- 1Department of Psychiatry, University of Oxford, Oxford, United Kingdom
- 2Institut Méditerranéen de Recherches Avancées (IMéRA), Aix-Marseille Université, Marseille, France
- 3Department of Mathematics and Namur Institute for Complex Systems (naXys), University of Namur, Namur, Belgium
- 4Centre for Music in the Brain, Department of Clinical Medicine, Aarhus University, Aarhus, Denmark
- 5Department of Neuroimaging, Institute of Psychiatry, Psychology and Neuroscience, King’s College London, London, United Kingdom
- 6Global Business School for Health, University College London, London, United Kingdom
In recent years, brain imaging studies have begun to shed light on the neural correlates of physiologically-reversible altered states of consciousness such as deep sleep, anesthesia, and psychedelic experiences. The emerging consensus is that normal waking consciousness requires the exploration of a dynamical repertoire enabling both global integration i.e., long-distance interactions between brain regions, and segregation, i.e., local processing in functionally specialized clusters. Altered states of consciousness have notably been characterized by a tipping of the integration/segregation balance away from this equilibrium. Historically, functional MRI (fMRI) has been the modality of choice for such investigations. However, fMRI does not enable characterization of the integration/segregation balance at sub-second temporal resolution. Here, we investigated global brain spatiotemporal patterns in electrocorticography (ECoG) data of a monkey (Macaca fuscata) under either ketamine or propofol general anesthesia. We first studied the effects of these anesthetics from the perspective of band-specific synchronization across the entire ECoG array, treating individual channels as oscillators. We further aimed to determine whether synchrony within spatially localized clusters of oscillators was differently affected by the drugs in comparison to synchronization over spatially distributed subsets of ECoG channels, thereby quantifying changes in integration/segregation balance on physiologically-relevant time scales. The findings reflect global brain dynamics characterized by a loss of long-range integration in multiple frequency bands under both ketamine and propofol anesthesia, most pronounced in the beta (13–30 Hz) and low-gamma bands (30–80 Hz), and with strongly preserved local synchrony in all bands.
1 Introduction
Brain activity is characterized by the dynamic exploration of a diverse and flexible repertoire of functional brain configurations over time (Kelso, 2012; Allen et al., 2014). These explorations of the brain’s repertoire notably enable both global integration, i.e., long-distance interactions between brain regions, and local processing in functionally specialized clusters, i.e., segregation (Sporns, 2013; Deco et al., 2015; Lord et al., 2017). The simultaneous occurrence of integration and segregation, a hallmark of complex systems displaying emerging properties (Turkheimer et al., 2019; Turkheimer et al., 2021), is thought to lead to activity patterns of sufficiently high neural complexity to underlie normal, waking consciousness (Tononi and Edelman, 1998; Cavanna et al., 2018).
It follows that events, be they pathological or pharmacological, which disrupt the integration/segregation balance should in turn modulate one’s behavior and/or subjective experience. Notably, functional MRI (fMRI) dynamic connectivity has been investigated in different sleep stages, pharmacologically induced anesthesia in humans and during psychedelic experiences (Damaraju et al., 2020; Girn et al., 2023). The emerging consensus is that unconscious states are associated with a loss of functional integration, as dynamic explorations become limited to specific patterns dominated by rigid functional configurations tied to the anatomical connectivity (Tagliazucchi et al., 2013; Cavanna et al., 2018; Demertzi et al., 2019; Luppi et al., 2020; Luppi et al., 2021a). On the other hand, psychedelic experiences, such as those induced by taking LSD or psilocybin, have been associated with increased global integration and dynamical explorations loosely constrained by the structural connectome (Carhart-Harris et al., 2014; Petri et al., 2014; Atasoy et al., 2017; Lord et al., 2019; Luppi et al., 2021b). It is however important to note that, because fMRI allows for brain activity measurements on the order of seconds, the aforementioned studies have not yet determined how the integration/segregation balance is modulated on a sub-second scale in physiologically-reversible states of altered consciousness, such as general anesthesia.
Here, we investigated global brain spatiotemporal patterns in electrocorticography (ECoG) data of monkeys under either ketamine or propofol general anesthesia from the publicly available NeuroTycho project (Yanagawa et al., 2013). While administration of both ketamine and propofol induces a rapid decrease in level of consciousness, the respective pharmacodynamics of these drugs markedly differs. Ketamine acts primarily as a NMDA receptor antagonist whereas propofol’s main target is the GABAA receptor on which it has a positive allosteric effect (Brown et al., 2011; Stevens, 2022). ECoG is an invasive imaging modality in which an electrode array is applied directly on the cortical surface. This allows for brain activity measurements on significantly faster timescales than fMRI. For example, synchronization in the beta (13–30 Hz) and low gamma (∼30–80 Hz) frequency bands between neuronal populations is thought play a crucial role in the binding of information into conscious representations (Kopell et al., 2000; Varela et al., 2001; Tallon-Baudry, 2009; Betti et al., 2021) and evidence shows it acts as a substrate for the emergence of functional networks (Lord et al., 2013a). These links between high-frequency electrical oscillations and higher level cognitive entities cannot be directly investigated with fMRI due to insufficient temporal resolution.
In the present study, we first investigated the effects of ketamine and propofol from the perspective of band-specific synchronization behavior across the entire ECoG array, analyzing the collective behavior of ECoG channels treated as oscillators. We further sought to determine whether synchrony within spatially localized clusters of oscillators was differently affected by ketamine and propofol in comparison to synchronization over spatially distributed subsets of ECoG channels. This analysis hence enabled a quantification of the dynamics underlying the integration/segregation balance under anesthesia on physiologically-relevant timescales. The findings reflect global brain dynamics characterized by a loss of global integration in multiple frequency bands under both ketamine and propofol anesthesia, most pronounced in the beta (13–30 Hz) and low-gamma bands (30–80 Hz). Furthermore, whilst long-range synchronization was reduced during anesthesia relative to wakeful rest in those same frequency bands, synchrony within spatially localized electrode clusters remained strongly conserved for both anesthetic agents. Thus, despite their distinct pharmacodynamics, the effects of ketamine and propofol strongly converge on the novel measures of neural synchronization here applied to ECoG data.
2 Methods
2.1 Electrocorticography data acquisition and preprocessing
Neuroimaging data were obtained from the NeuroTycho dataset, an open-source set of 128-channel invasive electrocorticography (ECoG) recordings in a macaca fuscata monkey collected at the Riken Institute (Tokyo, Japan) (Yanagawa et al., 2013). The ECoG array covered the left hemisphere only, with electrodes placed on both the lateral and medial brain surfaces. Data were recorded during both a resting-state baseline with eyes closed and during either propofol or ketamine-induced anesthesia in separate experiment. Each experiment was carried out least 4 days apart. During each experiment, the monkey was seated in a primate chair with both arms and head movement restricted. The “anesthesia” condition was considered to have begun once the monkey failed to respond to sensory stimulation (i.e., stimulation of the nose with a cotton swab and unresponsiveness to have their forepaw touched). In the propofol condition, the monkey was given a single bolus of intravenous propofol (5.2 mg/kg), until loss of consciousness was observed. For the ketamine condition, a single bolus of intramuscular ketamine (5.1 mg/kg) was administered. For either drug, no maintenance anesthesia was given following initial induction.
ECoG data were acquired at a 1,000 Hz sampling rate. As is common practice in electrophysiological studies, the quality of ECoG signals was assessed by visually inspecting the power spectra of individual ECoG channels, and no channels were discarded on this basis. The data were notch filtered at 50 Hz to account for electrical line noise in Japan. While we did not downsample the data, we re-binned the timecourses into 100 ms windows in order for our measures of inter-areal synchrony (see sections below) to operate on physiologically meaningful timescales. To ensure a consistent number of time points across all experimental conditions (ketamine/propofol and baseline), we considered the first 5,000 time points of each experimental run, which yields a 5,000*100 ms = 500 s (8.33 min) recording interval.
2.2 Calculation of band-specific phase angles
We bandpassed each ECoG channel timecourse for the frequency-band of interest: δ = 0–4 Hz; θ = 4–8 Hz; α = 8–13 Hz; β = 13–30 Hz, and γ = 30–80 Hz. The bandpassed filtered data were normalized by removing the mean of each channel and dividing it by its standard deviation. For each ECoG channel n, we compute its analytic signal
We visualized the time-evolution of the phase angles for each ECoG channel in different frequency bands for both experimental conditions. For visualization purposes, only the first 500 time steps were plotted. To illustrate this output, the time-evolution of phase angles
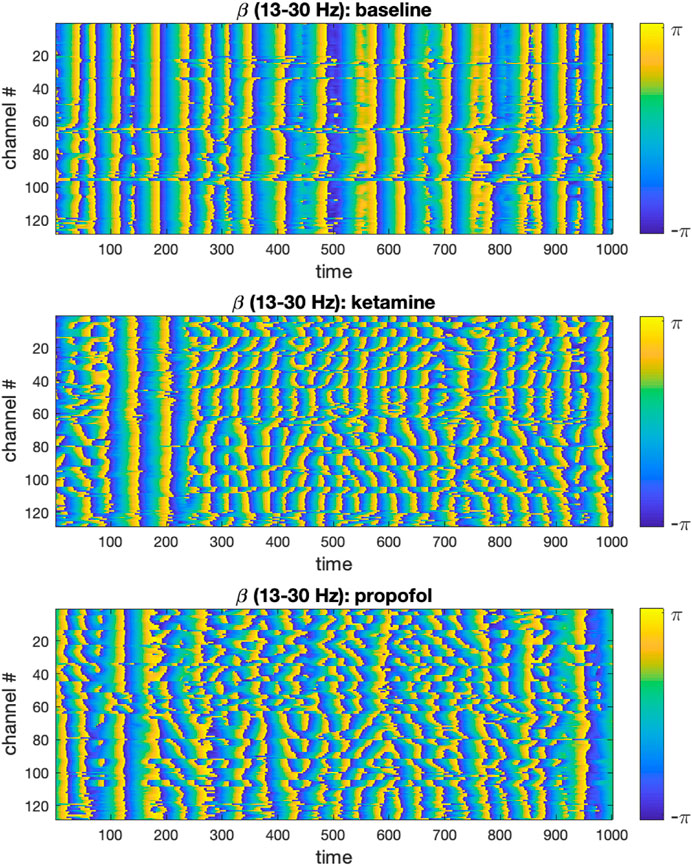
FIGURE 1. Time-evolution of phase angles
2.3 Analysis of global synchronization of ECoG channels
For each of the aforementioned frequency bands, we computed the order parameter (OP) (Kuramoto, 1975) of the whole set of recording electrodes (n = 128 ECoG channels) at each time point (t = 5,000):
This measure enables us to quantify the extent of the global desynchronization induced by ketamine and propofol anesthesia relative to the wakeful rest baseline. The time-evolution of the OP in each frequency band for the anesthesia and baseline conditions were plotted, see Figure 4. Differences in the average value of the OP were compared between the baseline and anesthesia conditions by using paired t-tests (undirected) with Bonferroni correction for multiple comparisons.
2.4 Comparison of local vs. distributed cluster synchrony
We then shifted our attention to studying the brain dynamics from the perspective of clusters of electrodes seen as oscillators. To do so, we applied k-means clustering, using the Matlab kmeans function, to obtain clusters of ECoG channels. K-means is a widely used unsupervised clustering method that clusters elements of a set based on the similarity of their features into k clusters (MacQueen, 1967), In our case, we clustered the electrodes using their spatial coordinates as features, effectively clustering together spatially local channels, for: k = 13, 14, 15. These k-values ensure that the number of electrodes per cluster is small enough to obtain well localized clusters, compared to the size of the brain area mapped by the electrodes, that also contain a non-trivial number of electrodes. To deal with the latter point, we introduced a constraint in the k-mean algorithm so that the smallest possible number of electrodes in a given cluster was n = 4. To account for the randomness involved in k-means initialization and ensure replicability of our findings, we performed 100 iterations of the k-means algorithm in our analyses. A typical exemplar of community assignments obtained for k = 14 is shown in Figure 2A. Where electrodes are assigned a number (and colour) that indicate which cluster they belong to.
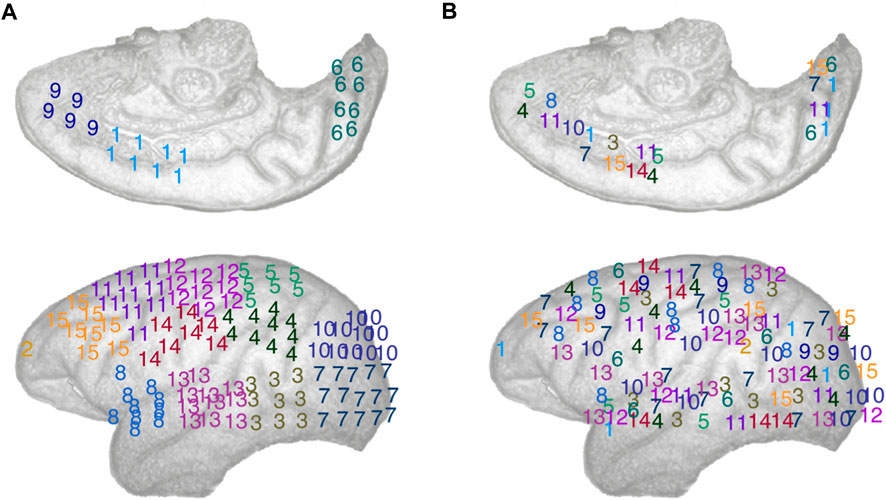
FIGURE 2. Schematic representation of the electrodes array implemented in the left cortical surface of the monkey, inside surface on the top row, outside surface on the bottom row. The electrodes are numbered according to: (A) A typical spatially local clustering for the electrodes using k-means clustering with k = 14. (B) A typical spatially distributed clustering from random electrodes assignment for k = 14. See Methods: Comparison of local vs. distributed cluster synchrony for details.
For each cluster of electrodes C, we calculated the internal synchrony
where |C| denotes the size of the cluster under scrutiny. Local synchrony was calculated for each frequency band on the clusters of ECoG channels defined by the k-means clustering algorithm, i.e., spatially compact clusters, for all k values (k = 13, 14, 15). This process was repeated for each of the 100 k-means iterations.
To measure synchrony across non spatially compact cluster of electrodes, i.e., distributed synchrony, we randomly assigned electrode to k clusters, thus creating spatially distributed clusters of electrodes, see Figure 2B for an illustration. We can see that the numbers (and colours), representing the cluster an electrode belongs to, are effectively spatially distributed. To ensure the robustness of this approach over a large number of randomly assigned electrode clusters, we computed local synchrony on 100 distinct sets of distributed clusters for k = 13, 14, and 15 respectively. For consistency, we introduced the same constraint as with the k-means, ensuring that the minimum size of any distributed cluster was n = 4 ECoG channels. As expected, randomly assigning the electrodes to k clusters statistically significantly increased the average Euclidian distance between electrodes in a given cluster compared to the corresponding k-means algorithm output, thus yielding spatially distributed cluster. See Figure 2 for a typical local and distributed electrodes and Figure 3 for the statistics on the spatial localization of the local and distributed clusters.
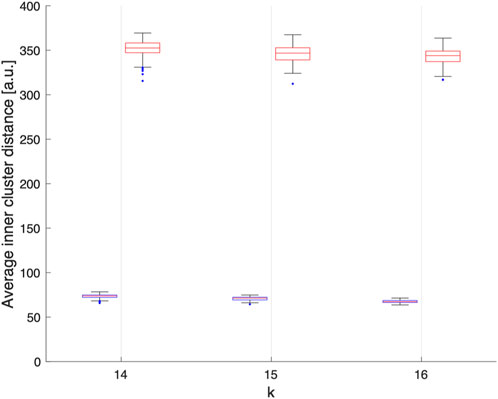
FIGURE 3. Average Euclidean distance, in arbitrary units, between electrodes within each clusters obtained assuming k = 14, 15 or 16 over 100 realisations. We can observe that electrodes grouped by k-means clustering are spatially compact (blue), while the electrodes grouped randomly are spatially delocalized (red).
For each frequency band and cluster number k, we compared the effects of ketamine and propofol anesthesia on the internal synchrony of local vs. distributed electrode clusters using repeated paired t-tests with Bonferroni correction for multiple comparisons. Thus, the statistical significance of pre vs. post-drug effect on cluster synchrony were separately calculated for 100 local cluster assignments, and 100 distributed cluster assignments respectively using a Bonferroni-adjusted significance threshold of α = 5*10-4.
3 Results
3.1 Ketamine and propofol induce global, frequency band-specific desynchronization of brain oscillations
In each frequency band under study, we find that both ketamine and propofol induce a global desynchronization of brain activity across the entire array of ECoG channels, as indicated by significant decreases in the Kuramoto order parameter (OP) of the system in all frequency bands considered, see Figure 4. For ketamine, mean percent decreases in OP during anesthesia relative to the wakeful rest baseline were: −21.3% (0–8 Hz); −40.9% (8–13 Hz); −59.1% (13–30 Hz); −65.6% (30–80 Hz) (all p-vals are sufficiently small to be considered as vanishing numerically). For propofol, mean percent decreases in OP during anesthesia relative to the wakeful rest baseline were: −16.7% (0–8 Hz); −5.9% (8–13 Hz); −41.8% (13–30 Hz); −49.1% (30–80 Hz) (all p-vals are sufficiently small to be considered as vanishing numerically). We note that for both drugs, the largest effects on global synchrony were observed in the β (13–30 Hz) and γ (30–80 Hz) frequency bands. Whilst still statistically significant, the weakest effect was observed for propofol in the α band (8–13 Hz).
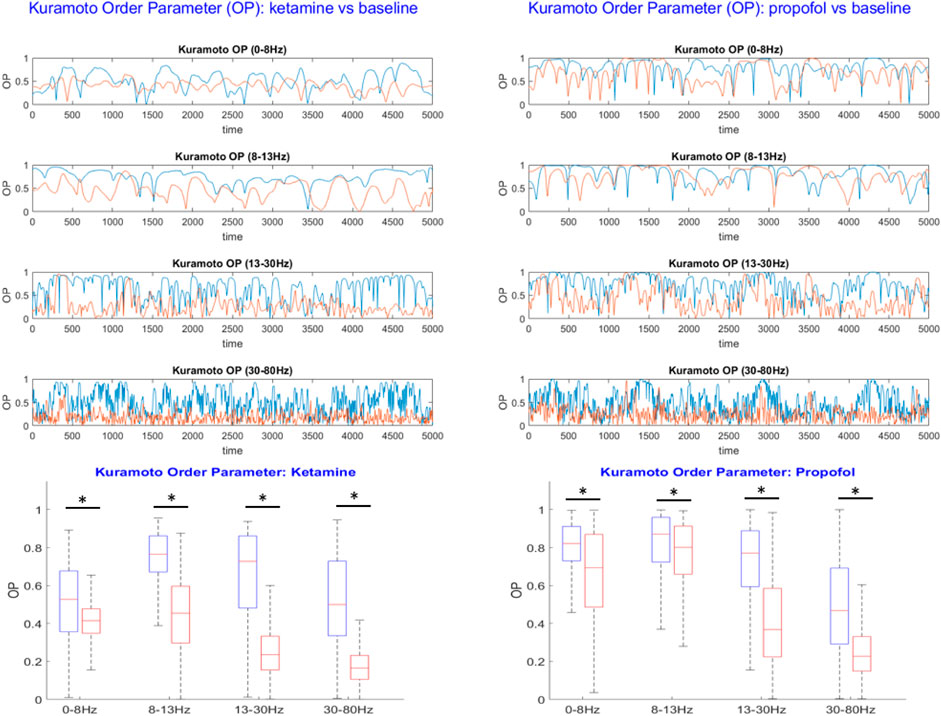
FIGURE 4. Top: The Kuramoto order parameter (OP) is plotted against time for both the anesthesia condition (red) and the wakeful rest baseline (blue) in each frequency band under study. Results from the ketamine anesthesia are shown on the lefthand side, and results for the propofol anesthesia on the right. Both ketamine and propofol induce a strong and statistically significant desynchronization of brain activity across the entire array of ECoG channels, persistent over all frequency bands under study. The largest effects of anesthetics on global synchrony were observed in the β (13–30 Hz) and γ (30–80 Hz) frequency bands, respectively. Bottom: Boxplots showing the mean OP over time ± standard deviation for the baseline (blue) and anesthesia conditions (red). Statistically significant reductions in global synchrony were observed across all frequency bands for both ketamine (left) and propofol (right). The largest effects on global synchrony were observed in the β (13–30 Hz) and γ (30–80 Hz) frequency bands.
3.2 Differential effects of general anesthetics on synchronization spatially localized vs. distributed electrode clusters
Because the OP considers the synchronization behavior of the entire electrode array, it does not allow to infer any distinction between local and distributed effects of the drugs on brain dynamics. We therefore followed up the initial analysis by comparing synchronization behavior of spatially localized and spatially distributed clusters of electrodes; the latter requiring long-range synchronization while the former does not. The internal synchrony, i.e., the local OP, of a given cluster at a given time step is denoted as
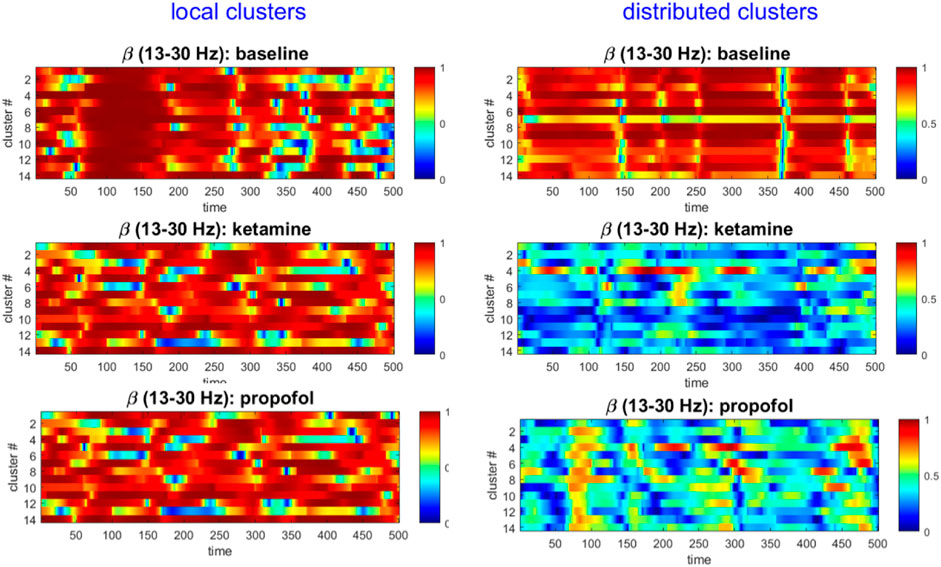
FIGURE 5. The local cluster synchrony
For both ketamine and propofol, synchrony was strongly maintained in spatially localized electrode clusters of ECoG channels, defined by k-means clustering; see Methods section, for k = 14. For ketamine, synchronization in spatially localized clusters was not significantly different in any of the frequency bands at the Bonferroni-adjusted significance threshold (α = 5*10-4). The average p-values were: 0.618, 0.010, 0.019, and 0.383 across all 100 k-means iterations for the δ, α, β, and γ bands, respectively, see Figure 6 left column. Similarly, for propofol anesthesia, synchronization within local clusters was also preserved relative to baseline in all frequency bands and across all cluster assignments. The averaged p-values, obtained across all 100 k-means iterations, were: 0.77, 0.28, 0.74, and 0.76 for δ, α, β, and γ frequency bands, see Figure 7 left column.
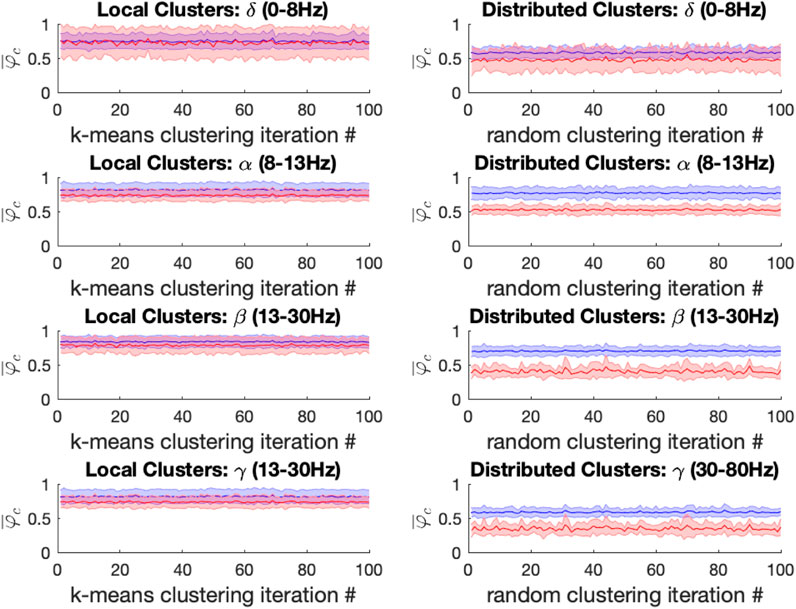
FIGURE 6. Left: For the ketamine (red) vs. baseline (blue) conditions, the mean local cluster synchrony
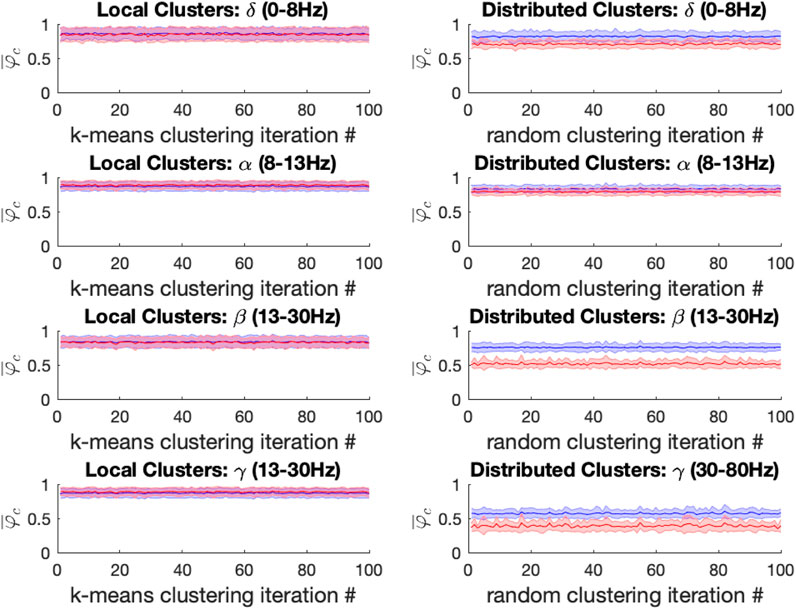
FIGURE 7. Left: For the propofol (red) vs. baseline (blue) conditions, the mean local cluster synchrony
Under ketamine anesthesia, synchronization in spatially distributed clusters was strongly and significantly reduced in every distributed cluster assignment (100/100) for the α, β, and γ bands, but no such reductions were observed in the δ band (0/100), see Figure 6 right column. The average p-vals were: 0.09, 3*10-7, 8*10-7, and 3*10-6 for δ, α, β, and γ frequency bands respectively (Bonferroni-adjusted significance threshold α = 5*10-4). Under propofol anesthesia distributed cluster synchrony was significantly reduced for every cluster assignment (100/100) in the β, and γ bands with average p-values of 1.1*10-7 and 2.7*10-5 respectively, see Figure 7 right column. Statistically significant reductions in distributed cluster synchrony were observed for 60/100 cluster assignments in the δ band (average p-val = 0.0012) but for only 6/100 cluster assignments in the α band (average p-val = 0.017).
Thus, for both ketamine and propofol, statistically significant reductions in distributed cluster synchrony, that are robust over cluster assignments, were observed in the β, and γ frequency bands. By contrast, synchronization in local clusters was not significantly impacted by either drug.
4 Discussion
The integration/segregation balance is intrinsically linked to some of the most complex and fascinating phenomena of modern neuroscience; from the spontaneous emergence of resting-state networks to higher cognition and consciousness (Varela et al., 2001; Sporns, 2013; Lord et al., 2017; Luppi et al., 2021a). Yet, relatively little is known about how changes in subjective experience relate to time-resolved changes in the integration/segregation balance. Here we applied novel analytical approaches to characterize changes in frequency band-specific synchronization patterns between clusters of ECoG channels in a monkey under general anesthesia, compared to a wakeful rest baseline. The effects of both ketamine and propofol anesthesia were studied across separate scanning sessions. We placed specific emphasis on investigating the dynamical integration/segregation balance during anesthesia-induced loss of consciousness by considering individual ECoG channels as oscillators.
Our findings describe a brain functional architecture characterized by a loss of global functional integration during both ketamine and propofol-induced loss of consciousness caused by a selective disruption in long-range synchrony, particularly in the β and γ frequency bands. On the other hand, synchronization in spatially localized clusters remained unaffected by both anesthetic drugs relative to a wakeful rest baseline in all frequencies under study. Furthermore, the results were highly consistent upon building electrode clusters of different sizes (k = 13, 14, 15) (see Supplementary Materials). Thus, despite their markedly different pharmacodynamics (Brown et al., 2011; Stevens, 2022), both ketamine and propofol had significant and directionally consistent effects on the synchronization measures employed in this study at general anesthetic doses, especially in the β and γ frequency bands.
The formation of dynamic links between brain regions via synchronization of oscillations in different frequency bands is one of the most plausible mechanisms for large-scale integration in the brain, and for the resulting binding of information into unified cognitive moments (Joliot et al., 1994; Varela et al., 2001; Tallon-Baudry, 2009; Lord et al., 2013a). For both ketamine and propofol anesthesia, we found that long-range synchronization was most strongly disrupted in the beta (13–30 Hz) and gamma (30–80 Hz) frequency bands. Interestingly, oscillations in the beta frequency range have been shown to facilitate long-range interactions at the cortical level (Gross et al., 2004; Sehatpour et al., 2008; Tallon-Baudry, 2009). Similarly, several lines of evidence have implicated gamma-oscillations in both long-range synchronization and as a neural correlate of conscious perception as well as sensory information-guided, goal-directed behaviors (Kopell et al., 2000; Varela et al., 2001; Melloni et al., 2007; Chand and Dhamala, 2017). Notably, the strength of synchronization in β and γ bands in large-scale cortical networks predicts the perception of audiovisual stimuli, further highlighting the important role of these frequency bands for cross-modal integration (Hipp et al., 2011). Thus, our findings of strongly reduced long-range synchrony in these particular frequencies during anesthesia-induced loss of consciousness provides further evidence for the contribution of the beta and gamma bands toward the integration of information into conscious representations.
Whilst detailed mechanistic explanations linking the pharmacodynamics of propofol and ketamine general anesthesia to the network effects reported in this study is beyond the scope of the current investigation, we nonetheless wish to expand on this idea. Binding of propofol to the widely distributed GABAA receptor hyperpolarizes pyramidal neurons, disrupting the balance between excitatory-to-inhibitory inputs in favor of inhibitory drive (Breshears et al., 2010; Brown et al., 2011). On the other hand, experimental evidence suggests that ketamine binds preferentially to NMDA receptors on GABAergic inhibitory interneurons, thereby disinhibiting pyramidal neurons and leading to a dissociative state in which multiple brain areas communicate through aberrant activity that lacks normal spatial and temporal coordination (Seamans, 2008; Brown et al., 2011). Thus, the most likely shared mechanism between those two drugs is an alteration of the ratio between excitation and inhibition (E/I ratio) in pyramidal neurons. Efficient reciprocal connections between fast-spiking inhibitory interneurons and pyramidal neurons is a crucial requirement for giving rise to coordinated, high-frequency oscillations in distributed circuits (Lord et al., 2013b). Notably, inhibition of fast-spiking interneurons using optogenetic techniques selectively suppresses γ-oscillations (Sohal et al., 2009). Moreover, NMDA receptor deletion results in a loss of neuronal synchronization in high frequency bands in vitro (Belforte et al., 2010). Therefore, we suggest that both ketamine and propofol alter the E/I ratio in pyramidal neurons, which may lead to significant disruptions in long-range synchronization in the β and γ bands, as observed in this study.
Whilst the focus of our analysis was on the β and γ frequency bands due to their well-documented roles in conscious perception, as discussed above, we wish to briefly comment on the results from the lower frequency bands under study (i.e., θ and α). We found decreased synchrony between distributed clusters of electrodes in those bands, albeit not as pronounced as in β and γ bands. This is interesting in light of the fact that oscillatory power in lower frequency bands is generally thought to be increased under propofol anesthesia (Ní Mhuircheartaigh et al., 2013). We note that biophysical modelling studies have found alternations between continuous low-frequency band spiking in thalamus and periods of co-occurring thalamic and cortical silence under propofol anesthesia (Soplata et al., 2023). Loss of cortical synchrony in low-frequency bands, and coordinated thalamocortical silent periods may therefore also contribute to the unconscious state; an observation consistent with our results.
While the available experimental data constrained our analysis to ECoG data from a single hemisphere, the available evidence suggests that both ketamine (Bonhomme et al., 2016; Lv et al., 2016) and propofol (Schrouff et al., 2011; Pujol et al., 2021) lead to the desynchronization of functional connectivity across spatially distant brain regions in both hemispheres; as such we would expect our results to remain consistent, and potentially even strengthen, should the analyses have included data from both hemispheres. Moreover, both GABAA receptors (propofol targets) (Bowery et al., 1987) and NMDA receptors (ketamine targets) (Hansen et al., 2017) are widely distributed in the brain, reducing the likelihood of hemisphere-specific effects.
The present results are also in agreement with prior studies which have used dynamical network analyses and related approaches to study physiologically-reversible changes in consciousness. The emerging consensus from this growing body of fMRI literature is that conscious wakefulness is characterized by global integration, (i.e., long-distance interactions between brain regions displaying scaling behaviour in time and space across a range of imaging modalities) (Linkenkaer-Hansen et al., 2001; Expert et al., 2011), as well as by the dynamic exploration of a diverse and flexible repertoire of functional brain configurations (Watanabe et al., 2014; Cabral et al., 2017; Gu et al., 2018). Conversely, during anesthesia or deep sleep, long-range functional integration is reduced and the dynamic explorations become limited to a smaller subset of specific activity patterns, more tightly constrained by the structural connectome (Demertzi et al., 2019). Our results therefore extend the idea of a dynamical imbalance between the integration and segregation of information during altered consciousness to electrophysiological data at much faster timescales than fMRI investigations allow for.
The results presented in this paper are functional and model agnostic. There is however a large body of theoretical work relating brain structure and function (Park and Friston, 2013). In particular, the modular structure of the brain (Meunier et al., 2010) has been shown to play a central role in the integration and segregation of brain function (Wang et al., 2021). Moreover, theoretical work has shown that transmission delays between neural populations (Pariz et al., 2021) or nodes of a Kuramoto model on modular network (Ziaeemehr et al., 2020) do have an important impact on the synchrony between brain regions and particularly in the transition between synchronous and asynchronous states. One might then conjecture that the pharmacodynamic effects of the two drugs considered have a functional effect on the transmission of information between brain regions, effectively playing the role of a global synchronization control parameter (Lynn and Bassett, 2019).
The measures introduced in this study capture distributed changes in the brain’s spatiotemporal organization induced by ketamine and propofol without considering the precise localization these changes within the brain. Specifically, they demonstrate the potential sensitivity of time-resolved measures of system-wide integration/segregation balance to changes in consciousness level and/or contents. We suggest that related measures could be extended beyond anesthesia and remain sensitive to a range of conditions in which behavior and/or subjective experience is measurably altered, i.e., psychopharmacological effects, neuropsychiatric illness, sleep, disorders of consciousness, etc. For example, we would notably expect psychedelic drugs, e.g., psilocybin and LSD, to have opposite effects as general anesthetic drugs on the measures employed in this study, since some fMRI studies have shown that these compounds may actually increase long-range functional integration (Petri et al., 2014; Tagliazucchi et al., 2016; Lord et al., 2019). An extension of this idea is that biomarkers for neurological and psychiatric disorders need not be necessarily based on changes in specific brain areas or circuits, but could also be reflected as altered global spatiotemporal patterns. For example, dynamical functional connectivity analyses of fMRI data from patients with major depressive disorder revealed abnormally stable patterns of long-range synchronization, consistent with various clinical features of depressive patients, including ruminative, slow, and monotonous thinking (Demirtaş et al., 2016; Figueroa et al., 2019). The present work lays a foundation for further studies in this direction.
In conclusion, we investigated changes in the dynamical balance between integration and segregation of information during anesthesia-induced loss of consciousness in ECoG data from a macaque monkey. The analysis was sensitive to the breakdown of global functional integration and long-range synchronization previously documented in fMRI investigations of physiologically reversible unconscious states. We also report that, in contrast to long-range synchronization, functional synchrony in spatially localized clusters of electrodes remained highly conserved under general anesthesia. We therefore propose that time-resolved measures of synchronization behavior in clusters of oscillators may be sensitive to departures from normal waking consciousness in various conditions and be clinically useful.
Data availability statement
Publicly available datasets were analyzed in this study. This data can be found here: http://www.www.neurotycho.org.
Ethics statement
Ethical approval was not required for the study involving animals in accordance with the local legislation and institutional requirements because the data is public available at http://www.www.neurotycho.org, from the RIKEN institute.
Author contributions
L-DL: Conceptualization, Data curation, Formal Analysis, Methodology, Writing–original draft, Writing–review and editing. TC: Conceptualization, Methodology, Writing–review and editing, Supervision. HF: Conceptualization, Writing–review and editing. FT: Validation, Writing–review and editing. PE: Conceptualization, Methodology, Supervision, Writing–review and editing, Formal Analysis.
Funding
The author(s) declare that no financial support was received for the research, authorship, and/or publication of this article.
Acknowledgments
L-DL, TC, HF, and PE conducted part of the study as Fellows of the Institut Méditeranéen d’Etudes et de Recherches Avancées (IMeRA) avec le soutien de l'Iméra, Aix-Marseille Université, Marseille, du Labex RFIEA + et de l'ANR-Investissements d'avenir.
Conflict of interest
The authors declare that the research was conducted in the absence of any commercial or financial relationships that could be construed as a potential conflict of interest.
Publisher’s note
All claims expressed in this article are solely those of the authors and do not necessarily represent those of their affiliated organizations, or those of the publisher, the editors and the reviewers. Any product that may be evaluated in this article, or claim that may be made by its manufacturer, is not guaranteed or endorsed by the publisher.
Supplementary material
The Supplementary Material for this article can be found online at: https://www.frontiersin.org/articles/10.3389/fnetp.2023.1279646/full#supplementary-material
References
Allen, E. A., Damaraju, E., Plis, S. M., Erhardt, E. B., Eichele, T., and Calhoun, V. D. (2014). Tracking whole-brain connectivity dynamics in the resting state. Cereb. cortex 24 (3), 663–676. doi:10.1093/cercor/bhs352
Atasoy, S., Roseman, L., Kaelen, M., Kringelbach, M. L., Deco, G., and Carhart-Harris, R. L. (2017). Connectome-harmonic decomposition of human brain activity reveals dynamical repertoire re-organization under LSD. Sci. Rep. 7 (1), 17661–17718. doi:10.1038/s41598-017-17546-0
Belforte, J. E., Zsiros, V., Sklar, E. R., Jiang, Z., Yu, G., Li, Y., et al. (2010). Postnatal NMDA receptor ablation in corticolimbic interneurons confers schizophrenia-like phenotypes. Nat. Neurosci. 13 (1), 76–83. doi:10.1038/nn.2447
Betti, V., Della Penna, S., de Pasquale, F., and Corbetta, M. (2021). Spontaneous beta band rhythms in the predictive coding of natural stimuli. Neurosci. 27 (2), 184–201. doi:10.1177/1073858420928988
Bonhomme, V., Vanhaudenhuyse, A., Demertzi, A., Bruno, M.-A., Jaquet, O., Bahri, M. A., et al. (2016). Resting-state network-specific breakdown of functional connectivity during ketamine alteration of consciousness in volunteers. Anesthesiology 125 (5), 873–888. doi:10.1097/ALN.0000000000001275
Bowery, N., Hudson, A., and Price, G. (1987). GABAA and GABAB receptor site distribution in the rat central nervous system. Neuroscience 20 (2), 365–383. doi:10.1016/0306-4522(87)90098-4
Breshears, J. D., Roland, J. L., Sharma, M., Gaona, C. M., Freudenburg, Z. V., Tempelhoff, R., et al. (2010). Stable and dynamic cortical electrophysiology of induction and emergence with propofol anesthesia. Proc. Natl. Acad. Sci. 107 (49), 21170–21175. doi:10.1073/pnas.1011949107
Brown, E. N., Purdon, P. L., and Van Dort, C. J. (2011). General anesthesia and altered states of arousal: a systems neuroscience analysis. Annu. Rev. Neurosci. 34, 601–628. doi:10.1146/annurev-neuro-060909-153200
Cabral, J., Vidaurre, D., Marques, P., Magalhães, R., Silva Moreira, P., Miguel Soares, J., et al. (2017). Cognitive performance in healthy older adults relates to spontaneous switching between states of functional connectivity during rest. Sci. Rep. 7 (1), 5135–5213. doi:10.1038/s41598-017-05425-7
Carhart-Harris, R. L., Leech, R., Hellyer, P. J., Shanahan, M., Feilding, A., Tagliazucchi, E., et al. (2014). The entropic brain: a theory of conscious states informed by neuroimaging research with psychedelic drugs. Front. Hum. Neurosci. 8, 20. doi:10.3389/fnhum.2014.00020
Cavanna, F., Vilas, M. G., Palmucci, M., and Tagliazucchi, E. (2018). Dynamic functional connectivity and brain metastability during altered states of consciousness. Neuroimage 180, 383–395. doi:10.1016/j.neuroimage.2017.09.065
Chand, G. B., and Dhamala, M. (2017). Interactions between the anterior cingulate-insula network and the fronto-parietal network during perceptual decision-making. Neuroimage 152, 381–389. doi:10.1016/j.neuroimage.2017.03.014
Damaraju, E., Tagliazucchi, E., Laufs, H., and Calhoun, V. D. (2020). Connectivity dynamics from wakefulness to sleep. NeuroImage 220, 117047. doi:10.1016/j.neuroimage.2020.117047
Deco, G., Tononi, G., Boly, M., and Kringelbach, M. L. (2015). Rethinking segregation and integration: contributions of whole-brain modelling. Nat. Rev. Neurosci. 16 (7), 430–439. doi:10.1038/nrn3963
Demertzi, A., Tagliazucchi, E., Dehaene, S., Deco, G., Barttfeld, P., Raimondo, F., et al. (2019). Human consciousness is supported by dynamic complex patterns of brain signal coordination. Sci. Adv. 5 (2), eaat7603. doi:10.1126/sciadv.aat7603
Demirtaş, M., Tornador, C., Falcón, C., López-Solà, M., Hernández-Ribas, R., Pujol, J., et al. (2016). Dynamic functional connectivity reveals altered variability in functional connectivity among patients with major depressive disorder. Hum. Brain Mapp. 37 (8), 2918–2930. doi:10.1002/hbm.23215
Expert, P., Lambiotte, R., Chialvo, D. R., Christensen, K., Jensen, H. J., Sharp, D. J., et al. (2011). Self-similar correlation function in brain resting-state functional magnetic resonance imaging. J. R. Soc. Interface 8 (57), 472–479. doi:10.1098/rsif.2010.0416
Figueroa, C. A., Cabral, J., Mocking, R. J., Rapuano, K. M., Van Hartevelt, T. J., Deco, G., et al. (2019). Altered ability to access a clinically relevant control network in patients remitted from major depressive disorder. Hum. Brain Mapp. 40 (9), 2771–2786. doi:10.1002/hbm.24559
Girn, M., Rosas, F. E., Daws, R. E., Gallen, C. L., Gazzaley, A., and Carhart-Harris, R. L. (2023). A complex systems perspective on psychedelic brain action. Trends Cognitive Sci. 27, 433–445. doi:10.1016/j.tics.2023.01.003
Gross, J., Schmitz, F., Schnitzler, I., Kessler, K., Shapiro, K., Hommel, B., et al. (2004). Modulation of long-range neural synchrony reflects temporal limitations of visual attention in humans. Proc. Natl. Acad. Sci. 101 (35), 13050–13055. doi:10.1073/pnas.0404944101
Gu, S., Cieslak, M., Baird, B., Muldoon, S. F., Grafton, S. T., Pasqualetti, F., et al. (2018). The energy landscape of neurophysiological activity implicit in brain network structure. Sci. Rep. 8 (1), 2507–2515. doi:10.1038/s41598-018-20123-8
Hansen, K. B., Yi, F., Perszyk, R. E., Menniti, F. S., and Traynelis, S. F. (2017). NMDA receptors in the central nervous system. NMDA Recept. Methods Protoc., 1–80. doi:10.1007/978-1-4939-7321-7_1
Hipp, J. F., Engel, A. K., and Siegel, M. (2011). Oscillatory synchronization in large-scale cortical networks predicts perception. Neuron 69 (2), 387–396. doi:10.1016/j.neuron.2010.12.027
Joliot, M., Ribary, U., and Llinas, R. (1994). Human oscillatory brain activity near 40 Hz coexists with cognitive temporal binding. Proc. Natl. Acad. Sci. 91 (24), 11748–11751. doi:10.1073/pnas.91.24.11748
Kelso, J. S. (2012). Multistability and metastability: understanding dynamic coordination in the brain. Philosophical Trans. R. Soc. B Biol. Sci. 367 (1591), 906–918. doi:10.1098/rstb.2011.0351
Kopell, N., Ermentrout, G., Whittington, M., and Traub, R. (2000). Gamma rhythms and beta rhythms have different synchronization properties. Proc. Natl. Acad. Sci. 97 (4), 1867–1872. doi:10.1073/pnas.97.4.1867
Y. Kuramoto (Editor) (1975). “Self-entrainment of a population of coupled non-linear oscillators,” International symposium on mathematical problems in theoretical physics (Germany: Springer).
Linkenkaer-Hansen, K., Nikouline, V. V., Palva, J. M., and Ilmoniemi, R. J. (2001). Long-range temporal correlations and scaling behavior in human brain oscillations. J. Neurosci. 21 (4), 1370–1377. doi:10.1523/JNEUROSCI.21-04-01370.2001
Lord, L.-D., Expert, P., Atasoy, S., Roseman, L., Rapuano, K., Lambiotte, R., et al. (2019). Dynamical exploration of the repertoire of brain networks at rest is modulated by psilocybin. Neuroimage 199, 127–142. doi:10.1016/j.neuroimage.2019.05.060
Lord, L. D., Expert, P., Huckins, J. F., and Turkheimer, F. E. (2013a). Cerebral energy metabolism and the brain's functional network architecture: an integrative review. J. Cereb. Blood Flow. Metab. 33 (9), 1347–1354. doi:10.1038/jcbfm.2013.94
Lord, L.-D., Expert, P., Huckins, J. F., and Turkheimer, F. E. (2013b). Cerebral energy metabolism and the brain’s functional network architecture: an integrative review. J. Cereb. Blood Flow Metabolism 33 (9), 1347–1354. doi:10.1038/jcbfm.2013.94
Lord, L.-D., Stevner, A. B., Deco, G., and Kringelbach, M. L. (2017). Understanding principles of integration and segregation using whole-brain computational connectomics: implications for neuropsychiatric disorders. Philosophical Trans. R. Soc. A Math. Phys. Eng. Sci. 375 (2096), 20160283. doi:10.1098/rsta.2016.0283
Luppi, A. I., Carhart-Harris, R. L., Roseman, L., Pappas, I., Menon, D. K., and Stamatakis, E. A. (2021b). LSD alters dynamic integration and segregation in the human brain. NeuroImage 227, 117653. doi:10.1016/j.neuroimage.2020.117653
Luppi, A. I., Golkowski, D., Ranft, A., Ilg, R., Jordan, D., Menon, D. K., et al. (2021a). Brain network integration dynamics are associated with loss and recovery of consciousness induced by sevoflurane. Hum. Brain Mapp. 42 (9), 2802–2822. doi:10.1002/hbm.25405
Luppi, A. I., Vohryzek, J., Kringelbach, M. L., Mediano, P. A., Craig, M. M., Adapa, R., et al. (2020). Connectome harmonic decomposition of human brain dynamics reveals a landscape of consciousness. BioRxiv. doi:10.1038/s42003-023-04474-1
Lv, Q., Yang, L., Li, G., Wang, Z., Shen, Z., Yu, W., et al. (2016). Large-scale persistent network reconfiguration induced by ketamine in anesthetized monkeys: relevance to mood disorders. Biol. psychiatry 79 (9), 765–775. doi:10.1016/j.biopsych.2015.02.028
Lynn, C. W., and Bassett, D. S. (2019). The physics of brain network structure, function and control. Nat. Rev. Phys. 1 (5), 318–332. doi:10.1038/s42254-019-0040-8
J. MacQueen (Editor) (1967). “Some methods for classification and analysis of multivariate observations,” Proceedings of the fifth Berkeley symposium on mathematical statistics and probability (Oakland, CA, USA: Springe).
Melloni, L., Molina, C., Pena, M., Torres, D., Singer, W., and Rodriguez, E. (2007). Synchronization of neural activity across cortical areas correlates with conscious perception. J. Neurosci. 27 (11), 2858–2865. doi:10.1523/JNEUROSCI.4623-06.2007
Meunier, D., Lambiotte, R., and Bullmore, E. T. (2010). Modular and hierarchically modular organization of brain networks. Front. Neurosci. 4, 200. doi:10.3389/fnins.2010.00200
Ní Mhuircheartaigh, R., Warnaby, C., Rogers, R., Jbabdi, S., and Tracey, I. (2013). Slow-wave activity saturation and thalamocortical isolation during propofol anesthesia in humans. Sci. Transl. Med. 5, 208ra148. doi:10.1126/scitranslmed.3006007
Pariz, A., Fischer, I., Valizadeh, A., and Mirasso, C. (2021). Transmission delays and frequency detuning can regulate information flow between brain regions. PLoS Comput. Biol. 17 (4), e1008129. doi:10.1371/journal.pcbi.1008129
Park, H.-J., and Friston, K. (2013). Structural and functional brain networks: from connections to cognition. Science 342 (6158), 1238411. doi:10.1126/science.1238411
Petri, G., Expert, P., Turkheimer, F., Carhart-Harris, R., Nutt, D., Hellyer, P. J., et al. (2014). Homological scaffolds of brain functional networks. J. R. Soc. Interface 11 (101), 20140873. doi:10.1098/rsif.2014.0873
Pujol, J., Blanco-Hinojo, L., Gallart, L., Moltó, L., Martínez-Vilavella, G., Vilà, E., et al. (2021). Largest scale dissociation of brain activity at propofol-induced loss of consciousness. Sleep 44 (1), zsaa152. doi:10.1093/sleep/zsaa152
Schrouff, J., Perlbarg, V., Boly, M., Marrelec, G., Boveroux, P., Vanhaudenhuyse, A., et al. (2011). Brain functional integration decreases during propofol-induced loss of consciousness. Neuroimage 57 (1), 198–205. doi:10.1016/j.neuroimage.2011.04.020
Seamans, J. (2008). Losing inhibition with ketamine. Nat. Chem. Biol. 4 (2), 91–93. doi:10.1038/nchembio0208-91
Sehatpour, P., Molholm, S., Schwartz, T. H., Mahoney, J. R., Mehta, A. D., Javitt, D. C., et al. (2008). A human intracranial study of long-range oscillatory coherence across a frontal–occipital–hippocampal brain network during visual object processing. Proc. Natl. Acad. Sci. 105 (11), 4399–4404. doi:10.1073/pnas.0708418105
Sohal, V. S., Zhang, F., Yizhar, O., and Deisseroth, K. (2009). Parvalbumin neurons and gamma rhythms enhance cortical circuit performance. Nature 459 (7247), 698–702. doi:10.1038/nature07991
Soplata, A. E., Adam, E., Brown, E. N., Purdon, P. L., McCarthy, M. M., and Kopell, N. (2023). Rapid thalamocortical network switching mediated by cortical synchronization underlies propofol-induced EEG signatures: a biophysical model. J. Neurophysiology 130, 86–103. doi:10.1152/jn.00068.2022
Sporns, O. (2013). Network attributes for segregation and integration in the human brain. Curr. Opin. Neurobiol. 23 (2), 162–171. doi:10.1016/j.conb.2012.11.015
Tagliazucchi, E., Roseman, L., Kaelen, M., Orban, C., Muthukumaraswamy, S. D., Murphy, K., et al. (2016). Increased global functional connectivity correlates with LSD-induced ego dissolution. Curr. Biol. 26 (8), 1043–1050. doi:10.1016/j.cub.2016.02.010
Tagliazucchi, E., von Wegner, F., Morzelewski, A., Brodbeck, V., Jahnke, K., and Laufs, H. (2013). Breakdown of long-range temporal dependence in default mode and attention networks during deep sleep. Proc. Natl. Acad. Sci. 110 (38), 15419–15424. doi:10.1073/pnas.1312848110
Tallon-Baudry, C. (2009). The roles of gamma-band oscillatory synchrony in human visual cognition. Front. Biosci. 14 (321-332), 321–332. doi:10.2741/3246
Tononi, G., and Edelman, G. M. (1998). Consciousness and complexity. science 282 (5395), 1846–1851. doi:10.1126/science.282.5395.1846
Turkheimer, F. E., Hellyer, P., Kehagia, A. A., Expert, P., Lord, L. D., Vohryzek, J., et al. (2019). Conflicting emergences. Weak vs. strong emergence for the modelling of brain function. Neurosci. Biobehav Rev. 99, 3–10. doi:10.1016/j.neubiorev.2019.01.023
Turkheimer, F. E., Rosas, F. E., Dipasquale, O., Martins, D., Fagerholm, E. D., Expert, P., et al. (2021). A complex systems perspective on neuroimaging studies of behavior and its disorders. Neuroscientist, 1073858421994784. doi:10.1177/1073858421994784
Varela, F., Lachaux, J.-P., Rodriguez, E., and Martinerie, J. (2001). The brainweb: phase synchronization and large-scale integration. Nat. Rev. Neurosci. 2 (4), 229–239. doi:10.1038/35067550
Wang, R., Liu, M., Cheng, X., Wu, Y., Hildebrandt, A., and Zhou, C. (2021). Segregation, integration, and balance of large-scale resting brain networks configure different cognitive abilities. Proc. Natl. Acad. Sci. 118 (23), e2022288118. doi:10.1073/pnas.2022288118
Watanabe, T., Hirose, S., Wada, H., Imai, Y., Machida, T., Shirouzu, I., et al. (2014). Energy landscapes of resting-state brain networks. Front. neuroinformatics 8, 12. doi:10.3389/fninf.2014.00012
Yanagawa, T., Chao, Z. C., Hasegawa, N., and Fujii, N. (2013). Large-scale information flow in conscious and unconscious states: an ECoG study in monkeys. PloS one 8 (11), e80845. doi:10.1371/journal.pone.0080845
Keywords: neural synchronisation, ECoG, altered state of consciousness, integration/segregation, anesthesia
Citation: Lord L-D, Carletti T, Fernandes H, Turkheimer FE and Expert P (2023) Altered dynamical integration/segregation balance during anesthesia-induced loss of consciousness. Front. Netw. Physiol. 3:1279646. doi: 10.3389/fnetp.2023.1279646
Received: 18 August 2023; Accepted: 20 November 2023;
Published: 05 December 2023.
Edited by:
Alexander N. Pisarchik, Universidad Politécnica de Madrid, SpainReviewed by:
Alireza Valizadeh, Institute for Advanced Studies in Basic Sciences (IASBS), IranGanesh Chand, Washington University in St. Louis, United States
Copyright © 2023 Lord, Carletti, Fernandes, Turkheimer and Expert. This is an open-access article distributed under the terms of the Creative Commons Attribution License (CC BY). The use, distribution or reproduction in other forums is permitted, provided the original author(s) and the copyright owner(s) are credited and that the original publication in this journal is cited, in accordance with accepted academic practice. No use, distribution or reproduction is permitted which does not comply with these terms.
*Correspondence: Paul Expert, cC5leHBlcnRAdWNsLmFjLnVr