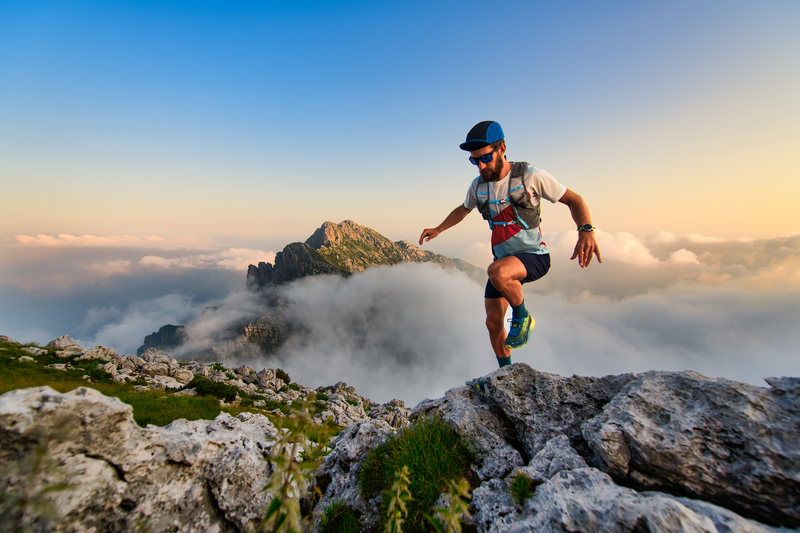
94% of researchers rate our articles as excellent or good
Learn more about the work of our research integrity team to safeguard the quality of each article we publish.
Find out more
CORRECTION article
Front. Comput. Neurosci. , 26 May 2021
Volume 15 - 2021 | https://doi.org/10.3389/fncom.2021.698574
This article is part of the Research Topic How Can Neuroscience Contribute to the Development of General Artificial Intelligence? View all 9 articles
This article is a correction to:
Attention in Psychology, Neuroscience, and Machine Learning
A Corrigendum on
Attention in Psychology, Neuroscience, and Machine Learning
by Lindsay, G. W. (2020). Front. Comput. Neurosci. 14:29. doi: 10.3389/fncom.2020.00029
In the original article, there was an error. A portion of the text was repeated unnecessarily.
A correction has been made to 4. Ideas for Future Interaction Between Artificial and Biological Attention, 4.2. How to Deploy Attention, Paragraph 4. The corrected paragraph is shown below.
Activities would likely need to flexibly decide which of several possible goals should be achieved at any time and therefore where attention should be placed. This problem clearly interacts closely with issues around reinforcement learning—particularly hierarchical reinforcement learning which involves the choosing of subtasks—as such decisions must be based on expected positive or negative outcomes. Indeed, there is a close relationship between attention and reward as previously rewarded stimuli attract attention even in contexts where they no longer provide reward (Camara et al., 2013). A better understanding of how humans choose which tasks to engage in and when should allow human behavior to inform the design of a multi-task AI.
The author apologizes for this error and states that this does not change the scientific conclusions of the article in any way. The original article has been updated.
Keywords: attention, artificial neural networks, machine learning, vision, memory, awareness
Citation: Lindsay GW (2021) Corrigendum: Attention in Psychology, Neuroscience, and Machine Learning. Front. Comput. Neurosci. 15:698574. doi: 10.3389/fncom.2021.698574
Received: 21 April 2021; Accepted: 30 April 2021;
Published: 26 May 2021.
Approved by:
Frontiers Editorial Office, Frontiers Media SA, SwitzerlandCopyright © 2021 Lindsay. This is an open-access article distributed under the terms of the Creative Commons Attribution License (CC BY). The use, distribution or reproduction in other forums is permitted, provided the original author(s) and the copyright owner(s) are credited and that the original publication in this journal is cited, in accordance with accepted academic practice. No use, distribution or reproduction is permitted which does not comply with these terms.
*Correspondence: Grace W. Lindsay, Z3JhY2V3bGluZHNheUBnbWFpbC5jb20=
Disclaimer: All claims expressed in this article are solely those of the authors and do not necessarily represent those of their affiliated organizations, or those of the publisher, the editors and the reviewers. Any product that may be evaluated in this article or claim that may be made by its manufacturer is not guaranteed or endorsed by the publisher.
Research integrity at Frontiers
Learn more about the work of our research integrity team to safeguard the quality of each article we publish.