- 1 Department of Information Engineering and Computer Science, University of Trento, Trento, Italy
- 2 European Alliance for Innovation, Gent, Belgium
- 3 Service Automation and Integration Lab, HP Labs, Palo Alto, CA, USA
- 4 Artificial Intelligence Research Institute (IIIA-CSIC), Barcelona, Catalonia, Spain
- 5 Exprivia SpA, Molfetta, Italy
- 6 Peerevaluation.org, Paris, France
In this paper we review several novel approaches for research evaluation. We start with a brief overview of the peer review, its controversies, and metrics for assessing efficiency and overall quality of the peer review. We then discuss five approaches, including reputation-based ones, that come out of the research carried out by the LiquidPub project and research groups collaborated with LiquidPub. Those approaches are alternative or complementary to traditional peer review. We discuss pros and cons of the proposed approaches and conclude with a vision for the future of the research evaluation, arguing that no single system can suit all stakeholders in various communities.
1. Introduction
Formal peer review of one kind or another has been part of the scientific publishing process since at least the eighteenth century (Kronick, 1990). While the precise norms and practices of review have varied extensively by historical period and by discipline (Burnham, 1990; Spier, 2002), key themes have remained consistent: a concern for ensuring the correctness of work and not allowing demonstrably false claims to distort the literature; the need for authors to have their work certified as valid; the reputation of the society, publisher, or editorial board responsible for the work; and at the same time, concern to not inhibit the introduction of valuable new ideas. Particularly with the increasing volume of publication through the twentieth century, the process has become an almost unavoidable necessity in determining what out of a huge range of submissions should be selected to appear in the limited (and costly) number of pages of the most prominent journals (Ingelfinger, 1974). One consequence of this competition for reader attention has been that reviewers are increasingly being asked to assess not just the technical correctness of work but also to make essentially editorial assessments such as the topical suitability and potential impact or importance of a piece of work (Lawrence, 2003).
Different practices for the evaluation of knowledge have been proposed and applied by the scientific community, including but not limited to single-blind review (where reviewers remain anonymous, but author identity is known to the reviewer); double-blind review (where the identities of both authors and reviewers are hidden); and open peer review where both authors and reviewers are aware of each other’s identity. Journal editors also have an important role, both in the initial assessment of whether to send a manuscript for review and in terms of management and final decision-making on the basis of reviewer recommendations; the precise degree of editor- versus reviewer-based selection can vary greatly between different publications (McCook, 2006). Yet despite its modern ubiquity, and a broad consensus among scientists upon its essential contribution to the research process (Ware and Monkman, 2008; Sense About Science, 2009), there are also widespread concerns about the known or perceived shortcomings of the review process: bias and inconsistency, ineffective filtering of error or fraud, and the suppression of innovation.
In this paper we discuss various models that offer complementary or replacement evaluation mechanisms to the traditional peer review process. The next section provides a brief overview of the conventional peer review process and its controversies, including a review of studies and analyses of peer review and reviewer behavior across a range of disciplines and review practices. This is followed by a review of a number of quantitative metrics to assess the overall quality and efficiency of peer review processes, to check the robustness of the process, the degree of agreement among and bias of the reviewers, and to check the ability of reviewers to predict the impact of papers in subsequent years.
We then proceed to introduce a number of different experiments in peer review, including comparisons between quick ranking of papers, bidding to review papers, and reviewing them in the traditional manner. We also discuss two approaches to research evaluation that are based on leveraging on the explicit or implicit feedback of the scientific community: OpinioNet and UCount. We conclude the paper with a discussion of the pros and cons of the presented approaches and our vision for the future of the research evaluation.
2. Peer Review History and Controversies
Review processes of one kind or another have been part of scientific publication since the first scientific journals – notably the Philosophical Transactions of the Royal Society – with the first formally defined peer review process being that of the journal Medical Essays and Observations, published in 1731 by the Royal Society of Edinburgh (Kronick, 1990). While historical practice varied greatly (Burnham, 1990), the growth of the scientific literature in the twentieth century has seen peer review become almost universal, being widely seen as the key evaluation mechanism of scholarly work (Ingelfinger, 1974; Ware and Monkman, 2008; Sense About Science, 2009).
Despite this ubiquity of the practice (or perhaps more properly, of a great diversity of practices coming under the same name), peer review has been little studied by scientists until the last decades. The results of these studies are perhaps surprising, being as they are often very equivocal about whether peer review really fulfills its supposed role as a gatekeeper for error correction and selection of quality work (Jefferson et al., 2007). A significant number of papers report that peer review is a process whose effectiveness “is a matter of faith rather than evidence” (Smith, 2006), that is “untested” and “uncertain” (Jefferson et al., 2002b), and that we know very little about its real effects because scientists are rarely given access to the relevant data.
For example, Lock (1994) claims that peer review can at most help detect major errors and that the real criterion for judging a paper is to look at how often its content is used and referred to several years after publication. Other experimental studies cast doubt on the ability of peer review to spot important errors in a paper (Godlee et al., 1998). At the same time, peer review is still considered a process to which no reasonable alternatives have been found (Kassirer and Campion, 1994; Smith, 2006).
Part of the problem is that the practice and goals of peer review can vary greatly by discipline and journal. Studies on peer review differ in the kind and amount of data available and use different metrics to analyze its effectiveness. Indeed, having precise objectives for the analysis is one of the key and hardest challenges as it is often unclear and debatable to define what it means for peer review to be effective (Jefferson et al., 2002a). Nevertheless, in general we can divide the metrics used into two groups: those aiming to determine the effectiveness or validity or peer review (discussed below), and those aiming at measuring what authors consider to be “good” properties of peer review (discussed in Section 3).
The first category of studies can itself broadly be divided into two categories: those testing the ability of peer review to detect errors, and those measuring reviewers’ ability to anticipate the future impact of work, usually measured using citation count.
Where error detection is concerned, a study was conducted by Goodman et al. (1994) who studied 111 manuscripts submitted to the Annals of Internal Medicine between March 1992 and March 1993. They studied the papers before and after the peer review process in order to find out whether peer review was able to detect errors. They did not find any substantial difference in the manuscripts before and after publication. Indeed, they state that peer review was able to detect only small flaws in the papers, such as figures, statistics, and description of the results. An interesting study was carried out by Godlee et al. (1998), who introduced deliberate errors in a paper already accepted by the British Medical Journal (BMJ)1 and asked 420 reviewers divided in 5 different groups to review the paper. Groups 1 and 2 did not know the identity of the authors, while 3 and 4 knew it. Groups 1 and 3 were asked to sign their reports, while 2 and 4 were asked to return their reports unsigned. The only difference between groups 4 and 5 was that reviewers from group 5 were aware that they were taking part in a study. Godlee et al. (1998) report that the mean number of major errors detected was 2 out of a total of 8, while there were 16% of reviewers that did not find any mistake, and 33% of reviewers went for acceptance despite the introduced mistakes. Unfortunately, the study does not report on whether the reviewers collectively identified all the errors (which might lend support to some of the community review processes discussed later in this article) or whether certain errors were noticed more often than others.
Citation count has been used extensively as a metric in studies by Bornmann and Daniel. The first of these reports on whether peer review committees are effective in selecting people that have higher citation statistics, and finds that there is indeed such a correlation (Bornmann and Daniel, 2005b). A later paper examines the initial assessments by staff editors of manuscripts submitted to a major chemistry journal, compared to the later assessments by external reviewers (Bornmann and Daniel, 2010a): where editors make an actual assessment this is indeed correlated with final citation count, but in 2/3 of cases they were unable or unwilling to venture an opinion. Final assessments after peer review were much more strongly correlated with final citation count, implying a positive effect whether or not editors were able to reach an initial decision. These results can be compared to those of Opthof et al. (2002) on submissions to a medical journal, where editors’ initial ratings were uncorrelated with later citation count, while external reviewers’ ratings were correlated, more strongly so where more reviewers were employed. The best predictive value, however, was a combination of reviewers’ and editors’ ratings, suggesting that differences in prediction ability are down to editors and reviewers picking up on different aspects of article quality.
3. Quantitative Analyses of Peer Review
In this section we review research approaches dealing with quantitative analysis of peer review. Effectiveness or validity of peer review can be measured taking into account different metrics, included but not limited to: ability to predict the future position of the paper in the citation ranking, the disagreement between reviewers, the bias of a reviewer.
An obvious quantitative analysis is to measure the correlation between reviewers’ assessments of manuscripts and their later impact, most readily measured by citation. As discussed in the previous section, results may be highly dependent on the particular context. For example, Bornmann and Daniel (2010b), studying a dataset of 1899 submissions to the Angewandte Chemie International Edition, found a positive correlation between reviewers’ recommendations and the later citation impact – with, interestingly, a stronger correlation where fewer reviewers were used2. On the other hand, Ragone et al. (2011), studying a large dataset of 9000 reviews covering circa 3000 submissions to 10 computer science conferences, observed few statistically significant correlations when the ranking of papers according to reviewer ratings was compared to the ranking according to citation3.
Another important metric for the peer review process is the inter-reviewer agreement (Casati et al., 2010), which measures how much the marks given by reviewers to a contribution differ. The rationale behind this metric is that while reviewers’ perspectives may differ according to background, areas of expertise and so on, we may expect there to be some degree of consensus among them on the core virtues (or lack thereof) of an article. If on the other hand the marks given by reviewers are comparable to marks given at random, then the results of the review process are also effectively random, which defeats its purpose. There are several reasons for having several reviewers per contribution: to evaluate based on consensus or majority opinion and to provide multiple expertise (e.g., having a more methodological reviewer and two more content reviewers).
Indeed, having a high disagreement value means, in some way, that the judgment of the involved peers is not sufficient to state the value of the contribution itself. This metric could be useful to improve the quality of the review process as could help to decide, based on the disagreement value, if three reviewers are enough to judge a contribution or if more reviewers are needed in order to ensure the quality of the process.
A significant portion of the research on peer review focuses on identifying reviewer biases and understanding their impact in the review process. Indeed, reviewers’ objectivity is often considered a fundamental quality of a review process: “the ideal reviewer,” notes Ingelfinger (1974), “should be totally objective, in other words, supernatural.” Approaches for analyzing bias in peer reviews identified several kinds of bias: affiliation bias, meaning that researchers from prominent institutions are favored in peer review (Ceci and Peters, 1982); bias in favor of US-based researchers (Link, 1998), gender bias against female researchers (Wenneras and Wold, 1997; Bornmann, 2007; Marsh et al., 2009; Ceci and Williams, 2011) and order bias (Bornmann and Daniel, 2005a), meaning that reviewing applications for doctoral and post-doctoral research scholarship in alphabetic order may favor those applicants having names at the beginning of the alphabet. Although it is not always easy to decouple these apparent biases from other factors such as quality differentials, at least some biases, such as those based on nationality of reviewers and authors, remain even when quality is taken into account (Lee et al., 2006; Lynch et al., 2007). Others, such as bias in favor of statistically significant results (Olson et al., 2002; Lee et al., 2006) or gender biases (Marsh et al., 2009; Ceci and Williams, 2011), appear to be down primarily to other factors than the review process itself. In addition, it is possible to compute the rating bias, i.e., reviewers consistently giving higher or lower marks, independently from the quality of the specific contribution they have to assess, which is a kind of bias that appears rather often, is easy to detect, and that can be corrected with rather simple procedures to improve the fairness of the review process (Ragone et al., 2011).
One of the ways to identify bias is to compare single- and double-blind review. Single-blind review provides anonymity to the reviewers and is used to protect the reviewers from author reprisals. In many research fields, single-blind review is the normative practice. However, in others, such as information systems, or at Association for Computing Machinery Special Interest Group on Management of Data (ACM SIGMOD) conferences, double-blind review, where identities of both authors and reviewers are hidden, is the norm. The purpose of the double-blind review is to help the reviewers to assess only scientific achievements of the paper, not taking into consideration other factors and therefore to be unbiased.
Analyses of the merit of the double-blind review process are somewhat equivocal. Early studies by McNutt et al. (1990) and Fisher et al. (1994) on double-blind review of journal submissions reported a positive effect on review quality as rated by editors, although the latter study may have been influenced by the fact that blinded reviewers knew they were taking part in a study while non-blinded reviewers did not. A later and much larger study by Justice et al. (1998), where all reviewers knew they were taking part in a study, revealed no statistically significant difference, while another by van Rooyen et al. (1999) including both informed and uninformed reviewers suggested no difference due to either the review style (single- or double-blind) or reviewer knowledge of whether they were partaking in a study. On the other hand an extensive study of abstract submissions to medical conferences by Ross et al. (2006) suggested that double-blind review was successful in eliminating a host of biases related to gender, nationality, prestige, and other factors.
One major factor that may explain these contradictory results is the question of whether the masking of author identity is actually successful, as authors frequently include identifying elements in their papers such as citations to their previous work (Cho et al., 1998; Katz et al., 2002). The likelihood of such accidental unblinding may be larger for extensive works like journal submissions, making it more difficult for double-blind review to succeed compared to shorter works such as abstracts. Unblinding rates vary widely between journals, and it may be that volume of submissions and the size of the contributing community also affect how easy it is to identify authors (Ross et al., 2006). It may also be possible for authors to identify reviewers from their comments. Potential positive effects of successfully blinded review may therefore be difficult to secure in practice.
Research on open peer review (where the reviewer’s name is known to the authors) is at present very limited. Initial studies showed that open reviews were of higher quality, were more courteous and reviewers spent typically more time to complete them (Walsh et al., 2000). An example of the open peer review, adopted mainly by *PLoP4 conferences, is shepherding, where a shepherd (reviewer) works together with the sheep (authors) on improving the paper. The major problem of open peer review is combating the unwillingness of some potential reviewers to agree to their identity being revealed (Ware and Monkman, 2008), although journals that have implemented open review have reported good experiences in practice (Godlee, 2002).
Research shows that to improve the peer review process, sometimes paying attention to details is enough. For instance, the mark scale can influence reviewers and lead them to use only specific marks, instead of the whole scale (Casati et al., 2010; Medo and Wakeling, 2010). It has been shown that in the scale from 1 to 5 with half-marks, reviewers tend to not use half-marks, while in the same scale without half-marks (1 to 10) reviewers use the entire scale to rate (Casati et al., 2010). In a scale from 1 to 7, reviewers’ marks tend to concentrate in the middle (Casati et al., 2010).
One of the main issues in peer review analysis is to have access to the data. Usually, works on peer review are restricted to analyzing only 1-2 conferences, grant applications processes or fellowships. Just to name a few: Reinhart (2009) analyzed 496 applications for project-base funding; Bornmann and Daniel (2005a) studied the selection process of 1,954 doctoral and 743 post-doctoral applications for fellowships; Bornmann et al. (2008) analyzed 668 applications for funding; Godlee et al. (1998) involved in their experiments 420 reviewers from the journal’s database; Goodman et al. (1994) analyzed 111 manuscripts accepted for publication. As already mentioned above, one of the largest datasets has been used in the work by Ragone et al. (2011) where they collected data from 10 conferences, for a total of 9032 reviews, 2797 submitted contributions and 2295 reviewers.
4. Experiments in Peer Review
Nowadays, scientists and editors are exploring alternative approaches to tackle some of the pervasive problems with traditional peer review (Akst, 2010). Alternatives include enabling authors to carry reviews from one journal to another (Akst, 2010), posting reviewer comments alongside the published paper5, or running the traditional peer review process simultaneously with a public review (Akst, 2010). The ACM SIGMOD conference has also experimented with variations of the classical peer review model where papers are evaluated in two phases, where the first phase filters out papers that are unlikely to be accepted allowing to focus the reviewers’ effort on a more limited set of papers. In Casati et al. (2010) authors provide a model for multi-phase review that can improve the peer review process in the sense of reducing the review effort required to reach a decision on a set of submitted papers while keeping the same quality of results.
In the following we focus on three experimental approaches for peer review: asking reviewers to rank papers instead of reviewing them, bidding for reviewing a paper, and open evaluation of research works.
4.1. Experiment on Ranking Papers vs Reviewing
For the Institute of Electrical and Electronics Engineers Business-Driven IT Management Workshop (IEEE BDIM) in 2010, the Technical Program Committee (TPC) chairs experimented with a “wisdom of the crowd” approach to selecting papers. The aim of the experiment was to assess the viability of an alternate selection mechanism where (some of the) reviewers can rank papers based on a quick read rather than providing an in-depth review with quality scores.
This is the process they followed:
• The TPC members were asked to split into two roughly equal-size groups: (a) “wisdom of the crowd” and (b) “traditional,” TPC chairs completed the split for those TPC member who did not reply or were indifferent6.
• TPC members obviously knew which group they were in, but had no direct knowledge of other members’ placement.
• Group (b) carried out the usual 3–4 traditional reviews.
• At the end of the review phase, reviews from group (b) were averaged as usual, resulting in a total order of all papers submitted.
• Group (a) got assigned a PDF containing all submissions (excluding conflicts of interest) with no author information, thus we followed double-blind review process.
• Group (a) was required to provide a total order of all (or most) of the papers submitted, spending no more than 3–5 min on each paper.
• They TPC chairs merged the lists giving equal weight to each, and the top papers were divided into tiers (extended presentation, regular presentation, short presentation, posters, rejected) according to the harmonized ordered list. TCP chairs performed tie-break where necessary.
• Authors received feedback containing
– Acceptance/rejection;
– Tier of acceptance if applicable (extended, regular, short, poster);
– Full explanation of the review process;
– at least 3 reviews for their submission;
– their paper’s rank in the traditional review process, and its rank in the “wisdom of the crowd” process.
Interesting findings were:
(1) reviewers split evenly between the two groups, with exactly half of the TPC choosing the “wisdom of the crowd” approach, and half choosing the traditional
(2) for selection, there were three traditional reviewers for each paper, so the TPC chairs counted the score from the wisdom of the crowd ranking with a weight equal to three reviewers. They transformed the ranking into a score by averaging ranks over all the reviewers, and normalizing linearly the average rank onto the range of scores of the traditional reviews
(3) results were such that the top three papers and the bottom four papers were identical for both the traditional and the fast ranking review. However, for the selection of the papers in the middle, the TPC chairs had to take into account not only review scores, but also the review content, and give more weight to more experienced reviewers. For the submissions falling in the in-between category, the wisdom of the crowd did not help, and it was mostly off what the end selection wound up being.
In conclusion, the experiment showed that fast ranking in the wisdom of the crowd approach could be applied to get to a fast selection of the top and bottom submissions. However, that does not help in selecting the papers that fall in-between these categories.
4.2. e-Scripts: Bidding for Reviewing
Most researchers maintain a strong preference for peer review as the key mechanism of research evaluation (Ware and Monkman, 2008; Sense About Science, 2009). A major motivating factor here is the ability of peer review not just to assess or filter work but to help improve it prior to publication (Goodman et al., 1994; Purcell et al., 1998; Sense About Science, 2009), and many researchers consider this opportunity to help their fellow scientists to be one of the key pleasures of contributing reviews (Sense About Science, 2009).
By contrast, some of the major frustrations of authors (and editors) with the review process relate to those occasions when the reviewer is unmotivated or unfamiliar with the subject matter. At conferences (e.g., at EuroPLoP), this factor is often dealt with by allowing members of the technical program committee to bid to review submissions on the basis of titles and abstracts. In this way, every program committee member can hope to have a paper to review which meets their interests and areas of expertise. The role of the program chair is also made easier, with less work to do in assigning referees to articles.
The e-Scripts submissions management system7, developed by the Institute for Computer Sciences, Social Informatics and Telecommunications Engineering (ICST) and the European Alliance for Innovation (EAI), attempts to bring the same principles and benefits to the peer review process of research journals. Titles and abstracts of submitted articles are posted publicly online after submission, and for a period of about 2 weeks thereafter interested readers can bid to review those which catch their fancy. At the end of the public bidding period, the editor approves an ordered list of candidate reviewers based on a mix of bidders, author- and editor-nominated candidates, and reviewer invitations are sent out automatically starting from the top of the list.
The aim here is principally to engage with the enthusiasm and willingness to help that motivate good reviewers, while not relying on it: as opposed to some unsuccessful attempts at community review (Greaves et al., 2006), the Editors still have a responsibility to nominate and secure reviewers, with bidding acting as a supplemental rather than replacement selection process. In addition the system maintains a level of confidentiality for unpublished work, with the journal Editor still controlling access.
Beyond improving the quality of individual reviews, this approach has the capacity to generate additional data to support editorial decision-making. First, just as early download statistics offer a reliable precursor of later citation impact (Brody et al., 2006), so we can anticipate bidding intensity to reflect the potential importance of a submitted article. Second, correlations in user bidding can be used to build a profile of reviewer interests that can help automate the process of reviewer nomination. This, together with other means of assessing and ranking potential reviewers, is the subject of EAI’s UCount project, which is discussed in Section 5.2.
4.3. Peerevaluation.org: Scientific Trust in the Social Web
For the Millennial generation, sharing, reviewing, disseminating, and receiving immediate feedback have become not only natural practices but also strong expectations. For almost a billion Facebook users, both practices and expectations are fully embedded in the daily flows of consumption, communication, entertainment, information, work, and access to knowledge.
4.3.1. The advent of social reputation
On the Social Web, all are empowered to become, all at once, producers, reviewers, disseminators, and consumers. With such empowerment and shuffling of roles, it is only logical that alternative mechanisms of reputation building would also emerge.
4.3.2. The story of John
John composed a song, uploaded it on YouTube and sent it to his friends. The song became a hit and triggered exponential viral dissemination. John has now a reputation as a composer and has built a network of 500,000 thousand listeners, fans, and reviewers. In John’s story, music publishers, distributors, and journalists had no implications in the realization of his endeavors. John relied on social dissemination, reviewing, and social reputation building. He was then offered a contract by a music label, which he chose to accept, for greater dissemination and recognition.
4.3.3. The story of Sophie
John’s younger sister, Sophie, is a neurobiologist who just defended her Ph.D. Sophie is as Web savvy as John and expects her career to be just as fluid. Sophie knows that her future as a researcher will depend on her capacity to contribute to neurobiology with original and valid methods and results, and sufficient funding. To convince research funding agencies, all that Sophie needs is a method to certify that her research projects are valuable to neurobiology and that her methods and results are valid. Sophie is of course aware that she could publish articles in peer reviewed journals to give tokens of trust to such agencies but, having knowledge of John’s experience, she is disappointed by the slowness of the peer reviewing process, publishing costs and the complex and opaque mechanisms of scientific reputation and impact measures. Indeed, like John, Sophie values empowerment, immediacy, transparency, and qualitative appreciation of her work, as opposed to automated and quantitative measures of her impact.
4.3.4. Sophie’s world
Sophie does not need 500,000 viewers or reviewers. In her smartphone, she has the email addresses of 20 peers around the World specializing in her field, 20 neurobiologists who could review her work. All she needs is a place where she can demonstrate that she has respected the rules of scientific trust and that her methods and results have indeed been reviewed by qualified and objective peers. This place should also be social dissemination friendly so that her work may be shared, discussed and recommended by an exclusive community of specialized peers.
Finally, because research funding agencies are usually overwhelmed by the number of proposals, Sophie will have to provide them with a summarized and comprehensive digest representing to what extent her research is indeed valid, original and endorsed by peers who believe it is useful to science, and to human development at large.
These are the issues peerevaluation.org is tackling all at once, aware that a platform supporting Open Science, collaborative peer reviewing and dissemination cannot succeed without powerful incentives, innovative intellectual property rights management and, finally, reliable representations of scientific trust that meet the expectations of policy makers and funding bodies.
Peerevaluation.org aims at becoming a place where scholars come to make sure that they are getting the best of online sharing: increased dissemination, visibility, accessibility, commentary, and discussion, fruitful collaborations and, finally, evidence of impact, influence and re-use.
The basic peerevaluation.org scenario – focusing on the dissemination and remote pre- or post- publication peer review and commentary – unfolds as follows: (a) you upload a PDF of your recent paper; (b) you export the PDF’s abstract and link to your blog, your Mendeley account and a repository like CiteSeerX. (c) simultaneously it gets indexed by Google Scholar and Microsoft Academic Search; (d) wherever your file is, people can comment it, discuss it, recommend it, share it, have access to your articles statistics, social impact measures; (e) all these remote social interactions are simultaneously aggregated and displayed in your peerevaluation.org account, for you and others to consult.
5. Approaches for Community-Based Evaluation
Existing problems in peer review and new tools brought by Web 2.0 triggered new directions in research evaluation, making trust and reputation an important topic for peer review (see, for instance, the Peerevaluation.org approach). Reputation reflects community opinion on the performance of an individual with respect to one or more criteria. In this section we review two approaches for research evaluation leveraging on the explicit or implicit feedback of the scientific community, namely: (1) OpinioNet computes the reputation of researchers based on the opinions, such as review scores or citations; (2) UCount employs dedicated surveys to elicit community opinion on individual’s performance either as a researcher, or as a reviewer.
5.1. OpinioNet: Reputation of Research Based on Opinion Propagation
OpinioNet is a tool that is based on the notion of the propagation of opinions in structural graphs. In OpinioNet, the reputation of a given research work is not only influenced by the opinions it receives, but also by its position in the publications’ structural graph. For instance, a conference is reputable because it accepts high quality papers. Similarly, people usually assume that in the absence of any information about a given paper, the fact that the paper has been accepted by a highly reputable journal implies that the paper should be of good quality. Hence, there is a notion of propagation of opinions along the part_of relation of structural graphs.
Figure 1 provides an example of a common structural graph of research work. In this figure, there is a conference series CS that has a set of conference proceedings, {CP1,…, CPn}, and each conference proceeding is composed of a set of papers. Similarly, there is a journal J that has a set of volumes, {V1,…, Vn}, each composed of a set of papers. We note that if papers were split into sections, {S1,…, Sn}, then it is possible for different papers to share some sections, such as the “Background” section.
Current reputation measures in the publications field have mainly focused on citation-based metrics, like the h-index. Explicit reviews (or opinions) have been neglected outside the review process due to the fact that this information is very scarce in the publications field, unlike e-commerce scenarios such as Amazon or eBay. OpinioNet addresses this problem by providing means that help a single researcher infer their opinion about some research work (or other researcher) based on their own opinions of bits and pieces of the global publications structural graph. Accordingly, the reputation (or group opinion) is calculated by aggregating individual researchers’ opinions.
Furthermore, OpinioNet may also be used with indirect opinions. When computing the reputation of researchers and their research work, we say there is a lot of information out there that may be interpreted as opinions about the given researcher or research work. For instance, the current publication system provides us with direct (explicit) opinions: the review scores. Additionally, direct (or implicit) opinions may also be considered. For example, citations may also be viewed as an indication of how good a given research work is, i.e., a positive opinion of the citing authors about the cited research work. Subscription to journals may be viewed as an indication of how good a journal is viewed in its community, i.e., a positive opinion of the subscriber about the journal. Massive volumes of information exist that may be interpreted as opinions. The OpinioNet algorithm (Osman et al., 2010b) uses these opinions, whether they were direct or indirect, to infer the opinion of a researcher about some given research work8, and then infer the opinion of a research community accordingly. More importantly, OpinioNet may be used for any combination of information sources, although different fields of research may give more weight to one information source over the other.
As such, OpinioNet is easily customizable to suit the requirements of different communities or disciplines. For example, it is known that different disciplines have very different traditions and attitudes toward the way in which research is evaluated. With OpinioNet, one can select the source(s) of opinions to focus on, possibly giving more weight to different sources. For instance, one may easily make OpinioNet run on one’s own personal opinions only, the direct opinions of the community, on citation-based opinions only, or on a combination of citation-based opinions and direct ones. OpinioNet may also give more weight to papers accepted by journals that conferences, or vice versa. And so on.
Furthermore, OpinioNet does not need an incentive to encourage people to change their current behavior. Of course, having an open system where people read and rate each others work would be hugely beneficial. But OpinioNet also works with the data which is available now. We argue that we already have massive numbers of opinions, both direct and indirect, such as reviews, citations, acceptance by journals/conferences, subscriptions to journals, references from untraditional sources (such as blogs), etc. What is needed is a system, such as OpinioNet that can access such data, interpret it, and deduce reputation of research work accordingly. At the time being, we believe that accessing and compiling this data is the main challenge.
As for potential bias, when considering an opinion, the reputation of the opinion source is used by OpinioNet to assess the reliability of the opinion. For example, we say a person that is considered very good in a certain field is usually considered to be very good as well in assessing how others are in that field. This is based on the ex cathedra argument. An example of a current practice following the application of this argument is the selection of members of committees, advisory boards, etc. Although, of course, instead of simply considering the expertise of the person in the field, complementary methods that may assess how good the person is in rating research work may be used to enrich OpinioNet against bias and attacks. For example, studying a person’s past reviews could tell whether the person is usually biased for a specific gender, ethnicity, scientific technique, etc. Also, analyzing past reviews, one may also tell how close a person’s past opinions were to the group’s opinion. Past experiences may also be used to assess potential attacks, such as collusion. All of this information is complementary to OpinioNet, and it may be used by OpinioNet to help determine the reliability of the opinion.
After introducing the basic concepts and goals of OpinioNet, we now provide a brief technical introduction to the algorithm. Of course, for further details, we refer the interested reader to Osman et al. (2010b). And for information about evaluating OpinioNet and its impact on research behavior via simulations, we refer interested readers to Osman et al. (2011).
5.1.1. Reputation of research work
The reputation of research work is based on the propagation and aggregation of opinions in a structural graph. OpinioNet’s propagation algorithm is based on three main concepts:
• Impact of a node. Since researchers may write and split their research work into different ‘child nodes’ (e.g., a section of a paper, or papers in conference proceedings), it is impossible to know what is the exact weight to assign to each child node when assessing its impact on its parent nodes (and vice versa). In OpinioNet, the impact of a given node n at time t is based on the proportion of nodes that have received a direct opinion in the structural sub-tree of n. In other words, OpinioNet relies on the attention that a node receives (whether positive or negative) to assess its impact. For example, if one paper of a journal received a huge number of reviews (positive or negative) while another received no attention at all, then the one that received a huge number of reviews will have a stronger impact on the reputation of the journal than the latter.
• Direction of propagation. The direction of propagation in the structural graph is crucial. Each holds a different meaning. The “downward” propagation is viewed to provide the default opinion, such as a paper inheriting the reputation of the journal that accepted it. The default opinion is understood to present the opinion about the node that is inherited from the parents, and is usually used when there is a lack of information about the children nodes that help compose the node in question. The “upward” propagation provides the developing opinion, such as a conference aggregating the reputation of its papers. Then, each time a new opinion is added to a node in the graph, the default and developing opinions of its neighboring nodes are updated accordingly. Then, the update of one node’s values triggers the update of its neighboring nodes, resulting in a propagation wave throughout the structural graph.
• Decay of information value. We say everything loses its value with time. Opinions are no exception, and an opinion about some node n made at time t loses its value (very) slowly by decaying toward the decay probability distribution (or the default opinion) following a decay function that makes the opinion converge to the default one with time.
We note that OpinioNet essentially propagates the opinions of one researcher on a given attribute (say quality of research) in a structural graph. However, opinions may be provided for several attributes, such as novelty, soundness of research work, etc. Opinions may also be provided by more that one researcher. In these cases, different aggregations may be used to obtain the final group opinion about a given piece of research work. Osman et al. (2010b) provides some examples on how to aggregate these opinions to obtain a final reputation measure. However, as discussed earlier, an important thing to note is that the reputation of each opinion holder is used to provide a measure on how reliable their opinions are. In other words, the reputations of opinion holders are used to provide the weights of the opinions being aggregated.
5.1.2. Reputation of researchers
Every node of a structural graph has its own author, or set of coauthors. The authors of different sections of a paper may be different, although there might be some overlap in the sets of authors. Similarly, the authors of different papers of a conference may be different. And so on. In OpinioNet, the reputation of an author at a given time is an aggregation of the reputation of its research work. However, the aggregation takes into consideration the number of coauthors that each paper has. The aggregation (see Osman et al., 2010a) essentially states that the more coauthors some research work has, the smaller the impact it leaves on each of its coauthors.
5.2. UCount: A Community-Based Approach for Research Evaluation
The UCount approach9 (Parra et al., 2011) provides the means for community-based evaluation of overall scientific excellence of researchers and their performance as reviewers. The evaluation of overall scientific excellence of researchers is done via surveys10 that aim at gathering community opinions on how valuable a given researcher’s contribution to science is. The results are aggregated to build rankings. In the current section we describe the use of UCount for assessing reviewers, since it better fits the scope of the special issue.
UCount for assessing reviewers is specifically designed to operate based on reviewer performance as reviewers, as opposed to other criteria such as bibliometric prominence: a high-profile researcher is not necessarily a good reviewer (Black et al., 1998). UCount is integrated in the above-mentioned e-Scripts, a review system used for the ICST Transactions. It enables authors and editors to provide feedback on the performance of reviewers using the Review Quality Instrument (RQI) developed by editors of the British Medical Journal (van Rooyen et al., 1999). This is a psychometrically validated instrument used in multiple studies of peer review (Jefferson et al., 2007).
The RQI consists of an 8-point scale (Figure 2), where each item is scored on a 5-point Likert scale (1 = poor, 5 = excellent). The first 7 points each enquire about a different aspect of the review, including the discussion of the importance and originality of the work, feedback on the strengths and weaknesses of the research method and the presentation of the results, the constructiveness of comments, and the substantiation of comments by reference to the paper. The 8th and final item is an overall assessment of the review quality, and can be compared to the total score calculated as the mean of the first 7 items.
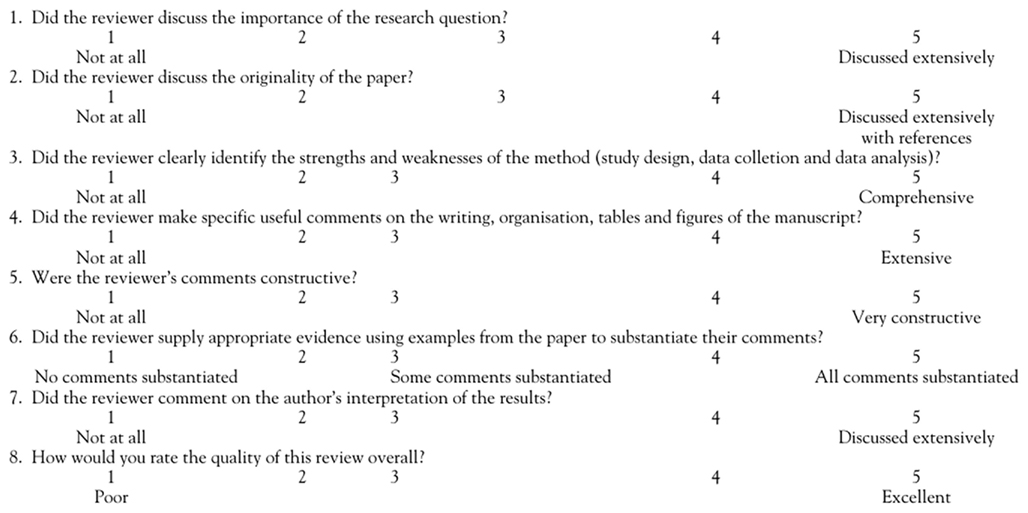
Figure 2. The 8-point Review Quality Instrument (RQI) developed by van Rooyen et al. (1999). The total score is calculated as the mean of the first 7 items, while the 8th “global item” provides an extra validation check.
On the basis of this feedback, every 3 months (linked with ICST Transactions issue schedule) public rankings of reviewers will be presented. Reviewers submitting at least three reviews will be ranked according to several criteria: overall best score, total number of reviews completed, and the usefulness, insight, and constructiveness of feedback. Moreover, during the process of choosing the reviewers for a paper, the editor will be able to see the ranking of the reviewers based on their past performance. The ranking will be based on RQI feedback:
• First-placed are candidates with a mean RQI score higher than a given threshold (suggest the median 3), ranked according to their RQI score.
• Next come candidates with no RQI, including both new reviewers and those who have completed less than 3 reviews in the last 12 months. These candidates will be ranked in the traditional bidder-author-editor order.
• Last come candidates whose mean RQI score is below the acceptable threshold, ranked in descending order of score.
Where available, RQI for candidates will be displayed in order to clarify the ranking. Editors will still be able to re-order the candidate list. We believe that this will lead to the selection of better reviewers and also to their recognition in the community as opposed to the current situation in most journals, where only the members of the editorial board get credits, while the reviewers remain unknown.
UCount is now being implemented for publication activities of the European Alliance for Innovation (EAI) and the Institute for Computer Sciences, Social Informatics and Telecommunications Engineering (ICST).
6. Conclusion and Discussion
In this paper we have presented a range of possible extensions or alternatives to the conventional peer review process. The diversity of these approaches reflects the wide range of complementary factors that can be considered when determining the value of a scientific contribution. Indeed, definitions of quality are often highly context-dependent: for example, in some cases a technically unreliable but imaginative and inspirational paper may be of more value than a thorough and careful examination (Underwood, 2004), while in other cases, the opposite will be true. Such a diversity of needs requires a diversity of solutions.
The particular selection of the approaches for research evaluation reviewed in this paper is by no means complete, reflecting primarily the research carried out by the LiquidPub11 project and its collaborators12. There exist many other approaches that we would see as complementary, for example expert expert post-publication review such as that carried out by the Faculty of 100013, or personalized recommender systems (Adomavicius and Tuzhilin, 2005; Zhou et al., 2010).
In the following we discuss controversial aspects of the approaches reviewed in the paper.
6.1. Bidding as an Indicator of Importance
Given the known results regarding article download statistics (Brody et al., 2006) and the findings from the experiment on bidding described in Section 1, we can expect that bid counts too will serve as a reliable (though not infallible) indicator of the future impact of research work. A concern here is that – as with citation – people may bid not just on papers which interest them topically, but on papers which they wish to criticize and see rejected. Our inclination is that this is less of a risk than might be thought, for two main reasons. First, results from online rating systems such as the 5-star system used on YouTube show that there is a very strong bias toward positive ratings, suggesting that people treat items which they dislike with indifference rather than active criticism (Hu et al., 2009): we can expect that a similar principle may apply in bidding, that potential reviewers will ignore bad papers rather than waste valuable time volunteering to critique something they will likely expect to be rejected anyway. Second, leaving aside bad papers, we may anticipate bidders volunteering to review papers with which they have a strong disagreement. This may certainly create an issue for the journal Editor who must control for the potential conflicts of interest, but it does not reflect a conflict with the potential impact of the paper. Papers on hotly contested topics are likely to be more, not less, highly cited.
An additional risk is that since bidding is based on title and abstract, it may attract attention to “over-sold” papers whose claims are made to sound more important than they actually are. This is of course a universal problem of research, not limited to bidding: authors try and over-hype their work to attract editorial, reviewer, and reader attention (Lawrence, 2003). The major question, which will have to be addressed on the basis of future experience, is whether this will distort the bidding statistics any more than it already does the citation and download counts.
On a more positive note, bidding is in line with one of the key motivations for scientists to engage in peer review, namely that by doing so they can help to improve and contribute to their colleagues’ work (Goodman et al., 1994; Purcell et al., 1998; Sense About Science, 2009). This strong ethic of professional altruism is more than likely to help offset the risks described above, and provides another reason why bidding is likely to reflect importance and impact – it is more exciting to contribute to work which you believe will be of lasting importance.
6.2. Peerevaluation.org vs. UCount
Peerevaluation.org and UCount both aim at more open and transparent peer review. However, while UCount aims at incremental change in the traditional journal review, by introducing feedback on the reviewers, Peerevaluation proposes a radical shift in the process, which in its case is no more managed by the editors. We believe that the two approaches can be combined in the future, for instance UCount findings can be used to suggest reviewers in Peerevaluation, while Peerevaluation past review history can be a valuable input to UCount.
6.3. Use of Community Opinions
OpinioNet and UCount approaches use community opinions to estimate the reputation of a researcher. To take into account that majority is not always right, OpinioNet weights opinions based on the credibility of the opinion source, e.g., the level of expertise of the person who provides the opinion. UCount, however, aims at catching the community opinion as it is, without any adjustments. Therefore, UCount does not aim at answering “is it true that person A is the best reviewer (researcher)?”, but rather at stating “community X thinks that person A is the best reviewer (researcher).” Both approaches rely on getting data about community opinions: while OpinioNet aims at collecting the data already available via citation, co-authorship, and publication networks, UCount requires that authors fill in a questionnaire, and the results can be used as direct opinions in OpinioNet.
6.4. Incentives to Participate
Providing direct opinions on reviewers in UCount might be seen as yet another action required from the author. However, providing ratings is a minimal effort comparing to writing a paper or writing a review. Therefore, we believe that if really good journals will require feedback on reviewers (e.g., as proposed by UCount), then people will participate and then other journals will have to follow. Moreover, in both the UCount and Peerevaluation approaches reviewers have incentives to submit good reviews because they know they are being assessed, either directly (UCount) or indirectly (Peerevaluation, because reviews are public). Moreover, reviewers will get publicity for doing a good job. UCount also offers incentives for authors, who are encouraged to participate because in this way they help editors to select better reviewers, and therefore, get better reviews. If at some point in time it appears that there are not enough good reviewers, maybe the incentives should be reconsidered. Controversial but possible incentives include paying reviewers, making it possible to submit a paper only after first reviewing three other papers, or reducing registration fees for people who spend time reviewing papers for a conference.
6.5. The Role of the Internet
It has long been recognized that the advent of the Web offers many opportunities to change the landscape of research publication and evaluation (Harnad, 1990; Ginsparg, 1994; Swan, 2007). At the most basic level, electronic publication effectively reduces storage, distribution, and communication costs to near zero, as well as greatly facilitating the creation and sharing of documents (Odlyzko, 1995). Electronic corpora considerably facilitate search and indexing of documents, and the speed of electronic communication has made it possible to greatly reduce the time to review and publish scholarly work (Spier, 2002). Electronic publishing also permits the distribution of a great many different types of media besides the conventional scholarly article, including datasets, software, videos, and many other forms of supporting material.
The same factors help to facilitate the kind of large-scale peer evaluation described in the present article, of which we already see a great deal of uptake in social networks, video-sharing sites, and other online communities. It is cheap and easy for an individual to rate or comment on a given electronic entity, yet the large-scale of commenting and rating activity enables a great many forms of valuable analysis, that in turn bring benefits back to the evaluating communities (Masum and Zhang, 2004).
One concern related to this approach is that while in principle electronic communication serves to widen access and availability, the practical effect of search, reputation and recommendation tools may in fact be to narrow it (Evans, 2008). On the one hand this may be due to improved filtering of inferior work; however, it is possible that electronic distribution and evaluation systems will heighten the already-known “rich-get-richer” phenomenon of citation (de Solla Price, 1976; Medo et al., 2011), and perhaps reinforce existing inequalities of attention. One means of addressing this may be to ensure that electronic evaluation systems place a strong focus on diversity as a useful service (Zhou et al., 2010). It certainly emphasizes the point made earlier in this article, that a diversity of metrics is required in order to ensure that the many different types of contribution are all properly recognized and rewarded.
A second concern relates to accessibility. Many of the tools and techniques described here assume ubiquitous access to the internet, something readily available in wealthier nations but still difficult to ensure elsewhere in the world (Best, 2004). Even where access is not an issue, bandwidth may be, for example where the distribution of multimedia files is concerned. However, electronic technologies and communities also serve to narrow geographic and economic inequalities, for example making it easier to create documents of equivalent quality (Ginsparg, 1994) and enabling virtual meetings where the cost of travel makes it otherwise difficult for researchers to communicate with their peers (Gichora et al., 2010). The move to online communities as a facilitator of scientific evaluation must certainly be accompanied by a strong push to ensure access.
6.6. Our Vision for Future of Research Evaluation
One of the conclusions that we might draw from the paper is that, as the landscape of the scientific publishing is undoubtedly changing, the processes for the evaluation of research outputs and of researchers are also changing. As we seen in Sections 2, 3, and 4.2, the purpose of the peer review (to find errors or to help improve the paper) is perceived differently by different communities. In the next years we envision the growth of various tools for research evaluation, including open source and those operating with open API/protocols. Such tools would primarily operate on the Web and include the variety of methods for research evaluation, so that PC chairs or journal editors (or even people playing some new emerging roles which do not exist yet) will be able to choose. Examples of tools with such functionalities already emerge (e.g., Mendeley, Peerevaluation.org, Interdisciplines), but it is not yet clear how these tools can be connected and which of them will be adopted widely enough to have a normative effect. We believe that different tools and practices will be adopted by different communities and there is no unique approach that will suit all the researchers on the planet. Moreover, the same researcher working in different contexts will need different tools, and effective evaluation systems should have these choices and alternatives built in by design14. With this in mind, attention should be paid less to designing “the” scientific evaluation system of tomorrow – something that, like “the” peer review process, will be an emergent phenomenon based on the different needs of different disciplines and communities. Instead, attention should focus on ensuring interoperability and diversity among the many possible tools that scientific evaluation can make use of.
Conflict of Interest Statement
The authors declare that the research was conducted in the absence of any commercial or financial relationships that could be construed as a potential conflict of interest.
Acknowledgments
This work has been supported by the EU ICT project LiquidPublication. The LiquidPub project acknowledges the financial support of the Future and Emerging Technologies (FET) programme within the Seventh Framework Programme for Research of the European Commission, under FET-Open grant number: 213360.
Footnotes
- ^“With the authors’ consent, the paper already peer reviewed and accepted for publication by BMJ was altered to introduce 8 weaknesses in design, analysis, or interpretation” (Godlee et al., 1998).
- ^This marks an odd contradiction to the results of Opthof et al. (2002), where more reviewers made for better prediction. One explanation might be that in medical research there could be a greater number of different factors that must be considered when assessing an article, hence several reviewers with different expertise might produce a better review.
- ^Correlation between reviewer- and citation-based rankings was measured using Kendall’s τ for 5 different conferences, of which 2 had weak but statistically significant correlations (τ = 0.392, p = 0.0001 and τ = 0.310, p = 0.005; the two conferences had respectively 150 and 100 submissions). The other, larger conferences had no statistically significant correlation (Mirylenka et al., unpublished).
- ^PLoP stands for Pattern Languages of Programs and *PLoP family of conferences includes: EuroPLoP, PLoP, VikingPLoP, etc. See http://www.hillside.net/europlop/europlop2011/links.html for a complete list.
- ^http://interdisciplines.org/, a website for interdisciplinary conferences run as conversations.
- ^Note that technically this experiment is closer to a quasi-experiment because the reviewers were allowed to choose the type of review process. If any of the groups was superior in terms of reviewing quality, this may have affected the results.
- ^http://escripts.icst.org/
- ^How indirect opinions may be defined is an issue that has been addressed by Osman et al. (2010a).
- ^http://icst.org/ucount/
- ^See examples of such surveys at http://icst.org/UCount-Survey/
- ^http://project.liquidpub.org/
- ^A complete overview of the research carried out by the project on these topics is available at http://project.liquidpub.org/research-areas/research-evaluation
- ^http://f1000.com/
- ^For instance, Confy, a submission system used by EAI and ICST, allows a choice of various models for conducting peer review – with or without bidding, customizable review forms, and other features. Confy is currently available at http://cameraready.eai.eu/and will become open source as the code becomes feature-complete.
References
Adomavicius, G., and Tuzhilin, A. (2005). Toward the next generation of recommender systems: a survey of the state-of-the-art and possible extensions. IEEE Trans. Knowl. Data Eng. 17, 734–749.
Black, N., van Rooyen, S., Godlee, F., Smith, R., and Evans, S. (1998). What makes a good reviewer and a good review for a general medical journal? J. Am. Med. Assoc. 280, 231–233.
Bornmann, L. (2007). Bias cut. women, it seems, often get a raw deal in science – so how can discrimination be tackled? Nature 445, 566.
Bornmann, L., and Daniel, H.-D. (2005a). Committee peer review at an international research foundation: predictive validity and fairness of selection decisions on post-graduate fellowship applications. Res. Eval. 14, 15–20.
Bornmann, L., and Daniel, H.-D. (2005b). Selection of research fellowship recipients by committee peer review. reliability, fairness and predictive validity of board of trustees’ decisions. Scientometrics 63, 297–320.
Bornmann, L., and Daniel, H.-D. (2010a). The validity of staff editors’ initial evaluations of manuscripts: a case study of Angewandte Chemie International Edition. Scientometrics 85, 681–687.
Bornmann, L., and Daniel, H.-D. (2010b). The usefulness of peer review for selecting manuscripts for publication: a utility analysis taking as an example a high-impact journal. PLoS ONE 5, e11344. doi:10.1371/journal.pone.0011344
Bornmann, L., Wallon, G., and Ledin, A. (2008). Does the committee peer review select the best applicants for funding? An investigation of the selection process for two European molecular biology organization programmes. PLoS ONE 3, e3480. doi:10.1371/journal.pone.0003480
Brody, T., Harnad, S., and Carr, L. (2006). Earlier web usage statistics as predictors of later citation impact. JASIST 58, 1060–1072.
Casati, F., Marchese, M., Mirylenka, K., and Ragone, A. (2010). Reviewing Peer Review: A Quantitative Analysis of Peer Review. Technical Report 1813. University of Trento. Available at: http://eprints.biblio.unitn.it/archive/00001813/
Ceci, S. J., and Williams, W. M. (2011). Understanding current causes of women’s underrepresentation in science. Proc. Natl. Acad. Sci. U.S.A. 108, 3157–3162.
Cho, M. K., Justice, A. C., Winker, M. A., Berlin, J. A., Waeckerle, J. F., Callaham, M. L., and Rennie, D. (1998). Masking author identity in peer review: what factors influence masking success? PEER Investigators. JAMA 280, 243–245.
de Solla Price, D. (1976). A general theory of bibliometric and other cumulative advantage processes. J. Am. Soc. Inf. Sci. 27, 292–306.
Evans, J. A. (2008). Electronic publication and the narrowing of science and scholarship. Science 321, 395–399.
Fisher, M., Friedman, S. B., and Strauss, B. (1994). The effects of blinding on acceptance of research papers by peer review. J. Am. Med. Assoc. 272, 143–146.
Gichora, N. N., Fatumo, S. A., Ngara, M. V., Chelbat, N., Ramdayal, K., Opap, K. B., Siwo, G. H., Adebiyi, M. O., El Gonnouni, A., Zofou, D., Maurady, A. A. M., Adebiyi, E. F., de Villiers, E. P., Masiga, D. K., Bizzaro, J. W., Suravajhala, P., Ommeh, S. C., and Hide, W. (2010). Ten simple rules for organizing a virtual conference – anywhere. PLoS Comput. Biol. 6, e1000650. doi:10.1371/journal.pcbi.1000650
Ginsparg, P. (1994). First steps towards electronic research communication. Comput. Phys. 8, 390–396.
Godlee, F. (2002). Making reviewers visible: openness, accountability, and credit. JAMA 287, 2762–2765.
Godlee, F., Gale, C. R., and Martyn, C. N. (1998). Effect on the quality of peer review of blinding reviewers and asking them to sign their reports a randomized controlled trial. JAMA 280, 237–240.
Goodman, S. N., Berlin, J., Fletcher, S. W., and Fletcher, R. H. (1994). Manuscript quality before and after peer review and editing at annals of internal medicine. Ann. Intern. Med. 121, 11–21.
Greaves, S., Scott, J., Clarke, M., Miller, L., Hannay, T., Thomas, A., and Campbell, P. (2006). Overview: Nature’s peer review trial. Nature. doi: 10.1038/nature05535.
Harnad, S. (1990). Scholarly skywriting and the prepublication continuum of scientific enquiry. Psychol. Sci. 1, 342–344.
Hu, N., Pavlou, P. A., and Zhang, J. (2009). Overcoming the J-shaped distribution of product reviews. Commun. ACM 52, 144–147.
Jefferson, T., Rudin, M., Folse, S. B., and Davidoff, F. (2007). Editorial peer review for improving the quality of reports of biomedical studies. Cochrane 41, MR000016.
Jefferson, T., Wager, E., and Davidoff, F. (2002a). Measuring the quality of editorial peer review. JAMA 287, 2786–2790.
Jefferson, T., Alderson, P., Wager, E., and Davidoff, F. (2002b). Effects of editorial peer review: a systematic review. JAMA 287, 2784–2786.
Justice, A. C., Cho, M. K., Winker, M. A., Berlin, J. A., Rennie, D., and PEER Investigators. (1998). Does masking author identity improve peer review quality? A randomized controlled trial. JAMA 280, 240–242.
Kassirer, J. P., and Campion, E. W. (1994). Peer review: crude and understudied, but indispensable. J. Am. Med. Assoc. 272, 96–97.
Katz, D. S., Proto, A. V., and Olmsted, W. W. (2002). Incidence and nature of unblinding by authors: our experience at two radiology journals with double-blinded peer review policies. Am. J. Roentgenol. 179, 1415–1417.
Lee, K., Boyd, E., Holroyd-Leduc, J., Bacchetti, P., and Bero, L. (2006). Predictors of publication: characteristics of submitted manuscripts associated with acceptance at major biomedical journals. Med. J. Aust. 184, 621.
Lynch, J. R., Cunningham, M. R., Warme, W. J., Schaad, D. C., Wolf, F. M., and Leopold, S. S. (2007). Commercially funded and united states-based research is more likely to be published; good-quality studies with negative outcomes are not. J. Bone Joint Surg. Am. 89, 1010–1018.
Marsh, H. W., Bornmann, L., Mutz, R., Daniel, H.-D., and O’Mara, A. (2009). Gender effects in the peer reviews of grant proposals: a comprehensive meta-analysis comparing traditional and multilevel approaches. Rev. Educ. Res. 79, 1290–1326.
McNutt, R. A., Evans, A. T., Fletcher, R. H., and Fletcher, S. W. (1990). The effects of blinding on the quality of peer review: a randomized trial. JAMA 263, 1371–1376.
Medo, M., Cimini, G., and Gualdi, S. (2011). Temporal effects in the growth of networks. Available at: http://arxiv.org/abs/1109.5560
Medo, M., and Wakeling, J. R. (2010). The effect of discrete vs. continuous-valued ratings on reputation and ranking systems. Europhys. Lett. 91, 48004.
Odlyzko, A. M. (1995). Tragic loss or good riddance? The impending demise of traditional scholarly journals. Int. J. Hum. Comput. Sci. 42, 71–122.
Olson, C. M., Rennie, D., Cook, D., Dickersin, K., Flanagin, A., Hogan, J. W., Zhu, Q., Reiling, J., and Pace, B. (2002). Publication bias in editorial decision making. JAMA 287, 2825–2828.
Opthof, T., Coronel, R., and Janse, M. J. (2002). The significance of the peer review process against the background of bias: priority ratings of reviewers and editors and the prediction of citation, the role of geographical bias. Cardiovasc. Res. 56, 339–346.
Osman, N., Sabater-Mir, J., and Sierra, C. (2011). “Simulating research behaviour,” in 12th International Workshop on Multi-Agent-Based Simulation (MABS’11), Taipei.
Osman, N., Sabater-Mir, J., Sierra, C., de Pinninck Bas, A. P., Imran, M., Marchese, M., and Ragone, A. (2010a). Credit attribution for liquid publications. Deliverable D4.1, Liquid Publications Project. Available at: https://dev.liquidpub.org/svn/liquidpub/papers/deliverables/LP_D4.1.pdf
Osman, N., Sierra, C., and Sabater-Mir, J. (2010b). “Propagation of opinions in structural graphs,” in ECAI 2010: Proceedings of the 19th European Conference on Artificial Intelligence, Vol. 215 of Frontiers in Artificial Intelligence and Applications, eds H. Coelho, R. Studer, and M. Wooldridge (Lisbon: IOS Press), 595–600.
Parra, C., Birukou, A., Casati, F., Saint-Paul, R., Wakeling, J. R., and Chlamtac, I. (2011). “UCount: a community-driven approach for measuring scientific reputation,” in Proceedings of Altmetrics11: Tracking Scholarly Impact on the Social Web, Koblenz.
Purcell, G. P., Donovan, S. L., and Davidoff, F. (1998). Changes to manuscripts during the editorial process: characterizing the evolution of a clinical paper. J. Am. Med. Assoc. 280, 227–228.
Ragone, A., Mirylenka, K., Casati, F., and Marchese, M. (2011). “A quantitative analysis of peer review,” in 13th International Society of Scientometrics and Informetrics Conference, Durban.
Reinhart, M. (2009). Peer review of grant applications in biology and medicine. reliability, fairness, and validity. Scientometrics 81, 789–809.
Ross, J. S., Gross, C. P., Desai, M. M., Hong, Y., Grant, A. O., Daniels, S. R., Hachinski, V. C., Gibbons, R. J., Gardner, T. J., and Krumholz, H. M. (2006). Effect of blinded peer review on abstract acceptance. J. Am. Med. Assoc. 295, 1675–1680.
Sense About Science. (2009). Peer Review Survey: Preliminary Results. Available at: http://www.senseaboutscience.org.uk/index.php/site/project/29/
Smith, R. (2006). Peer review: a flawed process at the heart of science and journals. J. R. Soc. Med. 99, 178–182.
Underwood, A. J. (2004). It would be better to create and maintain quality rather than worrying about its measurement. Mar. Ecol. Prog. Ser. 270, 283–286.
van Rooyen, S., Black, N., and Godlee, F. (1999). Development of the review quality instrument (RQI) for assessing peer reviews of manuscripts. J. Clin. Epidemiol. 52, 625–629.
Walsh, E., Rooney, M., Appleby, L., and Wilkinson, G. (2000). Open peer review: a randomised controlled trial. Br. J. Psychiatry 176, 47–51.
Ware, M., and Monkman, M. (2008). Peer Review in Scholarly Journals: Perspective of the Scholarly Community – An International Study. Survey Commissioned by the Publishing Research Consortium. Available at: http://www.publishingresearch.net/PeerReview.htm
Keywords: research evaluation, peer review, metrics, bidding, opinions, LiquidPub, UCount
Citation: Birukou A, Wakeling JR, Bartolini C, Casati F, Marchese M, Mirylenka K, Osman N, Ragone A, Sierra C and Wassef A (2011) Alternatives to peer review: novel approaches for research evaluation. Front. Comput. Neurosci. 5:56. doi: 10.3389/fncom.2011.00056
Received: 12 July 2011;
Paper pending published: 07 August 2011;
Accepted: 11 November 2011;
Published online: 14 December 2011.
Edited by:
Diana Deca, University of Amsterdam, NetherlandsReviewed by:
Jelte M. Wicherts, University of Amsterdam, NetherlandsH. Steven Scholte, University of Amsterdam, Netherlands
Diana Deca, University of Amsterdam, Netherlands
Dietrich Samuel Schwarzkopf, Wellcome Trust Centre for Neuroimaging at UCL, UK
Copyright: © 2011 Birukou, Wakeling, Bartolini, Casati, Marchese, Mirylenka, Osman, Ragone, Sierra and Wassef. This is an open-access article subject to a non-exclusive license between the authors and Frontiers Media SA, which permits use, distribution and reproduction in other forums, provided the original authors and source are credited and other Frontiers conditions are complied with.
*Correspondence: Aliaksandr Birukou and Joseph Rushton Wakeling, European Alliance for Innovation, Gent, Belgium, via alla Cascata 56/D, 38123 Trento, TN, Italy. e-mail: birukou@gmail.com; joseph.wakeling@gmail.com