- 1Department of Neurology, Beidahuang Industry Group General Hospital, Harbin, China
- 2Institute for Stroke and Dementia Research (ISD), LMU Klinikum, Ludwig-Maximilian-University (LMU), Munich, Germany
- 3Munich Medical Research School (MMRS), LMU Klinikum, Ludwig-Maximilian-University (LMU), Munich, Germany
Neural circuits are characterized as interconnecting neuron networks connected by synapses. Some kinds of gene expression and/or functional changes of neurons and synaptic connections may result in aberrant neural circuits, which has been recognized as one crucial pathological mechanism for the onset of many neurological diseases. Gradual advances in single-cell sequencing approaches with strong technological advantages, as exemplified by high throughput and increased resolution for live cells, have enabled it to assist us in understanding neuronal diversity across diverse brain regions and further transformed our knowledge of cellular building blocks of neural circuits through revealing numerous molecular signatures. Currently published transcriptomic studies have elucidated various neuronal subpopulations as well as their distribution across prefrontal cortex, hippocampus, hypothalamus, and dorsal root ganglion, etc. Better characterization of brain region-specific circuits may shed light on new pathological mechanisms involved and assist in selecting potential targets for the prevention and treatment of specific neurological disorders based on their established roles. Given diverse neuronal populations across different brain regions, we aim to give a brief sketch of current progress in understanding neuronal diversity and neural circuit complexity according to their locations. With the special focus on the application of single-cell sequencing, we thereby summarize relevant region-specific findings. Considering the importance of spatial context and connectivity in neural circuits, we also discuss a few published results obtained by spatial transcriptomics. Taken together, these single-cell sequencing data may lay a mechanistic basis for functional identification of brain circuit components, which links their molecular signatures to anatomical regions, connectivity, morphology, and physiology. Furthermore, the comprehensive characterization of neuron subtypes, their distributions, and connectivity patterns via single-cell sequencing is critical for understanding neural circuit properties and how they generate region-dependent interactions in different context.
Introduction
Neurons, the most critical structural as well as functional components of the nervous system, are divided into sensory neurons, motor neurons, and interneurons in the functional sense (Peng et al., 2021; Zeng, 2022). Due to their genetic diversity and wide distribution across brain regions, specific types of neurons generally have distinct functions (Beine et al., 2022; Breton-Provencher et al., 2022). Interestingly, these neurons do not work individually. In other words, developing neurons and their synaptic partners interconnect with each other, forming complex neural circuits to exert specific functions once activated (Sanes and Zipursky, 2020; Luo, 2021; Brinkman et al., 2022). In fact, there are a vast number of neural circuits in the brain, governing diverse functions (Hu et al., 2020; Kuner and Kuner, 2021). From a clinical perspective, aberrant neural circuits have been observed to participate in the initiation and progression of a variety of neurological disorders, such as autism spectrum disorder (ASD) and neurodegenerative diseases, through pathological neuronal activities and abnormal axon guidance protein changes (Van Battum et al., 2015; Sudarov et al., 2018; Barth and Ray, 2019; Moussa and Wester, 2022). Taking ASD as an example, an imbalance in the ratio of excitation to inhibition within cortical circuits has been hypothesized as a specific developmental mechanism (Golden et al., 2018; Sohal and Rubenstein, 2019). Therefore, it is reasonable to focus on and further reveal region-specific neuronal diversity, which supports us to better understand the complexity of neural circuits that underlie autistic phenotypes or others.
To this end, quite a lot of methods to study neural circuits have been emerging, such as optogenetics and calcium imaging, nicely linking distinct circuit abnormalities to specific disease dimensions, as shown in the above part of Figure 1. However, they do not take neuronal diversity across different brain regions into account (Bugeon et al., 2022; Lin et al., 2022; Russell et al., 2022). In contrast, recent application of multiple single-cell sequencing techniques, as detailedly compared in Table 1, through identifying various molecular signatures in about 1,000∼1,000,000 individual cells, provides high-resolution genomic information for neurons, with more details in neuronal subpopulation identification (Lange et al., 2020; Cebrian-Silla et al., 2021), although it also meets some technical challenges (Stegle et al., 2015; Stubbington et al., 2017). In terms of the assessment of neural cells, one of the limitations of single-cell sequencing is that some subtypes of neurons, for example cortical layer five pyramidal tract neurons, may not easily survive during the cell isolation process (Tasic et al., 2018). Therefore, most researchers performed their experiments on neurons via single nuclei RNA sequencing (snRNA-Seq) (Cameron et al., 2022; Smajić et al., 2022). On the other hand, recent progress in single-cell RNA sequencing (scRNA-seq) techniques also makes it possible to perform the transcriptional cataloging of neural cells, including neurons and astrocytes, as summarized by several nice review articles (Mu et al., 2019; Yuste et al., 2020; Armand et al., 2021). Of note, the comprehensive characterization of neuronal diversity and the precise identification of neuron-specific transcriptional features via snRNA-seq can extend our current understanding of neural circuits and further predict state modulations of different functional neurons. When combining regular snRNA-seq with spatial transcriptomics, such as the multiplexed error robust fluorescence in situ hybridization (MERFISH), as a notable tool (Moffitt et al., 2016; Burgess, 2019; Eng et al., 2019), it brings great potential to draw more complicated molecular maps. Major technical features of different single-cell sequencing approaches, including applied protocols, the number of detected genes, and sample requirements, etc., have been summarized in Table 1.
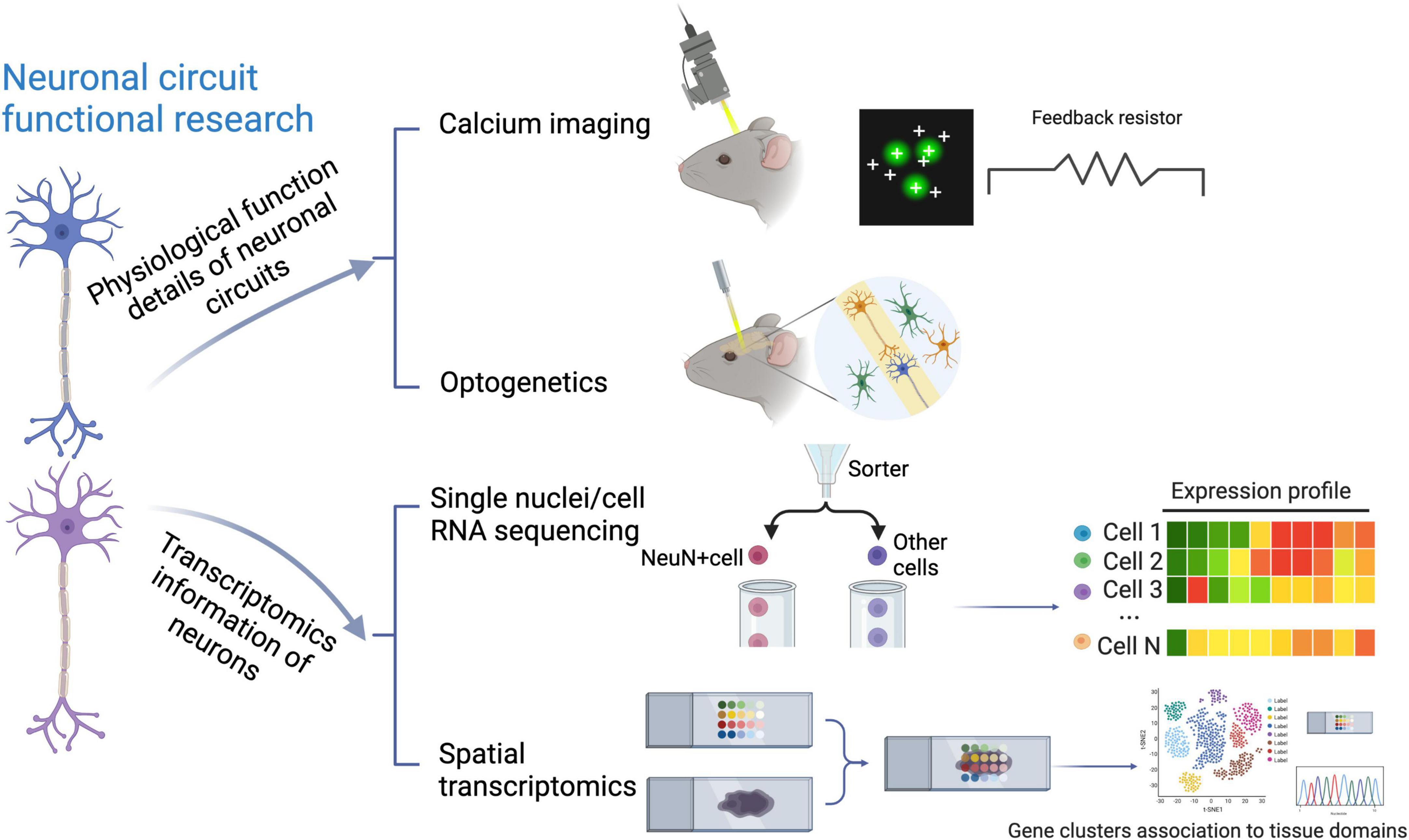
Figure 1. Traditional and transcriptomic techniques to study neural circuits. Depicted are the traditional techniques including optogenetics and calcium imaging, and recently-applied single-cell transcriptomic approaches, which have been used to investigate neural circuits from different aspects.
Taking current progress in understanding neuronal diversity and neural circuit complexity, as well as their potential significance in neurological diseases and psychiatric disorders, into account, we would like to make a brief summary of the latest studies identifying uncharacterized subtypes of neurons and new neural circuits by scRNA-seq in accordance with their specific distributions in the brain. In addition, common scRNA-seq techniques applied in these studies have been reviewed in Table 1. While primarily focusing on the application of scRNA-seq, we keep updates on recent single-cell transcriptomics studies showing intriguing findings about neuronal diversity and region-specific neural circuits among prefrontal cortex, subpallium, hypothalamus, hippocampus, dorsal root ganglion, and brainstem in different species including humans, mice, and birds, which have been listed in Table 2, and vividly visualized in Figure 2, and will be elaborated in the following chapters as well. Of note, some spatially resolved advances demonstrated by spatial transcriptomics will be additionally discussed here, giving new insights into how multi-regional neural circuits are organized in this context.
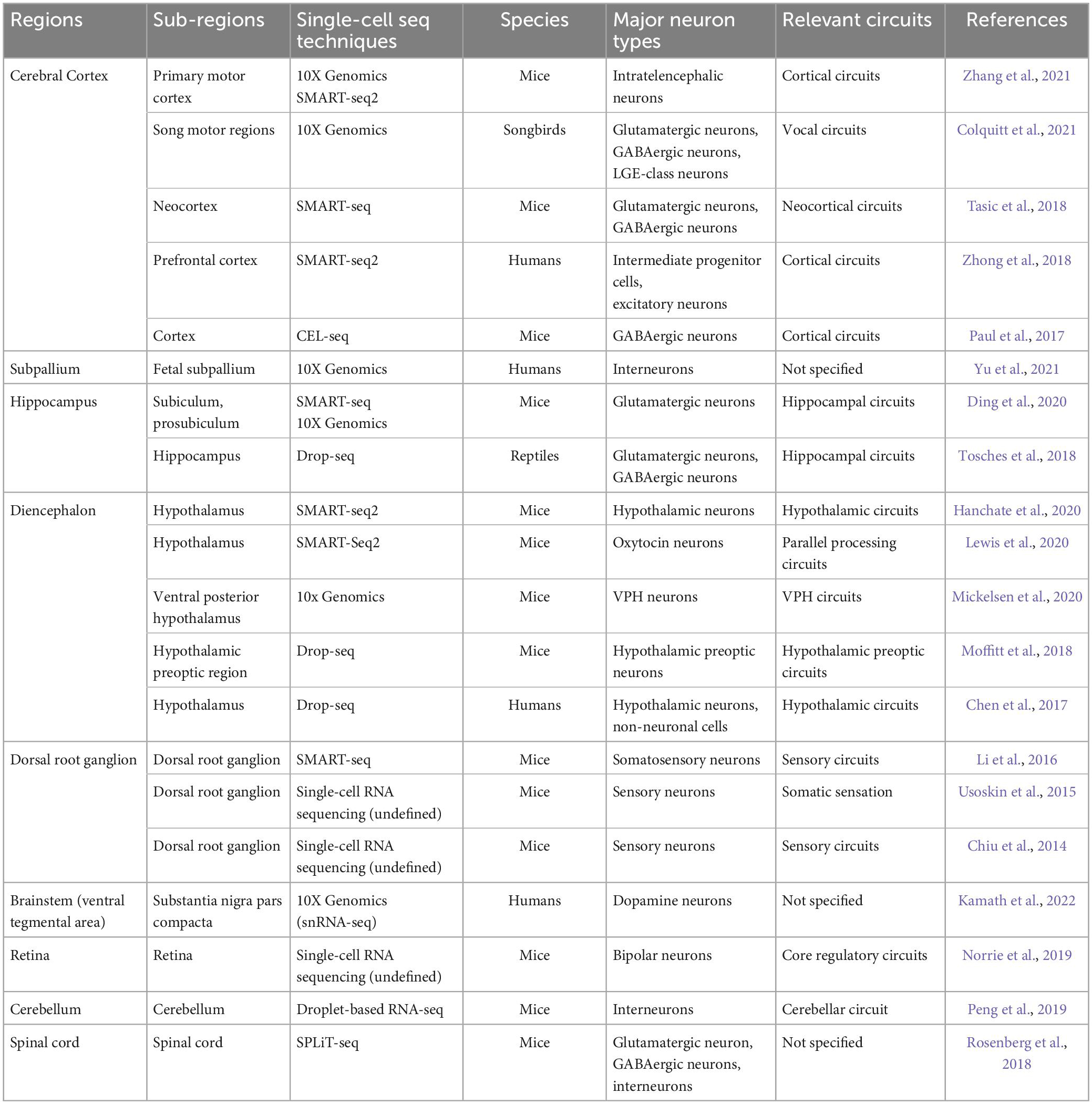
Table 2. Examples investigating neuronal diversity and neural circuit complexity across different regions using single-cell sequencing.
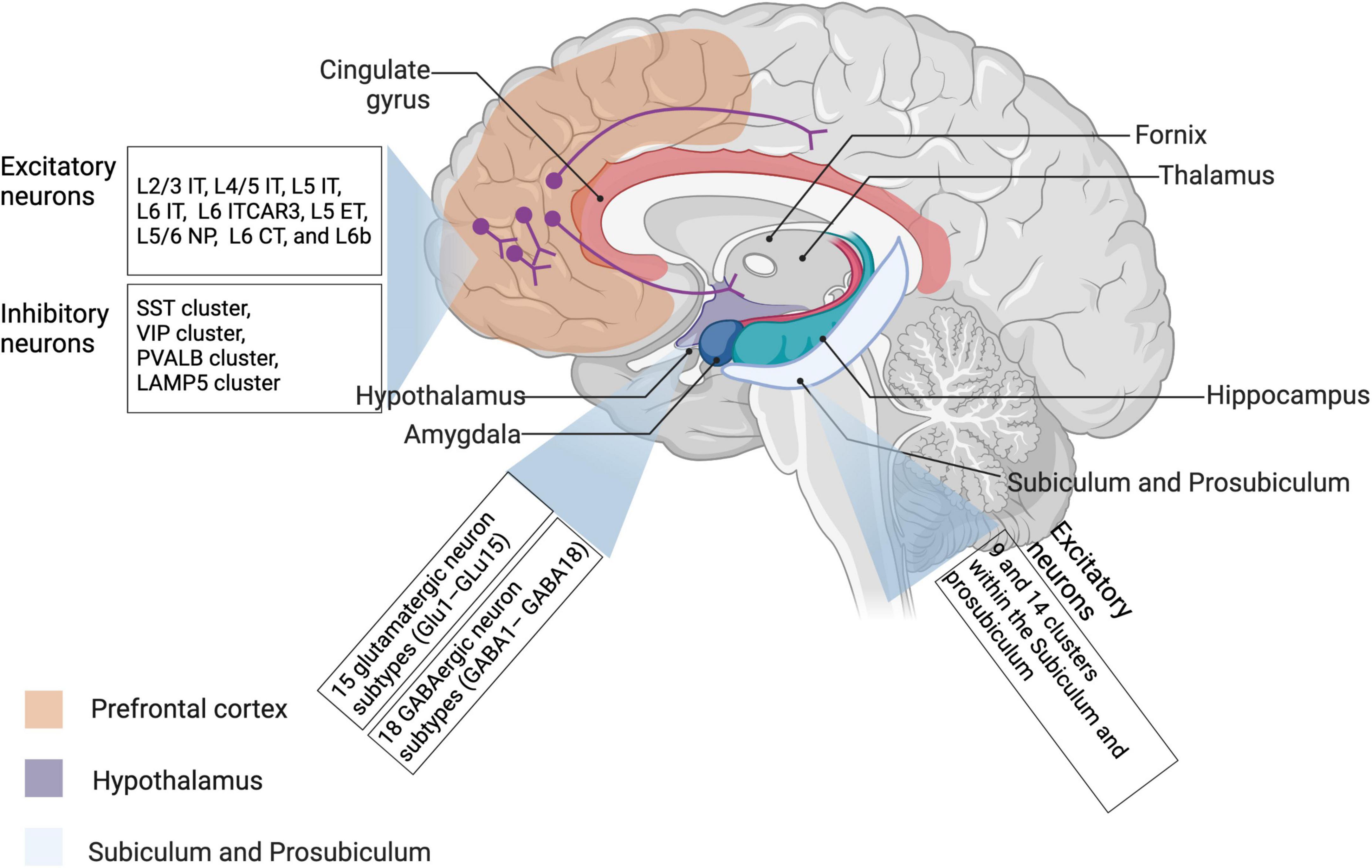
Figure 2. Neuronal diversity across different brain regions revealed by single-cell transcriptomic studies. Most recent literature about neuronal transcriptomes have investigated neuron subpopulations from prefrontal cortex, hippocampus, and hypothalamus. Relevant details have been also described in Table 2. IT, intratelencephalic-projecting neurons; ET, extratelencephalic-projecting neurons; NP, near-projecting neurons; CT, corticothalamic projecting neurons.
Potential value of single-cell sequencing in studying neuronal diversity and neural circuit complexity
Beyond different regions in the brain and among several species, individual neurons generally process information through forming neuronal circuits with their synaptic partners, involving precise synaptic connectivity. Of note, the evolution and development of neuronal circuit architectures have been comprehensively reviewed by Luo (2021) recently, at least partially showing how different architectures of neuronal circuits cooperate in an individual nervous system. In addition, identifying new subpopulations of region-specific neurons and even their molecular signatures is of importance to a deeper understanding of neural circuits underlying abnormal behaviors, as exemplified by the identification of GluLHA neurons in a neural circuit for the inhibition of feeding under persistent pain (Tang et al., 2022). In this review, a wide range of applications of traditional techniques, including optogenetics and calcium imaging versus currently applied single-cell sequencing approaches have been visualized in Figure 1. Interestingly, optogenetics and calcium imaging using two-photon microscopy give some insights into physiological functions of neuronal circuits by detecting the spiking activity of neuronal populations, whereas single-cell sequencing techniques additionally provide the transcriptomic information for neurons at the single-cell resolution (Quirin et al., 2016; Armand et al., 2021; Rodriguez-Romaguera et al., 2022). These three techniques complement each other to elucidate neural circuits (Figure 1). Recently, several comparative studies encompassing different species have suggested that investigating various subtypes of neurons in homologous brain regions via single-cell transcriptomics is a useful first step (Tosches et al., 2018; Hodge et al., 2019; Kebschull et al., 2020). Afterward, with the development of single-cell transcriptomics in the past decade, plenty of original studies revealing region-specific neuron subpopulations and related neural circuits have been emerging, as detailedly summarized in Table 2.
So far, there are more than 20 kinds of established protocols for single-cell sequencing since this technique was first introduced by Tang et al. (2009). Transcriptomic data generated by different protocols display significant heterogeneity (Ziegenhain et al., 2017; Hwang et al., 2018; Mereu et al., 2020). For this, Mereu et al. (2020) have systematically compared thirteen kinds of commonly used protocols of scRNA-seq and snRNA-seq applied to a heterogeneous reference sample resource and discussed their major differences with regards to the RNA capture efficiency, bias, scale and costs, comprehensiveness, and integrability. Of interest, most of the improved versions of plate-based methods, such as Quartz-seq2, CEL-seq2 and Smart-seq2, have been found to generate high-resolution transcriptome profiles. As shown in current studies, Smart-seq/Smart-seq2 and 10X Genomics, as the most representative sequencing protocols, have been extensively applied to reveal genetic gene-expression heterogeneity of neural cells (Hanchate et al., 2020; Maynard et al., 2021; Wang et al., 2021). These protocols also hold true for the identification of diverse neuron subtypes and new neural circuits in humans (Zhong et al., 2018; Yu et al., 2021; Kamath et al., 2022), and mice (Ding et al., 2020; Hanchate et al., 2020; Zhang et al., 2021), details of which have been summarized in Table 2. Additionally, this review also includes a few studies utilizing other sequencing protocols, such as CEL-seq and Drop-seq (Chen et al., 2017; Paul et al., 2017).
It is worth noting that even though sc/snRNA-seq assists in characterizing the transcriptomes of rare types of cells and accurately understanding gene expression regulatory mechanisms, one of the most striking disadvantages drawing our attention is the sacrifice of the spatial context in neural circuits due to the disruption of tissues into isolated cells (Kolodziejczyk et al., 2015; Armand et al., 2021). To tackle this problem, spatial transcriptomics have emerged as a collection of genomic technologies, which can dissect neural circuits with complex anatomical organization, to provide comprehensive anatomical and functional information with spatial localization messages (Lein et al., 2017; Moffitt et al., 2018; Kim et al., 2019). However, analyses of spatial transcriptomic data are computationally challenging (Wang et al., 2018; Shang and Zhou, 2022). Nevertheless, spatially resolved transcriptomics of brain tissue retain the spatial context at the regional, cellular or sub-cellular levels, providing more gene expression information for clustering single-neuron populations. Interestingly, Shah et al. (2016, 2017) have applied sequential fluorescence in situ hybridization (seqFISH) using mouse brain tissues to detect the gene expression in single cells within a large dynamic range, profiling a complex molecular map of hippocampal neurons with high spatial heterogeneity. However, spatial transcriptomics have some technical limitations as well, in comparison with conventional single-cell sequencing methods, as exemplified by the predefinition of candidate genes, the complicated experimental setup, high requirements for computational images, and high cost, and the time-consuming imaging, which all limit their applications, and further ask researchers for more experimental inputs (Rodriques et al., 2019; Armand et al., 2021).
Even so, spatial transcriptomics, as an intriguing and emerging technique, geniusly integrate cellular transcriptomics with their spatial coordinates within tissues, together allowing a deeper understanding of cellular composition, and heterogeneity as well as cell-cell communications (Wolock et al., 2019; Anderson et al., 2022; Fang S. et al., 2022; Shen et al., 2022). On the other hand, neuronal activities in local neural circuits have been thought to be organized for information processing both spatially and temporally, which can be at least partially explained by spatial transcriptomics and other structural analyses (Weisenburger and Vaziri, 2018; Parra-Damas and Saura, 2020; Endo et al., 2021). In addition, given specific cognitive functions as well as distinct computational properties of different brain areas, we would like to discuss these single-cell transcriptomic studies addressing neuronal diversity and neural circuit complexity according to the distribution of neurons in the brain in the following chapters, respectively.
Advances in studying cortical neurons and circuits via single-cell sequencing
Due to the size and the intricate folding, the cerebral cortex takes a predominant place in the brain among species (Fernández et al., 2016; Llinares-Benadero and Borrell, 2019; Bhattacharjee et al., 2021). Thus, the cortex remains the most well-studied brain region through single-cell sequencing so far, attracting a lot of attention from researchers. Lodato et al. (2015) have profiled various molecular signatures of 36 neurons from the cerebral cortex of three healthy individuals by single-cell sequencing and identified thousands of somatic single-nucleotide variants. Furthermore, Heavner et al. (2020) have defined many subclasses of developing projection neurons in the cerebral cortex according to the transcription factor expression, which is in line with single-cell RNA-seq subtypes, as confirmed through multidimensional approaches. In this chapter, involved cortical neurons associated with neural circuit formation and changes mainly include intratelencephalic neurons, glutamatergic neurons, GABAergic neurons, LGE-class neurons, and intermediate progenitor cells (Paul et al., 2017; Tasic et al., 2018; Zhong et al., 2018; Colquitt et al., 2021; Zhang et al., 2021). More recently, Endo and coworkers have comprehensively reviewed the emerging technologies for studying local neural circuits in the cerebral cortex and given new insight into local neural circuits obtained by these technologies, such as single-cell sequencing and tissue clearing, etc. (Endo et al., 2021). In this review, we mainly focus on the application of single-cell sequencing in studying neuronal diversity and neural circuit complexity. For this, relevant details of these original findings, including applied single-cell sequencing techniques, major studied species, and involved brain regions, have been systemically summarized in Table 2, and visualized in Figure 2 as well.
From a conventional hierarchical view of cortical circuits, neurons are regarded as specialized structures in response to specific stimuli, process these signals, and transmit this information to neurons in the following hierarchical order. These cortical neurons collect information that they receive from other circuits and encode a percept (Solari et al., 2019; Brinkman et al., 2022). However, Zhou et al. (2018) have suggested this hierarchical view of visual cortical processing may not apply to the mouse visual cortex, as tested in their study. What single-cell sequencing can add to this view is to extend the understanding of the diversity of neuronal types in the brain and give more molecular details for neuron-neuron communications. To this end, significant diversity in excitatory and inhibitory neurons in the cerebral cortex revealed by single-cell sequencing will be discussed. Glutamatergic neurons, as the most common and widely studied excitatory neurons in cortical circuits, have important molecular signatures and specific physiological properties (Birey et al., 2017; Chapman et al., 2022; Zhong et al., 2022). This importance also holds true for GABAergic neurons in inhibitory circuits and long-range projections (Rock et al., 2017; Kirmse and Zhang, 2022). Several transcriptomic studies have consistently demonstrated that glutamatergic neurons show a greater diversity across brain regions and species in comparison with GABAergic neurons (Tasic et al., 2018; Bakken et al., 2021; Yao et al., 2021), which is in line with single-cell DNA methylation data from Luo et al. (2017).
Through utilizing SMART-seq, Tasic and coworkers have identified some new types of glutamatergic and GABAergic neurons in mouse neocortex, which is responsible for coordination of learned behaviors. Of interest, some markers used for cell type assignment are novel, such as Slc30a3, Osr1, and Fam84b, etc. In their study, glutamatergic neurons display a region-specific pattern, whereas most of GABAergic neurons are ubiquitously located across two neocortical areas, i.e., the primary visual cortex, and the anterior lateral motor cortex. Specifically, glutamatergic neurons from two areas whereas belonging to the same cluster have a median of 78 differentially expressed genes (DEGs), whereas GABAergic types only have a median of two DEGs, as demonstrated by the best-matched tests (Tasic et al., 2018). In a similar vein, Paul et al. (2017) have applied CEL-seq to characterize murine GABAergic subpopulations in mouse cerebral cortex, which are distinguished by their transcriptional architectures. These identified gene families can be divided into 6 functional categories, including transcription factors, cell-adhesion molecules, regulatory components of membrane-proximal signaling pathways, ion channels, neurotransmitter and modulator receptors, and neuropeptides and vesicular release components. In addition, some special features of GABA have been found to associate with distinct spatiotemporal patterns of receptor activation and post-synaptic cell firing that affect circuit computation (Markram et al., 2015). Paul et al. (2017) have also revealed 190 kinds of DEGs, and confirmed some known markers for medial ganglionic eminence- and caudal ganglionic eminence-derived interneurons, which may link the altered gene expression to aberrant cellular and circuit properties. It is worth noting that there exists a huge difference in the number of DEGs between the above-mentioned two studies from Tasic et al. (2018), Paul et al. (2017), which could be due to different protocols of single-cell sequencing applied in their experiments.
Zhong et al. (2018) have revealed molecular signatures of neural progenitor cells, excitatory neurons, and interneurons, etc., through analyzing more than 2,300 single cells in human prefrontal cortex that is associated with advanced cognitive functions, and neurogenesis dynamics during neuronal differentiation (Zhou et al., 2018; Lui et al., 2021). In turn, dysfunction of the prefrontal cortex may lead to cognitive deficits and most of neurodevelopmental disorders (Butt and Lak, 2020; Anderson et al., 2021). As addressed in the above part, given their origin across the dorsal telencephalon, transcriptomic profiles of cortical excitatory neurons may show a more heterogeneous pattern compared with GABAergic neurons (Mayer et al., 2018). Therefore, these transcriptomic findings together outline general characteristics of different neuronal populations that may actively participate in cortical circuits. Interestingly enough, Colquitt et al. (2021) have studied another species except for humans and mice, birds, using 10X Genomics, and demonstrated that glutamatergic vocal neurons of birds are quite similar to neocortical projection neurons of mammals concerning their transcriptional activities. As shown in Table 2, glutamatergic neurons have been especially investigated in mouse hippocampus using 10X Genomics, which will be discussed in the next paragraph (Tasic et al., 2018; Ding et al., 2020). More recently, Zhang and coworkers have applied both 10X Genomics and SMART-seq2, together with MERFISH to establish a spatially resolved cell atlas, with special emphasis on intratelencephalic neurons. In their study, 95 neuronal and non-neuronal cell clusters have been profiled in the murine primary motor cortex, and further a comprehensive spatial map of excitatory as well as inhibitory neuronal clusters has been revealed based on the application of MERFISH (Zhang et al., 2021).
Moreover, single-cell spatial transcriptomics together with retrograde labeling can provide the laminar and regional location of neurons with specific projections. Notably, the integration of MERFISH in their experiment shows a complex network among multiple neuronal clusters beyond brain regions (Xia et al., 2019; Zhang et al., 2020). Even though there are a limited number of spatial transcriptomic studies on neural circuits, MERFISH seems to be able to catch the spatial complexity of neuronal circuits via collecting various forms of spatial data from the same region in the brain. Of note, cell type-specific inference of differential expression (C-SIDE), a novel statistical method, supports to identify cell type-specific differential expression in spatial transcriptomics, providing additional possibilities for mechanistic exploration of diverse circuits (Cable et al., 2022). Furthermore, Fang R. et al. (2022) have utilized MERFISH to reveal differences in somatic interactions as well as conservation in the laminar organization of cells between mice and humans, suggesting potential commonalities and features in neural circuits across species
At last, in addition to neuronal development, single-cell sequencing could evaluate gene expression changes induced by neural activity and plasticity, as exemplified by visual cortical neurons exposed to light (Hrvatin et al., 2018; Wei et al., 2022). In turn, the analysis of activity-regulated genes is also helpful to identify active cells via single-nucleus RNA-seq (Hu et al., 2017). These findings together suggest that single-cell sequencing not only reveals neuron diversity to explore putative functions, but also assesses DEGs to analyze context-specific neurons. It is worthy to mention here that more researchers prefer to use multiple single-cell sequencing techniques to strengthen the credibility of those findings nowadays (Ding et al., 2020; Zhang et al., 2021). In summary, categorizing cortical neurons into specific subtypes by single-cell sequencing techniques, and investigating the roles of different types of neurons in the function of the circuit, is an essential step toward understanding how different cortical circuits produce distinct computations.
Advances in studying hypothalamic neurons and circuits via single-cell sequencing
In addition to the cerebral cortex addressed in the above part, the diencephalon, including the hypothalamus, is the second most well-studied brain region by researchers using single-cell sequencing (Chen et al., 2017; Ding et al., 2020; Hanchate et al., 2020). In general, hypothalamic neurons are highly diverse and participate in a wide range of processes and behaviors which are essential for organismal survival (Sternson, 2013; Li et al., 2018; Fu et al., 2019). On the one hand, both hypothalamus and hippocampus belong to the limbic system, which is in charge of advanced mental functions, such as emotion processing and time perception (Gley et al., 2021). On the other hand, hypothalamus-hippocampus circuitry emerges as an important neural pathway to control various activities, for example, behavioral impulsivity and stress response (Noble et al., 2019). To sum up, studying hypothalamic and/or hippocampal circuits separately or jointly by single-cell sequencing is essential. Therefore, in addition to hippocampal circuits discussed in the following chapter, we mainly summarize some transcriptomic studies about hypothalamic neurons and circuits by single-cell sequencing here, which are also discussed in Table 2.
In the functional sense, hypothalamic circuits are mainly responsible for maintaining homeostatic challenges, as exemplified by immune responses to coronavirus disease 2019 (COVID-19) (Poon, 2020; Mussa et al., 2021). Hanchate et al. (2020) have found that numerous neuropeptides are expressed in upstream neurons isolated from the hypothalamus by using Connect-seq, which can generate a complex molecular map and further allow the molecular and genetic interrogation of how neuronal components control its function in neural circuits. Of interest, 39 neuropeptides are coexpressed with glutamate or GABA, indicating the potential mechanisms for their excitatory and inhibitory effects. In detail, Avp has been observed for PRV+ neurons in four areas of the hypothalamus. By contrast, Tac1 and Npy have been detected in PRV+ neurons in only one hypothalamic area, respectively, i.e., the dorsomedial hypothalamic nucleus (DMH) for Tac1 and the arcuate hypothalamic nucleus (ARC) for Npy. These findings together show the diversity of molecular signatures among different subtypes of hypothalamic neurons (Hanchate et al., 2020). Moreover, Chen et al. (2017) have comprehensively evaluated hypothalamic neuron diversity in mice utilizing Drop-seq and defined 34 novel clusters of neurons with distinct transcriptional signatures. The neuropeptide expression profile across different subtypes of hypothalamic neurons has also been checked, and Crabp1+ neurons in arcuate hypothalamic nucleus (ARH) and Pax6+ neurons in the zona incerta (ZI) have been identified, suggesting that there are still many uncharacterized neuron subpopulations in the hypothalamus (Chen et al., 2017). In addition, the expression pattern of several transcription factors, for example, Foxb1, Npas1, and Lhx8 have displayed a neuron subtype-specific pattern, which is in line with their functions of promoting neuron differentiation as well as identity (Chen et al., 2017).
Besides, some single-cell transcriptomic studies have focused on the specified regions of the hypothalamus, such as the hypothalamic preoptic region and the ventral posterior hypothalamus. To this end, Moffitt et al. (2016) have applied Drop-seq and MERFISH in the hypothalamic preoptic region and identified ∼70 neuronal populations among ∼1 million cells, including 43 subpopulations for inhibitory neurons and 23 subpopulations for excitatory neurons, together outlining a high-resolution framework for mechanistic exploration of behavior circuits in the hypothalamus. Of interest, the combination of MERFISH with measurements of immediate early genes can help to define specific neuron subpopulations activated by specific social behaviors in different physiological states (Moffitt et al., 2018). Recently, Lewis et al. (2020) have particularly targeted oxytocin neurons through SMART-Seq2 in combination with molecular targeting approaches and revealed that some autism risk genes, such as Calb1, Kcnmb4, Reln, and Cnr1, are enriched in parvocellular oxytocin neurons in comparison to magnocellular oxytocin neurons in the context of parallel processing circuits. Mickelsen et al. (2020) have investigated novel neuronal cell types in the ventral posterior hypothalamus and defined 20 neuronal and 18 non-neuronal cell populations by analyzing more than 16,000 single cells, providing a resource for investigating the circuit-level mechanisms.
On the other hand, increasing interest in aging-associated cognitive declines have given rise to the compensation-related utilization of neural circuits hypothesis, drawing more attention to age-related signature changes of hypothalamic neurons in neural circuits (Furusawa and Emoto, 2021; Kang et al., 2022). Hajdarovic et al. (2022a) have performed snRNA-seq of 40,064 hypothalamic nuclei obtained from young and aged female mice, respectively, and revealed an unexpected female-specific feature of hypothalamic aging. Of note, the master regulator of X-inactivation, Xist, has been found to be upregulated with age, especially in hypothalamic neurons, providing some correlative explanations for aging-related hypothalamic changes between neural circuits and behaviors (Hajdarovic et al., 2022a,b).
Advances in studying other region neurons and neural circuits via single-cell sequencing
In the last chapter of this review, we would like to summarize some transcriptomic findings about neuronal diversity and neural circuit complexity in other regions in addition to the cerebral cortex and the hypothalamus, mainly involving hippocampus, subpallium, dorsal root ganglion (DRG), and ventral tegmental area (Li et al., 2016; Yu et al., 2021; Kamath et al., 2022), which are also included in Table 2. Moreover, representative single-cell transcriptomic studies in other central nervous system (CNS) regions, such as, retina, cerebellum, spinal cord, have been briefly summarized in this table as well. Yu et al. (2021) have identified interneuron diversity and complex interneuron lineages in the human subpallium using 10X Genomics. This study highlights the temporal and spatial specification of interneuron subpopulations, which could be linked to neurodevelopmental and psychiatric disorders due to neural circuit dysregulation (Sah et al., 2020; Burket et al., 2021). Importantly, they have selected some representative region-specific markers in the subpallium, for instance, NKX2-1 and LHX6 for the medial ganglionic eminence (MGE), MEIS2 and ZFHX3 for the lateral ganglionic eminence (LGE), and NR2F1 and NR2F2 for the caudal ganglionic eminence (CGE), giving some candidates used for cell classification (Yu et al., 2021).
As for the hippocampus, Ding et al. (2020) have identified the subiculum and the prosubiculum as two important regions of the mouse brain with differential transcriptomic cell types as well as hippocampal circuits. In the meanwhile, they have defined 27 types of transcriptomic cells in both two areas by applying both SMART-seq and 10X Genomics, where two kinds of molecularly and anatomically distinct circuits, i.e., subiculum and prosubiculum circuits, are centered, shedding light into historical findings (Cembrowski et al., 2018; Ding et al., 2020). Interestingly, some genes, such as Col23a1, Id4, Rab3b, Abca8a, Unc5d, Lpl, Gpc3, and Car10, associated with circuit formation, have shown a region-enriched expression pattern in the hippocampus-amygdaloid transition area, as highlighted in Figure 2 (Ding et al., 2020). Tosches et al. (2018) have investigated transcriptomic signatures of glutamatergic and GABAergic neurons in the hippocampus in reptiles, and revealed significant heterogeneity in the ZBTB20+ ETV1+ cells. Recently, novel technologies to characterize new neurons in the adult hippocampus, including single-cell RNA sequencing, intravital imaging, etc., have been generalized by one review article (Denoth-Lippuner and Jessberger, 2021). More importantly, the characterization of hippocampal circuits would better explain the onset of several neurological and psychiatric diseases and further provide some promising therapeutic targets.
Dorsal root ganglion neurons, as an important type of sensory neurons, are involved in the generation, transmission, as well as regulation of different somatosensory signals. In turn, their dysfunction occurs in a variety of neuronal diseases (Nascimento et al., 2018; Meltzer et al., 2021; Balogh et al., 2022). Li et al. (2016) have categorized large and small diameter somatosensory neurons in the mouse DRG. Of special interest, large DRG neurons are classified into four types, and small diameter DRG neurons are categorized into six types, together providing a new classification system for understanding somatosensory neuron subtypes in sensory circuits (Li et al., 2016). Meanwhile, they have revealed 1745 DEGs from 197 neurons for principal component analysis. Single-cell real-time PCR have further verified the differential expression of marker genes in C1 (n = 6), C2 (n = 6), C3 (n = 6), C4 (n = 3), and C5 (n = 6) neurons (Li et al., 2016). At last, Kamath et al. (2022) have profiled 22,048 dopamine neurons in the context of Parkinson’s disease through snRNA-seq analysis, and addressed the importance of neuronal vulnerability in disease-associated degeneration, as exemplified by a representative single subtype with the expression of AGTR1. Usoskin et al. (2015) have revealed eleven subtypes of sensory neurons with markedly different molecular and operational properties using single-cell RNA sequencing. In a similar vein, Chiu et al. (2014) have applied the same procedure to identify three populations and six distinct subgroups from 334 single DRG sensory neurons. These findings together reveal the complexity and diversity of these new subsets of neurons underlying somatosensation.
To sum up, characterization of diverse neuron subpopulations in specific brain regions through single-cell sequencing facilitates the accurate identification of region- and/or disease-specific circuits. The correlation among various functional activities induced by similar or distinct neural circuits beyond regions will be better deciphered. Of special note, to figure out how various cortical circuits are related to distinct functional behaviors, is essential to categorize cortical neurons into subtypes, and study their disparate roles in the context of certain neural circuits. Furthermore, the combinative application of multiple sc/snRNA-seq techniques will validate these preliminary findings and give more robust indications for further mechanistic investigation.
Concluding remarks
To date, single-cell sequencing has been widely applied in identifying neuronal diversity and neural circuit complexity across different brain regions and among various species. Technical advances in sc/snRNA-seq combined with spatial transcriptomics have enabled it to provide comprehensive anatomical and functional information with spatial localization messages and extend our conventional understanding of how region-specific neural circuits work and abnormal neural circuits contribute to neurological disorders. With the identification of new neuron subpopulations in different brain regions and the characterization of these region-specific circuits, enormous information obtained by single-cell sequencing will be translated into organized and systematical knowledge about brain cell ontogeny and function. Moreover, it is worth noting that most current studies have shown cell atlases of normal brain tissues. By contrast, the profile characterization in pathological conditions remains poorly understood, which will be an interesting direction in the future. Overall, understanding nervous system organization beyond the level of individual neurons supports deciphering specific molecular mechanisms of neuropsychiatric diseases.
Author contributions
YX and LL conceived and designed the contents and layout of the mini-review with help from CZ. YX and LL wrote the first draft of the manuscript and CZ edited it. All authors read and approved the final manuscript.
Acknowledgments
The authors acknowledge support from the China Scholarship Council (CSC) fellowship program CSC/LMU. Figures were created with BioRender.com.
Conflict of interest
The authors declare that the research was conducted in the absence of any commercial or financial relationships that could be construed as a potential conflict of interest.
Publisher’s note
All claims expressed in this article are solely those of the authors and do not necessarily represent those of their affiliated organizations, or those of the publisher, the editors and the reviewers. Any product that may be evaluated in this article, or claim that may be made by its manufacturer, is not guaranteed or endorsed by the publisher.
References
Anderson, A. C., Yanai, I., Yates, L. R., Wang, L., Swarbrick, A., Sorger, P., et al. (2022). Spatial transcriptomics. Cancer Cell 40, 895–900. doi: 10.1016/j.ccell.2022.08.021
Anderson, E. M., Engelhardt, A., Demis, S., Porath, E., and Hearing, M. C. (2021). Remifentanil self-administration in mice promotes sex-specific prefrontal cortex dysfunction underlying deficits in cognitive flexibility. Neuropsychopharmacology 46, 1734–1745. doi: 10.1038/s41386-021-01028-z
Armand, E. J., Li, J., Xie, F., Luo, C., and Mukamel, E. A. (2021). Single-cell sequencing of brain cell transcriptomes and epigenomes. Neuron 109, 11–26. doi: 10.1016/j.neuron.2020.12.010
Bakken, T. E., Jorstad, N. L., Hu, Q., Lake, B. B., Tian, W., Kalmbach, B. E., et al. (2021). Comparative cellular analysis of motor cortex in human, marmoset and mouse. Nature 598, 111–119. doi: 10.1038/s41586-021-03465-8
Balogh, M., Zhang, J., Gaffney, C. M., Kalakuntla, N., Nguyen, N. T., Trinh, R. T., et al. (2022). Sensory neuron dysfunction in orthotopic mouse models of colon cancer. J. Neuroinflammation 19:204. doi: 10.1186/s12974-022-02566-z
Barth, A. L., and Ray, A. (2019). Progressive circuit changes during learning and disease. Neuron 104, 37–46. doi: 10.1016/j.neuron.2019.09.032
Beine, Z., Wang, Z., Tsoulfas, P., and Blackmore, M. G. (2022). Single nuclei analyses reveal transcriptional profiles and marker genes for diverse supraspinal populations. J. Neurosci. 42, 8780–8794. doi: 10.1523/JNEUROSCI.1197-22.2022
Bhattacharjee, S., Kashyap, R., Abualait, T., Annabel Chen, S. H., Yoo, W. K., and Bashir, S. (2021). The role of primary motor cortex: More than movement execution. J. Mot. Behav. 53, 258–274. doi: 10.1080/00222895.2020.1738992
Birey, F., Andersen, J., Makinson, C. D., Islam, S., Wei, W., Huber, N., et al. (2017). Assembly of functionally integrated human forebrain spheroids. Nature 545, 54–59. doi: 10.1038/nature22330
Breton-Provencher, V., Drummond, G., Feng, J., Li, Y., and Sur, M. (2022). Spatiotemporal dynamics of noradrenaline during learned behaviour. Nature 606, 732–738. doi: 10.1038/s41586-022-04782-2
Brinkman, B. A., Yan, H., Maffei, A., Park, I. M., Fontanini, A., Wang, J., et al. (2022). Metastable dynamics of neural circuits and networks. Appl. Phys. Rev. 9:011313. doi: 10.1063/5.0062603
Bugeon, S., Duffield, J., Dipoppa, M., Ritoux, A., Prankerd, I., Nicoloutsopoulos, D., et al. (2022). A transcriptomic axis predicts state modulation of cortical interneurons. Nature 607, 330–338. doi: 10.1038/s41586-022-04915-7
Burgess, D. J. (2019). Spatial transcriptomics coming of age. Nat. Rev. Genet. 20, 317–317. doi: 10.1038/s41576-019-0129-z
Burket, J. A., Webb, J. D., and Deutsch, S. I. (2021). Perineuronal nets and metal cation concentrations in the microenvironments of fast-spiking, parvalbumin-expressing GABAergic interneurons: Relevance to neurodevelopment and neurodevelopmental disorders. Biomolecules 11:1235. doi: 10.3390/biom11081235
Butt, S. J. B., and Lak, A. (2020). The spineless origins of prefrontal cortex dysfunction and psychiatric disorders. Neuron 105, 4–6. doi: 10.1016/j.neuron.2019.12.009
Cable, D. M., Murray, E., Shanmugam, V., Zhang, S., Zou, L. S., Diao, M., et al. (2022). Cell type-specific inference of differential expression in spatial transcriptomics. Nat. Methods 19, 1076–1087. doi: 10.1038/s41592-022-01575-3
Cameron, D., Mi, D., Vinh, N. N., Webber, C., Li, M., Marín, O., et al. (2022). Single-nuclei RNA sequencing of 5 regions of the human prenatal brain implicates developing neuron populations in genetic risk for schizophrenia. Biol. Psychiatry. 93, 157–166. doi: 10.1016/j.biopsych.2022.06.033
Cebrian-Silla, A., Nascimento, M. A., Redmond, S. A., Mansky, B., Wu, D., Obernier, K., et al. (2021). Single-cell analysis of the ventricular-subventricular zone reveals signatures of dorsal and ventral adult neurogenesis. Elife 10:e67436. doi: 10.7554/eLife.67436
Cembrowski, M. S., Phillips, M. G., Dilisio, S. F., Shields, B. C., Winnubst, J., Chandrashekar, J., et al. (2018). Dissociable structural and functional hippocampal outputs via distinct subiculum cell classes. Cell 173, 1280–1292. doi: 10.1016/j.cell.2018.03.031
Chapman, C. A., Nuwer, J. L., and Jacob, T. C. (2022). The Yin and Yang of GABAergic and glutamatergic synaptic plasticity: Opposites in balance by crosstalking mechanisms. Front. Synaptic Neurosci. 14:911020. doi: 10.3389/fnsyn.2022.911020
Chen, R., Wu, X., Jiang, L., and Zhang, Y. (2017). Single-cell RNA-seq reveals hypothalamic cell diversity. Cell Rep. 18, 3227–3241. doi: 10.1016/j.celrep.2017.03.004
Chiu, I. M., Barrett, L. B., Williams, E. K., Strochlic, D. E., Lee, S., Weyer, A. D., et al. (2014). Transcriptional profiling at whole population and single cell levels reveals somatosensory neuron molecular diversity. Elife 3:e04660. doi: 10.7554/eLife.04660
Colquitt, B. M., Merullo, D. P., Konopka, G., Roberts, T. F., and Brainard, M. S. (2021). Cellular transcriptomics reveals evolutionary identities of songbird vocal circuits. Science 371:eabd9704. doi: 10.1126/science.abd9704
Denoth-Lippuner, A., and Jessberger, S. (2021). Formation and integration of new neurons in the adult hippocampus. Nat. Rev. Neurosci. 22, 223–236. doi: 10.1038/s41583-021-00433-z
Ding, S. L., Yao, Z., Hirokawa, K. E., Nguyen, T. N., Graybuck, L. T., Fong, O., et al. (2020). Distinct transcriptomic cell types and neural circuits of the subiculum and prosubiculum along the dorsal-ventral axis. Cell Rep. 31:107648. doi: 10.1016/j.celrep.2020.107648
Endo, M., Maruoka, H., and Okabe, S. (2021). Advanced technologies for local neural circuits in the cerebral cortex. Front. Neuroanat. 15:757499. doi: 10.3389/fnana.2021.757499
Eng, C.-H. L., Lawson, M., Zhu, Q., Dries, R., Koulena, N., Takei, Y., et al. (2019). Transcriptome-scale super-resolved imaging in tissues by RNA seqFISH+. Nature 568, 235–239. doi: 10.1038/s41586-019-1049-y
Fang, R., Xia, C., Close, J. L., Zhang, M., He, J., Huang, Z., et al. (2022). Conservation and divergence of cortical cell organization in human and mouse revealed by MERFISH. Science 377, 56–62. doi: 10.1126/science.abm1741
Fang, S., Chen, B., Zhang, Y., Sun, H., Liu, L., Liu, S., et al. (2022). Computational approaches and challenges in spatial transcriptomics. Genom. Proteom. Bioinform. doi: 10.1016/j.gpb.2022.10.001 [Epub ahead of print].
Fernández, V., Llinares-Benadero, C., and Borrell, V. (2016). Cerebral cortex expansion and folding: What have we learned? EMBO J. 35, 1021–1044. doi: 10.15252/embj.201593701
Fu, O., Iwai, Y., Narukawa, M., Ishikawa, A. W., Ishii, K. K., Murata, K., et al. (2019). Hypothalamic neuronal circuits regulating hunger-induced taste modification. Nat. Commun. 10:4560. doi: 10.1038/s41467-019-12478-x
Furusawa, K., and Emoto, K. (2021). Scrap and build for functional neural circuits: Spatiotemporal regulation of dendrite degeneration and regeneration in neural development and disease. Front. Cell Neurosci. 14:613320. doi: 10.3389/fncel.2020.613320
Gley, K., Hadlich, F., Trakooljul, N., Haack, F., Murani, E., Gimsa, U., et al. (2021). Multi-transcript level profiling revealed distinct mRNA, miRNA, and tRNA-derived fragment bio-signatures for coping behavior linked haplotypes in HPA axis and limbic system. Front. Genet. 12:635794. doi: 10.3389/fgene.2021.635794
Golden, C. E., Buxbaum, J. D., and De Rubeis, S. (2018). Disrupted circuits in mouse models of autism spectrum disorder and intellectual disability. Curr. Opin. Neurobiol. 48, 106–112. doi: 10.1016/j.conb.2017.11.006
Hajdarovic, K. H., Yu, D., Hassell, L. A., Evans, S., Packer, S., Neretti, N., et al. (2022a). Single-cell analysis of the aging female mouse hypothalamus. Nat. Aging 2, 662–678. doi: 10.1038/s43587-022-00246-4
Hajdarovic, K. H., Yu, D., and Webb, A. E. (2022b). Understanding the aging hypothalamus, one cell at a time. Trends Neurosci. 45, 942–954. doi: 10.1016/j.tins.2022.10.004
Hanchate, N. K., Lee, E. J., Ellis, A., Kondoh, K., Kuang, D., Basom, R., et al. (2020). Connect-seq to superimpose molecular on anatomical neural circuit maps. Proc. Natl. Acad. Sci. U.S.A. 117, 4375–4384. doi: 10.1073/pnas.1912176117
Heavner, W. E., Ji, S., Notwell, J. H., Dyer, E. S., Tseng, A. M., Birgmeier, J., et al. (2020). Transcription factor expression defines subclasses of developing projection neurons highly similar to single-cell RNA-seq subtypes. Proc. Natl. Acad. Sci. U.S.A. 117, 25074–25084. doi: 10.1073/pnas.2008013117
Hodge, R. D., Bakken, T. E., Miller, J. A., Smith, K. A., Barkan, E. R., Graybuck, L. T., et al. (2019). Conserved cell types with divergent features in human versus mouse cortex. Nature 573, 61–68. doi: 10.1038/s41586-019-1506-7
Hrvatin, S., Hochbaum, D. R., Nagy, M. A., Cicconet, M., Robertson, K., Cheadle, L., et al. (2018). Single-cell analysis of experience-dependent transcriptomic states in the mouse visual cortex. Nat. Neurosci. 21, 120–129. doi: 10.1038/s41593-017-0029-5
Hu, H., Cui, Y., and Yang, Y. (2020). Circuits and functions of the lateral habenula in health and in disease. Nat. Rev. Neurosci. 21, 277–295.
Hu, P., Fabyanic, E., Kwon, D. Y., Tang, S., Zhou, Z., and Wu, H. (2017). Dissecting cell-type composition and activity-dependent transcriptional state in mammalian brains by massively parallel single-nucleus RNA-seq. Mol. Cell 68, 1006–1015.e7. doi: 10.1016/j.molcel.2017.11.017
Hwang, B., Lee, J. H., and Bang, D. (2018). Single-cell RNA sequencing technologies and bioinformatics pipelines. Exp. Mol. Med. 50, 1–14. doi: 10.1038/s12276-018-0071-8
Kamath, T., Abdulraouf, A., Burris, S. J., Langlieb, J., Gazestani, V., Nadaf, N. M., et al. (2022). Single-cell genomic profiling of human dopamine neurons identifies a population that selectively degenerates in Parkinson’s disease. Nat. Neurosci. 25, 588–595. doi: 10.1038/s41593-022-01061-1
Kang, W., Wang, J., and Malvaso, A. (2022). Inhibitory control in aging: The compensation-related utilization of neural circuits hypothesis. Front. Aging Neurosci. 13:771885. doi: 10.3389/fnagi.2021.771885
Kebschull, J. M., Richman, E. B., Ringach, N., Friedmann, D., Albarran, E., Kolluru, S. S., et al. (2020). Cerebellar nuclei evolved by repeatedly duplicating a conserved cell-type set. Science 370:eabd5059. doi: 10.1126/science.abd5059
Kim, D.-W., Yao, Z., Graybuck, L. T., Kim, T. K., Nguyen, T. N., Smith, K. A., et al. (2019). Multimodal analysis of cell types in a hypothalamic node controlling social behavior. Cell 179, 713–728.e17. doi: 10.1016/j.cell.2019.09.020
Kirmse, K., and Zhang, C. (2022). Principles of GABAergic signaling in developing cortical network dynamics. Cell Rep. 38:110568. doi: 10.1016/j.celrep.2022.110568
Kolodziejczyk, A. A., Kim, J. K., Svensson, V., Marioni, J. C., and Teichmann, S. A. (2015). The technology and biology of single-cell RNA sequencing. Mol. Cell 58, 610–620. doi: 10.1016/j.molcel.2015.04.005
Kuner, R., and Kuner, T. (2021). Cellular circuits in the brain and their modulation in acute and chronic pain. Physiol. Rev. 101, 213–258. doi: 10.1152/physrev.00040.2019
Lange, C., Rost, F., Machate, A., Reinhardt, S., Lesche, M., Weber, A., et al. (2020). Single cell sequencing of radial glia progeny reveals the diversity of newborn neurons in the adult zebrafish brain. Development 147:dev185595. doi: 10.1242/dev.185595
Lein, E., Borm, L. E., and Linnarsson, S. (2017). The promise of spatial transcriptomics for neuroscience in the era of molecular cell typing. Science 358, 64–69. doi: 10.1126/science.aan6827
Lewis, E. M., Stein-O’brien, G. L., Patino, A. V., Nardou, R., Grossman, C. D., Brown, M., et al. (2020). Parallel social information processing circuits are differentially impacted in autism. Neuron 108, 659–675.e6. doi: 10.1016/j.neuron.2020.10.002
Li, C. L., Li, K. C., Wu, D., Chen, Y., Luo, H., Zhao, J. R., et al. (2016). Somatosensory neuron types identified by high-coverage single-cell RNA-sequencing and functional heterogeneity. Cell Res. 26, 83–102. doi: 10.1038/cr.2015.149
Li, Y., Zeng, J., Zhang, J., Yue, C., Zhong, W., Liu, Z., et al. (2018). Hypothalamic circuits for predation and evasion. Neuron 97, 911–924.e5. doi: 10.1016/j.neuron.2018.01.005
Lin, S., Du, Y., Xia, Y., Xie, Y., Xiao, L., and Wang, G. (2022). Advances in optogenetic studies of depressive-like behaviors and underlying neural circuit mechanisms. Front. Psychiatry 13:950910. doi: 10.3389/fpsyt.2022.950910
Llinares-Benadero, C., and Borrell, V. (2019). Deconstructing cortical folding: Genetic, cellular and mechanical determinants. Nat. Rev. Neurosci. 20, 161–176. doi: 10.1038/s41583-018-0112-2
Lodato, M. A., Woodworth, M. B., Lee, S., Evrony, G. D., Mehta, B. K., Karger, A., et al. (2015). Somatic mutation in single human neurons tracks developmental and transcriptional history. Science 350, 94–98. doi: 10.1126/science.aab1785
Lui, J. H., Nguyen, N. D., Grutzner, S. M., Darmanis, S., Peixoto, D., Wagner, M. J., et al. (2021). Differential encoding in prefrontal cortex projection neuron classes across cognitive tasks. Cell 184, 489–506.e26. doi: 10.1016/j.cell.2020.11.046
Luo, C., Keown, C. L., Kurihara, L., Zhou, J., He, Y., Li, J., et al. (2017). Single-cell methylomes identify neuronal subtypes and regulatory elements in mammalian cortex. Science 357, 600–604. doi: 10.1126/science.aan3351
Luo, L. (2021). Architectures of neuronal circuits. Science 373:eabg7285. doi: 10.1126/science.abg7285
Markram, H., Muller, E., Ramaswamy, S., Reimann, M. W., Abdellah, M., Sanchez, C. A., et al. (2015). Reconstruction and simulation of neocortical microcircuitry. Cell 163, 456–492. doi: 10.1016/j.cell.2015.09.029
Mayer, C., Hafemeister, C., Bandler, R. C., Machold, R., Batista Brito, R., Jaglin, X., et al. (2018). Developmental diversification of cortical inhibitory interneurons. Nature 555, 457–462. doi: 10.1038/nature25999
Maynard, K. R., Collado-Torres, L., Weber, L. M., Uytingco, C., Barry, B. K., Williams, S. R., et al. (2021). Transcriptome-scale spatial gene expression in the human dorsolateral prefrontal cortex. Nat. Neurosci. 24, 425–436. doi: 10.1038/s41593-020-00787-0
Meltzer, S., Santiago, C., Sharma, N., and Ginty, D. D. (2021). The cellular and molecular basis of somatosensory neuron development. Neuron 109, 3736–3757. doi: 10.1016/j.neuron.2021.09.004
Mereu, E., Lafzi, A., Moutinho, C., Ziegenhain, C., Mccarthy, D. J., Álvarez-Varela, A., et al. (2020). Benchmarking single-cell RNA-sequencing protocols for cell atlas projects. Nat. Biotechnol. 38, 747–755. doi: 10.1038/s41587-020-0469-4
Mickelsen, L. E., Flynn, W. F., Springer, K., Wilson, L., Beltrami, E. J., Bolisetty, M., et al. (2020). Cellular taxonomy and spatial organization of the murine ventral posterior hypothalamus. Elife 9:e58901. doi: 10.7554/eLife.58901
Moffitt, J. R., Bambah-Mukku, D., Eichhorn, S. W., Vaughn, E., Shekhar, K., Perez, J. D., et al. (2018). Molecular, spatial, and functional single-cell profiling of the hypothalamic preoptic region. Science 362:eaau5324. doi: 10.1126/science.aau5324
Moffitt, J. R., Hao, J., Wang, G., Chen, K. H., Babcock, H. P., and Zhuang, X. (2016). High-throughput single-cell gene-expression profiling with multiplexed error-robust fluorescence in situ hybridization. Proc. Natl. Acad. Sci. U.S.A. 113, 11046–11051. doi: 10.1073/pnas.1612826113
Moussa, A. J., and Wester, J. C. (2022). Cell-type specific transcriptomic signatures of neocortical circuit organization and their relevance to autism. Front. Neural Circuits 16:982721. doi: 10.3389/fncir.2022.982721
Mu, Q., Chen, Y., and Wang, J. (2019). Deciphering brain complexity using single-cell sequencing. Genom. Proteom. Bioinform. 17, 344–366. doi: 10.1016/j.gpb.2018.07.007
Mussa, B. M., Srivastava, A., and Verberne, A. J. M. (2021). COVID-19 and neurological impairment: Hypothalamic circuits and beyond. Viruses 13:498. doi: 10.3390/v13030498
Nascimento, A. I., Mar, F. M., and Sousa, M. M. (2018). The intriguing nature of dorsal root ganglion neurons: Linking structure with polarity and function. Prog. Neurobiol. 168, 86–103. doi: 10.1016/j.pneurobio.2018.05.002
Noble, E. E., Wang, Z., Liu, C. M., Davis, E. A., Suarez, A. N., Stein, L. M., et al. (2019). Hypothalamus-hippocampus circuitry regulates impulsivity via melanin-concentrating hormone. Nat. Commun. 10:4923. doi: 10.1038/s41467-019-12895-y
Norrie, J. L., Lupo, M. S., Xu, B., Al Diri, I., Valentine, M., Putnam, D., et al. (2019). Nucleome dynamics during retinal development. Neuron 104, 512–528.e11. doi: 10.1016/j.neuron.2019.08.002
Parra-Damas, A., and Saura, C. A. (2020). Tissue clearing and expansion methods for imaging brain pathology in neurodegeneration: From circuits to synapses and beyond. Front. Neurosci. 14:914. doi: 10.3389/fnins.2020.00914
Paul, A., Crow, M., Raudales, R., He, M., Gillis, J., and Huang, Z. J. (2017). Transcriptional architecture of synaptic communication delineates GABAergic neuron identity. Cell 171, 522–539.e20. doi: 10.1016/j.cell.2017.08.032
Peng, H., Xie, P., Liu, L., Kuang, X., Wang, Y., Qu, L., et al. (2021). Morphological diversity of single neurons in molecularly defined cell types. Nature 598, 174–181. doi: 10.1038/s41586-021-03941-1
Peng, J., Sheng, A. L., Xiao, Q., Shen, L., Ju, X. C., Zhang, M., et al. (2019). Single-cell transcriptomes reveal molecular specializations of neuronal cell types in the developing cerebellum. J. Mol. Cell Biol. 11, 636–648. doi: 10.1093/jmcb/mjy089
Poon, K. (2020). Behavioral feeding circuit: Dietary fat-induced effects of inflammatory mediators in the hypothalamus. Front. Endocrinol. 11:591559. doi: 10.3389/fendo.2020.591559
Quirin, S., Vladimirov, N., Yang, C. T., Peterka, D. S., Yuste, R., and Ahrens, M. B. (2016). Calcium imaging of neural circuits with extended depth-of-field light-sheet microscopy. Opt. Lett. 41, 855–858. doi: 10.1364/OL.41.000855
Rock, C., Zurita, H., Lebby, S., Wilson, C. J., and Apicella, A. J. (2017). Cortical circuits of callosal GABAergic neurons. Cereb. Cortex 28, 1154–1167. doi: 10.1093/cercor/bhx025
Rodriguez-Romaguera, J., Namboodiri, V. M. K., Basiri, M. L., Stamatakis, A. M., and Stuber, G. D. (2022). Developments from bulk optogenetics to single-cell strategies to dissect the neural circuits that underlie aberrant motivational states. Cold Spring Harb. Perspect. Med. 12:a039792. doi: 10.1101/cshperspect.a039792
Rodriques, S. G., Stickels, R. R., Goeva, A., Martin, C. A., Murray, E., Vanderburg, C. R., et al. (2019). Slide-seq: A scalable technology for measuring genome-wide expression at high spatial resolution. Science 363, 1463–1467. doi: 10.1126/science.aaw1219
Rosenberg, A. B., Roco, C. M., Muscat, R. A., Kuchina, A., Sample, P., Yao, Z., et al. (2018). Single-cell profiling of the developing mouse brain and spinal cord with split-pool barcoding. Science 360, 176–182. doi: 10.1126/science.aam8999
Russell, L. E., Dalgleish, H. W. P., Nutbrown, R., Gauld, O. M., Herrmann, D., Fişek, M., et al. (2022). All-optical interrogation of neural circuits in behaving mice. Nat. Protoc. 17, 1579–1620. doi: 10.1038/s41596-022-00691-w
Sah, M., Shore, A. N., Petri, S., Kanber, A., Yang, M., Weston, M. C., et al. (2020). Altered excitatory transmission onto hippocampal interneurons in the IQSEC2 mouse model of X-linked neurodevelopmental disease. Neurobiol. Dis. 137:104758. doi: 10.1016/j.nbd.2020.104758
Sanes, J. R., and Zipursky, S. L. (2020). Synaptic specificity, recognition molecules, and assembly of neural circuits. Cell 181, 536–556. doi: 10.1016/j.cell.2020.04.008
Shah, S., Lubeck, E., Zhou, W., and Cai, L. (2016). In situ transcription profiling of single cells reveals spatial organization of cells in the mouse hippocampus. Neuron 92, 342–357. doi: 10.1016/j.neuron.2016.10.001
Shah, S., Lubeck, E., Zhou, W., and Cai, L. (2017). seqFISH accurately detects transcripts in single cells and reveals robust spatial organization in the hippocampus. Neuron 94, 752–758.e1. doi: 10.1016/j.neuron.2017.05.008
Shang, L., and Zhou, X. (2022). Spatially aware dimension reduction for spatial transcriptomics. Nat. Commun. 13:7203. doi: 10.1038/s41467-022-34879-1
Shen, X., Zhao, Y., Wang, Z., and Shi, Q. (2022). Recent advances in high-throughput single-cell transcriptomics and spatial transcriptomics. Lab Chip 22, 4774–4791. doi: 10.1039/d2lc00633b
Smajić, S., Prada-Medina, C. A., Landoulsi, Z., Ghelfi, J., Delcambre, S., Dietrich, C., et al. (2022). Single-cell sequencing of human midbrain reveals glial activation and a Parkinson-specific neuronal state. Brain 145, 964–978. doi: 10.1093/brain/awab446
Sohal, V. S., and Rubenstein, J. L. R. (2019). Excitation-inhibition balance as a framework for investigating mechanisms in neuropsychiatric disorders. Mol. Psychiatry 24, 1248–1257. doi: 10.1038/s41380-019-0426-0
Solari, F., Caramenti, M., Chessa, M., Pretto, P., Bülthoff, H. H., and Bresciani, J. P. (2019). A biologically-inspired model to predict perceived visual speed as a function of the stimulated portion of the visual field. Front. Neural Circuits 13:68. doi: 10.3389/fncir.2019.00068
Stegle, O., Teichmann, S. A., and Marioni, J. C. (2015). Computational and analytical challenges in single-cell transcriptomics. Nat. Rev. Genet. 16, 133–145. doi: 10.1038/nrg3833
Sternson, S. M. (2013). Hypothalamic survival circuits: Blueprints for purposive behaviors. Neuron 77, 810–824. doi: 10.1016/j.neuron.2013.02.018
Stubbington, M. J., Rozenblatt-Rosen, O., Regev, A., and Teichmann, S. A. (2017). Single-cell transcriptomics to explore the immune system in health and disease. Science 358, 58–63. doi: 10.1126/science.aan6828
Sudarov, A., Zhang, X.-J., Braunstein, L., Locastro, E., Singh, S., Taniguchi, Y., et al. (2018). Mature hippocampal neurons require LIS1 for synaptic integrity: Implications for cognition. Biol. Psychiatry 83, 518–529. doi: 10.1016/j.biopsych.2017.09.011
Tang, F., Barbacioru, C., Wang, Y., Nordman, E., Lee, C., Xu, N., et al. (2009). mRNA-Seq whole-transcriptome analysis of a single cell. Nat. Methods 6, 377–382. doi: 10.1038/nmeth.1315
Tang, H. D., Dong, W. Y., Hu, R., Huang, J. Y., Huang, Z. H., Xiong, W., et al. (2022). A neural circuit for the suppression of feeding under persistent pain. Nat. Metab. 4, 1746–1755. doi: 10.1038/s42255-022-00688-5
Tasic, B., Yao, Z., Graybuck, L. T., Smith, K. A., Nguyen, T. N., Bertagnolli, D., et al. (2018). Shared and distinct transcriptomic cell types across neocortical areas. Nature 563, 72–78. doi: 10.1038/s41586-018-0654-5
Tosches, M. A., Yamawaki, T. M., Naumann, R. K., Jacobi, A. A., Tushev, G., and Laurent, G. (2018). Evolution of pallium, hippocampus, and cortical cell types revealed by single-cell transcriptomics in reptiles. Science 360, 881–888. doi: 10.1126/science.aar4237
Usoskin, D., Furlan, A., Islam, S., Abdo, H., Lönnerberg, P., Lou, D., et al. (2015). Unbiased classification of sensory neuron types by large-scale single-cell RNA sequencing. Nat. Neurosci. 18, 145–153. doi: 10.1038/nn.3881
Van Battum, E. Y., Brignani, S., and Pasterkamp, R. J. (2015). Axon guidance proteins in neurological disorders. Lancet Neurol. 14, 532–546. doi: 10.1016/S1474-4422(14)70257-1
Wang, X., Allen, W. E., Wright, M. A., Sylwestrak, E. L., Samusik, N., Vesuna, S., et al. (2018). Three-dimensional intact-tissue sequencing of single-cell transcriptional states. Science 361:eaat5691. doi: 10.1126/science.aat5691
Wang, X., He, Y., Zhang, Q., Ren, X., and Zhang, Z. (2021). Direct comparative analyses of 10X genomics chromium and smart-seq2. Genom. Proteom. Bioinform. 19, 253–266. doi: 10.1016/j.gpb.2020.02.005
Wei, J. R., Hao, Z. Z., Xu, C., Huang, M., Tang, L., Xu, N., et al. (2022). Identification of visual cortex cell types and species differences using single-cell RNA sequencing. Nat. Commun. 13:6902. doi: 10.1038/s41467-022-34590-1
Weisenburger, S., and Vaziri, A. (2018). A guide to emerging technologies for large-scale and whole-brain optical imaging of neuronal activity. Annu. Rev. Neurosci. 41, 431–452. doi: 10.1146/annurev-neuro-072116-031458
Wolock, S. L., Lopez, R., and Klein, A. M. (2019). Scrublet: Computational identification of cell doublets in single-cell transcriptomic data. Cell Syst. 8, 281–291.e9. doi: 10.1016/j.cels.2018.11.005
Xia, C., Fan, J., Emanuel, G., Hao, J., and Zhuang, X. (2019). Spatial transcriptome profiling by MERFISH reveals subcellular RNA compartmentalization and cell cycle-dependent gene expression. Proc. Natl. Acad. Sci. U.S.A. 116, 19490–19499. doi: 10.1073/pnas.1912459116
Yao, Z., Van Velthoven, C. T., Nguyen, T. N., Goldy, J., Sedeno-Cortes, A. E., Baftizadeh, F., et al. (2021). A taxonomy of transcriptomic cell types across the isocortex and hippocampal formation. Cell 184, 3222–3241.e26. doi: 10.1016/j.cell.2021.04.021
Yu, Y., Zeng, Z., Xie, D., Chen, R., Sha, Y., Huang, S., et al. (2021). Interneuron origin and molecular diversity in the human fetal brain. Nat. Neurosci. 24, 1745–1756. doi: 10.1038/s41593-021-00940-3
Yuste, R., Hawrylycz, M., Aalling, N., Aguilar-Valles, A., Arendt, D., Armañanzas, R., et al. (2020). A community-based transcriptomics classification and nomenclature of neocortical cell types. Nat. Neurosci. 23, 1456–1468. doi: 10.1038/s41593-020-0685-8
Zeng, H. (2022). What is a cell type and how to define it? Cell 185, 2739–2755. doi: 10.1016/j.cell.2022.06.031
Zhang, M., Eichhorn, S. W., Zingg, B., Yao, Z., Cotter, K., Zeng, H., et al. (2021). Spatially resolved cell atlas of the mouse primary motor cortex by MERFISH. Nature 598, 137–143. doi: 10.1038/s41586-021-03705-x
Zhang, M., Eichhorn, S. W., Zingg, B., Yao, Z., Zeng, H., Dong, H., et al. (2020). Molecular, spatial and projection diversity of neurons in primary motor cortex revealed by in situ single-cell transcriptomics. Biorxiv doi: 10.1101/2020.06.04.105700
Zhong, P., Cao, Q., and Yan, Z. (2022). Selective impairment of circuits between prefrontal cortex glutamatergic neurons and basal forebrain cholinergic neurons in a tauopathy mouse model. Cereb. Cortex 32, 5569–5579. doi: 10.1093/cercor/bhac036
Zhong, S., Zhang, S., Fan, X., Wu, Q., Yan, L., Dong, J., et al. (2018). A single-cell RNA-seq survey of the developmental landscape of the human prefrontal cortex. Nature 555, 524–528. doi: 10.1038/nature25980
Zhou, N., Masterson, S. P., Damron, J. K., Guido, W., and Bickford, M. E. (2018). The mouse pulvinar nucleus links the lateral extrastriate cortex, striatum, and amygdala. J. Neurosci. 38, 347–362. doi: 10.1523/JNEUROSCI.1279-17.2017
Keywords: single-cell sequencing, neurons, neural circuits, cortex, hypothalamus, hippocampus, synaptic connection, neuroscience
Citation: Xing Y, Zan C and Liu L (2023) Recent advances in understanding neuronal diversity and neural circuit complexity across different brain regions using single-cell sequencing. Front. Neural Circuits 17:1007755. doi: 10.3389/fncir.2023.1007755
Received: 30 July 2022; Accepted: 16 February 2023;
Published: 30 March 2023.
Edited by:
Masahito Yamagata, Harvard University, United StatesReviewed by:
Hui Yu, University of Michigan, United StatesKa-Chun Wong, City University of Hong Kong, Hong Kong SAR, China
Copyright © 2023 Xing, Zan and Liu. This is an open-access article distributed under the terms of the Creative Commons Attribution License (CC BY). The use, distribution or reproduction in other forums is permitted, provided the original author(s) and the copyright owner(s) are credited and that the original publication in this journal is cited, in accordance with accepted academic practice. No use, distribution or reproduction is permitted which does not comply with these terms.
*Correspondence: Lu Liu, TGF1cmFsaXVsdTE5OTFAZ21haWwuY29t, THUuTGl1QG1lZC51bmktbXVlbmNoZW4uZGU=