- 1Neuroscience Institute, Lithuanian University of Health Sciences, Kaunas, Lithuania
- 2Department of Informatics, Vytautas Magnus University, Kaunas, Lithuania
- 3Institute of Biophysics, National Research Council, Palermo, Italy
- 4Université Côte d'Azur, Centre National de la Recherche Scientifique (CNRS), Institut de Pharmacologie Moléculaire et Cellulaire (IPMC), Valbonne, France
Alzheimer's disease (AD) is a progressive memory loss and cognitive dysfunction brain disorder brought on by the dysfunctional amyloid precursor protein (APP) processing and clearance of APP peptides. Increased APP levels lead to the production of AD-related peptides including the amyloid APP intracellular domain (AICD) and amyloid beta (Aβ), and consequently modify the intrinsic excitability of the hippocampal CA1 pyramidal neurons, synaptic protein activity, and impair synaptic plasticity at hippocampal CA1–CA3 synapses. The goal of the present study is to build computational models that incorporate the effect of AD-related peptides on CA1 pyramidal neuron and hippocampal synaptic plasticity under the AD conditions and investigate the potential pharmacological treatments that could normalize hippocampal synaptic plasticity and learning in AD. We employ a phenomenological N-methyl-D-aspartate (NMDA) receptor-based voltage-dependent synaptic plasticity model that includes the separate receptor contributions on long-term potentiation (LTP) and long-term depression (LTD) and embed it into the a detailed compartmental model of CA1 pyramidal neuron. Modeling results show that partial blockade of Glu2NB-NMDAR-gated channel restores intrinsic excitability of a CA1 pyramidal neuron and rescues LTP in AICD and Aβ conditions. The model provides insight into the complex interactions in AD pathophysiology and suggests the conditions under which the synchronous activation of a cluster of synaptic inputs targeting the dendritic tree of CA1 pyramidal neuron leads to restored synaptic plasticity.
1 Introduction
Alzheimer's disease (AD) has a long preclinical stage and, before any clinical symptoms appear, pathological processes are observed in the hippocampus and entorhinal cortex, key brain structures responsible for memory encoding and retrieval. AD cannot be prevented, halted, or cured today, and new interdisciplinary ways are urgently needed for the understanding and treatment of this devastating disease. Recent experimental evidence supports the fundamental role of AD-related peptides early in the pathology: in particular, the most widely studied amyloid beta (Aβ) and the less investigated amyloid precursor protein (APP) C-terminal peptide (AICD). Their differential effects on synaptic function and intrinsic excitability of hippocampal CA1 pyramidal neuron at a single cell level are currently being investigated. However, the impact and complex interaction effects of Aβ and AICD on hippocampal synaptic plasticity and CA1 neuron activity remain largely unknown.
It is widely believed that, before any ongoing tau or neuroinflammation pathologies, the first molecular events occurring in the AD brain are alterations of APP processing and/or clearance of APP peptides. This leads to the well-documented alteration in levels of Aβ, which readily aggregates (Haass and Selkoe, 2007). There is also evidence that the levels of AICD, the production of which is intimately linked with Aβ, processing, are also elevated in early AD (Ghosal et al., 2009; Rajão-Saraiva et al., 2023). Accumulating experimental evidence suggests that each peptide plays a role in modifying hippocampus function in the early stages of the disease. AICD has a strong impact on synapse function, as recently shown by Pousinha et al. (2017) and on intrinsic excitability (Pousinha et al., 2019). Increased levels of AICD, as previously observed in AD mouse models and human patients, enhance GluN2B-NMDAR contribution, overactivate SK channels, and strongly perturb long-term potentiation (LTP), but spare long-term depression (LTD) in CA1 pyramidal neurons. Partial antagonism of GluN2B-NMDAR rescues LTP in early AD.
Oligomeric forms of Aβ prevented induction of LTP in hippocampal cultured neurons (Opazo et al., 2018). Incubation with oligomeric Aβ led to a dose-dependent activation of calcium/calmodulin-dependent kinase II (CaMKII) via GluN2B-NMDAR (Opazo et al., 2018). CaMKII is a key protein for LTP expression, activated directly by Ca2+ influx through GluN2B-NMDAR and leading to phosphorylation of synaptic proteins and increase in the number of active AMPARs or their single-channel conductance (Shipton and Paulsen, 2014; Park et al., 2021; Yasuda et al., 2022). Oligomeric Aβ increased CaMKII overall activity, prevented its subsequent T286 autophosphorylation by plasticity-inducing stimulation, and led to the LTP-mediated immobilization of CaMKII at dendritic spines and diffusional trapping of AMPARs. In addition, oligomeric Aβ caused dendritic spine loss in a GluN2B-NMDAR-dependent manner (Opazo et al., 2018). CaMKII inhibitors KN93, tatCN21, and specific GluN2B-NMDAR antagonist ifenprodil completely rescued Aβ-induced inhibition of LTP by preventing CaMKII overactivation and dendritic spine loss (Opazo et al., 2018). The results demonstrated that Aβ prevents LTP induction by activating CaMKII in a GluN2B-NMDAR-dependent manner.
This study aims at explaining early hippocampus-dependent learning and memory deficits induced by increased levels of AICD and Aβ, a condition that mimics early AD. To model the effects of AICD and Aβ on excitatory neuron activity, we focused on recent publications analyzing the acute effect (1–5 h) of peptide-delivered ex-vivo at physiopathologically relevant concentrations (nanomolar range; Abramov et al., 2009; Pousinha et al., 2017, 2019; Opazo et al., 2018; Taylor et al., 2021).
We developed a data-driven in silico model of the hippocampal CA1 pyramidal neuron under AD conditions, with the main objectives being to incorporate the effects of AICD and Aβ into computational models of CA1 pyramidal neuron and hippocampal synaptic plasticity. The goal of this study was to explain complex interactions of synaptic and cellular-level mechanisms of altered hippocampal function that leads to impaired learning and progressive irreversible memory loss in AD, and finally, to identify and assess potential targets for innovative pharmacological treatment of AD.
2 Methods
We used a modified NMDAR subunit-dependent voltage-based model of synaptic weight change at hippocampal CA3–CA1 synapses (Dainauskas et al., 2023) to investigate the effect of elevated levels of Aβ and AICD. We embedded this model into a multicompartmental CA1 pyramidal neuron model (Peng et al., 2016; Migliore et al., 2018) to study the influence of AICD and of Aβ on synaptic weights within a cluster of randomly distributed CA3–CA1 synapses onto apical dendrites of a CA1 neuron in the stratum radiatum (SR) region. We modeled LTP and LTD, induced by high and low frequency stimulation, respectively, and the alterations due to the increased levels of AD peptides. Finally, we analyzed the effect of GluN2B-NMDAR function on the synaptic properties and a possible pharmacological treatment to restore normal synaptic function in AD.
2.1 Synapse model under AICD and Aβ conditions
We employed a phenomenological voltage-dependent NMDAR-based synaptic plasticity model, developed in our previous study (Dainauskas et al., 2023) (ModelDB accession number 267680), and extended it to incorporate the effects of AICD and Aβ in AD. The model relies on the functioning of the GluN2A-NMDAR and GluN2B-NMDAR subunits as the separate mediators of LTD and LTP, respectively. It is assumed that LTP is mainly dependent on GluN2B-NMDAR (Morishita et al., 2007; Andrade-Talavera et al., 2016; Pousinha et al., 2017), and GluN2A-NMDAR is the main mediator of LTD (Morishita et al., 2007).
Schematic diagram of the synapse model in control conditions and under the influence of increased AICD and Aβ is presented in Figure 1. In the model, active NDMARs, consisting of GluN2B-NMDAR and GluN2A-NMDAR subunits, trigger LTP and LTD functions ϕNMDALTP and ϕNMDALTD, respectively, that represent second messenger pathways responsible for LTP and LTD induction, such as CaMKII and phosphatase activation, in a phenomenological manner. These functions take a form of a Hill equation and are activated mainly by the postsynaptic GluN2B-NMDAR for LTP term and by postsynaptic GluN2A-NMDAR for the LTD term. Subsequently, the functions ϕNMDALTP and ϕNMDALTD are multiplied by the low-filtered membrane potential at a synapse location and , correspondingly, to form the LTP and LTD components that linearly add to calculate the instantaneous change of the AMPAR weight wAMPA (Figure 1A). In addition, the model diagram shows dendritic Ca2+-dependent K+ channels CagK and L-type Ca2+ channels CaL, that shape synaptic plasticity properties in AD. Specifically, intracellular NMDAR-mediated Ca2+ activates the nearby Ca2+-dependent K+ channels CagK, that may lead to hyperpolarization of the membrane potential and in turn inhibit NMDAR. Moreover, these Ca2+-dependent K+ channels CagK are activated by Ca2+ through L-type Ca2+ channels CaL. As dendritic ion channels CagK and CaL influence synaptic weight wAMPAR indirectly through NMDAR and membrane potential filtered values and , these elements are presented in gray boxes. The model equations are described in Supplementary material.
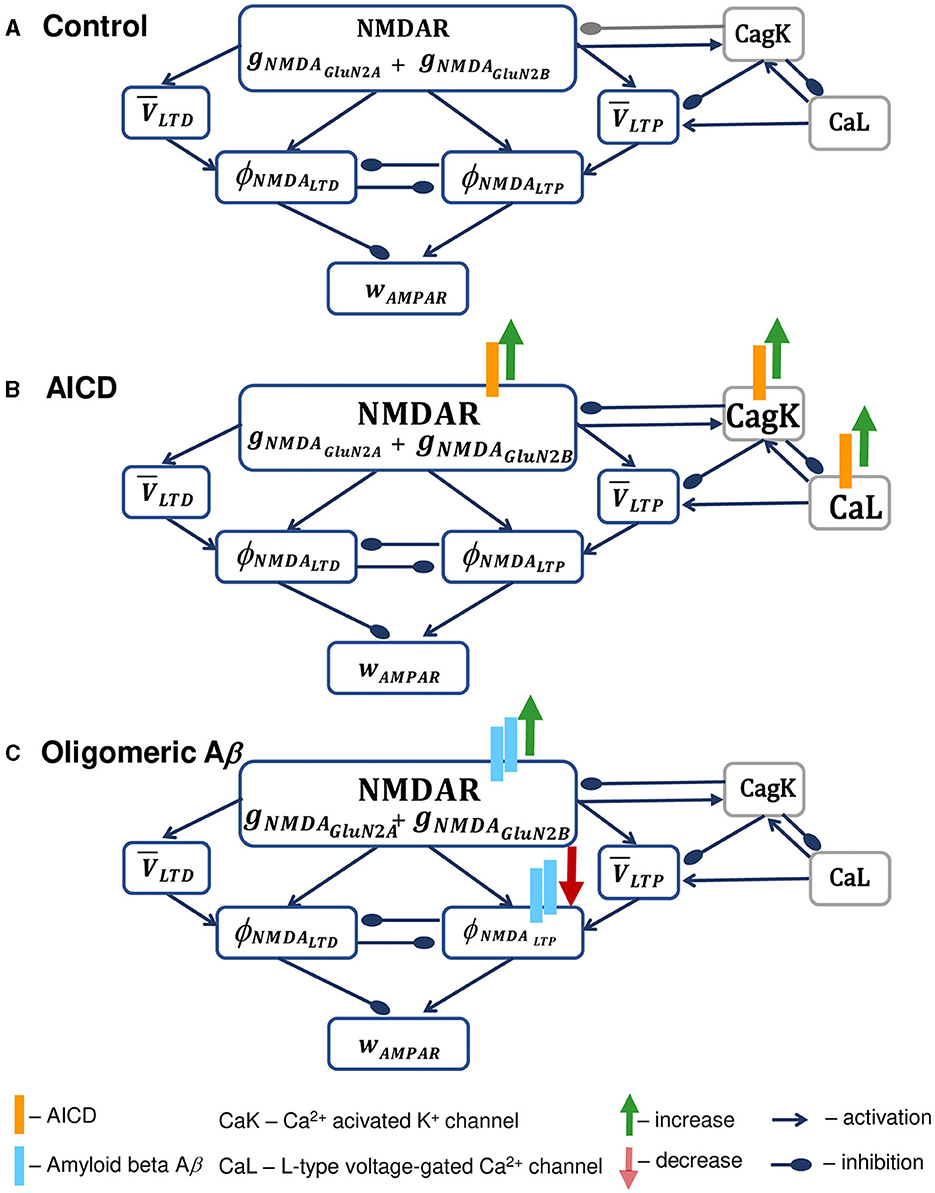
Figure 1. Schematic diagram of the synapse model in control conditions (A), under the increased AICD (B), and Aβ (C) concentrations in AD. (A) Presynaptic action potential activates NMDAR, composed of GluN2B-NMDAR and GluN2A-NMDAR subunits gNMDAGluN2B and gNMDAGluN2A, and triggers LTP and LTD functions ϕNMDALTP and ϕNMDALTD, respectively. These LTP and LTD functions ϕNMDALTP and ϕNMDALTD mutually inhibit each other. NMDAR contributes to the postsynaptic local membrane potential that is low-pass filtered, and the resulting LTP and LTD variables and are multiplied by the corresponding NMDAR-dependent functions ϕNMDALTP and ϕNMDALTD to form the LTP and LTD components of the AMPAR weight wAMPAR. NMDAR is inhibited by the activity of the nearby Ca2+-dependent K+ channels CagK, that are in turn triggered by the NMDAR activation and lead to the hyperpolarization of the membrane potential. In a similar manner, Ca2+-dependent K+ channels CagK are activated by L-type Ca2+ channels CaL. Dendritic ion channels CagK and CaL exhibit the effect on synaptic weight wAMPAR indirectly through the influence on NMDAR and membrane potential filtered values and , and are therefore presented in gray boxes. (B) Elevated AICD concentrations (orange bars) lead to GluN2B-NMDAR-gated channel conductance upregulation (green arrow) and increase in Ca2+-activated K+ channel CagK conductance (green arrow) and L-type Ca2+ channel CaL conductance (green arrow; Pousinha et al., 2017, 2019). The resulting high intracellular Ca2+ levels overactivate Ca2+-dependent K+ channels and cause hyperpolarization of the neuron membrane, thus leading to the failure in LTP induction. Increase in specific membrane conductance and in axonal, somatic, and dendritic M-type K+ current and decrease in axonal, somatic, and basal Na+ current are omitted. (C) Oligomeric Aβ (light blue bars) increases NMDAR activation (green arrow) through glutamate transmission facilitation (Abramov et al., 2009; Taylor et al., 2021) and prevents the activation of LTP protein CaMKII expressed as a phenomenological function ϕNMDALTP, thus leading to deficits in LTP (Opazo et al., 2018). Spine loss caused by Aβ is not indicated.
To study the effect of AICD on synaptic plasticity, we incorporated the AICD influence on NMDAR and ion channels following Pousinha et al. (2017, 2019). Specifically, as shown in Figure 1B, elevated AICD levels (orange bars) result in the increased GluN2B-NMDAR—gated channel conductance (green arrow), and increased Ca2+—dependent K+ channel CagK conductance (green arrow), increased L-type Ca2+ channel CaL conductance (green arrow). In addition, AICD leads to the increase in specific membrane conductance and axonal, somatic, and dendritic M-type K+ current, and decrease in axonal, somatic, and basal Na+ current. These modifications are omitted in the model diagram because the changes in channels are not observed in the proximity of a synapse. As a result of the elevated AICD levels, intracellular Ca2+ concentration rises, overactivates Ca2+—dependent K+ channels, and leads to the hypoexcitability of the neuron preventing LTP induction.
We modeled the influence of AICD by increasing conductances of GluN2B-NMDAR-gated channels by 400% (Pousinha et al., 2019); dendritic and somatic Ca2+-dependent K+ channels by 120%; somatic and dendritic L-type Ca2+ channels by 200%; axonal, somatic, and dendritic M-type channels by 120, 200, and 200%, respectively, decreasing the conductance of axonal, somatic, and basal Na+ channels by 22.2, 66.6, and 33.3%, respectively, and increasing specific membrane conductance by 180%.
Furthermore, we analyzed the influence of increasing oligomeric Aβ levels (Figure 1C). Oligomeric forms of Aβ (light blue bars) lead to the increase in glutamate transmission and higher NMDAR (green arrow) and AMPAR activations, prevention of CaMKII phosphorylation in a GluN2B-NMDAR-dependent manner (red arrow), and spine loss (Opazo et al., 2018). A critical role of GluN2B-NMDAR in oligomeric Aβ-mediated LTP impairment in hippocampal slices was also shown by Rönicke et al. (2011). Aβ oligomers also increase glutamate release probability (Abramov et al., 2009) and enhance LTD (Taylor et al., 2021).
We model the effect of Aβ by increasing the glutamate concentration by 120% (Abramov et al., 2009), that causes enhancement of LTD (Taylor et al., 2021). We reduce synaptic density to 80% (Opazo et al., 2018) and transform the GluN2B-NMDAR-dependent function for LTP component ϕNMDALTP into the bell-shape function so that it remains close to zero even when GluN2B-NMDAR is activated, thus failing to trigger LTP (Equation S4, Supplementary material).
Modifications of the synaptic plasticity model parameters under AICD and Aβ conditions are given in Table 1.
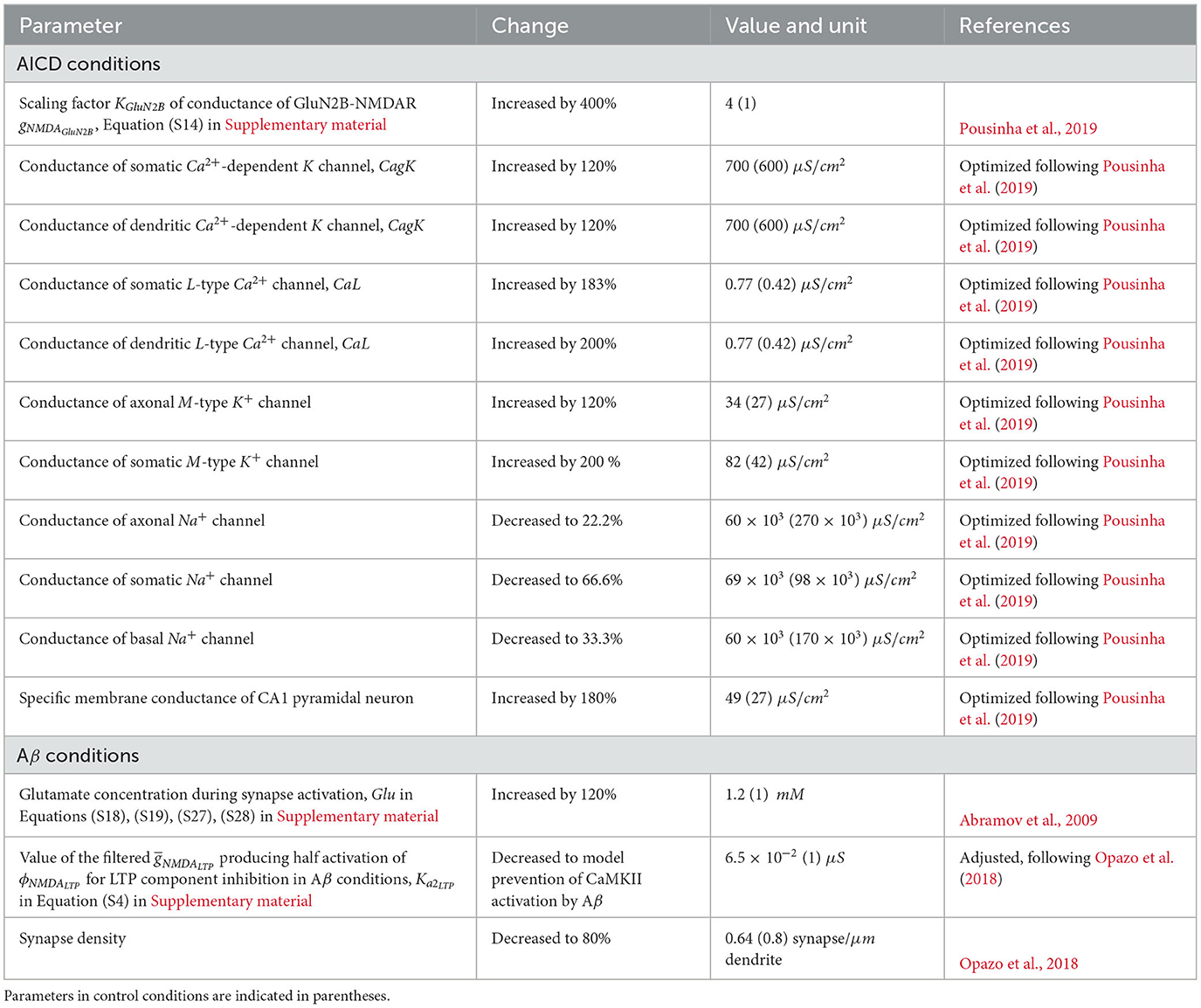
Table 1. Modifications of parameters of synaptic plasticity model and multicompartmental CA1 pyramidal neuron model in AICD and Aβ conditions.
2.2 Multicompartmental model of CA1 pyramidal neuron
We used a morphology reconstruction of a CA1 pyramidal neuron downloaded from: http://www.neuromorpho.org (Peng et al., 2016) (cell fx_CA1_7.CNG.swc). The channel kinetics were based on those used in previously published articles on CA1 hippocampal neurons and validated against a number of experimental findings. The model was implemented with channel kinetics used by Migliore et al. (2018) (ModelDB accession number 244688). In particular, we used a delayed-rectifier type current (KDR), two A-type potassium (KA) currents (for proximal and distal dendrites), a delayed type current (KD), three types of voltage-dependent Ca2+ currents (CaL, CaT, and CaN), the slow afterhyperpolarization (AHP) Ca2+-dependent K+ current (kCa), a Na+ current, and a calcium pump. The kinetics for the non-specific hyperpolarization-activated current (Ih), a M-type potassium, KM, and the medium afterhyperpolarization (AHP) Ca2+-dependent K+ currents (CagK) were optimized for the specific classical accommodating traces by using both the standard Run Fitter tool available in NEURON and the BluePyOpt. In particular, the genetic algorithm used calculates the best seed among 128 offspring produced during a maximum of 100 generations. We performed single-cell optimizations by running four seeds at the same time. Electrophysiological features were extracted from experimental recordings using a tool of the EBRAINS Cellular Level Modeling workflows (https://ebrains.eu/service/cls-interactive/), based on the open-source Electrophysiological Feature Extraction Library (eFEL). The optimizations were carried out simultaneously on any given set of experimental traces until they converged into a good solution (error lower than 0.3 mV using Neuron Run Fitter and three standard deviation in the case of BluePyOpt). The ionic channels were distributed on the membrane according to experimental findings. The KA and Ih increased with distance from the soma (Hoffman and Johnston, 1999), while KD decreased with the distance from the soma. Passive properties and peak conductance for each channel were adapted from their original values to qualitatively reproduce the experimental recordings used as a reference. The specific membrane capacitance (Cm) and the time constant of the calcium pump were included as fitting parameters during the optimization. The values for the peak conductance of each channel were independently optimized in each type of compartment (soma, axon, basal, and apical dendrites).
Starting from results reported by Pousinha et al. (2019) and using the experimental recordings from control mouse and AICD pyramidal neurons herein analyzed, we decided to implement a single cell model optimization, using one typical set of data for each phenotype. In the study by Pousinha et al. (2019), it was shown that AICD causes a decrease in the firing frequency through the enhancement of the afterhyperpolarization. In accordance with several studies, this peculiar behavior is related to the alteration of L-type Ca2+ channels, the Ca2+-activated K+ channels (SK or CagK) and the Kv7/M channels (Kumar and Foster, 2002). For this reason, we first implemented the model for the control condition. Then, keeping constant all the peak channel conductances not directly involved in the firing frequency alteration discussed by Pousinha et al. (2019), we optimized the model to reproduce AICD conditions by re-optimizing only passive properties and the peak conductances for CaL, CagK, KM, and Na+ channels. The results suggested that AICD neurons will have a lower Na+ conductance and ionic channels modulating adaptation would be at higher density, with respect to control. While searching the literature, we could only find one publication (Tamagnini et al., 2015) reporting a minimal impact of oligomeric Aβ on CA1 pyramidal excitability profile in conditions identical to the description of Aβ's effects on synaptic function used by Opazo et al. (2018) (500 nM oligomeric Aβ application for a few hours on hippocampal slices). Under the Aβ conditions, the excitability profile of CA1 pyramidal neuron was thus not modified. Modifications of the multicompartmental CA1 pyramidal neuron model parameters under AICD conditions are given in Table 1.
We formed a cluster of 50 AMPARs and GluN2A/GluN2B-NMDARs synapses, randomly distributed on the apical dendrites of CA1 pyramidal neurons in the SR region. Synapses were defined with a distance of 100–300 μm from the soma and a synaptic density of 0.8 synapses/μm of dendrite (Gasparini et al., 2004; Bezaire et al., 2016).
Synapses were stimulated at 100 Hz for 1 s (LTP protocol) or at 1 Hz for 500 s (LTD protocol) as in the study by Pousinha et al. (2017). To estimate the change in the somatic postsynaptic excitatory potential (EPSP), a presynaptic stimulus was delivered before and after the conditioning stimulation, and the resulting ratio between the maximal values of the resulting EPSPs was calculated. The enhancement and partial blockade of the GluN2B-NMDAR-gated channel in AICD and Aβ conditions was simulated by varying the conductance gNMDAGluN2B (Equation S14 in Supplementary material).
All simulations were carried out the NEURON simulation environment (Hines and Carnevale, 1997) integrated with Python (Van Geit et al., 2016). Optimization of the neuron parameters was performed as a parallel code executed on different high-performance computing systems: JURECA (Juelich Supercomputing Center, Germany), Galileo100 (CINECA, Italy), and Piz Daint (Swiss National Supercomputing Center CSCS).
All model files in NEURON and Python are available for public download under the ModelDB section of the Senselab database, accession numbers 2014822 and 2015000 (https://senselab.med.yale.edu/ModelDB/).
3 Results
We used the extended synaptic plasticity model, embedded into the multicompartmental model of a CA pyramidal neuron, to study how high levels of AICD and Aβ influence synaptic changes at CA3–CA1 synapses in AD. First, we validated the model against the experimental data on impaired synaptic plasticity for increased levels of AICD (Pousinha et al., 2017, 2019) and Aβ (Opazo et al., 2018), and then analyzed the effect of the GluN2B-NMDAR blockade to rescue LTP in conditions when AICD, Aβ, or both toxic peptides are present. In summary, we modeled the following scenarios for LTP and LTD induction at a cluster of AMPARs and GluN2A/GluN2B-NMDARs synapses on the proximal apical dendrites of CA1 pyramidal neuron:
• Control conditions.
• AD conditions:
– increased levels of AICD concentration;
– increased levels of Aβ concentration;
– increased levels of AICD and Aβ.
• AD conditions and restored synaptic plasticity:
– increased levels of AICD concentration with a partial blockade of Glu2NB-NMDAR;
– increased levels of Aβ concentration with a partial blockade of Glu2NB-NMDAR;
– increased levels of AICD and Aβ with a partial blockade of Glu2NB-NMDAR.
3.1 Model validation against experimental findings of impaired synaptic plasticity under AICD and Aβ conditions
The distributions of synaptic weights, the traces of somatic and dendritic membrane potential, and synaptic conductances of GluN2A-NMDAR and GluN2B-NMDAR, crucial for synaptic modification induction at CA3–CA1 synapses, are presented for LTP and LTD stimulation protocols in control, AICD, Aβ, and AICD & Aβ conditions in Figures 2, 3.
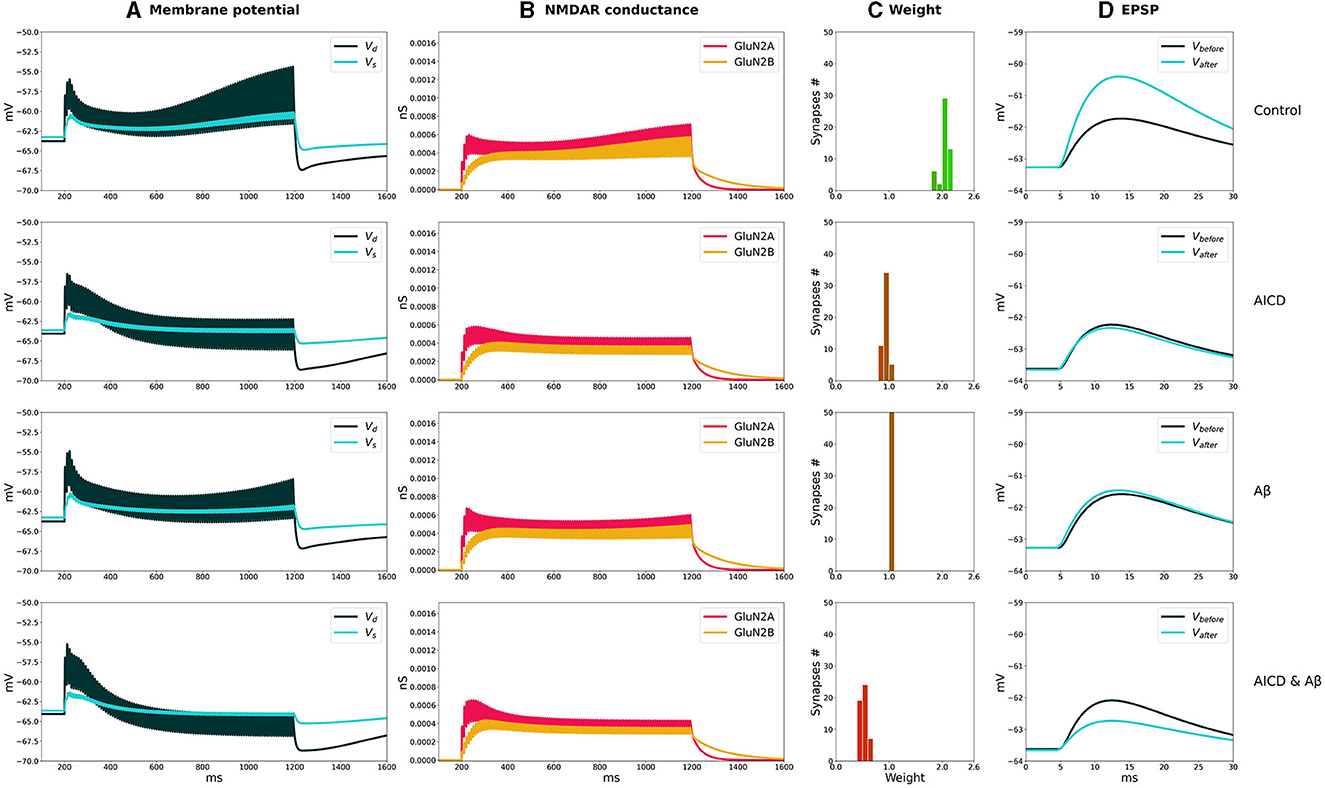
Figure 2. LTP induction in control, AICD, Aβ, and AICD & Aβ conditions. Presynaptic inputs were stimulated at 100 Hz for 1 s. GluN2B-NMDAR synaptic conductance was increased by a factor of 4 in AICD and AICD & Aβ conditions. (A) Membrane potential in soma Vs (cyan line) and at a single synapse Vd (dark green line). (B) GluN2A-NMDAR synaptic conductance (red line) and GluN2B-NMDAR synaptic conductance (yellow line). (C) Synaptic weight distribution after the LTP induction protocol. (D) Somatic EPSP before (black line) and after (cyan line) LTP induction protocol. Rows top to bottom: (Control) Somatic EPSP change is 183%. (AICD) Somatic EPSP change is 94%. (Aβ) Somatic EPSP change is 105%. (AICD & Aβ) Somatic EPSP change is 60%.
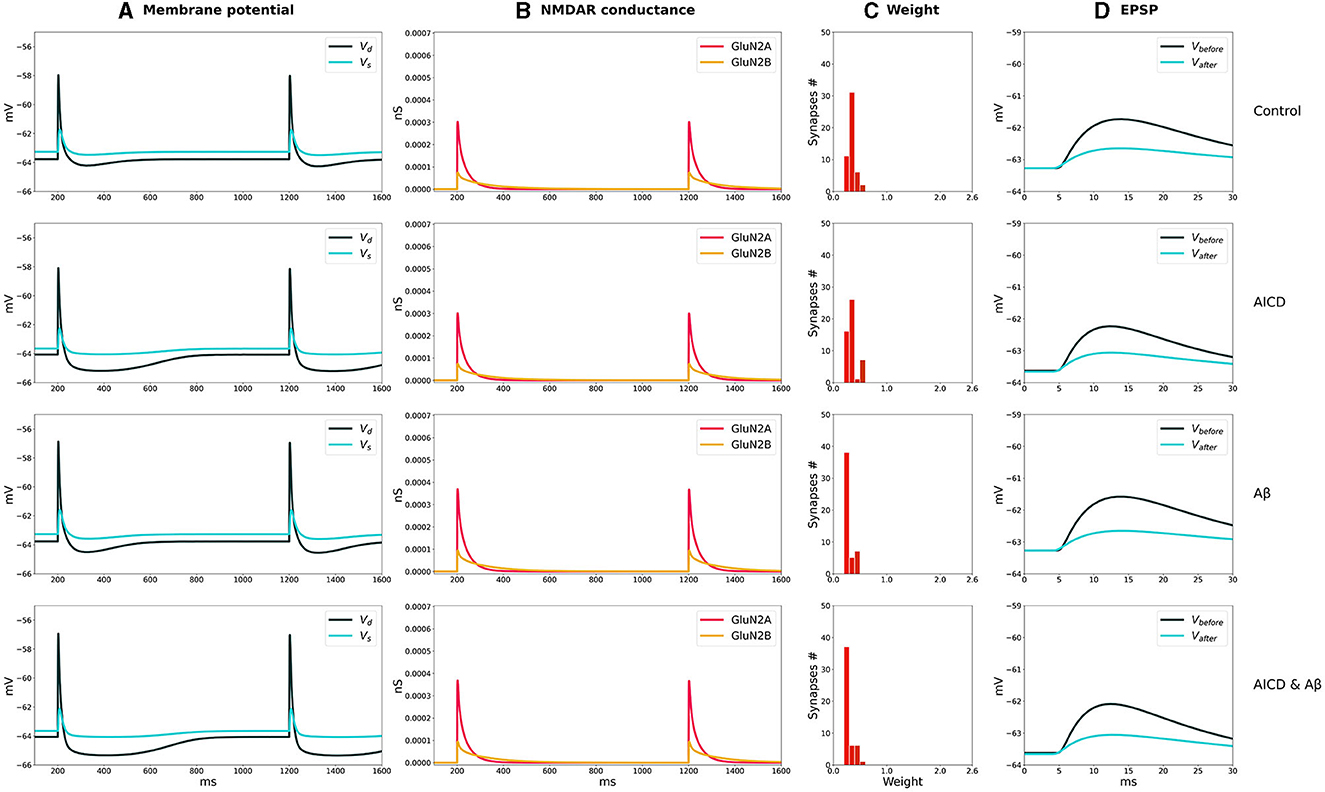
Figure 3. LTD induction in control, AICD, Aβ, and AICD & Aβ conditions. Presynaptic inputs were stimulated at 1 Hz for 500 s. GluN2B-NMDAR synaptic conductance was increased by a factor of 4 in AICD and AICD & Aβ conditions. (A) Membrane potential in soma Vs (cyan line) and at a single synapse Vd (dark green line). (B) GluN2A-NMDAR synaptic conductance (red line) and GluN2B-NMDAR synaptic conductance (yellow line). (C) Synaptic weight distribution after the LTD induction protocol. (D) Somatic EPSP before (black line) and after (cyan line) LTD induction protocol. Rows top to bottom: (Control) Somatic EPSP change is 40%. (AICD) Somatic EPSP change is 42%. (Aβ) Somatic EPSP change is 36%. (AICD & Aβ) Somatic EPSP change is 39%.
In control conditions, the dendritic depolarization caused by a conditioning stimulation at 100 Hz for 1 s, activated Glu2NA-NMDAR and Glu2NB-NMDAR. During this period, the weight of each synapse in a cluster independently evolved according to the local membrane potential and NMDAR-gated synaptic conductance function. Increased Glu2NB-NMDAR triggered LTP induction and inhibited LTD induction. Synaptic weights increased up to the peak value, as shown in the histogram, and the somatic EPSP response increased by 183% (Figure 2, Control).
AICD-mediated alterations in Glu2NB-NMDAR, i.e., upregulation of Ca2+-dependent K+ channels CagK and L-type Ca2+ channels CaL resulted in a neuron hypoexcitability (Pousinha et al., 2019), effectively preventing synaptic plasticity (Figure 2, AICD). Increased concentration of oligomeric Aβ also impaired LTP, limiting its induction to 105% (Figure 2, Aβ). The model suggested that the observed changes were caused by the oligomeric Aβ-mediated synapse loss and inhibition of CaMKII activation via Glu2NB-NMDAR-dependent pathway, with these processes not counterbalanced by the increased glutamate concentration (Opazo et al., 2018). Simultaneous application of AICD and Aβ led to hypoexcitability of the CA1 pyramidal neuron. Although the Glu2NB-NMDAR contribution was high, synaptic weights were reduced leading to an LTD of 60% (Figure 2, AICD & Aβ). The interplay between the upregulation of Glu2NB-NMDAR, Ca2+-dependent K+ channels CagK, L-type Ca2+ channels CaL, and CaMKII inhibition with synapse loss impaired LTP for high-frequency stimulation protocol.
The weak depolarization generated by 1-Hz low-frequency stimulation resulted in a weak NMDAR activation under control conditions. Synaptic weights were reduced to the minimal value of 0.6 leading to a somatic EPSP change of 40% (Figure 3, Control). AICD did not affect LTD as the hypoexcitability of the CA1 pyramidal neuron was not triggered by a low-frequency presynaptic stimulation (Figure 3, AICD).
Oligomeric Aβ led to a stronger LTD of 36% as the elevated glutamate transmission provided stronger local depolarization, while hypoexcitability was not triggered (Figure 3, Aβ). The majority of synaptic weights reached a minimal value of 0.6. Increased levels of both AICD and oligomeric Aβ slightly strengthened LTD up to 39% due to the enhanced glutamate transmission and increased Glu2NB-NMDAR synaptic conductance (Figure 3, AICD & Aβ).
The results show the qualitative agreement with experimental findings. In control conditions, 100 pulses at 100 Hz induced 191% LTP and 500 pulses at 1 Hz led to 57% LTD in hippocampal CA1 pyramidal neurons in rats (Pousinha et al., 2017; Taylor et al., 2021). Elevated AICD concentrations prevented LTP and did not affect LTD (Pousinha et al., 2017). Oligomeric Aβ impaired LTP (Opazo et al., 2018) and strengthened LTD (Taylor et al., 2021). We modeled the joint influence of AICD and Aβ, and observed abolishment of LTP and upregulated LTD.
3.2 GluN2B-NMDAR blockade rescues synaptic functioning under AICD and Aβ conditions
We analyzed how synaptic plasticity was affected by a partial GluN2B-NMDAR blockade in the presence of increased concentrations of AICD, Aβ, for high- and low-frequency stimulations (Figures 4, 5).
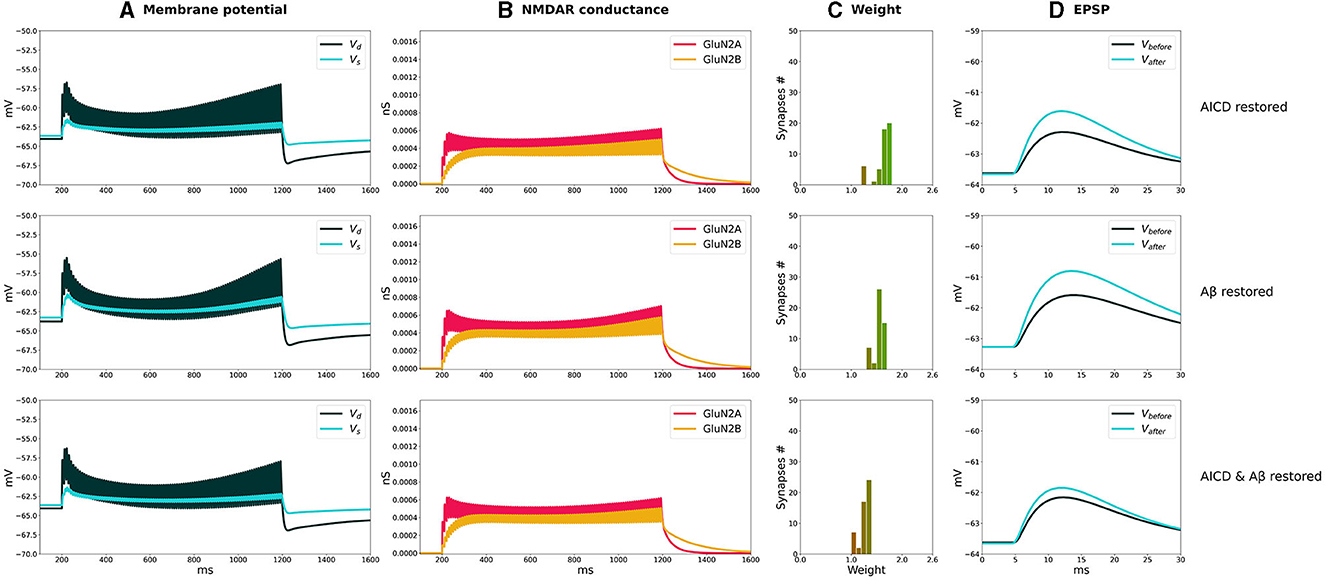
Figure 4. Partial blockade of Glu2NB-NMDARS restores LTP in control, AICD, Aβ, and AICD & Aβ conditions. Presynaptic inputs were stimulated at 100 Hz for 1 s. GluN2B-NMDAR synaptic conductance was increased by a factor of 4 in AICD and AICD & Aβ conditions. (A) Membrane potential in soma Vs (cyan line) and at a single synapse Vd (dark green line). (B) GluN2A-NMDAR synaptic conductance (red line) and GluN2B-NMDAR synaptic conductance (yellow line). (C) Synaptic weight distribution after the LTP induction protocol. (D) Somatic EPSP before (black line) and after (cyan line) LTP induction protocol. Rows top to bottom: (AICD restored). Partial blockade of Glu2NB-NMDAR leaving 0.25 fraction of its active elevated value leads to the EPSP change of 152%. (Aβ restored) Partial blockade of Glu2NB-NMDAR leaving 0.6 fraction of its active value leads to the EPSP change of 144%. (AICD & Aβ restored) Partial blockade of Glu2NB-NMDAR leaving 0.18 fraction of its active elevated value leads to the EPSP change of 122%.
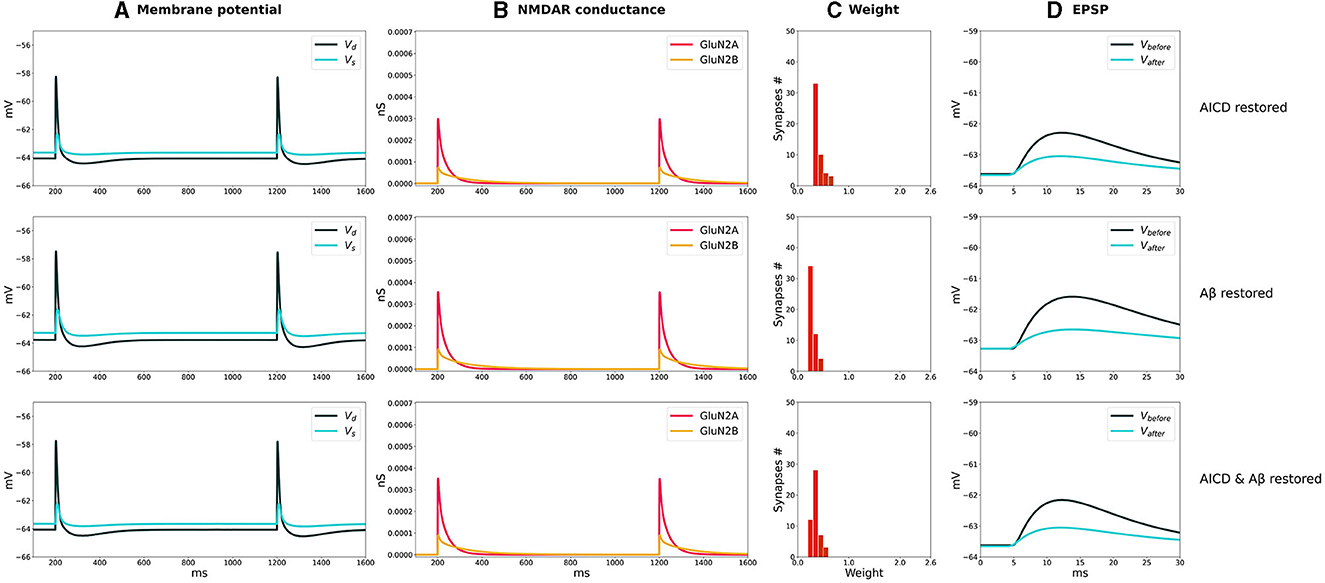
Figure 5. Partial blockade of Glu2NB-NMDARS normalizes LTD in control, AICD, Aβ, and AICD & Aβ conditions. Presynaptic inputs were stimulated at 1 Hz for 500 s. GluN2B-NMDAR synaptic conductance was increased by a factor of 4 in AICD and AICD & Aβ conditions. (A) Membrane potential in soma Vs (cyan line) and at a single synapse Vd (dark green line). (B) GluN2A-NMDAR synaptic conductance (red line) and GluN2B-NMDAR synaptic conductance (yellow line). (C) Synaptic weight distribution after the LTD induction protocol. (D) Somatic EPSP before (black line) and after (cyan line) LTD induction protocol. Rows top to bottom: (AICD restored) Partial blockade of Glu2NB-NMDAR leaving 0.25 fraction of its active elevated value leads to the EPSP change of 45%. (Aβ restored) Partial blockade of Glu2NB-NMDAR leaving 0.6 fraction of its active value leads to the EPSP change of 36%. (AICD & Aβ restored) Partial blockade of Glu2NB-NMDAR leaving 0.18 fraction of its active elevated value leads to the EPSP change of 40%.
In AICD conditions, Glu2NB-NMDAR synaptic conductance was increased by a factor of 4. Partial blockade of Glu2NB-NMDAR leaving 0.25 fraction of its elevated active value rescued LTP (Figure 4, AICD restored). Glu2NB-NMDAR synaptic conductance was returned to its basal value, thus preventing high Ca2+ influx, overactivation of Ca2+-dependent K+ channels, and membrane potential hyperpolarization allowing synaptic weights to reach values close to 2 and the resulting somatic EPSP increase by 152%.
An oligomeric Aβ-induced LTP impairment was restored by a partial blockade of Glu2NB-NMDAR leaving 0.6 fraction of its active value. After the stimulation, the weights increased and somatic EPSP changed by 144% as the Glu2NB-NMDAR-mediated prior overactivation of CaMKII was prevented (Figure 4, Aβ restored). In a similar manner, partial blockade of Glu2NB-NMDAR leaving 0.18 of its active elevated fraction rescued LTP under the conditions of increased AICD and oligomeric Aβ concentrations. Membrane potential was sufficiently depolarized and prior CaMKII overactivation was prevented, enabling synaptic weights to be strengthened and somatic EPSP to increase by 122% (Figure 4, AICD & Aβ restored).
For the low-frequency stimulation at 1 Hz, downregulation of Glu2NB-NMDAR leaving 0.25 fraction of its elevated active value did not show pronounced influence in AICD conditions. Membrane depolarization at the locations of the synapses on the dendritic branches was slightly lower to induce a weight decay, and the somatic EPSP change was equal to 45%, close to the control conditions of 40% (Figure 5, AICD restored). For the elevated oligomeric Aβ concentrations, LTD of 36% was not affected by the lower Glu2NB-NMDAR function with 0.6 fraction of its active value due to the enhanced glutamate transmission (Figure 5, Aβ restored). In the conditions of elevated AICD and oligomeric Aβ concentrations, partial blockade of Glu2NB-NMDAR synaptic conductance with 0.18 fraction of its active elevated value allowed synaptic depression to return to its normal levels of 40% (Figure 5, AICD & Aβ restored).
The results obtained align well with the experimental evidence on the Glu2NB-NMDAR function effect in synaptic plasticity in the presence of the elevated AICD and oligomeric Aβ concentrations. It was shown that ifenprodil, a specific Glu2NB-NMDAR antagonist, rescued LTP in AICD (Pousinha et al., 2017). Ifenprodil reverted oligomeric Aβ-induced inhibition of LTP (Rönicke et al., 2011). Our modeling results suggest that partial blockade of Glu2NB-NMDAR prevents impairment of LTP and LTD when both toxic peptides AICD and Aβ are present by normalizing excitability of CA1 pyramidal neuron and preventing prior CaMKII activation, thus ensuring sufficient membrane depolarization and activity of the second-messenger cascades, necessary for LTP and LTD inductions.
Furthermore, we analyzed how the degree of Glu2NB-NMDAR hypofunction influences synaptic plasticity properties under both normal and AD conditions. The relative somatic EPSP changes as a function of partial Glu2NB-NMDAR blockade for elevated AICD, Aβ, and AICD & Aβ concentrations versus healthy control state are shown in Figures 6–8. The proportion of active GluN2B-NMDAR was calculated in relation to the maximum GluN2B-NMDAR conductance under normal conditions and was converted to the active fraction by normalizing it by the maximum GluN2B-NMDAR conductance in AICD, Aβ, and AICD & Aβ conditions, respectively. Maximum GluN2B-NMDAR conductance was increased by a factor of 4 to account for AICD and AICD & Aβ effect, while it was maintained the same for the Aβ conditions.
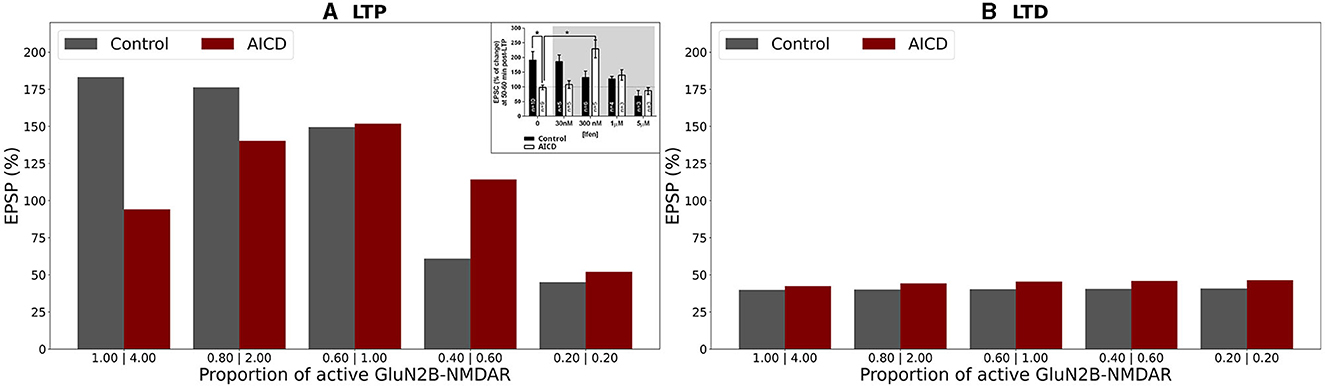
Figure 6. Effect of GluN2B-NMDAR blockade on somatic EPSP change in control and AICD conditions. GluN2B-NMDAR synaptic conductance was increased by a factor of 4 in AICD conditions. (A) LTP induction protocol. Presynaptic inputs were stimulated at 100 Hz for 1 s. Partial blockade of GluN2B-NMDAR leads to LTP impairment and prevention in control conditions (gray bars) and restores LTP in AICD conditions (red bars). Inset shows the experimental results modified from the study by Pousinha et al. (2017). Average LTP magnitude normalized to pre-LTP baseline values during 0–5 μM in vitro bath application of idenprodil in control conditions (black bars) and in AICD conditions (white bars). Courtesy of Pousinha et al. (2017). (B) LTD induction protocol. Presynaptic inputs were stimulated at 1 Hz for 500 s. Blockade of GluN2B-NMDAR does not affect LTD in control conditions (gray bars) and AICD conditions (red bars).
In the absence of AD peptides, the LTP induction protocol led to reduced somatic EPSP from 183% to 45% as the hypofunction in GluN2B-NMDAR increased, i.e., as the proportion of active GluN2B-NMDAR was lowered from 1 to 0.2 (Figure 6A, gray columns). Under AICD conditions, the bell-shape effect of partial GluN2B-NMDAR blockade was observed, where the optimal active proportion corresponded to the strongest LTP for high frequency stimulation protocol (Figure 6A, red columns). Specifically, partial blockade of the upregulated GluN2B-NMDAR channel and restoration of its active proportion to 1 instead of 4 (i.e., maintaining 0.25 fraction of its active elevated value) resulted in LTP of 152%. The proportions 2 and 0.6 (fractions 0.5 and 0.15) led to a weaker LTP of 140% and 114%, respectively, while the absence of GluN2B-NMDAR blockade or almost full inhibition of GluN2B-NMDAR (proportions 4 and 0.2, fractions 1 and 0.05) converted LTP to LTD of 94% and 45%, respectively. Partial blockade of GluN2B-NMDAR left LTD unaffected at 39%–41% in control conditions (Figure 6B, gray columns). The impairment of GluN2B-NMDAR functioning did not show such a strong non-linear effect on LTD leaving the EPSP change at 42%–46% (Figure 6B, red columns).
The modeling study effectively replicated the experimental findings on the impact of the GluN2B-NMDAR inhibitor ifenprodil on synaptic plasticity (Figure 6A inset; Pousinha et al., 2017). In control conditions, increasing ifenprodil concentration up to 1 μM reduced LTP, and 5 μM converted 190% LTP to 75% LTD for high-frequency stimulation. Ifenprodil application did not affect LTD for low-frequency stimulation. In AICD conditions, a bell-shape effect was observed for LTP induction protocol, and 300 nM of ifenprodil rescued LTP.
In the presence of oligomeric Aβ forms, optimal GluN2B-NMDAR blockade maintaining a proportion of 0.6 (or a fraction 0.6 of its active not-elevated value) restored LTP to 144% if compared to 105% for the fully functional GluN2B-NMDAR and high-frequency stimulation (Figure 7A, blue bars). Increased hypofunction of GluN2B-NMDAR led to the induction of LTD. The blockage of this receptor did not alter LTD for low frequency stimulation protocol (Figure 7B, blue bars). The results are consistent with the experimental work (Opazo et al., 2018) which demonstrated that oligomeric Aβ promoted CaMKII pre-activation and impairment of LTP. Blockade of GluN2B-NMDAR by ipenfrodil prevented CaMKII activation, and CaMKII inhibition was sufficient to rescue LTP.
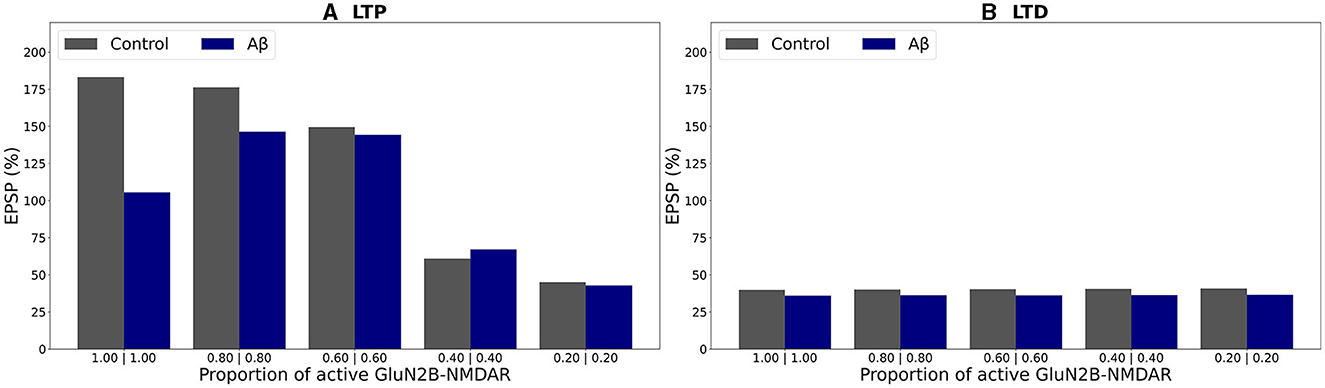
Figure 7. Effect of GluN2B-NMDAR blockade on somatic EPSP change in control and Aβ conditions. (A) LTP induction protocol. Presynaptic inputs were stimulated at 100 Hz for 1 s. Partial blockade of GluN2B-NMDAR leads to LTP impairment and prevention in control conditions (gray bars) and restores LTP in Aβ conditions (blue bars). (B) LTD induction protocol. Presynaptic inputs were stimulated at 1 Hz for 500 s. Blockade of GluN2B-NMDAR does not affect LTD in control conditions (gray bars) and Aβ conditions (blue bars).
In a similar manner, alterations in somatic EPSP appeared as a bell-shape curve, dependent on GluN2B-NMDAR function, when the concentrations of both AICD and Aβ were elevated (Figure 8A, violet bars). Optimal GluN2B-NMDAR blockade with 0.72 proportion (0.18 fraction of its active elevated value) restored LTP to 122 %, while the higher of lower levels of its activity failed to strengthen the synapses sufficiently. For low-frequency stimulation, LTD was not affected by the GluN2B-NMDAR hypofunction (Figure 8B, violet bars).
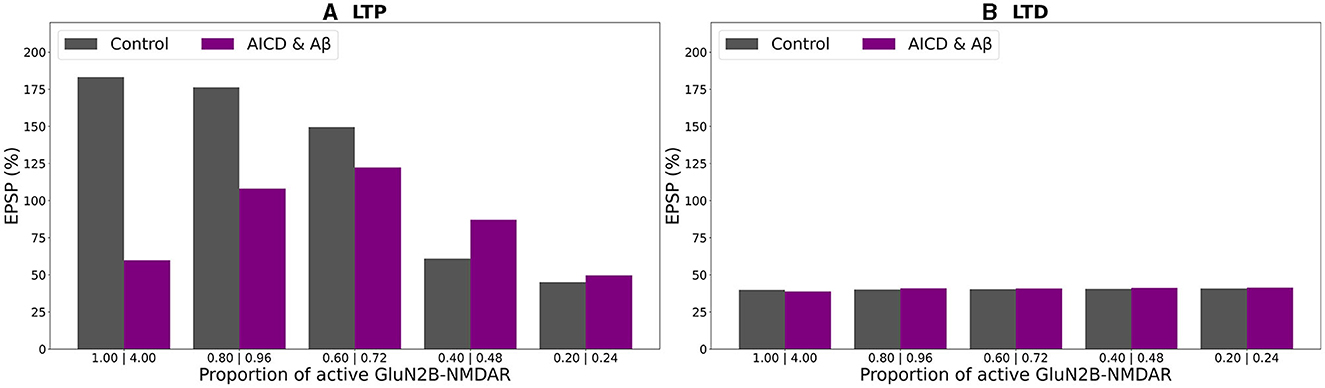
Figure 8. Effect of GluN2B-NMDAR blockade on somatic EPSP change in control and AICD & Aβ conditions. GluN2B-NMDAR synaptic conductance was increased by a factor of 4 in AICD & Aβ conditions. (A) LTP induction protocol. Presynaptic inputs were stimulated at 100 Hz for 1 s. Partial blockade of GluN2B-NMDAR leads to LTP impairment and prevention in control conditions (gray bars) and restores LTP in AICD & Aβ conditions (violet bars). (B) LTD induction protocol. Presynaptic inputs were stimulated at 1 Hz for 500 s. Blockade of GluN2B-NMDAR does not affect LTD in control conditions (gray bars) and AICD & Aβ conditions (violet bars).
Summary of the results is presented in Figure 9. In control conditions, LTP reached 183% for high frequency stimulation (Figure 9A, gray bar). Increased AICD levels led to a slight LTD of 94%, and GluN2B-NMDAR blockade to its active initial proportion 1 restored LTP to 152% (Figure 9A, red and light red bars). Oligomeric Aβ impaired LTP to 105% that was restored to 144% by the GluN2B-NMDAR blockade leaving 0.6 of its active proportion (Figure 9A, blue and light blue bars). For the elevated concentrations of both AICD and Aβ, somatic EPSP LTD to 60% was prevented and converted to LTP of 122% by the GluN2B-NMDAR blockade leading to 0.72 of its active proportion (Figure 9A, violet and pink bars).
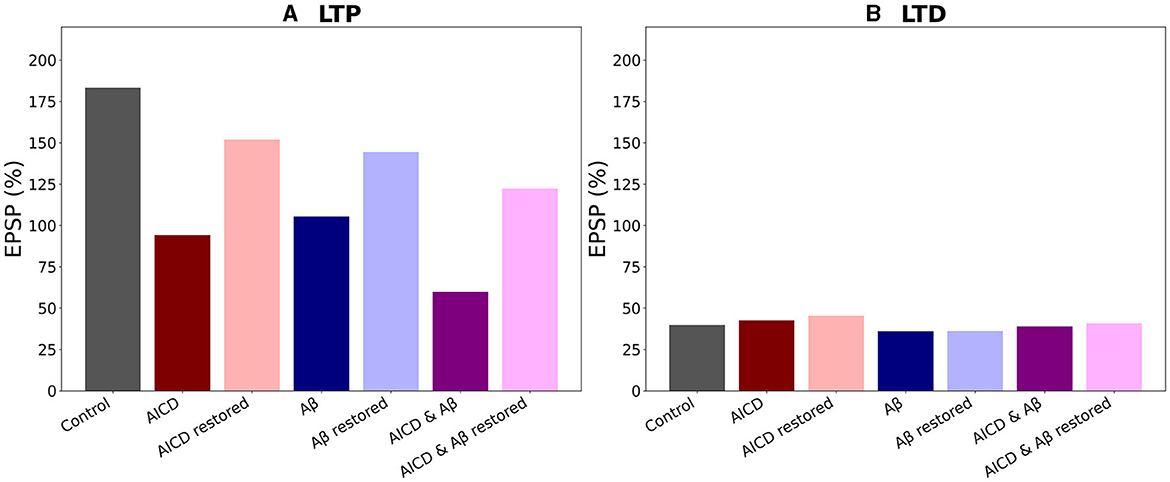
Figure 9. Optimal blockade of GluN2B-NMDAR restores synaptic functionality. (A) Somatic EPSP change for LTP induction protocol. GluN2B-NMDAR synaptic conductance was increased by a factor of 4 in AICD conditions and AICD conditions & Aβ conditions. Left to right columns: (Control) Somatic EPSP change is 183% (gray bar); (AICD) Somatic EPSP change is 94% (red bar); (AICD restored) Active GluN2B-NMDAR proportion is 1 of its elevated value of 4, i.e., the active fraction is 0.25; somatic EPSP change is 152% (light red bar); (Aβ) Somatic EPSP change is 105% (blue bar); (Aβ restored) Active GluN2B-NMDAR proportion is 0.6; somatic EPSP change is 144% (light blue bar); (AICD & Aβ) Somatic EPSP change is 60% (violet bar); (AICD & Aβ restored) Active GluN2B-NMDAR proportion is 0.72 of its elevated value of 4, i.e., the active fraction is 0.18; somatic EPSP change is 122% (pink bar). (B) Somatic EPSP change for LTD induction protocol. Left to right columns: (Control) Somatic EPSP change is 40% (gray bar); (AICD) Somatic EPSP change is 42% (red bar); (AICD restored) Active GluN2B-NMDAR proportion is 1 of its elevated value of 4, i.e., the active fraction is 0.25; somatic EPSP change is 45% (light red bar); (Aβ) Somatic EPSP change is 36% (blue bar); (Aβ restored) Active GluN2B-NMDAR proportion is 0.6; somatic EPSP change is 36% (light blue bar); (AICD & Aβ) Somatic EPSP change is 39% (violet bar); (AICD & Aβ restored) Active GluN2B-NMDAR proportion is 0.72 of its elevated value of 4, i.e., the active fraction is 0.18; somatic EPSP change is 40% (pink bar).
Taken together, the results obtained in this study indicated that optimal antagonism of GluN2B-NMDAR allowed to restore synaptic functionality in AD through normalizing intrinsic excitability and molecular pathways, responsible for LTP induction.
4 Discussion
We extended the Glu2NB-NMDAR and Glu2NA-NMDAR subunit-dependent voltage-based model of synaptic plasticity at hippocampal CA3-CA1 synapses (Dainauskas et al., 2023) to analyse the effects of elevated levels of two AID peptides, AICD and oligomeric Aβ, and modeled synaptic modifications in a cluster of synapses distributed on the proximal apical dendrites of a detailed compartmental model of a CA1 pyramidal neuron. We optimized the ion channels of the CA1 pyramidal neuron model to account for reduced intrinsic excitability in AICD conditions (Pousinha et al., 2019). We validated the models against the experimental data of synaptic plasticity impairment under the AICD and oligomeric Aβ conditions and in the presence of GluN2B-NMDAR blockade (Pousinha et al., 2017, 2019; Opazo et al., 2018). We confirmed that GluN2B-NMDAR dysfunction leads to LTP impairment and transformation to LTD in control conditions (Pousinha et al., 2017). We successfully replicated the bell-shape effect of GluN2B-NMDAR downregulation in the presence of the elevated AICD concentration for high-frequency stimulation and LTP rescue by the optimal blockade of GluN2B-NMDAR (Pousinha et al., 2017). We qualitatively reproduced experimental findings that GluN2B-NMDAR downregulation restores LTP in oligomeric Aβ conditions. Increased AICD levels enhanced intracellular Ca2+ concentration via GluN2B-NMDAR and L-type Ca2+ channels, which activated the nearby Ca2+-dependent K+ channels and led to hypoexcitability of a CA1 pyramidal neuron. Oligomeric forms of Aβ caused synapse loss and prevented CaMKII activation via Glu2NB-NMDAR-dependent pathway. Optimal blockade of GluN2B-NMDAR normalized Ca2+-dependent K+ channel functioning, restored excitability and allowed CaMKII to be phoshorylated to induce LTP.
We investigated the effect of simultaneous application of AICD and Aβ and showed that AICD-triggered hypoexcitability and Aβ-induced prevention of CaMKII activation can be averted by downregulation of Glu2NB-NMDAR functioning. Our modeling studies predict that AICD & Aβ -mediated impairment in LTP and LTD can be reversed by the optimal GluN2B-NMDAR antagonism. We demonstrated in a mechanistic way that impairment in synaptic plasticity in AD conditions depends on the interplay between upregulation of Glu2NB-NMDAR, Ca2+-dependent K+ channels CagK, L-type Ca2+ channels CaL, CaMKII inhibition, and synapse loss.
We showed that a reduction of Glu2NB-NMDAR conductance is sufficient to restore synaptic plasticity in AICD, Aβ, and AICD & Aβ conditions, despite the fact that ion channels, neurotransmitter release, and spine density were also affected by AD. Moreover, our model makes the experimentally testable prediction that lower or higher values of active Glu2NB-NMDAR conductance cannot result in LTP rescue. For AICD conditions, this optimal active fraction of Glu2NB-NMDAR corresponded to the initial value of Glu2NB-NMDAR in control condition. Reduced activity of the overexpressed Glu2NB-NMDAR prevented upregulation of Ca2+-dependent K+ channels CagK, restored excitability of CA1 pyramidal neuron, and led to the rescue of LTP. The model suggested that smaller values of active Glu2NB-NMDAR were not sufficient to trigger the LTP induction pathways, while large values resulted in the overactivation of CagK channels, insufficient membrane potential depolarization, and impaired LTP. For Aβ and AICD & Aβ conditions, the optimal active fraction of Glu2NB-NMDAR was lower than in control conditions. For increased oligomeric Aβ concentrations, the optimal active fraction of Glu2NB-NMDAR was equal to 0.6 if compared to the control conditions. The reason behind this effect was that normal functioning of Glu2NB-NMDAR overactivated CaMKII, the main protein in the LTP molecular pathway, and prevented LTP induction. Small active fraction of Glu2NB-NMDAR failed to sufficiently activate CaMKII and trigger LTP. For AICD & Aβ conditions, the optimal active fraction of Glu2NB-NMDAR was equal to 0.72 of its active value, if compared to the control conditions. In this case, block of Glu2NB-NMDAR overexpression prevented both upregulation of Ca2+-dependent K+ channels CagK and overactivation of CaMKII, and rescued LTP.
The modeling study links complex interactions among Glu2NB-NMDAR, excitability of CA1 pyramidal neuron, and LTP induction pathways, and allows to get insights into the mechanisms of LTP impairment and its rescue.
Our modeling study enables to analyse these processes and allows prediction of the joint effect of AD peptides and the influence of partial GluN2B-NMDAR blockade to restore the properties of synaptic plasticity.
Computational modeling offers a powerful technique to interpret the mechanisms of AD, including its pathophysiology, neurobiology, and potential interventions, and allows the integration of experimental findings at many different scales, from genes, molecules, and synapses up to neurons, networks, and the whole brain. Computational models of AD can be categorized into molecular and sub-cellular, single cell, neural circuits, and large-scale brain network models. Molecular and subcellular models of AD pathophysiology focus on detailed description of complex biochemical interactions of APP processing, Aβ, tau, tangles, inflammation, intracellular Ca2+ concentration, and a network of proteins and phosphatases responsible for LTP and LTD induction (Pallitto and Murphy, 2001; Proctor and Gray, 2010; Anastasio, 2011, 2013, 2014; Schmidt et al., 2012; De Caluwé and Dupont, 2013; Kyrtsos and Baras, 2013; Ortega et al., 2013; Proctor et al., 2013). At a single neuron level, detailed biophysical models of CA1 pyramidal and cortical neurons were employed to analyse the influence of Aβ on neuronal excitability (Morse et al., 2010; Culmone and Migliore, 2012; Romani et al., 2013). Neural circuit models of AD is another increasingly expanding class of studies representing specific brain networks affected by the disease, and typically focus on the altered synaptic function, activity of individual neurons, excitatory-inhibitory balance, and oscillations (Hasselmo, 1997; Bhattacharya et al., 2011; Zou et al., 2011; Abuhassan et al., 2012; Bianchi et al., 2014; Rowan et al., 2014; Bachmann et al., 2020; Li et al., 2020, 2023; Ness and Schultz, 2021). Large-scale brain network models simulate the development, manifestation, and progression of AD at a macroscopic level of brain regions connecting mean field models of neural activity to multimodal EEG, MEG, and fMRI data (Haan et al., 2017). Recently, a whole-brain simulation neuroinformatics platform The Virtual Brain (www.thevirtualbrain.org) has been developed, driven by the neural mass modeling approach and multi-modal structural, functional (MRI, diffusion MRI, and PET), neurophysiological (EEG and MEG), genetic, molecular, and cognitive data (Ritter et al., 2013; Spiegler and Jirsa, 2013; Zimmermann et al., 2018; Stefanovski et al., 2019, 2021).
Our modeling study falls into a class of single neuron models. Previous studies of hippocampal CA1 neuron properties in AD analyzed the effect of Aβ on the intrinsic excitability and short-term plasticity, influence of CREB on pattern storage and recall (Culmone and Migliore, 2012; Romani et al., 2013; Bianchi et al., 2014). At a single neuron level, Culmone and Migliore (2012) investigated the progressive effect of Aβ accumulation on membrane properties of hippocampal CA1 pyramidal neuron and showed that Aβ-mediated reduction of KA, KDR, and Na+ peak conductances in the sections of dendritic tree and decrease in synaptic conductances led to the decreased firing probability. Although basing the model on experimental data obtained with very high concentrations of Aβ (100 μM). The modeling study predicted that increase of KA or Na+ currents combined with a similar increase in synaptic conductance may restore spike probability of a CA1 neuron. Romani et al. (2013) implemented the Aβ-induced enhancement in the initial release probability at the CA3-CA1 synapses of the hippocampus and demonstrated that the altered synaptic short-term plasticity of the synapse favored synaptic depression over facilitation, and significantly modified synaptic integration properties. The potential pharmacological treatment was suggested as the increase in KA, Na+ conductances, and enhancement of AMPAR synaptic conductance as a result of CREB activity boosting.
We extended the studies on CA1 pyramidal neurons and analyzed the joint effect of AICD and Aβ on intrinsic excitability, and in particular, on synaptic plasticity in AD. We integrated the experimental findings on AICD and Aβ and presented prediction of the complex interaction effects of AD peptides and the influence of partial GluN2B-NMDAR blockade to normalize the properties of synaptic plasticity. The novelty of our study is the demonstration that modulation of GluN2B-NMDAR activity by its partial blockade has a potential to prevent Aβ—mediated synaptotoxicity and AICD-induced hypoexcitability, and restore synaptic plasticity in early AD. We aim to extend our study to modeling the storage and recall in hippocampal CA1 network under the influence of elevated concentrations of AD peptides. Hippocampal synaptic plasticity plays a critical role in memory formation and retrieval; therefore, mechanistic framework of complex processes facilitates understanding of cognitive impairment in neurodegenerative diseases and suggests potential targets to preserve and maintain brain network functionality.
Data availability statement
The datasets presented in this study can be found in online repositories. The names of the repository/repositories and accession number(s) can be found in the article/Supplementary material.
Author contributions
JD: Data curation, Formal analysis, Investigation, Methodology, Software, Visualization, Writing – review & editing. PV: Data curation, Investigation, Methodology, Software, Writing – review & editing. SM: Conceptualization, Investigation, Writing – review & editing. HM: Conceptualization, Data curation, Funding acquisition, Investigation, Methodology, Supervision, Writing – review & editing. MM: Conceptualization, Funding acquisition, Supervision, Writing – review & editing. AS: Conceptualization, Funding acquisition, Investigation, Methodology, Project administration, Resources, Supervision, Validation, Writing – original draft, Writing – review & editing.
Funding
The author(s) declare financial support was received for the research, authorship, and/or publication of this article. This research was funded by the Research Council of Lithuania, Agence Nationale de la Recherche (France) (Flagship ERA-NET Joint Transnational Call JTC 2019 in synergy with the Human Brain Project, No. S-FLAG-ERA-20-1/2020-PRO-28), the EU Horizon 2020 Framework Program for Research and Innovation (Specific Grant 945539, Human Brain Project SGA3), the Fenix computing and storage resources was provided under Specific Grant Agreement No. 800858 (Human Brain Project ICEI), and a grant from the Swiss National Supercomputing Center (CSCS) under project ID ich011. MM also acknowledges administrative and technical assistance from Alessia Bonafede and a contribution from the Italian National Recovery and Resilience Plan (NRRP), M4C2 and funded by the European Union - NextGenerationEU (Project IR0000011, CUP B51E22000150006, EBRAINS-Italy).
Conflict of interest
The authors declare that the research was conducted in the absence of any commercial or financial relationships that could be construed as a potential conflict of interest.
Publisher's note
All claims expressed in this article are solely those of the authors and do not necessarily represent those of their affiliated organizations, or those of the publisher, the editors and the reviewers. Any product that may be evaluated in this article, or claim that may be made by its manufacturer, is not guaranteed or endorsed by the publisher.
Supplementary material
The Supplementary Material for this article can be found online at: https://www.frontiersin.org/articles/10.3389/fncom.2023.1305169/full#supplementary-material
References
Abramov, E., Dolev, I., Fogel, H., Ciccotosto, G. D., Ruff, E., and Slutsky, I. (2009). Amyloid-beta as a positive endogenous regulator of release probability at hippocampal synapses. Nat. Neurosci. 12, 1567–1576. doi: 10.1038/nn.2433
Abuhassan, K., Coyle, D., and Maguire, L. P. (2012). Investigating the neural correlates of pathological cortical networks in Alzheimer's disease using heterogeneous neuronal models. IEEE Trans. Bio-Med. Eng. 59, 890–896. doi: 10.1109/TBME.2011.2181843
Anastasio, T. J. (2011). Data-driven modeling of Alzheimer disease pathogenesis. J. Theor. Biol. 290, 60–72. doi: 10.1016/j.jtbi.2011.08.038
Anastasio, T. J. (2013). Exploring the contribution of estrogen to amyloid-β regulation: a novel multifactorial computational modeling approach. Front. Pharmacol. 4:16. doi: 10.3389/fphar.2013.00016
Anastasio, T. J. (2014). Computational identification of potential multitarget treatments for ameliorating the adverse effects of amyloid-β on synaptic plasticity. Front. Pharmacol. 5:85. doi: 10.3389/fphar.2014.00085
Andrade-Talavera, Y., Duque-Feria, P., Paulsen, O., and Rodríguez-Moreno, A. (2016). Presynaptic spike timing-dependent long-term depression in the mouse hippocampus. Cereb. Cortex 26, 3637–3654. doi: 10.1093/cercor/bhw172
Bachmann, C., Tetzlaff, T., Duarte, R., and Morrison, A. (2020). Firing rate homeostasis counteracts changes in stability of recurrent neural networks caused by synapse loss in Alzheimer's disease. PLoS Comput. Biol. 16:e1007790. doi: 10.1371/journal.pcbi.1007790
Bezaire, M. J., Raikov, I., Burk, K., Vyas, D., and Soltesz, I. (2016). Interneuronal mechanisms of hippocampal theta oscillations in a full-scale model of the rodent CA1 circuit. eLife 5:e18566. doi: 10.7554/eLife.18566
Bhattacharya, B. S., Coyle, D., and Maguire, L. P. (2011). Alpha and theta rhythm abnormality in Alzheimer's Disease: a study using a computational model. Adv. Exp. Med. Biol. 718, 57–73. doi: 10.1007/978-1-4614-0164-3_6
Bianchi, D., De Michele, P., Marchetti, C., Tirozzi, B., Cuomo, S., Marie, H., et al. (2014). Effects of increasing CREB-dependent transcription on the storage and recall processes in a hippocampal CA1 microcircuit. Hippocampus 24, 165–177. doi: 10.1002/hipo.22212
Culmone, V., and Migliore, M. (2012). Progressive effect of beta amyloid peptides accumulation on CA1 pyramidal neurons: a model study suggesting possible treatments. Front. Comput. Neurosci. 6:52. doi: 10.3389/fncom.2012.00052
Dainauskas, J. J., Marie, H., Migliore, M., and Saudargiene, A. (2023). GluN2B-NMDAR subunit contribution on synaptic plasticity: a phenomenological model for CA3-CA1 synapses. Front. Synapt. Neurosci. 15:1113957. doi: 10.3389/fnsyn.2023.1113957
De Caluwé, J., and Dupont, G. (2013). The progression towards Alzheimer's disease described as a bistable switch arising from the positive loop between amyloids and Ca(2+). J. Theor. Biol. 331, 12–18. doi: 10.1016/j.jtbi.2013.04.015
Gasparini, S., Migliore, M., and Magee, J. C. (2004). On the initiation and propagation of dendritic spikes in CA1 pyramidal neurons. J. Neurosci. 24, 11046–11056. doi: 10.1523/JNEUROSCI.2520-04.2004
Ghosal, K., Vogt, D. L., Liang, M., Shen, Y., Lamb, B. T., and Pimplikar, S. W. (2009). Alzheimer's disease-like pathological features in transgenic mice expressing the APP intracellular domain. Proc. Natl. Acad. Sci. U.S.A. 106, 18367–18372. doi: 10.1073/pnas.0907652106
Haan, W. d., Straaten, E. C. W., Gouw, A. A., and Stam, C. J. (2017). Altering neuronal excitability to preserve network connectivity in a computational model of Alzheimer's disease. PLOS Comput. Biol. 13:e1005707. doi: 10.1371/journal.pcbi.1005707
Haass, C., and Selkoe, D. J. (2007). Soluble protein oligomers in neurodegeneration: lessons from the Alzheimer's amyloid β-peptide. Nat. Rev. Mol. Cell Biol. 8, 101–112. doi: 10.1038/nrm2101
Hasselmo, M. E. (1997). A computational model of the progression of Alzheimer's disease. Computing 14, 181–191.
Hines, M. L., and Carnevale, N. T. (1997). The NEURON simulation environment. Neural Comput. 9, 1179–1209. doi: 10.1162/neco.1997.9.6.1179
Hoffman, D. A., and Johnston, D. (1999). Neuromodulation of dendritic action potentials. J. Neurophysiol. 81, 408–411. doi: 10.1152/jn.1999.81.1.408
Kumar, A., and Foster, T. C. (2002). 17beta-estradiol benzoate decreases the AHP amplitude in CA1 pyramidal neurons. J. Neurophysiol. 88, 621–626. doi: 10.1152/jn.2002.88.2.621
Kyrtsos, C. R., and Baras, J. S. (2013). Studying the role of ApoE in Alzheimer's disease pathogenesis using a systems biology model. J. Bioinform. Comput. Biol. 11:1342003. doi: 10.1142/S0219720013420031
Li, K. T., Ji, D., and Zhou, C. (2023). Memory rescue and learning in synaptic impaired neuronal circuits. iScience 26:106931. doi: 10.1016/j.isci.2023.106931
Li, X., Yang, X., and Sun, Z. (2020). Alpha rhythm slowing in a modified thalamo-cortico-thalamic model related with Alzheimer?s disease. PLoS ONE 15:e0229950. doi: 10.1371/journal.pone.0229950
Migliore, R., Lupascu, C. A., Bologna, L. L., Romani, A., Courcol, J.-D., Antonel, S., et al. (2018). The physiological variability of channel density in hippocampal CA1 pyramidal cells and interneurons explored using a unified data-driven modeling workflow. PLoS Comput. Biol. 14:e1006423. doi: 10.1371/journal.pcbi.1006423
Morishita, W., Lu, W., Smith, G. B., Nicoll, R. A., Bear, M. F., and Malenka, R. C. (2007). Activation of NR2B-containing NMDA receptors is not required for NMDA receptor-dependent long-term depression. Neuropharmacology 52, 71–76. doi: 10.1016/j.neuropharm.2006.07.005
Morse, T., Carnevale, N., Mutalik, P., Migliore, M., and Shepherd, G. (2010). Abnormal excitability of oblique dendrites implicated in early Alzheimer's: a computational study. Front. Neural Circuits 4:16. doi: 10.3389/fncir.2010.00016
Ness, N., and Schultz, S. R. (2021). A computational grid-to-place-cell transformation model indicates a synaptic driver of place cell impairment in early-stage Alzheimer's Disease. PLoS Comput. Biol. 17:e1009115. doi: 10.1371/journal.pcbi.1009115
Opazo, P., Viana da Silva, S., Carta, M., Breillat, C., Coultrap, S. J., Grillo-Bosch, D., et al. (2018). CaMKII metaplasticity drives Aβ oligomer-mediated synaptotoxicity. Cell Rep. 23, 3137–3145. doi: 10.1016/j.celrep.2018.05.036
Ortega, F., Stott, J., Visser, S. A. G., and Bendtsen, C. (2013). Interplay between α, β, and γ-secretases determines biphasic amyloid-β protein level in the presence of a γ-secretase inhibitor. J. Biol. Chem. 288, 785–792. doi: 10.1074/jbc.M112.419135
Pallitto, M. M., and Murphy, R. M. (2001). A mathematical model of the kinetics of beta-amyloid fibril growth from the denatured state. Biophys. J. 81, 1805–1822. doi: 10.1016/S0006-3495(01)75831-6
Park, P., Georgiou, J., Sanderson, T. M., Ko, K.-H., Kang, H., Kim, J.-I., et al. (2021). PKA drives an increase in AMPA receptor unitary conductance during LTP in the hippocampus. Nat. Commun. 12:413. doi: 10.1038/s41467-020-20523-3
Peng, Y., Lu, Z., Li, G., Piechowicz, M., Anderson, M., Uddin, Y., et al. (2016). The autism-associated MET receptor tyrosine kinase engages early neuronal growth mechanism and controls glutamatergic circuits development in the forebrain. Mol. Psychiatry 21, 925–935. doi: 10.1038/mp.2015.182
Pousinha, P. A., Mouska, X., Bianchi, D., Temido-Ferreira, M., Raj ao-Saraiva, J., Gomes, R., et al. (2019). The amyloid precursor protein C-terminal domain alters CA1 neuron firing, modifying hippocampus oscillations and impairing spatial memory encoding. Cell Rep. 29, 317–331.e5. doi: 10.1016/j.celrep.2019.08.103
Pousinha, P. A., Mouska, X., Raymond, E. F., Gwizdek, C., Dhib, G., Poupon, G., et al. (2017). Physiological and pathophysiological control of synaptic GluN2B-NMDA receptors by the C-terminal domain of amyloid precursor protein. eLife 6:e25659. doi: 10.7554/eLife.25659
Proctor, C. J., Boche, D., Gray, D. A., and Nicoll, J. A. R. (2013). Investigating interventions in Alzheimer's disease with computer simulation models. PLoS ONE 8:e73631. doi: 10.1371/journal.pone.0073631
Proctor, C. J., and Gray, D. A. (2010). GSK3 and p53 - is there a link in Alzheimer's disease? Mol. Neurodegen. 5:7. doi: 10.1186/1750-1326-5-7
Rajão-Saraiva, J., Dunot, J., Ribera, A., Temido-Ferreira, M., Coelho, J. E., König, S., et al. (2023). Age-dependent NMDA receptor function is regulated by the amyloid precursor protein. Aging Cell 22:e13778. doi: 10.1111/acel.13778
Ritter, P., Schirner, M., McIntosh, A. R., and Jirsa, V. K. (2013). The virtual brain integrates computational modeling and multimodal neuroimaging. Brain Connect. 3, 121–145. doi: 10.1089/brain.2012.0120
Romani, A., Marchetti, C., Bianchi, D., Leinekugel, X., Poirazi, P., Migliore, M., et al. (2013). Computational modeling of the effects of amyloid-β on release probability at hippocampal synapses. Front. Comput. Neurosci. 7:1. doi: 10.3389/fncom.2013.00001
Rönicke, R., Mikhaylova, M., Rönicke, S., Meinhardt, J., Schröder, U. H., Fändrich, M., et al. (2011). Early neuronal dysfunction by amyloid β oligomers depends on activation of NR2B-containing NMDA receptors. Neurobiol. Aging 32, 2219–2228. doi: 10.1016/j.neurobiolaging.2010.01.011
Rowan, M. S., Neymotin, S. A., and Lytton, W. W. (2014). Electrostimulation to reduce synaptic scaling driven progression of Alzheimer's disease. Front. Comput. Neurosci. 8:39. doi: 10.3389/fncom.2014.00039
Schmidt, V., Baum, K., Lao, A., Rateitschak, K., Schmitz, Y., Teichmann, A., et al. (2012). Quantitative modelling of amyloidogenic processing and its influence by SORLA in Alzheimer's disease. EMBO J. 31, 187–200. doi: 10.1038/emboj.2011.352
Shipton, O. A., and Paulsen, O. (2014). GluN2A and GluN2B subunit-containing NMDA receptors in hippocampal plasticity. Philos. Trans. R. Soc. Lond. Ser. B Biol. Sci. 369:20130163. doi: 10.1098/rstb.2013.0163
Spiegler, A., and Jirsa, V. (2013). Systematic approximations of neural fields through networks of neural masses in the virtual brain. NeuroImage 83, 704–725. doi: 10.1016/j.neuroimage.2013.06.018
Stefanovski, L., Meier, J. M., Pai, R. K., Triebkorn, P., Lett, T., Martin, L., et al. (2021). Bridging scales in Alzheimer's disease: biological framework for brain simulation with the virtual brain. Front. Neuroinform. 15:630172. doi: 10.3389/fninf.2021.630172
Stefanovski, L., Triebkorn, P., Spiegler, A., Diaz-Cortes, M.-A., Solodkin, A., Jirsa, V., et al. (2019). Linking molecular pathways and large-scale computational modeling to assess candidate disease mechanisms and pharmacodynamics in Alzheimer's disease. Front. Comput. Neurosci. 13:54. doi: 10.3389/fncom.2019.00054
Tamagnini, F., Scullion, S., Brown, J. T., and Randall, A. D. (2015). Intrinsic excitability changes induced by acute treatment of hippocampal CA1 pyramidal neurons with exogenous amyloid β peptide. Hippocampus 25, 786–797. doi: 10.1002/hipo.22403
Taylor, H. B. C., Emptage, N. J., and Jeans, A. F. (2021). Long-term depression links amyloid-β to the pathological hyperphosphorylation of tau. Cell Rep. 36:109638. doi: 10.1016/j.celrep.2021.109638
Van Geit, W., Gevaert, M., Chindemi, G., Rössert, C., Courcol, J.-D., Muller, E. B., et al. (2016). BluePyOpt: leveraging open source software and cloud infrastructure to optimise model parameters in neuroscience. Front. Neuroinform. 10:17. doi: 10.3389/fninf.2016.00017
Yasuda, R., Hayashi, Y., and Hell, J. W. (2022). CaMKII: a central molecular organizer of synaptic plasticity, learning and memory. Nat. Rev. Neurosci. 23, 666–682. doi: 10.1038/s41583-022-00624-2
Zimmermann, J., Perry, A., Breakspear, M., Schirner, M., Sachdev, P., Wen, W., et al. (2018). Differentiation of Alzheimer's disease based on local and global parameters in personalized Virtual Brain models. NeuroImage 19, 240–251. doi: 10.1016/j.nicl.2018.04.017
Keywords: Alzheimer's disease, amyloid precursor protein intracellular domain, amyloid beta, synaptic plasticity, NMDA receptor, GluN2B-NMDA receptor subunit, Schaffer collateral synapses, CA1 pyramidal neuron
Citation: Dainauskas JJ, Vitale P, Moreno S, Marie H, Migliore M and Saudargiene A (2023) Altered synaptic plasticity at hippocampal CA1–CA3 synapses in Alzheimer's disease: integration of amyloid precursor protein intracellular domain and amyloid beta effects into computational models. Front. Comput. Neurosci. 17:1305169. doi: 10.3389/fncom.2023.1305169
Received: 30 September 2023; Accepted: 07 November 2023;
Published: 07 December 2023.
Edited by:
Nicolangelo Iannella, University of Oslo, NorwayReviewed by:
Fidel Santamaria, University of Texas at San Antonio, United StatesLubica Benuskova, Comenius University, Slovakia
Copyright © 2023 Dainauskas, Vitale, Moreno, Marie, Migliore and Saudargiene. This is an open-access article distributed under the terms of the Creative Commons Attribution License (CC BY). The use, distribution or reproduction in other forums is permitted, provided the original author(s) and the copyright owner(s) are credited and that the original publication in this journal is cited, in accordance with accepted academic practice. No use, distribution or reproduction is permitted which does not comply with these terms.
*Correspondence: Ausra Saudargiene, YXVzcmEuc2F1ZGFyZ2llbmVAbHNtdS5sdA==