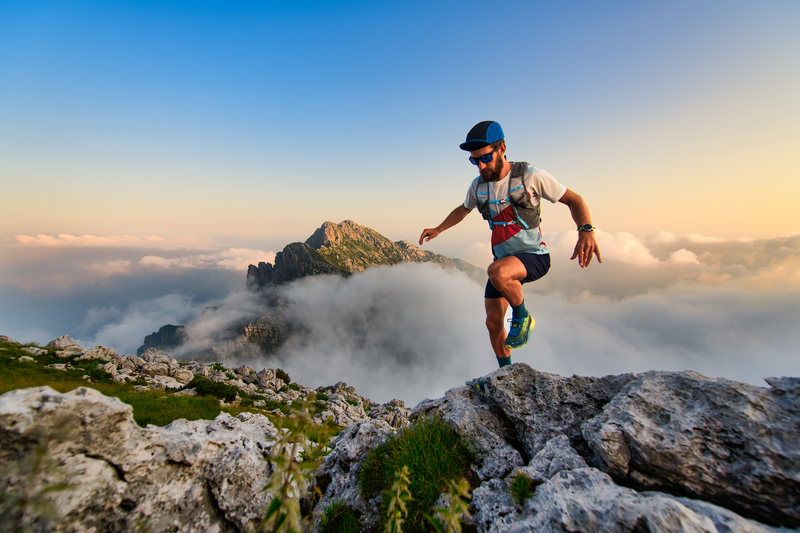
95% of researchers rate our articles as excellent or good
Learn more about the work of our research integrity team to safeguard the quality of each article we publish.
Find out more
ORIGINAL RESEARCH article
Front. Netw. Physiol. , 21 August 2024
Sec. Networks of Dynamical Systems
Volume 4 - 2024 | https://doi.org/10.3389/fnetp.2024.1436046
This article is part of the Research Topic Metastable Dynamics in Physiological Networks View all articles
Oscillatory complex networks in the metastable regime have been used to study the emergence of integrated and segregated activity in the brain, which are hypothesised to be fundamental for cognition. Yet, the parameters and the underlying mechanisms necessary to achieve the metastable regime are hard to identify, often relying on maximising the correlation with empirical functional connectivity dynamics. Here, we propose and show that the brain’s hierarchically modular mesoscale structure alone can give rise to robust metastable dynamics and (metastable) chimera states in the presence of phase frustration. We construct unweighted 3-layer hierarchical networks of identical Kuramoto-Sakaguchi oscillators, parameterized by the average degree of the network and a structural parameter determining the ratio of connections between and within blocks in the upper two layers. Together, these parameters affect the characteristic timescales of the system. Away from the critical synchronization point, we detect the emergence of metastable states in the lowest hierarchical layer coexisting with chimera and metastable states in the upper layers. Using the Laplacian renormalization group flow approach, we uncover two distinct pathways towards achieving the metastable regimes detected in these distinct layers. In the upper layers, we show how the symmetry-breaking states depend on the slow eigenmodes of the system. In the lowest layer instead, metastable dynamics can be achieved as the separation of timescales between layers reaches a critical threshold. Our results show an explicit relationship between metastability, chimera states, and the eigenmodes of the system, bridging the gap between harmonic based studies of empirical data and oscillatory models.
The macroscopic dynamics of the human brain are characterized by a balance and flexible switching between integrated and segregated activity (Fox and Raichle, 2007). The coexistence of both segregative and integrative tendencies is thought to be essential for healthy brain functioning and cognitive processing (Tognoli and Kelso, 2014; López-González et al., 2021; Wang et al., 2021; Capouskova et al., 2023). In coordination dynamics (Kelso, 2013), this coexistence is the defining feature of complex dynamical systems in the metastable regime (Tognoli and Kelso, 2014; Hancock et al., 2023a). In the last 2 decades, various indexes of metastability have been proposed to quantify these dynamical behaviours from empirical data (Hancock et al., 2023a). Recently, measures of metastability have been successful in characterizing changes in the brain’s metastable-like dynamical features in the presence of pathological or pharmacological alterations (Lee et al., 2018; Lord et al., 2019; Hancock et al., 2023b), brain injury (Hellyer et al., 2015), the aging brain (Cabral et al., 2017) [as well as the aging rat brain (Alteriis et al., 2024)], and after brain stimulation (Bapat et al., 2024).
Motivated by these empirical observations, numerous whole-brain dynamical models have been employed to reproduce the metastable features of macroscopic brain dynamics (Cabral et al., 2014; Hansen et al., 2015). A whole-brain model consists of a complex dynamical system in which each of the constituent elements interacts with other elements according to the structural connectivity of real brains. The most frequently used indexes of metastability are the variation of the Kuramoto Order Parameter (KOP) (Shanahan, 2010) and the variation of the leading eigenvector of the instantaneous phase locking matrix and its eigenvalue (Cabral et al., 2017; Alteriis et al., 2024) (see review (Hancock et al., 2023a) for an in-depth discussion). Whole-brain oscillatory based models, in particular, allow for an extensive exploration of the structural and dynamical parameter space, enabling researchers to identify key parameters that dictate the models’ behaviours in the metastable regime (Cabral et al., 2011; Deco et al., 2017; Torres et al., 2024). A large number of key control parameters have been suggested to play a vital role in the emergence of metastable-like features. For instance, heterogeneity in couplings, delays, and connectivity has been shown to be crucial for the emergence of metastable states that synchronize at frequencies lower than the average oscillator frequency (Cabral et al., 2022). Turning to the role of structure, graph theoretical properties of connectomes have been shown to alter indexes of metastability (Váša et al., 2015) and similar measures of functional complexity (Zamora-López et al., 2016).
In these models, indexes of metastability have been hypothesised to peak when the correlation with empirical dynamical functional connectivity (dFC) is maximised (Deco and Kringelbach, 2016) [however, see also (Pope et al., 2023)]. Thus, most studies primarily focus on the properties of a system in the metastable regime and how the system’s parameters change these observations. However, the criteria that should be satisfied by an oscillatory system to achieve the metastable regime, and the mechanisms underpinning metastability, are not fully understood. As suggested in (Cabral et al., 2022), understanding the mechanisms that give rise to the emergence of metastable states is essential to be able to make predictions about the appearance and duration of specific metastable modes, and eventually to design possible therapeutic interventions.
In dynamical systems theory, metastable-like dynamics have been observed in various systems of oscillators. Here, metastability is usually associated with instability of chimera states: symmetry breaking states characterised by the coexistence of coherent and incoherent patterns in systems of phase-lagged identical oscillators (Abrams and Strogatz, 2004; Panaggio and Abrams, 2015; Haugland, 2021). For instance, in (Shanahan, 2010), metastable chimera states arise as a result of winnerless competition between modules of identical oscillators to join the coalition of synchronized modules. Similar metastable behaviours can be found in (Bick, 2018) due to the addition of higher-order interactions. Chaos, turbulence, and other dynamics with metastable-like features have also been shown to arise in the case of heterogeneous couplings and phase lags in the two-population model (Bick et al., 2018). Thus, these studies suggest that the interplay between modular structures, coupling heterogeneities, and phase frustration is necessary for metastable chimera states to arise. The effects of non-local hierarchical topologies on chimera states have also been investigated. In these settings, the structural symmetries and the clustering coefficient have been found to be important parameters for the emergence of chimera states in networks of Van der Pol oscillators (Ulonska et al., 2016). Closer to the approach taken in this study, authors in (Makarov et al., 2019) analyzed a system of Kuramoto-Sakaguchi oscillators composed of subnetworks connected via hub nodes placed on a ring. The authors showed how the size and connectivity of the subnetworks promote a competition mechanism between scales which results in an expansion of the range of parameters within which chimera states arise when compared to the case without subnetworks. Hence, hierarchical network properties seem to promote the emergence of chimera states, but their role in achieving the metastable regime and the specific mechanisms enabled by hierarchical structures remain unclear.
Some insights have come from computational neuroscience studies that explored in more detail effects of the hierarchically modular structure of the brain (Meunier et al., 2010), such as Griffith phases (Rubinov et al., 2011; Moretti and Muñoz, 2013; Ódor et al., 2015). In particular, recent research suggested that including the brain’s mesoscale connectivity in whole-brain oscillatory models can help us identify and understand the mechanisms underlying metastable dynamics. In (Mackay et al., 2023), the authors generated spatially constrained random networks to approximate the mesoscale neocortex, allowing the implementation of heterogeneous phase delays in a model of non-identical Kuramoto oscillators. The authors observed how the topology of these constrained networks widens the range of critical couplings within which metastable behaviours arise, analogous to Griffith phases. In (Villegas et al., 2014) instead, the authors studied in detail real and synthetic hierarchically modular brain networks of oscillators in the absence of phase frustration. Whilst it is well known that the hierarchical network structure affects the dynamics of oscillatory systems in the path towards global synchronization (Arenas et al., 2006; Arenas and Díaz-Guilera, 2007; Villegas et al., 2022), in (Villegas et al., 2014) the authors observed that modules of identical units at the bottom of the hierarchy synchronize first (fast timescale dynamics). However, synchronization can be lost as interactions with other modules become more significant at longer timescales, giving rise to transient metastable states on the path towards synchronization. Thus, these observations highlight how the slow dynamics associated with the lowest eigenvalues of the graph Laplacian affect the faster timescale dynamics that dictate the oscillators’ behaviour within lower-layer modules. This study, however, did not consider the effect of phase frustrations, such as phase lags or phase delays, and whether robust metastable and chimera states can persist, preventing global synchronization even in the case of identical oscillators.
Taken together, these studies suggest that modular or hierarchically modular structures, combined with either higher-order (non-additive) interactions, or the heterogeneity of both couplings and delays, are crucial to achieve the metastable regime [but also see the case for nonlinear couplings (Haugland et al., 2015; Haugland, 2021)]. In this work, we investigate the more parsimonious hypothesis that the brain’s hierarchically modular mesoscale structure alone can give rise to robust chimera states and modulate metastable dynamics in the presence of phase-frustration. Under the assumption that dFC patterns in real brains are supported by the brain’s mesoscale structural connectivity, our hypothesis is motivated by the fact that dFC patterns can be constructed using the spectral information of structural brain networks (Atasoy et al., 2016; Atasoy et al., 2017). Inspired by the network structures used in (Villegas et al., 2014; Zamora-López et al., 2016; Kang et al., 2019; Fuscà et al., 2023), we construct 3-layer hierarchical networks using a variation of the nested Stochastic Block Model (nSBM) (Peixoto, 2014). Our variation of the nSBM is parametrized by two quantities: the average degree of the network, and a structural parameter controlling the ratio of connections between and within blocks at high hierarchical layers while keeping the average degree of the network conserved and homogeneous. In the absence of coupling and oscillator frequency heterogeneities, we show how the Kuramoto-Sakaguchi dynamics on these networks display robust metastable and chimera states at different hierarchical layers depending on the mesoscale structure of the network. Through explaining the mechanisms underpinning the emergence of metastable and chimera states we elucidate an explicit relationship between these states and the eigenmodes of the graph Laplacian. In particular, we show how the system we investigated may be reduced to the classic two-population model for a specific range of the structural parameter via the Laplacian Renormalization Group (LRG) flow. Our results suggest that, while the slow eigenmodes determine the functional organization in higher coarse-grained layers, the spectral gap between fast and slow eigenmodes affects the stability of cluster synchronization in the lower fine-grained layers. We conclude by pointing towards possible future extensions of this work to further bridge the gap between harmonic based studies of empirical dFC and oscillatory based whole-brain models.
To test our hypothesis, we seek to construct hierarchically modular networks while avoiding the presence of structural heterogeneities such as network motifs, hubs and the rich club, as well as non-homogeneous degree distributions, all of which have already been studied in (Ulonska et al., 2016; Zamora-López et al., 2016; Krishnagopal et al., 2017). Hence, we seek to construct hierarchical networks with a single control parameter that smoothly varies the mesoscale organization of the network while maintaining the average degree of the network fixed and homogeneous across all vertices. Critical mesoscale structures, which, in the context of information-diffusion and synchronization dynamics, are identified as structures that emerge at a characteristic timescale in the dynamical process, are an important feature of hierarchically modular networks such as the brain (Villegas et al., 2022). Such structures naturally arise in community-structured networks such as the Stochastic Block Model (SBM) and its nested variations (nSBM) used in various computational neuroscience studies such as (Villegas et al., 2014; Kang et al., 2019; Villegas et al., 2022; Fuscà et al., 2023). Therefore, nSBMs are a good starting point for our study. In the next subsection, we introduce a single-parameter nSBM variation, allowing us to smoothly vary the system from a SBM to a nSBM for a given desired average degree.
Given an arbitrary partition
The first two constraints ensure structural homogeneity at layer level for all layers, while the third constraint was chosen to model the brain’s two-hemisphere configuration, similar to previous studies (Villegas et al., 2014; Kang et al., 2019). We assign non-zero probabilities only for edges between nodes in the same block of any layer. Then, since layer
These constraints allow to smoothly vary the system configuration from a 2-layer community structured network to a 3-layer hierarchically modular network while keeping blocks in layer 1 always almost fully connected. Finally, we obtain expressions for
where the factor
allowing us to find
Hence our model allows to choose an average degree in the range between
where each
Figure 1. Example of a 3-layer hierarchical network. (A) At each layer
Figure 2. (top) Example adjacency matrices for
We consider the underlying network to be static, and associate each node to a Kuramoto-Sakaguchi oscillator. The phase
where
To quantify the degree of synchrony of the whole system we used the Kuramoto Order Parameter (KOP)
where the real part
In a similar fashion, we defined the local KOP for a subset
Similarly to Equation 6, the real part of this measure,
Oscillatory systems are said to be in the metastable regime when they exhibit spontaneous flexible switching between segregative (incoherence) and integrative (synchronization) tendencies1 (Kelso, 2013). To quantify this switching behaviour, here we used the metastability index
The term chimera state is generally invoked to denote the coexistence of coherent and incoherent patterns in systems of identical oscillators. Over the past decade, various measures have been defined to characterize different types of chimera states (Kemeth et al., 2016; Haugland, 2021). In the case of identical sinusoidally-coupled oscillators with fixed amplitude, the local KOPs can be used to measure the degree of phase-coherence (Panaggio and Abrams, 2015) between the system’s parts. However, since an analytical characterization of chimera states is only possible in the limit of an infinite number of oscillators, an arbitrary threshold needs to be introduced,
where
where thresholds
All code and notebooks related to this work can be downloaded from (Caprioglio, 2024).
To detect metastable dynamics and chimera states, we numerically analyzed the dynamics of Kuramoto-Sakaguchi oscillators (governed by Equation 5) on 3-layer hierarchical networks at different levels of observation. We started by examining the dynamics at the whole-system level, presenting the KOP and
Figure 3. (A) Degree of synchronization,
To understand why metastable dynamics are detected as the connectivity within population increases, we analysed the populations’ local KOPs,
Figure 4. (A) Mean degree of synchronization
In good agreement with our results of Section 3.1, metastability was found to reach its minimum in region
In the same region of the parameter space in which metastable dynamics are initially detected at the whole-system layer, we encounter stable and breathing chimera states (regions
Metastable and alternating chimeras are detected in the range
In region
The remaining question is whether modules in the first layer also exhibit metastable dynamics, and whether modules in different populations display distinct dynamics depending on the symmetry-breaking states detected in layer 2.
To answer the first question, we computed the degree of metastability
Figure 5. (A) Left: Metastability index
To address our second question, we computed the average local KOP and the degree of metastability of modules within each population separately, with fixed
In the previous sections, we provided numerical evidence for the emergence of chimera and metastable states in hierarchically modular networks at different levels of observation. While in layer 2 different types of chimera states were identified depending on the mesoscale structure of the network (determined by
The systems we investigated are structurally homogeneous in each layer (uniform degree distribution). Nonetheless, a peculiar property of diffusive-like dynamics on hierarchically modular networks, such as synchronization dynamics, is the richness of distinct information-diffusion pathways across different timescales (Villegas et al., 2022). In the absence of phase frustration, blocks of oscillators synchronize hierarchically, from layer 1,until the whole system is synchronized (Arenas et al., 2006). As shown in (Arenas et al., 2006), the linearized Kuramoto model (without phase lags) reduces to a linear diffusion model governed by the graph Laplacian. The graph Laplacian is defined as
where
Figure 6. Gaps between consecutive eigenvalues
To test hypothesis (a), we adopted the Laplacian Renormalization Group (LRG) flow approach recently introduced in (Villegas et al., 2022; Villegas et al., 2023). In summary, this approach allows us to rescale the system up to a timescale
and constructed the time-rescaled Laplacian at
discarding the
Figure 7. (A) Left: example of populations’ dynamics in a stable chimera state in layer 2, where
While
To test hypothesis (b), we computed the size of the second spectral gap,
Our numerical analyses have shown the relationship between different symmetry-breaking states and the system’s hierarchically modular structure. In layers 2 and 3, chimera and metastable states are detected as the populations become more defined (with increasing
Figure 8. Structural perturbation analysis for fixed
Figure 9. Impact of heterogeneous frequencies with increasing
By randomly rewiring
With the addition of natural frequencies, the system’s coupling becomes an important factor in the dynamics. It is well known that oscillatory systems display various degrees of metastability within the range of critical couplings (Villegas et al., 2014). However, we seek to investigate the behaviour of the system away from the critical synchronization point to assess in what range of values of
One of the hallmarks of complex systems is the presence of hierarchically modular structures: systems composed of interrelated substructures whose interaction across scales is thought to be fundamental for robust and efficient information processing (Simon, 1991). The identification of interrelated subsystems and their role in shaping the dynamics and in the emergence of macroscopic collective patterns is an important ongoing research endeavour in complexity science (Jensen, 2023) and particularly in network neuroscience (Bassett and Gazzaniga, 2011). In this work, we hypothesised that the interplay between nested structures alone can give rise to chimera and metastable states in minimal brain-inspired models of phase-frustrated oscillatory systems. First, we provided evidence for the emergence of symmetry-breaking states in the absence of heterogeneous couplings, delays, and structural heterogeneities. Second, we revealed the relationships between the symmetry-breaking states we observed and the spectral properties typical of hierarchically modular networks.
Our analysis highlighted two distinct pathways towards achieving the metastable regime in different layers. First, metastable dynamics were detected in layers 2 and 3 at a point in which stable and breathing chimeras cease to exist. While it was possible to explain the emergence of stable and breathing chimera states by integrating out the fast modes of the system and reducing the network to a structure similar to that of the two-population model, the precise mechanism that leads to metastable chimera states remains unclear. Due to the small size of the rescaled system, we cannot exclude the possibility that the behaviours we observed are the result of finite size effects. Additionally, a good agreement with the results in (Abrams et al., 2008) was only possible for low values of
Second, the emergence of spontaneous (de) synchronization patterns in layer 1 was found to be related to the size of the second spectral gap. When there is enough separation of timescales and modules are structurally well defined (high modularity), each oscillator within a module has time to integrate with other units in the same module the phase-frustrated inter-module interactions. When the separation of timescales is not large enough, however, modules do not have time to integrate those interactions, resulting in the spontaneous desynchronization of oscillators within the module. This behaviour has been observed in Sections 3.3, 3.4, in which we systematically increased the average degree of the network, leading to the loss of modularity in layer 1, as well as in Section 3.5, in which modularity was lost by randomly rewiring edges in the network. We also considered the more parsimonious hypothesis that spontaneous (de) synchronization is caused by increasing the average degree of the network since this increases the number of phase-frustrated interactions in the system. However, as the average degree of the network is increased beyond a threshold value, layer 1 modules do not display increasing metastability; instead metastability reaches a plateau for all degrees
An important distinction evident in our model is that between metastability at the critical synchronization point and metastability away from the critical synchronization point. In general, at the phase transition between coherence and incoherence indexes of metastability detect the presence of large fluctuations in the global and local order parameters (Arenas et al., 2008; Villegas et al., 2014; Mackay et al., 2023) indicative of the metastable regime. In fact, these behaviours can be observed in our robustness analysis in Section 3.5 with the addition of heterogeneous frequencies. More specifically, in Figure 9 we comment on the observation that at the transition between coherence and incoherence, at around
Our systematic numerical analysis across different levels of observation allowed us to detect and compare metastable dynamics and chimera states in different hierarchical layers. However, it also highlighted the limitations of the variation of the whole-system KOP as a measure of metastability. For example, while we detected metastability at the whole-system level when the structural parameter ranged between 0.3 and 0.49, layer 2 analysis revealed the presence of stable and breathing chimera states instead. At the same time, metastable dynamics in layer 1 remained entirely undetected in our analysis at the whole-system level. In computational neuroscience, the variation of the whole-system KOP is often used to tune whole-brain dynamical models, and has been hypothesized to peak when the correlation with dFC is maximised (Deco and Kringelbach, 2016). In the model we studied, and which allows a more systematic analysis of the indexes of metastability due to the model’s inherent modular construction, the peaks in metastability were all found to be in the same region of the parameter space, except for the value of metastability of the modules in the unstable population (Figure 5C). Therefore, our findings suggest that a thorough analysis across scales should be performed when possible, and that the index of metastability should be properly defined in terms of the relevant order parameters of the system. This approach may help resolve inconsistencies in the current literature, where maximising the variation of the whole-system order parameter has not necessarily aligned with the best empirical fit (Pope et al., 2023).
When dealing with empirical structural brain networks, such analysis is often not possible due to the lack of well defined communities and consequently the absence of clearly identified relevant local order parameters of the system. Both in our model and the Shanahan model (Shanahan, 2010), where the metastability index was originally formulated, the systems exhibit a clear modular structure, inherent to the system’s construction. Consequently, the issue of identifying the relevant local KOPs is absent. Novel approaches based on the harmonic decomposition of structural connectomes (Atasoy et al., 2016; Atasoy et al., 2017) have been proposed [see also the eigen-microstate approach (Zheng et al., 2024)], which we suggest could overcome this problem. Within this framework, dynamical functional connectivities can be characterized by computing the contribution of each eigenmode of the system at each point in time (Luppi et al., 2024; Zheng et al., 2024). Inspired by the harmonic brain modes framework and the work of Villegas and colleagues (Villegas et al., 2014; Villegas et al., 2022; Villegas et al., 2023), we analysed our systems using the spectral information of the graph Laplacian. Our analysis in layer 1 showed that when the relative separation of timescales between modes is large enough the fast eigenmodes associated with dynamics in the first layer do not contribute to the dynamics of the system, since modules remain completely synchronized at all times. In layer 2, instead, we found that the slow modes of the system encode the relevant information about the system’s behaviours at higher coarse-grainings. In particular, the time-rescaled system reduced to the two-population model for a specific range of parameters. These results highlight the effectiveness of the LRG approach to identify the critical mesoscale structures with a characteristic timescale, and suggest that a similar approach may be used to identify the relevant order parameters of the system at different levels of observation, as well as the spectral profiles that may facilitate hierarchical integration (Wang et al., 2021) in oscillatory systems.
The limitations imposed on the system have allowed us to study the effects of the presence of a mesoscale structure in isolation, however, it is unclear if the behaviours we observed would persist with the addition of structural heterogeneities such as core-periphery structures, and, in particular, the presence of hubs. The rich club has been shown to change the path towards synchronization (Gómez-Gardeñes et al., 2007) when compared to random networks: rather than small clusters synchronizing first, the subset of hubs and the nodes more strongly connected to them synchronize first. The combination of rich club and modular structure has also been investigated in (Arenas and Díaz-Guilera, 2007; Zamora-López et al., 2016), highlighting the role hubs play in the formation of isolated synchronized communities and their contribution to the overall functional complexity. It remains unclear how these structural heterogeneities affect systems of phase-lagged or phase-delayed oscillators and, in particular, away from the critical regime. As noticed in (Arenas and Díaz-Guilera, 2007), the dynamical equation corresponding to a hub node is a topological average of the phases of its neighbours. In the presence of frustration, it is unclear if the frustrations get cancelled out, promoting integration and synchronization, or have the opposite effect. The answer to this will likely depend on the choice between uniform or heterogeneous lags, or, with the addition of an underlying metric space, heterogeneous delays.
Finally, we believe that the study of metastability in hyperbolic networks (Krioukov et al., 2010) of oscillators might help address these limitations. Hyperbolic networks have been shown to display many of the universal properties of real-world networks (Krioukov et al., 2010), including clustering, hubs, rich club, and modularity (Kovács and Palla, 2021; Balogh et al., 2023). Crucially, hyperbolic networks come equipped with an underlying metric space, allowing a geometric renormalization procedure (García-Pérez et al., 2018) which has been shown to detect the possible organizing principles of the brain (Zheng et al., 2020) across scales.
The original contributions presented in the study are included in the article/Supplementary Material, further inquiries can be directed to the corresponding authors.
EC: Conceptualization, Formal Analysis, Methodology, Software, Writing–original draft, Writing–review and editing. LB: Conceptualization, Funding acquisition, Methodology, Supervision, Writing–review and editing.
The author(s) declare that financial support was received for the research, authorship, and/or publication of this article. The PhD studentship of the first author was fully funded by Moogsoft Ltd. The funder had no role in the research.
The authors declare that the research was conducted in the absence of any commercial or financial relationships that could be construed as a potential conflict of interest.
All claims expressed in this article are solely those of the authors and do not necessarily represent those of their affiliated organizations, or those of the publisher, the editors and the reviewers. Any product that may be evaluated in this article, or claim that may be made by its manufacturer, is not guaranteed or endorsed by the publisher.
The Supplementary Material for this article can be found online at: https://www.frontiersin.org/articles/10.3389/fnetp.2024.1436046/full#supplementary-material
1Note the distinction between metastability and multistability. While in multistable systems a trajectory can escape a basin of attraction only due to external perturbations or noise, trajectories in metastable systems do this spontaneously (Hancock et al., 2023a).
Abrams, D. M., Mirollo, R., Strogatz, S. H., and Wiley, D. A. (2008). Solvable model for chimera states of coupled oscillators. Phys. Rev. Lett. 101 (8), 084103. doi:10.1103/physrevlett.101.084103
Abrams, D. M., and Strogatz, S. H. (2004). Chimera states for coupled oscillators. Phys. Rev. Lett. 93 (17), 174102. doi:10.1103/physrevlett.93.174102
Alteriis, G. de, MacNicol, E., Hancock, F., Ciaramella, A., Cash, D., Expert, P., et al. (2024). EiDA: a lossless approach for dynamic functional connectivity; application to fMRI data of a model of ageing. Imaging Neurosci. 2, 1–22. doi:10.1162/imag_a_00113
Arenas, A., and Díaz-Guilera, A. (2007). Synchronization and modularity in complex networks. Eur. Phys. J. Special Top. 143 (1), 19–25. doi:10.1140/epjst/e2007-00066-2
Arenas, A., Díaz-Guilera, A., Kurths, J., Moreno, Y., and Zhou, C. (2008). Synchronization in complex networks. Phys. Rep. 469 (3), 93–153. doi:10.1016/j.physrep.2008.09.002
Arenas, A., Díaz-Guilera, A., and Pérez-Vicente, C. J. (2006). Synchronization reveals topological scales in complex networks. Phys. Rev. Lett. 96 (11), 114102. doi:10.1103/physrevlett.96.114102
Atasoy, S., Deco, G., Kringelbach, M. L., and Pearson, J. (2017). Harmonic brain modes: a unifying framework for linking space and time in brain dynamics. Neurosci. 24 (3), 277–293. doi:10.1177/1073858417728032
Atasoy, S., Donnelly, I., and Pearson, J. (2016). Human brain networks function in connectome-specific harmonic waves. Nat. Commun. 7 (1), 10340. doi:10.1038/ncomms10340
Balogh, S. G., Kovács, B., and Palla, G. (2023). Maximally modular structure of growing hyperbolic networks. Commun. Phys. 6 (1), 76. doi:10.1038/s42005-023-01182-4
Bapat, R., Pathak, A., and Banerjee, A. (2024). Metastability indexes global changes in the dynamic working point of the brain following brain stimulation. Front. Neurorobotics 18, 1336438. doi:10.3389/fnbot.2024.1336438
Bassett, D. S., and Gazzaniga, M. S. (2011). Understanding complexity in the human brain. Trends Cognitive Sci. 15 (5), 200–209. doi:10.1016/j.tics.2011.03.006
Bick, C. (2018). Heteroclinic switching between chimeras. Phys. Rev. E 97 (5), 050201. doi:10.1103/physreve.97.050201
Bick, C., Panaggio, M. J., and Martens, E. A. (2018). Chaos in Kuramoto oscillator networks. Chaos Interdiscip. J. Nonlinear Sci. 28 (7), 071102. doi:10.1063/1.5041444
Buscarino, A., Frasca, M., Gambuzza, L. V., and Hövel, P. (2015). Chimera states in time-varying complex networks. Phys. Rev. E 91 (2), 022817. doi:10.1103/physreve.91.022817
Cabral, J., Castaldo, F., Vohryzek, J., Litvak, V., Bick, C., Lambiotte, R., et al. (2022). Metastable oscillatory modes emerge from synchronization in the brain spacetime connectome. Commun. Phys. 5 (1), 184. doi:10.1038/s42005-022-00950-y
Cabral, J., Hugues, E., Sporns, O., and Deco, G. (2011). Role of local network oscillations in resting-state functional connectivity. NeuroImage 57 (1), 130–139. doi:10.1016/j.neuroimage.2011.04.010
Cabral, J., Kringelbach, M. L., and Deco, G. (2014). Exploring the network dynamics underlying brain activity during rest. Prog. Neurobiol. 114, 102–131. doi:10.1016/j.pneurobio.2013.12.005
Cabral, J., Vidaurre, D., Marques, P., Magalhães, R., Silva Moreira, P., Miguel Soares, J., et al. (2017). Cognitive performance in healthy older adults relates to spontaneous switching between states of functional connectivity during rest. Sci. Rep. 7 (1), 5135. doi:10.1038/s41598-017-05425-7
Capouskova, K., Zamora-López, G., Kringelbach, M. L., and Deco, G. (2023). Integration and segregation manifolds in the brain ensure cognitive flexibility during tasks and rest. Hum. Brain Mapp. 44 (18), 6349–6363. doi:10.1002/hbm.26511
Caprioglio, E. (2024). Emergence of metastability in frustrated oscillatory networks: the key role of hierarchical modularity. Available at: https://github.com/EnricoCaprioglio/Emergence-of-metastability-in-frustrated-oscillatory-networks.
Deco, G., and Kringelbach, M. L. (2016). Metastability and coherence: extending the communication through coherence hypothesis using A whole-brain computational perspective. Trends Neurosci. 39 (3), 125–135. doi:10.1016/j.tins.2016.01.001
Deco, G., Kringelbach, M. L., Jirsa, V. K., and Ritter, P. (2017). The dynamics of resting fluctuations in the brain: metastability and its dynamical cortical core. Sci. Rep. 7 (1), 3095. doi:10.1038/s41598-017-03073-5
Feng, P., Yang, J., Wu, Y., and Liu, Z. (2023). Alternating chimera states in complex networks with modular structures. Chaos Interdiscip. J. Nonlinear Sci. 33 (3), 033136. doi:10.1063/5.0132072
Fox, M. D., and Raichle, M. E. (2007). Spontaneous fluctuations in brain activity observed with functional magnetic resonance imaging. Nat. Rev. Neurosci. 8 (9), 700–711. doi:10.1038/nrn2201
Fuscà, M., Siebenhühner, F., Wang, S. H., Myrov, V., Arnulfo, G., Nobili, L., et al. (2023). Brain criticality predicts individual levels of inter-areal synchronization in human electrophysiological data. Nat. Commun. 14 (1), 4736. doi:10.1038/s41467-023-40056-9
García-Pérez, G., Boguñá, M., and Serrano, M. Á. (2018). Multiscale unfolding of real networks by geometric renormalization. Nat. Phys. 14 (6), 583–589. doi:10.1038/s41567-018-0072-5
Gómez-Gardeñes, J., Moreno, Y., and Arenas, A. (2007). Paths to synchronization on complex networks. Phys. Rev. Lett. 98 (3), 034101. doi:10.1103/physrevlett.98.034101
Hancock, F., Rosas, F. E., McCutcheon, R. A., Cabral, J., Dipasquale, O., and Turkheimer, F. E. (2023b). Metastability as a candidate neuromechanistic biomarker of schizophrenia pathology. PLOS ONE 18 (3), e0282707. doi:10.1371/journal.pone.0282707
Hancock, F., Rosas, F. E., Zhang, M., Mediano, P. A. M., Luppi, A., Cabral, J., et al. (2023a). Metastability demystified — the foundational past, the pragmatic present, and the potential future. Preprints. doi:10.20944/preprints202307.1445.v1
Hansen, E. C., Battaglia, D., Spiegler, A., Deco, G., and Jirsa, V. K. (2015). Functional connectivity dynamics: modeling the switching behavior of the resting state. NeuroImage 105, 525–535. doi:10.1016/j.neuroimage.2014.11.001
Haugland, S. W. (2021). The changing notion of chimera states, a critical review. J. Phys. Complex. 2 (3), 032001. doi:10.1088/2632-072x/ac0810
Haugland, S. W., Schmidt, L., and Krischer, K. (2015). Self-organized alternating chimera states in oscillatory media. Sci. Rep. 5 (1), 9883. doi:10.1038/srep09883
Hellyer, P. J., Scott, G., Shanahan, M., Sharp, D. J., and Leech, R. (2015). Cognitive flexibility through metastable neural dynamics is disrupted by damage to the structural connectome. J. Neurosci. 35 (24), 9050–9063. doi:10.1523/jneurosci.4648-14.2015
Jensen, H. J. (2023). Complexity science: the study of emergence. New York, NY: Cambridge University Press.
Kang, L., Tian, C., Huo, S., and Liu, Z. (2019). A two-layered brain network model and its chimera state. Sci. Rep. 9 (1), 14389. doi:10.1038/s41598-019-50969-5
Kelso, J. A. S. (2013). “Coordination dynamics,” in Encyclopedia of complexity and systems science. New York: Springer, 1–41.
Kemeth, F. P., Haugland, S. W., Schmidt, L., Kevrekidis, I. G., and Krischer, K. (2016). A classification scheme for chimera states. Chaos Interdiscip. J. Nonlinear Sci. 26 (9), 094815. doi:10.1063/1.4959804
Kovács, B., and Palla, G. (2021). The inherent community structure of hyperbolic networks. Sci. Rep. 11 (1), 16050. doi:10.1038/s41598-021-93921-2
Krioukov, D., Papadopoulos, F., Kitsak, M., Vahdat, A., and Boguñá, M. (2010). Hyperbolic geometry of complex networks. Phys. Rev. E 82 (3), 036106. doi:10.1103/physreve.82.036106
Krishnagopal, S., Lehnert, J., Poel, W., Zakharova, A., and Schöll, E. (2017). Synchronization patterns: from network motifs to hierarchical networks. Philosophical Trans. R. Soc. A Math. Phys. Eng. Sci. 375 (2088), 20160216. doi:10.1098/rsta.2016.0216
Lee, W. H., Doucet, G. E., Leibu, E., and Frangou, S. (2018). Resting-state network connectivity and metastability predict clinical symptoms in schizophrenia. Schizophrenia Res. 201, 208–216. doi:10.1016/j.schres.2018.04.029
López-González, A., Panda, R., Ponce-Alvarez, A., Zamora-López, G., Escrichs, A., Martial, C., et al. (2021). Loss of consciousness reduces the stability of brain hubs and the heterogeneity of brain dynamics. Commun. Biol. 4 (1), 1037. doi:10.1038/s42003-021-02537-9
Lord, L. D., Expert, P., Atasoy, S., Roseman, L., Rapuano, K., Lambiotte, R., et al. (2019). Dynamical exploration of the repertoire of brain networks at rest is modulated by psilocybin. NeuroImage 199, 127–142. doi:10.1016/j.neuroimage.2019.05.060
Luppi, A. I., Uhrig, L., Tasserie, J., Signorelli, C. M., Stamatakis, E. A., Destexhe, A., et al. (2024). Local orchestration of distributed functional patterns supporting loss and restoration of consciousness in the primate brain. Nat. Commun. 15 (1), 2171. doi:10.1038/s41467-024-46382-w
Mackay, M., Huo, S., and Kaiser, M. (2023). Spatial organisation of the mesoscale connectome: a feature influencing synchrony and metastability of network dynamics. PLOS Comput. Biol. 19 (8), e1011349. doi:10.1371/journal.pcbi.1011349
Makarov, V. V., Kundu, S., Kirsanov, D. V., Frolov, N. S., Maksimenko, V. A., Ghosh, D., et al. (2019). Multiscale interaction promotes chimera states in complex networks. Commun. Nonlinear Sci. Numer. Simul. 71, 118–129. doi:10.1016/j.cnsns.2018.11.015
Meunier, D., Lambiotte, R., and Bullmore, E. T. (2010). Modular and hierarchically modular organization of brain networks. Front. Neurosci. 4, 200. doi:10.3389/fnins.2010.00200
Moretti, P., and Muñoz, M. A. (2013). Griffiths phases and the stretching of criticality in brain networks. Nat. Commun. 4 (1), 2521. doi:10.1038/ncomms3521
Ódor, G., Dickman, R., and Ódor, G. (2015). Griffiths phases and localization in hierarchical modular networks. Sci. Rep. 5 (1), 14451. doi:10.1038/srep14451
Ott, E., and Antonsen, T. M. (2008). Low dimensional behavior of large systems of globally coupled oscillators. Chaos Interdiscip. J. Nonlinear Sci. 18 (3), 037113. doi:10.1063/1.2930766
Panaggio, M. J., and Abrams, D. M. (2015). Chimera states: coexistence of coherence and incoherence in networks of coupled oscillators. Nonlinearity 28 (3), R67–R87. doi:10.1088/0951-7715/28/3/r67
Peixoto, T. P. (2014). Hierarchical block structures and high-resolution model selection in large networks. Phys. Rev. X 4 (1), 011047. doi:10.1103/physrevx.4.011047
Pope, M., Seguin, C., Varley, T. F., Faskowitz, J., and Sporns, O. (2023). Co-evolving dynamics and topology in a coupled oscillator model of resting brain function. NeuroImage 277, 120266. doi:10.1016/j.neuroimage.2023.120266
Rubinov, M., Sporns, O., Thivierge, J. P., and Breakspear, M. (2011). Neurobiologically realistic determinants of self-organized criticality in networks of spiking neurons. PLoS Comput. Biol. 7 (6), e1002038. doi:10.1371/journal.pcbi.1002038
Shanahan, M. (2010). Metastable chimera states in community-structured oscillator networks. Chaos Interdiscip. J. Nonlinear Sci. 20 (1), 013108. doi:10.1063/1.3305451
Simon, H. A. (1991). “The architecture of complexity,” in Facets of systems science. Springer US, 457–476.
Tognoli, E., and Kelso, J. A. S. (2014). The metastable brain. Neuron 81 (1), 35–48. doi:10.1016/j.neuron.2013.12.022
Torres, F. A., Otero, M., Lea-Carnall, C. A., Cabral, J., Weinstein, A., and El-Deredy, W. (2024). Emergence of multiple spontaneous coherent subnetworks from a single configuration of human connectome-coupled oscillators model. bioRxiv. doi:10.1101/2024.01.09.574845
Ulonska, S., Omelchenko, I., Zakharova, A., and Schöll, E. (2016). Chimera states in networks of Van der Pol oscillators with hierarchical connectivities. Chaos Interdiscip. J. Nonlinear Sci. 26 (9), 094825. doi:10.1063/1.4962913
Váša, F., Shanahan, M., Hellyer, P. J., Scott, G., Cabral, J., and Leech, R. (2015). Effects of lesions on synchrony and metastability in cortical networks. NeuroImage 118, 456–467. doi:10.1016/j.neuroimage.2015.05.042
Villegas, P., Gabrielli, A., Santucci, F., Caldarelli, G., and Gili, T. (2022). Laplacian paths in complex networks: information core emerges from entropic transitions. Phys. Rev. Res. 4 (3), 033196. doi:10.1103/physrevresearch.4.033196
Villegas, P., Gili, T., Caldarelli, G., and Gabrielli, A. (2023). Laplacian renormalization group for heterogeneous networks. Nat. Phys. 19 (3), 445–450. doi:10.1038/s41567-022-01866-8
Villegas, P., Moretti, P., and Muñoz, M. A. (2014). Frustrated hierarchical synchronization and emergent complexity in the human connectome network. Sci. Rep. 4 (1), 5990. doi:10.1038/srep05990
Wang, R., Liu, M., Cheng, X., Wu, Y., Hildebrandt, A., and Zhou, C. (2021). Segregation, integration, and balance of large-scale resting brain networks configure different cognitive abilities. Proc. Natl. Acad. Sci. 118 (23), e2022288118. doi:10.1073/pnas.2022288118
Zamora-López, G., Chen, Y., Deco, G., Kringelbach, M. L., and Zhou, C. (2016). Functional complexity emerging from anatomical constraints in the brain: the significance of network modularity and rich-clubs. Sci. Rep. 6 (1), 38424. doi:10.1038/srep38424
Zheng, M., Allard, A., Hagmann, P., Alemán-Gómez, Y., and Serrano, M. Á. (2020). Geometric renormalization unravels self-similarity of the multiscale human connectome. Proc. Natl. Acad. Sci. 117 (33), 20244–20253. doi:10.1073/pnas.1922248117
Keywords: metastability, network physiology, renormalisation, oscillations, chimera states, hierarchical modularity, criticality, whole-brain
Citation: Caprioglio E and Berthouze L (2024) Emergence of metastability in frustrated oscillatory networks: the key role of hierarchical modularity. Front. Netw. Physiol. 4:1436046. doi: 10.3389/fnetp.2024.1436046
Received: 21 May 2024; Accepted: 07 August 2024;
Published: 21 August 2024.
Edited by:
Joana Cabral, University of Minho, PortugalReviewed by:
Michele Allegra, Dipartimento di Fisica e Astronomia “G. Galilei”, ItalyCopyright © 2024 Caprioglio and Berthouze. This is an open-access article distributed under the terms of the Creative Commons Attribution License (CC BY). The use, distribution or reproduction in other forums is permitted, provided the original author(s) and the copyright owner(s) are credited and that the original publication in this journal is cited, in accordance with accepted academic practice. No use, distribution or reproduction is permitted which does not comply with these terms.
*Correspondence: Enrico Caprioglio, ZS5jYXByaW9nbGlvQHN1c3NleC5hYy51aw==; Luc Berthouze, bC5iZXJ0aG91emVAc3Vzc2V4LmFjLnVr
Disclaimer: All claims expressed in this article are solely those of the authors and do not necessarily represent those of their affiliated organizations, or those of the publisher, the editors and the reviewers. Any product that may be evaluated in this article or claim that may be made by its manufacturer is not guaranteed or endorsed by the publisher.
Research integrity at Frontiers
Learn more about the work of our research integrity team to safeguard the quality of each article we publish.