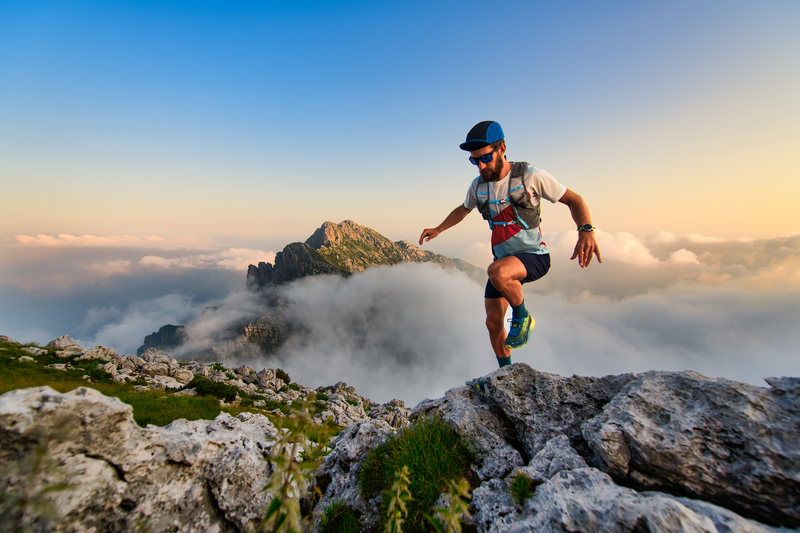
94% of researchers rate our articles as excellent or good
Learn more about the work of our research integrity team to safeguard the quality of each article we publish.
Find out more
REVIEW article
Front. Comput. Neurosci. , 01 July 2010
Volume 4 - 2010 | https://doi.org/10.3389/fncom.2010.00019
This article is part of the Research Topic Theory and models of spike-timing dependent plasticity View all 14 articles
Spike timing dependent plasticity (STDP) is a phenomenon in which the precise timing of spikes affects the sign and magnitude of changes in synaptic strength. STDP is often interpreted as the comprehensive learning rule for a synapse – the “first law” of synaptic plasticity. This interpretation is made explicit in theoretical models in which the total plasticity produced by complex spike patterns results from a superposition of the effects of all spike pairs. Although such models are appealing for their simplicity, they can fail dramatically. For example, the measured single-spike learning rule between hippocampal CA3 and CA1 pyramidal neurons does not predict the existence of long-term potentiation one of the best-known forms of synaptic plasticity. Layers of complexity have been added to the basic STDP model to repair predictive failures, but they have been outstripped by experimental data. We propose an alternate first law: neural activity triggers changes in key biochemical intermediates, which act as a more direct trigger of plasticity mechanisms. One particularly successful model uses intracellular calcium as the intermediate and can account for many observed properties of bidirectional plasticity. In this formulation, STDP is not itself the basis for explaining other forms of plasticity, but is instead a consequence of changes in the biochemical intermediate, calcium. Eventually a mechanism-based framework for learning rules should include other messengers, discrete change at individual synapses, spread of plasticity among neighboring synapses, and priming of hidden processes that change a synapse’s susceptibility to future change. Mechanism-based models provide a rich framework for the computational representation of synaptic plasticity.
It is amateurs who have one big bright beautiful idea that they can never abandon. Professionals know that they have to produce theory after theory before they are likely to hit the jackpot.
-Francis Crick.
The term “spike timing dependent plasticity” (STDP) refers to the observation that the precise timing of spikes significantly affects the sign and magnitude of synaptic plasticity (Bell et al., 1997; Markram et al., 1997; Bi and Poo, 1998). For example, at connections between mammalian pyramidal neurons (Markram et al., 1997; Bi and Poo, 1998; Feldman, 2000; Nishiyama et al., 2000; Sjöström et al., 2001; Wittenberg and Wang, 2006) a presynaptic spike preceding a postsynaptic spike within a narrow time window leads to long-term potentiation (LTP); if the order is reversed, long-term depression (LTD) results. In a common experimental paradigm, presynaptic and postsynaptic spike pairs are evoked repeatedly with a fixed time interval, Δt. This pairing is repeated at low frequency and the resulting change in synaptic response size is measured. Repeating this experiment for many values of Δt gives the timing-dependence of plasticity. Such an STDP curve is assumed to be useful for predicting the plasticity that results when Δt is variable, e.g., for arbitrary trains of presynaptic and postsynaptic spikes that occur under less controlled conditions. In this way the STDP curve is interpreted as a learning rule that defines how a particular type of synapse participates in information storage and ultimately brain circuit function.
Certainly the discovery of STDP represented a major advance over previous means of inducing synaptic plasticity, which relied on less controlled stimulation such as the delivery of strong (tetanic) stimuli to entire presynaptic axon tracts. In contrast, the minimal nature of STDP protocols carried with it two hopes: that the activity patterns used were more realistic, and that the various properties of synaptic plasticity could eventually be accounted for by knowing the timing of all the spikes. This is realized in theoretical models by assuming that cumulative plasticity is predicted by a simple superposition of spike pairs that repeatedly sample the STDP curve (“linear STDP”) (Gerstner et al., 1996; Kempter et al., 1999; Abbott and Nelson, 2000; Song et al., 2000; van Rossum et al., 2000; Gütig et al., 2003; Izhikevich and Desai, 2003). In this sense STDP has been considered as a possible first law of synaptic plasticity.
This appealingly simple viewpoint neglects the actual mechanisms that change synaptic strength. Synaptic plasticity is induced by a variety of receptor-generated second messengers, which in turn activate kinases, phosphatases, and other downstream targets. A first-law view of STDP largely disregard these molecular and cellular mechanisms in favor of the view that the essential character of synaptic plasticity can be separated from messy biological details.
In this article we review the considerable experimental evidence that real learning rules occupy a parameter space of high dimensionality that is not easily reduced or even approximated using spike pairs alone. Such parameters as stimulation frequency or even the total number of presynaptic and postsynaptic spikes have a large influence on the sign and magnitude of net plasticity. In addition, major nonlinearities arise from the history of spike activity on timescales longer than the width of the STDP curve as well as the location and spatial pattern of synaptic activity on and across neurons. Finally, on time scales of tens of minutes and shorter, single synapses undergo plasticity in what appears to be a sudden and all-or-none manner.
Can these and other nonlinearities be tamed without losing the conceptual appeal of a rule-based approach? We suggest that this complexity is naturally captured by models of synaptic plasticity that are based on cellular mechanisms. Consideration of signaling machinery allows the creation of a model that can be driven by any activity pattern to mimic a variety of experimental induction protocols, as well as natural activity patterns that occur in living animals. We focus in particular on one messenger, calcium, that can potentially account for much of the complexity seen in several commonly studied forms of synaptic plasticity.
The hypothesis that memory formation may correspond to changes in the connections between neurons dates back to Konorski, (1948), Hebb, (1949) and other work reviewed in Squire, (1987). In a striking early formulation, Hebb cited the functional notion of causality by postulating that a presynaptic neuron that repeatedly drives a postsynaptic neuron to fire should eventually cause the presynaptic neuron to become more efficient in driving the postsynaptic neuron. For this to occur, the presynaptic neuron would presumably fire immediately before the postsynaptic neuron. Hebb’s rule has profoundly influenced neuroscience and machine learning.
The discovery of long-term potentiation in the perforant path input to the dentate gyrus of the hippocampus (Bliss and Gardner-Medwin, 1973; Bliss and Lømo, 1973) provided the first experimental evidence for synaptic plasticity. Now the most widely used experimental model is a nearby type of synapse between pyramidal neurons of hippocampal areas CA3 and CA1. High-frequency tetanic stimulation of CA3 axons, which drive postsynaptic CA1 neurons to fire, leads to long-term potentiation (LTP), an increase in the synaptic response in CA1 to single stimuli (Figure 1A).
Figure 1. Classical induction protocols for synaptic plasticity. (A) Changing the stimulation frequency of robust extracellular stimulation affects the sign and magnitude of synaptic plasticity. Left: high-frequency stimulation results in LTP whereas low-frequency stimulation produces LTD. Right: frequency vs. plasticity curve (from O’Connor et al., 2005a). (B) Low-frequency stimulation paired with voltage clamping of the postsynaptic cell can also result in LTP or LTD depending on the postsynaptic voltage. Left: moderate depolarization produces LTD where as large depolarization produces LTP. Right: depolarization vs. plasticity curve (from Ngezahayo et al., 2000). (C) Theta-burst stimulation tries to mimic more naturalistic conditions. In the hippocampus of awake behaving animals there is a strong theta-frequency oscillation (right). Left: In a theta-burst induction protocol, short high-frequency bursts are delivered each 200 ms, or at a frequency of 5 Hz, within the theta range (from Hirase et al., 1999). (D) STDP protocols are induced by precisely stimulating the presynaptic afferents at a specific time (Δt) before or after a postsynaptic spike. Right: The precise Δt determines the sign and magnitude of synaptic plasticity (from Bi and Poo, 1998).
Subsequently Hebb’s postulate was extended to encompass LTD as a necessary converse of LTP (Stent, 1973; Sejnowski, 1977). Based on observations of the development and plasticity of visual cortex (Wiesel and Hubel, 1963), Bienenstock, Cooper, and Munro (BCM) theorized (Bienenstock et al., 1982) and several groups (Dudek and Bear, 1992; Mulkey and Malenka, 1992) demonstrated experimentally that if a presynaptic neuron drives a postsynaptic neuron only weakly, LTD of the active synapses (homosynaptic LTD) would occur. These results are consistent with the bidirectional “BCM rule” in which the direction and magnitude of plasticity depends on a postsynaptic activity variable (Figure 1A).
Empirical search revealed that LTP was robustly induced with 1-s-long stimuli (high-frequency tetanus, 100 Hz), while LTD required 15 min of stimulation (low-frequency, 1 Hz; Figure 1A). Pairing low-frequency presynaptic stimulation with postsynaptic depolarization (to 0 mV for LTP, to −30 mV for LTD) was also found to elicit these phenomena robustly in voltage-clamp experiments (Figure 1B), suggesting that the strength of postsynaptic activation determines the sign of plasticity (Artola et al., 1990; Ngezahayo et al., 2000). These protocols were not physiologically realistic, but they did enable detailed studies of molecular mechanisms of inducing, expressing and maintaining synaptic plasticity. Another thread of research was the exploration of naturalistic-seeming patterns of neural activity. It was found that a necessary part of LTP induction at CA3–CA1 synapses was bursts of stimulation at intervals corresponding approximately to the theta frequency (Figure 1C; Rose and Dunwiddie, 1986; Larson and Lynch, 1988), which occurs in vivo in the hippocampus during active behavior and REM sleep. However, in neither thread of work was spiking in the postsynaptic neuron controlled or measured (though see Levy and Steward, 1983).
At the time, computational neuroscience was oriented toward connectionist-inspired learning models in which a neuron’s activity was described by a continuously varying firing rate (Wilson and Cowan, 1973; Sejnowski, 1977; Bienenstock et al., 1982; Oja, 1982; Hopfield, 1984; Rumelhart and McClelland, 1987) with very little work considering the timing of discrete spikes. Currently much of the attention has shifted to computational models with spiking neurons in which spike timing might actually carry information, or where computations are too quick for obtaining good rate estimates (Hopfield, 1995; Amit and Brunel, 1997; Gerstner and Kistler, 2002). This shift to spiking models has intensified considerably following the demonstration of STDP (Figure 1D). Spike timing-dependence has since become a foundation on which both theorists and experimentalists seek to build a general understanding of synaptic change, learning, and memory recall.
The discovery of diverse forms of timing-dependent plasticity at different synapses generated excitement because it appeared that such learning rules reflected different information processing and storage needs depending on the particular neural circuit (Figure 2A). These timing-dependent rules are sometimes interpreted as kernels, timing-dependent functions that can predict other properties of synaptic plasticity simply by a superposition of the effects of all pre/post spike pairs. A large body of theoretical work now models plasticity in such a manner (Kempter et al., 1999; Song et al., 2000; van Rossum et al., 2000; Rao and Sejnowski, 2001; Gütig et al., 2003; Izhikevich and Desai, 2003; Cassenaer and Laurent, 2007; Fiete et al., 2010). In this simplifying approach STDP is viewed as the “first law” of synaptic plasticity.
Figure 2. Spike timing dependent plasticity as the first law of synaptic plasticity. (A) Measurements of synaptic plasticity for protocols in which presynaptic and postsynaptic action potentials are repeatedly separated in time by an interval Δtij are made to construct an STDP “kernel” (see STDP as the “first law” of synaptic plasticity? for definition) for a given synapse type. Kernel shapes have been taken to be synapse-specific representations of learning rules (for review see Abbott and Nelson, 2000; Wittenberg and Wang, 2006). (B) Illustration of two common methods for using STDP kernels to predict plasticity from an epoch of neural activity. Left: contributions to plasticity from all pairwise combinations of presynaptic and postsynaptic spikes are included. Right: Only nearest neighbor spike pairs are included. (C) Experiments have demonstrated that very different kernels can be measured at a single synapse. Left: At the CA3–CA1 synapse pairing single presynaptic and postsynaptic action potentials leads to an LTD-only rule. Based on the linear STDP model illustrated in (A,B), no spike pattern would ever result in LTP. Middle: By adding a second postsynaptic action potential, LTP can be induced. This is not predicted by linear STDP. Dashed vertical line corresponds to the time of the first postsynaptic action potential. Right: By decreasing the number of pairings to 20–30, the depression window disappears and an LTP-only kernel is measured. From such a kernel, the existence of LTD would not be predicted. Data from Wittenberg and Wang (2006).
A first-law use of plasticity curves induced by pairs of spikes requires one to assume a strong form of linearity. Timing-dependent learning curves as shown in Figure 1D are typically measured by giving of order 100 pairs of spikes. Computational models assume that one pair of spikes evokes of order 1/100th the amount of plasticity seen in the curve (Figure 2A). The result of all induction protocols, including pre-STDP-era experiments, is then calculated by summing the impact of all spike pairs produced during induction (Figure 2B, red line segments).
Such an approach has been successfully applied to certain systems such as barrel cortex (for instance see Feldman, 2000; Allen et al., 2003; Feldman and Brecht, 2005). To account for further complexity, from this starting point an ever more intricate series of computational models has grown.
The simplest and most common assumption is that all spike pairs count equally (Kempter et al., 1999; Song et al., 2000). Each spike pair has a given Δt associated with it, the time between the presynaptic and postsynaptic spike in the pair (Figure 2B, top). Using the kernel for that synapse, each Δt translates into a change in synaptic weight (Δw), and the total synaptic weight change is simply the linear sum of all the changes. Mathematically, this is done by convolving the STDP kernel with the cross-correlation function between the presynaptic and postsynaptic neuron.
An alternative to counting all spike pairs in two complex trains is to count only plasticity arising from neighboring spike pairs (Izhikevich and Desai, 2003) (Figure 2B, bottom). This rule requires a definition of neighbor pairs, for instance counting the nearest postsynaptic spike to each presynaptic spike (Figure 2B, green lines). Alternatively, one could start from each postsynaptic spike, leading to a different set of spike pairs. A generalization of “nearest neighbor takes all” is “nearest neighbor takes more,” in which a discount function is used to weight near spike pairs more heavily than distant spike pairs (Froemke and Dan, 2002). Such a spike suppression model adds some physiological plausibility, and additional degrees of freedom for fitting, and consequently an improved fit to data.
Another move toward realism is the replacement of additive plasticity with a multiplicative rule (van Rossum et al., 2000; Gütig et al., 2003). LTP and LTD are known to saturate. In an additive model spike pairs contribute until an upper or lower bound is reached. In a multiplicative model the magnitude of change depends on the current synaptic weight, with diminishing contributions as synaptic weight approaches the upper or lower limit. This provides a smoother form of saturation.
A variety of ways in which experiments can deviate from a kernel-style approach can be seen in an applet http://nba.uth.tmc.edu/homepage/shouval/applets/v1/applet02.htm that explores the ensuing predictions. This applet allows the reader to choose a kernel as well as frequency and other parameters. In addition, a rich body of experimental work, starting before the discovery of STDP and continuing after it, can be used to test kernel-based superposition models for inconsistencies.
The first quandary, recognized almost immediately, is the need to explain why low-frequency stimulation of CA3 presynaptic axons results in CA3–CA1 LTD. An STDP kernel with equal-duration timing windows for potentiation and depression suggests two scenarios, neither of which predicts LTD: (1) Presynaptic stimulation drives postsynaptic firing, in which case the timing is in the causal direction and should result in LTP. (2) The presynaptic stimulation does not drive postsynaptic firing, which should result in no plasticity.
In one suggested repair to the model, it was noted that if the postsynaptic neuron fired spontaneously and randomly, and the STDP depression window was larger in area than the potentiation window, LTD would result (Kempter et al., 1999; Song et al., 2000). However, this hypothesis is directly falsified by recordings of the postsynaptic neuron during low-frequency (1 Hz) stimulation of CA3–CA1 synapses in the classical protocol (Dudek and Bear, 1992; Mulkey and Malenka, 1992). Under this condition, all evoked postsynaptic action potentials occurred within 25 ms after presynaptic stimulation yet resulted in LTD (Wittenberg and Wang, 2006). In fact, pairing single presynaptic and postsynaptic action potentials led to an LTD-only STDP kernel (Pike et al., 1999; Wittenberg and Wang, 2006; Figure 2C, left). Such a kernel can never generate LTP by superposition1. Considering that much of what is known about LTP arises from studies of CA3–CA1 synapses, this finding presents a major roadblock to the general applicability of STDP as a first law.
Similarly, starting from an entirely negative STDP kernel, increasing the presynaptic frequency cannot convert LTD to LTP. This problem dates back to the first observations of STDP. Markram et al. (1997) showed in neocortical synapses that causal pairings with Δt = 10 ms led to LTP-only when pairing was done at frequencies above 10 Hz. Conversely, Sjöström et al. (2001) additionally showed that at high enough frequencies the timing-dependent rule becomes LTP-only, i.e., both positive and negative timings produce LTP. Thus plasticity for a particular timing can adopt positive or negative sign depending on pairing frequency.
At CA3–CA1 synapses, several additional mechanisms have been observed to convert causal timing-dependent LTD to causal timing-dependent LTP at CA3–CA1 synapses. The first of these is a firing burst (Pike et al., 1999) or even a pair of spikes (Wittenberg and Wang, 2006) in the postsynaptic neuron, both of which lead to LTP where single spikes lead to LTD. Thus the contribution to plasticity of postsynaptic spikes is affected quite strongly by their arrival in bursts.
Plasticity also accumulates in a nonlinear fashion with respect to the number of pairings (Dudek and Bear, 1992; Yang et al., 1999; Mizuno et al., 2001). At CA3–CA1 synapses, under conditions that allow LTP – pairing presynaptic action potentials with postsynaptic bursts – an LTP-only rule emerges after 10 pairings, but a bidirectional rule requires 100 pairings (Wittenberg and Wang, 2006). Thus plasticity must accumulate as a nonlinear function of the number of stimuli, with depression accumulating more slowly than potentiation.
Finally, neural activity can influence later plasticity for seconds and minutes significantly longer than the STDP window. It has long been understood that neural activity that does not trigger measurable plasticity may have a profound influence on the effects of subsequent neural activity on in synapse strength. A classic example is priming. Rose and Dunwiddie (1986) demonstrated that LTP could be induced with as few as four stimuli to the CA3 pathway, so long as the stimuli were preceded 170 ms earlier by a single priming stimulus. A single burst of five stimuli at 100 Hz without the priming pulse failed to generate plasticity. None of the variations of STDP models described above can explain this primed-burst potentiation. Other work supports the interpretation that activity on this longer timescale is a requirement for LTP (Larson and Lynch, 1988).
In summary, the concept of STDP as the first law of synaptic plasticity is inconsistent with a large body of prior and subsequent existing work. Many parameters other than spike timing have a great enough influence on synaptic plasticity as to generate timing-dependent rules that are either LTD-only or LTP-only, even at the same synapse (Figure 2C).
Although linear superposition of STDP kernels fails, it has still been used as a starting point for making corrections or arguments. Such corrections have met with limited success.
Spike suppression models (Froemke and Dan, 2002; Froemke et al., 2006) were constructed to account for the failure of linear superposition of spike pairs to account for spike triplets and quadruplets in experiments in visual cortex. Although this model does improve fits to data in neocortical slices, it cannot account for the qualitative failures we have described. In particular, if low-frequency pairings lead to a depression-only rule (Markram et al., 1997; Sjöström et al., 2001; Wittenberg and Wang, 2006), the spike suppression model does not explain the emergence of LTP when the pairing frequency is increased or when bursts of postsynaptic spikes are used.
In the case of hippocampal cultures (Wang et al., 2005), results measured using spike triplets have led to a further correction to the linear STDP model. The spike suppression model accounts for the fact that a pre-post-pre spike sequence produces LTP, but not the fact that a post-pre-post sequence can do the same. The patch to the model is an additional rule in which LTP wins over LTD if LTP is triggered first. The generalization of this rule to more complex spike patterns with multiple presynaptic and postsynaptic spikes, resulting in interleaved LTP and LTD epochs, is unclear. A related, more rigorous approach includes higher-order multispike kernels (Pfister and Gerstner, 2006), which by virtue of having more parameters can account for more of the variance in a data set. The multispike kernel method has been separately applied to hippocampal culture data (Wang et al., 2005) and to some neocortical data (Sjöström et al., 2001) but has not been applied, to our knowledge, to triplet and quadruplet data in visual cortical slices (Froemke and Dan, 2002) or to data from hippocampal slices (Nishiyama et al., 2000; Wittenberg and Wang, 2006). This approach requires a new fit for every system, and constitutes a descriptive approach for summarizing the findings at a particular synapse. Also, several problems – nonlinear accumulation and long-timescale effects such as priming – remain unexplained. More rules could undoubtedly be created.
At this point, the initial appeal of the STDP concept has started to dim. The intricacy of the approach is starting to resemble the tax code of a developed country. Does another framework exist in which rules for plasticity arise more naturally?
Here we present an approach based on known biochemical intermediates in the induction of plasticity. In this approach, a mechanistic model is constructed by converting known biological mechanisms to assumptions that are formulated mathematically. These assumptions constitute a model that can be simulated or analyzed under different conditions. The model is constrained by matching the output of the model to experimental results.
As an example we present the calcium-dependent plasticity model (CaDP) of (Shouval et al., 2002). The CaDP model can explain several observed experimental nonlinearities and can be easily modified by adding components that may account for further experimental observations. Such a model can also be used to simulate various slice plasticity protocols (Shouval et al., 2002; Cai et al., 2007) and receptive field plasticity in vivo (Yeung et al., 2004; Yu et al., 2008). Here we focus on STDP-style experiments that are hard to explain by linear superposition models.
The CaDP model is based on three key assumptions.
(1) Calcium elevation in spines determines the sign, magnitude and rate of synaptic plasticity. A moderate elevation in calcium results in LTD whereas a large elevation in calcium levels results in long-term potentiation (LTP) (Figure 3A, left). We also assume that the rate of plasticity is a monotonically increasing function of calcium, η (Figure 3A, middle).
Figure 3. The CaDP model can account for various forms of spike timing dependent plasticity. (A) The key functions controlling the CaDP model. Left: The Ω function controls the sign and magnitude of calcium-dependent synaptic plasticity, the gray shading marks the LTD region. Center: the η function controls the calcium-dependent rate of plasticity. Right: the shape of the back-propagating action potential with its long tail current. (B) The results of an STDP induction protocol, simulating the CaDP model with GNMDA = 1/420 (μM/mV). Left: the calcium transients for baseline, Δt = −10 ms, 0 ms and 30 ms. Here the LTD threshold is θd = 0.35 and the LTP threshold is θp = 0.55. The LTD region is indicated by the gray shading. Right: the complete STDP curve, which exhibits, post-pre LTD, pre-post LTP and also pre-post LTD. (C) The same as (B) but with GNMDA = 1/600 (μM/mV). Here all values of Δt produce LTD. (D) The same as (C) but with two postsynaptic spikes. The timing of the two postsynaptic spikes is indicated by the vertical lines, and the time between the two post spikes is 10 ms. Here we get a complete STDP curve with one LTP window and two LTD windows.
The calcium assumption is based on experimental evidence (Cummings et al., 1996; Yang et al., 1999) and has been previously suggested in models of calcium-dependent kinase-phosphatase systems in postsynaptic spines (Lisman, 1989). Mathematically it is described by the equation:
where wi is the synaptic efficacy of synapse i, [Ca]i is the calcium concentration at synapse i, and λ is a decay time constant. The functions Ω and η (Shouval et al., 2002) determine the sign and rate of synaptic plasticity and are depicted in Figure 3A. Ω is a function of calcium concentration and is defined by two thresholds θd and θp (Figure 3A) that control the sign and magnitude of synaptic plasticity.
(2) The source of calcium is influx through NMDA receptors which pass calcium and are gated by both glutamate and voltage. NMDA receptors can therefore report the coincidence of presynaptically released glutamate and postsynaptic depolarization by allowing calcium into a dendritic spine. NMDA receptors are relatively slow-gating receptors, with time constants in the range of 50–200 ms, a scale comparable to time windows for timing-dependent plasticity.
(3) Back-propagating action potentials (BPAP) in the postsynaptic neuron leave a lingering post-action potential current in the dendrite. The BPAP is the source of depolarization. The assumption of a lingering tail is necessary in order to explain a time window for LTD when the postsynaptic spike precedes the presynaptic spike.
The results of this model depend on a variety of parameter assumptions. Although we will focus on accounting for CA3–CA1 plasticity rules, parameters can be adjusted to account for plasticity properties at other synapses.
In Figure 3B we show induction of STDP with the CaDP model. The functions for Ω, η, and the voltage response of the back- propagating action potential are depicted in Figure 3A, and the NMDA receptor conductance for calcium ions (GNMDA) is set at an appropriate value. These assumptions produce a three-peaked learning rule (Figure 3B): post-pre LTD, pre-post LTP, and pre-post LTD at larger values of Δt. This second LTD window is seen at some synapses (Nishiyama et al., 2000; Woodin et al., 2003; Wittenberg and Wang, 2006) whereas it is absent or less prominent in neocortical synapses examined to date.
If the NMDA conductance is reduced by 30%, single postsynaptic spikes no longer produce LTP at low pairing frequencies (Figure 3C). Now if a burst of two postsynaptic spikes or more is paired with each presynaptic spike, a three-peaked timing-dependent plasticity curve again results (Figure 3D). This rule resembles the triphasic rule that is possible at CA3–CA1 synapses (Nishiyama et al., 2000; Wittenberg and Wang, 2006). This is illustrated in the CaDP applet available at: http://nba.uth.tmc.edu/homepage/shouval/applets/v1/applet01.htm. Other proposed mechanistic models also generate a second LTD window (Kitajima and Hara, 2000; Abarbanel et al., 2002; Karmarkar et al., 2002).
Yet neocortical synapses have multiple mechanisms for LTD including metabotropic glutamate receptor or cannabinoid receptor- dependent signaling (van Rossum et al., 2000; Sjöström et al., 2003; Bender et al., 2006) but lack a prominent second LTD window. Biochemical veto mechanisms have been proposed that can overrule the second LTD window in neocortical synapses (Rubin et al., 2005) but allow it to be expressed at CA3–CA1 synapses. A difference could also be based on biological heterogeneity, for instance the relative abundance of calcium release in CA1 neurons compared with neocortical pyramidal neurons (Nakamura et al., 2000). Finally, stochastic properties of synaptic transmission in conjunction with the CaDP model may significantly reduce the magnitude of the second LTD window (Shouval and Kalantzis, 2005).
In neocortical synapses, LTP results from single postsynaptic spikes at high pairing frequencies, but not at low pairing frequencies (Markram et al., 1997; Sjöström et al., 2001). At high enough frequencies LTD is eliminated entirely. This frequency- dependence is qualitatively consistent with results at CA3–CA1 synapses (Wittenberg and Wang, 2006). Such a transition from bidirectionality to all-LTP falls naturally from the function Ω.
In this simple example we have not included the effects of short-term synaptic dynamics (Tsodyks et al., 1998). In models, short-term facilitation and depression can alter the frequency-dependence of plasticity (Cai et al., 2007) and may account for properties of the plasticity induced by multi-spike protocols (Froemke and Dan, 2002; Wang et al., 2005).
Models that are based on biophysical mechanisms show promise in capturing the fullness of real learning rules. The simple CaDP model described here can account for a number of key aspects of the observed malleability of STDP. In this final section we survey some salient experimental observations that suggest ways in which the CaDP model could be amended and improved. Incorporation of additional mechanism-based rules can move modeling efficiently toward a more complete representation of synaptic learning rules. The point of view of modeling biophysical mechanisms goes beyond calcium: in synapses where other second messengers drive plasticity (Huang et al., 1994; Salin et al., 1996), calcium is not the appropriate target for modeling. Finally, although parsimony argues against adding all the mechanisms described at once, one or more mechanisms could be incorporated for a particular need.
Additional mechanisms that may influence learning rules fall into three broad categories: (a) additional properties of calcium signaling and other messengers, which may influence the dependence of plasticity on temporal activity on time scales of seconds; (b) dendritic excitation and other locally spreading signals, which may influence the dependence of plasticity on the spatial location and pattern of synaptic input; and (c) additional properties of plasticity such as saturable, binary, and irreversible changes in synaptic strength, which may contribute to very long time scale rules, such as metaplasticity. We describe some of these mechanisms and their consequences.
Although the existing CaDP model assumes that calcium rises only from NMDA receptor opening, calcium may be elevated by calcium-permeable AMPA receptors, voltage-gated calcium channels, and calcium release from internal stores (Higley and Sabatini, 2008). Each of these sources is known to contribute to the induction of synaptic plasticity, and may shape the rule. For example, calcium entry through AMPA receptors would be relatively timing- independent and therefore broaden timing windows for plasticity. At cerebellar parallel fiber-Purkinje cell synapses timing- dependence of LTD (Wang et al., 2000a; Safo and Regehr, 2008) may arise from the properties of calcium release driven by the second messenger IP3 (Sarkisov and Wang, 2008).
Synaptic plasticity is regulated by activity on time scales longer than the width of measured STDP kernels. In this regard it is interesting to note that calcium signals are buffered and therefore attenuated by intracellular binding molecules. Some of these molecules are proteins such as calbindin and parvalbumin, which have binding kinetics on the order of 0.1–1 s and saturate at moderate levels of calcium. They are found in hippocampal CA1 pyramidal neurons, cerebellar Purkinje neurons, and many interneurons. A high- frequency pairing requirement for LTP could arise from saturation of buffer proteins. Accumulated calcium also could trigger further calcium release, again leading to a dependence on long time scales. For example, in primed-burst LTP, in which LTP induction depends on activity at the 5 Hz theta frequency at the CA3–CA1 synapse (Rose and Dunwiddie, 1986; Larson and Lynch, 1988; Wittenberg and Wang, 2006) calcium accumulated during the priming activity might saturate buffers or enhance calcium-induced calcium release during the subsequent burst (Schiegg et al., 1995).
Measured calcium dynamics alone are insufficient to account for the direction of synaptic plasticity in the basal dendrites of layer 2/3 pyramidal neurons of the somatosensory cortex (Schultz, 2002; Nevian and Sakmann, 2006). This finding suggests that fluorescence measurements may not capture the key variables that predict plasticity, such as fine spatial and temporal calcium dynamics, or because messengers apart from calcium play a significant role. Indeed, calcium entry through voltage-gated channels may be needed for plasticity in the absence of a measurable calcium signal (Yasuda et al., 2003). Additionally, some signal transduction pathways activated during plasticity might depend on other messengers such as cAMP (Huang et al., 1994; Salin et al., 1996) and endocannabinoids (Safo and Regehr, 2008).
Spike timing dependent plasticity is also modulated by neuromodulatory neurotransmitters. Recently Seol et al. (2007) have shown that in slices of visual cortex, β-adrenergic receptors are necessary for inducing spike timing dependent LTP and muscarinic (M1) receptors are essential for inducing LTD, results that are consistent with in vivo observations. Neuromodulation may translate behavioral state into a capacity for change: for instance, dopamine may act as a reward signal to drive reinforcement learning (Schultz, 2002). Dopamine is capable of subsecond dynamics (Gonon, 1997) providing a substrate for rapid regulation of learning rules (Pawlak and Kerr, 2008). Such effects may be modeled by including messenger molecules such as cyclic AMP, or perhaps simply the neurotransmitters themselves. Recent observations have shown that in cultured synapses, dopamine acting through D1 receptors can convert an antisymmetric STDP rule to a potentiation-only rule with broad timing-dependence that spans both sides of the zero timing condition (Zhang et al., 2009). Such a phenomenon is consistent with enhancements in dendritic excitability, NMDA receptor function, or other calcium signaling or detection machinery.
In spike pair-generated plasticity, the sign and amount of change is known to depend on the dendritic location of the synapse (Froemke et al., 2005). Thus even in a simple case, dendrites are electrically inhomogeneous. In addition, dendrites show a rich range of excitable properties (Sjöström et al., 2008). Dendritic spikes are commonly evoked by sufficiently dense excitation to activate voltage-gated channels (Miyakawa et al., 1992; Wang et al., 2000a) (Larkum and Nevian, 2008; Sjöström et al., 2008) or NMDA receptors themselves (Schiller and Schiller, 2001; Major et al., 2008). Consequently, plasticity can be evoked via local dendritic spikes independent of somatic firing (Hartell, 1996; Golding et al., 2002).
Such observations can naturally be incorporated into CaDP models as upstream steps that regulate the amount of calcium entry. This requires modeling of active dendritic conductances, or identification of rules that map cellular activity to patterns of change in dendritic voltage. Such models should be able to account for the properties of plasticity driven by dendritic spikes (Larkum and Nevian, 2008; Sjöström et al., 2008). Dendritic excitation may also account for locally spreading heterosynaptic LTP and LTD, in which synaptic activity can cause plasticity at near (Johnston et al., 2003) by synapses (Engert and Bonhoeffer, 1997; Wang et al., 2000b).
Signaling molecules may spread from active to inactive synapses. Svoboda and colleagues (Yasuda et al., 2006; Harvey and Svoboda, 2007; Zhong et al., 2009) have demonstrated that activity at one synapse on a CA1 neuron can increase the sensitivity of that synapse to further change without inducing plasticity. This effect lasts approximately 10 min and requires the phosphorylation of Ras, a calcium-dependent G protein that regulates MAP kinase. They also demonstrated that the priming effect can spread about 10 μ, thereby sensitizing neighboring spines to an induction stimulus that would otherwise not lead to plasticity.
Such spreading signals are not limited to synaptic plasticity, nor are they always local. An old phenomenon somewhat unappreciated in models is the fact that the induction of plasticity is typically accompanied by changes in the excitability of the postsynaptic neuron. It has also been shown that activity can lead to local changes in dendritic excitability on a scale of microns (Johnston et al., 2003; Sjöström et al., 2008) comprising a form of information storage (Narayanan and Johnston, 2007).
These complexities suggest that molecular mechanisms of plasticity may account for priming on a location and proximity- dependent basis. In one attractive hypothesis, co-activation of nearby inputs on an excitable dendrite could serve to drive plasticity in a group of synapses. In this scenario, functionally related inputs could become clustered if the plasticity signal drives LTP (Mehta, 2004; Larkum and Nevian, 2008). Conversely, LTD driven by large calcium signals, which occurs at parallel fiber-Purkinje cell synapses, might lead to repulsion of related inputs from one another and thus sparse mapping on the dendritic arbor.
The CaDP model described here produces graded synaptic weights. However, the induction of plasticity appears experimentally to be sudden and discrete, and possibly even a two-state system of binary strength (Petersen et al., 1998; O’Connor et al., 2005b). Several models have taken the observations of discrete plasticity states into account (Abarbanel et al., 2005; Graupner and Brunel, 2007; Clopath et al., 2008). Possible substrates for discrete states include CaMKII autophosphorylation (Lisman and Zhabotinsky, 2001) and other maintenance mechanisms are also likely to form discrete states (Aslam et al., 2009). Such binary changes have been observed on time scales of tens of minutes; on longer time scales, the levels of such states could change. For instance, the “high” state could be defined by the number of slots for AMPA receptor insertion (McCormack et al., 2006), which could change via metaplastic and homeostatic mechanisms (Rioult-Pedotti et al., 2007).
Stepwise, saturable change in synaptic strength has two consequences. First, the saturation of plasticity suggests that even for a fixed rule for mapping calcium to plasticity, the measured learning rule will depend on the initial synaptic strength. This can account for the finding that after saturation of LTD, a potentiation-only learning rule results, and vice versa, a simple form of change in a learning rule over time, or metaplasticity (O’Connor et al., 2005a). Second, when the number of active synapses is small, as occurs between pairs of neurons, the amount and sign of plasticity can vary considerably based on counting statistics alone. Saturable change at individual terminals could even account for the observation that a given amplitude of calcium transient can evoke either LTP or LTD (Nevian and Sakmann, 2006) without revoking assumption (1) of the CaDP model.
For both Hebbian rate-based learning rules and linear STDP-based rules, runaway synaptic plasticity can occur (Bienenstock et al., 1982; Oja, 1982). The problem is not solved by imposing upper and lower bounds on synaptic weights, since synaptic weights can still saturate, leading to nonselective cells that respond equally to many input patterns. This problem of linear STDP models is associated with causality, which tends to result in presynaptic neurons firing slightly before postsynaptic neurons, and thus producing LTP. Therefore, synaptic saturation observed in linear STDP can be addressed by using an STDP kernel with slightly more LTD than LTP (Kempter et al., 1999; Song et al., 2000).
Mechanistic models, which try to account for system level phenomena, like rate-based models, require stabilization mechanisms (Yeung et al., 2004; Yu et al., 2008; Clopath et al., 2010). It has been suggested that synaptic scaling (Turrigiano et al., 1998) might result in overall homeostatic change that reduces change in total synaptic strength on a neuron, which can prevent runaway plasticity.
Such stabilization mechanisms seem related to metaplasticity observed experimentally (Abraham and Bear, 1996). Metaplasticity is at times used as a catch-all term for changes in learning rule. It is feasible that metaplasticity and synaptic scaling (Yeung et al., 2004) may arise naturally from cellular mechanisms that have a stabilizing influence. Synaptic plasticity models should therefore incorporate biophysical implementations of stabilization mechanisms for comparison to experiment.
Experimentally, LTP is not the same on all time scales. For example, the late phase of LTP (L-LTP) has elements of consolidation, lasting for hours or longer, and requires protein synthesis and stronger activation than most LTP induction protocols (Frey et al., 1993). On shorter time scales, a related phenomenon, is the irreversibility of LTP under stimulation conditions more intense than the minimum needed to induce potentiation (Stäubli and Chun, 1996; O’Connor et al., 2005a). Such a “lock-in” (O’Connor et al., 2005a) concept has been modeled using deeper levels of plasticity (Stäubli and Chun, 1996; Fusi et al., 2005; O’Connor et al., 2005b). This approach has not yet been combined with spike timing-dependent models of learning.
This review has focused on bringing a directed dose of mechanistic complexity to theoretical models, moving beyond the initial notion that STDP is essentially a first law of synaptic plasticity. We advocate the use of simple biophysical models of plasticity that can be constrained both by the realism of their mechanistic assumptions and by comparison with experiment.
Although the high dimensionality of the parameter space governing synaptic plasticity appears daunting (Figure 4), new approaches may be helpful. At present, sampling this parameter space typically requires monitoring the electrophysiological response of one postsynaptic cell for up to an hour. Simultaneous patching of multiple neurons can increase the number of experiments which can be performed in parallel, but this approach is not scalable. However, technologies such as patterned and spatially resolved uncaging (Civillico et al., 2010) and optogenetic manipulation of identified neuron types (Gradinaru et al., 2007) enable many connections to be probed on a high-throughput basis. These experimental directions promise to provide quantities of data necessary to constrain models better. As progress in these areas continues, the ability to sample the parameter space will improve.
Figure 4. Spike timing is merely one dimension in the high-dimensional synaptic learning rule. A conceptual illustration of a learning rule in three dimensions is shown. Depending on the choice of activity parameters other than spike timing, many different STDP rules can be measured at a synapse (from Wittenberg and Wang, 2006). The second axis represents the transition from parameters that more strongly activate depression (D-rule) to parameters that more strongly activate potentiation (P-rule). By choosing parameters that activate only a single rule, the spike timing-dependence of LTP and LTD can be measured separately. At the CA3–CA1 synapse, potentiation is initiated by as few as 20 causal pairings of presynaptic action potentials with postsynaptic bursts repeated at 5 Hz or higher. Depression does not require high-frequency stimulation or postsynaptic bursts but requires more pairings than LTP. Stimulus conditions that satisfy the temporal requirements for both the potentiation rule and the depression rule lead to a bidirectional spike-timing-dependent plasticity curve. In neocortex one can shift along the P–D axis by changing the pairing frequency (Sjöström et al., 2001), or by neuromodulator concentration (Seol et al., 2007).
Biophysically oriented models of plasticity may also eventually be useful in network models in order to predict the properties of circuit structure. For example, standard STDP models favor the elimination of reciprocal connections between cortical neurons because any given timing would lead to LTP in one direction and LTD in the other direction. This is contradicted by the fact that early experiments (Markram et al., 1997) were done at reciprocally connected pairs, as well as the well-known phenomenon that distant neocortical areas are often reciprocally connected (Felleman and Van Essen, 1991). Of interest is recent work (Clopath et al., 2010) presenting a phenomenological model of plasticity that includes additional aspects of plasticity such as frequency-dependence, and that allows both unidirectional and bidirectional connections to develop. Additional rules such as these come easily from mechanistic considerations, suggesting that a biophysical approach can eventually help account for circuit-level phenomena.
Ultimately we should strive to create biophysically based system levels model of neuronal circuits. Such models will enable us to connect the molecular and cellular level basis of plasticity to its consequences on the circuit and system levels, and to test the models both on the basis of their underlying low-level assumptions as well as on their higher-level predictions. The space of models and activity parameters to explore can be reduced considerably by sampling activity patterns likely to occur in a behaving animal, and advances in recording methods promise to provide continuing new sources of data. In this regard a valuable source of insight into any synapse’s function is the experimental literature on multiple levels: in vivo activity patterns, plasticity, and behavior.
An open question is whether, over the natural stimuli of a synapse in a given region, final plasticity rules will be so high-dimensional that very complex, essentially descriptive rules will be needed for realistic modeling. We take the optimistic view that “real rules”, which are nevertheless relatively simple, can be found, and that this is more easily accomplished using a biophysical approach. Although the linear STDP models do not account for most of the experimental results, much of the original appeal of this approach lay in the possibility that it could be used in higher-level models. A key future question is whether a biophysically based approach can contribute a simple description sufficient to help model working neural circuits. Such an approach may also aid the eventual understanding of the role of spike timing – and many other factors – in determining the principles by which neural circuits learn.
The authors declare that the research was conducted in the absence of any commercial or financial relationships that could be construed as a potential conflict of interest.
Harel Z. Shouval was supported by a grant from the NIH (2P01-NS038310). Samuel S.-H. Wang was supported by a grant from the NIH (1R01-NS045193) and is a W. M. Keck Distinguished Young Scholar in Medical Research. We thank Mark S. Goldman for his comments on the manuscript and Michael J. Byrne for the Java applets.
Abarbanel, H. D., Huerta, R., and Rabinovich, M. I. (2002). Dynamical model of long-term synaptic plasticity. Proc. Natl. Acad. Sci. U.S.A. 99, 10132–10137.
Abarbanel, H. D., Talathi, S. S., Gibb, L., and Rabinovich, M. I. L. (2005). Synaptic plasticity with discrete state synapses. Phys. Rev. E Stat. Nonlin. Soft Matter Phys. 72, 031914.
Abbott, L. F., and Nelson, S. B. (2000). Synaptic plasticity: taming the beast. Nat. Neurosci. 3(Suppl.), 1178–1183.
Abraham, W. C., and Bear, M. F. (1996). Metaplasticity: the plasticity of synaptic plasticity. Trends Neurosci. 19, 126–130.
Allen, C. B., Celikel, T., and Feldman, D. E. (2003). Long-term depression induced by sensory deprivation during cortical map plasticity in vivo. Nat. Neurosci. 6, 291–299.
Amit, D. J., and Brunel, N. (1997). Model of global spontaneous activity and local structured activity during delay periods in the cerebral cortex. Cereb. Cortex 7, 237–252.
Artola, A., Brocher, S., and Singer, W. (1990). Different voltage-dependent thresholds for inducing long-term depression and long-term potentiation in slices of rat visual cortex. Nature 347, 69–72.
Aslam, N., Kubota, Y., Wells, D., and Shouval, H. Z. (2009). Translational switch for long-term maintenance of synaptic plasticity. Mol. Syst. Biol. 5, 284.
Bell, C. C., Han, V. Z., Sugawara, Y., and Grant, K. (1997). Synaptic plasticity in a cerebellum-like structure depends on temporal order. Nature 387, 278–281.
Bender, V. A., Bender, K. J., Brasier, D. J., and Feldman, D. E. (2006). Two coincidence detectors for spike timing-dependent plasticity in somatosensory cortex. J. Neurosci. 26, 4166–4177.
Bi, G. Q., and Poo, M. M. (1998). Synaptic modifications in cultured hippocampal neurons: dependence on spike timing, synaptic strength, and postsynaptic cell type. J. Neurosci. 18, 10464–10472.
Bienenstock, E. L., Cooper, L. N., and Munro, P. W. (1982). Theory for the development of neuron selectivity: orientation specificity and binocular interaction in visual cortex. J. Neurosci. 2, 32–48.
Bliss, T. V. P., and Gardner-Medwin, A. R. (1973). Long-lasting potentiation of synaptic transmission in the dentate area of the unanaestetized rabbit following stimulation of the perforant path. J. Physiol. 232, 357–374.
Bliss, T. V. P., and Lømo, T. (1973). Long-lasting potentiation of synaptic transmission in the dentate area of the anaesthetized rabbit following stimulation of the perforant path. J. Physiol. 232, 331–356.
Cai, Y., Gavornik, J. P., Cooper, L. N., Yeung, L. C., and Shouval, H. Z. (2007). Effect of stochastic synaptic and dendritic dynamics on synaptic plasticity in visual cortex and hippocampus. J. Neurophysiol. 97, 375–386.
Cassenaer, S., and Laurent, G. (2007). Hebbian STDP in mushroom bodies facilitates the synchronous flow of olfactory information in locusts. Nature 448, 709–713.
Civillico, E. F., Rickgauer, J. P., and Wang, S. S.-H. (2010). “Targeting and excitation of photoactivatable molecules: design considerations for neurophysiology experiments,” in Photoactivatable Molecules for Biology, eds J. J. Chambers and R. H. Kramer (New York: Humana Press), (in press).
Clopath, C., Busing, L., Vasilaki, E., and Gerstner, W. (2010). Connectivity reflects coding: a model of voltage-based STDP with homeostasis. Nat. Neurosci. 13, 344–352.
Clopath, C., Ziegler, L., Vasilaki, E., Busing, L., and Gerstner, W. (2008). Tag-trigger-consolidation: a model of early and late long-term-potentiation and depression. PLoS Comput. Biol. 4, e1000248. doi:10.1371/journal.pcbi.1000248.
Cummings, J. A., Mulkey, R. M., Nicoll, R. A., and Malenka, R. C. (1996). Ca2+ signaling requirements for long-term depression in the hippocampus. Neuron 16, 825–833.
Dudek, S. M., and Bear, M. F. (1992). Homosynaptic long-term depression in area CA1 of hippocampus and effects of N-methyl-D-aspartate receptor blockade. Proc. Natl. Acad. Sci. U.S.A. 89, 4363–4367.
Engert, F., and Bonhoeffer, T. (1997). Synapse specificity of long-term potentiation breaks down at short distances. Nature 388, 279–284.
Feldman, D. E. (2000). Timing-based LTP and LTD at vertical inputs to layer II/III pyramidal cells in rat barrel cortex. Neuron 27, 45–56.
Feldman, D. E., and Brecht, M. (2005). Map plasticity in somatosensory cortex. Science 310, 810–815.
Felleman, D. J., and Van Essen, D. C. (1991). Distributed hierarchical processing in the primate cerebral cortex. Cereb. Cortex 1, 1–47.
Fiete, I. R., Senn, W., Wang, C. Z. H., and Hahnloser, R. H. R. (2010). Spike-time-dependent plasticity and heterosynaptic competition organize networks to produce long scale-free sequences of neural activity. Neuron 65, 563–576.
Frey, U., Huang, Y. Y., and Kandel, E. R. (1993). Effects of cAMP simulate a late stage of LTP in hippocampal CA1 neurons. Science 260, 1661–1664.
Froemke, R. C., and Dan, Y. (2002). Spike-timing-dependent synaptic modification induced by natural spike trains. Nature 416, 433–438.
Froemke, R. C., Poo, M. M., and Dan, Y. (2005). Spike-timing-dependent synaptic plasticity depends on dendritic location. Nature 434, 221–225.
Froemke, R. C., Tsay, I. A., Raad, M., Long, J. D., and Dan, Y. (2006). Contribution of individual spikes in burst-induced long-term synaptic modification. J. Neurophysiol. 95, 1620–1629.
Fusi, S., Drew, P. J., and Abbott, L. F. (2005). Cascade models of synaptically stored memories. Neuron 45, 599–611.
Gerstner, W., Kempter, R., van Hemmen, J. L., and Wagner, H. (1996). A neuronal learning rule for sub-millisecond temporal coding. Nature 383, 76–81.
Gerstner, W., and Kistler, W. M. (2002). Spiking Neuron Models: Single Neurons, Populations, Plasticity. Cambridge: Cambridge University Press.
Golding, N. L., Staff, N. P., and Spruston, N. (2002). Dendritic spikes as a mechanism for cooperative long-term potentiation. Nature 418, 326–331.
Gonon, F. (1997). Prolonged and extrasynaptic excitatory action of dopamine mediated by D1 receptors in the rat striatum in vivo. J. Neurosci. 17, 5972–5978.
Gradinaru, V., Thompson, K. R., Zhang, F., Mogri, M., Kay, K., Schneider, M. B., and Deisseroth, K. (2007). Targeting and readout strategies for fast optical neural control in vitro and in vivo. J. Neurosci. 27, 14231–14238.
Graupner, M., and Brunel, N. (2007). STDP in a bistable synapse model based on CaMKII and associated signaling pathways. PLoS Comput. Biol. 3, e221. doi:10.1371/journal.pcbi.0030221.
Gütig, R., Aharonov, R., Rotter, S., and Sompolinsky, H. (2003). Learning input correlations through nonlinear temporally asymmetric Hebbian plasticity. J. Neurosci. 23, 3697–3714.
Hartell, N. A. (1996). Strong activation of parallel fibers produces localized calcium transients and a form of LTD that spreads to distant synapses. Neuron 16, 601–610.
Harvey, C. D., and Svoboda, K. (2007). Locally dynamic synaptic learning rules in pyramidal neuron dendrites. Nature 450, 1195–1200.
Higley, M. J., and Sabatini, B. L. (2008). Calcium signaling in dendrites and spines: practical and functional considerations. Neuron 59, 902–913.
Hirase, H., Czurko, A., Csicsvari, J., and Buzsaki, G. (1999). Firing rate and theta-phase coding by hippocampal pyramidal neurons during ‘space clamping’. Eur. J. Neurosci. 11, 4373–4380.
Hopfield, J. J. (1984). Neurons with graded response have collective computational properties like those of two-state neurons. Proc. Natl. Acad. Sci. U.S.A. 81, 3088–3092.
Hopfield, J. J. (1995). Pattern recognition computation using action potential timing for stimulus representation. Nature 376, 33–36.
Huang, Y. Y., Li, X. C., and Kandel, E. R. (1994). cAMP contributes to mossy fiber LTP by initiating both a covalently mediated early phase and macromolecular synthesis-dependent late phase. Cell 79, 69–79.
Johnston, D., Christie, B. R., Frick, A., Gray, R., Hoffman, D. A., Schexnayder, L. K., Watanabe, S., and Yuan, L. L. (2003). Active dendrites, potassium channels and synaptic plasticity. Philos. Trans. R. Soc. Lond., B, Biol. Sci. 358, 667–674.
Karmarkar, U. R., Najarian, M. T., and Buonomano, D. V. (2002). Mechanisms and significance of spike-timing dependent plasticity. Biol. Cybern. 87, 373–382.
Kempter, R., Gerstner, W., and van Hemmen, L. (1999). Hebbian learning and spiking neurons. Phys. Rev. E 59, 4498–4514.
Kitajima, T., and Hara, K. (2000). A generalized Hebbian rule for activity-dependent synaptic modifications. Neural. Netw. 13, 445–454.
Konorski, J. (1948). Conditioned Reflexes and Neuron Organization. Cambridge: Cambridge University Press.
Larkum, M. E., and Nevian, T. (2008). Synaptic clustering by dendritic signalling mechanisms. Curr. Opin. Neurobiol. 18, 321–331.
Larson, J., and Lynch, G. (1988). Role of N-methyl-D-aspartate receptors in the induction of synaptic potentiation by burst stimulation patterned after the hippocampal theta-rhythm. Brain Res. 441, 111–118.
Levy, W. B., and Steward, O. (1983). Temporal contiguity requirements for long-term associative potentiation/depression in the hippocampus. Neuroscience 8, 791–797.
Lisman, J. (1989). A mechanism for the Hebb and the anti-Hebb processes underlying learning and memory. Proc. Natl. Acad. Sci. U.S.A. 86, 9574–9578.
Lisman, J. E., and Zhabotinsky, A. M. (2001). A model of synaptic memory: a CaMKII/PP1 switch that potentiates transmission by organizing an AMPA receptor anchoring assembly. Neuron 31, 191–201.
Major, G., Polsky, A., Denk, W., Schiller, J., and Tank, D. W. (2008). Spatiotemporally graded NMDA spike/plateau potentials in basal dendrites of neocortical pyramidal neurons. J. Neurophysiol. 99, 2584–2601.
Markram, H., Lubke, J., Frotscher, M., and Sakmann, B. (1997). Regulation of synaptic efficacy by coincidence of postsynaptic APs and EPSPs. Science 275, 213–215.
McCormack, S. G., Stornetta, R. L., and Zhu, J. J. (2006). Synaptic AMPA receptor exchange maintains bidirectional plasticity. Neuron 50, 75–88.
Mehta, M. R. (2004). Cooperative LTP can map memory sequences on dendritic branches. Trends Neurosci. 27, 69–72.
Miyakawa, H., Lev-Ram, V., Lasser-Ross, N., and Ross, W. N. (1992). Calcium transients evoked by climbing fiber and parallel fiber synaptic inputs in guinea pig cerebellar Purkinje neurons. J. Neurophysiol. 68, 1178–1189.
Mizuno, T., Kanazawa, I., and Sakurai, M. (2001). Differential induction of LTP and LTD is not determined solely by instantaneous calcium concentration: an essential involvement of a temporal factor. Eur. J. Neurosci. 14, 701–708.
Mulkey, R. M., and Malenka, R. C. (1992). Mechanisms underlying induction of homosynaptic long-term depression in area CA1 of the hippocampus. Neuron 9, 967–975.
Nakamura, T., Nakamura, K., Lasser-Ross, N., Barbara, J. G., Sandler, V. M., and Ross, W. N. (2000). Inositol 1,4,5-trisphosphate (IP3)-mediated Ca2+ release evoked by metabotropic agonists and backpropagating action potentials in hippocampal CA1 pyramidal neurons. J. Neurosci. 20, 8365–8376.
Narayanan, R., and Johnston, D. (2007). Long-term potentiation in rat hippocampal neurons is accompanied by spatially widespread changes in intrinsic oscillatory dynamics and excitability. Neuron 56, 1061–1075.
Nevian, T., and Sakmann, B. (2006). Spine Ca2+ signaling in spike-timing-dependent plasticity. J. Neurosci. 26, 11001–11013.
Ngezahayo, A., Schachner, M., and Artola, A. (2000). Synaptic activity modulates the induction of bidirectional synaptic changes in adult mouse hippocampus. J. Neurosci. 20, 2451–2458.
Nishiyama, M., Hong, K., Mikoshiba, K., Poo, M. M., and Kato, K. (2000). Calcium stores regulate the polarity and input specificity of synaptic modification. Nature 408, 584–588.
O’Connor, D. H., Wittenberg, G. M., and Wang, S. S.-H. (2005a). Dissection of bidirectional synaptic plasticity into saturable unidirectional processes. J. Neurophysiol. 94, 1565–1573.
O’Connor, D. H., Wittenberg, G. M., and Wang, S. S.-H. (2005b). Graded bidirectional synaptic plasticity is composed of switch-like unitary events. Proc. Natl. Acad. Sci. U.S.A. 102, 9679–9684.
Oja, E. (1982). A simplified neuron model as a principal component analyzer. J. Math. Biol. 15, 267–273.
Pawlak, V., and Kerr, J. N. (2008). Dopamine receptor activation is required for corticostriatal spike- timing-dependent plasticity. J. Neurosci. 28, 2435–2446.
Petersen, C. C., Malenka, R. C., Nicoll, R. A., and Hopfield, J. J. (1998). All-or-none potentiation at CA3-CA1 synapses. Proc. Natl. Acad. Sci. U.S.A. 95, 4732–4737.
Pfister, J. P., and Gerstner, W. (2006). Triplets of spikes in a model of spike timing-dependent plasticity. J. Neurosci. 26, 9673–9682.
Pike, F. G., Meredith, R. M., Olding, A. W., and Paulsen, O. (1999). Rapid report: postsynaptic bursting is essential for ‘Hebbian’ induction of associative long-term potentiation at excitatory synapses in rat hippocampus. J. Physiol. 518(Pt 2), 571–576.
Rao, R. P., and Sejnowski, T. J. (2001). Spike-timing-dependent Hebbian plasticity as temporal difference learning. Neural. Comput. 13, 2221–2237.
Rioult-Pedotti, M. S., Donoghue, J. P., and Dunaevsky, A. (2007). Plasticity of the synaptic modification range. J. Neurophysiol. 98, 3688–3695.
Rose, G. M., and Dunwiddie, T. V. (1986). Induction of hippocampal long-term potentiation using physiologically patterned stimulation. Neurosci. Lett. 69, 244–248.
Rubin, J. E., Gerkin, R. C., Bi, G. Q., and Chow, C. C. (2005). Calcium time course as a signal for spike-timing-dependent plasticity. J. Neurophysiol. 93, 2600–2613.
Rumelhart, D. E., and McClelland, J. E. (1987). Parallel Distributed Processing, Vol.1 Foundations. Cambridge, MA: MIT press.
Safo, P., and Regehr, W. G. (2008). Timing dependence of the induction of cerebellar LTD. Neuropharmacology 54, 213–218.
Salin, P. A., Malenka, R. C., and Nicoll, R. A. (1996). Cyclic AMP mediates a presynaptic form of LTP at cerebellar parallel fiber synapses. Neuron 16, 797–803.
Sarkisov, D. V., and Wang, S. S. -H. (2008). Order-dependent coincidence detection in cerebellar Purkinje neurons at the inositol trisphosphate receptor. J. Neurosci. 28, 133–142.
Schiegg, A., Gerstner, W., Ritz, R., and van Hemmen, J. L. (1995). Intracellular Ca2+ stores can account for the time course of LTP induction: a model of Ca2+ dynamics in dendritic spines. J. Neurophysiol. 74, 1046–1055.
Schiller, J., and Schiller, Y. (2001). NMDA receptor-mediated dendritic spikes and coincident signal amplification. Curr. Opin. Neurobiol. 11, 343–348.
Sejnowski, T. J. (1977). Storing covariance with nonlinearly interacting neurons. J. Math. Biol. 4, 303–321.
Seol, G. H., Ziburkus, J., Huang, S., Song, L., Kim, I. T., Takamiya, K., Huganir, R. L., Lee, H. K., and Kirkwood, A. (2007). Neuromodulators control the polarity of spike-timing-dependent synaptic plasticity. Neuron 55, 919–929.
Shouval, H. Z., Bear, M. F., and Cooper, L. N. (2002). A unified model of NMDA receptor-dependent bidirectional synaptic plasticity. Proc. Natl. Acad. Sci. U.S.A. 99, 10831–10836.
Shouval, H. Z., and Kalantzis, G. (2005). Stochastic properties of synaptic transmission affect the shape of spike time-dependent plasticity curves. J. Neurophysiol. 93, 1069–1073.
Sjöström, P. J., Rancz, E. A., Roth, A., and Häusser, M. (2008). Dendritic excitability and synaptic plasticity. Physiol. Rev. 88, 769–840.
Sjöström, P. J., Turrigiano, G. G., and Nelson, S. B. (2001). Rate, timing, and cooperativity jointly determine cortical synaptic plasticity. Neuron 32, 1149–1164.
Sjöström, P. J., Turrigiano, G. G., and Nelson, S. B. (2003). Neocortical LTD via coincident activation of presynaptic NMDA and cannabinoid receptors. Neuron 39, 641–654.
Song, S., Miller, K. D., and Abbott, L. F. (2000). Competitive Hebbian learning through spike-timing-dependent synaptic plasticity. Nat. Neurosci. 3, 919–926.
Stäubli, U., and Chun, D. (1996). Factors regulating the reversibility of long-term potentiation. J. Neurosci. 16, 853–860.
Stent, G. S. (1973). A physiological mechanism for Hebb’s postulate of learning. Proc. Natl. Acad. Sci. U.S.A. 70, 997–1001.
Tsodyks, M., Pawelzik, K., and Markram, H. (1998). Neural networks with dynamic synapses. Neural. Comput. 10, 821–835.
Turrigiano, G. G., Leslie, K. R., Desai, N. S., Rutherford, L. C., and Nelson, S. B. (1998). Activity-dependent scaling of quantal amplitude in neocortical neurons. Nature 391, 892–896.
van Rossum, M. C., Bi, G. Q., and Turrigiano, G. G. (2000). Stable Hebbian learning from spike timing-dependent plasticity. J. Neurosci. 20, 8812–8821.
Wang, H. X., Gerkin, R. C., Nauen, D. W., and Bi, G. Q. (2005). Coactivation and timing-dependent integration of synaptic potentiation and depression. Nat. Neurosci. 8, 187–193.
Wang, S. S.-H., Denk, W., and Häusser, M. (2000a). Coincidence detection in single dendritic spines mediated by calcium release. Nat. Neurosci. 3, 1266–1273.
Wang, S. S.-H., Khiroug, L., and Augustine, G. J. (2000b). Quantification of spread of cerebellar long-term depression with chemical two-photon uncaging of glutamate. Proc. Natl. Acad. Sci. U.S.A. 97, 8635–8640.
Wiesel, T. N., and Hubel, D. H. (1963). Effects of Visual Deprivation on Morphology and Physiology of Cells in the Cats Lateral Geniculate Body. J. Neurophysiol. 26, 978–993.
Wilson, H. R., and Cowan, J. D. (1973). A mathematical theory of the functional dynamics of cortical and thalamic nervous tissue. Kybernetik 13, 55–80.
Wittenberg, G. M., and Wang, S. S.-H. (2006). Malleability of spike-timing-dependent plasticity at the CA3-CA1 synapse. J. Neurosci. 26, 6610–6617.
Woodin, M. A., Ganguly, K., and Poo, M. M. (2003). Coincident pre- and postsynaptic activity modifies GABAergic synapses by postsynaptic changes in Cl- transporter activity. Neuron 39, 807–820.
Yang, S. N., Tang, Y. G., and Zucker, R. S. (1999). Selective induction of LTP and LTD by postsynaptic [Ca2+]i elevation. J. Neurophysiol. 81, 781–787.
Yasuda, R., Harvey, C. D., Zhong, H., Sobczyk, A., van Aelst, L., and Svoboda, K. (2006). Supersensitive Ras activation in dendrites and spines revealed by two-photon fluorescence lifetime imaging. Nat. Neurosci. 9, 283–291.
Yasuda, R., Sabatini, B. L., and Svoboda, K. (2003). Plasticity of calcium channels in dendritic spines. Nat. Neurosci. 6, 948–955.
Yeung, L. C., Shouval, H. Z., Blais, B. S., and Cooper, L. N. (2004). Synaptic homeostasis and input selectivity follow from a calcium-dependent plasticity model. Proc. Natl. Acad. Sci. U.S.A. 101, 14943–14948.
Yu, X., Shouval, H. Z., and Knierim, J. J. (2008). A biophysical model of synaptic plasticity and metaplasticity can account for the dynamics of the backward shift of hippocampal place fields. J. Neurophysiol. 100, 983–992.
Zhang, J. C., Lau, P. M., and Bi, G. Q. (2009). Gain in sensitivity and loss in temporal contrast of STDP by dopaminergic modulation at hippocampal synapses. Proc. Natl. Acad. Sci. U.S.A. 106, 13028–13033.
Keywords: STDP, synaptic plasticity, mechanistic models, calcium, learning rules, long-term depression, long-term potentiation
Citation: Shouval HZ, Wang SS-H and Wittenberg GM (2010) Spike timing dependent plasticity: a consequence of more fundamental learning rules. Front. Comput. Neurosci. 4:19. doi: 10.3389/fncom.2010.00019
Received: 07 March 2010;
Paper pending published: 17 April 2010;
Accepted: 07 June 2010;
Published online: 01 July 2010
Edited by:
Per Jesper Sjöström, University College London, UKReviewed by:
Wulfram Gerstner, Ecole Polytechnique Fédérale de Lausanne, SwitzerlandCopyright: © 2010 Shouval, Wang and Wittenberg. This is an open-access article subject to an exclusive license agreement between the authors and the Frontiers Research Foundation, which permits unrestricted use, distribution, and reproduction in any medium, provided the original authors and source are credited.
*Correspondence: Harel Z. Shouval, Department of Neurobiology and Anatomy, The University of Texas Medical School at Houston, 6431 Fannin St., Houston, TX 77303, USA. e-mail:aGFyZWwuc2hvdXZhbEB1dGgudG1jLmVkdQ==
Disclaimer: All claims expressed in this article are solely those of the authors and do not necessarily represent those of their affiliated organizations, or those of the publisher, the editors and the reviewers. Any product that may be evaluated in this article or claim that may be made by its manufacturer is not guaranteed or endorsed by the publisher.
Research integrity at Frontiers
Learn more about the work of our research integrity team to safeguard the quality of each article we publish.