- 1Clinical and Data Intelligence Systems-Advanced Analytics, Fresenius Medical Care Deutschland GmbH, Vaiano Cremasco, Italy
- 2Center for Preclinical Research, Fondazione IRCCS Ca’ Granda Ospedale Maggiore Policlinico, Milan, Italy
- 3Department of Medical Biometry, Informatics, and Epidemiology (IMBIE), Faculty of Medicine, University of Bonn, Bonn, Germany
- 4Department of Nephrology and Hypertension, Universitätsklinikum Erlangen, Friedrich-Alexander Universität Erlangen-Nürnber, Erlangen, Germany
- 5Medical Centre for Information and Communication Technology (MIK), University Hospital Erlangen, Erlangen, Germany
- 6Fresenius Medical Care, Deutschland GmbH, Bad Homburg, Germany
- 7Institute of Genetic Epidemiology, Faculty of Medicine and Medical Center - University of Freiburg, Freiburg, Germany
- 8Department of Medicine IV – Nephrology and Primary Care, Faculty of Medicine and Medical Center - University of Freiburg, Freiburg, Germany
- 9Department of Nephrology and Medical Intensive Care, Charité Universitätsmedizin Berlin, Berlin, Germany
Background and Objectives: Cardiovascular (CV) disease is the main cause of morbidity and mortality in patients suffering from chronic kidney disease (CKD). Although it is widely recognized that CV risk assessment represents an essential prerequisite for clinical management, existing prognostic models appear not to be entirely adequate for CKD patients. We derived a literature-based, naïve-bayes model predicting the yearly risk of CV hospitalizations among patients suffering from CKD, referred as the CArdiovascular, LIterature-Based, Risk Algorithm (CALIBRA).
Methods: CALIBRA incorporates 31 variables including traditional and CKD-specific risk factors. It was validated in two independent CKD populations: the FMC NephroCare cohort (European Clinical Database, EuCliD®) and the German Chronic Kidney Disease (GCKD) study prospective cohort. CALIBRA performance was evaluated by c-statistics and calibration charts. In addition, CALIBRA discrimination was compared with that of three validated tools currently used for CV prediction in CKD, namely the Framingham Heart Study (FHS) risk score, the atherosclerotic cardiovascular disease risk score (ASCVD), and the Individual Data Analysis of Antihypertensive Intervention Trials (INDANA) calculator. Superiority was defined as a ΔAUC>0.05.
Results: CALIBRA showed good discrimination in both the EuCliD® medical registry (AUC 0.79, 95%CI 0.76-0.81) and the GCKD cohort (AUC 0.73, 95%CI 0.70-0.76). CALIBRA demonstrated improved accuracy compared to the benchmark models in EuCliD® (FHS: ΔAUC=-0.22, p<0.001; ASCVD: ΔAUC=-0.17, p<0.001; INDANA: ΔAUC=-0.14, p<0.001) and GCKD (FHS: ΔAUC=-0.16, p<0.001; ASCVD: ΔAUC=-0.12, p<0.001; INDANA: ΔAUC=-0.04, p<0.001) populations. Accuracy of the CALIBRA score was stable also for patients showing missing variables.
Conclusion: CALIBRA provides accurate and robust stratification of CKD patients according to CV risk and allows score calculations with improved accuracy compared to established CV risk scores also in real-world clinical cohorts with considerable missingness rates. Our results support the generalizability of CALIBRA across different CKD populations and clinical settings.
1 Introduction
Patients with non-dialysis dependent chronic kidney disease (NDD-CKD) show higher cardiovascular morbidity and mortality relative to the general population (1, 2). The spectrum of cardiovascular disease (CVD) in CKD includes heart failure (HF), atrial fibrillation (AF), coronary artery disease (CAD), myocardial infarction (MI), cerebrovascular disease (CRVD), and peripheral arterial (occlusive) disease (PA(O)D) (3). About half of all NDD-CKD patients die subsequent to a cardiovascular event without progressing to kidney failure requiring renal replacement therapy (2, 4, 5). Given the significant burden of CVD in patients with NDD-CKD, cardiovascular risk stratification would enhance the effectiveness and efficiency of prevention programs (6).
Cardiovascular risk prediction in NDD-CKD is currently performed using predictive tools developed for the general population (3, 6, 7). However, the pathology and clinical manifestations of CVD differ in the presence of impaired kidney function (2, 3) and traditional risk factors sub-optimally predict CVD in CKD patients (3, 8, 9). The Kidney Disease: Improving Global Outcomes (KDIGO) clinical practice guidelines recommend using both estimated glomerular filtration rate (eGFR) and albuminuria for CVD risk assessment (7). Accordingly, A recent meta-analysis of the Chronic Kidney Disease Prognosis Consortium (CKD-PC) showed that inclusion of combined urinary albumin-to-creatinine ratio (ACR) and eGFR improves cardiovascular risk prediction in NDD-CKD populations (10). Of note, measures reflecting pathophysiological processes in the cardiovascular system, such as coronary artery calcium score or high-sensitivity cardiac troponin T (hs-cTnT), improved cardiovascular risk estimation in NDD-CKD (3, 11).
Given the lack of well-performing CVD risk stratification tools specifically addressing NDD-CKD patients, we derived a knowledge-based, Naïve-Bayes (NB) model predicting the yearly risk of cardiovascular hospitalizations in this patient group, namely the CArdiovascular, LIterature-Based, Risk Algorithm (CALIBRA). In the present study, we sought to evaluate the accuracy of CALIBRA in two independent cohorts of CKD patients, namely the FMC NephroCare cohort (European Clinical Database, EuCliD®) (12) and the German Chronic Kidney Disease (GCKD) study cohort (13). Moreover, we compared the performance of CALIBRA with that of three validated cardiovascular scores which are currently used for cardiovascular prediction in CKD patients.
2 Methods
2.1 Description of CALIBRA
CALIBRA is a literature-based naïve-bayes classifier (NBC) predicting the risk of at least one cardiovascular hospitalization within 1 year among NDD-CKD. An NBC is a simple probabilistic model based on application of the Bayes’ theorem. These tools are represented as directed acyclic graphs and their weights can be learned by data-driven algorithms or by codifying existing medical knowledge obtained from domain experts (14). NBCs can be exploited to generate metrics enabling broad prognostic reasoning. First, they generate a risk score representing the expected incidence of disease given a vector of known patients’ characteristics. Second, NBCs can be used to generate value of information (VOI) statistics and impact metrics. VOI statistics represent the reduction in uncertainty (i.e. entropy) in the outcome variable by measuring/observing the status of previously unknown variables (15). Therefore, it can be used to prioritize additional diagnostic testing or biomarker assays for patients with incomplete medical records. Conversely, impact metrics evaluates the magnitude of association of different subsets of evidence on the outcome variable (16). As a consequence, it can be used to estimate the potential impact of an intervention addressing some or all modifiable risk factors.
2.2 Derivation of CALIBRA
We derived a NB prognostic model entirely based on knowledge abstracted from medical literature.
CALIBRA graphical structure is shown in Figure 1. Model parameters feeding the conditional probability tables representing the relationship between each independent risk factor and outcomes were computed by abstracting and pooling effect size estimates from observational studies (Supplementary Material; Appendix 1). We used fixed effect meta-analysis using normalized study sample size as the weighting parameter. We included studies reporting data on fatal and non-fatal cardiovascular outcomes, whereas we excluded studies with follow up shorter than 1 year and those not reporting sufficient information for knowledge extraction. We also excluded researches focused on pediatric population, patients on renal replacement therapy or other disease populations. The process adopted to abstract medical knowledge from the literature and compute model parameters is reported in Supplementary Material; Appendix 2. We included in the final model 31 variables (Table 1). Details of model derivation have been described previously (17).
2.3 Design and Setting of CALIBRA Validation Studies
CALIBRA was validated in two independent cohorts, one derived from the FMC NephroCare network and the other derived from the GCKD study. This design allowed testing the accuracy of the model in different clinical settings henceforth enhancing the robustness of our results.
2.3.1 FMC NephroCare Cohort
All patients treated in the FMC NephroCare network from 2017 to 2018 were screened for eligibility. A total of 22,535 subjects who underwent at least 2 visits one year apart and one serum creatinine assessment (s-Cr) during their pre-dialysis care were included. We abstracted all data from the EuCliD® database (Fresenius Medical Care, Deutschland GmbH), as previously described (18, 19).
2.3.2 GCKD Cohort
A second analysis evaluated CALIBRA performance using data of the GCKD cohort (13). Briefly, the GCKD study is an ongoing prospective observational cohort study investigating determinants of kidney disease progression and cardiovascular complications. The GCKD study enrolled 5217 patients with CKD of various etiologies between 2010 and 2012. Patient recruitment and follow-up are organized through a network of academic nephrology centers collaborating with practicing nephrologists throughout Germany. At the time of recruitment, patients were under nephrological care and either had an eGFR of 30–60 mL/min/1.73m2 or overt proteinuria in the presence of an eGFR >60 mL/min/1.73m2. In our validation analysis, only patients with a s-Cr value available at baseline were considered (N=5,159).
In both cohorts, the study endpoint, i.e. cardiovascular hospitalization, was assessed over a 12-month follow up period.
2.4 Measures
2.4.1 Endpoint Definition
The primary endpoint was the occurrence of any hospitalization due to CVD events within 1 year after the index date (i.e., the date of the first visit recorded for each patient). The short follow up time was chosen to reflect the relatively high incidence rate of cardiovascular event in NDD-CKD patients. Given that NDD-CKD patients undergo repeated visits during the year, longer prediction horizons may reduce the ability to address changes in risk profiles that merit a timely change in treatment or referral to intensified prevention program. CVD events were defined as the composite of HF, CAD, MI, CRVD, AF, PAD, and sudden cardiac death (SCD). In both validation cohorts, endpoint for CALIBRA validation study was extracted from patients’ chart reports. In particular, concerning patients included in the EuCliD® dataset, endpoints were ascertained by occurrence of suggestive ICD10 codes (Supplementary Material; Appendix 3 (20),) that occurred during a hospitalization. In the GCKD study all endpoints are extracted from patient discharge reports; the process of endpoint adjudication and abstraction in the GCKD study has been described previously (18, 19).
2.4.2 Definition of CKD Stages
Patients were classified according to established GFR categories (21, 22) using eGFR estimates derived by the CKD-EPI equation (23).
2.4.3 Other Input Variables
We assessed demographic, anthropometric, life-style variables at index visit; blood biomarkers were collected and averaged over 12 months before the index date; finally, we abstracted comorbidities and CKD etiologies recorded at the index date.
2.5 Statistical Analysis and Model Performance Evaluation
We calculated the incidence density and 95% confidence interval (CI) of CVD events in the study population based on the Poisson distribution.
Since data collected in routine clinical practice are largely incomplete, a suitable prognostic algorithm should be able to handle heavy missingness generation process without loss of accuracy. Since CALIBRA is based on NB networks, no data manipulation was required to explicitly handle missing variables. Therefore, one important objective of the study consisted in assessing the computability and accuracy of equation-based risk scores against CALIBRA.
Model performance was evaluated by concordance statistic and calibration charts. Calibration was visually inspected by plotting the incidence of observed outcomes by quintiles of the risk score. We used the calibration plot to evaluate model discrimination properties across the distribution of the risk score. Overall discrimination was quantified by calculating the area under the receiver operating characteristic curve (ROC AUC) in both validation cohorts.
Furthermore, we investigated CALIBRA non-inferiority relative to scores whose performance has been previously assessed in NDD-CKD patients, including the FHS risk score (24), the INDANA calculator (25), and the ASCVD score of the American College of Cardiology (ACC)/American Heart Association (AHA) guidelines (26). Even though such scores evaluate the risk of cardiovascular events over a longer time horizon, it is important to assess their suitability for clinical practice for a more useful endpoint among NDD-CKD patients. Other risk scores, such as the SCORE2 algorithm have never been validated among CKD-NDD patients and demonstrated a wide range of performance across different European Countries SCORE2 (27). A list of variables included in these tools is provided in Appendix 4. Non-inferiority was assessed by checking whether a one-sided confidence interval of the AUC remained entirely above the non-inferiority threshold (σ=0.05). If non-inferiority was achieved, we evaluated whether CALIBRA was superior, defined as ΔAUC>0·05 compared to an existing risk score. Superiority was tested with DeLong non-parametric approach (28). Given the sequential nature of testing in a fixed order method approach, type I error is not inflated by multiple testing. Statistical significance was claimed at α<0.05.
3 Results
3.1 Sample Characteristics
Baseline demographic and clinical data of the FMC-NephroCare and the GCKD cohorts are reported in Table 2. In the EuCliD® dataset, patients were older and had lower eGFR levels. Number of patients lost to follow-up was 7,655 (34%) in the FMC-NephroCare cohort and 337 (6.5%) participants in the GCKD study. CVD hospital admissions were 309 (0.021 events/person*year), and 197 (0.041 events/person*year) in the EuCliD® database and the GCKD cohort, respectively.
3.2 CALIBRA Discrimination in the FMC-NephroCare Cohort
Our model showed good discriminative ability in the FMC-NephroCare cohort. Concordance statistic was 0.79 (95% CI 0.76-0.81; Stage G3-5). Calibration of predicted versus observed risk is represented in Figure 2A. Risk ratios between adjacent quintiles showed that model discrimination was highest at the extreme of predicted risk distribution.
Table 3 shows performances of CALIBRA and existing CVD risk models. Based on the pre-defined superiority criteria, CALIBRA outperformed FHS (ΔAUC = -0.22 p<0.001), ASCVD (ΔAUC = -0.17, p<0.001) and INDANA (ΔAUC = -0.14, p<0.001).
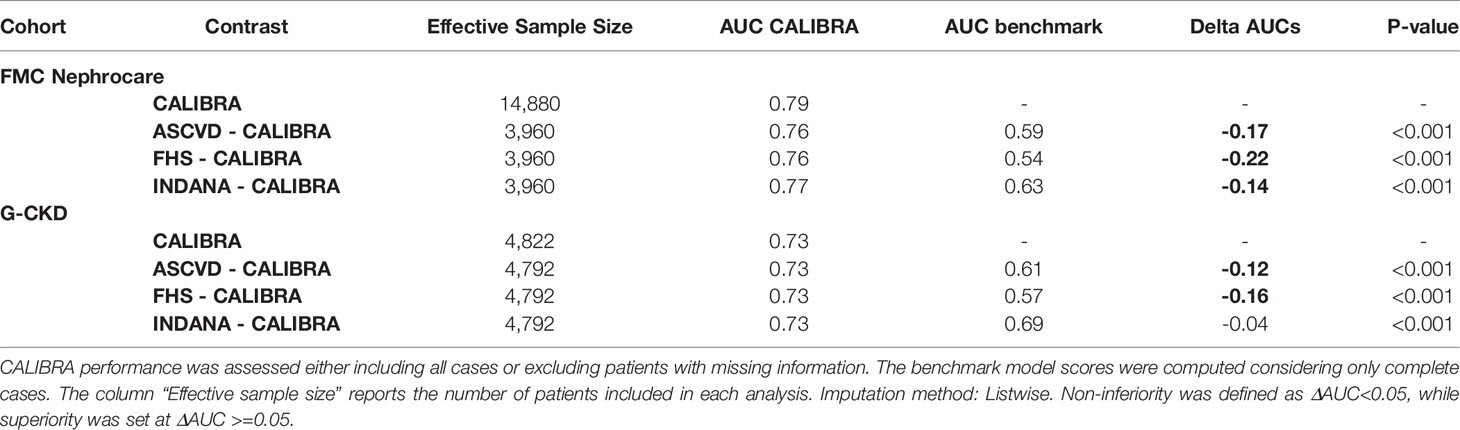
Table 3 Discrimination ability of CALIBRA. Analysis was performed both in the FMC NephroCare cohort and in the GCKD cohort; endpoint horizon: 1 year.
3.3 CALIBRA Discrimination in the GCKD Cohort
Discrimination in the GCKD study was moderate (AUC=0.73 95% CI 0.70-0.76; GCKD, Stage G1-5). Calibration of predicted versus observed risk is represented in Figure 2B. The model better discriminated low and high-risk patients compared to those classified in the central quintiles of risk score distribution.
Based on pre-specified superiority criteria, CALIBRA outperformed FHS (ΔAUC= -0.16, p<0.001), and ASCVD (ΔAUC= -0.12, p<0.001, Table 3). Even though statistically significant, the difference in performance between CALIBRA and INDANA did not meet the pre-defined superiority criteria (ΔAUC = -0.04, p<0.001, Table 3).
3.4 Case Study
To better illustrate the full scope of CALIBRA in facilitating broad prognostic reasoning, we report model metrics generated from a routine clinical examination for a specific patient who presented to an FMC-NephroCare NDD-CKD outpatient clinic (Supplementary Material; Appendix 5). The patient was 45 years old, male, obese (BMI=41), suffering from stage G4 CKD (eGFR=25), most likely caused by diabetes; he was a past-smoker. Known comorbidities were hypertension and chronic obstructive pulmonary disease (COPD). Blood biomarkers: serum albumin=4.00 g/dl; calcium=8.9 mg/dl; hemoglobin=12.53 g/dl; phosphate=4.19 mg/dl, PTH=289 ng/l, proteinuria=2.49 g/24h.
Based on these data CALIBRA predicted a 14% risk of CVD hospitalization within 1 year.
According to Impact Analysis, proteinuria (normalized likelihood, NL=1.52), diabetes (NL=1.43), COPD (NL=1.35), male sex (NL=1.24), eGFR (NL=1.06), hypertension (NL=1.05), PTH (NL=1.02) increased CVD risk. Conversely, young age was determined as a protective factor (NL=0.35).
VOI metrics were indexed to the highest in rank. For the present case, the greatest reduction in information entropy (i.e. reduction in uncertainty around risk estimated) would be achieved by measuring, in decreasing order of relative importance, C-Reactive Protein (Indexed VOI=0.46), resting hsTNT (Indexed VOI=0.39), and s-Uric Acid (Indexed VOI=0.22).
4 Discussion
In this large validation study, CALIBRA consistently showed better discrimination in predicting the risk of NDD-CKD patients to experience a CV hospitalization within 1 year compared to the FHS (24), the ASCVD (26), and INDANA (25) CV risk algorithms.
CALIBRA overcomes inherent limitations of existing CVD risk scores in forecasting CV events among NDD-CKD patients by making use of both traditional and CKD-specific risk factors (i.e. kidney function measures eGFR (10), albuminuria (9, 29) or proteinuria (10, 11), uric acid (9), C-reactive protein (30), among others). To address the lack of comprehensive and sufficiently large dataset for model derivation, we used a novel literature-based Bayesian Network approach by incorporating pooled effect size estimates obtained from published reports of large historical and longitudinal cohort studies; such an approach proved to enhance model discrimination compared to traditional methods. Of interest, one important corollary of literature-based NBCs is their unlimited scalability, in that additional risk factors can be easily added to the model when sufficient evidence would be collected from future studies. As an instance, CALIBRA already includes biomarkers such as hs-cTnT (3, 10) and IL-6 (31) which have been previously shown to provide independent cardiovascular prognostic signals. Unfortunately, these biomarkers were not available in the two study populations, so that their potential incremental discrimination in CALIBRA could not be assessed.
Of importance, CALIBRA superiority against existing cardiovascular risk scores was demonstrated in two independent cohorts, namely the FMC-NephroCare and the GCKD cohorts. These cohorts show substantial differences in their design, geographical area of recruitment, inclusion/exclusion criteria, measurements, and data collection strategies. Indeed, EuCliD® is a medical registry archiving FMC-NephroCare real-world medical data of stage G 3-5 CKD and dialysis patients from multiple countries in Europe, South America, Africa, Middle-East and the Asia-Pacific region with exclusion of Germany. Conversely, the GCKD study is a large prospective observational research that enrolled patients with stages G3, A1-3 or stages G1-2, A3 in Germany (13). In addition, in the EuCliD® registry, medical data were collected by clinicians and nurses as part of their routine activity; biochemical tests, despite automatically uploaded from lab results files, were purposefully prescribed by physicians. On the other hand, in the GCKD cohort, data were collected per protocol at pre-specified assessment timepoints. Consequently, the EuCliD® registry was associated with a higher percentage of missing variables, while the GCKD dataset was almost complete. Overall, our results support the generalizability of CALIBRA across different CKD populations and clinical settings.
Consistent with previous reports (8), our findings showed that the accuracy of FHS in predicting CV events among NDD-CKD patients is poor. Superiority of CALIBRA against the FHS score is not surprising. Indeed, FHS was derived from a general population-based cohort with a low NDD-CKD prevalence; furthermore, it includes traditional risk factors only (24). CALIBRA performance was likewise superior to that of the ASCVD risk prediction tool which considers additional variables including the regular use of medications for diabetes and high blood pressure (26). A third comparison was made relative to INDANA that, in contrast to the other algorithms, includes a measure of kidney function (25). Our findings indicate superior discrimination of CALIBRA compared to that of INDANA. The difference in performance was particularly evident in the FMC-NephroCare cohort. The reduced performance of the INDANA calculator likely depends on its derivation from a randomized controlled trial. In fact, despite this design vouches for the accuracy of data collection in the INDANA study, it may limit the generalizability of the risk score in real life setting where data generation process is driven by clinical decision making and, as a consequence, may lack consistency. On the contrary, CALIBRA was robust to missing variables due to the inherent properties of NB networks. It is worth mentioning that two novel risk models were recently developed to predict CVD risk among CKD patients. In the first study, a novel algorithm incorporatingkidney function and proteinuria to existing risk equations has been derived and validated in a very large collaborative cohort by the CKD Prognosis Consortium (CKD-PC) study group (32). The augmented risk equations showed improved discrimination compared to original risk scores (GFR Patch: AUC=0.75; 95%CI 0.70-0.77; CKD Patch, including both eGFR and ACR: AUC=0.70; 95%CI 0.67-0.73). The second study provides two models for 10-year risk prediction of atherosclerotic CVD, namely a model composed of readily clinically available variables and a biomarker-enriched model (33). Both tools were derived using data from the Chronic Renal Insufficiency Cohort (CRIC) study and showed an AUC of 0.76 (95%CI 0.68-0.85) and 0.77 (95%CI 0.67-0.85), respectively. Of interest, the discrimination ability observed in these studieswas comparable to CALIBRA performance.
Irrespective of discrimination superiority, NB networks have several advantages over equation-based risk scores. First, BNCs account for uncertainty related to variable missingness and provide realistic risk estimation by incorporating distributional assumptions for unmeasured input variables (14). While this property may not provide any apparent advantage in longitudinal cohorts such as the GCKD study given its almost complete datasets, it is particularly useful in real-life applications where traditional, equation-based approaches may not be calculable for many patients with missing information on key risk factors. As expected, the FHS, the INDANA, and the ASCVD scores could not be computed for a large proportion of patients in the FMC-NephroCare cohort due to many parameters not being documented in clinical practice. On the contrary, CALIBRA could be used in all patients and its accuracy remained consistently superior to other risk score.
Finally, NB networks provide additional metrics beyond risk estimations which further support prognostic reasoning in clinical practice. In order to demonstrate the enhanced clinical utility of metrics generated by NBCs, we showed CALIBRA predictions in an exemplary case. Beside accurate CVD risk prediction, NBCs facilitate the assessment of prediction robustness to missing information on risk factors by inspecting VOI. Therefore, VOI metrics may help healthcare professionals prioritize further diagnostic testing by highlighting those that would provide the highest amount of additional prognostic information for the case at hand. Moreover, NBCs rank the impact of observed evidence on each patient’s risk based on NL metrics (16), thus suggesting how individual patients’ risk may be reduced by manipulating modifiable risk factors. This appears particularly relevant for those applications in which changes in lifestyle or medication can be recommended. In fact, the optimal intervention mix may be different for patients with the same overall risk based on the relative contribution of each risk component.
Our study shows some limitations. First, we opted not to include novel markers of glomerular filtration rate, e.g. cystatin C and Beta2-microglobulin (3, 10), into CALIBRA due to their limited availability in the real-world clinical records. Nevertheless, our method for the derivation of Literature-Based NBCs allows to add any further risk factor as soon as sufficient evidence is published and the candidate biomarker is ready to be adopted in clinical practice. Second, CALIBRA shows a slightly reduced discrimination in the GCKD population compared to that achieved in the FMC-NephroCare cohort. This observation could suggest that our model may not fully capture the underlying CVD data generation process in NDD-CKD patients. In fact, CALIBRA was derived using data from both longitudinal and historical cohorts in which endpoint definition, measures, and data collection may be subjected to underreporting of less severe cases. Whereas this potential weakness deserves further investigation, it is important to emphasize that CALIBRA still demonstrated superior accuracy compared to established cardiovascular risk scores even in a rigorous longitudinal cohort study setting.
5 Conclusions
In contrast to widely used CVD risk profiles, CALIBRA incorporates both traditional risk factors and CKD-specific prognostic variables to obtain an accurate cardiovascular prediction in non-dialysis dependent CKD patients. Contrary to equation-based scores which may not be calculable for a large proportion of patients with incomplete data, CALIBRA extends to all patients and allows explicit assessment of prediction robustness in case of missing values for key risk factors. Furthermore, CALIBRA can be easily updated by incorporating novel predictors allowing to make full use of the new advancements of research in the field. Our analysis suggests that CALIBRA is a valuable addition to the current tools used by nephrologists to stratify patients’ risk and inform referral to cardiovascular prevention programs.
Data Availability Statement
The datasets presented in this article were acquired from FMC Nephrocare and GCKD cohorts. The raw clinical data are not readily available because data sharing would violate the terms and conditions under which Fresenius Medical Care acquired the data. Pseudo-anonymized raw data may be accessible under a data sharing agreement upon well-motivated requests by qualified scientists upon approval by the CALIBRA study steering committee.
Author Contributions
FB and LN contributed to study concept, design, statistical analysis, interpretation of results, manuscript drafting, and approved the final version of the manuscript. CL performed literature search, contributed to interpretation of results, drafted the first version of the manuscript, and approved the final version of the manuscript. JT contributed to interpretation of results and drafted the first version of the manuscript, and approved the final version of the manuscript. JN, MH, MatS, BB, MarS, US, and K-UE contributed to data acquisition, interpretation of results, and reviewed and approved the final version of the manuscript. UT performed literature search, project conceptualization and project administration, and reviewed and approved the final version of the manuscript. CB contributed to study concept, interpretation of results, and approved the final version of the manuscript. CM contributed to interpretation of results, and approved the final version of the manuscript. SoS contributed to interpretation of results and approved the final version of the manuscript. StS contributed to interpretation of results, and reviewed and approved the final version of the manuscript. All authors contributed to the article and approved the submitted version.
Funding
The GCKD study was/is supported by the German Ministry of Education and Research (Bundesministerium für Bildung und Forschung, FKZ 01ER 0804, 01ER 0818, 01ER 0819, 01ER 0820, and 01ER 0821), KfH Foundation for Preventive Medicine, Innovative Medicines Initiative 2 Joint Undertaking (BEAt-DKD, grant number 115974) and corporate sponsors (www.gckd.org). The funder was not involved in the study design, collection, analysis, interpretation of data, the writing of this article or the decision to submit it for publication.
Conflict of Interest
LN, JT, FB, SoS, StS, CM, CB, and UT are full time employees at Fresenius Medical Care. CL provided medical writing services on behalf of Fresenius Medical Care. HM reports grants from KfH Foundation of Preventive Medicine, and grants from German ministry of Education and Research. MatS reports grants from Fresenius Medical Care during the conduct of the study. BB reports grants from the Federal Ministry of Education and Research (Bundesministerium für Bildung und Forschung (www.bmbf.de), FKZ 01ER 0804, 01ER 0818, 01ER 0819, 01ER 0820 und 01ER 0821), and grants from Foundation for Preventive Medicine of the KfH (Kuratorium für Heimdialyse und Nierentransplantation e.V.–Stiftung Präventivmedizin; www.kfh-stiftung-praeventivmedizin.de). MarS reports grants from Fresenius Medical Care outside the submitted work. K-UE reports grants from: Astra Zeneca, Bayer, Fresenius Medical Care, Vifor, and Amgen during the conduct of the study, personal fees from Akebia, Astellas, Astra Zeneca, Bayer, and Boehringer Ingelheim, and grants from Genzyme, Shire, and Vifor outside the submitted work.
The remaining authors declare that the research was conducted in the absence of any commercial or financial relationships that could be construed as a potential conflict of interest.
Publisher’s Note
All claims expressed in this article are solely those of the authors and do not necessarily represent those of their affiliated organizations, or those of the publisher, the editors and the reviewers. Any product that may be evaluated in this article, or claim that may be made by its manufacturer, is not guaranteed or endorsed by the publisher.
Acknowledgments
This research was supported by Fresenius Medical Care. The views and opinions expressed in the article are those of the individual authors and do not necessarily reflect the official position of the contributor company.
Supplementary Material
The Supplementary Material for this article can be found online at: https://www.frontiersin.org/articles/10.3389/fneph.2022.922251/full#supplementary-material
References
1. Webster AC, Nagler EV, Morton RL, Masson P. Chronic Kidney Disease. Lancet (2017) 389(10075):1238–52. doi: 10.1016/S0140-6736(16)32064-5
2. Herzog CA, Asinger RW, Berger AK, Charytan DM, Díez J, Hart RG, et al. Cardiovascular Disease in Chronic Kidney Disease. A Clinical Update From Kidney Disease: Improving Global Outcomes (KDIGO). Kidney Int (2011) 80(6):572–86. doi: 10.1038/ki.2011.223
3. Ballew SH, Matsushita K. Cardiovascular Risk Prediction in CKD. Semin Nephrol (2018) 38(3):208–16. doi: 10.1016/j.semnephrol.2018.02.002
4. Gansevoort RT, Correa-Rotter R, Hemmelgarn BR, Jafar TH, Heerspink HJL, Mann JF, et al. Chronic Kidney Disease and Cardiovascular Risk: Epidemiology, Mechanisms, and Prevention. Lancet (2013) 382(9889):339–52. doi: 10.1016/S0140-6736(13)60595-4
5. Thompson S, James M, Wiebe N, Hemmelgarn B, Manns B, Klarenbach S, et al. Cause of Death in Patients With Reduced Kidney Function. J Am Soc Nephrol (2015) 26(10):2504–11. doi: 10.1681/ASN.2014070714
6. Levin A, Stevens PE. Summary of KDIGO 2012 CKD Guideline: Behind the Scenes, Need for Guidance, and a Framework for Moving Forward. Kidney Int (2014) 85(1):49–61. doi: 10.1038/ki.2013.192
7. Levin A, Stevens PE, Bilous RW, Coresh J, De Francisco ALM, De Jong PE, et al. Kidney Disease: Improving Global Outcomes (KDIGO) CKD Work Group. KDIGO 2012 Clinical Practice Guideline for the Evaluation and Management of Chronic Kidney Disease. Kidney Int Suppl (2013) 3(1):1–150. doi: 10.1038/kisup.2012.73
8. Weiner DE, Tighiouart H, Elsayed EF, Griffith JL, Salem DN, Levey AS, et al. The Framingham Predictive Instrument in Chronic Kidney Disease. J Am Coll Cardiol (2007) 50(3):217–24. doi: 10.1016/j.jacc.2007.03.037
9. Chen SC, Su HM, Tsai YC, Huang JC, Chang JM, Hwang SJ, et al. Framingham Risk Score With Cardiovascular Events in Chronic Kidney Disease. PloS One (2013) 8(3):e60008. doi: 10.1371/journal.pone.0060008
10. Matsushita K, Coresh J, Sang Y, Chalmers J, Fox C, Guallar E, et al. Kidney Measures Beyond Traditional Risk Factors for Cardiovascular Prediction: A Collaborative Meta-Analysis HHS Public Access. Lancet Diabetes Endocrinol (2015) 3(7):514–25. doi: 10.1016/S2213-8587(15)00040-6
11. McMurray JJV, Uno H, Jarolim P, Desai AS, De Zeeuw D, Eckardt KU, et al. Predictors of Fatal and Nonfatal Cardiovascular Events in Patients With Type 2 Diabetes Mellitus, Chronic Kidney Disease, and Anemia: An Analysis of the Trial to Reduce Cardiovascular Events With Aranesp (Darbepoetin-Alfa) Therapy (TREAT). Am Heart J (2011) 162(4):748–755.e3. doi: 10.1016/j.ahj.2011.07.016
12. Steil H, Amato C, Carioni C, Kirchgessner J, Marcelli D, Mitteregger A, et al. EuCliD® - A Medical Registry. Methods Inf Med (2004) 43(1):83–8. doi: 10.1055/s-0038-1633841
13. Eckardt KU, Bärthlein B, Seema BA, Beck A, Busch M, Eitner F, et al. The German Chronic Kidney Disease (GCKD) Study: Design and Methods. Nephrol Dial Transplant (2012) 27(4):1454–60. doi: 10.1093/ndt/gfr456.
15. Constantinou AC, Yet B, Fenton N, Neil M, Marsh W. Value of Information Analysis for Interventional and Counterfactual Bayesian Networks in Forensic Medical Sciences. Artif Intell Med (2016) 66:41–52. doi: 10.1016/j.artmed.2015.09.002
16. Kjærulff UB, Madsen AL. Bayesian Networks and Influence Diagrams: A Guide to Construction and Analysis. (2013). doi: 10.1007/978-1-4614-5104-4
17. Tschulena U, Bellocchio F, Neri L, Amsen W, Barbieri C. Medical Device and Computer-Implemented Method of Predicting Risk, Occurrence or Progression of Adverse Health Conditions in Test Subjects in Subpopulations Arbitrarily Selected From a Totalò Population. (2019).
18. Bellocchio F, Carioni P, Lonati C, Garbelli M, Martínez-Martínez F, Stuard S, et al. Enhanced Sentinel Surveillance System for Covid-19 Outbreak Prediction in a Large European Dialysis Clinics Network. Int J Environ Res Public Health (2021) 18(18):9739. doi: 10.3390/ijerph18189739
19. Bellocchio F, Lonati C, Titapiccolo JI, Nadal J, Meiselbach H, Schmid M, et al. Validation of a Novel Predictive Algorithm for Kidney Failure in Patients Suffering From Chronic Kidney Disease: The Prognostic Reasoning System for Chronic Kidney Disease (PROGRES-CKD). Int J Environ Res Public Health (2021) 18(23):12649. doi: 10.3390/ijerph182312649
20. Quan H, Sundararajan V, Halfon P, Fong A, Burnand B, Luthi JC, et al. Coding Algorithms for Defining Comorbidities in ICD-9-CM and ICD-10 Administrative Data. Med Care (2005) 43(11):1130–9. doi: 10.1097/01.mlr.0000182534.19832.83
21. Levey AS, Eckardt KU, Tsukamoto Y, Levin A, Coresh J, Rossert J, et al. Definition and Classification of Chronic Kidney Disease: A Position Statement From Kidney Disease: Improving Global Outcomes (KDIGO)Z. Kidney Int (2005) 67(6):2089–100. doi: 10.1111/j.1523-1755.2005.00365.x
22. Levey AS, De Jong PE, Coresh J, El NM, BC A, Matsushita K, et al. The Definition, Classification, and Prognosis of Chronic Kidney Disease: A KDIGO Controversies Conference Report. Kidney Int (2011) 80(1):17–28. doi: 10.1038/ki.2010.483
23. Levey AS, Stevens LA, Schmid CH, Zhang Y, Castro AF, Feldman HI, et al. A New Equation to Estimate Glomerular Filtration Rate. Ann Intern Med (2009) 150(9):604–12. doi: 10.7326/0003-4819-150-9-200905050-00006
24. D’Agostino RB, Grundy S, Sullivan LM, Wilson P. Validation of the Framingham Coronary Heart Disease Prediction Scores: Results of a Multiple Ethnic Groups Investigation. J Am Med Assoc (2001) 286(2):180–7. doi: 10.1001/jama.286.2.180
25. Pocock SJ, McCormack V, Gueyffier F, Boutitie F, Fagard RH, Boissel JP. A Score for Predicting Risk of Death From Cardiovascular Disease in Adults With Raised Blood Pressure, Based on Individual Patient Data From Randomised Controlled Trials. Br Med J (2001) 323(7304):75–81. doi: 10.1136/bmj.323.7304.75
26. Goff DC, Lloyd-Jones DM, Bennett G, Coady S, D’Agostino RB, Gibbons R, et al. 2013 ACC/AHA Guideline on the Assessment of Cardiovascular Risk: A Report of the American College of Cardiology/American Heart Association Task Force on Practice Guidelines. J Am Coll Cardiol (2014) 129(25 Suppl 2):S49–73. doi: 10.1161/01.cir.0000437741.48606.98
27. Cardiovascular ESC. SCORE2-OP Risk Prediction Algorithms: Estimating Incident Cardiovascular Event Risk in Older Persons in Four Geographical Risk Regions. Eur Heart J (2021) 42(25):2455–67. doi: 10.1093/eurheartj/ehab312
28. DeLong ER, DeLong DM, Clarke-Pearson DL. Comparing the Areas Under Two or More Correlated Receiver Operating Characteristic Curves: A Nonparametric Approach. Biometrics (1988) 44(3):837–45. doi: 10.2307/2531595
29. Matsushita K, Coresh J, Sang Y, Chalmers J, Fox C, Guallar E, et al. Estimated Glomerular Filtration Rate and Albuminuria for Prediction of Cardiovascular Outcomes: A Collaborative Meta-Analysis of Individual Participant Data. Lancet Diabetes Endocrinol (2015) 3(7):514–25. doi: 10.1016/S2213-8587(15)00040-6
30. Zimmermann J, Herrlinger S, Pruy A, Metzger T, Wanner C. Inflammation Enhances Cardiovascular Risk and Mortality in Hemodialysis Patients. Kidney Int (1999) 55(2):648–58. doi: 10.1046/j.1523-1755.1999.00273.x
31. Liu Y, Berthier-Schaad Y, Fallin MD, Fink NE, Tracy RP, Klag MJ, et al. IL-6 Haplotypes, Inflammation, and Risk for Cardiovascular Disease in a Multiethnic Dialysis Cohort. J Am Soc Nephrol (2006) 17(3):863–70. doi: 10.1681/ASN.2005050465
32. Matsushita K, Jassal SK, Sang Y, Ballew SH, Grams ME, Surapaneni A, et al. Incorporating Kidney Disease Measures Into Cardiovascular Risk Prediction: Development and Validation in 9 Million Adults From 72 Datasets. EClinicalMedicine (2020) 27:100552. doi: 10.1016/j.eclinm.2020.100552
Keywords: cardiovascular risk score, chronic kidney disease, cardiovascular events, hospitalization, machine learning, personalized medicine
Citation: Neri L, Lonati C, Titapiccolo JI, Nadal J, Meiselbach H, Schmid M, Baerthlein B, Tschulena U, Schneider MP, Schultheiss UT, Barbieri C, Moore C, Steppan S, Eckardt K-U, Stuard S and Bellocchio F (2022) The Cardiovascular Literature-Based Risk Algorithm (CALIBRA): Predicting Cardiovascular Events in Patients With Non-Dialysis Dependent Chronic Kidney Disease. Front. Nephrol. 2:922251. doi: 10.3389/fneph.2022.922251
Received: 17 April 2022; Accepted: 20 May 2022;
Published: 12 July 2022.
Edited by:
Michal Nowicki, Medical University of Lodz, PolandReviewed by:
Mei Liu, University of Kansas Medical Center, United StatesGiulia Capitoli, University of Milano Bicocca, Italy
Copyright © 2022 Neri, Lonati, Titapiccolo, Nadal, Meiselbach, Schmid, Baerthlein, Tschulena, Schneider, Schultheiss, Barbieri, Moore, Steppan, Eckardt, Stuard and Bellocchio. This is an open-access article distributed under the terms of the Creative Commons Attribution License (CC BY). The use, distribution or reproduction in other forums is permitted, provided the original author(s) and the copyright owner(s) are credited and that the original publication in this journal is cited, in accordance with accepted academic practice. No use, distribution or reproduction is permitted which does not comply with these terms.
*Correspondence: Luca Neri, Luca.Neri@fmc-ag.com