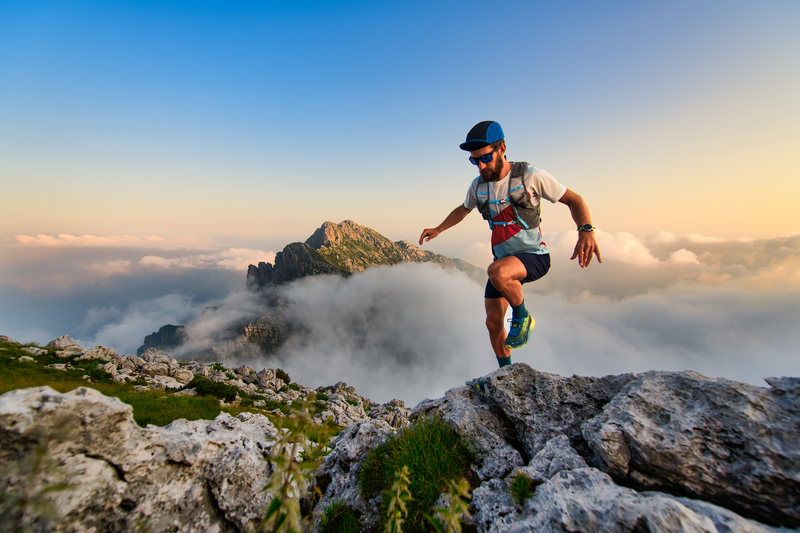
94% of researchers rate our articles as excellent or good
Learn more about the work of our research integrity team to safeguard the quality of each article we publish.
Find out more
ERRATUM article
Front. Comput. Neurosci. , 01 September 2023
Volume 17 - 2023 | https://doi.org/10.3389/fncom.2023.1282093
This article is part of the Research Topic Symmetry as a Guiding Principle in Artificial and Brain Neural Networks, Volume II View all 7 articles
This article is an erratum on:
Covariance properties under natural image transformations for the generalised Gaussian derivative model for visual receptive fields
by Lindeberg, T. (2023). Front. Comput. Neurosci. 17:1189949. doi: 10.3389/fncom.2023.1189949
Due to a production error, there was a mistake in Footnote 2 in the HTML version of the article. A correction has been made to the mathematical expression. The corrected footnote appears below.
2. In the deep learning literature, the property that we refer to as “covariance” is often referred to as “equivariance.” In this paper, we use the term “covariance” because of the traditional use of this terminology in physics, and to maintain consistency with the previous work in scale-space theory that this paper builds upon. An operator O is said to be covariant under a transformation group Tp with parameter p, if the operator essentially commutes with the transformation group, in the sense that O'(Tp(f)) = Tp(Of) for some possibly transformed operator O' within the same family of operators as O.
The publisher apologizes for this mistake. The original article has been updated.
Keywords: receptive field, Image transformations, scale covariance, affine covariance, Galilean covariance, primary visual cortex, vision, theoretical neuroscience
Citation: Frontiers Production Office (2023) Erratum: Covariance properties under natural image transformations for the generalised Gaussian derivative model for visual receptive fields. Front. Comput. Neurosci. 17:1282093. doi: 10.3389/fncom.2023.1282093
Received: 23 August 2023; Accepted: 23 August 2023;
Published: 01 September 2023.
Approved by:
Frontiers Editorial Office, Frontiers Media SA, SwitzerlandCopyright © 2023 Frontiers Production Office. This is an open-access article distributed under the terms of the Creative Commons Attribution License (CC BY). The use, distribution or reproduction in other forums is permitted, provided the original author(s) and the copyright owner(s) are credited and that the original publication in this journal is cited, in accordance with accepted academic practice. No use, distribution or reproduction is permitted which does not comply with these terms.
*Correspondence: Frontiers Production Office, cHJvZHVjdGlvbi5vZmZpY2VAZnJvbnRpZXJzaW4ub3Jn
Disclaimer: All claims expressed in this article are solely those of the authors and do not necessarily represent those of their affiliated organizations, or those of the publisher, the editors and the reviewers. Any product that may be evaluated in this article or claim that may be made by its manufacturer is not guaranteed or endorsed by the publisher.
Research integrity at Frontiers
Learn more about the work of our research integrity team to safeguard the quality of each article we publish.