- 1Department of Communication Science and Disorders, University of Pittsburgh, Pittsburgh, PA, United States
- 2Center for Education in Health Sciences, Northwestern University, Chicago, IL, United States
- 3Department of Speech, Language, and Hearing Sciences, The University of Texas at Austin, Austin, TX, United States
- 4Department of Communication Sciences and Disorders, University of Houston, Houston, TX, United States
This mini review is aimed at a clinician-scientist seeking to understand the role of oscillations in neural processing and their functional relevance in speech and music perception. We present an overview of neural oscillations, methods used to study them, and their functional relevance with respect to music processing, aging, hearing loss, and disorders affecting speech and language. We first review the oscillatory frequency bands and their associations with speech and music processing. Next we describe commonly used metrics for quantifying neural oscillations, briefly touching upon the still-debated mechanisms underpinning oscillatory alignment. Following this, we highlight key findings from research on neural oscillations in speech and music perception, as well as contributions of this work to our understanding of disordered perception in clinical populations. Finally, we conclude with a look toward the future of oscillatory research in speech and music perception, including promising methods and potential avenues for future work. We note that the intention of this mini review is not to systematically review all literature on cortical tracking of speech and music. Rather, we seek to provide the clinician-scientist with foundational information that can be used to evaluate and design research studies targeting the functional role of oscillations in speech and music processing in typical and clinical populations.
Introduction
The past decade has seen a surge in research on the role of neural oscillations in sensory processing. Neural oscillations are self-sustained rhythmic activity of neural populations that occur at multiple time scales, are generally observed in local field potentials, and may modulate the spiking activity of single neurons (Howard and Poeppel, 2010; Giraud and Poeppel, 2012). They can be seen at rest, and changes in their power and phase can be elicited by external (e.g., sensory stimulation) and internal factors (e.g., self-initiated movements, mind-wandering). Recent research demonstrated that neural oscillations can reliably track ongoing changes in a stimulus (for review, see Haegens and Zion Golumbic, 2018; Meyer, 2018; Myers et al., 2019; Obleser and Kayser, 2019). Consequently, it has been purported that they play an important role in speech and music perception. In this mini-review, we address three main points: (1) oscillatory frequency bands and their functional role, (2) analysis metrics used to study neural oscillations, and (3) the functional relevance of neural oscillations in aging, hearing loss, disorders affecting speech and language (Palana et al., 2022), and music processing. Lastly, we discuss promising methods and future directions for studying neural oscillations.
Oscillatory Frequency Bands and Their Purported Functional Roles in Speech and Music Processing
Neural oscillations can be observed using a variety of electrophysiological methods with millisecond temporal precision [electroencephalography (EEG), electrocorticography, and magnetoencephalography (MEG)]. Most auditory research on neural oscillations takes advantage of the non-invasive nature of M/EEG to investigate how well neural oscillations align in phase or power with acoustic and linguistic rhythms in speech. This is often referred to as tracking or entrainment, although the use of these terms is debated (e.g., Obleser and Kayser, 2019; Meyer et al., 2020a,b).
It is unclear whether oscillatory alignment is a result of (1) the summation of delayed, passive, transient, evoked responses to rhythmic stimulus events; (2) active, intrinsic brain oscillations aligning to rhythmic stimulus events; or (3) both (Haegens and Zion Golumbic, 2018; Rimmele et al., 2018; Coffey et al., 2021; cf. Doelling et al., 2019; Zou et al., 2021). In the auditory domain, the mechanistic role of neural oscillations is the subject of ongoing investigation, especially regarding whether oscillations are an emergent property of the auditory system (evoked) vs. an inherent part of that system (intrinsic). A summary of evidence for evoked vs. intrinsic accounts of oscillatory alignment is beyond the scope of this mini-review; we refer the reader to numerous papers on this topic (Haegens and Zion Golumbic, 2018; Lakatos et al., 2019; Poeppel and Assaneo, 2020; cf., Doelling and Assaneo, 2021).
Neural oscillations are typically grouped into frequency bands. These bands arguably play a role in encoding acoustic and linguistic information that unfolds across timescales equivalent to the frequency of the oscillations (Ding et al., 2016; Meyer, 2018; Myers et al., 2019). The slower bands are more engaged in processing information that unfolds across longer periods of time, whereas the faster bands are more engaged for rapidly unfolding information. The delta band (0.5–4 Hz) is thought to encode words, syntactic structures, and prosodic cues in speech and music (Ghitza, 2017; Meyer et al., 2017, 2020a; Keitel et al., 2018; Teoh et al., 2019; Rimmele et al., 2021). The theta band (4–8 Hz) oscillates at a similar rate as syllable production and has been implicated in syllabic processing (Ghitza, 2013; Poeppel and Assaneo, 2020). The alpha (8–12 Hz) and beta (12–25 Hz) bands have been implicated in attention (Wöstmann et al., 2017) and auditory-motor coupling (Fujioka et al., 2012), respectively. The gamma band (25–140 Hz) is hypothesized to encode rapid fluctuations in the auditory signal and be critical for encoding phonetic features (Masuda and Doiron, 2007; Giraud and Poeppel, 2012). Whereas gamma is posited to reflect more bottom-up, lower-level processing of acoustic and phonetic structures in speech, delta and theta may reflect the synthesis of higher auditory and linguistic objects and may modulate gamma activity (Hyafil et al., 2015b). Researchers have proposed a theta-gamma coupling mechanism, with theta oscillations tracking the syllabic structure of speech and providing a temporal frame to group phonetic features encoded by gamma oscillations (Hyafil et al., 2015a; Lizarazu et al., 2019; Hovsepyan et al., 2020).
Analysis Metrics for Investigating Neural Oscillations
A variety of metrics have been used to infer the role of neural oscillations in speech and music processing, including (but not limited to) cross-correlation (Ahissar et al., 2001), multivariate temporal response functions (Crosse et al., 2016), mutual information (Nelken and Chechik, 2007), inter-trial phase coherence (Rimmele et al., 2015), cerebro-acoustic coherence (Peelle et al., 2013), and cross-frequency coupling (Hovsepyan et al., 2020; Figure 1 and Table 1). These metrics convey how well rhythms are tracked by neural oscillations, providing different yet complementary insights into underlying neural mechanisms across frequency bands, acoustic and linguistic rhythms, and brain regions of interest (e.g., cortical vs. subcortical, sensory vs. motor).
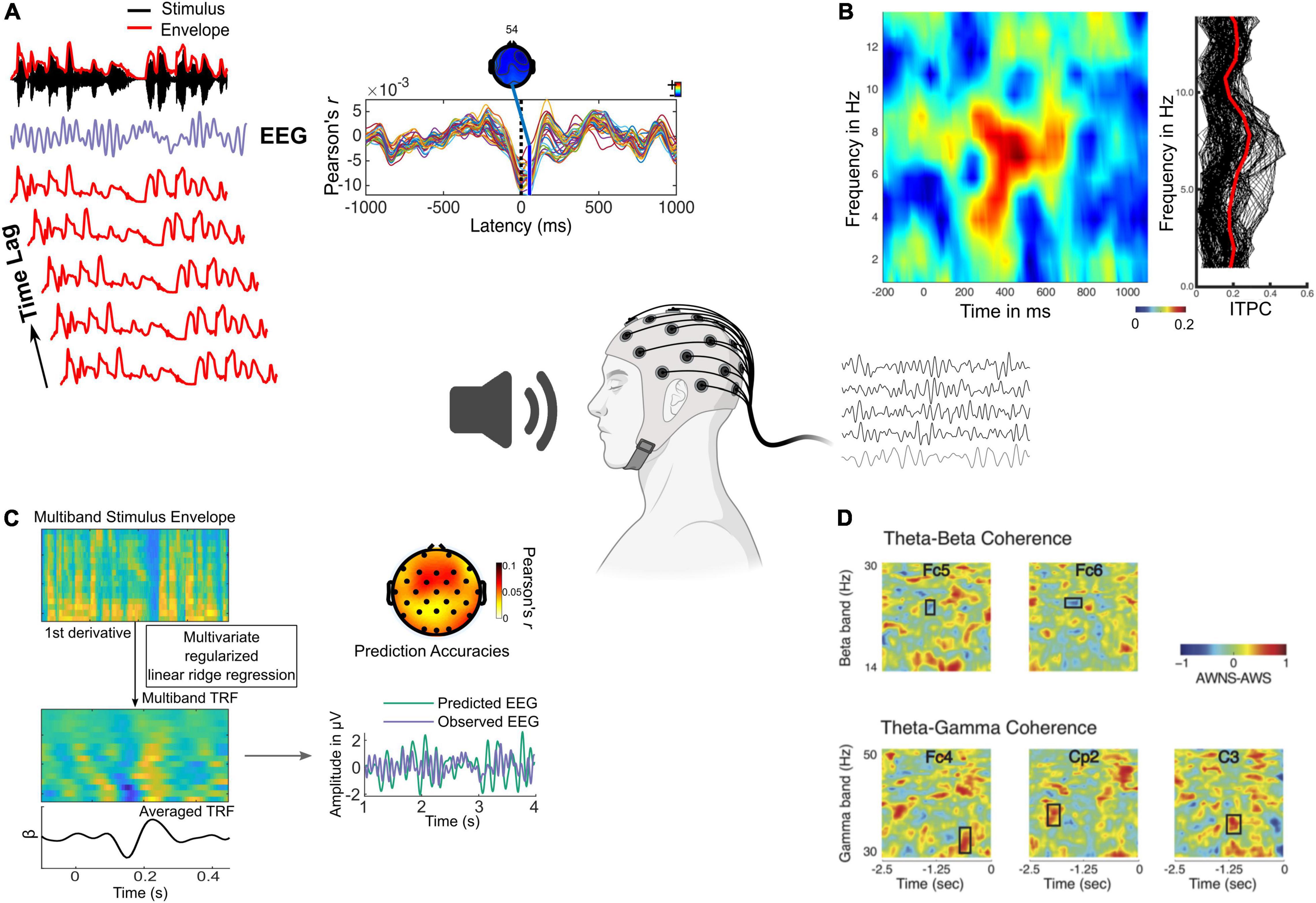
Figure 1. Different analysis metrics used to investigate oscillatory contributions to speech and music perception. (A) Cross correlation between stimulus envelope (lag time series) and EEG extracted for sentences. (B) Left panel shows time-frequency representation of inter-trial phase coherence for a 6 Hz modulated tone showing stronger values corresponding to the modulation frequency. Right panel shows single trial phase values collapsed across time. (C) Multivariate TRF modeling of multiband stimulus envelope to map a kernel function onto the EEG. The observed EEG, multivariate TRF predicted EEG (at a single electrode) and their correlation at each electrode is shown (adapted from Dial et al., 2021). (D) Time-frequency representation of cross frequency coupling showing phase amplitude coherence between the theta phase and beta power (above), and theta phase and gamma power (below) in adults who do (AWS) and do not stutter (AWNS) (Sengupta et al., 2019).
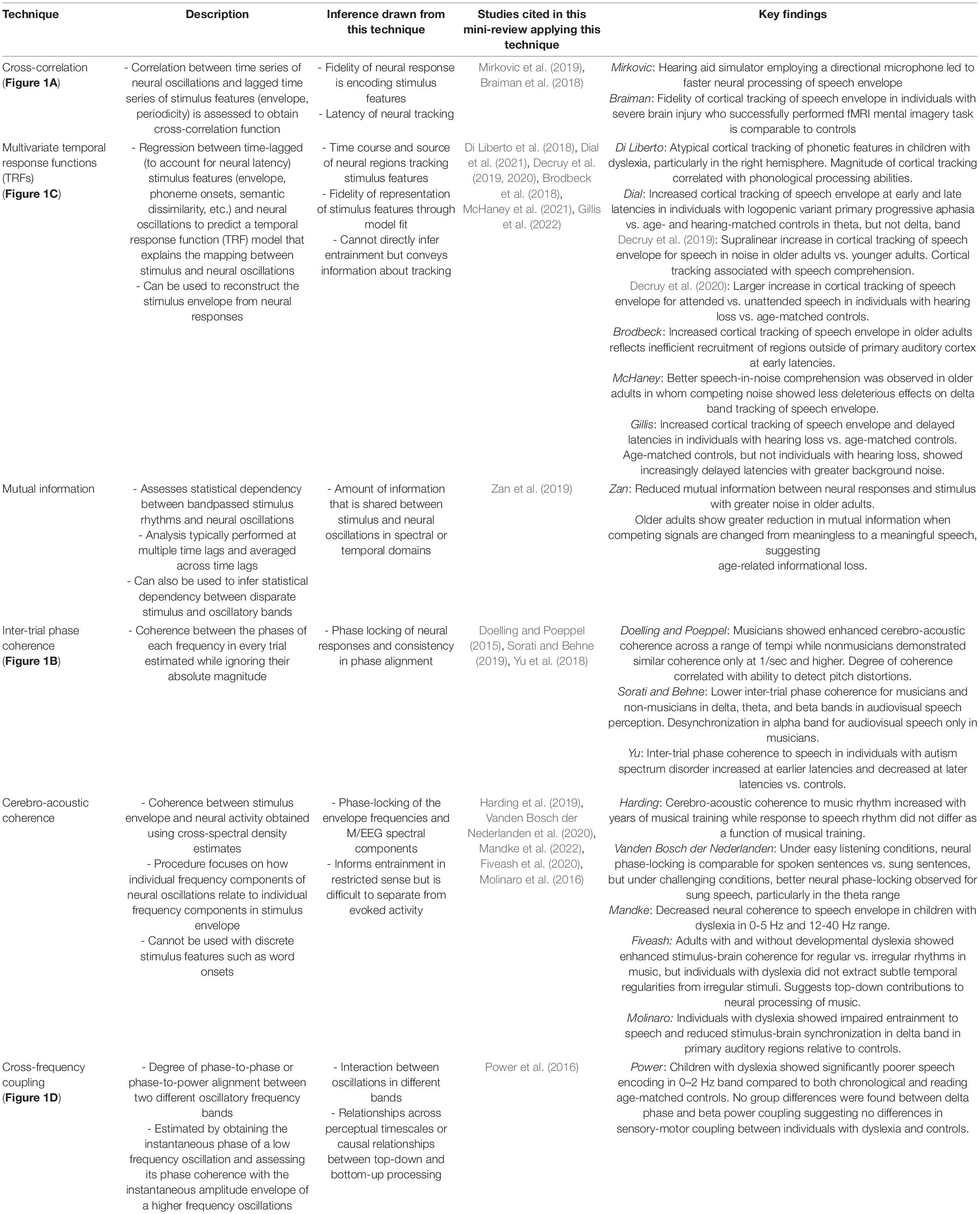
Table 1. Overview of analysis techniques used to study the role of neural oscillations in speech and music processing, the possible inferences that can be drawn when utilizing each technique, and the references in the present mini-review that applied this technique in older adults, clinical populations or music processing (see in-text references for additional context regarding each study).
Oscillations in Speech and Music Processing
Neural oscillations can be observed in response to both speech and music. Recent advances in computational modeling have enabled new insights into the mechanisms underlying auditory neural processing. Doelling et al. (2019) modeled MEG responses to music of varying rates, providing evidence for a combination of evoked and intrinsic mechanisms supporting neural processing of musical stimuli. Zou et al. (2021) subsequently utilized this approach with EEG responses to Mandarin narratives. They observed a linear change in phase lag between cortical activity and the speech envelope across frequency bands, suggesting that these oscillations could be modeled as evoked responses. Neural responses to speech and music may thus reflect both evoked and intrinsic oscillatory alignment, depending on the timescale of the stimulus and the oscillatory frequencies of interest. This is of particular interest in music, where the incoming stimulus is generally more isochronous than in speech (Peelle and Davis, 2012; Nolan and Jeon, 2014; cf. Jadoul et al., 2016). However, there is also considerable variability across styles of music and languages [e.g., syllable-timed languages (Spanish) feature a more isochronous rhythm than stress-timed languages (English, Mandarin); Pike, 1945; cf. Grabe and Low, 2002].
These computational findings add to a growing body of research supporting a role for oscillatory alignment in music processing. Doelling and Poeppel (2015) showed that musicians may have enhanced oscillatory alignment in response to musical rhythms, indicating higher perceptual acuity to subtle spectrotemporal variations. Fujioka et al. (2012) investigated whether MEG beta band oscillations (∼20 Hz) show power and phase coherence to auditory rhythms (i.e., musical beats) across auditory and motor neural systems. Their results suggest that oscillations reflect functional coordination across these systems, referred to as auditory-motor coupling. Fujioka et al. (2015) replicated and extended prior findings specifying that beat encoding by beta band oscillations was influenced by metrical structure (i.e., 4/4 or 12/8 time). Thus, auditory-motor coupling driven by beta oscillations provides an explanation as to why many find it difficult to resist tapping along to one’s favorite song. Further, tests of auditory-motor synchronization to speech rhythm differentiates participants into low and high “synchronizers” (Lizcano-Cortés et al., 2022). However, the precise basis of these distinct behavioral phenotypes have yet to be explored and are a promising avenue for future research in neurotypical and clinical populations.
Harding et al. (2019) studied oscillatory tracking of speech and music using matched stimulus rhythms. They found that individuals with extensive music training showed increased cortical tracking for music vs. speech, whereas tracking in individuals with limited musical training did not differ between music and speech. While such findings point to differences in neural mechanisms supporting speech and music processing, factors like task demands (Devaraju et al., 2021), individual differences (e.g., experience-dependent plasticity; Harding et al., 2019; Sorati and Behne, 2019), and stimulus properties (e.g., “sharpness” of stimulus onset events; Doelling et al., 2019) are important considerations when interpreting study findings.
Oscillations Across Different Populations
Although most work on cortical tracking of speech has been conducted with neurotypical younger adults, it holds promise as an ecologically-valid tool for assessing speech and language processing in different populations (see systematic review by Palana et al., 2022). For example, Braiman et al. (2018) examined cortical tracking of the speech envelope in individuals with severe brain injury who could not produce overt responses. Individuals who showed evidence of minimally conscious state during a fMRI mental imagery task also showed preserved speech envelope tracking. The benefit of the cortical tracking approach is that it provides an inexpensive, temporally precise measure of rhythmic encoding. Examining cortical tracking of speech is thus an attractive approach for the study of speech perception in neurotypical and clinical populations across the lifespan (Ríos-López et al., 2020; Kolozsvári et al., 2021; Ortiz Barajas et al., 2021).
Recently, increased speech envelope tracking has been observed in the delta-theta range in neurotypical older adults relative to younger adults (Presacco et al., 2016; Brodbeck et al., 2018; Decruy et al., 2019; Broderick et al., 2021) and in individuals with vs. without hearing loss (e.g., Mirkovic et al., 2019; Decruy et al., 2020; Gillis et al., 2022). Similarly, increased speech tracking was observed in the theta range (i.e., syllabic rate) in individuals with logopenic variant primary progressive aphasia (lvPPA), a disorder characterized by impaired phonological processing due to neurodegenerative disease (Dial et al., 2021). This finding was highly reliable across narratives differing in acoustic and linguistic features, further supporting the utility of this method in clinical populations.
In contrast, decreased cortical tracking has been observed in the delta-theta range in children and adults with developmental disorders (e.g., children with dyslexia: Molinaro et al., 2016; Power et al., 2016; Di Liberto et al., 2018; Mandke et al., 2022; adults with dyslexia: Molinaro et al., 2016; Fiveash et al., 2020; children with autism spectrum disorder: Wang et al., 2021; c.f., Yu et al., 2018). Individuals with developmental dyslexia exhibit impaired perception of syllabic stress, prosody, and metrical structure, pointing toward a deviant oscillatory network (Goswami, 2019). This was interpreted in the context of the temporal sampling hypothesis (Goswami, 2011, 2019), which states that delta and theta oscillations in auditory cortex are important for prosody perception and temporal integration at the syllable rate, respectively. The temporal sampling hypothesis is also applicable to other communication disorders like stuttering, wherein individuals exhibit impaired rhythm processing (Wieland et al., 2015), poor temporal resolution (Devaraju et al., 2020), and aberrant neural phase coherence when planning speech utterances (Sengupta et al., 2019).
More recent theories also address atypical rhythm processing in individuals with developmental speech and language disorders. Two such theories are the processing rhythm in speech and music (PRISM) framework (Fiveash et al., 2021) and the atypical rhythm risk hypothesis (ARRH; Ladányi et al., 2020). PRISM highlights the importance of evoked oscillatory alignment to external rhythmic stimuli along with precise auditory timing and sensorimotor coupling. Similarly, ARRH stresses early identification of risk factors (e.g., genetic predisposition) and addressing atypical rhythm processing early. A potentially promising approach for addressing atypical rhythm processing is the use of more song-like speech stimuli, as research has shown that, under challenging listening conditions, neural phase-locking is stronger when speech is sung vs. when it is spoken (Vanden Bosch der Nederlanden et al., 2020). With early identification, individuals may have access to better treatment approaches, leading to better long-term outcomes.
Does the Magnitude of Cortical Tracking Reflect the Quality of Processing?
As indicated above, both increased and decreased tracking have been observed in older adults and individuals with communication disorders. Some researchers have characterized the relation between tracking and behavior as non-linear, with increased tracking associated with better performance to a certain level, beyond which increases relate to poorer performance (e.g., Schmidt et al., 2021). Increased tracking in older adults and individuals with hearing loss has been interpreted as reflecting the recruitment of regions outside of primary auditory cortex and an imbalance between excitatory and inhibitory mechanisms, leading to over-excitability, and consequently, inefficient processing of acoustic cues in the speech envelope (e.g., Decruy et al., 2019). For example, Brodbeck et al. (2018) found that the largest difference between older and younger adults occurred at a relatively early latency in regions outside primary auditory cortex, suggesting that older adults recruit a larger network of brain regions to process acoustic cues, even at early stages of processing. Increased cortical tracking could also represent a compensatory mechanism to improve speech perception. In fact, increased envelope tracking in older adults (Decruy et al., 2019) and individuals with hearing loss (Decruy et al., 2020) has been related to better speech understanding.
The relation between cortical tracking and speech processing might also be confounded by differential effects across delta and theta bands. In younger adults, Etard and Reichenbach (2019) found increased delta band tracking related to better comprehension. Similarly, McHaney et al. (2021) found that better speech-in-noise comprehension in older adults was related to larger increases in delta band tracking for speech in noise relative to quiet. Dial et al. (2021) found that individuals with lvPPA had increased theta band envelope tracking relative to neurotypical older adults, despite demonstrating worse speech understanding. Thus, increased cortical tracking in the delta band may reflect better comprehension, whereas increased tracking in the theta band may reflect poorer comprehension. However, contradictory evidence exists. Etard and Reichenbach (2019) found that increased tracking in the theta band in younger adults positively related to perceived speech clarity. Drawing strong conclusions about the unique roles of the delta and theta bands in speech processing is difficult (partially because many studies examine the delta-theta range or an even broader range; e.g., Gillis et al., 2022). Future work should examine these bands separately and, perhaps, instantiate non-linear analysis methods to further elucidate the role of cortical tracking in speech and music processing.
Discussion
Neural oscillations play a critical role in speech and music processing, contributing to our understanding of these processes in various populations. In this mini-review, we presented an overview of neural oscillations, methods for studying them, and their functional relevance to aging, hearing loss, speech and language disorders, and music processing. In the following, we discuss methodological advances that may further elucidate the role of oscillations in auditory processing.
To date, neural oscillations have been studied using M/EEG with high temporal but poor spatial precision. Recent advances in neuroimaging methods with good spatial precision enable acquisition with higher temporal resolution (≤1 s) (Lin et al., 2013; Lewis et al., 2016). For instance, a recent fMRI study with 1 Hz sampling found that hemodynamic responses tracked the envelope of attended speech, particularly in right hemisphere non-primary auditory cortex (Hausfeld and Formisano, 2021). A related technique, functional near-infrared spectroscopy (fNIRS), has superior temporal resolution, higher motion tolerance, and fewer contraindications (e.g., cochlear implants) than fMRI. This makes it a strong candidate for research seeking to localize brain areas where typical/atypical oscillatory mechanisms exist in various populations.
Neuroimaging methods can be applied in tandem, compensating for individual methods’ shortcomings. For example, pairing EEG and fMRI allows for temporally precise localization of neural patterns (Philiastides et al., 2021; cf. Chang and Chen, 2021; Scrivener, 2021). Combined EEG-fMRI has already been applied in research on hemispheric specialization of neural oscillations in dyslexia (Schulz et al., 2008; Lehongre et al., 2013) and in neurotypical individuals to examine cortical tracking of speech in noise (Puschmann et al., 2017). To our knowledge, combined EEG-fMRI has yet to be applied to cortical tracking in clinical populations. This could improve our understanding of the loci of neural lesions contributing to functional differences in cortical tracking, further informing treatment approaches (Lehongre et al., 2013).
Another promising technique is transcranial alternating current stimulation (tACS), which uses a signal matched to different rhythms in incoming stimuli to stimulate brain areas involved in perception. tACS provides a direct method for establishing a causal relationship between external rhythms and neural oscillations. Several studies demonstrated improved acoustic and speech processing following tACS (Jones et al., 2020; Keshavarzi and Reichenbach, 2020; Erkens et al., 2021; Keshavarzi et al., 2021; for recent reviews, see Riecke and Zoefel, 2018; Nooristani et al., 2021). Researchers have also argued for a relation between aberrant cortical tracking of speech and speech-in-noise difficulties in individuals with hearing loss (Fuglsang et al., 2020; Vander Ghinst et al., 2021). tACS is thus a promising method for studying neural oscillations and may improve perception in clinical populations. Moreover, combined M/EEG-tACS may further elucidate intrinsic vs. evoked accounts of neural oscillations (e.g., van Bree et al., 2021).
Beyond multimodal neuroimaging, advances in computational approaches (Doelling et al., 2019; Accou et al., 2021; Guest and Martin, 2021) provide exciting avenues for research on cortical tracking of speech and music and a deeper investigation into the unique contributions of different oscillatory bands. For example, researchers recently utilized neural networks to model predictions based on theta-gamma coupling in syllable recognition and speech prediction (Donhauser and Baillet, 2020; Hovsepyan et al., 2020). Additionally, improvements in natural language processing have resulted in stimulus models representing higher-level linguistic processing, allowing researchers to examine cortical tracking of features like semantic dissimilarity (Broderick et al., 2018).
Research in clinical populations and across the lifespan has just begun to explore cortical tracking of linguistic features at sublexical (e.g., phonetic features), lexical (e.g., word entropy), semantic (e.g., semantic dissimilarity), and syntactic (e.g., surprisal based on part of speech) levels (e.g., Mesik et al., 2021). Such investigations could elucidate the mechanistic underpinnings of impaired processing and assist in identifying deficits in clinical populations, avoiding confounds associated with traditional neuropsychological assessment (e.g., overt responses). This, in turn, could provide treatment targets and a way to assess treatment-induced changes. In sum, the study of neural oscillations provides a unique window into the brain through which we can assay the neurobiological computations supporting speech and music processing in neurotypical and clinical populations. The rapid evolution of this field is promising for basic and applied research and has immense potential for steering neurobiologically informed treatment methods.
Author Contributions
All authors listed have made a substantial, direct, and intellectual contribution to the work, and approved it for publication.
Funding
This work was supported by the NIH/NIDCD NRSA Postdoctoral Fellowship F32DC016812 (awarded to HD), University of Houston Faculty research startup (awarded to HD), and NIH/NIDCD K01DC019421 (awarded to KS).
Conflict of Interest
The authors declare that the research was conducted in the absence of any commercial or financial relationships that could be construed as a potential conflict of interest.
Publisher’s Note
All claims expressed in this article are solely those of the authors and do not necessarily represent those of their affiliated organizations, or those of the publisher, the editors and the reviewers. Any product that may be evaluated in this article, or claim that may be made by its manufacturer, is not guaranteed or endorsed by the publisher.
References
Accou, B., Monesi, M. J., Montoya, J., and Francart, T. (2021). Modeling the relationship between acoustic stimulus and EEG with a dilated convolutional neural network. Eur. Signal Process. Conf. 28, 1175–1179. doi: 10.23919/Eusipco47968.2020.9287417
Ahissar, E., Nagarajan, S., Ahissar, M., Protopapas, A., Mahncke, H., and Merzenich, M. M. (2001). Speech comprehension is correlated with temporal response patterns recorded from the auditory cortex. Proc. Natl. Acad. Sci. U. S. A. 98, 13367–13372. doi: 10.1073/pnas.201400998
Braiman, C., Fridman, E. A., Conte, M. M., Voss, H. U., Reichenbach, C. S., Reichenbach, T., et al. (2018). Cortical response to the natural speech envelope correlates with neuroimaging evidence of cognition in severe brain injury. Curr. Biol. 28, 3833–3839.e3. doi: 10.1016/j.cub.2018.10.057
Brodbeck, C., Presacco, A., Anderson, S., and Simon, J. Z. (2018). Over-representation of speech in older adults originates from early response in higher order auditory cortex. Acta Acust. United Acust. 104, 774–777. doi: 10.3813/AAA.919221
Broderick, M. P., Anderson, A. J., Di Liberto, G. M., Crosse, M. J., and Lalor, E. C. (2018). Electrophysiological correlates of semantic dissimilarity reflect the comprehension of natural, narrative speech. Current Biology, 28, 803–809.
Broderick, M. P., Di Liberto, G. M., Anderson, A. J., Rofes, A., and Lalor, E. C. (2021). Dissociable electrophysiological measures of natural language processing reveal differences in speech comprehension strategy in healthy ageing. Sci. Rep. 11:4963. doi: 10.1038/s41598-021-84597-9
Chang, C., and Chen, J. E. (2021). Multimodal EEG-fMRI: advancing insight into large-scale human brain dynamics. Curr. Opin. Biomed. Eng. 18:100279. doi: 10.1016/j.cobme.2021.100279
Coffey, E. B. J., Arseneau-Bruneau, I., Zhang, X., Baillet, S., and Zatorre, R. J. (2021). Oscillatory entrainment of the frequency following response in auditory cortical and subcortical structures. J. Neurosci. 41, 4073–4087. doi: 10.1523/JNEUROSCI.2313-20.2021
Crosse, M. J., Di Liberto, G. M., Bednar, A., and Lalor, E. C. (2016). The multivariate temporal response function (mTRF) toolbox: a MATLAB toolbox for relating neural signals to continuous stimuli. Front. Hum. Neurosci. 10:604. doi: 10.3389/fnhum.2016.00604
Decruy, L., Vanthornhout, J., and Francart, T. (2019). Evidence for enhanced neural tracking of the speech envelope underlying age-related speech-in-noise difficulties. J. Neurophysiol. 122, 601–615. doi: 10.1152/jn.00687.2018
Decruy, L., Vanthornhout, J., and Francart, T. (2020). Hearing impairment is associated with enhanced neural tracking of the speech envelope. Hear. Res. 393:107961. doi: 10.1016/j.heares.2020.107961
Devaraju, D. S., Kemp, A., Eddins, D. A., Shrivastav, R., Chandrasekaran, B., and Hampton Wray, A. (2021). Effects of task demands on neural correlates of acoustic and semantic processing in challenging listening conditions. J. Speech Lang. Hear. Res. 64, 3697–3706. doi: 10.1044/2021_JSLHR-21-00006
Devaraju, D. S., Maruthy, S., and Kumar, A. U. (2020). Detection of gap and modulations: auditory temporal resolution deficits in adults who stutter. Folia Phoniatr. Logop. 72, 13–21. doi: 10.1159/000499565
Di Liberto, G. M., Peter, V., Kalashnikova, M., Goswami, U., Burnham, D., and Lalor, E. C. (2018). Atypical cortical entrainment to speech in the right hemisphere underpins phonemic deficits in dyslexia. NeuroImage 175, 70–79. doi: 10.1016/j.neuroimage.2018.03.072
Dial, H. R., Gnanateja, G. N., Tessmer, R. S., Gorno Tempini, M. L., Chandrasekaran, B., and Henry, M. L. (2021). Cortical tracking of the speech envelope in logopenic variant primary progressive aphasia. Front. Hum. Neurosci. 14:597694. doi: 10.3389/fnhum.2020.597694
Ding, N., Melloni, L., Zhang, H., Tian, X., and Poeppel, D. (2016). Cortical tracking of hierarchical linguistic structures in connected speech. Nat. Neurosci. 19, 158–164. doi: 10.1038/nn.4186
Doelling, K. B., and Assaneo, M. F. (2021). Neural oscillations are a start toward understanding brain activity rather than the end. PLoS Biol. 19:e3001234. doi: 10.1371/journal.pbio.3001234
Doelling, K. B., Assaneo, M. F., Bevilacqua, D., Pesaran, B., and Poeppel, D. (2019). An oscillator model better predicts cortical entrainment to music. Proc. Natl. Acad. Sci. U. S. A. 116, 10113–10121. doi: 10.1073/pnas.1816414116
Doelling, K. B., and Poeppel, D. (2015). Cortical entrainment to music and its modulation by expertise. Proc. Natl. Acad. Sci. U. S. A. 112, E6233–E6242. doi: 10.1073/pnas.1508431112
Donhauser, P. W., and Baillet, S. (2020). Two distinct neural timescales for predictive speech processing. Neuron 105, 385–393. doi: 10.1016/j.neuron.2019.10.019
Erkens, J., Schulte, M., Vormann, M., Wilsch, A., and Herrmann, C. S. (2021). Hearing impaired participants improve more under envelope-transcranial alternating current stimulation when signal to noise ratio is high. Neurosci. Insights 16:2633105520988854. doi: 10.1177/2633105520988854
Etard, O., and Reichenbach, T. (2019). Neural speech tracking in the theta and in the delta frequency band differentially encode clarity and comprehension of speech in noise. J. Neurosci. 39, 5750–5759. doi: 10.1523/JNEUROSCI.1828-18.2019
Fiveash, A., Bedoin, N., Gordon, R. L., and Tillmann, B. (2021). Processing rhythm in speech and music: shared mechanisms and implications for developmental speech and language disorders. Neuropsychology 35, 771–791. doi: 10.1037/neu0000766
Fiveash, A., Schön, D., Canette, L. H., Morillon, B., Bedoin, N., and Tillmann, B. (2020). A stimulus-brain coupling analysis of regular and irregular rhythms in adults with dyslexia and controls. Brain Cogn. 140:105531. doi: 10.1016/j.bandc.2020.105531
Fuglsang, S. A., Märcher-Rørsted, J., Dau, T., and Hjortkjær, J. (2020). Effects of sensorineural hearing loss on cortical synchronization to competing speech during selective attention. J. Neurosci. 40, 2562–2572. doi: 10.1523/JNEUROSCI.1936-19.2020
Fujioka, T., Ross, B., and Trainor, L. J. (2015). Beta-band oscillations represent auditory beat and its metrical hierarchy in perception and imagery. J. Neurosci. 35, 15187–15198. doi: 10.1523/JNEUROSCI.2397-15.2015
Fujioka, T., Trainor, L. J., Large, E. W., and Ross, B. (2012). Internalized timing of isochronous sounds is represented in neuromagnetic β oscillations. J. Neurosci. 23, 1791–1802. doi: 10.1523/JNEUROSCI.4107-11.2012
Ghitza, O. (2013). The theta-syllable: a unit of speech information defined by cortical function. Front. Psychol. 4:138. doi: 10.3389/fpsyg.2013.00138
Ghitza, O. (2017). Acoustic-driven delta rhythms as prosodic markers. Lang. Cogn. Neurosci. 32, 545–561. doi: 10.1080/23273798.2016.1232419
Gillis, M., Decruy, L., Vanthornhout, J., and Francart, T. (2022). Hearing loss is associated with delayed neural responses to continuous speech. Eur. J. Neurosci. 55, 1671–1690. doi: 10.1111/ejn.15644
Giraud, A.-L., and Poeppel, D. (2012). Cortical oscillations and speech processing: emerging computational principles and operations. Nat. Neurosci. 15, 511–517. doi: 10.1038/nn.3063
Goswami, U. (2011). A temporal sampling framework for developmental dyslexia. Trends Cogn. Sci. 15, 3–10. doi: 10.1016/j.tics.2010.10.001
Goswami, U. (2019). A neural oscillations perspective on phonological development and phonological processing in developmental dyslexia. Linguistics Lang. Compass 13:e12328. doi: 10.1111/lnc3.12328
Grabe, E., and Low, E. L. (2002). Durational variability in speech and the rhythm class hypothesis. Lab. Phonol. 7, 515–546. doi: 10.1515/9783110197105
Guest, O., and Martin, A. E. (2021). How computational modeling can force theory building in psychological science. Perspect. Psychol. Sci. 16, 789–802. doi: 10.1177/1745691620970585
Haegens, S., and Zion Golumbic, E. (2018). Rhythmic facilitation of sensory processing: a critical review. Neurosci. Biobehav. Rev. 86, 150–165. doi: 10.1016/j.neubiorev.2017.12.002
Harding, E. E., Sammler, D., Henry, M. J., Large, E. W., and Kotz, S. A. (2019). Cortical tracking of rhythm in music and speech. NeuroImage 185, 96–101. doi: 10.1016/j.neuroimage.2018.10.037
Hausfeld, L., and Formisano, E. (2021). Listening to speech in noisy scenes: antithetical contribution of primary and non-primary auditory cortex. bioRxiv [Preprint]. doi: 10.1101/2021.10.26.465858
Hovsepyan, S., Olasagasti, I., and Giraud, A. L. (2020). Combining predictive coding and neural oscillations enables online syllable recognition in natural speech. Nat. Commun. 11:3117. doi: 10.1038/s41467-020-16956-5
Howard, M. F., and Poeppel, D. (2010). Discrimination of speech stimuli based on neuronal response phase patterns depends on acoustics but not comprehension. J. Neurophysiol. 104, 2500–2511. doi: 10.1152/jn.00251.2010
Hyafil, A., Giraud, A. L., Fontolan, L., and Gutkin, B. (2015b). Neural cross-frequency coupling: connecting architectures, mechanisms, and functions. Trends Neurosci. 38, 725–740. doi: 10.1016/j.tins.2015.09.001
Hyafil, A., Fontolan, L., Kabdebon, C., Gutkin, B., and Giraud, A.-L. (2015a). Speech encoding by coupled cortical theta and gamma oscillations. eLife 4:e06213. doi: 10.7554/eLife.06213
Jadoul, Y., Ravignani, A., Thompson, B., Filippi, P., and De Boer, B. (2016). Seeking temporal predictability in speech: comparing statistical approaches on 18 world languages. Front. Hum. Neurosci. 10:586. doi: 10.3389/fnhum.2016.00586
Jones, K. T., Johnson, E. L., Tauxe, Z. S., and Rojas, D. C. (2020). Modulation of auditory gamma-band responses using transcranial electrical stimulation. J. Neurophysiol. 123, 2504–2514. doi: 10.1152/jn.00003.2020
Keitel, A., Gross, J., and Kayser, C. (2018). Perceptually relevant speech tracking in auditory and motor cortex reflects distinct linguistic features. PLoS Biol. 16:e2004473. doi: 10.1371/journal.pbio.2004473
Keshavarzi, M., and Reichenbach, T. (2020). Transcranial alternating current stimulation with the theta-band portion of the temporally-aligned speech envelope improves speech-in-noise comprehension. Front. Hum. Neurosci. 14:187. doi: 10.3389/fnhum.2020.00187
Keshavarzi, M., Varano, E., and Reichenbach, T. (2021). Cortical tracking of a background speaker modulates the comprehension of a foreground speech signal. J. Neurosci. 41:5093. doi: 10.1523/JNEUROSCI.3200-20.2021
Kolozsvári, O. B., Xu, W., Gerike, G., Parviainen, T., Nieminen, L., Noiray, A., et al. (2021). Coherence between brain activation and speech envelope at word and sentence levels showed age-related differences in low frequency bands. Neurobiol. Lang. 2, 226–253. doi: 10.1162/nol_a_00033
Ladányi, E., Persici, V., Fiveash, A., Tillmann, B., and Gordon, R. L. (2020). Is atypical rhythm a risk factor for developmental speech and language disorders? Interdiscip. Rev. Cogn. Sci. 11:e1528. doi: 10.1002/wcs.1528
Lakatos, P., Gross, J., and Thut, G. (2019). A new unifying account of the roles of neuronal entrainment. Curr. Biol. 29, R890–R905. doi: 10.1016/j.cub.2019.07.075
Lehongre, K., Morillon, B., Giraud, A. L., and Ramus, F. (2013). Impaired auditory sampling in dyslexia: further evidence from combined fMRI and EEG. Front. Hum. Neurosci. 7:454. doi: 10.3389/fnhum.2013.00454
Lewis, L. D., Setsompop, K., Rosen, B. R., and Polimeni, J. R. (2016). Fast fMRI can detect oscillatory neural activity in humans. Proc. Natl. Acad. Sci. U. S. A. 113, E6679–E6685. doi: 10.1073/pnas.1608117113
Lin, F. H., Witzel, T., Raij, T., Ahveninen, J., Tsai, K. W., Chu, Y. H., et al. (2013). fMRI hemodynamics accurately reflects neuronal timing in the human brain measured by MEG. Neuroimage 78, 372–384. doi: 10.1016/j.neuroimage.2013.04.017
Lizarazu, M., Lallier, M., and Molinaro, N. (2019). Phase-amplitude coupling between theta and gamma oscillations adapts to speech rate. Ann. N. Y. Acad. Sci. 1453:140. doi: 10.1111/nyas.14099
Lizcano-Cortés, F., Gómez-Varela, I., Mares, C., Wallisch, P., Orpella, J., Poeppel, D., et al. (2022). Speech-to-Speech Synchronization protocol to classify human participants as high or low auditory-motor synchronizers. STAR Protocols 3:101248. doi: 10.1016/j.xpro.2022.101248
Mandke, K., Flanagan, S., Macfarlane, A., Gabrielczyk, F., Wilson, A., Gross, J., et al. (2022). Neural sampling of the speech signal at different timescales by children with dyslexia. NeuroImage 253:119077. doi: 10.1016/j.neuroimage.2022.119077
Masuda, N., and Doiron, B. (2007). Gamma oscillations of spiking neural populations enhance signal discrimination. PLoS Comput. Biol. 3:e236. doi: 10.1371/journal.pcbi.0030236
McHaney, J. R., Gnanateja, G. N., Smayda, K. E., Zinszer, B. D., and Chandrasekaran, B. (2021). Cortical tracking of speech in delta band relates to individual differences in speech in noise comprehension in older adults. Ear Hear. 42, 343–354. doi: 10.1097/AUD.0000000000000923
Mesik, J., Ray, L., and Wojtczak, M. (2021). Effects of age on cortical tracking of word-level features of continuous competing speech. Front. Neurosci. 15:363. doi: 10.3389/fnins.2021.635126
Meyer, L. (2018). The neural oscillations of speech processing and language comprehension: state of the art and emerging mechanisms. Eur. J. Neurosci. 48, 2609–2621. doi: 10.1111/ejn.13748
Meyer, L., Henry, M. J., Gaston, P., Schmuck, N., and Friederici, A. D. (2017). Linguistic bias modulates interpretation of speech via neural delta-band oscillations. Cereb. Cortex 27, 4293–4302. doi: 10.1093/cercor/bhw228
Meyer, L., Sun, Y., and Martin, A. E. (2020a). Synchronous, but not entrained: exogenous and endogenous cortical rhythms of speech and language processing. Lang. Cogn. Neurosci. 35, 1089–1099. doi: 10.1080/23273798.2019.1693050
Meyer, L., Sun, Y., and Martin, A. E. (2020b). “Entraining” to speech, generating language? Lang. Cogn. Neurosci. 35, 1138–1148. doi: 10.1080/23273798.2020.1827155
Mirkovic, B., Debener, S., Schmidt, J., Jaeger, M., and Neher, T. (2019). Effects of directional sound processing and listener’s motivation on EEG responses to continuous noisy speech: do normal-hearing and aided hearing-impaired listeners differ? Hear. Res. 377, 260–270. doi: 10.1016/j.heares.2019.04.005
Molinaro, N., Lizarazu, M., Lallier, M., Bourguignon, M., and Carreiras, M. (2016). Out-of-synchrony speech entrainment in developmental dyslexia. Hum. Brain Mapp. 37, 2767–2783. doi: 10.1002/hbm.23206
Myers, B. R., Lense, M. D., and Gordon, R. L. (2019). Pushing the envelope: developments in neural entrainment to speech and the biological underpinnings of prosody perception. Brain Sci. 9:70. doi: 10.3390/brainsci9030070
Nelken, I., and Chechik, G. (2007). Information theory in auditory research. Hear. Res. 229, 94–105.
Nolan, F., and Jeon, H.-S. (2014). Speech rhythm: a metaphor? Philos. Trans. R. Soc. Lond. B Biol. Sci. 369:20130396. doi: 10.1098/rstb.2013.0396
Nooristani, M., Augereau, T., Moïn-Darbari, K., Bacon, B. A., and Champoux, F. (2021). Using transcranial electrical stimulation in audiological practice: the gaps to be filled. Front. Hum. Neurosci. 15:735561. doi: 10.3389/fnhum.2021.735561
Obleser, J., and Kayser, C. (2019). Neural entrainment and attentional selection in the listening brain. Trends Cogn. Sci. 23, 913–926. doi: 10.1016/j.tics.2019.08.004
Ortiz Barajas, M. C., Guevara, R., and Gervain, J. (2021). The origins and development of speech envelope tracking during the first months of life. Dev. Cogn. Neurosci. 48:100915. doi: 10.1016/j.dcn.2021.100915
Palana, J., Schwartz, S., and Tager-Flusberg, H. (2022). Evaluating the use of cortical entrainment to measure atypical speech processing: a systematic review. Neurosci. Biobehav. Rev. 133:104506. doi: 10.1016/j.neubiorev.2021.12.029
Peelle, J., and Davis, M. (2012). Neural oscillations carry speech rhythm through to comprehension. Front. Psychol. 3:320. doi: 10.3389/fpsyg.2012.00320
Peelle, J. E., Gross, J., and Davis, M. H. (2013). Phase-locked responses to speech in human auditory cortex are enhanced during comprehension. Cereb. Cortex. 23, 1378–1387. doi: 10.1093/cercor/bhs118
Philiastides, M. G., Tu, T., and Sajda, P. (2021). Inferring macroscale brain dynamics via fusion of simultaneous EEG-fMRI. Annu. Rev. Neurosci. 44, 315–334. doi: 10.1146/annurev-neuro-100220-093239
Poeppel, D., and Assaneo, M. F. (2020). Speech rhythms and their neural foundations. Nat. Rev. Neurosci. 21, 322–334. doi: 10.1038/s41583-020-0304-4
Power, A. J., Colling, L. J., Mead, N., Barnes, L., and Goswami, U. (2016). Neural encoding of the speech envelope by children with developmental dyslexia. Brain Lang. 160, 1–10. doi: 10.1016/j.bandl.2016.06.006
Presacco, A., Simon, J. Z., and Anderson, S. (2016). Evidence of degraded representation of speech in noise, in the aging midbrain and cortex. J. Neurophysiol. 116, 2346–2355. doi: 10.1152/jn.00372.2016
Puschmann, S., Steinkamp, S., Gillich, I., Mirkovic, B., Debener, S., and Thiel, C. M. (2017). The right temporoparietal junction supports speech tracking during selective listening: evidence from concurrent EEG-fMRI. J. Neurosci. 37, 11505–11516. doi: 10.1523/JNEUROSCI.1007-17.2017
Riecke, L., and Zoefel, B. (2018). Conveying temporal information to the auditory system via transcranial current stimulation. Acta Acust. United Acust. 104, 883–886. doi: 10.3813/AAA.919235
Rimmele, J. M., Golumbic, E. Z., Schröger, E., and Poeppel, D. (2015). The effects of selective attention and speech acoustics on neural speech-tracking in a multi-talker scene. Cortex 68, 144–154. doi: 10.1016/j.cortex.2014.12.014
Rimmele, J. M., Morillon, B., Poeppel, D., and Arnal, L. H. (2018). Proactive sensing of periodic and aperiodic auditory patterns. Trends Cogn. Sci. 22, 870–882. doi: 10.1016/j.tics.2018.08.003
Rimmele, J. M., Poeppel, D., and Ghitza, O. (2021). Acoustically driven cortical δ oscillations underpin prosodic chunking. Eneuro 8:4. doi: 10.1523/ENEURO.0562-20.2021
Ríos-López, P., Molinaro, N., Bourguignon, M., and Lallier, M. (2020). Development of neural oscillatory activity in response to speech in children from 4 to 6 years old. Dev. Sci. 23:e12947. doi: 10.1111/desc.12947
Schmidt, F., Chen, Y. P., Keitel, A., Rösch, S., Hannemann, R., Serman, M., et al. (2021). Neural speech tracking shifts from the syllabic to the modulation rate of speech as intelligibility decreases. bioRxiv [Preprint]. doi: 10.1101/2021.03.25.437033v1
Schulz, E., Maurer, U., van der Mark, S., Bucher, K., Brem, S., Martin, E., et al. (2008). Impaired semantic processing during sentence reading in children with dyslexia: combined fMRI and ERP evidence. Neuroimage 41, 153–168. doi: 10.1016/j.neuroimage.2008.02.012
Scrivener, C. L. (2021). When is simultaneous recording necessary? A guide for researchers considering combined EEG-fMRI. Front. Neurosci. 15:774. doi: 10.3389/fnins.2021.636424
Sengupta, R., Yaruss, J. S., Loucks, T. M., Gracco, V. L., Pelczarski, K., and Nasir, S. M. (2019). Theta modulated neural phase coherence facilitates speech fluency in adults who stutter. Front. Hum. Neurosci. 13:394. doi: 10.3389/fnhum.2019.00394
Sorati, M., and Behne, D. M. (2019). Musical expertise affects audiovisual speech perception: findings from event-related potentials and inter-trial phase coherence. Front. Psychol. 10:2562. doi: 10.3389/fpsyg.2019.02562
Teoh, E. S., Cappelloni, M. S., and Lalor, E. C. (2019). Prosodic pitch processing is represented in delta-band EEG and is dissociable from the cortical tracking of other acoustic and phonetic features. Eur. J. Neurosci. 50, 3831–3842. doi: 10.1111/ejn.14510
van Bree, S., Sohoglu, E., Davis, M. H., and Zoefel, B. (2021). Sustained neural rhythms reveal endogenous oscillations supporting speech perception. PLoS Biol. 19:e3001142. doi: 10.1371/journal.pbio.3001142
Vanden Bosch der Nederlanden, C. M., Joanisse, M. F., and Grahn, J. A. (2020). Music as a scaffold for listening to speech: better neural phase-locking to song than speech. NeuroImage 214:116767. doi: 10.1016/j.neuroimage.2020.116767
Vander Ghinst, M., Bourguignon, M., Wens, V., Naeije, G., Ducène, C., Niesen, M., et al. (2021). Inaccurate cortical tracking of speech in adults with impaired speech perception in noise. Brain Commun. 3:fcab186. doi: 10.1093/braincomms/fcab186
Wang, X., Saa, J. F. D., Marchesotti, S., Kojovic, N., Sperdin, H. F., Rihs, T. A., et al. (2021). Neural oscillation coupling selectively predicts speech reception in young children with Autism Spectrum Disorder. bioRxiv [Preprint]. doi: 10.1101/2021.09.27.461214
Wieland, E. A., McAuley, J. D., Dilley, L. C., and Chang, S. E. (2015). Evidence for a rhythm perception deficit in children who stutter. Brain Lang. 144, 26–34. doi: 10.1016/j.bandl.2015.03.008
Wöstmann, M., Lim, S.-J., and Obleser, J. (2017). The human neural alpha response to speech is a proxy of attentional control. Cereb. Cortex 27, 3307–3317. doi: 10.1093/cercor/bhx074
Yu, L., Wang, S., Huang, D., Wu, X., and Zhang, Y. (2018). Role of inter-trial phase coherence in atypical auditory evoked potentials to speech and nonspeech stimuli in children with autism. Clin. Neurophysiol. 129, 1374–1382. doi: 10.1016/j.clinph.2018.04.599
Zan, P., Presacco, A., Anderson, S., and Simon, J. Z. (2019). Mutual information analysis of neural representations of speech in noise in the aging midbrain. J. Neurophys. 122, 2372–2387. doi: 10.1152/jn.00270.2019
Keywords: neural oscillations, cortical tracking, speech tracking, cortical entrainment, neurogenic communication disorders, electrophysiology, speech processing, music processing
Citation: Gnanateja GN, Devaraju DS, Heyne M, Quique YM, Sitek KR, Tardif MC, Tessmer R and Dial HR (2022) On the Role of Neural Oscillations Across Timescales in Speech and Music Processing. Front. Comput. Neurosci. 16:872093. doi: 10.3389/fncom.2022.872093
Received: 09 February 2022; Accepted: 24 May 2022;
Published: 23 June 2022.
Edited by:
Johanna Maria Rimmele, Max Planck Institute for Empirical Aesthetics, GermanyReviewed by:
Katharina S. Rufener, University Hospital Magdeburg, GermanyAnna Kasdan, Vanderbilt University, United States
Copyright © 2022 Gnanateja, Devaraju, Heyne, Quique, Sitek, Tardif, Tessmer and Dial. This is an open-access article distributed under the terms of the Creative Commons Attribution License (CC BY). The use, distribution or reproduction in other forums is permitted, provided the original author(s) and the copyright owner(s) are credited and that the original publication in this journal is cited, in accordance with accepted academic practice. No use, distribution or reproduction is permitted which does not comply with these terms.
*Correspondence: Heather R. Dial, aHJkaWFsQGNlbnRyYWwudWguZWR1
†These authors have contributed equally to this work