Erratum: fMRI studies evaluating central respiratory control in humans
- 1Department of Clinical Neurosciences, Lausanne University Hospital and University of Lausanne, Lausanne, Switzerland
- 2Lyon Neuroscience Research Center, Institut National de la Santé et de la Recherche Médicale U1028/CNRS UMR 5292 Lyon 1 University, Bron, France
- 3IDEE Epilepsy Institute, Lyon, France
- 4Department of Functional Neurology and Epileptology, Hospices Civils de Lyon, Lyon, France
A plethora of neural centers in the central nervous system control the fundamental respiratory pattern. This control is ensured by neurons that act as pacemakers, modulating activity through chemical control driven by changes in the O2/CO2 balance. Most of the respiratory neural centers are located in the brainstem, but difficult to localize on magnetic resonance imaging (MRI) due to their small size, lack of visually-detectable borders with neighboring areas, and significant physiological noise hampering detection of its activity with functional MRI (fMRI). Yet, several approaches make it possible to study the normal response to different abnormal stimuli or conditions such as CO2 inhalation, induced hypercapnia, volitional apnea, induced hypoxia etc. This review provides a comprehensive overview of the majority of available studies on central respiratory control in humans.
Introduction
Regulating breathing is a response to alterations in blood levels of oxygen (O2) and carbon dioxide (CO2). Traditionally, this regulation process was attributed primarily on respiratory control centers located in the brainstem, particularly in the medulla and the pons. However, more recently, suprapontine structures such as the limbic areas, the diencephalon, the striatum and the cortex were ascribed to be essential in modulation of the respiratory drive of the brainstem (Horn and Waldrop, 1998; Pattinson et al., 2009b; Feldman et al., 2013). The basic pattern of respiration is generated in the medulla, and primarily regulated by pontine centers. These areas constantly regulate respiration, so that oxygen, carbon dioxide and acid levels are kept within normal limits. It is possible for someone to deliberately breathe faster or slower or to hold their breath, and it is also possible to not breathe at all for a period of time. This active control is regulated by the cerebral cortex, the amygdala and the hypothalamus, which participate in normal or exaggerated respiratory control, such as in stressful conditions (Horn and Waldrop, 1998). There are many brain regions sensitive to hypoxia and hypercapnia, and so the overriding of the will not to breathe comes from many regions (Guz, 1997). Both voluntary and automatic respiratory control systems are primarily integrated within the brainstem. However, in animal models, electrical stimulation of the cortex produces respiratory responses directly through the dorsal cord and the respiratory motor neurons and indirectly via the corticobulbar pathways (Shea, 1996). Brainstem reflex respiratory response is inhibited if cortical inputs are altered, as shown in decorticated animals (Tenney and Ou, 1977), in patients with bilateral infarction (Heyman et al., 1958) or in opioid administration (Pattinson et al., 2009a).
Several acute and chronic neurological conditions are associated with altered breathing patterns. This is caused by changes that occur in central respiratory control centers located in the brainstem or in the forebrain (Nogues and Benarroch, 2008). In general, these changes are less severe in chronic diseases, like multiple sclerosis, compared to acute diseases like stroke (Nogues et al., 2002). They can also occur intermittently, such as during or after an epileptic seizure, where they might lead to sudden unexpected death in epilepsy (SUDEP).
Central nervous control
Medulla
Respiratory centers in the medulla are divided into the dorsal respiratory group (DRG) and the ventral respiratory column (VRC). The DRG represents the ventro-lateral portion of the nucleus tractus solitarius (NTS) and is mainly an inspiratory group (Alheid and Mccrimmon, 2008). It is described as a center of integration for afferents from peripheral chemoreceptors via the glossopharyngeal and vagus nerves (Alheid et al., 2011). The DRG sends constant bursts to respiratory motor neurons (Lalley, 1986). The VRC is a bilateral column formed by neurons in the lateral tegmentum and extending from the caudal part of the facial nucleus to the spino-medullary junction (Alheid and Mccrimmon, 2008; Figure 1). The caudal half of the VRC, termed the ventral respiratory group (VRG), contains bulbospinal respiratory premotoneurons that receive converging inputs from VRC rhythm generating neurons and from neurons outside the VRC, sculpting the activity pattern distributed to various pools of respiratory motoneurons (Alheid and Mccrimmon, 2008). The VRG is subdivided into the rostral (rVRG) and caudal (cVRG) group, based on the peak concentrations of inspiratory (rVRG) vs. expiratory (cVRG) bulbospinal neurons (Smith et al., 2013). The VRG is described as primarily expiratory, but it also contains inspiratory neurons. It consists of four groups of neurons, which generate a breathing rhythm through communication with each other: (1) the Bötzinger complex (BC); (2) caudal VRG jointly control the voluntary forced exhalation by sending input to intercostal and abdominal muscles. This is opposing: (3) the Prebötzinger complex (PBC); and (4) the rostral VRG that jointly acts to increase the force of inspiration (Ikeda et al., 2017). The PBC and BC are believed to be the central pattern generators of respiration (Smith et al., 2009).
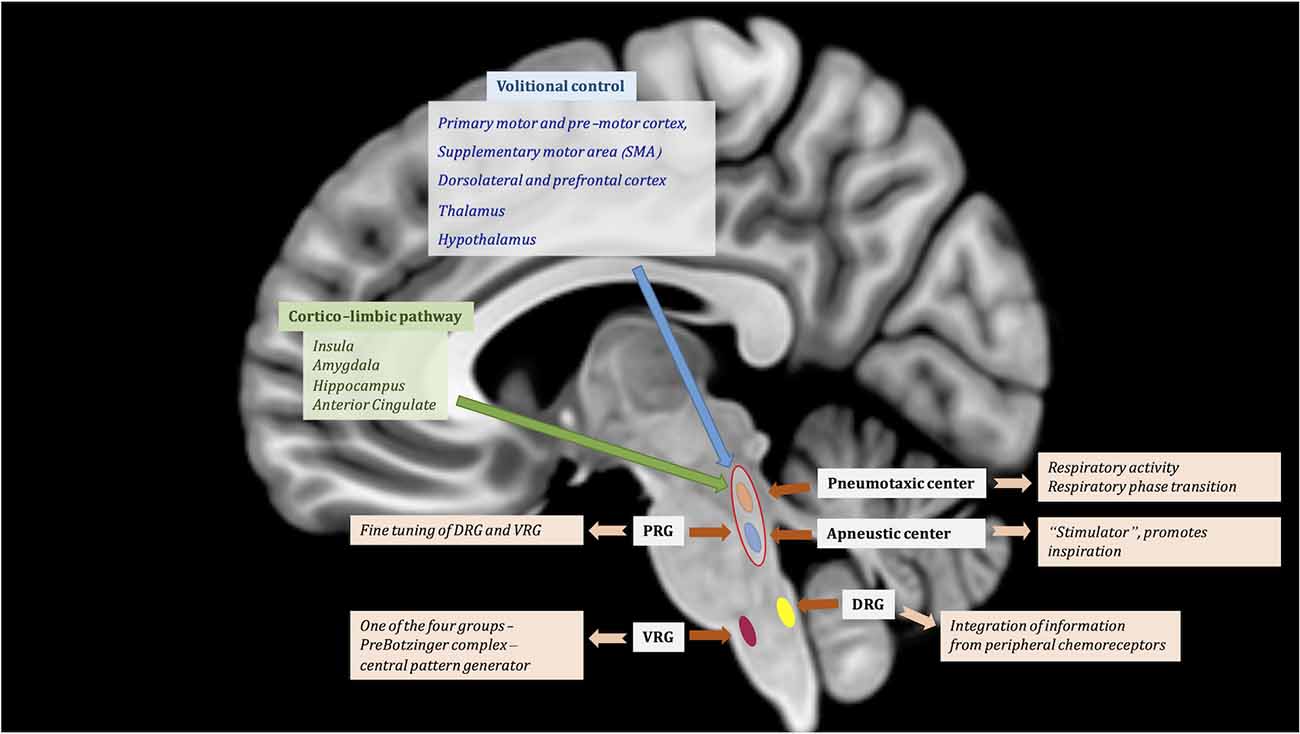
Figure 1. Central regulation of respiratory function. Suprapontine modulation of breathing through volitional control (blue) and corticolimbic pathway (green). Automatic regulation is assured by the brainstem centers contributing to the respiratory drive: (1) Pontine respiratory group (PRG) is represented by the pneumotaxic center and the apneustic center; and (2) Medulla—respiratory centers comprised of the dorsal respiratory group (DRG) and ventral respiratory group (VRG), the latter is part of the ventral respiratory column. The VRG is composed of four groups of neurons: (1) the Bötzinger complex; (2) caudal VRG; (3) the Prebötzinger complex; and (4) the rostral VRG. MNI T1 in sagittal projection was used for illustration.
Pons
The pons has two significant centers implicated in breathing regulation, both being part of the pontine respiratory group (PRG): (1) the pneumotaxic center; and (2) the apneustic center (Figure 1). The pneumotaxic center, located bilaterally in the dorsal rostral pons, is composed of the Kölliker-Fuse (KöF) and the parabrabrachial nuclei (PB) complex (Ikeda et al., 2017) and is involved in inspiratory off-switch. The KoF/PB complex is responsible for regulation of respiratory activity and respiratory phase transition (Ikeda et al., 2017). The apneustic center is located in the lower pons (Kahn and Wang, 1967). The PRG exerts “fine-tuning” influences over the medullary respiratory centers to help produce normal smooth inspirations and expirations (Douglas et al., 2004; Figure 1). Both centers communicate in order to control the rate and depth of breathing. The apneustic part is a “stimulator” and promotes inspiration by sending inputs to neurons in the DRG and VRG controlling inspiration. Although neurons involved with respiration are aggregated in certain parts of the brainstem, neurons that are active during inspiration are intermingled with those active during expiration (Smith et al., 2013).
Chemical control
Of vital importance in the control of respiration are chemoreceptors. These receptors respond to the CO2 level in circulating blood, but the gas acts indirectly. CO2 is capable of diffusing through the capillary blood-brain barrier. In the blood, dissolved CO2 is neutralized by the bicarbonate-carbon dioxide buffer system and carbonic acid is formed, leading to the production of hydrogen and bicarbonate ions, and allowing the body to maintain a physiological pH (Alheid and Mccrimmon, 2008). When CO2 is elevated, the concentration of hydrogen ions in the blood increases, lowering pH and resulting in acidosis. The central chemoreceptors are stimulated and respond to this pH change. The rise in blood CO2 level, known as hypercapnia, thus triggers ventilation. The chemoreceptors that regulate respiration are located centrally near the medullary respiratory centers and peripherally in the arteries. Central chemoreceptors in the brainstem are continuously regulating breathing through monitoring of pH, the partial pressure of carbon dioxide (PCO2) and oxygen (pO2) in the blood. This regulation is insured by afferents from peripheral chemoreceptors located in the carotid body that are primarily targeting the solitary tractus (Lahiri et al., 1978). The partial pressure of oxygen (PO2) will stimulate respiration when it reaches severe hypoxemic levels (Javaheri and Kazemi, 1987). The medullar structures playing the role of central chemosensors responding to changes in pH and PaCO2 in arterial blood or cerebral spinal fluid (CSF) are: (1) the raphe nuclei; (2) the arcuate nucleus; (3) the retrotrapezoid nucleus (RTN); and (4) the parafacial respiratory group (pFRG; Smith et al., 2013). The raphe nuclei are partly formed of serotoninergic neurons that participate in cardiorespiratory regulation (Morris et al., 2010). The arcuate nucleus is a group of neurons involved in the breathing rate control and is located bilaterally on both sides of the midline in the medulla (Mikhail and Ahmed, 1975). The pFRG and RTN, the most rostral structures of the ventral medulla, are respiratory-modulators and are also regulated by hypercapnia (Smith et al., 2013).
Peripheral chemoreceptors that regulate breathing are found in structures known as the carotid and aortic bodies. These bodies contain sensory neurons which react primarily to a reduction in oxygen supply. They are generally not involved in regulating respiration, since they do not act until O2 drops to a very low level (Memmler et al., 1992). Recent studies have shown that astrocytes are also involved in chemosensing regulation in the brainstem. Low PO2 stimulates astrocytes in the brainstem, and they promote a general respiratory response to hypoxia to deliver adequate oxygen to arterial blood (Angelova et al., 2015; Sheikhbahaei et al., 2018). Since there is usually a sufficient supply of O2 in the blood, CO2 has the most immediate effect on regulating breathing in the central chemoreceptors. As the level of CO2 increases, increased respiration is required to remove excess gas. Chemoreceptors play an important role in developing the uncomfortable sensation of dyspnea, mostly due to direct connection to forebrain regions particularly in the limbic system, and they stimulate the respiratory system due to hypoxia/hypercapnia (Buchanan and Richerson, 2009).
Cerebral and limbic system control
While it is universally accepted that the brainstem drives the autonomic respiratory pattern, cortical modulation of central respiratory rhythm and the conscious perception of breathing are still poorly understood (Evans, 2010). Through the cerebral cortex, it is possible to consciously or unconsciously increase or decrease the rate and depth of the respiratory movements. The extra-pontine and extra-bulbar respiratory centers are involved in volitional control, autonomic control, and cortico-limbic control (Figure 1).
The cortico-limbic pathway involved in modulation of respiratory control is very similar to the one active during strong emotional or affective states (Ledoux, 2000; Nagai et al., 2010; Feinstein et al., 2022). It is mostly represented by the insula and associated operculum (its anterior agranular part mostly), the head of the hippocampus, the amygdala, and the anterior cingulate cortex (ACC; Evans, 2010). Imaging studies have showed involvement of the amygdala in experimentally induced anxiety (Feinstein et al., 2022), of limbic structures in sleep disorders (Harper et al., 2014), and of the insula in obstructive sleep apnea (OSA; Li et al., 2015).
The autonomic regulation is mainly controlled by the brainstem, and it was mainly covered above, but inputs and outputs are generated by central structures acting in the control of cardio-respiratory functions, including the insula, temporal lobe, central operculum, and periaqueductal gray matter (Benarroch, 1993; Linnman et al., 2012). The fastigial nucleus of the cerebellum also contains CO2/H+ intrinsic chemoreceptors and plays an essential role in compensating for extreme changes in blood pressure and modulating hypercapnia-induced respiratory response via monosynaptic projections to the medullary gigantocellular nuclei (Martino et al., 2007). The cerebellum is also thought to participate mainly in the expiration phase of breathing (Prasad et al., 2021).
The volitional respiratory control is mediated by the primary motor and pre-motor cortex, the supplementary motor area (SMA), the dorsolateral and prefrontal cortex for decision making and motor planning, and by thalamic nuclei (ventro-posterolateral, ventrolateral, anterior, pulvinar), as well as the hypothalamus. Various studies highlight the essential role of the dorsomedial hypothalamus in respiratory regulation as a result of different types of stressful situations (for review see Dampney et al., 2008). The caudal hypothalamus is also responsible for integration of respiratory output in relation to changes in homeostasis (Horn and Waldrop, 1998).
Afferents from pontine and medullary respiratory centers, cortical, limbic and other suprapontine structures descend along the anterolateral column of the spinal cord to the phrenic, intercostal and abdominal muscle motor neurons and generate respiratory movements.
Functional MRI of The Brainstem in Relation to Respiration
The original fMRI studies of respiration were designed to detect central respiratory control in humans, but suffered from very limited sample size, lack of statistical power, and difficult interpretation of clusters observed around the midline (Gozal et al., 1995; Harper et al., 1998; Evans et al., 1999; Šmejkal et al., 2000). Furthermore, there was no correction for the various artifacts (motion artifacts caused by breathing, cardiac movement, cerebral spinal fluid pulsation) that impact BOLD signals generated in the brainstem or suprapontine structures (Dagli et al., 1999).
In recent years, the quality of MR imaging of the midline structures has improved significantly through the development of high field and ultra-high field MRI. However, imaging of the human brainstem remains challenging. Very few structures or nuclei can be reliably identified on a structural MRI, even on a high-field MRI scanner, let alone on a 1.5 or 3T scan. This becomes even more challenging for fMRI studies, since the BOLD signal is far more difficult to detect in the brainstem than in cortical areas due to a much lower signal-to-noise ratio (Beissner et al., 2014). However, there are solutions to tackle these issues, such as reducing the size of the acquisition field of view, which will consequently increase the spatial resolution and lead to a smaller voxel size, or correcting images for physiological movements.
Spontaneous fluctuations of BOLD signal during fMRI acquisitions are influenced by a multitude of physiological variables, including cardiac rhythm (Piche et al., 2009), respiratory movements (Hu et al., 1995; Kruger and Glover, 2001; Birn et al., 2006), head movements and changes in CO2 (Iacovella and Hasson, 2011). The presence of major arteries and CSF in the vicinity of the brainstem adds to this physiological noise and further decreases the signal-to-noise ratio in fMRI studies of respiratory centers (Beissner et al., 2014; Beissner, 2015).
It is customary for fMRI studies dedicated to central respiratory control research to record all above-mentioned physiological signals to correct for their impact on BOLD signal. This requires using a pneumatic belt, end-tidal gas monitoring and head positioning foam pads during fMRI acquisition (Chang and Glover, 2009). De-noising of data from physiologically occurring signals can then be applied (Glover et al., 2000; Birn et al., 2006, 2008b; Chang and Glover, 2009). However, one needs to keep in mind that the removal of these signals, and in particular those related to respiratory movements, might partly hide the specific activation of respiratory centers elicited by the fMRI experiment (Iacovella and Hasson, 2011). Physiological noise can also be removed by computing both low frequency [physiological oscillations (~0.01–0.15 Hz)] and high-frequency (driven by cardiac rhythm and normal breathing) physiological regressors (Windischberger et al., 2002; Birn et al., 2008a, b; Chang and Glover, 2009; Chang et al., 2009; Yuan et al., 2013; Cordes et al., 2014). Removal of these components from the fMRI series can induce significant changes and allow for a more reliable interpretation of the BOLD signal (Chang et al., 2009; Tong et al., 2019). This is especially valid for the reduction of susceptibility artifacts occurring along the vertebrobasilar arterial system and neighboring brainstem (Dagli et al., 1999). There are also other susceptibility artifacts that can induce changes in the magnetic field, such as the oscillatory chest movement, diaphragm shifting, and changes in the inhaled/exhaled gas. These artifacts will generate respiration-induced B0 fluctuations and produce shifts in the phase of the MR image (Van De Moortele et al., 2002). A potential solution is parallel imaging, which increases the contrast to noise of echo-planar image (EPI) data by acquiring multiecho EPI (Poser et al., 2006). Multiecho EPI samples the data at multiple repeated short echo times (TE). This type of acquisition with optimized echo weighting can reduce susceptibility-induced distortion and dropout artifacts in EPI images, while improving BOLD contrast sensitivity (Poser et al., 2006). The technique, in combination with Independent Component Analysis (ICA), has been shown to be effective in significantly reducing susceptibility artifacts in the brainstem (Kundu et al., 2012; Beissner and Baudrexel, 2014).
While total removal of these artifacts is improbable, their reduction is feasible either during the acquisition or in the pre-processing stage, by applying different denoising algorithms (Caballero-Gaudes and Reynolds, 2017). Yet, the intrinsic temporal relationship between the activation of the brainstem respiratory centers and respiration related movements makes such denoising a risk of masking the BOLD signal of interest. In addition, one needs to consider the degrees of freedom lost in this process. One of the most commonly used method in the field is RETROICOR, which is based on the Fourier transformation of cardiac and breathing rhythmic activities to correct for their related movement artifacts (Glover et al., 2000). A modified RETROICOR method has been developed to avoid overfitting the noise from physiological signals (Harvey et al., 2008; Jones et al., 2008; Wallace et al., 2017). Other approaches use ICA, CompCor toolbox from the CONN toolbox, or masked ICA (mICA) with a brainstem mask, to perform a signal decomposition directly from the acquired images into various components, including physiological artifacts such as breathing or heartbeat (Beissner et al., 2014; Moher Alsady et al., 2016; Jarrahi, 2021). Overall, modified RETROICOR and mICA are thought to enable removal of respiration-induced movements without masking the activity of brainstem respiratory centers (Beissner et al., 2014).
Reducing physiological noise in the brainstem is necessary for studies investigating its functions, such as control of breathing (Dunckley et al., 2005; Harvey et al., 2008). Denoising is particularly important in distinguishing between the true response of respiratory centers and the spill-over effect from neighboring vessels (Khalili-Mahani et al., 2013). Only few structures within the brainstem can be reliably identified on the traditional MRI (Matt et al., 2019). Parcellation techniques, widely used for segmenting MRIs, usually have one single label for the brainstem, or in some atlases, the brainstem is segmented into midbrain, pons, and medulla. Comparison of different preprocessing techniques for the brainstem described five methods that were sensitive to brainstem activation, including use of various parameters for normalization and smoothing in several commonly used software packages (Beissner et al., 2011). The small size of brainstem respiratory centers represents a significant issue in fMRI studies of respiratory control. Indeed, classical gradient echo images have a low spatial resolution, 3 mm3 or lower (Weibull et al., 2008). This issue can be addressed by reducing the acquisition field of view by focusing on the brainstem (Pattinson et al., 2009b), or by using ultra-high field MR scanners which have provided promising results in the identification of brainstem nuclei. In addition, smoothing enables improving the high signal-to-noise ratio (SNR) of brainstem fMRI (Worsley and Friston, 1995; Beissner, 2015). Also, recent studies indicate that due to the particularly small size of nuclei involved in autonomic control, a small smoothing kernel of 6 mm for smoothing the data during preprocessing is advisable (Mckay et al., 2003, 2008; Evans et al., 2009; Hess et al., 2013). Yet, this results in blurring the distinction of closely spaced brainstem nuclei.
Correction models for respiratory rate and cardiac rhythm, along with direct measurements of CO2 have also been applied successfully to quantify cerebrovascular reactivity (CVR) in the cerebrum (Golestani et al., 2015; Moreton et al., 2016; Prokopiou et al., 2019; Golestani and Chen, 2020). CVR is defined as the percentage signal change in CBF per mmHg change in arterial partial pressure of CO2 (PaCO2; Poublanc et al., 2015). It is often referred to as the ability of cerebral blood vessels to undergo diastolic contraction under the influence of hypercapnic challenges (Liu et al., 2019). CVR is also accompanied by mild hypoxia (Tancredi and Hoge, 2013; Chan et al., 2020). CVR is a marker of vascular reserve, and is complementary to basal cerebral hemodynamic measurements such as cerebral blood flow (CBF) and cerebral blood volume (CBV; Liu et al., 2019). Hypercapnia induced by CO2 gas blend administration in patients with various neurological diseases effectively triggers CVR, enabling to evaluate their perfusion reserve (Spano et al., 2013). Cerebral regions that exhibit less or little BOLD CVR are usually affected in conditions such as gliomas, trauma, amyloid deposition, occlusion of major arteries etc. (Vernieri et al., 1999; Pindzola et al., 2001; Hsu et al., 2004; Ziyeh et al., 2005; Mandell et al., 2008). The CVR response to breathing challenges is different from the one induced by CO2 inhalation, suggesting that CVR measurements in pathologies affecting the respiratory system may be inaccurate (Ogoh et al., 2019). One important issue in fMRI studies of respiratory centers is to distinguish BOLD signal changes due to CVR from those reflecting activation of brain respiratory response. In task fMRI the increase in the cerebral metabolic rate of O2 and CBV is associated with increased local neuronal activity, which results in local decrease in the concentration of deoxyhemoglobin, which decreases the tissue-blood susceptibility differential. As a result there is a decrease in spin dephasing, and consequential BOLD signal increase (Bandettini et al., 1992). This process makes it feasible to measure the task related response from the brain areas involved in the processing of the task. For the CVR measurement, use of vasodilatory stimulus such as breath holding (BH) or exogenous CO2 gas will induce only slight changes to cerebral metabolic rate of O2 but a very robust global increased BOLD signal, primarily reflecting the augmentation of CBF (due to vasodilatation effect of the CO2). The distinction between these two is the local and global response to the task and the ways to measure the signal. The CVR BOLD quantifies the overall signal variance, the ratio between changes in the BOLD signal and end-tidal CO2 change, whereas the BOLD response of the respiratory centers measures the local changes in BOLD intensity, which has a typical temporal profile known as the hemodynamic response function (HRF).
Removal of global signal changes is also fairly common (Macey P. M. et al., 2004). For the correction of CO2 fluctuations on the fMRI signal, the HRF CO2 can be convolved with end-tidal CO2 data, and the output regressed out of the BOLD signal (Prokopiou et al., 2019). Use of end-tidal CO2 measurements can also be useful when the user requires creation of the estimated CO2 arrival time at each brain region and quantification of the hemodynamic response following elevation of CO2 (Yao et al., 2021).
Using fMRI, there are several ways to obtain a response from the respiratory centers of the brain. This can be done either by: (i) inducing hypercapnia (through inhaling high concentration of CO2 or through imposing prolonged breath holding); (ii) inducing hypoxia (through reducing the concentration of inhaled O2); or (iii) through voluntary modulations of breathing (i.e., hyperpnea, breath-holding, slowing of respiratory pace). In particular, compliance with the breath-holding task is critical, given the role of prolonged volitional control in triggering an effective BOLD response from the respiratory centers. Breath-holding experiments can be either timed, using a fixed BH duration, or maintained for as long as participants can feel comfortable (Thomason et al., 2005; Mckay et al., 2008). Other methods to activate brainstem respiratory centers include hypercapnia and hypoxia challenges. Hypercapnia can be induced by administering a mixed gas with high concentration of CO2 (5%) and 95% O2, delivered for 2 min (Harper et al., 2005). For the hypoxia challenges, several paradigms have been used, including breathing a mixed concentration of 15% O2 and 85% N2 for 2 min (Macey et al., 2005), or undergoing five hypoxic episodes of breathing a mixed gas with 10% O2 and 90% N2 for 180 s followed by 90 s of normoxia (Gerlach et al., 2021). Because task-related changes in arterial gases, and notably pCO2, can be affected by manipulation of breathing, some respiratory imaging studies have used mechanical ventilation as a passive condition and volitional control of respiration as an active condition, which allowed observing BOLD signal activations, unaffected by manipulation of pCO2 (Ramsay et al., 1993; Evans et al., 1999).
Some of these studies are reviewed below.
Hypercapnia
Increased levels of pCO2 and reduced pH trigger a response from the raphe pallidus, pFRG/RTN, and the NTS (Okada et al., 2008). Stimulation of these medullary chemoreceptors through an increase in pCO2 leads to an excitatory response from the respiratory neuronal network, and to a hypercapnic ventilatory response (in vivo and in vitro study; Gourine et al., 2005; Fukushi et al., 2021). Usually, elevation of pCO2 induces dyspnea or breathlessness in healthy subjects (Chonan et al., 1987; Burki and Lee, 2010) and this is used as a stimulus in investigations directed toward studying central respiratory control in dyspnea or air hunger (behavioral and pharmacological studies). The regional fMRI signal responses to hypercapnia, using 5% CO2-95% O2 for 120 s mixture, showed a pronounced increased response in regions not classically associated with breathing control, but traditionally related to affect, autonomic regulation, or motor coordination (Harper et al., 2005; i.e., the thalamus, dorsal striatum, insula, hippocampus, cingulate cortex, amygdala and hypothalamus; Brannan et al., 2001; Liotti et al., 2001; Von Leupoldt and Dahme, 2005). Limbic structures are also involved in breathing regulation such as air hunger (Banzett et al., 2000; Brannan et al., 2001; Liotti et al., 2001), urge-to-cough (Mazzone et al., 2007), or respiratory challenges such as induced hypercapnia by inhaling 5% CO2 (Harper et al., 2005) or inspiratory breath holding (Macefield et al., 2006) and forced expiratory loading (Macey K. E. et al., 2004). Limbic activations are more notable in highly anxious subjects compared to low anxious participants when subjected to hypercapnia (Chan et al., 2019). The cerebellar and more rostral (midbrain, pons) involvement in mediating hypercapnia was also reported (Gozal et al., 1994; Harper et al., 1998, 2005; Kastrup et al., 1999b; Brannan et al., 2001; Parsons et al., 2001).
Hypercapnia-based studies are typically confronted by questions on how to obtain the response—either by volitional manipulation—end-expiratory or end-inspiratory BH, hyperventilation or by CO2 gas mixture (which requires special equipment for the gas delivery, calibration, MR-safety measurements of all equipment etc). The advantage of such delivery is the control of inhaled and exhaled gas (Moreton et al., 2016), whereas the main constraint is the requirement of special equipment. Voluntary manipulations of breathing rely fully on subjects’ compliance, which also can be viewed as a constraint. The advantage is that it can be easily implemented by means of finding a suitable stimulus onset/offset software and deciding upon which type of breathing manipulation to use in the experimental setting. Studies cited above have studied CVR (Okada et al., 2008) or central response to hypercapnia (Harper et al., 2005), voluntary expiration (Macey K. E. et al., 2004), inspiratory occlusion (Chan et al., 2019), Valsava maneuver (Harper et al., 1998), end-expiratory BH (Kastrup et al., 1999b). Few studies were conducted using CO2 gas mixture (Gozal et al., 1994; Harper et al., 1998, 2005; Brannan et al., 2001; Liotti et al., 2001; Parsons et al., 2001). Some reference articles were using a PET technique (Brannan et al., 2001; Liotti et al., 2001; Parsons et al., 2001). Regarding the preprocessing of the data, some authors used data motion correction and global signal change removal (Macey K. E. et al., 2004; Harper et al., 2005), while some opted for no correction (Harper et al., 1998; Kastrup et al., 1999b; Chan et al., 2019), or only the motion correction (Gozal et al., 1994).
Hypoxia
Hypoxia, reduction in pO2, is detected by the carotid chemoreceptors, which trigger a response from the brainstem respiratory centers that leads to hyperventilation, for review please see Gourine and Funk (2017). Another review points to peripheral chemoreceptors, that also trigger forebrain response, descending through the hypothalamus to the DRG and VRG (Fukushi et al., 2021). Indeed, the hypothalamus is one of the key regions driving the central respiratory response to hypoxia (Horn and Waldrop, 1998). Hypoxia is an effective way to experimentally trigger dyspnea, and can be easily reversed with inhalation of O2 (Fukushi et al., 2021). In an fMRI study, hypoxia was induced by repeated inhalation of 10% oxygen and 90% nitrogen, and contrasted with normoxia (Gerlach et al., 2021). Five distinct hypoxia-responsive regions were detected around the NTS (the nucleus ambiguous, intermediate reticular nucleus, dorsal motor nucleus of the vagal nerve, spinal trigeminal nucleus, and the inferior olivary nucleus; Gerlach et al., 2021), as well as three hypothalamic regions (the arcuate nucleus, anterior hypothalamic area/lateral hypothalamic area, and paraventricular nucleus; Gerlach et al., 2021). However, they did not monitor the end-tidal pCO2 during their experiment, nor did they apply a physiological correction to the BOLD series, raising the possibility that the central chemoreflex response could have been merged with the activations detected during their experiment. They also employed mICA focusing on the lower brainstem and the hypothalamus. As for the hypercapnia induced by CO2 inhalation, the advantage of inducing the hypoxia with a mixture of 10% O2 and 90% N2 is that it allows full control over the inhaled gas. The issue is that this method of gas delivery requires special MR safe equipment.
Voluntary Modulation
Forebrain regions, such as the primary motor cortex, premotor area and supplementary motor area, are activated by volitional breathing (Brannan et al., 2001; Liotti et al., 2001; Von Leupoldt and Dahme, 2005). Voluntary modulation of breathing in 20 healthy volunteers who breathed at a slower pace than usual (i.e., 5.5 breaths per minute, similar to yoga practice, compared to 10 breaths per minute) resulted in increased BOLD activation pattern within the brainstem, across the dorsal length of the pons, in hypothalamic and thalamic regions, within cerebellar vermis and lateral cortices and in the striatum, the hippocampus and the motor, supplementary motor and parietal cortices (Critchley et al., 2015). These areas are part of an executive homeostatic network (Zaccaro et al., 2018). The observed activations hint to the link between the control of breathing and the baroreflex sensitivity. Hypoxic challenge (breathing 13% of O2) in the same group of subjects led to activation within the dorsal pons, bilateral amygdala, thalamus and cerebellar cortices, along with activation of occipital, medial and dorsolateral prefrontal regions, an activation pattern typically observed in stressful conditions (Critchley et al., 2015). In the modeling of fMRI data, they added end-tidal CO2, arterial oxygen saturation SaO2, respiratory rate, tidal and minute volume (ventilation), heart rate and standard deviation of inter-beat interval. Another modulation of breathing, which consists of 6 s breath (3 s breathe in and 3 s breathe out) or 12 s breath (6 s breathe in and 6 s breathe out), provided comparable end-tidal CO2 values and patterns of BOLD response compared to those resulting from inhalation of CO2 (Liu et al., 2020). Authors accounted for variation of end-tidal CO2 in their data preprocessing. An early fMRI study performed in five healthy men assessing volitional inspiratory control (voluntary hyperpnea ensured by a ventilator with creation of large tidal inspiratory volumes compared to passive expirations) showed activation within the superior motor cortex, premotor cortex and supplementary motor area (Evans et al., 1999). They used a 15 mm smoothing kernel, and no correction for any susceptibility artifacts was applied, but also no activation in the brainstem was reported. In another study where healthy participants executed voluntary hyperpnea (about three times faster as the normal breathing), the same cortical areas, along with medullar activation were detected (Mckay et al., 2003). They accounted for global signal changes in the volumes, and used a much smaller smoothing kernel—6 mm. Another paradigm consisted in contrasting unconscious to conscious breathing, by asking healthy volunteers to focus their attention (or not) on each inspiratory and expiratory movements. This resulted in modulation of the activation pattern in the premotor and parietal cortex (Šmejkal et al., 1999, 2000). Authors did not provide any information about data preprocessing.
The advantage of using voluntary respiration modulation relies on: (1) very little equipment required to perform the task; and (2) the facility to train subjects to perform the experiment. The drawback of this approach is: (1) little control over compliance; and (2) variability in subjects’ ability to hold long breath holds.
Resting State fMRI
Resting state fMRI enables study of the synchronous spontaneous fluctuations between various cortical regions (Biswal et al., 1995). Several resting state networks have been described, however most studies focus on the default brain mode (DMN; Greicius et al., 2003). In the DMN, consistent regions of the brain are active at rest but reduce their activity when cognitive tasks are carried out. Abnormal resting state connectivity has been observed in various diseases, including conditions where respiratory and cardiovascular regulation is impaired. Confounds, such as bulk motion, cardiac-related motion, white matter fluctuations, respiratory-related motion, and variations in end-tidal CO2 account for 46% of signal variance in resting state fMRI data and must be considered when investigating low frequency variations of the BOLD signal (Harita and Stroman, 2017). Modulation of respiration also proved to influence resting state fMRI, with enhanced connections when pCO2 is increased (Mcketton et al., 2021). Authors corrected for the end-tidal PCO2 and for head motion, and used CompCor toolbox to extract physiologically related noise in the data. The same correction was used in a recent study, which reported that voluntary normal breathing through the mouth compared to nose breathing also resulted in increased functional connectivity (FC) throughout the DMN nodes (Jung et al., 2020). The authors linked this finding to the potential cognitive disturbances observed in subjects suffering from mouth breathing syndrome (Jung et al., 2020). Another study showed synchronized neural activity through a distributed network of limbic/paralimbic and brainstem regions during uninterrupted spontaneous respiration (Evans et al., 2009). Authors used a 6 mm kernel for smoothing, and used global regressors for correcting for variation of BOLD globally and the PCO2 regressor.
Breath-Holding fMRI
Breath holding (BH) is commonly used to simulate the effect of apnea and hypercapnia on the brain, resulting in autonomic down-regulation of heart rate, vasodilatation and simultaneous reduction in blood flow to the brain (Kastrup et al., 1998; Corfield et al., 2001). Breath-holding results not only in rapid increase in pCO2 but also in reduction of PO2 in the first 20–30 s (Dubois, 1952; Hong et al., 1971), and the cumulative amount of CO2 for longer BHs (30–40 s) is lower than when the BHs last 20 s or less (Lindholm and Linnarsson, 2002). Hyperventilation before BHs will reduce the CO2 and increase the reserve of O2, and will diminish the urge to breath. While the PO2 levels after very long BHs will descend to 20 mmHg, the levels of CO2 will stay normal or slightly decreased (Lindholm and Lundgren, 2006). The breaking point of BH happens when the sum of lower PO2 and higher CO2 is sufficient to induce alveolar ventilation eight times higher the normal (Otis et al., 1948). The increase in PCO2 will stimulate the central respiratory rhythm, which keeps its rhythmicity throughout the BH and cannot be controlled voluntarily (Parkes, 2006). The breathing patterns correlate with fluctuations in PO2 and PCO2 during BH, which are vasoactive triggers that modify global cerebral perfusion (Kastrup et al., 1999c). While the majority of fMRI studies cited in this review had reported PCO2 or end-tidal CO2 variations measured in their studies, only very few also focused on PO2 variations (Gozal et al., 1995; Chan et al., 2020). That is probably due to the common concept that an increase in PCO2 will imminently decrease the PO2, as they work synergistically in the normoxic condition to stimulate peripheral chemoreceptors (Lahiri et al., 1978). Comparison of studies with and without measurement of PO2, but with measurement of PCO2 showed that variation of PCO2 during BH and after BH are very similar (Otis et al., 1948; Gozal et al., 1995; Chan et al., 2020). Studies in which PO2 was measured (Gozal et al., 1995; Chan et al., 2020) indicated that hypoxia and hypercapnia have synergistic effect, and reproduce results observed in animal studies (Honda et al., 1963; Lahiri et al., 1978; Chan et al., 2020).
Compared to inhalation of CO2, where special equipment is needed, BH is safe, easy to use, suitable for various age groups, and offers fairly robust results (Godfrey and Campbell, 1968; Strohl and Altose, 1984; Li et al., 1999; Liu et al., 2002; Parkes, 2006; Mckay et al., 2008; Magon et al., 2009; Roberts et al., 2009; Bright and Murphy, 2013; Sutterlin et al., 2013; Tancredi and Hoge, 2013; Iranmahboob et al., 2016). Strong cortical, subcortical and medullary activations are usually observed during BH (data corrected for global intensity and smoothed with 6 mm kernel; Mckay et al., 2008) and the signal increase occurs with no apparent change in mean arterial pressure, but no information about data correction was reported (Kannurpatti et al., 2002). BH can be performed in different ways, either by: (1) end-expiratory BH (shortest due to lack of inhibitory lung stretch and a small lung volume reservoir to mix atmospheric air with arterial blood); (2) end-inspiratory BH (longer than expiratory BH); (3) end-inspiratory BH with previous hyperventilation (results in reduction of CO2 due to hyperventilation, which allows this type of BH to last longer); and (4) end-inspiratory BH with previous hyperoxia [similar to (3)] (Skow et al., 2015). The most commonly used BHs in fMRI are standard end-expiratory and end-inspiratory BHs. Computer paced end-expiratory BHs were suggested to show a more intense BOLD signal compared to self-paced breathing, and data were corrected for the delay in onset of the BH (Scouten and Schwarzbauer, 2008). Breathing pace in inspiratory BH also influences the level of fMRI activation, with greater BOLD changes with faster breathing when data was corrected for head motion and delay in BH onset (Chen et al., 2021). A recent review recommended to practice expiratory BHs of 15 s with self-paced recovery period for optimal results (Pinto et al., 2021). BH fMRI studies can be applied in younger populations but suffer from significantly noisier and less activated voxels in children than in adults (Thomason et al., 2005).
A very early fMRI study (1993) of the effect of hypoxia following inspiratory BH reported a decrease in the intensity of BOLD, but no information about correction applied is provided in the article (Stehling et al., 1993). However, subsequent fMRI studies that used the inspiratory and expiratory BH maneuvers consistently observed an increase in activations, however no particular correction for the physiological noise or else was used (Kastrup et al., 1998, 1999a; Li et al., 1999). Yet, end-expiratory BH was found to be associated with both increased BOLD response in the right insula, dorsal anterior cingulate, cerebellum, and fronto-parietal cortex, and decreased BOLD signal in the left insula, ventral anterior cingulate, precentral gyrus and hippocampus (Kimmerly et al., 2013; Sharman et al., 2014). Reported data was corrected for global signal intensity change (Kimmerly et al., 2013) and for respiration and heartbeat (Sharman et al., 2014). These regions are viewed as an autonomic cortical network controlling apnea-induced muscle sympathetic nerve activity (Kimmerly et al., 2013). Inspiratory BH was associated with fMRI activations in the midbrain, pons, cerebellum and lentiform nuclei, but no correction for the data was reported (Gozal et al., 1995). During resistive inspiratory load, higher BOLD activations were observed in ventrolateral and dorsal medulla (PBC) than in caudal ventro-lateral pons (pFRG; Hess et al., 2013). Data were corrected for respiratory volume per time, end-tidal CO2, RR cardiac interval and saturation, and a small smoothing kernel was used, 6 mm.
Most of the studies examined here took into account the variation of end-tidal CO2, likely because it is easy to measure and reflects the variation of O2 in expired air. However, as discussed at the beginning of this section, CO2 and O2 fluctuations will also affect the BOLD signal and these confounds should be considered as part of the data model (Moreton et al., 2016). The first fMRI studies of central control of breathing have used little or no correction for susceptibility and movement artifacts, and also suffered from poor spatial resolution. Due to these limitations, findings from these pioneering studies were difficult to interpret, both in terms of the mechanisms underlying changes in BOLD signal and their precise anatomical location. Yet, most of these findings were confirmed in subsequent studies which have applied an appropriate methodology (Sharman et al., 2014; Chen et al., 2021).
fMRI of Central Respiratory Control in Different Diseases
Sudden unexpected death in epilepsy (SUDEP)
Sudden unexpected death in epilepsy (SUDEP) is the most shattering outcome in patients with epilepsy, typically affecting adolescents and young adults between 20 and 40 years of age with drug resistant epilepsy. SUDEP may account for up to 1/3 of all causes of non-suicidal, non-accidental sudden death in this age range. Recent progresses have pointed to the primary role of post-ictal central respiratory distress (Patodia et al., 2021). Individuals who are at high risk of SUDEP, based on various clinical risk factors, exhibit regional brain structural and FC alterations compared with low-risk patients. In the former, FC was found reduced between pons and thalamus, between midbrain and thalamus (Tang et al., 2014), between the thalamus, brainstem, anterior cingulate, putamen and amygdala, and was elevated between medial/orbital frontal cortex, insula, hippocampus, amygdala, subcallosal cortex, brain stem, thalamus, caudate, and putamen (Allen et al., 2017, 2019). Structural alterations were also identified in patients who died from SUDEP, such as the presence of brainstem atrophy (Mueller et al., 2014) and reduced posterior thalamic gray matter volume, possibly linked to hypoxic challenges from apnea (Wandschneider et al., 2015). Finally, in a mixed population of SUDEP cases and individuals at high risk of SUDEP, fMRI showed impaired communication between several nodes involved in respiratory and cardiovascular regulation (La et al., 2019).
Congenital Central Hypoventilation Syndrome
Congenital central hypoventilation syndrome (CCHS) is a genetic condition characterized specifically by a lack of sensitivity to CO2 and is defined by an important alteration of the automatic control of breathing. Hypoxia, induced through inhalation of 10% oxygen and 90% nitrogen, elicited comparable BOLD responses in CCHS and control subjects in medullary and hypothalamic structures but significantly different patterns in cerebellar, dorsolateral pontine, thalamic, basal ganglia, limbic, and midbrain areas (Macey et al., 2005) and the prefrontal cortex (Zhang et al., 2011). The altered suprapontine control of respiratory function could also account for a dysfunction in the other cognitive processes supported by the same brain regions. In a single CCHS case study, spontaneous breathing showed an increased functional connectivity between the brainstem and the frontal cortex, whereas assisted breathing (mechanical ventilation) resulted in restoration of physiological DMN low frequency oscillations and improved patients’ executive functions (Sharman et al., 2014). During Valsalva maneuvre, a forced exhale and hold task, the overall BOLD response in nine patients with CCHS was muted compared to healthy controls, leading authors to conclude that in CCHS the structures that mediate sympathetic and parasympathetic output are impaired (Ogren et al., 2010).
Obstructive Sleep Apnea
Obstructive sleep apnea (OSA) is characterized by episodes of complete or partial repetitive upper airway collapse during sleep. In 12 patients with OSA, the BH maneuver resulted in less CVR than in controls, while brain regions of decreased CVR were larger in these patients than in controls (Buterbaugh et al., 2015). Expiratory loading in nine patients with OSA resulted in decreased global gray matter signal intensity, which was less pronounced than in healthy participants, and occurred twice as fast in OSA than in controls (20 s into the challenge compared to 40 s in controls; Macey et al., 2003). During this expiratory loading challenge, patients with OSA also showed increased activation in the ventral midbrain and the hippocampus, and decreased activation in the middle frontal gyrus and Broca’s area, insula and anterior cingulate (Macey et al., 2003). Inspiratory loading in seven patients resulted in decreased BOLD signal in the dorsal and ventral striatum, frontal cortex, insula, hippocampus and midbrain, while there were significant increases in activation in the dorsal midbrain, medial cingulate, temporal and cerebellar cortex (Macey et al., 2006). fMRI study using the Valsava maneuver in 21 male subjects with OSA showed a decreased response compared to controls in the left inferior parietal cortex, anterior superior temporal gyrus, posterior insular cortex, cerebellar cortex, fastigial nucleus, and hippocampus (Henderson et al., 2003). Also, in patients with OSA, the DMN pattern is selectively altered (Li et al., 2016; Wu et al., 2020). The areas that show altered BOLD response are usually expressing regional structural changes in the white and gray matter and reduced cortical thickness (Macey et al., 2008, 2018; Canessa et al., 2011).
Conclusion
MR imaging in humans has demonstrated its ability to investigate the neural centers involved in the CNS control of respiration, using various types of experimental protocols. In particular, controlled hypercapnia, hypoxia, as well as breath holding maneuvers enable fMRI activation of brainstem respiratory centers and their hypothalamic, limbic and cortical controlling networks. Such investigations can now be used to explore medical conditions associated with known or suspected dysfunction of central respiratory control, in order to better understand their pathophysiology and to develop novel clinically relevant biomarkers.
Author Contributions
CC collected books and articles for the review, conceived, designed, and wrote the review. SR and PR provided a methodological/clinical perspective and editing of the review, provided general advice on the review. All authors contributed to the article and approved the submitted version.
Funding
The study was supported by two grants from InnoCentive Challenges—The SUDEP Institute Challenge 9933784: Developing Predictive Biomarkers of Epilepsy Seizures. Open access funding was provided by the University of Lausanne.
Conflict of Interest
The authors declare that the research was conducted in the absence of any commercial or financial relationships that could be construed as a potential conflict of interest.
Publisher’s Note
All claims expressed in this article are solely those of the authors and do not necessarily represent those of their affiliated organizations, or those of the publisher, the editors and the reviewers. Any product that may be evaluated in this article, or claim that may be made by its manufacturer, is not guaranteed or endorsed by the publisher.
References
Alheid, G. F., Jiao, W., and Mccrimmon, D. R. (2011). Caudal nuclei of the rat nucleus of the solitary tract differentially innervate respiratory compartments within the ventrolateral medulla. Neuroscience 190, 207–227. doi: 10.1016/j.neuroscience.2011.06.005
Alheid, G. F., and Mccrimmon, D. R. (2008). The chemical neuroanatomy of breathing. Respir. Physiol. Neurobiol. 164, 3–11. doi: 10.1016/j.resp.2008.07.014
Allen, L. A., Harper, R. M., Kumar, R., Guye, M., Ogren, J. A., Lhatoo, S. D., et al. (2017). Dysfunctional brain networking among autonomic regulatory structures in temporal lobe epilepsy patients at high risk of sudden unexpected death in epilepsy. Front. Neurol. 8:544. doi: 10.3389/fneur.2017.00544
Allen, L. A., Harper, R. M., Lhatoo, S., Lemieux, L., and Diehl, B. (2019). Neuroimaging of sudden unexpected death in epilepsy (SUDEP): insights from structural and resting-state functional MRI studies. Front. Neurol. 10:185. doi: 10.3389/fneur.2019.00185
Angelova, P. R., Kasymov, V., Christie, I., Sheikhbahaei, S., Turovsky, E., Marina, N., et al. (2015). Functional oxygen sensitivity of astrocytes. J. Neurosci. 35, 10460–10473. doi: 10.1523/JNEUROSCI.0045-15.2015
Bandettini, P. A., Wong, E. C., Hinks, R. S., Tikofsky, R. S., and Hyde, J. S. (1992). Time course EPI of human brain function during task activation. Magn. Reson. Med. 25, 390–397. doi: 10.1002/mrm.1910250220
Banzett, R. B., Mulnier, H. E., Murphy, K., Rosen, S. D., Wise, R. J., and Adams, L. (2000). Breathlessness in humans activates insular cortex. Neuroreport 11, 2117–2120. doi: 10.1097/00001756-200007140-00012
Beissner, F. (2015). Functional MRI of the brainstem: common problems and their solutions. Clin. Neuroradiol. 25, 251–257. doi: 10.1007/s00062-015-0404-0
Beissner, F., and Baudrexel, S. (2014). Investigating the human brainstem with structural and functional MRI. Front. Hum. Neurosci. 8:116. doi: 10.3389/fnhum.2014.00116
Beissner, F., Deichmann, R., and Baudrexel, S. (2011). fMRI of the brainstem using dual-echo EPI. Neuroimage 55, 1593–1599. doi: 10.1016/j.neuroimage.2011.01.042
Beissner, F., Schumann, A., Brunn, F., Eisenträger, D., and Bär, K.-J. (2014). Advances in functional magnetic resonance imaging of the human brainstem. Neuroimage 86, 91–98. doi: 10.1016/j.neuroimage.2013.07.081
Benarroch, E. E. (1993). The central autonomic network: functional organization, dysfunction and perspective. Mayo Clin. Proc. 68, 988–1001. doi: 10.1016/s0025-6196(12)62272-1
Birn, R. M., Diamond, J. B., Smith, M. A., and Bandettini, P. A. (2006). Separating respiratory-variation-related fluctuations from neuronal-activity-related fluctuations in fMRI. Neuroimage 31, 1536–1548. doi: 10.1016/j.neuroimage.2006.02.048
Birn, R. M., Murphy, K., and Bandettini, P. A. (2008a). The effect of respiration variations on independent component analysis results of resting state functional connectivity. Hum. Brain Mapp. 29, 740–750. doi: 10.1002/hbm.20577
Birn, R. M., Smith, M. A., Jones, T. B., and Bandettini, P. A. (2008b). The respiration response function: the temporal dynamics of fMRI signal fluctuations related to changes in respiration. Neuroimage 40, 644–654. doi: 10.1016/j.neuroimage.2007.11.059
Biswal, B., Yetkin, F. Z., Haughton, V. M., and Hyde, J. S. (1995). Functional connectivity in the motor cortex of resting human brain using echo-planar MRI. Magn. Reson. Med. 34, 537–541. doi: 10.1002/mrm.1910340409
Brannan, S., Liotti, M., Egan, G., Shade, R., Madden, L., Robillard, R., et al. (2001). Neuroimaging of cerebral activations and deactivations associated with hypercapnia and hunger for air. Proc. Natl. Acad. Sci. U S A 98, 2029–2034. doi: 10.1073/pnas.98.4.2029
Bright, M. G., and Murphy, K. (2013). Reliable quantification of BOLD fMRI cerebrovascular reactivity despite poor breath-hold performance. Neuroimage 83, 559–568. doi: 10.1016/j.neuroimage.2013.07.007
Buchanan, G. F., and Richerson, G. B. (2009). Role of chemoreceptors in mediating dyspnea. Respir. Physiol. Neurobiol. 167, 9–19. doi: 10.1016/j.resp.2008.12.002
Burki, N. K., and Lee, L. Y. (2010). Blockade of airway sensory nerves and dyspnea in humans. Pulm. Pharmacol. Ther. 23, 279–282. doi: 10.1016/j.pupt.2010.02.002
Buterbaugh, J., Wynstra, C., Provencio, N., Combs, D., Gilbert, M., and Parthasarathy, S. (2015). Cerebrovascular reactivity in young subjects with sleep apnea. Sleep 38, 241–250. doi: 10.5665/sleep.4406
Caballero-Gaudes, C., and Reynolds, R. C. (2017). Methods for cleaning the BOLD fMRI signal. Neuroimage 154, 128–149. doi: 10.1016/j.neuroimage.2016.12.018
Canessa, N., Castronovo, V., Cappa, S. F., Aloia, M. S., Marelli, S., Falini, A., et al. (2011). Obstructive sleep apnea: brain structural changes and neurocognitive function before and after treatment. Am. J. Respir. Crit. Care Med. 183, 1419–1426. doi: 10.1164/rccm.201005-0693OC
Chan, S. T., Evans, K. C., Song, T. Y., Selb, J., Van Der Kouwe, A., Rosen, B. R., et al. (2020). Cerebrovascular reactivity assessment with O2-CO2 exchange ratio under brief breath hold challenge. PLoS One 15:e0225915. doi: 10.1371/journal.pone.0225915
Chan, P.-Y. S., Wu, Y. T., Hsu, A. L., Li, C. W., Wu, C. W., Von Leupoldt, A., et al. (2019). The effect of anxiety on brain activation patterns in response to inspiratory occlusions: an fMRI study. Sci. Rep. 9:15045. doi: 10.1038/s41598-019-51396-2
Chang, C., Cunningham, J. P., and Glover, G. H. (2009). Influence of heart rate on the BOLD signal: the cardiac response function. Neuroimage 44, 857–869. doi: 10.1016/j.neuroimage.2008.09.029
Chang, C., and Glover, G. H. (2009). Relationship between respiration, end-tidal CO2 and BOLD signals in resting-state fMRI. Neuroimage 47, 1381–1393. doi: 10.1016/j.neuroimage.2009.04.048
Chen, K., Yang, H., Zhang, H., Meng, C., Becker, B., and Biswal, B. (2021). Altered cerebrovascular reactivity due to respiratory rate and breath holding: a BOLD-fMRI study on healthy adults. Brain Struct. Funct. 226, 1229–1239. doi: 10.1007/s00429-021-02236-5
Chonan, T., Mulholland, M. B., Cherniack, N. S., and Altose, M. D. (1987). Effects of voluntary constraining of thoracic displacement during hypercapnia. J. Appl. Physiol. (1985) 63, 1822–1828. doi: 10.1152/jappl.1987.63.5.1822
Cordes, D., Nandy, R. R., Schafer, S., and Wager, T. D. (2014). Characterization and reduction of cardiac- and respiratory-induced noise as a function of the sampling rate (TR) in fMRI. Neuroimage 89, 314–330. doi: 10.1016/j.neuroimage.2013.12.013
Corfield, D. R., Murphy, K., Josephs, O., Adams, L., and Turner, R. (2001). Does hypercapnia-induced cerebral vasodilation modulate the hemodynamic response to neural activation? Neuroimage 13, 1207–1211. doi: 10.1006/nimg.2001.0760
Critchley, H. D., Nicotra, A., Chiesa, P. A., Nagai, Y., Gray, M. A., Minati, L., et al. (2015). Slow breathing and hypoxic challenge: cardiorespiratory consequences and their central neural substrates. PLoS One 10:e0127082. doi: 10.1371/journal.pone.0127082
Dagli, M. S., Ingeholm, J. E., and Haxby, J. V. (1999). Localization of cardiac-induced signal change in fMRI. Neuroimage 9, 407–415. doi: 10.1006/nimg.1998.0424
Dampney, R. A., Horiuchi, J., and Mcdowall, L. M. (2008). Hypothalamic mechanisms coordinating cardiorespiratory function during exercise and defensive behaviour. Auton. Neurosci. 142, 3–10. doi: 10.1016/j.autneu.2008.07.005
Douglas, C. L., Demarco, G. J., Baghdoyan, H. A., and Lydic, R. (2004). Pontine and basal forebrain cholinergic interaction: implications for sleep and breathing. Respir. Physiol. Neurobiol. 143, 251–262. doi: 10.1016/j.resp.2004.02.011
Dubois, A. B. (1952). Alveolar CO2 and O2 during breath holding, expiration and inspiration. J. Appl. Physiol. 5, 1–12. doi: 10.1152/jappl.1952.5.1.1
Dunckley, P., Wise, R. G., Fairhurst, M., Hobden, P., Aziz, Q., Chang, L., et al. (2005). A comparison of visceral and somatic pain processing in the human brainstem using functional magnetic resonance imaging. J. Neurosci. 25, 7333–7341. doi: 10.1523/JNEUROSCI.1100-05.2005
Evans, K. C. (2010). Cortico-limbic circuitry and the airways: insights from functional neuroimaging of respiratory afferents and efferents. Biol. Psychol. 84, 13–25. doi: 10.1016/j.biopsycho.2010.02.005
Evans, K. C., Dougherty, D. D., Schmid, A. M., Scannell, E., Mccallister, A., Benson, H., et al. (2009). Modulation of spontaneous breathing via limbic/paralimbic-bulbar circuitry: an event-related fMRI study. Neuroimage 47, 961–971. doi: 10.1016/j.neuroimage.2009.05.025
Evans, K. C., Shea, S. A., and Saykin, A. J. (1999). Functional MRI localisation of central nervous system regions associated with volitional inspiration in humans. J. Physiol. 520, 383–392. doi: 10.1111/j.1469-7793.1999.00383.x
Feinstein, J. S., Gould, D., and Khalsa, S. S. (2022). Amygdala-driven apnea and the chemoreceptive origin of anxiety. Biol. Psychol. 170:108305. doi: 10.1016/j.biopsycho.2022.108305
Feldman, J. L., Del Negro, C. A., and Gray, P. A. (2013). Understanding the rhythm of breathing: so near, yet so far. Annu. Rev. Physiol. 75, 423–452. doi: 10.1146/annurev-physiol-040510-130049
Fukushi, I., Pokorski, M., and Okada, Y. (2021). Mechanisms underlying the sensation of dyspnea. Respir. Investig. 59, 66–80. doi: 10.1016/j.resinv.2020.10.007
Gerlach, D. A., Manuel, J., Hoff, A., Kronsbein, H., Hoffmann, F., Heusser, K., et al. (2021). Medullary and hypothalamic functional magnetic imaging during acute hypoxia in tracing human peripheral chemoreflex responses. Hypertension 77, 1372–1382. doi: 10.1161/HYPERTENSIONAHA.120.16385
Glover, G. H., Li, T. Q., and Ress, D. (2000). Image-based method for retrospective correction of physiological motion effects in fMRI: RETROICOR. Magn. Reson. Med. 44, 162–167. doi: 10.1002/1522-2594(200007)44:1<162::aid-mrm23>3.0.co;2-e
Godfrey, S., and Campbell, E. J. M. (1968). The control of breath holding. Respir. Physiol. 5, 385–400. doi: 10.1016/0034-5687(68)90030-3
Golestani, A. M., Chang, C., Kwinta, J. B., Khatamian, Y. B., and Jean Chen, J. (2015). Mapping the end-tidal CO2 response function in the resting-state BOLD fMRI signal: spatial specificity, test-retest reliability and effect of fMRI sampling rate. Neuroimage 104, 266–277. doi: 10.1016/j.neuroimage.2014.10.031
Golestani, A. M., and Chen, J. J. (2020). Controlling for the effect of arterial-CO2 fluctuations in resting-state fMRI: comparing end-tidal CO2 clamping and retroactive CO2 correction. Neuroimage 216:116874. doi: 10.1016/j.neuroimage.2020.116874
Gourine, A. V., and Funk, G. D. (2017). On the existence of a central respiratory oxygen sensor. J. Appl. Physiol. (1985) 123, 1344–1349. doi: 10.1152/japplphysiol.00194.2017
Gourine, A. V., Llaudet, E., Dale, N., and Spyer, K. M. (2005). ATP is a mediator of chemosensory transduction in the central nervous system. Nature 436, 108–111. doi: 10.1038/nature03690
Gozal, D., Hathout, G. M., Kirlew, K. A., Tang, H., Woo, M. S., Zhang, J., et al. (1994). Localization of putative neural respiratory regions in the human by functional magnetic resonance imaging. J. Appl. Physiol. (1985) 76, 2076–2083. doi: 10.1152/jappl.1994.76.5.2076
Gozal, D., Omidvar, O., Kirlew, K. A., Hathout, G. M., Hamilton, R., Lufkin, R. B., et al. (1995). Identification of human brain regions underlying responses to resistive inspiratory loading with functional magnetic resonance imaging. Proc. Natl. Acad. Sci. U S A 92, 6607–6611. doi: 10.1073/pnas.92.14.6607
Greicius, M. D., Krasnow, B., Reiss, A. L., and Menon, V. (2003). Functional connectivity in the resting brain: a network analysis of the default mode hypothesis. Proc. Natl. Acad. Sci. U S A 100, 253–258. doi: 10.1073/pnas.0135058100
Guz, A. (1997). Brain, breathing and breathlessness. Respir. Physiol. 109, 197–204. doi: 10.1016/s0034-5687(97)00050-9
Harita, S., and Stroman, P. W. (2017). Confirmation of resting-state BOLD fluctuations in the human brainstem and spinal cord after identification and removal of physiological noise. Magn. Reson. Med. 78, 2149–2156. doi: 10.1002/mrm.26606
Harper, R. M., Gozal, D., Bandler, R., Spriggs, D., Lee, J., and Alger, J. (1998). Regional brain activation in humans during respiratory and blood pressure challenges. Clin. Exp. Pharmacol. Physiol. 25, 483–486. doi: 10.1111/j.1440-1681.1998.tb02240.x
Harper, R. M., Kumar, R., Macey, P. M., Woo, M. A., and Ogren, J. A. (2014). Affective brain areas and sleep-disordered breathing. Prog. Brain Res. 209, 275–293. doi: 10.1016/B978-0-444-63274-6.00014-X
Harper, R. M., Macey, P. M., Woo, M. A., Macey, K. E., Keens, T. G., Gozal, D., et al. (2005). Hypercapnic exposure in congenital central hypoventilation syndrome reveals CNS respiratory control mechanisms. J. Neurophysiol. 93, 1647–1658. doi: 10.1152/jn.00863.2004
Harvey, A. K., Pattinson, K. T., Brooks, J. C., Mayhew, S. D., Jenkinson, M., and Wise, R. G. (2008). Brainstem functional magnetic resonance imaging: disentangling signal from physiological noise. J. Magn. Reson. Imaging 28, 1337–1344. doi: 10.1002/jmri.21623
Henderson, L. A., Woo, M. A., Macey, P. M., Macey, K. E., Frysinger, R. C., Alger, J. R., et al. (2003). Neural responses during Valsalva maneuvers in obstructive sleep apnea syndrome. J. Appl. Physiol. (1985) 94, 1063–1074. doi: 10.1152/japplphysiol.00702.2002
Hess, A., Yu, L., Klein, I., De Mazancourt, M., Jebrak, G., Mal, H., et al. (2013). Neural mechanisms underlying breathing complexity. PLoS One 8:e75740. doi: 10.1371/journal.pone.0075740
Heyman, A., Birchfield, R. I., and Sieker, H. O. (1958). Effects of bilateral cerebral infarction on respiratory center sensitivity. Neurology 8, 694–700. doi: 10.1212/wnl.8.9.694
Honda, Y., Natsui, T., Hasumura, N., and Nakamura, K. (1963). Threshold Pco2 for respiratory system in acute hypoxia of dogs. J. Appl. Physiol. 18, 1053–1056. doi: 10.1152/jappl.1963.18.6.1053
Hong, S. K., Lin, Y. C., Lally, D. A., Yim, B. J., Kominami, N., Hong, P. W., et al. (1971). Alveolar gas exchanges and cardiovascular functions during breath holding with air. J. Appl. Physiol. 30, 540–547. doi: 10.1152/jappl.1971.30.4.540
Horn, E. M., and Waldrop, T. G. (1998). Suprapontine control of respiration. Respir. Physiol. 114, 201–211. doi: 10.1016/s0034-5687(98)00087-5
Hsu, Y. Y., Chang, C. N., Jung, S. M., Lim, K. E., Huang, J. C., Fang, S. Y., et al. (2004). Blood oxygenation level-dependent MRI of cerebral gliomas during breath holding. J. Magn. Reson. Imaging 19, 160–167. doi: 10.1002/jmri.10447
Hu, X., Le, T. H., Parrish, T., and Erhard, P. (1995). Retrospective estimation and correction of physiological fluctuation in functional MRI. Magn. Reson. Med. 34, 201–212. doi: 10.1002/mrm.1910340211
Iacovella, V., and Hasson, U. (2011). The relationship between BOLD signal and autonomic nervous system functions: implications for processing of “physiological noise”. Magn. Reson. Imaging 29, 1338–1345. doi: 10.1016/j.mri.2011.03.006
Ikeda, K., Kawakami, K., Onimaru, H., Okada, Y., Yokota, S., Koshiya, N., et al. (2017). The respiratory control mechanisms in the brainstem and spinal cord: integrative views of the neuroanatomy and neurophysiology. J. Physiol. Sci. 67, 45–62. doi: 10.1007/s12576-016-0475-y
Iranmahboob, A., Peck, K. K., Brennan, N. P., Karimi, S., Fisicaro, R., Hou, B., et al. (2016). Vascular reactivity maps in patients with gliomas using breath-holding BOLD fMRI. J. Neuroimaging 26, 232–239. doi: 10.1111/jon.12278
Jarrahi, B. (2021). An ICA investigation into the effect of physiological noise correction on dimensionality and spatial maps of intrinsic connectivity networks. Annu. Int. Conf. IEEE Eng. Med. Biol. Soc. 2021, 3145–3148. doi: 10.1109/EMBC46164.2021.9629877
Javaheri, S., and Kazemi, H. (1987). Metabolic alkalosis and hypoventilation in humans. Am. Rev. Respir. Dis. 136, 1011–1016. doi: 10.1164/ajrccm/136.4.1011
Jones, T. B., Bandettini, P. A., and Birn, R. M. (2008). Integration of motion correction and physiological noise regression in fMRI. Neuroimage 42, 582–590. doi: 10.1016/j.neuroimage.2008.05.019
Jung, J. Y., Park, C. A., Lee, Y. B., and Kang, C. K. (2020). Investigation of functional connectivity differences between voluntary respirations via mouth and nose using resting state fMRI. Brain Sci. 10:704. doi: 10.3390/brainsci10100704
Kahn, N., and Wang, S. C. (1967). Electrophysiologic basis for pontine apneustic center and its role in integration of the Hering-Breuer reflex. J. Neurophysiol. 30, 301–318. doi: 10.1152/jn.1967.30.2.301
Kannurpatti, S. S., Biswal, B. B., and Hudetz, A. G. (2002). Differential fMRI-BOLD signal response to apnea in humans and anesthetized rats. Magn. Reson. Med. 47, 864–870. doi: 10.1002/mrm.10131
Kastrup, A., Kruger, G., Glover, G. H., and Moseley, M. E. (1999a). Assessment of cerebral oxidative metabolism with breath holding and fMRI. Magn. Reson. Med. 42, 608–611. doi: 10.1002/(sici)1522-2594(199909)42:3<608::aid-mrm26>3.0.co;2-i
Kastrup, A., Krüger, G., Glover, G. H., Neumann-Haefelin, T., and Moseley, M. E. (1999b). Regional variability of cerebral blood oxygenation response to hypercapnia. Neuroimage 10, 675–681. doi: 10.1006/nimg.1999.0505
Kastrup, A., Li, T. Q., Glover, G. H., and Moseley, M. E. (1999c). Cerebral blood flow-related signal changes during breath-holding. Am. J. Neuroradiol. 20, 1233–1238.
Kastrup, A., Li, T. Q., Takahashi, A., Glover, G. H., and Moseley, M. E. (1998). Functional magnetic resonance imaging of regional cerebral blood oxygenation changes during breath holding. Stroke 29, 2641–2645. doi: 10.1161/01.str.29.12.2641
Khalili-Mahani, N., Chang, C., Van Osch, M. J., Veer, I. M., Van Buchem, M. A., Dahan, A., et al. (2013). The impact of “physiological correction” on functional connectivity analysis of pharmacological resting state fMRI. Neuroimage 65, 499–510. doi: 10.1016/j.neuroimage.2012.09.044
Kimmerly, D. S., Morris, B. L., and Floras, J. S. (2013). Apnea-induced cortical BOLD-fMRI and peripheral sympathoneural firing response patterns of awake healthy humans. PLoS One 8:e82525. doi: 10.1371/journal.pone.0082525
Kruger, G., and Glover, G. H. (2001). Physiological noise in oxygenation-sensitive magnetic resonance imaging. Magn. Reson. Med. 46, 631–637. doi: 10.1002/mrm.1240
Kundu, P., Inati, S. J., Evans, J. W., Luh, W. M., and Bandettini, P. A. (2012). Differentiating BOLD and non-BOLD signals in fMRI time series using multi-echo EPI. Neuroimage 60, 1759–1770. doi: 10.1016/j.neuroimage.2011.12.028
La, A., Rm, H., M, G., R, K., Ja, O., Sb, V., et al. (2019). Altered brain connectivity in sudden unexpected death in epilepsy (SUDEP) revealed using resting-state fMRI. Neuroimage Clin. 24:102060. doi: 10.1016/j.nicl.2019.102060
Lahiri, S., Mokashi, A., Delaney, R. G., and Fishman, A. P. (1978). Arterial PO2 and PCO2 stimulus threshold for carotid chemoreceptors and breathing. Respir. Physiol. 34, 359–375. doi: 10.1016/0034-5687(78)90134-2
Lalley, P. M. (1986). Responses of phrenic motoneurones of the cat to stimulation of medullary raphe nuclei. J. Physiol. 380, 349–371. doi: 10.1113/jphysiol.1986.sp016290
Ledoux, J. E. (2000). Emotion circuits in the brain. Annu. Rev. Neurosci. 23, 155–184. doi: 10.1146/annurev.neuro.23.1.155
Li, T.-Q., Kastrup, A., Takahashi, A. M., and Moseley, M. E. (1999). Functional MRI of human brain during breath holding by BOLD and FAIR techniques. NeuroImage 9, 243–249. doi: 10.1006/nimg.1998.0399
Li, H. J., Nie, X., Gong, H. H., Zhang, W., Nie, S., and Peng, D. C. (2016). Abnormal resting-state functional connectivity within the default mode network subregions in male patients with obstructive sleep apnea. Neuropsychiatr. Dis. Treat. 12, 203–212. doi: 10.2147/NDT.S97449
Li, M. X., Yan, C. Y., and Wang, S. (2015). New insights on the role of the insular cortex and habenula in OSA. Sleep Breath. 19, 1347–1353. doi: 10.1007/s11325-015-1168-0
Lindholm, P., and Linnarsson, D. (2002). Pulmonary gas exchange during apnoea in exercising men. Eur. J. Appl. Physiol. 86, 487–491. doi: 10.1007/s00421-002-0581-9
Lindholm, P., and Lundgren, C. E. G. (2006). Alveolar gas composition before and after maximal breath-holds in competitive divers. Undersea Hyperb. Med. 33, 463–467.
Linnman, C., Moulton, E. A., Barmettler, G., Becerra, L., and Borsook, D. (2012). Neuroimaging of the periaqueductal gray: state of the field. Neuroimage 60, 505–522. doi: 10.1016/j.neuroimage.2011.11.095
Liotti, M., Brannan, S., Egan, G., Shade, R., Madden, L., Abplanalp, B., et al. (2001). Brain responses associated with consciousness of breathlessness (air hunger). Proc. Natl. Acad. Sci. U S A 98, 2035–2040. doi: 10.1073/pnas.98.4.2035
Liu, P., De Vis, J. B., and Lu, H. (2019). Cerebrovascular reactivity (CVR) MRI with CO2 challenge: a technical review. Neuroimage 187, 104–115. doi: 10.1016/j.neuroimage.2018.03.047
Liu, H. L., Huang, J., Wu, C. T., and Hsu, Y. Y. (2002). Detectability of blood oxygenation level-dependent signal changes during short breath hold duration. Magn. Reson. Imaging 20, 643–648. doi: 10.1016/s0730-725x(02)00595-7
Liu, P., Xu, C., Lin, Z., Sur, S., Li, Y., Yasar, S., et al. (2020). Cerebrovascular reactivity mapping using intermittent breath modulation. Neuroimage 215:116787. doi: 10.1016/j.neuroimage.2020.116787
Macefield, V. G., Gandevia, S. C., and Henderson, L. A. (2006). Neural sites involved in the sustained increase in muscle sympathetic nerve activity induced by inspiratory capacity apnea: a fMRI study. J. Appl. Physiol. (1985) 100, 266–273. doi: 10.1152/japplphysiol.00588.2005
Macey, P. M., Haris, N., Kumar, R., Thomas, M. A., Woo, M. A., and Harper, R. M. (2018). Obstructive sleep apnea and cortical thickness in females and males. PLoS One 13:e0193854. doi: 10.1371/journal.pone.0193854
Macey, P. M., Kumar, R., Woo, M. A., Valladares, E. M., Yan-Go, F. L., and Harper, R. M. (2008). Brain structural changes in obstructive sleep apnea. Sleep 31, 967–977. doi: 10.5665/sleep/31.7.967
Macey, P. M., Macey, K. E., Henderson, L. A., Alger, J. R., Frysinger, R. C., Woo, M. A., et al. (2003). Functional magnetic resonance imaging responses to expiratory loading in obstructive sleep apnea. Respir. Physiol. Neurobiol. 138, 275–290. doi: 10.1016/j.resp.2003.09.002
Macey, P. M., Macey, K. E., Kumar, R., and Harper, R. M. (2004). A method for removal of global effects from fMRI time series. Neuroimage 22, 360–366. doi: 10.1016/j.neuroimage.2003.12.042
Macey, K. E., Macey, P. M., Woo, M. A., Harper, R. K., Alger, J. R., Keens, T. G., et al. (2004). fMRI signal changes in response to forced expiratory loading in congenital central hypoventilation syndrome. J. Appl. Physiol. (1985) 97, 1897–1907. doi: 10.1152/japplphysiol.00359.2004
Macey, K. E., Macey, P. M., Woo, M. A., Henderson, L. A., Frysinger, R. C., Harper, R. K., et al. (2006). Inspiratory loading elicits aberrant fMRI signal changes in obstructive sleep apnea. Respir. Physiol. Neurobiol. 151, 44–60. doi: 10.1016/j.resp.2005.05.024
Macey, P. M., Woo, M. A., Macey, K. E., Keens, T. G., Saeed, M. M., Alger, J. R., et al. (2005). Hypoxia reveals posterior thalamic, cerebellar, midbrain and limbic deficits in congenital central hypoventilation syndrome. J. Appl. Physiol. (1985) 98, 958–969. doi: 10.1152/japplphysiol.00969.2004
Magon, S., Basso, G., Farace, P., Ricciardi, G. K., Beltramello, A., and Sbarbati, A. (2009). Reproducibility of BOLD signal change induced by breath holding. Neuroimage 45, 702–712. doi: 10.1016/j.neuroimage.2008.12.059
Mandell, D. M., Han, J. S., Poublanc, J., Crawley, A. P., Stainsby, J. A., Fisher, J. A., et al. (2008). Mapping cerebrovascular reactivity using blood oxygen level-dependent MRI in Patients with arterial steno-occlusive disease: comparison with arterial spin labeling MRI. Stroke 39, 2021–2028. doi: 10.1161/STROKEAHA.107.506709
Martino, P. F., Davis, S., Opansky, C., Krause, K., Bonis, J. M., Pan, L. G., et al. (2007). The cerebellar fastigial nucleus contributes to CO2-H+ ventilatory sensitivity in awake goats. Respir. Physiol. Neurobiol. 157, 242–251. doi: 10.1016/j.resp.2007.01.019
Matt, E., Fischmeister, F. P. S., Amini, A., Robinson, S. D., Weber, A., Foki, T., et al. (2019). Improving sensitivity, specificity and reproducibility of individual brainstem activation. Brain Struct. Funct. 224, 2823–2838. doi: 10.1007/s00429-019-01936-3
Mazzone, S. B., Mclennan, L., Mcgovern, A. E., Egan, G. F., and Farrell, M. J. (2007). Representation of capsaicin-evoked urge-to-cough in the human brain using functional magnetic resonance imaging. Am. J. Respir. Crit. Care Med. 176, 327–332. doi: 10.1164/rccm.200612-1856OC
Mckay, L. C., Adams, L., Frackowiak, R. S., and Corfield, D. R. (2008). A bilateral cortico-bulbar network associated with breath holding in humans, determined by functional magnetic resonance imaging. Neuroimage 40, 1824–1832. doi: 10.1016/j.neuroimage.2008.01.058
Mckay, L. C., Evans, K. C., Frackowiak, R. S. J., and Corfield, D. R. (2003). Neural correlates of voluntary breathing in humans. J. Appl. Physiol. (1985) 95, 1170–1178. doi: 10.1152/japplphysiol.00641.2002
Mcketton, L., Sam, K., Poublanc, J., Crawley, A. P., Sobczyk, O., Venkatraghavan, L., et al. (2021). The effect of CO2 on resting-state functional connectivity: Isocapnia vs. Poikilocapnia. Front. Physiol. 12:639782. doi: 10.3389/fphys.2021.639782
Memmler, R. L., Cohen, B. J., and Wood, D. L. (1992). The Human Body in Health and Disease. Philadelphia; New York [et autres]: J. B. Lippincott.
Mikhail, Y., and Ahmed, Y. Y. (1975). Outline of the arcuate nucleus in the human medulla oblongata. Acta Anat. (Basel) 92, 285–291. doi: 10.1159/000144447
Moher Alsady, T., Blessing, E. M., and Beissner, F. (2016). MICA-A toolbox for masked independent component analysis of fMRI data. Hum. Brain Mapp. 37, 3544–3556. doi: 10.1002/hbm.23258
Moreton, F. C., Dani, K. A., Goutcher, C., O’hare, K., and Muir, K. W. (2016). Respiratory challenge MRI: practical aspects. Neuroimage Clin. 11, 667–677. doi: 10.1016/j.nicl.2016.05.003
Morris, K. F., Nuding, S. C., Segers, L. S., Baekey, D. M., Shannon, R., Lindsey, B. G., et al. (2010). Respiratory and Mayer wave-related discharge patterns of raphe and pontine neurons change with vagotomy. J. Appl. Physiol. (1985) 109, 189–202. doi: 10.1152/japplphysiol.01324.2009
Mueller, S. G., Bateman, L. M., and Laxer, K. D. (2014). Evidence for brainstem network disruption in temporal lobe epilepsy and sudden unexplained death in epilepsy. Neuroimage Clin. 5, 208–216. doi: 10.1016/j.nicl.2014.06.010
Nagai, M., Hoshide, S., and Kario, K. (2010). The insular cortex and cardiovascular system: a new insight into the brain-heart axis. J. Am. Soc. Hypertens. 4, 174–182. doi: 10.1016/j.jash.2010.05.001
Nogues, M. A., and Benarroch, E. (2008). Abnormalities of respiratory control and the respiratory motor unit. Neurologist 14, 273–288. doi: 10.1097/NRL.0b013e318173e830
Nogues, M. A., Roncoroni, A. J., and Benarroch, E. (2002). Breathing control in neurological diseases. Clin. Auton. Res. 12, 440–449. doi: 10.1007/s10286-002-0067-1
Ogoh, S., Suzuki, K., Washio, T., Tamiya, K., Saito, S., Bailey, T. G., et al. (2019). Does respiratory drive modify the cerebral vascular response to changes in end-tidal carbon dioxide? Exp. Physiol. 104, 1363–1370. doi: 10.1113/EP087744
Ogren, J. A., Macey, P. M., Kumar, R., Woo, M. A., and Harper, R. M. (2010). Central autonomic regulation in congenital central hypoventilation syndrome. Neuroscience 167, 1249–1256. doi: 10.1016/j.neuroscience.2010.02.078
Okada, Y., Kuwana, S., Masumiya, H., Kimura, N., Chen, Z., and Oku, Y. (2008). Chemosensitive neuronal network organization in the ventral medulla analyzed by dynamic voltage-imaging. Adv. Exp. Med. Biol. 605, 353–357. doi: 10.1007/978-0-387-73693-8_62
Otis, A. B., Rahn, H., and Fenn, W. O. (1948). Alveolar gas changes during breath holding. Am. J. Physiol. 152, 674–686. doi: 10.1152/ajplegacy.1948.152.3.674
Parkes, M. J. (2006). Breath-holding and its breakpoint. Exp. Physiol. 91, 1–15. doi: 10.1113/expphysiol.2005.031625
Parsons, L. M., Egan, G., Liotti, M., Brannan, S., Denton, D., Shade, R., et al. (2001). Neuroimaging evidence implicating cerebellum in the experience of hypercapnia and hunger for air. Proc. Natl. Acad. Sci. U S A 98, 2041–2046. doi: 10.1073/pnas.98.4.2041
Patodia, S., Somani, A., and Thom, M. (2021). Review: neuropathology findings in autonomic brain regions in SUDEP and future research directions. Auton. Neurosci. 235:102862. doi: 10.1016/j.autneu.2021.102862
Pattinson, K. T. S., Governo, R. J., Macintosh, B. J., Russell, E. C., Corfield, D. R., Tracey, I., et al. (2009a). Opioids depress cortical centers responsible for the volitional control of respiration. J. Neurosci. 29, 8177–8186. doi: 10.1523/JNEUROSCI.1375-09.2009
Pattinson, K. T. S., Mitsis, G. D., Harvey, A. K., Jbabdi, S., Dirckx, S., Mayhew, S. D., et al. (2009b). Determination of the human brainstem respiratory control network and its cortical connections in vivo using functional and structural imaging. Neuroimage 44, 295–305. doi: 10.1016/j.neuroimage.2008.09.007
Piche, M., Cohen-Adad, J., Nejad, M. K., Perlbarg, V., Xie, G., Beaudoin, G., et al. (2009). Characterization of cardiac-related noise in fMRI of the cervical spinal cord. Magn. Reson. Imaging 27, 300–310. doi: 10.1016/j.mri.2008.07.019
Pindzola, R. R., Balzer, J. R., Nemoto, E. M., Goldstein, S., and Yonas, H. (2001). Cerebrovascular reserve in patients with carotid occlusive disease assessed by stable xenon-enhanced ct cerebral blood flow and transcranial Doppler. Stroke 32, 1811–1817. doi: 10.1161/01.str.32.8.1811
Pinto, J., Bright, M. G., Bulte, D. P., and Figueiredo, P. (2021). Cerebrovascular reactivity mapping without gas challenges: a methodological guide. Front. Physiol. 11:608475. doi: 10.3389/fphys.2020.608475
Poser, B. A., Versluis, M. J., Hoogduin, J. M., and Norris, D. G. (2006). BOLD contrast sensitivity enhancement and artifact reduction with multiecho EPI: parallel-acquired inhomogeneity-desensitized fMRI. Magn. Reson. Med. 55, 1227–1235. doi: 10.1002/mrm.20900
Poublanc, J., Crawley, A. P., Sobczyk, O., Montandon, G., Sam, K., Mandell, D. M., et al. (2015). Measuring cerebrovascular reactivity: the dynamic response to a step hypercapnic stimulus. J. Cereb. Blood Flow Metab. 35, 1746–1756. doi: 10.1038/jcbfm.2015.114
Prasad, S., Pal, P. K., and Chen, R. (2021). “Chapter 1 - breathing and the nervous system,” in Aminoff’s Neurology and General Medicine (Sixth Edition), eds M. J. Aminoff and S. A. Josephson (Boston: Academic Press), 3–19.
Prokopiou, P. C., Pattinson, K. T. S., Wise, R. G., and Mitsis, G. D. (2019). Modeling of dynamic cerebrovascular reactivity to spontaneous and externally induced CO2 fluctuations in the human brain using BOLD-fMRI. Neuroimage 186, 533–548. doi: 10.1016/j.neuroimage.2018.10.084
Ramsay, S. C., Adams, L., Murphy, K., Corfield, D. R., Grootoonk, S., Bailey, D. L., et al. (1993). Regional cerebral blood flow during volitional expiration in man: a comparison with volitional inspiration. J. Physiol. 461, 85–101. doi: 10.1113/jphysiol.1993.sp019503
Roberts, P., Jezzard, P., and Bulte, D. (2009). Comparison of breath holding techniques for the calibration of FMRI measurements of oxygen metabolism. Proc. Intl. Soc. Mag. Reson. Med. 17:1532.
Scouten, A., and Schwarzbauer, C. (2008). Paced respiration with end-expiration technique offers superior BOLD signal repeatability for breath-hold studies. Neuroimage 43, 250–257. doi: 10.1016/j.neuroimage.2008.03.052
Sharman, M., Gallea, C., Lehongre, K., Galanaud, D., Nicolas, N., Similowski, T., et al. (2014). The cerebral cost of breathing: an FMRI case-study in congenital central hypoventilation syndrome. PLoS One 9:e107850. doi: 10.1371/journal.pone.0107850
Shea, S. A. (1996). Behavioural and arousal-related influences on breathing in humans. Exp. Physiol. 81, 1–26. doi: 10.1113/expphysiol.1996.sp003911
Sheikhbahaei, S., Turovsky, E. A., Hosford, P. S., Hadjihambi, A., Theparambil, S. M., Liu, B., et al. (2018). Astrocytes modulate brainstem respiratory rhythm-generating circuits and determine exercise capacity. Nat. Commun. 9:370. doi: 10.1038/s41467-017-02723-6
Skow, R. J., Day, T. A., Fuller, J. E., Bruce, C. D., and Steinback, C. D. (2015). The ins and outs of breath holding: simple demonstrations of complex respiratory physiology. Adv. Physiol. Educ. 39, 223–231. doi: 10.1152/advan.00030.2015
Šmejkal, V., Druga, R., and Tintěra, J. (1999). Control of breathing and brain activation in human subjects seen by functional magnetic resonance imaging. Physiol. Res. 48, 21–25.
Šmejkal, V., Druga, R., and Tintěra, J. (2000). Brain activation during volitional control of breathing. Physiol. Res. 49, 659–663.
Smith, J. C., Abdala, A. P., Borgmann, A., Rybak, I. A., and Paton, J. F. (2013). Brainstem respiratory networks: building blocks and microcircuits. Trends Neurosci. 36, 152–162. doi: 10.1016/j.tins.2012.11.004
Smith, J. C., Abdala, A. P., Rybak, I. A., and Paton, J. F. (2009). Structural and functional architecture of respiratory networks in the mammalian brainstem. Philos. Trans. R. Soc. Lond. B Biol. Sci. 364, 2577–2587. doi: 10.1098/rstb.2009.0081
Spano, V. R., Mandell, D. M., Poublanc, J., Sam, K., Battisti-Charbonney, A., Pucci, O., et al. (2013). CO2 blood oxygen level-dependent MR mapping of cerebrovascular reserve in a clinical population: safety, tolerability and technical feasibility. Radiology 266, 592–598. doi: 10.1148/radiol.12112795
Stehling, M. K., Schmitt, F., and Ladebeck, R. (1993). Echo-planar MR imaging of human brain oxygenation changes. J. Magn. Reson. Imaging 3, 471–474. doi: 10.1002/jmri.1880030307
Strohl, K. P., and Altose, M. D. (1984). Oxygen saturation during breath-holding and during Apneas in sleep. Chest 85, 181–186. doi: 10.1378/chest.85.2.181
Sutterlin, S., Schroijen, M., Constantinou, E., Smets, E., Van Den Bergh, O., and Van Diest, I. (2013). Breath holding duration as a measure of distress tolerance: examining its relation to measures of executive control. Front. Psychol. 4:483. doi: 10.3389/fpsyg.2013.00483
Tancredi, F. B., and Hoge, R. D. (2013). Comparison of cerebral vascular reactivity measures obtained using breath-holding and CO2 inhalation. J. Cereb. Blood Flow Metab. 33, 1066–1074. doi: 10.1038/jcbfm.2013.48
Tang, Y., Chen, Q., Yu, X., Xia, W., Luo, C., Huang, X., et al. (2014). A resting-state functional connectivity study in patients at high risk for sudden unexpected death in epilepsy. Epilepsy Behav. 41, 33–38. doi: 10.1016/j.yebeh.2014.08.140
Tenney, S. M., and Ou, L. C. (1977). Ventilatory response of decorticate and decerebrate cats to hypoxia and CO2. Respir. Physiol. 29, 81–92. doi: 10.1016/0034-5687(77)90119-0
Thomason, M. E., Burrows, B. E., Gabrieli, J. D., and Glover, G. H. (2005). Breath holding reveals differences in fMRI BOLD signal in children and adults. Neuroimage 25, 824–837. doi: 10.1016/j.neuroimage.2004.12.026
Tong, Y., Hocke, L. M., and Frederick, B. B. (2019). Low frequency systemic hemodynamic "noise" in resting state BOLD fMRI: characteristics, causes, implications, mitigation strategies and applications. Front. Neurosci. 13:787. doi: 10.3389/fnins.2019.00787
Van De Moortele, P. F., Pfeuffer, J., Glover, G. H., Ugurbil, K., and Hu, X. (2002). Respiration-induced B0 fluctuations and their spatial distribution in the human brain at 7 Tesla. Magn. Reson. Med. 47, 888–895. doi: 10.1002/mrm.10145
Vernieri, F., Pasqualetti, P., Passarelli, F., Rossini, P. M., and Silvestrini, M. (1999). Outcome of carotid artery occlusion is predicted by cerebrovascular reactivity. Stroke 30, 593–598. doi: 10.1161/01.str.30.3.593
Von Leupoldt, A., and Dahme, B. (2005). Cortical substrates for the perception of dyspnea. Chest 128, 345–354. doi: 10.1378/chest.128.1.345
Wallace, T. E., Manavaki, R., Graves, M. J., Patterson, A. J., and Gilbert, F. J. (2017). Impact of physiological noise correction on detecting blood oxygenation level-dependent contrast in the breast. Phys. Med. Biol. 62, 127–145. doi: 10.1088/1361-6560/62/1/127
Wandschneider, B., Koepp, M., Scott, C., Micallef, C., Balestrini, S., Sisodiya, S. M., et al. (2015). Structural imaging biomarkers of sudden unexpected death in epilepsy. Brain 138, 2907–2919. doi: 10.1093/brain/awv233
Weibull, A., Gustavsson, H., Mattsson, S., and Svensson, J. (2008). Investigation of spatial resolution, partial volume effects and smoothing in functional MRI using artificial 3D time series. Neuroimage 41, 346–353. doi: 10.1016/j.neuroimage.2008.02.015
Windischberger, C., Langenberger, H., Sycha, T., Tschernko, E. M., Fuchsjager-Mayerl, G., Schmetterer, L., et al. (2002). On the origin of respiratory artifacts in BOLD-EPI of the human brain. Magn. Reson. Imaging 20, 575–582. doi: 10.1016/s0730-725x(02)00563-5
Worsley, K. J., and Friston, K. J. (1995). Analysis of fMRI time-series revisited–again. Neuroimage 2, 173–181. doi: 10.1006/nimg.1995.1023
Wu, Y., Zhao, W., Chen, X., Wan, X., and Lei, X. (2020). Aberrant awake spontaneous brain activity in obstructive sleep apnea: a review focused on resting-state EEG and resting-state fMRI. Front. Neurol. 11:768. doi: 10.3389/fneur.2020.00768
Yao, J. F., Yang, H. S., Wang, J. H., Liang, Z., Talavage, T. M., Tamer, G. G., et al. (2021). A novel method of quantifying hemodynamic delays to improve hemodynamic response and CVR estimates in CO2 challenge fMRI. J. Cereb. Blood Flow Metab. 41, 1886–1898. doi: 10.1177/0271678X20978582
Yuan, H., Zotev, V., Phillips, R., and Bodurka, J. (2013). Correlated slow fluctuations in respiration, EEG and BOLD fMRI. Neuroimage 79, 81–93. doi: 10.1016/j.neuroimage.2013.04.068
Zaccaro, A., Piarulli, A., Laurino, M., Garbella, E., Menicucci, D., Neri, B., et al. (2018). How breath-control can change your life: a systematic review on psycho-physiological correlates of slow breathing. Front. Hum. Neurosci. 12:353. doi: 10.3389/fnhum.2018.00353
Zhang, X., Ma, L., Li, S., Wang, Y., and Wang, L. (2011). A functional MRI evaluation of frontal dysfunction in patients with severe obstructive sleep apnea. Sleep Med. 12, 335–340. doi: 10.1016/j.sleep.2010.08.015
Keywords: central respiratory control, brainstem, fMRI, breathing, forebrain
Citation: Ciumas C, Rheims S and Ryvlin P (2022) fMRI studies evaluating central respiratory control in humans. Front. Neural Circuits 16:982963. doi: 10.3389/fncir.2022.982963
Received: 30 June 2022; Accepted: 01 September 2022;
Published: 23 September 2022
Edited by:
Steven Allen Crone, Cincinnati Children’s Hospital Medical Center, United StatesReviewed by:
Ali Golestani, Tom Baker Cancer Centre, CanadaPaul M. Macey, University of California, Los Angeles, United States
Copyright © 2022 Ciumas, Rheims and Ryvlin. This is an open-access article distributed under the terms of the Creative Commons Attribution License (CC BY). The use, distribution or reproduction in other forums is permitted, provided the original author(s) and the copyright owner(s) are credited and that the original publication in this journal is cited, in accordance with accepted academic practice. No use, distribution or reproduction is permitted which does not comply with these terms.
*Correspondence: Carolina Ciumas, carolina.ciumas@gmail.com