure 2B, which arises as a combination of a slow motion (order of
The robustness/vulnerability to external stimuli can be inspected by analyzing the system’s Phase Response Curve (PRC), which quantifies the phase shifts induced in the trajectory due to very short perturbations (
Figure 3. (A). Comparison between the limit cycle solution for the undriven system (unforced) and the delayed trajectory forced by a short external stimulus of the form of Equations 2, 3 (B). Short current stimulus applied during a negative portion of the PRC. The parameters for the current are
When subject to an external current of the form of Equation 2, the forced solutions
It has been shown that a train of pulses at appropriate frequencies and phases is able to induce time-changes in the orbit of modified Van der Pol systems (Zebrowski et al., 2007). In fact, Figures 4A–C show the impact of the effective current when the envelope of Equation 3 is applied continuously. When the frequency and starting point of the envelope train are chosen such that the external effective current is nearly zero for most of the positive regions of the Phase Response Curve, the action potential is slowed down (Figure 4A). Note that the sign in Equation 4 makes the current impulse positive whenever
Figure 4. Comparison between the limit cycle solution for the undriven system (unforced) and the trajectory forced by a continuous external stimulus represented by an effective current (Equation 4), with envelope evolving as Equation 3. Parameters
Therefore, the proposed EMF coupling can model changes in heartbeat intervals observed experimentally (Figure 8), based on ion flow dynamics. Furthermore, if a significant difference in the model parameters is expected (e.g., normal vs. cancer cells bioelectric properties), we can likely consider radical behavioral shifts in ion flow dynamics across the membrane.
When the EMF signal is delivered, the forced solution of Equation 1 can be expressed in terms of the deviations
As shown in Figures 3A, D, because of the difference between the carrier wave frequency
Here, the initial time was chosen such that the perturbations were absent (
Moving up one order in the dual time approach, it can be shown that:
Averaging over the fast variable (one cycle of
Therefore, the fast-varying current exerts an effective pull on the slow time evolution of the perturbation that is proportional to the potential (and opposite in sign with it), and proportional to the square of the envelope amplitude. Equation 8 motivates the substitution of the exact current in the original system (Equation 1) by its effective slower evolving counterpart (Equation 4).
Considering a train of pulses at appropriate frequencies and phases that interferes with the heart dynamics causing changes in heart rate variability, by time-domain, frequency domains and nonlinear analysis, we examine how the heart’s qualitative behavior changes with variations in action potential trajectories.
A difference equation model discretizes events in time by expressing each term as a function of its immediately preceding term, enabling step-by-step computation of the sequence (Buchner and Jan Żebrowski, 2001; Buchner and Żebrowski, 2000; May, 1976). We use the logistic function for the interval x of two successive spikes:
which gives the logistic difference equation, defined for
where non-trivial behavior occurs only if
Figure 5. (A). displays Difference Equation curves xt+1 = axt (1−xt) for seven cancer patients, each characterized by a specific growth rate constant
Four strata were considered: If 0 <
The RRI time series displayed a nonlinear, quasi-periodic oscillation with an average RRI of 813.257 ± 143.593 milliseconds. We observed a shift in heart rate variability when comparing conditions of non-exposure and exposure in the same patient as illustrated in Figure 6, representing a change in nonlinear dynamics of the heart induced by EMF modulation. Further results are detailed in Capareli et al. (2023). Outlier heartbeats were identified from each participant’s 15-minute tachogram and are summarized in Table 1. All participants exhibited outlier heartbeats, either as isolated incidents or cluster of events (burst), associated with specific frequencies of amplitude modulation exposure. These outlier heartbeats were significantly longer or shorter than neigborhood RRI, as shown in Figure 7. Additionally, there were more instances of decelerated (longer) outlier heartbeats than accelerated (shorter) ones. However, the mean difference in RRI was in the order of +16.57 ± 27.97 milliseconds. A number of heart rate variability metrics for time domain, frequency domain and nonlinear analysis were studied as potential predictors for <360-day survival from the first exposure, using data from patients with advanced hepatocellular carcinoma restricted to the first exposure for each patient p=1,2,....22. However, all of the heart rate variability metrics showed a low predictive accuracy determined by Receiver-Operating Characteristic (ROC) curve. On the other hand, when a difference logistic equation was used, there was a significant improvement in accuracy (Table 2). A ROC curve was constructed using the constant
Figure 6. Comparison between non-exposure vs. exposure 15-minute tachograms recorded from a cancer patient. EMF exposure caused a delay in the heart periodic oscillations. In the time-domain, EMF interference resulted in increase in RRI duration, increase in SDNNI, decrease in RMSSD. In the frequency-domain, there is a shift for high VLF power, large increment in total power and LF/HF ratio. Graphic produced by Kubios. Note: RRI: R-R intervals; RMSSD: Square root of the mean squared differences between successive RR intervals; SDNNI: Mean of the standard deviations of RR intervals in 5-min segments; VLF: very low band peak frequencies; LF: low frequency band peak frequencies; HF: high frequency band peak frequencies.
Table 1. Description of outlier heart beats identified by automated process in the 15-minute tachograms recorded from each participant during their first EMF exposure.
Figure 7. (A) The boxplot displays outlier heartbeats categorized into decelerated and accelerated types, compared to the neighboring R-R interval values. The neighboring beats consist of a total of 8–10 heartbeats, divided equally before and after the outlier heartbeat. The comparison of two sequential 10-second tachograms shows (B) progressive larger RRI and (C) trajectory effect in gradient phase plot vectograms, when no EMF effect is observed, or the deceleration effect is introduced into the time series of heart beats.
Table 2. Sensitivity and specificity by ROC curve for prediction of survival longer than 360 days from initial EMF exposure procedure in 22 patients with advanced HCC.
Figure 8. A ROC curve for 22 cancer patients is presented (left). The Kaplan-Meier curve showed significantly better overall survival for cancer patients with constant
This retrospective study with 22 cancer patients is the first to demonstrate a closed-loop control method to exam the heart dynamics under the exposure to an external low energy EMF signal at appropriate frequencies and phases acting upon the limit cycle attractor of the oscillating heart.
This noninvasive, safe and simple method provides for the first time a measurement of the deterministic interference with the ionic flow dynamics of the plasma membrane observed in excitable cells (e.g., cardiomyocytes) with a large potential of application in medicine (Mattsson et al., 2009). Moreover, the determination of heart dynamics in this setting may serve as a novel strategy to access not only cancer patients’ health status but a novel cancer treatment approach. Therefore, this novel technology could open a new treatment area combining diagnostics with therapy using systemic EMF signals (e.g., theranostics).
The heart generates by far the strongest EMF in the body, surpassing the brain’s output in both electric and magnetic strength. As the predominant source of EMF oscillations, the heart produces nonlinear interactions affecting the entire complex system’s behavior of a human being (McCraty, 2016). The heart may act as a predominant node in the body’s physiological EMF network (Schubert, 1993; Becker and Selden, 1985). The heart’s dominant EMF oscillations exert influence over practically all EMF (also called bioelectromagnetic fields) produced by the human body through its biological processes because of the electrical activities of the cells, tissues, and organs (Young et al., 2021; Abdul Kadir et al., 2018; Kaestner et al., 2018). By introducing resilient limit cycle attractors at appropriate frequency and phase determined by feedback, one can leverage the sensitivity of chaotic systems to initial conditions and provide control of chaos through feedback control. These interventions are aimed to modify the system’s energy landscape, potentially stabilizing chaotic fluctuations, and steering the system towards predictable and regular behavior. This method uses minimal energy, and it is yet crucial for enhancing control in systems where chaos is a disruptive force, making it applicable to the entire physiological system (e.g., human body). This study presents a straightforward control strategy for managing chaotic oscillations in systems such as cardiac rhythms by stabilizing a previously unstable periodic behavior inherent in the system’s natural dynamics. Our approach uses a series of pulse trains that introduce delays in the heart dynamics, potentially leading to the natural stabilization of the periodic orbit, reducing the system’s chaotic fluctuations with minimal energy loss (Pyragas, 1992; Pyragas and Tamaševičius, 1993; Pyragas, 2006; Schöll and Schuster, 2008; Purewal et al., 2014).
Heart dynamics alterations induced by EMF occur significantly more frequently in cancer patients than in healthy volunteers as observed by Capareli et al. (p < 0.0001) (Capareli et al., 2023). Low-energy EMF appear insufficient to disrupt heart dynamics in healthy volunteers, based on our experimental observation. This suggests that internal oscillators in healthy physiological states, governed by stable dynamics with feedback and flexibility, are robust and adaptable to external interference. However, as in disease, damped systems exhibit instabilities that can drive physiological networks toward phase transitions, making them more susceptible to smooth subtle external disturbances (Pokorný et al., 2020). Furthermore, the initial conditions of a system significantly influence its long-term behavior, particularly in damped systems. This importance arises from the nonlinear dynamics of these systems, where even minor differences in initial states can result in dramatically divergent outcomes, which is referred to as sensitivity to initial conditions. Consequently, by examining the heart dynamics as response to the first exposure to EMF, we can gain insights into the individual health status as reflected in the dynamics of the system’s responses. Besides, big data containing frequency-time information combined with clinical outcomes represent a powerful and unique data source. Patients exhibiting different dynamics such as bifurcation of periodic oscillations, odd-cycle periodic windows or other dynamics, appear to experience better survival outcomes. Due to the limitations of the existing experiments, the long-term effects at various timescales, such as on circadian rhythms, cannot be determined from the current clinical data. Further research using a larger dataset in prospective clinical trials is required to explore the effects of EMF on survival outcomes and long-lasting effects.
As to the underlying molecular mechanism, the resting membrane potential Vm varies across and within cell types. Mature ‘quiescent’ cells, such as neurons and cardiomyocytes, typically maintain a membrane potential Vm of about -70 mV, while cancer cells are significantly more depolarized, Vm in resting state ranging from −50 to −10 mV or even less (Di Gregorio et al., 2022). Additionally, cancer cells demonstrate heightened electrical activity, with evoked Vm ranging from 100 to 400 μV in comparison to excitable cells ranging in mV (Restaino et al., 2023). Unlike normal cells, the membrane potential of cancer cells fluctuates more frequently, with hyperpolarization events occurring 2 ± 0.2 times per cell per 1,000 s and showing about a 3% variation in Vm (Quicke et al., 2022; Quicke et al., 2021). Analysis of cellular Vm time series indicates both synchronous and asynchronous intercellular crosstalk between cancer cells, with temporal events ranging from 0.01 to 1 Hz (Quicke et al., 2022). Additionally, within the realm of cancer, membrane depolarization may play a crucial role in the development, proliferation and persistence of cancer stem cells, contributing to continuous tumor growth (Yang and Brackenbury, 2013). Given that the systemic electric field generated by the heart is around 50 mV/m, and the external EMF signal delivers an electric field energy output 100 times higher, we hypothesize that a train of pulses at specific frequencies and phases could modulate the membrane potential through ionic flow dynamics of cancer cells, reverting it to a hyperpolarized Vm through the activity of voltage-gated channels as observed in the heart (Tsong, 1988; Nawarathna et al., 2005). It is of note here that 5V/m produced by the AutEMdev is below the safety limits of EMF exposure in humans (Mattsson et al., 2009). In fact, the EMF coupling term of Equation 4 favours damping of the action potential trajectory, depending on the bioelectrical parameters of the cell (hence the resting potential), which may drive the action potential towards a polarized state. Furthermore, translational studies support the concept that the effects of EMF under the given conditions cause a calcium flux in cell culture and xenograft models leading to an activation of calcium gated channels (Jimenez et al., 2019; Sharma et al., 2019).
In conclusion, the presented data offer a new theranostic concept by a minimally invasive and rapid method of controlling ionic flow dynamics via the plasma membrane with a large potential of application in medicine. By assessing heart dynamics, this method potentially allows the diagnostic recognition of individual EMF in cancer patients (e.g., patient-specific frequencies) thereby finding new means for the translation of these diagnostic signals into therapy and possibly prognostication by EMF.
The original contributions presented in the study are included in the article/Supplementary Material, further inquiries can be directed to the corresponding author.
The studies involving humans were approved by Ethical in Research Review Board, Hospital Sírio Libanês, São Paulo Brazil. The studies were conducted in accordance with the local legislation and institutional requirements. The participants provided their written informed consent to participate in this study.
FC: Conceptualization, Data curation, Formal Analysis, Funding acquisition, Investigation, Methodology, Project administration, Resources, Software, Supervision, Validation, Visualization, Writing–original draft, Writing–review and editing. JT: Conceptualization, Data curation, Formal Analysis, Methodology, Supervision, Validation, Writing–original draft, Writing–review and editing, Investigation, Resources, Visualization. AI: Data curation, Formal Analysis, Investigation, Methodology, Supervision, Validation, Writing–original draft, Writing–review and editing. WT: Formal Analysis, Investigation, Methodology, Supervision, Validation, Writing–original draft, Writing–review and editing. BW: Formal Analysis, Investigation, Methodology, Supervision, Validation, Writing–original draft, Writing–review and editing, Project administration. ES: Formal Analysis, Methodology, Supervision, Validation, Writing–original draft, Writing–review and editing, Conceptualization, Data curation.
The author(s) declare that no financial support was received for the research, authorship, and/or publication of this article.
The authors (FPC, BW, JT, AI) disclose their employment and financial relationships with Autem Therapeutics (AT) (Autem Therapeutics LLC, Suite 208, 35 South Main Street, Hanover, NH 03755, USA). The authors receive honoraria and have stock from AT. AT produced a medical device using a non-thermal radiofrequency amplitude modulated electromagnetic fields and is conducting new clinical trials to confirm its application as a systemic cancer treatment.
The remaining authors declare that the research was conducted in the absence of any commercial or financial relationships that could be construed as a potential conflict of interest.
The author(s) declared that they were an editorial board member of Frontiers, at the time of submission. This had no impact on the peer review process and the final decision.
The author(s) declare that Generative AI was used in the creation of this manuscript. The author(s) verify and take full responsibility for the use of generative AI in the preparation of this manuscript. Generative AI was used The authors declare the use of generative artificial intelligence (AI) and AI-assisted technologies only to improve readability, grammar and spelling check in the writing process. The authors carefully reviewed and edited the results produced by AI and take full responsibility for the content of the publication.
All claims expressed in this article are solely those of the authors and do not necessarily represent those of their affiliated organizations, or those of the publisher, the editors and the reviewers. Any product that may be evaluated in this article, or claim that may be made by its manufacturer, is not guaranteed or endorsed by the publisher.
The Supplementary Material for this article can be found online at: https://www.frontiersin.org/articles/10.3389/fnetp.2024.1525135/full#supplementary-material
Abdul Kadir, L., Stacey, M., and Barrett-Jolley, R. (2018). 'Emerging roles of the membrane potential: action beyond the action potential. Front. Physiol. 9, 1661. doi:10.3389/fphys.2018.01661
Anishchenko, V. S., Balanov, A. G., Janson, N. B., Igosheva, N. B., and Bordyugov, G. V. (2000). Entrainment between heart rate and weak noninvasive forcing self-organization. Int. J. Bifurcation Chaos 10, 2339–2348. doi:10.1142/s0218127400001468
Becker, R. O., and Selden, G. C. 1985. “The body electric: electromagnetism and the foundation of life,” in William Morrow Publishers.
Berenfeld, O., and Abboud, S. (1996). Simulation of cardiac activity and the ECG using a heart model with a reaction-diffusion action potential. Med. Eng. Phys. 18, 615–625. doi:10.1016/s1350-4533(96)00028-8
Berner, R., Sawicki, J., Thiele, M., Löser, T., and Schöll, E. (2022). Critical parameters in dynamic network modeling of sepsis. Front. Netw. Physiology 2, 904480. doi:10.3389/fnetp.2022.904480
Boccaletti, S., Pisarchik, A., Genio, C., and Amann, A. 2018. Synchronization: from coupled systems to complex networks. Cambridge University Press.
Buchner, T.Jan Żebrowski (2001). Logistic map with a delayed feedback: stability of a discrete time-delay control of chaos. Phys. Rev. E, Stat. nonlinear, soft matter Phys. 63, 016210. Cambridge University Press. doi:10.1103/PhysRevE.63.016210
Buchner, T., and Żebrowski, J. J. (2000). Logistic map with a delayed feedback: stability of a discrete time-delay control of chaos. Phys. Rev. E 63, 016210. doi:10.1103/PhysRevE.63.016210
Capareli, F., Costa, F., Tuszynski, J. A., Sousa, M. C., Setogute, Y. C., Lima, P. D., et al. (2023). 'Low-energy amplitude-modulated electromagnetic field exposure: feasibility study in patients with hepatocellular carcinoma. Cancer Med. 12, 12402–12412. doi:10.1002/cam4.5944
Costa, F., Wiedenmann, B., Schöll, E., and Tuszynski, J. A. (2024). Emerging cancer therapies: targeting physiological networks and cellular bioelectrical differences with non-thermal systemic electromagnetic fields in the human body – a comprehensive review. Front. Netw. Physiology 4, 1483401. doi:10.3389/fnetp.2024.1483401
D'Angelo, J., Ritchie, S. D., Oddson, B., Gagnon, D. D., Mrozewski, T., Little, J., et al. (2023). 'Using heart rate variability methods for health-related outcomes in outdoor contexts: a scoping review of empirical studies. Int. J. Environ. Res. Public Health 20, 1330. doi:10.3390/ijerph20021330
Davies, P. C., Demetrius, L., and Tuszynski, J. A. (2011). Cancer as a dynamical phase transition. Theor. Biol. Med. Model. 8, 30. doi:10.1186/1742-4682-8-30
Di Gregorio, E., Israel, S., Staelens, M., Tankel, G., Shankar, K., and Tuszynski, J. A. (2022). The distinguishing electrical properties of cancer cells. Phys. Life Rev. 43, 139–188. doi:10.1016/j.plrev.2022.09.003
Force, E. S. C. T., Bigger, J. T., Camm, A. J., Kleiger, R. E., Malliani, A., Moss, A. J., et al. (1996). Heart rate variability: standards of measurement, physiological interpretation, and clinical use. Eur. Heart J. 17, 354–381. doi:10.1093/oxfordjournals.eurheartj.a014868
Gad-el-Hak, M. (2000). Flow control: passive, active, and reactive flow management. Cambridge University Press.
García, P., Acosta, A., Gallo, R., and Peluffo-Ordóñez, D. (2023). Positive invariant regions for a modified Van Der Pol equation modeling heart action. Appl. Math. Comput. 442, 127732. doi:10.1016/j.amc.2022.127732
Grant, A. O. (2009). Cardiac ion channels. Circulation Arrhythmia Electrophysiol. 2, 185–194. doi:10.1161/CIRCEP.108.789081
Haken, H. (1977). Synergetics: an introduction: Nonequilibrium phase transitions and self-organization in Physics, Chemistry and Biology. Springer-Verlag.
Haken, H. (2006). Information and self-organization: a macroscopic approach to complex systems. Berlin, Germany: Springer.
Haken, H., and Portugali, J. 2016. Information and self-organization: a unifying approach and applications applications. Entropy 18, 197, doi:10.3390/e18060197
Heltberg, M. L., Krishna, S., Kadanoff, L. P., and Jensen, M. H. (2021). A tale of two rhythms: locked clocks and chaos in biology. Cell. Syst. 12, 291–303. doi:10.1016/j.cels.2021.03.003
Ivanov, P.Ch (2021). The new field of network physiology: building the human physiolome. Front. Netw. Physiology 1, 711778. doi:10.3389/fnetp.2021.711778
Jimenez, H., Wang, M., Zimmerman, J. W., Pennison, M. J., Sharma, S., Surratt, T., et al. (2019). Tumour-specific amplitude-modulated radiofrequency electromagnetic fields induce differentiation of hepatocellular carcinoma via targeting Ca(v)3.2 T-type voltage-gated calcium channels and Ca(2+) influx. EBioMedicine 44, 209–224. doi:10.1016/j.ebiom.2019.05.034
Kadji, H. G., Enjieu, H., Chabi Orou, J. B., Yamapi, R., and Woafo, P. (2007). Nonlinear dynamics and strange attractors in the biological system. Chaos, Solit. and Fractals 32, 862–882. doi:10.1016/j.chaos.2005.11.063
Kaestner, L., Wang, X., Hertz, L., and Bernhardt, I. (2018). Voltage-activated ion channels in non-excitable cells-A viewpoint regarding their physiological justification. Front. Physiol. 9, 450. doi:10.3389/fphys.2018.00450
Kumai, T. (2017). Isn't there an inductance factor in the plasma membrane of nerves? Biophys. Physicobiol 14, 147–152. doi:10.2142/biophysico.14.0_147
Macau, E. E. N., and Grebogi, C. (2007). “Controlling chaos,” in Handbook of chaos control. Editors E. Schöll, and H. G. Schuster (Weinheim: Wiley VCH).
Mattsson, M.-O., Auvinen, A., Bridges, J., Norppa, H., Schüz, J., Juutilainen, J., et al. 2009. SCENIHR (Scientific Committee on Emerging and Newly Identified Health Risks), Research needs and methodology to address the remaining knowledge gaps on the potential health effects of EMF, 6 July, 2009.
May, R. M. (1976). Simple mathematical models with very complicated dynamics. Nature 261, 459–467. doi:10.1038/261459a0
McCraty, R. 2016. Science of the heart, volume 2 exploring the role of the heart in human performance an overview of research conducted by the HeartMath Institute.
Nawarathna, D., Miller, J. H., Claycomb, J. R., Cardenas, G., and Warmflash, D. (2005). Harmonic response of cellular membrane pumps to low frequency electric fields. Phys. Rev. Lett. 95, 158103. doi:10.1103/PhysRevLett.95.158103
Omelchenko, I., Omel'chenko, O., Hövel, P., and Schöll, E. (2013). When nonlocal coupling between oscillators becomes stronger: patched synchrony or multi-chimera states. Phys. Rev. Lett. 110, 224101. doi:10.1103/PhysRevLett.110.224101
Pikovsky, A., Rosenblum, M., and Kurths, J. (2001). Synchronization: a universal concept in nonlinear sciences. Cambridge: Cambridge University Press.
Pincus, S. M. (1994). 'Greater signal regularity may indicate increased system isolation. Math. Biosci. 122, 161–181. doi:10.1016/0025-5564(94)90056-6
Pokorný, J., Pokorný, J., Kobilková, J., Jandová, A., and Holaj, R. (2020). Cancer development and damped electromagnetic activity. Appl. Sci. 10, 1826. doi:10.3390/app10051826
Purewal, A. S., Postlethwaite, C. M., and Krauskopf, B. (2014). Effect of delay mismatch in Pyragas feedback control. Phys. Rev. E 90, 052905. doi:10.1103/PhysRevE.90.052905
Pyragas, K. (1992). Continuous control of chaos by self-controlling feedback. Phys. Lett. A 170, 421–428. doi:10.1016/0375-9601(92)90745-8
Pyragas, K. (2006). Delayed feedback control of chaos. Philos. Trans. A Math. Phys. Eng. Sci. 364, 2309–2334. doi:10.1098/rsta.2006.1827
Pyragas, K., and Tamaševičius, A. (1993). Experimental control of chaos by delayed self-controlling feedback. Phys. Lett. A 180, 99–102. doi:10.1016/0375-9601(93)90501-p
Quicke, P., Sun, Y., Arias-Garcia, M., Acker, C. D., Djamgoz, M. B. A., Bakal, C., et al. (2021). Membrane voltage fluctuations in human breast cancer cells. bioRxiv 2021. doi:10.1101/2021.12.20.473148
Quicke, P., Sun, Y., Arias-Garcia, M., Beykou, M., Acker, C. D., Djamgoz, M. B. A., et al. (2022). Voltage imaging reveals the dynamic electrical signatures of human breast cancer cells. Commun. Biol. 5, 1178. doi:10.1038/s42003-022-04077-2
Restaino, A. C., Walz, A., Vermeer, S. J., Barr, J., Kovács, A., Fettig, R. R., et al. (2023). Functional neuronal circuits promote disease progression in cancer. Sci. Adv. 9, eade4443. doi:10.1126/sciadv.ade4443
Rosenblum, M. G., and Pikovsky, A. S. (2004). Controlling synchronization in an ensemble of globally coupled oscillators. Phys. Rev. Lett. 92, 114102. doi:10.1103/PhysRevLett.92.114102
Salvador, A. (2008). 'Uri alon, an introduction to systems biology: design principles of biological circuits, 215. London: Chapman and Hall/CRC, 193–195. ISBN 1584886420, GBP 30.99, 2007 (320 pp.)', Mathematical Biosciences - MATH BIOSCI.
Sawicki, J., Berner, R., Löser, T., and Schöll, E. (2022a). Modeling tumor disease and sepsis by networks of adaptively coupled phase oscillators. Front. Netw. Physiology 1, 730385. doi:10.3389/fnetp.2021.730385
Sawicki, J., Hartmann, L., Bader, R., and Schöll, E. (2022b). Modelling the perception of music in brain network dynamics. Front. Netw. Physiol. 2, 910920. doi:10.3389/fnetp.2022.910920
Schöll, E. (2021). Partial synchronization patterns in brain networks. Europhys. Lett. 136, 18001. doi:10.1209/0295-5075/ac3b97
Schöll, E. (2022). Editorial: network physiology, insights in dynamical systems: 2021. Front. Netw. Physiology 2, 961339. doi:10.3389/fnetp.2022.961339
Schöll, E. (2024). “Delayed feedback control of synchronization patterns: Comment on “Control of movement of underwater swimmers: Animals, simulated animates and swimming robots” by S.Yu. Gordleeva et al,”Phys. Life Rev., 49, 112–114. doi:10.1016/j.plrev.2024.03.010
Schöll, E., Hiller, G., Hövel, P., and Dahlem, M. A. (2009). 'Time-delayed feedback in neurosystems. Philos. Trans. A Math. Phys. Eng. Sci. 367, 1079–1096. doi:10.1098/rsta.2008.0258
E. Schöll, S. Klapp, and P. Hövel (2016). Control of self-organizing nonlinear systems (Berlin: Springer).
Schubert, E. (1993). Spatial and temporal informations from the cardiac electric field. Physiol. Res. 42, 61–67.
Schultheiss, N. W., Prinz, A. A., and Butera, R. J. (2012). Phase response curves in neuroscience: theory, experiment, and analysis. New York: Springer.
Sharma, S., Wu, S. Y., Jimenez, H., Xing, F., Zhu, D., Liu, Y., et al. (2019). Ca2+ and CACNA1H mediate targeted suppression of breast cancer brain metastasis by AM RF EMF. EBioMedicine 44, 194–208. doi:10.1016/j.ebiom.2019.05.038
Sun, G., Li, J., Zhou, W., Hoyle, R. G., and Zhao, Y. (2022). Electromagnetic interactions in regulations of cell behaviors and morphogenesis. Front. Cell. Dev. Biol. 10, 1014030. doi:10.3389/fcell.2022.1014030
Tiwari, R., Kumar, R., Malik, S., Raj, T., and Kumar, P. (2021). Analysis of heart rate variability and implication of different factors on heart rate variability. Curr. Cardiol. Rev. 17, e160721189770. doi:10.2174/1573403X16999201231203854
Tsong, T. Y. (1988). Active cation pumping of Na+,K+-ATPase and sarcoplasmic reticulum Ca2+-ATPase induced by an electric field. Methods Enzymol. 157, 240–251. doi:10.1016/0076-6879(88)57080-5
Tuszynski, J. A., and Costa, F. (2022). 'Low-energy amplitude-modulated radiofrequency electromagnetic fields as a systemic treatment for cancer: review and proposed mechanisms of action. Front. Med. Technol. 4, 869155. doi:10.3389/fmedt.2022.869155
Uthamacumaran, A. (2020). 'Cancer: a turbulence problem. Neoplasia 22, 759–769. doi:10.1016/j.neo.2020.09.008
Van der Pol, B., and Van der Mark, J. (1927). 'Frequency demultiplication. Nature 120, 363–364. doi:10.1038/120363a0
Winkler, M., Dumont, G., Schöll, E., and Gutkin, B. (2022). Phase response approaches to neural activity models with distributed delay. Biol. Cybern. 116, 191–203. doi:10.1007/s00422-021-00910-9
Yang, M., and Brackenbury, W. J. (2013). 'Membrane potential and cancer progression. Front. Physiol. 4, 185. doi:10.3389/fphys.2013.00185
Young, A., Hunt, T., and Ericson, M. (2021). The slowest shared resonance: a review of electromagnetic field oscillations between central and peripheral nervous systems. Front. Hum. Neurosci. 15, 796455. doi:10.3389/fnhum.2021.796455
Keywords: non-thermal electromagnetic fields, radiofrequency, cancer treatment, cancer cells, oscillations, resonance, synchronization, chaos control
Citation: Costa FP, Tuszynski J, Iemma AF, Trevizan WA, Wiedenmann B and Schöll E (2025) External low energy electromagnetic fields affect heart dynamics: surrogate for system synchronization, chaos control and cancer patient’s health. Front. Netw. Physiol. 4:1525135. doi: 10.3389/fnetp.2024.1525135
Received: 08 November 2024; Accepted: 03 December 2024;
Published: 03 January 2025.
Edited by:
Igor Franović, University of Belgrade, SerbiaReviewed by:
Alexander E. Hramov, Immanuel Kant Baltic Federal University, RussiaCopyright © 2025 Costa, Tuszynski, Iemma, Trevizan, Wiedenmann and Schöll. This is an open-access article distributed under the terms of the Creative Commons Attribution License (CC BY). The use, distribution or reproduction in other forums is permitted, provided the original author(s) and the copyright owner(s) are credited and that the original publication in this journal is cited, in accordance with accepted academic practice. No use, distribution or reproduction is permitted which does not comply with these terms.
*Correspondence: Frederico P. Costa, ZnJlZGVyaWNvLmNvc3RhQGhzbC5vcmcuYnI=
Disclaimer: All claims expressed in this article are solely those of the authors and do not necessarily represent those of their affiliated organizations, or those of the publisher, the editors and the reviewers. Any product that may be evaluated in this article or claim that may be made by its manufacturer is not guaranteed or endorsed by the publisher.
Research integrity at Frontiers
Learn more about the work of our research integrity team to safeguard the quality of each article we publish.