- School of Electrical and Electronic Engineering, The University of Adelaide, Adelaide, SA, Australia
Brain computer interfaces (BCI) for the rehabilitation of motor impairments exploit sensorimotor rhythms (SMR) in the electroencephalogram (EEG). However, the neurophysiological processes underpinning the SMR often vary over time and across subjects. Inherent intra- and inter-subject variability causes covariate shift in data distributions that impede the transferability of model parameters amongst sessions/subjects. Transfer learning includes machine learning-based methods to compensate for inter-subject and inter-session (intra-subject) variability manifested in EEG-derived feature distributions as a covariate shift for BCI. Besides transfer learning approaches, recent studies have explored psychological and neurophysiological predictors as well as inter-subject associativity assessment, which may augment transfer learning in EEG-based BCI. Here, we highlight the importance of measuring inter-session/subject performance predictors for generalized BCI frameworks for both normal and motor-impaired people, reducing the necessity for tedious and annoying calibration sessions and BCI training.
1. Introduction
Brain computer interfaces (BCI) exploiting sensorimotor rhythms (SMR) have shown promise for both the improvement of motor performance in normal subjects and the rehabilitation of motor function in patients (Dobkin, 2007; Wang and Jung, 2011). The SMR can be elicited by motor imagery (MI) that shares common neurophysiological mechanisms with overt motor execution (ME), the former being more convenient for BCI users who cannot perform an overt ME task due to some degree of motor disability (Jeannerod, 1995; Lotze and Halsband, 2006; Zich et al., 2015; Vyas et al., 2018). ME supplements the MI-based motor learning process for people with intact cognitive functions (Allami et al., 2008; Ruffino et al., 2017).
Since the motor learning processes differ across individuals (Herzfeld and Shadmehr, 2014; Wu et al., 2014), significant inter-subject variability in motor behavior is anticipated that manifests in the task-specific electrical activities in the cortico-subcortical networks (Seghier and Price, 2018). Consequently, the cortical activity observed in electroencephalogram (EEG) varies across subjects during MI, impeding its utility for BCI applications (Saha et al., 2017b). A study has suggested that time-variant brain functions cause unreliable EEG signatures with poor reproducibility even within a particular subject (Meyer et al., 2013). Such inter-session, intra-subject variability together with even larger inter-subject variability confounds BCI using SMR. This review discusses how inter-session and inter-subject performance predictors could potentially augment transfer learning to improve SMR-based BCI performance while reducing calibration efforts significantly.
2. Sensorimotor Dynamics and BCI
2.1. Motor Learning Process and Brain Function
Motor variability due to variability in human kinematic parameters, e.g., force field adaptation, speed and trajectory, and motivational factors such as level of user engagement, arousal and feelings of competence, necessary for performing a motor task is an integral part of the motor learning process (Duarte and Reinkensmeyer, 2015; Úbeda et al., 2015; Edelman et al., 2019; Faller et al., 2019). Such variability does not necessarily represent noise contents only, but may potentially be a manifestation of motor and perceptual learning processes. Motor variability may augment reinforcement-based motor learning (Herzfeld and Shadmehr, 2014; Wu et al., 2014; Singh et al., 2016). Individuals with higher motor variability may learn a skill faster than individuals with lower motor variability (Wu et al., 2014; Singh et al., 2016). The EEG patterns associated with motor variability could therefore partly explain intra-individual variability in SMR-based BCI (Bradberry et al., 2010; Úbeda et al., 2015; Ostry and Gribble, 2016). Furthermore, structural and functional differences between subjects are associated with motor learning process, which might explain the motor learning variability (Tomassini et al., 2011). On the other hand, motor variability could be leveraged to augment the motor learning and rehabilitation (Krakauer, 2006; Singh et al., 2016). A study has demonstrated that alterations in EEG signatures due to motor training are dependent on intra- and inter-subject variability (Jochumsen et al., 2017).
2.2. Motor Imagery vs. Motor Execution
Motor imagery is the kinesthetic anticipation of corresponding overt ME without producing an actual motor output. Jeannerod stated that MI is functionally equivalent to its ME counterpart (Jeannerod, 1995). More specifically, MI is related to the preparation of ME and represents meaningful neurophysiological dynamics of human motor functions (Zich et al., 2015). Consequently, both MI and ME share common sensorimotor areas such as primary motor area (M1), supplementary motor area (SMA) and premotor cortex (PMC) (Jeannerod, 1995; Lotze and Halsband, 2006; Zich et al., 2015).
The neurophysiology underlying MI may differ in healthy people and patients with motor-impairing conditions (Lotze et al., 2001). MI-based BCI may augment the motor learning process in healthy subjects (Ruffino et al., 2017). In patients with impaired motor functions, MI is often the only viable option to drive rehabilitative BCI due to users' inability to perform overt ME (Jackson et al., 2001; Lotze and Halsband, 2006). The individuality and severity of motor impairments impact the underlying neurophysiology, for example, post-stroke neurophysiology relies on the lesion locations (Niazi et al., 2013). Studies are essential to further delineate the roles of MI and ME in motor learning or relearning for both healthy and impaired subjects to refine the design of BCI for supplementing the motor learning process.
2.3. Neuroplasticity and BCI-Driven Motor Rehabilitation
Rehabilitative BCI designs either attach neural prostheses to the impaired upper/lower limb or restimulate the damaged synaptic networks. In either case, the idea is to exploit and promote neural plasticity (Dobkin, 2007; Wang et al., 2010). The plastic characteristics of the brain are created by the time-variant behavior of the synapses within complex neural networks, first illustrated by Hebb, 1949 (Brown and Milner, 2003). The motor learning process and associated variability promote plasticity in the sensorimotor networks and adjust both motor and perceptual skills (Ostry and Gribble, 2016). This inherent plasticity is exploited by BCI systems to rehabilitate impaired motor functions (Dobkin, 2007). Ruffino et al. demonstrated that MI-based mental training can contribute to corticospinal plasticity (Ruffino et al., 2017). This might lead to BCI-driven rehabilitation systems for stroke and spinal cord injury patients (Niazi et al., 2013; Müller-Putz et al., 2014). Recent studies showed that BCI skill acquisition and associated physiological changes may improve BCI performance in both patients and healthy users (Perdikis et al., 2018; Edelman et al., 2019). Complex or cognitively entertaining tasks that require greater user engagement or motivation can compensate for intra- and inter-subject variability, leading to enhanced BCI learning in adverse operating conditions (Perdikis et al., 2018; Edelman et al., 2019; Faller et al., 2019; Li et al., 2019).
BCI-driven prostheses can extend the degree of freedom of users with motor impairments. The success of BCI control and rehabilitation depends on the user's capacity to modulate the intact neural ensembles (Dobkin, 2007). Substantial changes in neural substrates that were observed following closed-loop BCI-driven motor learning of prosthesis control provide evidence of neuroplasticity (Orsborn et al., 2014). In stroke patients, post-rehabilitation electromyographic recordings showed increased activity in the paretic finger following BCI-driven rehabilitation using an orthosis, which exhibits improvement in neuromuscular coherence for movement control (Ramos-Murguialday et al., 2013). Furthermore, BCI-driven proprioceptive feedback-based and functional electrical stimulation-based rehabilitation strategies could reinforce motor control (Zhao et al., 2016; Darvishi et al., 2017; Selfslagh et al., 2019).
The structural and functional changes in neural substrates induced by MI-based training with transcranial direct current stimulation or transcranial magnetic stimulation provide further evidence for the induction of neuroplasticity that is essential for motor recovery (Hong et al., 2017; Johnson et al., 2018). Because the induction of plasticity by rehabilitation varies across subjects (Leamy et al., 2014; Vallence et al., 2015), subject-specific training sessions may be required. Since the neurophysiology associated with SMR dynamics varies between individuals, quantification of variability in healthy user groups could be beneficial first step that may guide the interpretation of altered neurophysiology in diverse conditions of motor-impairment (Müller-Putz et al., 2014).
3. Brain Topography and BCI Performance Predictors
3.1. Intra- and Inter-subject Variability in Brain Topography
The functional relevance of brain topographical variability with the anatomical boundaries is still not fully understood; however, significant structure-function correspondences may be derived at the aggregate level (Honey et al., 2009, 2010). Smith et al. delineated structural differences, suggesting that the number of folds and thickness of the cortex could be associated with whole-brain functional networks (Smith et al., 2019). Furthermore, inter-subject variability in topography occurs due to subject-specific cognitive style and strategy to perform a task over time (Seghier and Price, 2018), which could augment the underlying learning processes, e.g., motor and perceptual learning (Krakauer, 2006; Baldassarre et al., 2012; Herzfeld and Shadmehr, 2014; Wu et al., 2014; Singh et al., 2016).
Intra- and inter-subject variability can be explained by scale-dependent brain networks in spatial, temporal and topological domains (Betzel and Bassett, 2017; Betzel et al., 2019). For example, diversity in spatial organization of the brain networks can be investigated either at cellular or system level. The sources of intra- and inter-subject variability in brain dynamics may be identifiable using multi-scale analysis tools (Betzel et al., 2019) although the interpretation of brain connectivity networks at different scales may not be straightforward (Raichle, 2009).
Integrating intrinsic brain activities (i.e., resting state activities) into BCI design could offer experimental and methodological advantages for scrutinizing task-specific brain dynamics (Northoff et al., 2010). While it has been argued that the brain is primarily reflexive, responding according to external stimuli/environmental demand, the brain also performs many intrinsic functions including signal acquisition, maintenance, and interpretation (Raichle, 2009, 2010). Supporting the critical role of intrinsic brain activity, it consumes 20% of the body's energy (Clarke, 1999). Thus, understanding the role of resting EEG might supplement BCI performance (Northoff et al., 2010; Suk et al., 2014; Morioka et al., 2015).
3.2. BCI Performance Predictors
Around 15–30% users are inherently unable to produce task-specific signature robust enough to control a BCI (Blankertz et al., 2009; Vidaurre and Blankertz, 2010). The underlying causes of this BCI illiteracy are not well-understood; however, diverse psychological and neurophysiological predictors appear to be associated with BCI performance (Blankertz et al., 2009; Vidaurre and Blankertz, 2010; Jensen et al., 2011; Hammer et al., 2012; Ahn and Jun, 2015; Jeunet et al., 2015; Reichert et al., 2015; Zhang et al., 2015; Acqualagna et al., 2016; Vasilyev et al., 2017; Sannelli et al., 2019).
Cognitive and neurological factors including functions and anatomy along with emotional and mental processes give rise to intra- and inter-subject variability affecting the performance of SMR-based BCI (Wens et al., 2014; Reichert et al., 2015; Zhang et al., 2015; Acqualagna et al., 2016; Betzel and Bassett, 2017; Vasilyev et al., 2017; Seghier and Price, 2018; Betzel et al., 2019; Smith et al., 2019). Time-variant cognitive factors such as fatigue, memory load, attention and reaction time modulate instantaneous brain activity, and can cause inconsistent SMR-based BCI performance (Hammer et al., 2012; Ahn and Jun, 2015; Fox et al., 2015; Jeunet et al., 2015; Darvishi et al., 2018; Sannelli et al., 2019). Furthermore, users' characteristics such as lifestyle, gender, and age can influence BCI performance (Ahn and Jun, 2015). Kasahara et al. illustrated that a neuroanatomical feature i.e., gray matter volume is associated with SMR-based BCI performance (Kasahara et al., 2015).
The structural and functional differences may characterize dynamic baseline activities manifested in resting-state network (RSN) dynamics. RSNs represent large-scale spatiotemporal structures exhibiting intrinsic brain activities that are thought to be functionally relevant (Deco et al., 2011). Studies have shown intra- and inter-subject variability in sensorimotor RSN, which may have implications for BCI performance variability (Jensen et al., 2011; Wens et al., 2014; Reichert et al., 2015; Zhang et al., 2015; Acqualagna et al., 2016; Vasilyev et al., 2017). It has been hypothesized that SMR-based BCI performance predictor is reliable for people who display strong resting EEG amplitudes (Blankertz et al., 2010; Suk et al., 2014; Sannelli et al., 2019). Table 1 shows a list of intra- and inter-subject BCI performance predictors.
4. Transfer Learning
4.1. Covariate Shift and Transfer Learning
Transfer learning techniques originating from the field of machine learning have been adopted to compensate BCI systems for inter-subject and inter-session variability of EEG feature distributions (Fazli et al., 2015; Jayaram et al., 2016). A key idea is to regularize BCI model parameters for covariate shift adaptation. Covariate shift occurs when distributions of training and test data differ significantly although their conditional distributions may remain unchanged (Krusienski et al., 2011). Figure 1 schematically illustrates the idea of covariate shift when the training and test data distributions are different. The underlying time-variant and subject-specific brain dynamics depends on associated psychological and neurophysiological factors (Blankertz et al., 2009; Vidaurre and Blankertz, 2010; Jensen et al., 2011; Hammer et al., 2012; Ahn and Jun, 2015; Jeunet et al., 2015; Reichert et al., 2015; Zhang et al., 2015; Acqualagna et al., 2016; Vasilyev et al., 2017; Sannelli et al., 2019) and cause covariate shift in EEG-derived feature distributions (Krusienski et al., 2011; Fazli et al., 2015; Jayaram et al., 2016).
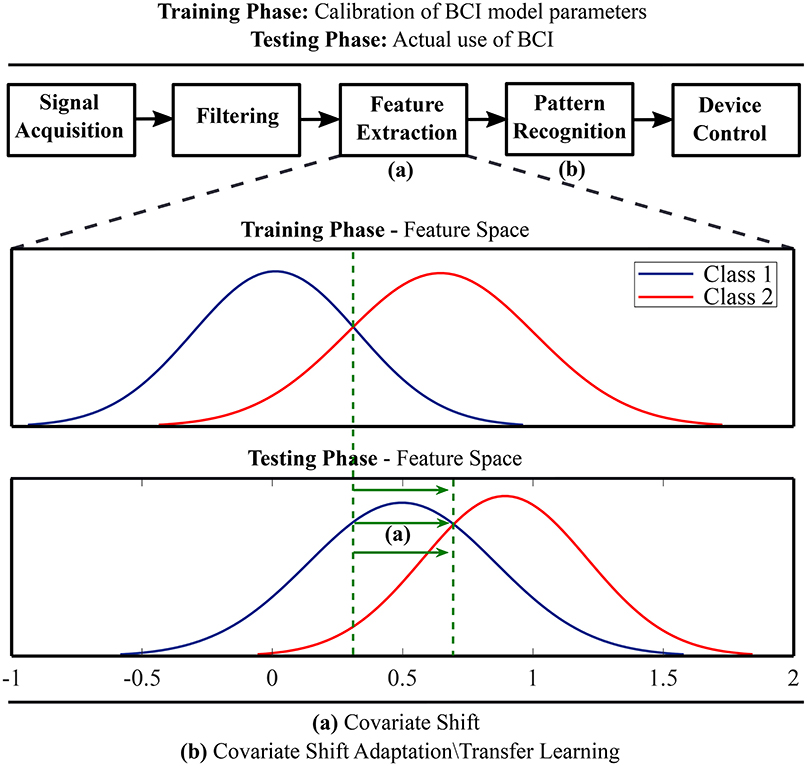
Figure 1. A schematic illustration of covariate shift in the feature space and application of transfer learning methods for covariate shift adaptation.
The earliest attempts to overcome inter-session variability include preliminary training sessions to enhance the user's ability to modulate brain signals robust enough to control BCI (Wolpaw et al., 1991; Wolpaw and McFarland, 1994; Birbaumer et al., 1999). The training sessions required for users are tedious and inconvenient. Therefore, machine learning-based BCI models were introduced to reduce individual training session for each BCI use, in which a model has to be calibrated based on the data at the beginning of each session (Ramoser et al., 2000; Blankertz et al., 2002). Recent studies have proposed SMR-based BCI without any session- and subject-specific calibration utilizing the concept of transfer learning (Kang et al., 2009; Li et al., 2010; Lu et al., 2010; Niazi et al., 2013; Kang and Choi, 2014; Fazli et al., 2015; Lotte, 2015; Jayaram et al., 2016; Saha et al., 2017a,b, 2019; Fahimi et al., 2018; He and Wu, 2019).
4.2. The Concept of Inter-subject Associativity
Most of the existing transfer learning approaches are based on regularization or inter-session/subject transfer of model parameters, indirectly transferring knowledge pertaining to the sources of intra- and inter-subject variability (Samek et al., 2013; Lotte, 2015). Many works on transfer learning for SMR-based BCI proposed the use of a very few training samples from the target subject (Kang et al., 2009; Lu et al., 2010; Kang and Choi, 2014; Fahimi et al., 2018; He and Wu, 2019). Recent studies have utilized resting EEG from the target subject incorporated into transfer learning model before proceeding to the actual experiment (Suk et al., 2014; Morioka et al., 2015). While time and effort for building those models could be significantly reduced, they still require training session. Others have recently demonstrated the feasibility of inter-subject BCI models without any training trial from the target subject (Saha et al., 2017a,b, 2019). However, the performance requires to be improved significantly prior to real-life use of such BCI systems.
A transfer learning method is worthwhile if the subjects share non-stationarities that can be modeled in an inter-subject context, but ineffective if the subjects exhibit unlike non-stationarities (Samek et al., 2013). The term inter-subject associativity refers to potential inter-subject BCI performance predictors, which could be incorporated into BCI design to augment transfer learning (Kang and Choi, 2014; Wronkiewicz et al., 2015; Saha et al., 2017a,b, 2019). Source-space analysis for detecting inter-subject associative EEG channels can improve SMR-based BCI performance (Wronkiewicz et al., 2015; Saha et al., 2017a, 2019). For example, the classification accuracies for two different subject pairs are 90.36±5.59% and 63.21±8.43%, respectively, suggesting not both subject pairs can be used to achieve a good performance (Saha et al., 2019).
A set of generalized BCI frameworks would be more feasible to implement as compared to a common BCI framework for all users. Because, it is evident to observe significant inter-subject variability in EEG signals (Saha et al., 2017b). Successful quantification of inter-subject associativity may suggest clustering of subjects, each cluster having subjects with EEG signal characteristics that are similar or can be interpreted in an inter-subject context. Considering the increasing volume of EEG-BCI databases, it may become feasible to quantify the exact sources of inter-subject/session variability as well as indicators of inter-subject associativity allowing to reduce training sessions to a minimum (Lotte, 2015). Recent advances in deep learning methods demonstrate a potential application that alleviates intra- and inter-subject variability in BCI settings (Chiarelli et al., 2018; Fahimi et al., 2018). Meanwhile, recent studies suggest that the quantification of inter-subject associativity could be equally important to increase the efficacy of exclusively machine learning-based transfer learning strategies for covariate shift adaptation (Kang et al., 2009; Kang and Choi, 2014; Wronkiewicz et al., 2015; Saha et al., 2017b, 2019; Perdikis et al., 2018).
5. Conclusion
Intra- and inter-subject variability is undeniable due to time-variant factors related to the experimental setting and underlying psychological and neurophysiological parameters. Besides the extensive use of transfer learning methods for the covariate shift adaptation, many recent works sought to find suitable psychological and neurological predictors for BCI performance. The assimilation of such predictors into a subject independent context may reduce or eliminate the tedious session or subject-specific training by supplementing the performance of existing transfer learning methods. However, collecting a priori information related to BCI performance predictors could be challenging. Inter-subject topographical associativity characterized by resting EEG could provide a viable alternative solution to reduce the calibration time to a minimum (Northoff et al., 2010; Suk et al., 2014; Morioka et al., 2015) assuming we understand the significance of intrinsic brain activities, i.e., resting EEG signals, and the role of RSN topographies on SMR-related brain functions.
Author Contributions
SS conceived the idea, prepared the figure and table, and wrote the first draft. MB reviewed and commented on the manuscript. SS and MB read and approved the final manuscript.
Conflict of Interest
The authors declare that the research was conducted in the absence of any commercial or financial relationships that could be construed as a potential conflict of interest.
References
Acqualagna, L., Botrel, L., Vidaurre, C., Kübler, A., and Blankertz, B. (2016). Large-scale assessment of a fully automatic co-adaptive motor imagery-based brain computer interface. PLoS ONE 11:e0148886. doi: 10.1371/journal.pone.0148886
Ahn, M., and Jun, S. C. (2015). Performance variation in motor imagery brain–computer interface: a brief review. J. Neuroscience Methods 243, 103–110. doi: 10.1016/j.jneumeth.2015.01.033
Allami, N., Paulignan, Y., Brovelli, A., and Boussaoud, D. (2008). Visuo-motor learning with combination of different rates of motor imagery and physical practice. Exp. Brain Res. 184, 105–113. doi: 10.1007/s00221-007-1086-x
Baldassarre, A., Lewis, C. M., Committeri, G., Snyder, A. Z., Romani, G. L., and Corbetta, M. (2012). Individual variability in functional connectivity predicts performance of a perceptual task. Proc. Natl. Acad. Sci. U.S.A. 109, 3516–3521. doi: 10.1073/pnas.1113148109
Betzel, R. F., and Bassett, D. S. (2017). Multi-scale brain networks. Neuroimage 160, 73–83. doi: 10.1016/j.neuroimage.2016.11.006
Betzel, R. F., Bertolero, M. A., Gordon, E. M., Gratton, C., Dosenbach, N. U., and Bassett, D. S. (2019). The community structure of functional brain networks exhibits scale-specific patterns of inter-and intra-subject variability. NeuroImage 202:115990. doi: 10.1016/j.neuroimage.2019.07.003
Birbaumer, N., Ghanayim, N., Hinterberger, T., Iversen, I., Kotchoubey, B., Kübler, A., et al. (1999). A spelling device for the paralysed. Nature 398:297.
Blankertz, B., Curio, G., and Müller, K.-R. (2002). “Classifying single trial eeg: towards brain computer interfacing,” in Advances in Neural Information Processing Systems, eds T. G. Dietterich, S. Becker, and Z. Ghahramani (Vancouver: MIT Press), 157–164.
Blankertz, B., Sanelli, C., Halder, S., Hammer, E., Kübler, A., Müller, K.-R., Curio, G., et al. (2009). Predicting bci performance to study bci illiteracy. BMC Neurosci. 10(Suppl. 1):P84. doi: 10.1186/1471-2202-10-S1-P84
Blankertz, B., Sannelli, C., Halder, S., Hammer, E. M., Kübler, A., Müller, K.-R., et al. (2010). Neurophysiological predictor of smr-based bci performance. Neuroimage 51, 1303–1309. doi: 10.1016/j.neuroimage.2010.03.022
Bradberry, T. J., Gentili, R. J., and Contreras-Vidal, J. L. (2010). Reconstructing three-dimensional hand movements from noninvasive electroencephalographic signals. J. Neurosci. 30, 3432–3437. doi: 10.1523/JNEUROSCI.6107-09.2010
Brown, R. E., and Milner, P. M. (2003). The legacy of donald o. hebb: more than the hebb synapse. Nat. Rev. Neurosci. 4, 1013–1019. doi: 10.1038/nrn1257.
Chiarelli, A. M., Croce, P., Merla, A., and Zappasodi, F. (2018). Deep learning for hybrid eeg-fnirs brain–computer interface: application to motor imagery classification. J. Neural Eng. 15:036028. doi: 10.1088/1741-2552/aaaf82
Clarke, D. D. (1999). “Circulation and energy metabolism of the brain,” Basic Neurochemistry: Molecular, Cellular, and Medical Aspects, eds G. J. Siegel, B. W. Agranoff, and R. W. Albers (Philadelphia: Lippincott-Raven), 637–670.
Darvishi, S., Gharabaghi, A., Boulay, C. B., Ridding, M. C., Abbott, D., and Baumert, M. (2017). Proprioceptive feedback facilitates motor imagery-related operant learning of sensorimotor β-band modulation. Front. Neurosci. 11:60. doi: 10.3389/fnins.2017.00060
Darvishi, S., Gharabaghi, A., Ridding, M. C., Abbott, D., and Baumert, M. (2018). Reaction time predicts brain–computer interface aptitude. IEEE J. Trans. Eng. Health Med. 6, 1–11. doi: 10.1109/JTEHM.2018.2875985
Deco, G., Jirsa, V. K., and McIntosh, A. R. (2011). Emerging concepts for the dynamical organization of resting-state activity in the brain. Nat. Rev. Neurosci. 12:43–56. doi: 10.1038/nrn2961
Dobkin, B. H. (2007). Brain–computer interface technology as a tool to augment plasticity and outcomes for neurological rehabilitation. J. Physiol. 579, 637–642. doi: 10.1113/jphysiol.2006.123067
Duarte, J. E., and Reinkensmeyer, D. J. (2015). Effects of robotically modulating kinematic variability on motor skill learning and motivation. J. Neurophysiol. 113, 2682–2691. doi: 10.1152/jn.00163.2014
Edelman, B., Meng, J., Suma, D., Zurn, C., Nagarajan, E., Baxter, B., et al. (2019). Noninvasive neuroimaging enhances continuous neural tracking for robotic device control. Sci. Robot. 4:eaaw6844. doi: 10.1126/scirobotics.aaw6844
Fahimi, F., Zhang, Z., Goh, W. B., Lee, T.-S., Ang, K. K., and Guan, C. (2018). Inter-subject transfer learning with end-to-end deep convolutional neural network for eeg-based bci. J. Neural Eng. 16:026007. doi: 10.1088/1741-2552/aaf3f6
Faller, J., Cummings, J., Saproo, S., and Sajda, P. (2019). Regulation of arousal via online neurofeedback improves human performance in a demanding sensory-motor task. Proc. Natl. Acad. Sci. U.S.A. 116, 6482–6490. doi: 10.1073/pnas.1817207116
Fazli, S., Dähne, S., Samek, W., Bießmann, F., and Mueller, K.-R. (2015). Learning from more than one data source: data fusion techniques for sensorimotor rhythm-based brain–computer interfaces. Proc. IEEE 103, 891–906. doi: 10.1109/JPROC.2015.2413993
Fox, K. C., Spreng, R. N., Ellamil, M., Andrews-Hanna, J. R., and Christoff, K. (2015). The wandering brain: meta-analysis of functional neuroimaging studies of mind-wandering and related spontaneous thought processes. Neuroimage 111, 611–621. doi: 10.1016/j.neuroimage.2015.02.039
Hammer, E. M., Halder, S., Blankertz, B., Sannelli, C., Dickhaus, T., Kleih, S., et al. (2012). Psychological predictors of smr-bci performance. Biol. Psychol. 89, 80–86. doi: 10.1016/j.biopsycho.2011.09.006
He, H., and Wu, D. (2019). Transfer learning for brain-computer interfaces: a euclidean space data alignment approach. IEEE Trans. Biomed. Eng. doi: 10.1109/TBME.2019.2913914. [Epub ahead of print].
Herzfeld, D. J., and Shadmehr, R. (2014). Motor variability is not noise, but grist for the learning mill. Nat. Neurosci. 17, 149–150. doi: 10.1038/nn.3633
Honey, C., Sporns, O., Cammoun, L., Gigandet, X., Thiran, J.-P., Meuli, R., et al. (2009). Predicting human resting-state functional connectivity from structural connectivity. Proc. Natl. Acad. Sci. U.S.A. 106, 2035–2040. doi: 10.1073/pnas.0811168106
Honey, C. J., Thivierge, J.-P., and Sporns, O. (2010). Can structure predict function in the human brain? Neuroimage 52, 766–776. doi: 10.1016/j.neuroimage.2010.01.071
Hong, X., Lu, Z. K., Teh, I., Nasrallah, F. A., Teo, W. P., Ang, K. K., et al. (2017). Brain plasticity following mi-bci training combined with tdcs in a randomized trial in chronic subcortical stroke subjects: a preliminary study. Sci. Rep. 7:9222. doi: 10.1038/s41598-017-08928-5
Jackson, P. L., Lafleur, M. F., Malouin, F., Richards, C., and Doyon, J. (2001). Potential role of mental practice using motor imagery in neurologic rehabilitation. Arch. Phys. Med. Rehabil. 82, 1133–1141. doi: 10.1053/apmr.2001.24286
Jayaram, V., Alamgir, M., Altun, Y., Scholkopf, B., and Grosse-Wentrup, M. (2016). Transfer learning in brain-computer interfaces. IEEE Comput. Intell. Magaz. 11, 20–31. doi: 10.1109/MCI.2015.2501545
Jensen, O., Bahramisharif, A., Oostenveld, R., Klanke, S., Hadjipapas, A., Okazaki, Y. O., et al. (2011). Using brain–computer interfaces and brain-state dependent stimulation as tools in cognitive neuroscience. Front. Psychol. 2:100. doi: 10.3389/fpsyg.2011.00100
Jeunet, C., NKaoua, B., Subramanian, S., Hachet, M., and Lotte, F. (2015). Predicting mental imagery-based bci performance from personality, cognitive profile and neurophysiological patterns. PLoS ONE 10:e0143962. doi: 10.1371/journal.pone.0143962
Jochumsen, M., Rovsing, C., Rovsing, H., Cremoux, S., Signal, N., Allen, K., et al. (2017). Quantification of movement-related eeg correlates associated with motor training: a study on movement-related cortical potentials and sensorimotor rhythms. Front. Hum. Neurosci. 11:604. doi: 10.3389/fnhum.2017.00604
Johnson, N., Carey, J., Edelman, B., Doud, A., Grande, A., Lakshminarayan, K., et al. (2018). Combined rtms and virtual reality brain–computer interface training for motor recovery after stroke. J. Neural Eng. 15:016009. doi: 10.1088/1741-2552/aa8ce3
Kang, H., and Choi, S. (2014). Bayesian common spatial patterns for multi-subject eeg classification. Neural Networks 57, 39–50. doi: 10.1016/j.neunet.2014.05.012
Kang, H., Nam, Y., and Choi, S. (2009). Composite common spatial pattern for subject-to-subject transfer. IEEE Signal Process. Lett. 16, 683–686. doi: 10.1109/LSP.2009.2022557
Kasahara, K., DaSalla, C. S., Honda, M., and Hanakawa, T. (2015). Neuroanatomical correlates of brain–computer interface performance. Neuroimage 110, 95–100. doi: 10.1016/j.neuroimage.2015.01.055
Krakauer, J. W. (2006). Motor learning: its relevance to stroke recovery and neurorehabilitation. Curr. Opin. Neurol. 19, 84–90. doi: 10.1097/01.wco.0000200544.29915.cc
Krusienski, D. J., Grosse-Wentrup, M., Galán, F., Coyle, D., Miller, K. J., Forney, E., et al. (2011). Critical issues in state-of-the-art brain–computer interface signal processing. J. Neural Eng. 8:025002. doi: 10.1088/1741-2560/8/2/025002
Leamy, D. J., Kocijan, J., Domijan, K., Duffin, J., Roche, R. A., Commins, S., et al. (2014). An exploration of eeg features during recovery following stroke–implications for bci-mediated neurorehabilitation therapy. J. Neuroeng. Rehabil. 11:9. doi: 10.1186/1743-0003-11-9
Li, Q., Lu, Z., Gao, N., and Yang, J. (2019). Optimizing the performance of the visual p300-speller through active mental tasks based on color distinction and modulation of task difficulty. Front. Human Neurosci. 13:130. doi: 10.3389/fnhum.2019.00130
Li, Y., Kambara, H., Koike, Y., and Sugiyama, M. (2010). Application of covariate shift adaptation techniques in brain–computer interfaces. IEEE Trans. Biomed. Eng. 57, 1318–1324. doi: 10.1109/TBME.2009.2039997
Lotte, F. (2015). Signal processing approaches to minimize or suppress calibration time in oscillatory activity-based brain–computer interfaces. Proc. IEEE 103, 871–890. doi: 10.1109/JPROC.2015.2404941
Lotze, M., Flor, H., Grodd, W., Larbig, W., and Birbaumer, N. (2001). Phantom movements and pain an fmri study in upper limb amputees. Brain 124, 2268–2277. doi: 10.1093/brain/124.11.2268
Lotze, M., and Halsband, U. (2006). Motor imagery. J. Physiol. Paris 99, 386–395. doi: 10.1016/j.jphysparis.2006.03.012
Lu, H., Eng, H.-L., Guan, C., Plataniotis, K. N., and Venetsanopoulos, A. N. (2010). Regularized common spatial pattern with aggregation for eeg classification in small-sample setting. IEEE Trans. Biomed. Eng. 57, 2936–2946. doi: 10.1109/TBME.2010.2082540
Meyer, M. C., van Oort, E. S., and Barth, M. (2013). Electrophysiological correlation patterns of resting state networks in single subjects: a combined eeg–fmri study. Brain Topography 26, 98–109. doi: 10.1007/s10548-012-0235-0
Morioka, H., Kanemura, A., Hirayama, J.-I., Shikauchi, M., Ogawa, T., Ikeda, S., et al. (2015). Learning a common dictionary for subject-transfer decoding with resting calibration. NeuroImage 111, 167–178. doi: 10.1016/j.neuroimage.2015.02.015
Müller-Putz, G. R., Daly, I., and Kaiser, V. (2014). Motor imagery-induced eeg patterns in individuals with spinal cord injury and their impact on brain–computer interface accuracy. J. Neural Eng. 11:035011. doi: 10.1088/1741-2560/11/3/035011
Niazi, I. K., Jiang, N., Jochumsen, M., Nielsen, J. F., Dremstrup, K., and Farina, D. (2013). Detection of movement-related cortical potentials based on subject-independent training. Med. Biol. Eng. Comput. 51, 507–512. doi: 10.1007/s11517-012-1018-1
Northoff, G., Duncan, N. W., and Hayes, D. J. (2010). The brain and its resting state activity-experimental and methodological implications. Progress Neurobiol. 92, 593–600. doi: 10.1016/j.pneurobio.2010.09.002
Orsborn, A. L., Moorman, H. G., Overduin, S. A., Shanechi, M. M., Dimitrov, D. F., and Carmena, J. M. (2014). Closed-loop decoder adaptation shapes neural plasticity for skillful neuroprosthetic control. Neuron 82, 1380–1393. doi: 10.1016/j.neuron.2014.04.048
Ostry, D. J., and Gribble, P. L. (2016). Sensory plasticity in human motor learning. Trends Neurosci. 39, 114–123. doi: 10.1016/j.tins.2015.12.006
Perdikis, S., Tonin, L., Saeedi, S., Schneider, C., and Millán, J. d. R. (2018). The cybathlon bci race: successful longitudinal mutual learning with two tetraplegic users. PLoS Biol. 16:e2003787. doi: 10.1371/journal.pbio.2003787
Raichle, M. E. (2009). A paradigm shift in functional brain imaging. J. Neurosci. 29, 12729–12734. doi: 10.1523/JNEUROSCI.4366-09.2009
Raichle, M. E. (2010). Two views of brain function. Trends Cognit. Sci. 14, 180–190. doi: 10.1016/j.tics.2010.01.008
Ramoser, H., Muller-Gerking, J., and Pfurtscheller, G. (2000). Optimal spatial filtering of single trial eeg during imagined hand movement. IEEE Trans. Rehabil. Eng. 8, 441–446. doi: 10.1109/86.895946
Ramos-Murguialday, A., Broetz, D., Rea, M., Läer, L., Yilmaz, Ö., Brasil, F. L., et al. (2013). Brain–machine interface in chronic stroke rehabilitation: a controlled study. Ann. Neurol. 74, 100–108. doi: 10.1002/ana.23879
Reichert, J. L., Kober, S. E., Neuper, C., and Wood, G. (2015). Resting-state sensorimotor rhythm (smr) power predicts the ability to up-regulate smr in an eeg-instrumental conditioning paradigm. Clin. Neurophysiol. 126, 2068–2077. doi: 10.1016/j.clinph.2014.09.032
Ruffino, C., Papaxanthis, C., and Lebon, F. (2017). Neural plasticity during motor learning with motor imagery practice: Review and perspectives. Neuroscience 341, 61–78. doi: 10.1016/j.neuroscience.2016.11.023
Saha, S., Ahmed, K. I., Mostafa, R., Khandoker, A. H., and Hadjileontiadis, L. (2017a). Enhanced inter-subject brain computer interface with associative sensorimotor oscillations. Healthcare Technol. Lett. 4, 39–43. doi: 10.1049/htl.2016.0073
Saha, S., Ahmed, K. I. U., Mostafa, R., Hadjileontiadis, L., and Khandoker, A. (2017b). Evidence of variabilities in eeg dynamics during motor imagery-based multiclass brain–computer interface. IEEE Trans. Neural Syst. Rehabil. Eng. 26, 371–382. doi: 10.1109/TNSRE.2017.2778178
Saha, S., Hossain, M. S., Ahmed, K., Mostafa, R., Hadjileontiadis, L., Khandoker, A., et al. (2019). Wavelet entropy-based inter-subject associative cortical source localization for sensorimotor BCI. Front. Neuroinform. 13:47. doi: 10.3389/fninf.2019.00047
Samek, W., Meinecke, F. C., and Müller, K.-R. (2013). Transferring subspaces between subjects in brain–computer interfacing. IEEE Trans. Biomed. Eng. 60, 2289–2298. doi: 10.1109/TBME.2013.2253608
Sannelli, C., Vidaurre, C., Müller, K.-R., and Blankertz, B. (2019). A large scale screening study with a smr-based bci: Categorization of bci users and differences in their smr activity. PLoS ONE 14:e0207351. doi: 10.1371/journal.pone.0207351
Seghier, M. L., and Price, C. J. (2018). Interpreting and utilising intersubject variability in brain function. Trends Cognit. Sci. 22, 517–530. doi: 10.1016/j.tics.2018.03.003
Selfslagh, A., Shokur, S., Campos, D. S., Donati, A. R., Almeida, S., Yamauti, S. Y., et al. (2019). Non-invasive, brain-controlled functional electrical stimulation for locomotion rehabilitation in individuals with paraplegia. Sci. Rep. 9:6782. doi: 10.1038/s41598-019-43041-9
Singh, P., Jana, S., Ghosal, A., and Murthy, A. (2016). Exploration of joint redundancy but not task space variability facilitates supervised motor learning. Proc. Natl. Acad. Sci. U.S.A. 113, 14414–14419. doi: 10.1073/pnas.1613383113
Smith, S., Duff, E., Groves, A., Nichols, T. E., Jbabdi, S., Westlye, L. T., et al. (2019). Structural variability in the human brain reflects fine-grained functional architecture at the population level. J. Neurosci. 39, 6136–6149. doi: 10.1523/JNEUROSCI.2912-18.2019
Suk, H.-I., Fazli, S., Mehnert, J., Müller, K.-R., and Lee, S.-W. (2014). Predicting BCI subject performance using probabilistic spatio-temporal filters. PLoS ONE 9:e87056. doi: 10.1371/journal.pone.0087056
Tomassini, V., Jbabdi, S., Kincses, Z. T., Bosnell, R., Douaud, G., Pozzilli, C., et al. (2011). Structural and functional bases for individual differences in motor learning. Human Brain Mapp. 32, 494–508. doi: 10.1002/hbm.21037
Úbeda, A., Hortal, E., Iáñez, E., Perez-Vidal, C., and Azorín, J. M. (2015). Assessing movement factors in upper limb kinematics decoding from eeg signals. PLoS ONE 10:e0128456. doi: 10.1371/journal.pone.0128456
Vallence, A.-M., Goldsworthy, M. R., Hodyl, N. A., Semmler, J. G., Pitcher, J. B., and Ridding, M. C. (2015). Inter-and intra-subject variability of motor cortex plasticity following continuous theta-burst stimulation. Neuroscience 304, 266–278. doi: 10.1016/j.neuroscience.2015.07.043
Vasilyev, A., Liburkina, S., Yakovlev, L., Perepelkina, O., and Kaplan, A. (2017). Assessing motor imagery in brain-computer interface training: psychological and neurophysiological correlates. Neuropsychologia 97, 56–65. doi: 10.1016/j.neuropsychologia.2017.02.005
Vidaurre, C., and Blankertz, B. (2010). Towards a cure for bci illiteracy. Brain Topography 23, 194–198. doi: 10.1007/s10548-009-0121-6
Vyas, S., Even-Chen, N., Stavisky, S. D., Ryu, S. I., Nuyujukian, P., and Shenoy, K. V. (2018). Neural population dynamics underlying motor learning transfer. Neuron 97, 1177–1186. doi: 10.1016/j.neuron.2018.01.040
Wang, W., Collinger, J. L., Perez, M. A., Tyler-Kabara, E. C., Cohen, L. G., Birbaumer, N., et al. (2010). Neural interface technology for rehabilitation: exploiting and promoting neuroplasticity. Phys. Med. Rehabil. Clinics 21, 157–178. doi: 10.1016/j.pmr.2009.07.003
Wang, Y., and Jung, T.-P. (2011). A collaborative brain-computer interface for improving human performance. PLoS ONE 6:e20422. doi: 10.1371/journal.pone.0020422
Wens, V., Bourguignon, M., Goldman, S., Marty, B., De Beeck, M. O., Clumeck, C., et al. (2014). Inter-and intra-subject variability of neuromagnetic resting state networks. Brain Topography 27, 620–634. doi: 10.1007/s10548-014-0364-8
Wolpaw, J. R., and McFarland, D. J. (1994). Multichannel eeg-based brain-computer communication. Electroencephal. Clin. Neurophysiol. 90, 444–449.
Wolpaw, J. R., McFarland, D. J., Neat, G. W., and Forneris, C. A. (1991). An eeg-based brain-computer interface for cursor control. Electroencephal. Clin. Neurophysiol. 78, 252–259.
Wronkiewicz, M., Larson, E., and Lee, A. K. (2015). Leveraging anatomical information to improve transfer learning in brain–computer interfaces. J. Neural Eng. 12:046027. doi: 10.1088/1741-2560/12/4/046027
Wu, H. G., Miyamoto, Y. R., Castro, L. N. G., Ölveczky, B. P., and Smith, M. A. (2014). Temporal structure of motor variability is dynamically regulated and predicts motor learning ability. Nat. Neurosci. 17, 312–321. doi: 10.1038/nn.3616
Zhang, R., Yao, D., Valdés-Sosa, P. A., Li, F., Li, P., Zhang, T., et al. (2015). Efficient resting-state eeg network facilitates motor imagery performance. J. Neural Eng. 12:066024. doi: 10.1088/1741-2560/12/6/066024
Zhao, X., Chu, Y., Han, J., and Zhang, Z. (2016). Ssvep-based brain–computer interface controlled functional electrical stimulation system for upper extremity rehabilitation. IEEE Trans. Syst. Man Cybernet. Syst. 46, 947–956. doi: 10.1109/TSMC.2016.2523762
Keywords: electroencephalography, brain computer interface, sensorimotor rhythms, transfer learning, inter-subject associativity
Citation: Saha S and Baumert M (2020) Intra- and Inter-subject Variability in EEG-Based Sensorimotor Brain Computer Interface: A Review. Front. Comput. Neurosci. 13:87. doi: 10.3389/fncom.2019.00087
Received: 21 October 2019; Accepted: 16 December 2019;
Published: 21 January 2020.
Edited by:
Chun-Shu Wei, National Chiao Tung University, TaiwanReviewed by:
Bradley Jay Edelman, School of Medicine, Stanford University, United StatesPo-Chih Kuo, Massachusetts Institute of Technology, United States
Yu-Kai Wang, University of Technology Sydney, Australia
Copyright © 2020 Saha and Baumert. This is an open-access article distributed under the terms of the Creative Commons Attribution License (CC BY). The use, distribution or reproduction in other forums is permitted, provided the original author(s) and the copyright owner(s) are credited and that the original publication in this journal is cited, in accordance with accepted academic practice. No use, distribution or reproduction is permitted which does not comply with these terms.
*Correspondence: Simanto Saha, c2ltYW50by5zYWhhQGllZWUub3Jn; Mathias Baumert, bWF0aGlhcy5iYXVtZXJ0QGFkZWxhaWRlLmVkdS5hdQ==