- Theoretical Division and Center for Nonlinear Studies (CNLS), Los Alamos National Laboratory, Los Alamos, NM, United States
Physiological networks are usually made of a large number of biological oscillators evolving on a multitude of different timescales. Phase oscillators are particularly useful in the modelling of the synchronization dynamics of such systems. If the coupling is strong enough compared to the heterogeneity of the internal parameters, synchronized states might emerge where phase oscillators start to behave coherently. Here, we focus on the case where synchronized oscillators are divided into a fast and a slow component so that the two subsets evolve on separated timescales. We assess the resilience of the slow component by, first, reducing the dynamics of the fast one using Mori-Zwanzig formalism. Second, we evaluate the variance of the phase deviations when the oscillators in the two components are subject to noise with possibly distinct correlation times. From the general expression for the variance, we consider specific network structures and show how the noise transmission between the fast and slow components is affected. Interestingly, we find that oscillators that are among the most robust when there is only a single timescale, might become the most vulnerable when the system undergoes a timescale separation. We also find that layered networks seem to be insensitive to such timescale separations.
1 Introduction
The synchronization dynamics of coupled phase oscillators finds numerous applications ranging from Josephson junctions and electrical power grids to physiological networks (Wiesenfeld et al., 1998; Pikovsky et al., 2003; Acebrón et al., 2005; Strogatz, 2014; Stiefel and Ermentrout, 2016; Ji et al., 2023). The collective behavior displayed by these systems is made possible by the interplay between the internal parameters of the individual dynamical units and the interaction coupling their degrees of freedom (Winfree, 1967; Kuramoto, 1975; Kuramoto, 1984; Strogatz, 2000). Due to the nonlinear nature of the coupling together with the complex network topology of the interaction, multiple synchronized states might exist for the same parameters and might be visited by the system due to perturbations or noise (Kramers, 1940; Dykman, 1990; DeVille, 2012; Rodrigues et al., 2016). Importantly, synchronization is not always a desirable feature. For example, in electrical power grids, a synchronous operational state ensures the good functioning and distribution of power (Blaabjerg et al., 2006; Machowski et al., 2008; Dörfler et al., 2013). The answer is less binary in physiological systems. Indeed, synchronization is of primal importance for some cognitive processes in the brain ensuring an adequate level of communication between neuronal groups (Fries, 2005). Also, synchronized dynamics emerge in healthy neuronal systems during sleep (González et al., 2023). Thus, a lack of synchronization might result in some impairment of physiological systems. However, direct connections have been drawn between the excess of synchronization in some neuronal groups and brain diseases (Uhlhaas and Singer, 2006; Popovych and Tass, 2014). Therefore, the synchronization dynamics as well as its resilience to external perturbations are topics of primal importance in order to better understand the interplay between synchronized groups of dynamical units.
Different types of synchronization might occur. For example, all the phases may converge to the same global value, which is usually referred to as phase synchronization. Another type of synchronization happens when the frequencies of all the oscillators converge to a common global value, which is referred to as phase-locked state, with time-independent phase differences. Perturbations of these synchronized states can take a great variety of forms such as external input signals injected into some internal parameters or noisy environments (DeVille, 2012; Hindes and Myers, 2015; Schäfer et al., 2017; Hindes and Schwartz, 2018; Ronellenfitsch et al., 2018; Tyloo et al., 2018; Halekotte and Feudel, 2020; Tyloo, 2022a), interruption of the interaction between some oscillators due to local failures (Soltan et al., 2017; Delabays et al., 2022), alteration of the dynamics of some units (Wang and Wang, 2019; Tyloo, 2023). Here, we are interested in networks of phase oscillators in a phase-locked state where, due to some damage to a subset of oscillators or simply because of their intrinsic characteristics, two separate timescales of the dynamics exist so that the system is divided into a fast and a slow component. This kind of timescale separation might occur for example, in the human physiological system thanks to the wide range of timescales reported (Gao et al., 2020). In such a scenario, the fast oscillators adapt to any input signal quickly compared to the ones in the slow component. Therefore, the input signals into the oscillators belonging to the fast component are transmitted differently to those in the slow component compared to the case where all oscillators evolve on the same timescale. Such timescale separation in systems of coupled phase oscillators have been used in the modelling of power systems (Kokotovic et al., 1980) and synchronization dynamics of Kuramoto oscillators with attractive and repulsive couplings (Kirillov et al., 2020). As a paradigmatic model to investigate synchronization, we use Kuramoto oscillators, but the framework presented here applies more generally to coupled dynamical systems evolving close to a stable fixed point. We consider time-correlated noisy inputs as in many relevant situations, dynamical systems are constantly pushed away from their synchronized fixed point by ambient noisy conditions (van Kampen, 1976). The resilience of the system to such perturbations can be assessed in various ways. One can estimate the size of the basin of attraction (Wiley et al., 2006; Menck et al., 2013), or evaluate the amplitude of the small fluctuations or the escape rate of large fluctuations (Tyloo, 2022b; Hindes et al., 2023). In this manuscript, we assess the resilience of the slow component in the small fluctuation regime by quantifying the phase deviations from the synchronized state. This is important as it clarifies how the features of the dynamical system affect its robustness to noise when coupled oscillators evolve over multiple timescales. Within the assumption of small fluctuation, we investigate the linear response of the system around a stable fixed point. We first account for the timescale separation applying Mori-Zwanzig formalism (Mori, 1965; Zwanzig, 1973) to the slow and fast components. This leads to a reduced dynamics of the oscillators which is equivalent to a Kron reduction of the Jacobian matrix (Kron, 1939; Dörfler and Bullo, 2012). The latter elucidate how the inputs in the fast component are transmitted to the slow one. Then, solving the linear system, we calculate the variance of the phase deviations in the slow component when time-correlated noise inputs with distinct typical correlation times are applied in each component. We show how the amplitude of the excursion essentially depends on the characteristics of the noise, as well as the system properties through the spectrum of its reduced Jacobian. In some specific settings, we are able to further predict the transmission of the noise from the fast to the slow component based on the properties of the oscillators in the fast component as well as the inter-component coupling structure. In particular, we find that some oscillators having smaller variance when there is no timescale separation, might become the ones with larger variance when there is a timescale separation, if they are well connected to the fast component. Also, when the slow and fast components are defined on a layered network, the variance is mostly insensitive to the timescale separation.
In Section 2, we introduce the model of Kuramoto oscillators with timescale separation and apply Mori-Zwanzig formalism to obtain a reduced dynamics for the slow component. In the same section, we then calculate the variance of the degrees of freedom of the oscillators in the slow component subject to time-correlated noise. In Section 3, we numerically confirm and illustrate the theory on various network structures. The conclusions are given in Section 4.
2 Timescale separation
Here, we first introduce the model of noisy phase oscillators we investigate and how the timescale separation is mathematically taken into account. Then, we describe the near-equilibrium dynamics which is captured by the linear response of the system, and apply Mori-Zwanzig formalism to obtain the time-evolution in the slow component. This enables us to calculate the moments of the phase deviations of each oscillator in the slow component. Eventually, we consider the strong coupling limit where the expression for the variance of the phase deviation can be explicitly calculated for specific network structures.
2.1 Networks of phase oscillators
We are interested in the situation where, due to an external perturbation or change in the environment, the intrinsic timescales of the individual oscillators separate into a fast and a slow subsystem. We consider a set of N oscillators each with a compact phase degree of freedom θi ∈ (−π, π] whose time-evolution is governed by the set of coupled differential equations (Kuramoto, 1975),
for i = 1, … N. The natural frequency of the ith oscillator is denoted ωi, the structure of the coupling network is given by elements bij of the adjacency matrix (Newman, 2018). Ambient noise is modelled at the ith oscillator by ηi and is taken as a time-correlated noise, uncorrelated in space, i.e.,
with
where we defined
2.2 Near-equilibrium and reduced dynamics
Even though we consider Kuramoto oscillators, the following approach applies in general to coupled dynamical systems that have a stable fixed point around which they evolve and where linearization is valid. To analyze the resilience of the slow component, we consider the dynamics of the system close to a fixed point
where we defined the matrix
which is the Jacobian of the system and is a Laplacian matrix when phase differences are between
where we denoted wα the eigenvectors of
where in the second line we defined the reduced Jacobian
Assuming a vanishing initial condition, the general solution to Eq. 7 is given by,
for
2.3 Fluctuations from the synchronized state
Various characteristics of the response can be used to determine the resilience of the coupled oscillators. When subject to stochastic inputs, a natural choice is to evaluate the magnitude of the deviations from the synchronized fixed point by calculating the variance of the phase deviations. Here, we consider time-correlated noise of the form given below Eq. 1, with
with the scalar
In the other limit where the noise correlation time is the longest timescale, one has,
Comparing the two limiting cases Eqs 11, 12, one remarks that in both variances, a significant contribution might come from the slowest eigenmodes. Note also that Eq. 10 is more generally valid in the case where
In the Supplementary Material Appendix D, we give the variance of the phases when there is no timescale separation.
To obtain more insights into the contribution from the fast component, let us consider specific situations in the strong coupling limit.
2.4 Strong coupling limit
In the strong coupling limit, one has
Within this coupling limit and some other assumptions that are specified below, one can further consider network structures that give more insights about Eq. 10.
2.4.1 Disconnected oscillators in the fast component
In the simple scenario where only a single oscillator l belongs to the fast component while all the others are in the slow one,
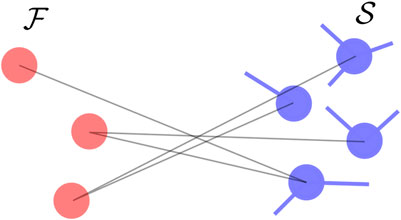
Figure 1. Connectivity where oscillators in the fast component are disconnected. The fast oscillators are shown in red while the slow oscillators are shown in blue.
2.4.2 All-to-all coupling from fast to slow component
When the oscillators in the fast component that have inter-component connections are connected to all the oscillators in the slow component with homogeneous coupling, i.e.,
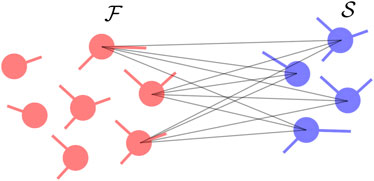
Figure 2. Connectivity where oscillators in the fast component with inter-component connections are connected to all the oscillators in the slow component (see inset). The fast oscillators are shown in red while the slow oscillators are shown in blue.
2.4.3 All-to-all from slow to fast component
In the opposite case where only a single oscillator in the slow component is homogeneously connected to all the oscillators in the fast one (see Figure 3), the intra-component structure of the coupling within the fast component does not influence the propagation of the noise. Indeed, if
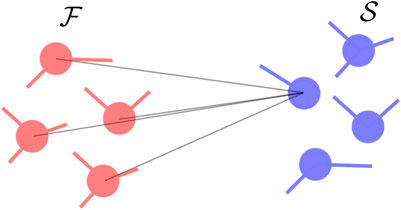
Figure 3. Connectivity where a single oscillator in the slow component is connected to all the oscillators in the fast one. The fast oscillators are shown in red while the slow oscillators are shown in blue.
2.4.4 Layered networks
An interesting case arises when oscillators are connected on layered networks so that the fast component is on one layer, the slow one on another layer, and the two layers are connected together. In the specific scenario where each fast oscillator is connected to a single distinct oscillator in the slow component and the number of units in the layers is the same, one has that
with
One can also calculate the variance when there is no timescale separation by remarking that the eigenmodes of the full Jacobian Eq. 5 are given by
While Eqs 16, 17 are different in general, they become similar when one takes the two limits of short and long correlation times. In particular, in the long correlation time limit, they only differ by a constant term given by the second term in the right-hand side of Eq. 17. We therefore expect similar variances in the cases with and without timescale separation.
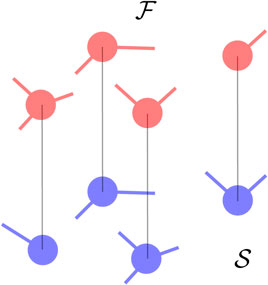
Figure 4. Connectivity in layers where the fast oscillators are shown in red in the top layer while the slow oscillators are shown in blue in the bottom layer. Each oscillator in one of the layers can be connected only to a single oscillator in the other layer.
In the following section, we illustrate and confirm numerically the results discussed so far.
3 Numerical results
Here, we first numerically confirm the theory by analyzing a network where the oscillators in the fast and slow components are randomly chosen. Then, we illustrate the theory for the specific structures discussed in Section 2.
3.1 Fast and slow components randomly chosen
We start by checking the analytical prediction when the oscillators in both components are randomly chosen. In Figure 5, we consider a Watts-Strogatz network (Newman, 2018) with m = 4 initial nearest neighbors and a rewiring probability prewiring = 0.1, of size
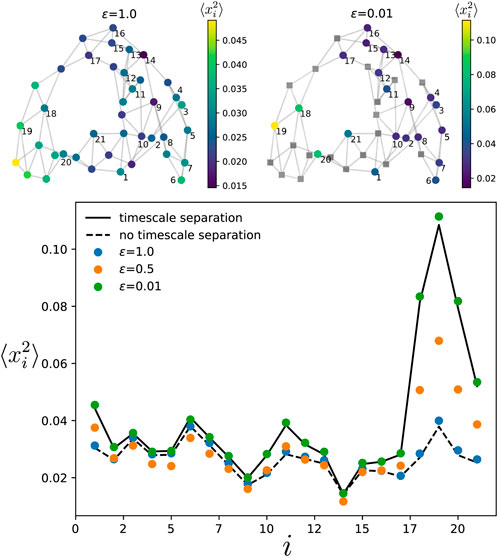
Figure 5. Variance of the phases in the slow component for the Watts-Strogatz network, with
3.2 Disconnected oscillators in the fast component
We then move to the case described Section 2.4 where the oscillators in the fast component are disconnected. In Figure 6, we numerically simulate the dynamics of Eq. 1 on a Watts-Strogatz network with m = 4 initial nearest neighbors and a rewiring probability prewiring = 0.3, of size
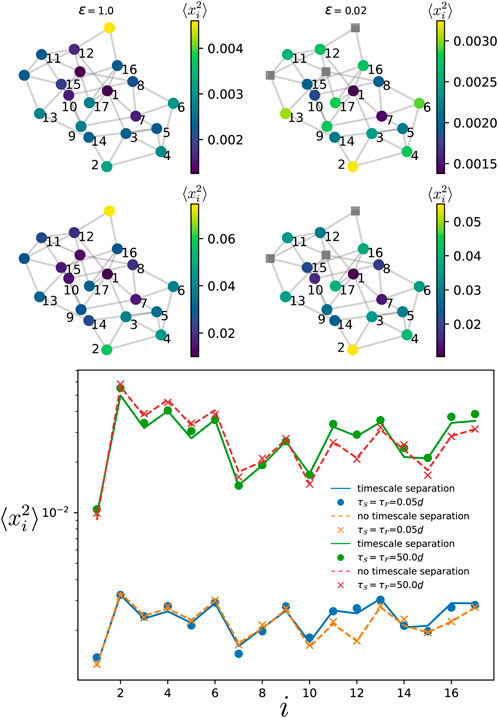
Figure 6. Variance of the phases in the slow component for the Watts-Strogatz network shown in the top panels, with
3.3 All-to-all coupling from fast to slow component
We consider the situation described in Section 2.4.2 where oscillators in the fast component that have some connections to the slow ones, are connected to all of them. The numerical results are shown on Figure 7 where this particular setting has been simulated for a modified Erdős-Rényi network of
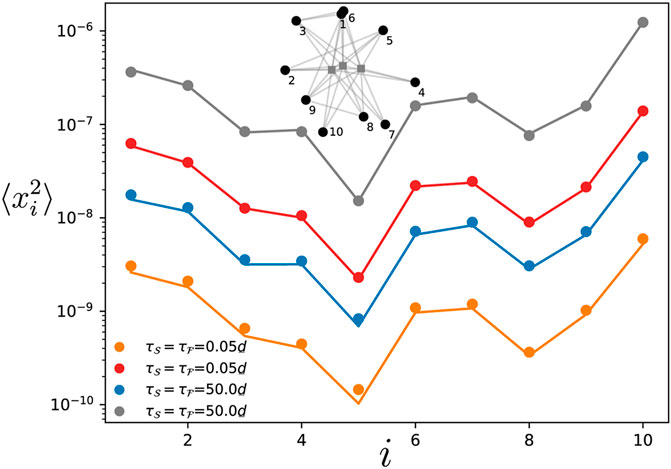
Figure 7. Variance of the phase deviations at every oscillator in the slow component. The variance is obtained by time-evolving Eq. 1 for a modified Erdős-Rényi network of 13 oscillators where three oscillators in the fast component are connected to all the oscillators in the slow component (see inset). The ratio of the damping parameters in the slow and fast component is
3.4 All-to-all from slow to fast component
In the other situation where some oscillators in the slow components are connected to a large fraction of the oscillators in the fast component, we showed in Section 2.4.3 that their variance is more important than oscillators with fewer or no connection to the fast component. This result is particularly interesting and intriguing, as in the regular situation where there is no timescale separation, oscillators that have a larger number of connections to the other elements typically have a smaller variance (Tyloo et al., 2019). Indeed, this is first illustrated in Figure 8 where the variance of each oscillator is given by the color map when there is no timescale separation (left top panels), and when there is a timescale separation (right top panels). In both correlation time limits (with
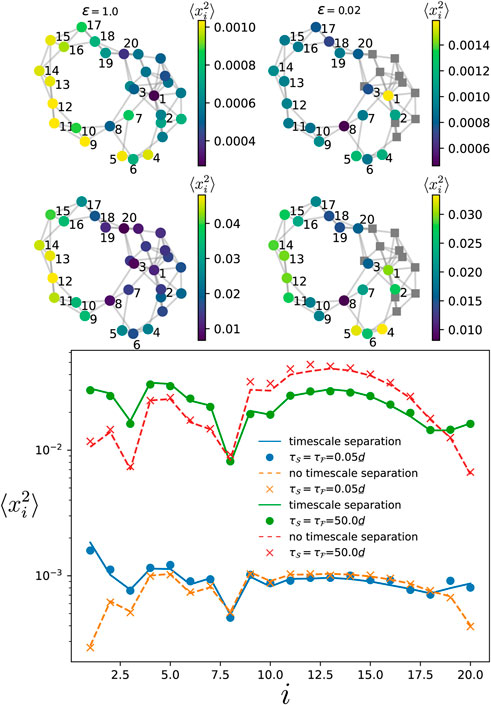
Figure 8. Variance of the phase deviations at every oscillator in the slow component when there is a timescale separation (right panels) and when there is no timescale separation (left panels), for a modified Watts-Strogatz network of
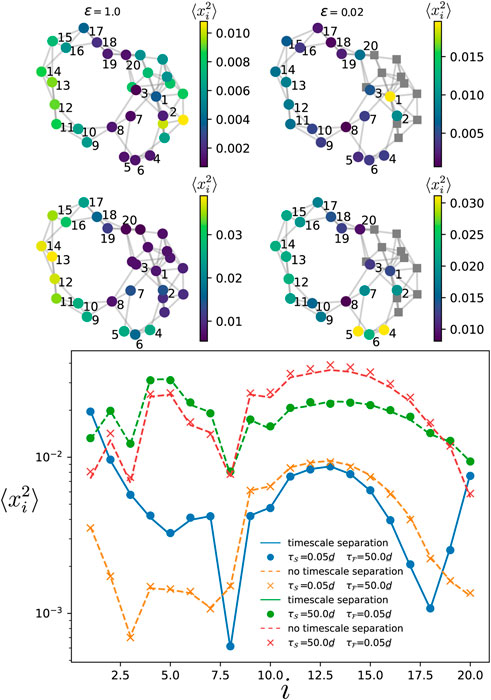
Figure 9. Variance of the phase deviations at every oscillator in the slow component when there is a timescale separation (right panels) and when there is no timescale separation (left panels), for a modified Watts-Strogatz network of
3.5 Layered networks
Here, we check the theory when the system is defined on a layered network, i.e., the slow and fast components each corresponds to one layer. We consider the specific setting where both layers have the same network connectivity and the inter-layer coupling is made through single connections between corresponding oscillators in each component (such structure are sometimes called multiplex (Newman, 2018)). In Figure 10, the numerical simulations for the variance (dots and crosses) match the theory Eqs. 10 and Eq. D1 in the Supplementary Material (solid and dashed lines) for various correlation times of the noise (homogeneous, i.e.,
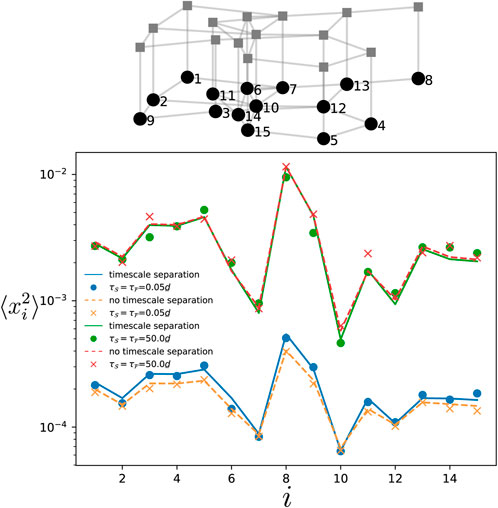
Figure 10. Variance of the phase deviations at every oscillator in the slow component. The variance is obtained by time-evolving Eq. 1 for a layered network made of two copies of an Erdős-Rényi network of 15 oscillators with edge probability 0.28 (see top panel). One layer is the fast component while the other is the slow one as depicted in the above network. The natural frequencies are identical and vanishing for all oscillators. Each dot and cross is obtained by time-averaging the variance over a single simulation of the dynamics. The solid and dashed curves give respectively, the theory Eq. 10 when there is no timescale separation (ϵ = 1), and when there is a timescale separation (ϵ =0.01). The noise amplitude is
4 Conclusion
Physiological systems are composed of a multitude of synchronized dynamical units evolving on various timescales. It is therefore relevant to investigate how these different timescales impact the synchronization dynamics of networked phase oscillators. Here, we considered networks of synchronized phase oscillators where a timescale separation divides the units into a slow and a fast component. Using Mori-Zwanzig formalism, we derived a reduced dynamical system describing the time-evolution of the slow component. We used the latter to assess the resilience of the slow component by calculating the variance of the phase deviations. We obtained a closed-form expression for the variance of each oscillator as a function of the eigenmodes of the reduced Jacobian. Interestingly, noise propagation from the fast to the slow component essentially depends on the mixing of the different eigenmodes. The precise mixing is given by the inter- and intra-component coupling structures. In particular, we showed that oscillators that have a small variance when there is no timescale separation, might have a strongly amplified variance when there is a timescale separation and they have numerous connections to the fast component. Also, we found that when the fast and slow components are connected over a layered structure, the variance of the oscillators is mostly insensitive to a timescale separation. When oscillators in the fast component are disconnected, the effect of the timescale separation remains local.
The theory presented here highlights the importance of timescales to assess the resilience of coupled phase oscillators. Some oscillators that might be the most robust within one ratio of the timescales, might become the most fragile ones for another ratio (see Figure 8, 9).
While the results of this manuscript were obtained for Kuramoto oscillators, they apply more generally to coupled dynamical system evolving close to a stable fixed point, so that the linear approximation is valid. For example, one could use the same framework to investigate coupled dynamical systems with adaptive coupling strength close to a stable fixed point. In this case, the linearization Eq. 4 will include the dynamics of the coupling strength. One can then choose which variables will undergo a timescale separation, i.e., which variables belong to the slow and fast components.
5 Future work
The present manuscript focused on a single timescale separation where the oscillators are separated into a slow and a fast component. Future research should consider more than one timescale separation to model more precisely dynamics such as physiological networks, as described in the introduction. Also, we focused here on the slow component, but one should evaluate the resilience of the fast component as well. Another assumption of these results, is that the noise is small enough such that we can only consider the small fluctuation of the slow component. One could consider transition between basins of attraction, and evaluate how the rate of large deviations is affected by the timescale separation.
Data availability statement
The original contributions presented in the study are included in the article/Supplementary Material, further inquiries can be directed to the corresponding author.
Author contributions
MT: Conceptualization, Data curation, Formal Analysis, Funding acquisition, Investigation, Methodology, Project administration, Resources, Software, Supervision, Validation, Visualization, Writing–original draft, Writing–review and editing.
Funding
The author(s) declare that financial support was received for the research, authorship, and/or publication of this article. This work has been supported by the Laboratory Directed Research and Development program of Los Alamos National Laboratory under project numbers 20220797PRD2 and 20220774ER and by U.S. DOE/OE as part of the DOE Advanced Sensor and Data Analytics Program.
Acknowledgments
We thank Francesco Caravelli for useful discussions.
Conflict of interest
The author declares that the research was conducted in the absence of any commercial or financial relationships that could be construed as a potential conflict of interest.
Publisher’s note
All claims expressed in this article are solely those of the authors and do not necessarily represent those of their affiliated organizations, or those of the publisher, the editors and the reviewers. Any product that may be evaluated in this article, or claim that may be made by its manufacturer, is not guaranteed or endorsed by the publisher.
Supplementary material
The Supplementary Material for this article can be found online at: https://www.frontiersin.org/articles/10.3389/fnetp.2024.1399352/full#supplementary-material
References
Acebrón, J. A., Bonilla, L. L., Pérez Vicente, C. J., Ritort, F., and Spigler, R. (2005). The Kuramoto model: a simple paradigm for synchronization phenomena. Rev. Mod. Phys. 77, 137–185. doi:10.1103/revmodphys.77.137
Blaabjerg, F., Teodorescu, R., Liserre, M., and Timbus, A. V. (2006). Overview of control and grid synchronization for distributed power generation systems. IEEE Trans. industrial Electron. 53, 1398–1409. doi:10.1109/tie.2006.881997
Caravelli, F., and Lin, Y. T. (2023). On the combinatorics of Lotka-Volterra equations. Available at: https://arxiv.org/abs/2308.13653.
Delabays, R., Pagnier, L., and Tyloo, M. (2022). Locating fast-varying line disturbances with the frequency mismatch. IFAC-PapersOnLine 55, 270–275. doi:10.1016/j.ifacol.2022.07.271
DeVille, L. (2012). Transitions amongst synchronous solutions in the stochastic Kuramoto model. Nonlinearity 25, 1473–1494. doi:10.1088/0951-7715/25/5/1473
Dörfler, F., and Bullo, F. (2012). Kron reduction of graphs with applications to electrical networks. IEEE Trans. Circuits Syst. I Regul. Pap. 60, 150–163. doi:10.1109/tcsi.2012.2215780
Dörfler, F., Chertkov, M., and Bullo, F. (2013). Synchronization in complex oscillator networks and smart grids. Proc. Natl. Acad. Sci. 110, 2005–2010. doi:10.1073/pnas.1212134110
Dykman, M. I. (1990). Large fluctuations and fluctuational transitions in systems driven by colored Gaussian noise: a high-frequency noise. Phys. Rev. A 42, 2020–2029. doi:10.1103/physreva.42.2020
Fries, P. (2005). A mechanism for cognitive dynamics: neuronal communication through neuronal coherence. Trends cognitive Sci. 9, 474–480. doi:10.1016/j.tics.2005.08.011
Gao, R., van den Brink, R. L., Pfeffer, T., and Voytek, B. (2020). Neuronal timescales are functionally dynamic and shaped by cortical microarchitecture. Elife 9, e61277. doi:10.7554/eLife.61277
González, J., Cavelli, M., Tort, A. B., Torterolo, P., and Rubido, N. (2023). Sleep disrupts complex spiking dynamics in the neocortex and hippocampus. Plos one 18, e0290146. doi:10.1371/journal.pone.0290146
Halekotte, L., and Feudel, U. (2020). Minimal fatal shocks in multistable complex networks. Sci. Rep. 10, 11783. doi:10.1038/s41598-020-68805-6
Hindes, J., and Myers, C. R. (2015). Driven synchronization in random networks of oscillators. Chaos 25, 073119. doi:10.1063/1.4927292
Hindes, J., and Schwartz, I. B. (2018). Rare slips in fluctuating synchronized oscillator networks. Chaos 28, 071106. doi:10.1063/1.5041377
Hindes, J., Schwartz, I. B., and Tyloo, M. (2023). Stability of Kuramoto networks subject to large and small fluctuations from heterogeneous and spatially correlated noise. Chaos 33. doi:10.1063/5.0163992
Ji, P., Wang, Y., Peron, T., Li, C., Nagler, J., and Du, J. (2023). Structure and function in artificial, zebrafish and human neural networks. Phys. Life Rev. 45, 74–111. doi:10.1016/j.plrev.2023.04.004
Kirillov, S. Y., Klinshov, V., and Nekorkin, V. I. (2020). The role of timescale separation in oscillatory ensembles with competitive coupling. Chaos Interdiscip. J. Nonlinear Sci. 30, 051101. doi:10.1063/5.0009074
Kokotovic, P. V., Allemong, J. J., Winkelman, J. R., and Chow, J. H. (1980). Singular perturbation and iterative separation of time scales. Automatica 16, 23–33. doi:10.1016/0005-1098(80)90083-7
Kramers, H. (1940). Brownian motion in a field of force and the diffusion model of chemical reactions. Physica 7, 284–304. doi:10.1016/s0031-8914(40)90098-2
Kuramoto, Y. (1975) International symposium on mathematical problems in theoretical Physics. Berlin, Heidelberg: Springer Berlin Heidelberg, 420–422.
Kuramoto, Y. (1984). “Chemical oscillations, waves and turbulence,” in Springer series in synergetics (Berlin, Heidelberg: Springer Berlin Heidelberg).
Machowski, J., Bialek, J. W., and Bumby, J. R. (2008) Power system dynamics. Chichester, U.K: Wiley.
Menck, P. J., Heitzig, J., Marwan, N., and Kurths, J. (2013). How basin stability complements the linear-stability paradigm. Nat. Phys. 9, 89–92. doi:10.1038/nphys2516
Mori, H. (1965). Transport, collective motion, and brownian motion. Prog. Theor. Phys. 33, 423–455. doi:10.1143/ptp.33.423
Pikovsky, A., Rosenblum, M., and Kurths, J. (2003) Synchronization: a universal concept in nonlinear sciences. Cambridge, United Kingdom: Cambridge University Press.
Popovych, O. V., and Tass, P. A. (2014). Control of abnormal synchronization in neurological disorders. Front. neurology 5, 268. doi:10.3389/fneur.2014.00268
Rodrigues, F. A., Peron, T. K. D., Ji, P., and Kurths, J. (2016). The Kuramoto model in complex networks. Phys. Rep. 610, 1–98. doi:10.1016/j.physrep.2015.10.008
Ronellenfitsch, H., Dunkel, J., and Wilczek, M. (2018). Optimal noise-canceling networks. Phys. Rev. Lett. 121, 208301. doi:10.1103/PhysRevLett.121.208301
Schäfer, B., Matthiae, M., Zhang, X., Rohden, M., Timme, M., and Witthaut, D. (2017). Escape routes, weak links, and desynchronization in fluctuation-driven networks. Phys. Rev. E 95, 060203(R). doi:10.1103/PhysRevE.95.060203
Soltan, S., Mazauric, D., and Zussman, G. (2017). Analysis of failures in power grids. IEEE Trans. Control Netw. Syst. 4, 288–300. doi:10.1109/tcns.2015.2498464
Stiefel, K. M., and Ermentrout, G. B. (2016). Neurons as oscillators. J. neurophysiology 116, 2950–2960. doi:10.1152/jn.00525.2015
Strogatz, S. H. (2000). From Kuramoto to Crawford: exploring the onset of synchronization in populations of coupled oscillators. Phys. D. 143, 1–20. doi:10.1016/s0167-2789(00)00094-4
Tyloo, M. (2022a). Layered complex networks as fluctuation amplifiers. J. Phys. Complex. 3, 03LT01. doi:10.1088/2632-072x/ac7e9d
Tyloo, M. (2022b). Faster network disruption from layered oscillatory dynamics. Chaos 32, 121102. doi:10.1063/5.0129123
Tyloo, M. (2023). Assessing the impact of Byzantine attacks on coupled phase oscillators. J. Phys. Complex. 4, 045005. doi:10.1088/2632-072x/ad0390
Tyloo, M., Coletta, T., and Jacquod, P. (2018). Robustness of synchrony in complex networks and generalized Kirchhoff indices. Phys. Rev. Lett. 120, 084101. doi:10.1103/PhysRevLett.120.084101
Tyloo, M., Pagnier, L., and Jacquod, P. (2019). The key player problem in complex oscillator networks and electric power grids: resistance centralities identify local vulnerabilities. Sci. Adv. 5, eaaw8359. doi:10.1126/sciadv.aaw8359
Tyloo, M., Vuffray, M., and Lokhov, A. Y. (2023). Forced oscillation source localization from generator measurements. Available at: https://arxiv.org/abs/2310.00458.
Uhlhaas, P. J., and Singer, W. (2006). Neural synchrony in brain disorders: relevance for cognitive dysfunctions and pathophysiology. neuron 52, 155–168. doi:10.1016/j.neuron.2006.09.020
van Kampen, N. G. (1976). Stochastic differential equations. Phys. Rep. 24, 171–228. doi:10.1016/0370-1573(76)90029-6
Wang, Z., and Wang, Y. (2019). Attack-resilient pulse-coupled synchronization. IEEE Trans. Control Netw. Syst. 6, 338–351. doi:10.1109/tcns.2018.2817922
Wiesenfeld, K., Colet, P., and Strogatz, S. H. (1998). Frequency locking in Josephson arrays: connection with the Kuramoto model. Phys. Rev. E 57, 1563–1569. doi:10.1103/physreve.57.1563
Wiley, D. A., Strogatz, S. H., and Girvan, M. (2006). The size of the sync basin. Chaos 16, 015103. doi:10.1063/1.2165594
Winfree, A. T. (1967). Biological rhythms and the behavior of populations of coupled oscillators. J. Theor. Biol. 16, 15–42. doi:10.1016/0022-5193(67)90051-3
Keywords: synchronization & phase locking, timescale separation, stochastic and deterministic stability, coupled oscillators, network physiology, complex networks
Citation: Tyloo M (2024) Resilience of the slow component in timescale-separated synchronized oscillators. Front. Netw. Physiol. 4:1399352. doi: 10.3389/fnetp.2024.1399352
Received: 11 March 2024; Accepted: 06 May 2024;
Published: 19 June 2024.
Edited by:
Eckehard Schöll, Technical University of Berlin, GermanyReviewed by:
Serhiy Yanchuk, University College Cork, IrelandAtefeh Ahmadi, Amirkabir University of Technology, Iran
Copyright © 2024 Tyloo. This is an open-access article distributed under the terms of the Creative Commons Attribution License (CC BY). The use, distribution or reproduction in other forums is permitted, provided the original author(s) and the copyright owner(s) are credited and that the original publication in this journal is cited, in accordance with accepted academic practice. No use, distribution or reproduction is permitted which does not comply with these terms.
*Correspondence: Melvyn Tyloo, bWVsdnluLnR5bG9vQGdtYWlsLmNvbQ==