- 1Department of Molecular and Human Genetics, Baylor College of Medicine, Houston, TX, United States
- 2Jan and Dan Duncan Neurological Research Institute, Texas Children’s Hospital, Houston, TX, United States
- 3Department of Neuroscience, Baylor College of Medicine, Houston, TX, United States
- 4Medical Scientist Training Program, Baylor College of Medicine, Houston, TX, United States
Neural circuits and the cells that comprise them represent the functional units of the brain. Circuits relay and process sensory information, maintain homeostasis, drive behaviors, and facilitate cognitive functions such as learning and memory. Creating a functionally-precise map of the mammalian brain requires anatomically tracing neural circuits, monitoring their activity patterns, and manipulating their activity to infer function. Advancements in cell-type-specific genetic tools allow interrogation of neural circuits with increased precision. This review provides a broad overview of recombination-based and activity-driven genetic targeting approaches, contemporary viral tracing strategies, electrophysiological recording methods, newly developed calcium, and voltage indicators, and neurotransmitter/neuropeptide biosensors currently being used to investigate circuit architecture and function. Finally, it discusses methods for acute or chronic manipulation of neural activity, including genetically-targeted cellular ablation, optogenetics, chemogenetics, and over-expression of ion channels. With this ever-evolving genetic toolbox, scientists are continuing to probe neural circuits with increasing resolution, elucidating the structure and function of the incredibly complex mammalian brain.
Introduction
Neural circuits are the functional building blocks of the brain, comprised of the unique inputs and outputs of connected sets of neurons with particular functions. Nodes within circuits are the keystone checkpoints of neural circuits. In other words, nodes represent anatomically and functionally distinct ensembles of cells that integrate and process neural information from many different inputs to dictate the output of that circuit. These complex networks of connectivity are responsible for everything from processing sensory information (Lohse et al., 2020), attaching valence to stimuli that drive motivated behaviors (Reynolds and Berridge, 2002; Root et al., 2014; Al-Hasani et al., 2015), maintaining physiological homeostasis (Atasoy et al., 2012; Madden and Morrison, 2019), learning, and consolidating memories (Ruder et al., 2021; Sharpe et al., 2021).
Initially, studies of the brain relied on gross anatomy, defining large structures at relatively low resolution, without knowledge of cell type (Scoville and Milner, 1957). Coarsely targeted lesions using electrical current or chemicals allowed researchers to impart functional significance to these anatomically distinct brain regions (Foster et al., 2003; Lavond and Steinmetz, 2003). Early studies also used dyes and microscopy to identify and trace neurons, characterize their morphology, and identify connectivity patterns within discrete brain regions (Golgi, 1886). Electrophysiology was used to classify different neurons by their electrical signature, and to record their inputs and outputs (Piccolino, 1997). Additionally, early electron microscopy experiments provided subcellular resolution of synaptic structures, affording an unparalleled but somewhat myopic view of the brain (Gray, 1959). Although groundbreaking in their time, these applications were limited due to their lack of cell-type specificity, and the constraints of static analysis due to the need to harvest tissue.
One of the continued challenges in neural systems research is dissecting circuit function despite incredible interconnectivity. Many neural circuits have multiple, and often redundant, functions. Adding to this complexity, each node within a circuit has numerous inputs and outputs, as well as feedback and feedforward patterns of interconnectivity. For example, in early lesion studies, it was found that the hypothalamus appeared to affect appetite and body weight (Cushing, 1932; Brobeck, 1946; Brooks et al., 1946; Stellar, 1953; King, 2006). As this circuit was dissected further, it was revealed that two molecularly defined cell types (AgRP/NPY and POMC neurons) appeared to work in opposition to each other to drive either appetite or satiation, respectively (Luquet et al., 2005; Aponte et al., 2011; Zhan et al., 2013). Further studies uncovered that these cells express different receptors with different input and output targets and that POMC neurons are even inhibited locally by AgRP neurons (Sohn, 2014; Sternson and Atasoy, 2014; Wang et al., 2015). Currently, it is now appreciated that this circuit influences diverse behaviors outside the scope of feeding, including locomotion and foraging (Huang et al., 2013; Dietrich et al., 2015). Additionally, numerous non-hypothalamic nodes are sufficient and necessary for normal appetite and body weight control, including regions such as the basal forebrain, amygdala, and dorsal raphe nucleus (Campos et al., 2016; Herman et al., 2016; Kim J. et al., 2017; Patel et al., 2019; Bond et al., 2020; Bruschetta et al., 2020; Ye et al., 2022). This example highlights the complexity of the functional circuitry underlying behaviors such as feeding. As the questions regarding neural circuit structure and function become progressively sophisticated, the tools used to answer them must also evolve.
Fortunately, the toolbox for interrogating neural circuits has expanded rapidly. More precise intersectional genetic approaches have allowed interrogation of circuits with higher resolution and greater cell-type specificity. Additionally, it is now feasible to label groups of neurons based on activity patterns rather than just molecular markers, which may reveal more information about functional ensembles within neural circuits. This review will highlight cell-type-specific tools for targeting neural circuits, which enable more precise (1) mapping and tracing, (2) dynamic monitoring of neural circuit activity, and (3) targeted manipulation of neural circuits via loss- or gain-of-function strategies to infer function (Figure 1). Foremost, intersectional genetic tools and activity-dependent recombinases are used to target molecularly- or activity-defined neuronal populations. These genetic approaches are fundamental for performing any mapping, monitoring, or manipulation experiment with cell type-specificity. Secondly, with advancements in viral tracing strategies, it is now commonplace to map monosynaptic inputs and outputs of circuit nodes with cell type-specificity. This review will describe the contemporary approaches being used for such labeling strategies. Thirdly, it is critical to monitor neural circuit activity to better correlate function. Advancements in activity recording methods, such as genetically encoded calcium and voltage indicators and neurotransmitter sensors, provide new avenues toward understanding how neural circuits function in awake, behaving animals. Finally, causally interrogating neural circuit function via targeted activation or inhibition of select neurons within that circuit lends critical insight into function. Toward this, increasingly sophisticated tools allow investigators to activate and inhibit neural circuits with approaches that include genetically-targeted cell ablation, chemogenetics, and optogenetics. Neuroscientists still have much to learn about the brain, but with these advancements in the ability to map, monitor, and manipulate neural activity, the field of systems neuroscience is in the midst of an intellectual renaissance.
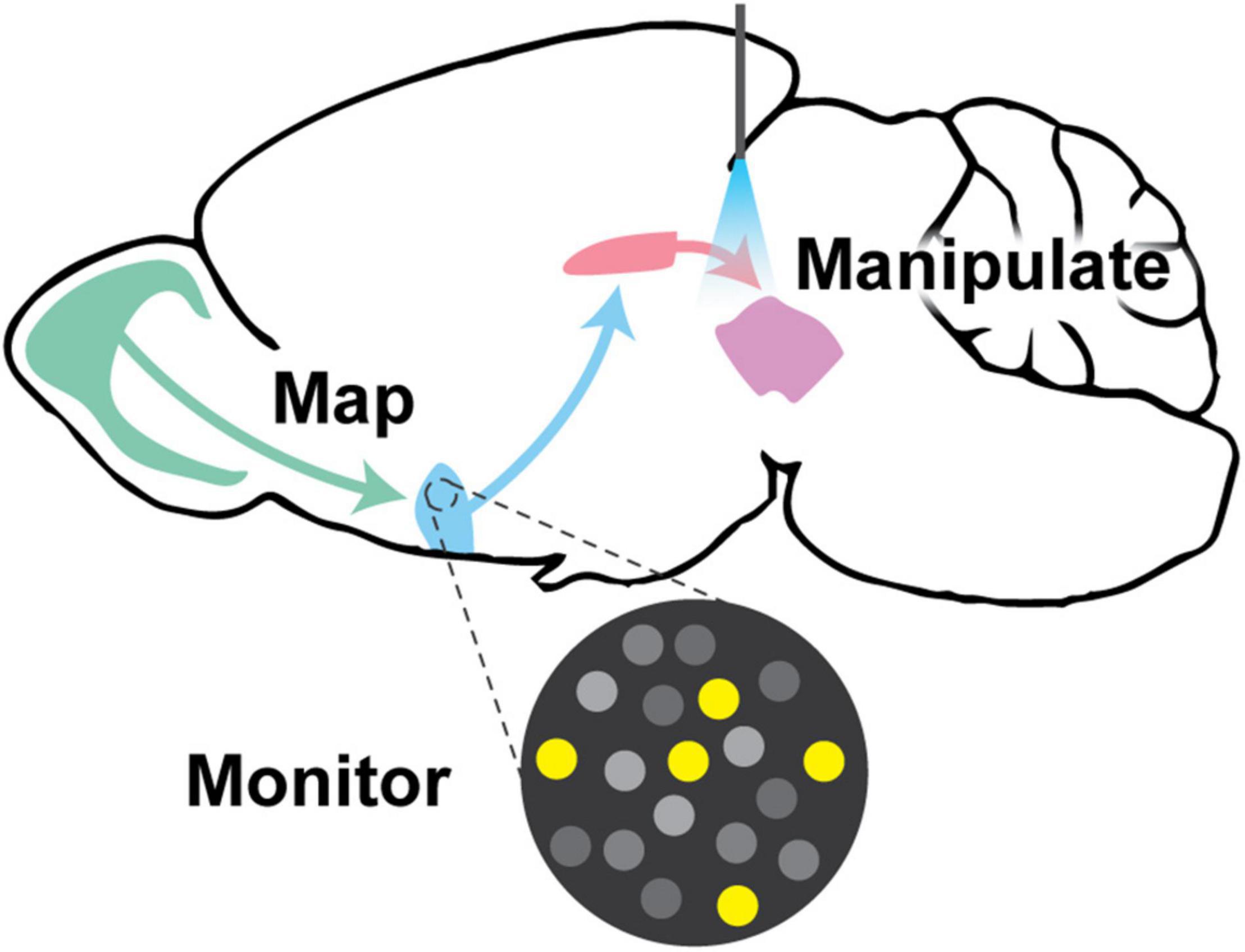
Figure 1. Visual abstract. This review discusses the paramount goals in neural circuitry research, which are to (1) map neural circuit connectivity using viral tracing approaches, (2) monitor circuit function using methods such as electrophysiology and genetically encoded indicator/voltage/sensor imaging, and (3) manipulate neural circuits to interrogate function via targeted ablation, expression of exogenous ion channels, chemogenetics, or optogenetics. All of these approaches require creative genetic tools to implement them in genetically-defined populations of neurons.
Genetic Approaches Toward Achieving Cell-Type Specificity: Site-Specific Recombination, Intersectional Genetics, and Activity-Based Targeting
Historically, brain circuits were investigated using tools that lacked cell type-specificity, such as lesions, pharmacology, and dyes. While this provided critical information about connectivity and function, we now know that each node in the brain is comprised of heterogeneous cell types, whose roles may be functionally distinct. Defining the neuronal constituents that contribute to circuit output is paramount to understanding brain architecture and function (McCulloch and Pitts, 1943). Thus, developing methods to label neurons in a molecularly- or activity-selective manner is imperative.
Site-Specific Recombination and Intersectional Genetics
Neuronal subtypes have been best defined and manipulated by their unique gene expression profiles (Colosimo et al., 2004; Gray et al., 2004; Baumgardt et al., 2007; Flames and Hobert, 2009; Winden et al., 2009). This has partially been made possible through the discovery and creative implementation of site-specific recombination (SSR). SSR affords the ability to selectively target neurons via controlled expression of Cre- and Flp-recombinases (Figure 2A; Dymecki, 1996; Dymecki and Tomasiewicz, 1998; Chai et al., 2000; Awatramani et al., 2003; Branda and Dymecki, 2004; Gong et al., 2007; Anastassiadis et al., 2009; Meinke et al., 2016). Such recombinases can be utilized to excise, invert, or conditionally express genes of interest via the “Lox-Stop-Lox” (LSL), “FRT-Stop-FRT” (Kuhlman and Huang, 2008), or “double-inverted orientation” [DIO; FLEx or FLEx (FRT)] methods (Atasoy et al., 2008; Schwarz et al., 2015). Of these, the DIO approach has proved extremely useful when paired with viral vectors to conditionally express genetic reporters or actuators in a region- or cell-type-specific manner. Additional recombinase/sequence pairs have recently been engineered to expand such approaches, including Dre/rox, VCre/VloxP, and SCre/SloxP (Anastassiadis et al., 2009; Suzuki and Nakayama, 2011). Similar to the Cre/lox system, these pairs can be used to delete, invert, and conditionally express genes of interest depending on the relative orientation of the rox, VloxP, or SloxP sites.
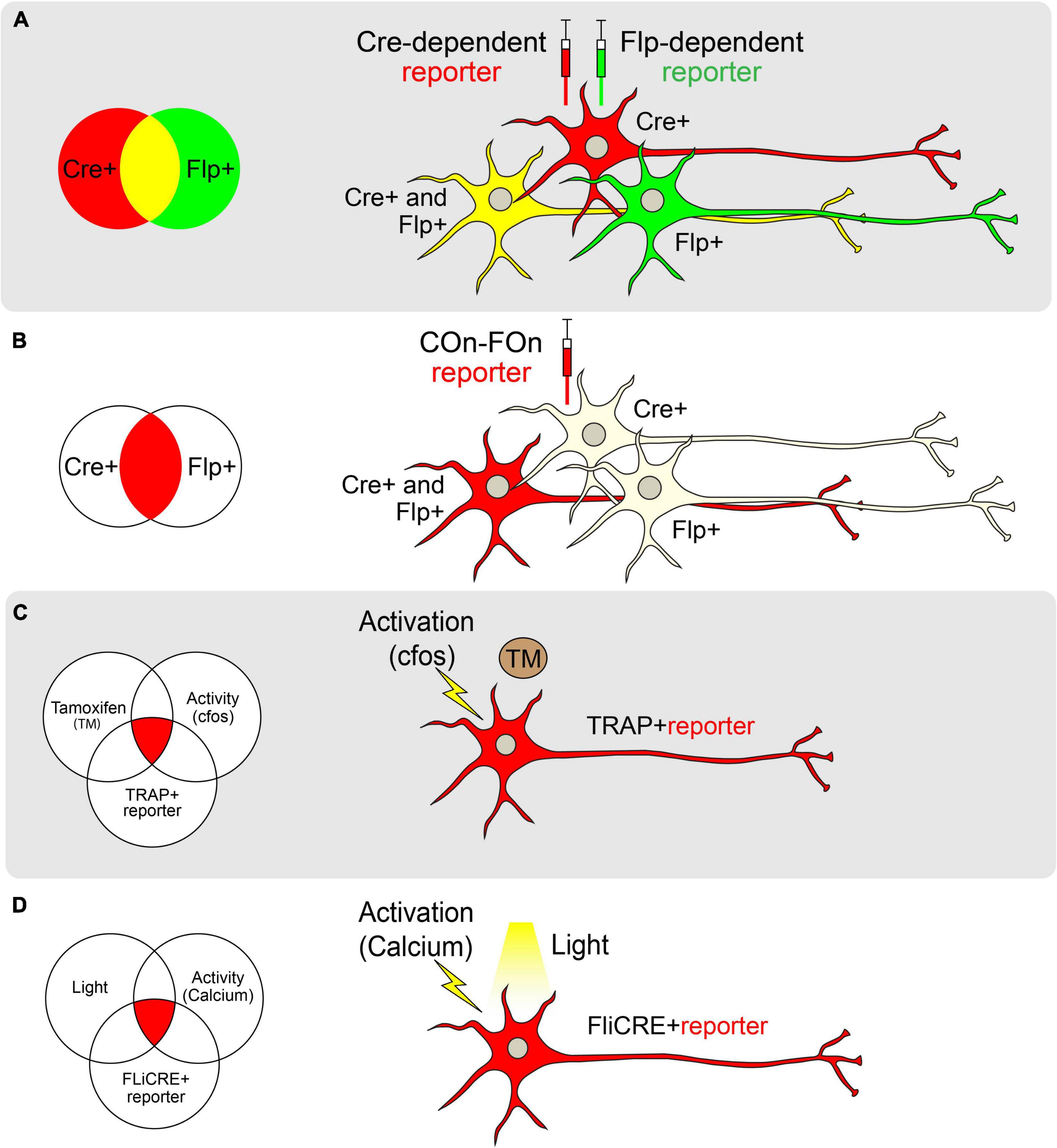
Figure 2. Genetic approaches toward achieving cell-type specificity. (A) Schematic of labeling neurons with different reporters using Cre (red) and Flp (green) recombinases separately. Cre and Flp-positive cells are visualized in yellow. (B) Schematic of labeling neurons with both Cre and Flp recombinases using the Con-Fon reporter, which is designed to be expressed only when both Cre and Flp recombinases exist. (C) Schematic of TRAP. The reporter of interest will be expressed only when Tamoxifen (TM) and neural activity (as indicated by cfos expression) exist in a TRAP + neuron. (D) Schematic of FLiCRE. When light and neuronal activity (as measured by an increase in calcium concentration) exist simultaneously, neurons will express the FLiCRE reporter of interest.
In cases where a neuronal population of interest lacks a single unifying molecular marker, multiple pairs of recombinases and sequences can be combined in an intersectional manner (Figure 2B). The Lox and FRT sites in these systems are oriented such that a gene will only be expressed in cells that express both Flp and Cre (for example, Frt-Stop-Frt followed by a FLEXed gene of interest; Dymecki et al., 2002; Awatramani et al., 2003). To reduce the possibility of leaky reporter expression due to stop codon read-through, an alternative intersectional strategy involves placing Lox and FRT sites at intronic sequences that divide a gene of interest, allowing for tighter control of gene expression. Moreover, intronically engineered sites and their adjacent exons can be oriented to facilitate genetic alterations only in cells that express both recombinases (ex. Cre-On, Flp-On or “COn-FOn”; Figure 2B), only one recombinase but not the other (ex. Cre-On/Flp-Off or Cre-Off/Flp-On), or in all cells except those that express both recombinases (ex. Cre-Off/Flp-Off; Fenno et al., 2014, 2020). Additionally, three-factor-dependent constructs have been generated (ex. Cre-On/Flp-On/VCre-On) to achieve even tighter genetic targeting control (Fenno et al., 2020). Such intersectional strategies also allow for the combination of spatially-restricted and cell type-specific drivers for genetic targeting. For example, using a retrogradely-transported Flp (Tervo et al., 2016) in combination with a cell type-specific Cre driver endows the ability to target cells that express a specific marker and project to a defined anatomical location [as in Rossi et al. (2021)].
While many experiments require the use of transgenic approaches to trace circuits or express actuators, engineering transgenic mice can be time-consuming, costly, and have limited experimental applications. The advent of viral genetics to express conditional genetic constructs (via the DIO or similar method) allows one to perform virtually any experiment in a cell type of interest if a Cre-driver is available (Haggerty et al., 2020; Nectow and Nestler, 2020). Viruses also provide better spatial control when targeting specific brain regions. Adeno-associated viruses (AAVs) are the most commonly used viruses in neuroscience. The simplicity of genomic structure, ease of packaging, and relatively non-pathogenic nature have made engineered AAVs ideal for genetic tracking, activity monitoring, and neuromodulation (Saleeba et al., 2019). Moreover, AAVs have selective tropism endowed by their capsid protein, which can be tailored for tissue of interest. For example, capsids such as AAV DJ/8, 2, and 9 are readily used for brain-specific expression (Haggerty et al., 2020; Nectow and Nestler, 2020). Due to the vast experimental possibilities brought about by viral genetics, teasing apart circuit function has been limited largely by the availability of recombinase-based genetic drivers that specify given cell populations. However, the advent of single-cell RNA sequencing is rapidly expanding to reveal potential gene candidates whose expression may serve as reliable and precise markers of different neuronal populations (Zeisel et al., 2015; Gokce et al., 2016; Chen et al., 2017b; Saunders et al., 2018; Tepe et al., 2018; Rossi et al., 2019; Hashikawa et al., 2020; Wallace et al., 2020). This novel information will provide a much larger repertoire of recombinase-expressing driver mice, a critical tool in the age of viral genetics.
Activity-Dependent Methods for Accessing Neuronal Ensembles
In some instances, single genes – or even sets of genes – are unable to adequately characterize the function of neuronal populations, which may operate as molecularly heterogeneous ensembles. Therefore, it is sometimes desirable to characterize neurons in an activity-dependent rather than a genetically-dependent manner. To this end, elegant methods have recently been developed to target neuronal populations based on their activity (DeNardo and Luo, 2017). These methods co-opt immediate-early genes such as Fos (Greenberg and Ziff, 1984) and Arc (Lyford et al., 1995) to drive recombinase expression in active neuronal populations.
Targeted Recombination in Active Populations (TRAP; Guenthner et al., 2013; DeNardo and Luo, 2017) is one such technology in which a tamoxifen-inducible Cre-ER(T2), has been inserted downstream of a Fos promoter. In the presence of tamoxifen, active cellular populations expressing Fos, and therefore Cre-ER(T2), permit translocation of Cre recombinase to the nucleus to facilitate the expression of a Cre-dependent reporter (Guenthner et al., 2013). Similar models exist that use Arc to drive Cre-ER(T2) expression (Guenthner et al., 2013; Figure 2C). A variation of this technology, termed the Tet-tag system, uses a tetracycline transactivator domain downstream of the Fos promoter (Reijmers et al., 2007). In this approach, tetracycline-controlled transactivator protein (tTA) binds to the tetracycline responsive promoter element (TRE) to drive gene expression. In the presence of tetracycline, tTA cannot bind the TRE, and target gene expression is dampened. To use this system, tetracycline must be continually supplied in chow. Upon cessation of tetracycline provision, active neurons produce tTA, allowing the transcription of downstream reporters in a temporally-precise and activity-dependent manner (Reijmers et al., 2007).
One of the major limitations of both the TRAP and Tet-tag systems is the potential for the temporal lag in accurately capturing real-time neuronal activation. Since TRAP relies on the systemic administration of tamoxifen (or similar analogs), active neurons may be labeled throughout a 24-h time window. Similarly, due to the slow time course of tTA activation following the removal of doxycycline, the Tet-Off system also captures cells over extended times (Reijmers et al., 2007; Guenthner et al., 2013). Thus, more temporally precise labeling methods have been sought-after. One such method called “CANE” (capturing neuronal ensembles) utilizes a transgenic mouse expressing destabilized avian-specific receptor TVA under the control of the Fos promoter (Fos-2A-dsTVA; Sakurai et al., 2016). The dsTVA is fused with a degradation signal so that TVA closely mirrors Fos expression patterns. Upon delivery of pseudotyped lentivirus or rabies virus, neurons actively expressing Fos may be tagged for tracing or manipulation experiments (Sakurai et al., 2016). Other methods that tag activated cells based on calcium signals rather than immediate early gene expression and drug delivery have been shown to be even more temporally constrained. For example, CaMPARI, a photoconvertible genetically encoded calcium indicator, labels neurons only when both calcium levels are high and light is delivered to cells, identifying activated neurons on the millisecond timescale with spatial precision (Fosque et al., 2015). Similarly, FLiCRE (Fast Light and Calcium-Regulated Expression) is a method that labels activated cells at the transcriptional level (Kim et al., 2020; Figure 2D). It not only tags activated cells following a calcium influx but also enables the direct manipulation of the tagged cells via the concomitant light-induced expression of Cre. Collectively, these novel activity-driven targeting approaches allow researchers to screen for neural circuits underlying behaviors of interest. This is useful when a molecular marker for a region/behavior is not known, or when more than one molecular marker may contribute to a particular behavior.
Mapping Neural Circuits: Viral and Multiplex Neural Tracing Strategies to Dissect Circuit Anatomy
One of the primary objectives in neural circuit research is to create maps that label neurons within a node, as well as their inputs and outputs. These maps are a critical first step toward understanding circuit function, providing information on cellular morphology, and ultimately, informing the creation of a brain-wide connectome. Initially, circuits were traced in a non-cell type-specific manner with small organic compounds or dyes. These conventional tracers – including lectins (PHA-L; Gerfen and Sawchenko, 1984) and dextran-amines (DAs; Gimlich and Braun, 1985) allowed researchers to label the neuronal membrane, visualizing the soma along with its axonal projections. Other compounds were discovered to label a neuron in a retrograde fashion, being taken up by axon terminals and transported to the soma of a neuron. Such compounds included Horseradish peroxidase (HRP; Kristensson and Olsson, 1971; Kristensson, 1977), Cholera toxin subunit B (CTB; Stoeckel et al., 1977), hydroxystilbamidine (FluoroGold™; Köbbert et al., 2000), and retrobeads (Katz et al., 1984; Katz and Larovici, 1990). Although conventional tracers robustly label neurons and provide glimpses into their complex morphology, they are not sufficient to reveal patterns of connectivity between molecularly defined cell types. As a result, viruses – which enable cell type-specificity and transsynaptic labeling – have largely replaced many conventional tracing methods.
Viral Tracers
Viruses provide many advantages over conventional tracers. Foremost, viral tracers may be engineered as conditional vectors compatible with diverse genetic markers, permitting cell type-specificity. Second, diverse promoters with varying strengths can be used to modulate expression levels or drive cell type-specificity. Lastly, viruses can be engineered to cross single synapses (Kuypers and Ugolini, 1990; Xu et al., 2020). Delivery of viral vectors using stereotaxic injections affords both temporal and spatial specificity, while also obviating the need to generate transgenic mouse models for genetic labeling experiments, so long as the recombinase driver of choice already exists. The following will give an overview of viruses used for both retrograde and anterograde labeling of neural circuits (Table 1).
Retrograde Viral Tracers
Retrograde viral tracers label inputs into designated populations of neurons (Figure 3A). HSV-1 was one of the first neurotropic viruses used to transfer genes into specific brain regions via stereotaxic injection (Carlezon et al., 1997; Lilley et al., 2001). While favoring retrograde transport, its ability to travel both anterograde and across the synapse rendered this virus limited in its utility (Ugolini, 2010). Thus, viruses that exclusively travel either retrogradely or anterogradely were sought. This led to the adoption of Pseudorabies (PRV; a misnomer since the virus actually belongs to the herpes virus family) and Rabies virus (RV; Martin and Dolivo, 1983; Ugolini, 1995; Enquist, 2002).

Figure 3. Retrograde viral tracing strategies. (A) Non-transsynaptic retrograde tracing using retro-AAV or CAV-2. (B) Transsynaptic retrograde tracing using pseudotyped rabies virus is used to trace monosynaptic connections. Rabies G (RB) is provided in trans and neurons are pseudotyped with TVA receptor to endow cell-type specificity. Once a neuron expressing TVA and RG is infected with RABV expressing EnvA, the RABV may jump across one synapse in a retrograde manner.
Since their discovery, PRV and RV have been widely used to infect the CNS of rodents and non-human primates for retrograde, transsynaptic labeling (Kuypers and Ugolini, 1990; Xu et al., 2020). However, results from these initial experiments proved challenging to interpret, as monosynaptic versus polysynaptic inputs were not discernable. Additionally, viral toxicity caused cell death. To address this problem, pseudotyped RV (RABV) was engineered to label the monosynaptic inputs of genetically and anatomically defined populations (Wickersham et al., 2007). This engineered RABV has a deletion of the endogenous envelope glycoprotein (RG), which is required for viral assembly and transsynaptic transport. By selectively supplying RG in trans to source-cells, RABV can replicate and cross one synapse, but no further. Furthermore, pseudotyping RABV with the avian leucosis virus coat protein EnvA restricts RABV to infect neurons that express the cognate TVA receptor, endowing greater control with cell type-specificity (Etessami et al., 2000; Arenkiel, 2011; Figure 3B). Using this approach, it is possible to trace different cell types within the same brain region, quantifying and comparing mapped inputs between targeted source cell types (Do et al., 2016).
Other types of viruses are also capable of revealing monosynaptic inputs to a node of interest. These retrograde viral tracers include canine adenovirus and modified capsids of AAV. Unlike the rabies virus, these viruses are not transsynaptic. Canine adenovirus type 2 (CAV-2) has high neuronal specificity and selectively infects axon terminals of neurons instead of the soma and dendrites, which makes it a powerful tool for mapping inputs to various brain regions (Soudais et al., 2001; Lavoie and Liu, 2020). For example, the use of CAV-2-Cre in conjunction with Cre-dependent fluorescent reporters allows for efficient mapping to targeted neuronal populations (as in Herman et al., 2016). Also, recent advances in AAV capsid engineering endow directionality-specific transduction. Of these, the rAAV2-retro capsid drives retrograde transport of AAV similar to CAV-2, being taken up by synaptic terminals and transported to the soma of the transduced neuron (Tervo et al., 2016). However, one caveat to both retro-AAVs and CAVs is that they may exhibit selective tropisms. For example, some studies have shown that CAV-2 more efficiently transduces certain neuronal populations compared to retro-AAV (Kakava-Georgiadou et al., 2019), while others have shown that retro-AAV is more efficient than CAV-2 for other neuronal populations (Tervo et al., 2016). Thus, potential biases in tropism may somewhat limit the utility of these viruses in certain brain regions or for targeting different cell types.
Anterograde Viral Tracers
In addition to mapping the inputs, it is also critical to map circuit node outputs, informing the next step in the wiring diagram. Many fluorescent markers labeling either the cytosol or neuronal membrane will label axon terminals, however, it may be difficult to differentiate axon terminals from fibers of passage using these markers (Figure 4A; Xu et al., 2020). A better way to perform anterograde viral tracing is to tag synaptic vesicle proteins with fluorescent markers. For example, synaptophysin::GFP selectively labels presynaptic axon terminals (Li et al., 2010). By conditionally expressing these fusion proteins, one may trace projections in a cell-type-specific manner (Figure 4B). However, close appositions visualized from synaptophysin tracing do not necessarily demonstrate functional synapses (Saleeba et al., 2019). Thus, functional connectivity studies must be performed to validate these projections, as described later in the review. Alternatively, GFP Reconstitution Across Synaptic Partners (GRASP) is a method to visualize anatomically-defined synaptic connections (Feinberg et al., 2008). GRASP relies on the targeted expression of complementary GFP fragments tethered to plasma membrane carrier proteins to detect the proximity of two cells. When two cells form functional synapses, split-GFP fragments reconstitute a functional, fluorescent GFP molecule at the point of contact, labeling the synapse (Feinberg et al., 2008; Kim et al., 2011). For example, when AAV-expressing pre-GRASP was targeted to the auditory cortex and post-GRASP to the inferior colliculus, it was revealed that these two regions are synaptically connected, as indicated by GFP puncta at the point of contact (Song et al., 2018).
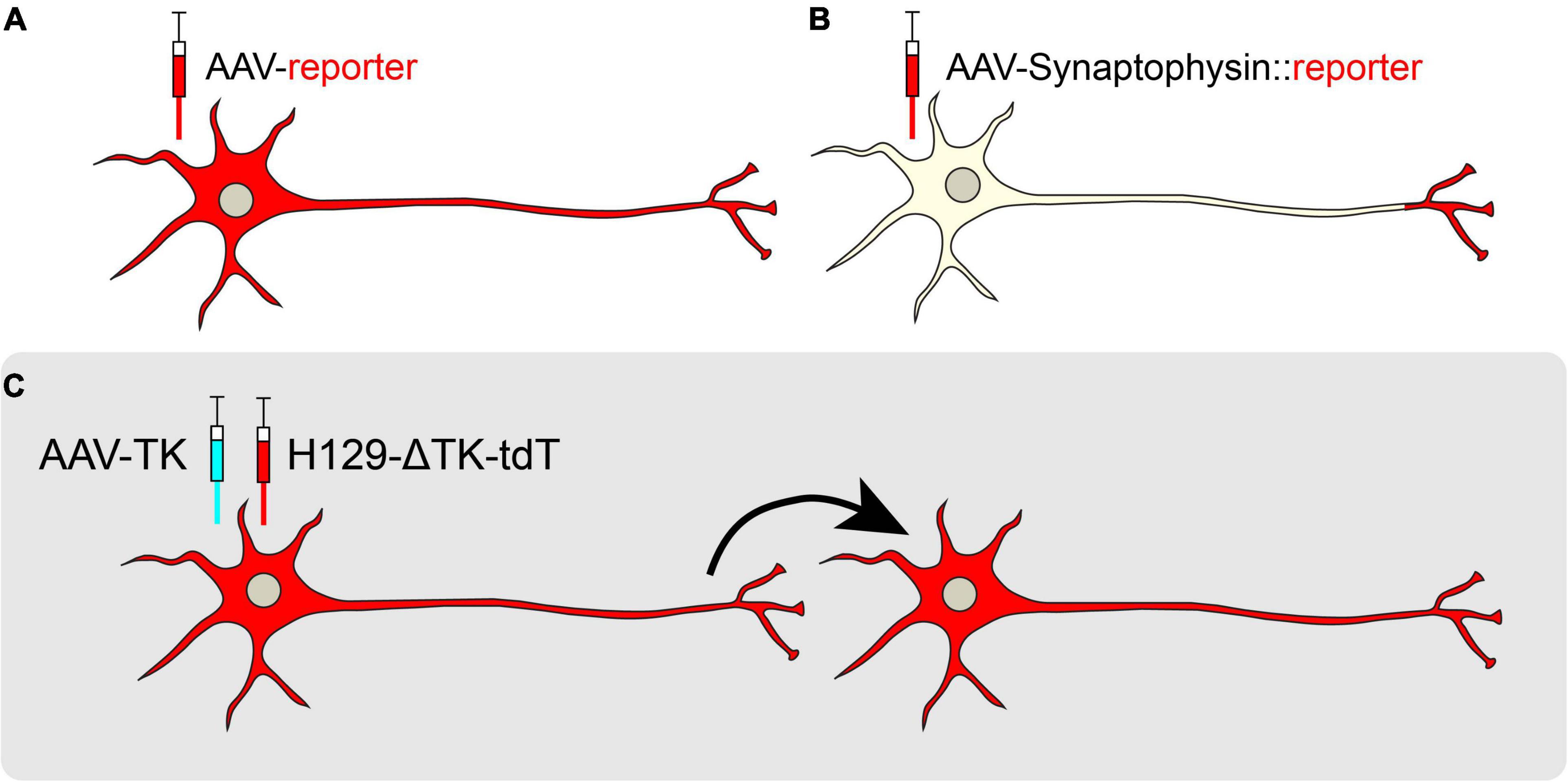
Figure 4. Anterograde viral tracing strategies. (A) Non-transsynaptic anterograde tracing using expression of a cytosolic or membrane-bound fluorescent reporter. (B) Non-transsynaptic anterograde tracing using expression of Synaptophysin fused to a fluorescent reporter. (C) Transsynaptic, monosynaptic tracing using the H129-ΔTK-tdT system. When TK is provided in trans, the H129 reporter virus may jump across one synapse in an anterograde manner.
Several other tools exist for transsynaptic anterograde mapping. Although most of the HSV-1 families are known to spread selectively in the retrograde direction, the H129 strain moves in the anterograde direction, crossing synapses to downstream target cells (Dix et al., 1983; Zemanick et al., 1991; Wojaczynski et al., 2015). Recently, a modified version of H129, H129-ΔTK-tdT, was developed for monosynaptic anterograde tracing. This genetically modified H129 virus has a deletion in the thymidine kinase (TK) gene that is replaced by a tdTomato reporter. Without TK, the H129 virus cannot replicate in neurons, but supplementation of TK from a helper virus allows H129-ΔTK-tdT to spread polysynaptically, labeling post-synaptic target neurons (Lo and Anderson, 2011; Zeng et al., 2017; Figure 4C). In a recent study, AAV-TK-GFP was stereotaxically targeted to the posteromedial thalamic nucleus (VPM) of wild-type mice, and H129-ΔTK-tdT was delivered 21 days later. Post-synaptic tdTomato was observed in cortical layer IV of the visual cortex (V1), revealing a novel connection from the VPM to V1 (Zeng et al., 2017). A polysynaptic version of H129 (H129-G4) has binary tandemly-connected GFP cassettes inserted into the H129 genome, allowing this variant to move anterogradely in a polysynaptic manner with robust labeling capable of revealing intricate neuronal morphologies (Zeng et al., 2017). However, since H129-G4 demonstrates polysynaptic spread, it may be difficult to discern the direct outputs of a particular node. Finally, capsid engineering has also produced AAVs capable of transsynaptic anterograde tracing. AAV1 is a novel capsid that endows preferential transport to postsynaptic neurons (Zingg et al., 2020). However, while AAV1 transsynaptically spreads in both glutamatergic and GABAergic neurons, it does not spread to neuromodulatory cell types, limiting its utility (Zingg et al., 2020).
Multiplexed Mapping Methods
Mapping neural circuits using fluorescent reporters combined with confocal microscopy remains a powerful method for navigating the brain. However, to identify connectivity with single-cell resolution, multiplexed mapping and sequencing approaches have evolved (Figure 5). For example, MAPseq (multiplexed analysis of projections by sequencing) and BRICseq (brain-wide individual animal connectome sequencing) are capable of surveying thousands of neurons in a single experiment (Kebschull et al., 2016; Han et al., 2018; Huang et al., 2020). These approaches utilize the Recombinant Sindbis virus, which rapidly achieves very high expression levels, to express unique mRNA barcodes in individual source cells. These barcodes spread throughout each source cell, including its axonal projections. Both the source area and downstream projections are then micro-dissected, dissociated, and sequenced. After sequencing, barcodes from projection areas are matched to the source area to reveal singe-cell projection patterns (Sun et al., 2021; Figures 5A,B). Recently, Gergues et al. (2020) used MAPseq to map projections from the ventral hippocampus (vHPC). They labeled vHPC neurons with a library of random RNA barcodes, examined 7 different target brain regions, and differentiated projection-specific populations of vHPC neurons (Gergues et al., 2020). One caveat with MAPseq/BRICseq is that the precise positions of individual cell bodies are lost due to tissue dissociation. To overcome this limitation, BARseq (barcoded anatomy resolved by sequencing), combines MAPseq with in situ sequencing to maintain cellular spatial localization (Chen et al., 2018, 2019; Figure 5C). BARseq has been applied to map projections from the mouse auditory cortex to 11 areas of the whole brain, confirming the laminar organization of the three top classes of projection neurons in the auditory cortex (Chen et al., 2019).
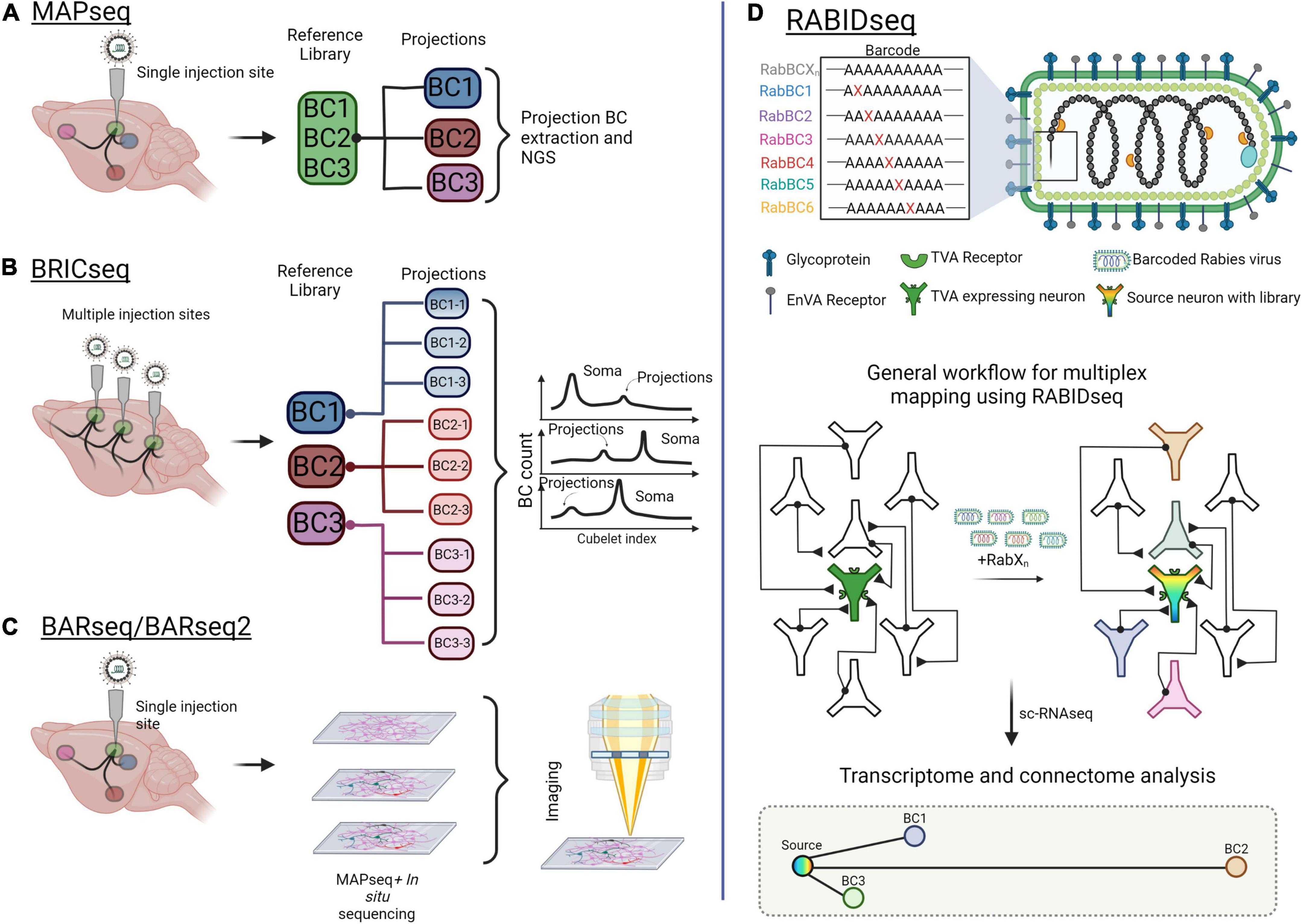
Figure 5. Mapping brain-wide connections with multiplexed, barcoded viral tools. (A) Multiplexed Analysis of Projections by Sequencing (MAPseq) uses barcoded viruses to identify projections from a single injection site. Barcodes are extracted from the injection site and from axons at the projections. (B) Brain-wide individual animal connectome (BRICseq) uses the same barcoded viral library strategy as MAPseq but for multiple sites in the brain. Different “zipcodes” are designated to a spatial location within the brain and small dissections of tissue are removed for analysis. Soma and projections are then determined by the total makeup of barcodes in the projections compared to soma. (C) Barcoded anatomy resolved by sequencing (BARseq) is a technique that combines MAPseq and in situ sequencing to maintain the physical position of neurons and their axons within the brain. (D) Rabies barcode interaction detection followed by sequencing (RABIDseq) uses rabies virus and sequencing to gather transcriptome data on mono-transsynaptic partners. Created with Biorender.com.
Other multiplexed mapping methods identify inputs through retrograde transport of barcodes. One such approach is Connect-seq, which determines the molecular identities of individual upstream neurons in a defined circuit by combining retrograde viral tracing and single-cell transcriptomics (Hanchate et al., 2020). Implementing this strategy, Hanchate et al., successfully targeted Cre-dependent PRV infection to corticotropin-releasing hormone neurons within the paraventricular nucleus of the hypothalamus (PVN). Flow cytometry was then used to isolate the upstream inputs, and single-cell RNA-sequencing (scRNA-seq) was used to determine the identities of these inputs (Hanchate et al., 2020). In a similar way, RABID-Seq utilizes pseudotyped rabies virus to label and deliver barcodes to presynaptic inputs in a cell type-specific manner, which may then be identified through scRNA-seq (Clark et al., 2021). Toward this, RABID-Seq has been used to identify axon guidance molecules as mediators of microglia-astrocyte interactions that promote CNS pathologies (Clark et al., 2021; Figure 5D).
In summary, these multiplexed input and output mapping approaches provide unprecedented resolution in the creation of accurate wiring diagrams, providing transcriptomic analyses that are lacking in other contemporary viral tracing approaches.
Clearing Methods for Whole-Brain Neural Circuit Mapping
As neural tracing strategies have evolved, so too have the microscopes and imaging technologies necessary to visualize them. A major obstacle to visualizing deep brain tissue is signal distortion due to light scattering and spherical aberrations. Consequently, imaging long-range projections were effectively impossible. To mitigate these challenges, emerging optical techniques such as CUBIC (Susaki et al., 2014; Murakami et al., 2018; Matsumoto et al., 2019), CLARITY (Chung et al., 2013; Tomer et al., 2014), ScaleS (Hama et al., 2011, 2015), and SeeDB (see deep brain; Ke et al., 2016) “clear” brain tissue. By removing lipids in whole-brain tissue while leaving proteins and nucleic acids intact, tissue effectively becomes transparent, allowing one to image three-dimensional brain architecture or brain-wide neural projections with minimal light scattering. When used in combination with light-sheet microscopy or similar imaging platforms, intricate circuit networks may be visualized in three dimensions, across the entire brain (Newmaster et al., 2021).
Monitoring Neural Circuits: Measuring Circuit Dynamics Via Electrophysiology and Genetically-Encoded Imaging Techniques
Once a circuit has been mapped, observing and quantifying circuit activity is necessary to better understand its function. Monitoring circuit activity using both traditional ex vivo and more recent in vivo methodologies provides information on circuit connectivity and action potential dynamics and correlates circuit function with a particular behavior, sensory perception, or cognitive task. One of the first methods for monitoring circuit activity was recording the electrical activity in the nerve tissue of a dissected frog leg Galvani (Piccolino, 1998). This discovery led to the birth of electrophysiology – the study of the intrinsic electrical properties of cells (Rubaiy, 2017). Electrophysiological techniques record activity dynamics with single-cell resolution, but they are relatively low-throughput in nature. Additionally, while neuron firing patterns can be used to classify certain cell types post hoc, these methods overall lack cell type-specificity. Recent advances have increased the throughput and cell type-specificity of electrical recordings. Furthermore, optical approaches, including genetically-encoded calcium/voltage indicators and neurotransmitter/neuropeptide sensors, have gained incredible momentum.
Electrophysiology
Classical electrophysiology centers around the “patch-clamp” technique, which creates a tight seal on a cell’s membrane using a glass electrode or micropipette to record membrane voltage or current (Neher and Sakmann, 1976). Patch clamping has remained the gold standard of electrophysiology for decades, allowing scientists to measure the properties of ion flow across the membrane and investigate the types of ion channels that regulate the electrical activity of cells. However, recent advancements in patch-clamp configuration allow increased flexibility and greater throughput recordings including cell-attached, inside-out, outside-out, perforated patch, and notably, the whole-cell configuration (Hill and Stephens, 2021).
In an increasingly multimodal research world, electrophysiology has adapted to interface with other sophisticated techniques, allowing users to measure multiple dimensions of neural activity. For example, patch-clamp electrophysiology has been combined with RNA sequencing in a technique known as Patch-Seq. Patch-Seq permits the collection of transcriptomic data after performing whole-cell electrophysiology recordings, as patched cells are drawn into a glass capillary and used for single-cell RNA sequencing (Bardy et al., 2016; Cadwell et al., 2016; Chen et al., 2016; Földy et al., 2016; Fuzik et al., 2016; Lipovsek et al., 2021). Using Patch-Seq, both neuronal activity and gene expression patterns are revealed with single-cell resolution. Indeed, this technique has been used to describe the diversity of neuronal subtypes within the mouse motor cortex, as well as to provide insights into the diversity of neuronal populations in the dorsal striatum (Muñoz-Manchado et al., 2018; Gouwens et al., 2020; Scala et al., 2021).
While recording the electrical properties of single cells has been critical for understanding the basic properties of individual neurons, interrogating the activity of interconnected circuits requires recording from multiple cells simultaneously. Toward this, extracellular recordings using wire or silicon-based electrodes measure action potentials in multiple parallel neurons. By using multiple geometrically arranged electrodes, or multielectrode arrays (MEAs), it is now possible to record many neurons simultaneously from one probe and analyze their summed electrical activity, or local field potentials (LFPs; Buzsáki, 2004; Einevoll et al., 2013). The past decade has seen the rise of increasingly powerful MEAs such as Neuropixels, a silicon-based probe with up to thousands of independent channels for recording hundreds of neurons simultaneously (Jun et al., 2017). With the advent of these new high-throughput recording methods, it is now possible to interrogate the electrical and synaptic properties of circuits with unprecedented spatiotemporal resolution (Csicsvari et al., 2003; Shobe et al., 2015; Jun et al., 2017).
Genetically-Encoded Calcium Indicators
Electrophysiology is optimized to measure the activity of neurons with high temporal precision but is limited in its ability to spatially localize the cells being recorded, or to identify their molecular cell type. With the advent of real-time neural activity imaging, activity-dependent fluorescent reporters have been developed to measure circuit dynamics with high spatial resolution in living brain tissue. Genetically Encoded Calcium Indicators (GECIs) are the most widely used activity-dependent fluorescent reporters. GECIs are proteins that bind cytosolic calcium and exhibit increased fluorescence with calcium influx, a proxy for neuronal firing, thereby translating neural activity into spatially resolved optical signals (Bootman and Berridge, 1995; Miyawaki et al., 1997; Oh et al., 2019). Since fluorescent activity reporters are genetically encoded, their expression can be targeted to specific populations through conditional viral or transgenic methods (Pérez Koldenkova and Nagai, 2013). While the temporal dynamics are less precise than electrophysiological techniques, advancements in imaging resolution and GECI sensitivity enable monitoring of diverse firing dynamics at high spatiotemporal resolution. Importantly, such imaging approaches facilitate the investigation of firing and anatomical properties of neuronal circuits from diverse brain structures, from the cortex to deep brain nuclei (Hoogland et al., 2009).
GCaMP reporters are currently one of the most widely used families of GECIs (Table 2). Structurally, GCaMP is a fusion protein between GFP, calmodulin (a calcium-binding protein), and the light chain myosin protein, which was rationally designed to facilitate increased GFP fluorescence with the influx and binding of free calcium (Shimomura et al., 1962; Porumb et al., 1994; Tallini et al., 2006; Akerboom et al., 2009). Additional improvements to GECI technology have been made via large-scale random mutagenesis (GECOs; Table 3), or semi-rational mutation strategies (GCaMP5 family) to identify indicators with stronger fluorescence intensity, varied activity kinetics (GCaMP6s-f), and greater dynamic ranges (Zhao et al., 2011; Akerboom et al., 2012; Helassa et al., 2015). Notably, GCaMP6 and GCaMP8 are widely used for their fast kinetics, stability, and high signal intensity. GCaMP8 has a higher signal intensity than GCaMP6, making it well suited for experiments to monitor population activity signals via imaging of the soma, while GCaMP6 is preferred for visualizing activity at dendritic spines (Ohkura et al., 2012a).
In parallel with the continued development of diverse fluorescent indicator proteins, imaging technologies have also advanced. Classical GECI recordings were often made in superficial brain areas due to the relative ease of access for optical observation. The development of miniaturized fiber optic-based photometry has facilitated studying deep-brain structures in awake, behaving animals in real-time (Helmchen et al., 2001; Grewe et al., 2010; Lütcke et al., 2010). However, fiber photometry measures bulk calcium dynamics, lacking cellular resolution. Now, experiments using implantable gradient refractive index (GRIN) lenses combined with mini-microscopes or 2-photon interfaces permit real-time dynamic recordings from individual cells deep within the brain (Helmchen et al., 2001; Barretto et al., 2009; Ghosh et al., 2011; Zong et al., 2017; Zhang et al., 2019). GECIs represent a powerful tool for monitoring the activity of neural circuits in a cell type-specific manner. This highly optimized and titratable system has revolutionized the field of systems neuroscience, allowing for bulk activity recordings and spike extractions from big data sets such as the Machine Intelligence from Cortical Networks (MICrONS) data set, which contains large scale tracing and functional connectivity data obtained through electron microscopy (Zhou et al., 2020). The combination of highly optimized calcium indicators, genetic targeting technology, sophisticated imaging techniques, and big data analysis through machine learning approaches have opened the door for an in-depth, mechanistic understanding of circuit dynamics with unprecedented precision.
Genetically-Encoded Voltage Indicators
While GECIs remains an invaluable tool to study cell-type-specific circuit dynamics, due to their dependence on calcium activity they represent an indirect measure of cell activity. The development of genetically encoded voltage indicators (GEVIs) directly measures neuronal activity, as indicated by neuronal membrane potential, in a more temporally precise manner than calcium indicators (Table 4). Although the advent of GEVIs predates GECIs until recently, circuit monitoring via GEVIs has suffered from limitations in imaging technologies and available voltage indicators. Advancements in image processing and GEVI development have paved the way for the visualization of voltage dynamics in genetically defined circuits (Chamberland et al., 2017). The first GEVIs were designed as fusion proteins between GFP and voltage-sensing domains (VSDs) like those found in voltage-gated ion channels (Siegel and Isacoff, 1997; Sakai et al., 2001; Dimitrov et al., 2007). Within the class of VSD-based GEVIs, two main families of indicators have been categorized by the number of fluorescent proteins fused to the VSD: FRET GEVIs (also known as ratiometric GEVIs) and single fluorescent protein (monochromatic) GEVIs.
Ratiometric GEVIs use voltage-dependent Förster resonance energy transfer (FRET) to modify the conformation of the VSD, in turn changing the conformation of two fluorescent proteins such that the readout is an anticorrelated switch from fluorescence in one color to another. FRET GEVIs are particularly useful for circuit monitoring applications in vivo, where the anticorrelated color-switching can be parsed out from hemodynamic correlated changes in fluorescents within the same color (Akemann et al., 2012, 2010). Monochromatic GEVIs, on the other hand, contain a single fluorescent protein that emits a single wavelength of light, best suited for cell culture or slice preparation experiments. The advantage of this kind of GEVI is that the optical imaging setup required for measuring its activity is less complex than that of the ratiometric GEVI. However, given that there is only a single wavelength emitted, performing in vivo imaging requires more complex corrective methods to account for hemodynamic changes and/or a multiplexed approach (Borden et al., 2017).
One of the major limitations of VSD-based GEVIs is the weak signal-to-noise ratio. Thus, a second major class of GEVIs was developed based on naturally occurring microbial opsins. These GEVIs take advantage of the native fluorescence of these opsins, but also amplify this signal by binding them to brighter fluorescent proteins, thus reducing the effect of photobleaching that is seen in other GEVIs (Isobe et al., 1983; Adam et al., 2019; Piatkevich et al., 2019). For example, FRET-opsins have been developed that couple rhodopsin to another fluorescent protein, such that the fast dynamics of the opsin can be coupled to the brightness of the additional fluorescent protein for better signal-to-noise ratios. Fluorescent proteins such as yellow mCitrine, the bright green mNeonGreen, or the orange mRuby3 and mScarlet, have been used to generate MacQ-mCitrine, Ace-mNeonGreen, VARNAM, and Ace-mScarlet, respectively (Gong et al., 2014, 2015; Gong, 2015; Kannan et al., 2018; Beck and Gong, 2019; Mollinedo-Gajate et al., 2021). These opsin-based GEVIs have been leveraged in the study of circuit dynamics in cell culture, slice preparations, and in vivo experiments within cerebellar Purkinje cells (Gong, 2015).
Förster resonance energy transfer-opsins represent an advancement in the design of GEVIs to overcome signal-to-noise limitations. However, due to a greater molecular size, they are susceptible to forming intracellular aggregates that impede their localization to the cell plasma membrane (Hochbaum et al., 2014; Kannan et al., 2019). To circumvent this limitation, a new class of chemigenetic hybrid GEVIs has been developed that consists of two components – the first which targets the indicator to a cell type of interest, while the fluorescent protein is separately delivered to the target tissue, often intravenously (Sundukova et al., 2019). Within the class of chemigenetic GEVIs, three main families of indicators exist: Opsin-Dye FRET chemigenetic indicators, fluorescent protein-dye FRET hybrid indicators, and indicators that use photo-induced electron transfer. The two most promising opsin-dye FRET chemigenetic indicators are Voltron and Positron – both of which use a microbial opsin fused to a self-labeling protein tag that allows for the binding of a fluorescent protein (Grimm et al., 2015; Abdelfattah et al., 2019, Abdelfattah et al., 2020). Fluorescent protein-dye FRET indicators use an exogenous chromophore coupled to a fluorescent protein, where the chromophore is used to quench fluorescence based on cell membrane voltage (Chanda et al., 2005; Wang et al., 2010). Lastly, photo-induced electron transfer GEVIs work using a voltage-sensitive fluorescent protein-tagged to the SpyTag protein which covalently binds to the SpyCatcher membrane protein in the cell (Grenier et al., 2019). Voltage sensing is achieved by photo-induced electron transfer through a synthetic wire in the membrane that reversibly quenches the activity of the fluorescent protein (Mollinedo-Gajate et al., 2021). The ability to target GEVI expression with cell-type specificity and improved membrane localization allows for greater reliability and precision in the quest to measure circuit dynamics.
Although fluorescent protein-based GEVIs represent a powerful way to measure neural activity, these indicators are limited in their photostability and potential for toxicity at high expression levels. A class of bioluminescent GEVIs has recently been developed that overcomes these limitations (Inagaki et al., 2019, 2017). Bioluminescent GEVIs provide cells with a substrate that can be converted into a fluorescent reporter based on changes in membrane potential. Traditional bioluminescent GEVIs combine a VSD, luciferase, and a fluorescent protein in a similar configuration to FRET-based GEVIs, whereby voltage changes produce a FRET-based anticorrelated signal between the luciferase and fluorescent reporter. While the anticorrelated design allows for better signal-to-noise ratios, it is limited by the requirement of the exogenous substrate, luciferin. To overcome this limitation, recent advancements have been made such that the bioluminescent GEVI self-generates an energy-rich substrate for light-emitting activity (Srinivasan et al., 2019; Mollinedo-Gajate et al., 2021).
While voltage indicator technology has advanced greatly in recent years, continued improvements to their photostability, signal intensity, and power of imaging platforms are required in order to more readily compete with calcium indicators (Yang and St-Pierre, 2016). However, new and improved GEVIs are continually being engineered to address these concerns, providing direct monitoring of electrical activity in living cells. Despite the high signal intensity provided by GECIs, this tool can only provide binary information on whether a neuron is active, lacking the temporal dynamics required to accurately determine neuronal firing patterns. Additionally, GECIs rely on indirect measures of neuronal activity via calcium. In contrast, GEVIs provide the necessary kinetics to accurately portray complex firing patterns, and even signal propagation throughout an individual neuron, but lack the appropriate signal intensity to generate high-quality data. A paradigm shift in the use of GECIs versus GEVIs may come in the future, but this requires continued advancements in both imaging techniques and protein engineering to improve GEVIs.
Neurotransmitter and Neuropeptide Sensors
Electrophysiology, GECIs, and GEVIs reveal detailed neuronal activity, and with the power of viral and transgenic targeting technologies, interrogate circuit dynamics with cell-type specificity. However, these techniques are limited in their ability to measure cell-cell communication dynamics at the synapse. To study the real-time communications between active neurons, novel protein-based sensors that bind to and report the release of neurotransmitters have emerged, providing an unprecedented view of the dynamics of communication between neurons (Table 5).
Neurotransmitter sensors come in two main classes – periplasmic binding protein (PBP)-based sensors and G-protein coupled receptor (GPCR)-based sensors. PBP-based sensors use microbial PBPs fused to fluorescent proteins to report the presence of neurotransmitters in the synaptic cleft. One of the first PBP-based sensors developed was iGluSnFR, which has been used to detect glutamate in vitro and in vivo in mice, worms, and zebrafish (Marvin et al., 2013). Since then, advanced versions of iGluSnFR with different color and kinetic properties have been developed. Other sensors in the PBP class of neurotransmitter sensors include iGABASnFR, iAChSnFR, and iSeroSnFR, as well as small molecule sensors like iATPSnFR, and iNicSnFR (Lobas et al., 2019; Marvin et al., 2019; Shivange et al., 2019; Borden et al., 2020; Unger et al., 2020). One caveat to using PBP-based sensors is that since they are microbially based, they may interfere with endogenous cellular processes and machinery.
GPCR-based sensors overcome this limitation, as they mimic the properties of endogenous receptors and are quite stable. GPCR sensors bind to neurotransmitters present in the synapse and cause a conformational change that results in a fluorescent signal. Some of the neurotransmitter sensors in this class include the dLight1 and GRABDA families of dopaminergic sensors as well as the GRABNE, GRAB5–HT, and GRABACh sensors for norepinephrine, serotonin, and acetylcholine, respectively (Jing et al., 2018; Patriarchi et al., 2018; Sun et al., 2018; Feng et al., 2019; Wan et al., 2021). Furthermore, the advent of neuropeptide GPCR-based sensors such as OxLight1, MTRIAOT, and GRABOT1.0 for orexin and oxytocin, respectively, permits the visualization of volume transmission from dendrites as well as axon terminals (Ino et al., 2021; Duffet et al., 2022; Qian et al., 2022). However, due to competitive interference from endogenous receptors, the readout of neurotransmitter activity using GPCR sensors will necessarily be based on the relative change in fluorescence rather than the absolute number of neurotransmitter molecules present at the synapse. Additionally, GPCR-based sensors cannot be used for pharmacological studies as they will interfere with the drug-receptor interactions. Thus, depending on the application, either PBP or GPCR sensors may be preferred.
The development of neurotransmitter sensors heralds a new era of neuroscientific discovery, where cell-cell neuronal communication is not only capable of being monitored at the cellular level, but at the synapse itself. In fact, biosensors are even being developed to monitor the activity patterns of second messengers downstream of synaptic transmission (Tewson et al., 2016; Massengill et al., 2021). For example, recent studies using a cAMP biosensor revealed that dopamine-triggered cAMP release in the basal amygdala facilitates learning (Lutas et al., 2022). With the advent of these sensors, neurotransmitter and signaling cascade dynamics may be studied to understand how these molecules play a role in the development and function of circuit architecture within the brain. Additionally, when used in tandem with GECIs or GEVIs, one may monitor both neural activity and neural signaling dynamics at once.
Manipulating Neural Circuits: Gain-And Loss-Of-Function Approaches Toward Interrogating Circuit Function
Mapping circuit connectivity and monitoring its activity patterns reveal critical knowledge regarding brain anatomy and function. However, monitoring a circuit even with cell-type-specific genetic tools only provides correlative data on circuit function. To test hypotheses, researchers often implement gain- or loss-of-function experimentation. About 200 years ago, through post-mortem observation of diseased patients, Dr. Broca identified a lesioned area in the left frontal cortex of an individual that contributed to speech production, as this patient had speech defects (Broca, 1861). While lesioning may still be used in neural systems research, the ability to draw correlations between brain structure and function has accelerated substantially in the last several decades due to the advent of genetically targeted gain- or loss-of-function strategies. Activating a circuit or node reveals its sufficiency to drive a particular phenotype. Reciprocally, inhibiting a circuit or node reveals whether it is necessary for a particular phenotype. Redundant and interdependent circuits add a layer of complexity to these experiments, as they may reveal a circuit to be only sufficient, only necessary, or both toward a particular phenotype. Recent advances in targeted genetic approaches have dramatically improved our ability to manipulate circuits with greater genetic, spatial, and temporal specificity.
Targeted Ablation
Although electrolytic or chemical lesioning methods remain powerful techniques to interrogate structure and function relationships in the brain (Foster et al., 2003; Lavond and Steinmetz, 2003), these approaches are non-specific, ablating all the cells in a given area. Alternatively, genetically-targeted ablation may be used for cell type-specific lesioning. One such system was adopted from the bacteria Corynebacterium diphtheriae. Its earliest rendition involved the use of transgenic lines that placed a diphtheria toxin (DT) gene under a promoter of interest (Breitman et al., 1987; Saito et al., 2001). Cells that used this promoter would express DT, driving their own death. The principal limitation of this technology was that the timing of cellular ablation could not be controlled. Since ablating cells early in development may result in compensatory plasticity mechanisms, it became desirable to temporally control ablation. In 2001, these limitations were abrogated by controlling cellular expression of the DT receptor (DTR), which is not endogenously expressed in the mouse. In this way, the experimenter could selectively express DTR in specific cell types, and provide exogenous DT at any point in development to selectively ablate cells expressing DTR (Saito et al., 2001). This technology led to the discovery that AgRP/NPY neurons are required for proper feeding behaviors in adult mice, but are not required in neonate mice, perhaps due to compensatory mechanisms (Luquet et al., 2005). One important consideration with cellular ablation is that it is permanent. Other experiments may require reversible manipulation, such as the techniques discussed below.
Pharmacology
Neuronal activity can be manipulated by specific chemicals. Commonly, this is due to agonistic or antagonistic effects on neural ion channels (Narahashi et al., 1964; Collingridge et al., 1983; Sweeney et al., 1995; Verderio et al., 1999; Corcoran and Quirk, 2007). By infusing pharmacological substances in particular brain regions, it is readily possible to investigate the effect of activating or inhibiting targeted areas. One common means of drug delivery is through a stereotaxically implanted hollow cannula (Sike et al., 2017). This can be combined with other technologies such as microdialysis or in vivo imaging to dissect the effects of drug treatments on neurotransmitter release, neural activity, and gene expression. Commonly used pharmacologically active chemicals used to manipulate neuronal activity are summarized in Table 6. Importantly, pharmacological manipulations are specific to cells that express a particular receptor but are not selective in the cell types they may target. For reversible and cell type-specific manipulation experiments, opto- or chemogenetics have proven invaluable.
Optogenetics
In the late 1970s, Francis Crick remarked that the real challenge of neuroscience would be to precisely control the activity of a desired set of neurons. Nearly three decades later, George Nagel, Peter Hegemann, and colleagues discovered Channelrhodopsin-2 (ChR2), a light-gated cation channel that demonstrated inward cationic current with millisecond time-scale resolution (Nagel et al., 2003). Shortly after this discovery, Boyden, Deisseroth, and colleagues showed that ChR2 could be leveraged to manipulate the activity of neurons using light in a technique known as optogenetics (Boyden et al., 2005). Currently, it remains one of the most temporally and spatially specific methodologies to manipulate neural activity, both ex vivo and in vivo, in which mice are implanted with fiber optics for light delivery (Yizhar et al., 2011). Optogenetics has led to many systems-level discoveries, such as the solidification of arcuate hypothalamic feeding circuitry. In this study, conditional expression of ChR2 and subsequent activation of AgRP cells in the arcuate nucleus of the hypothalamus led to robust acute food consumption, while optogenetic activation of POMC neurons led to decreased food consumption (Aponte et al., 2011).
At the core of optogenetics is the use of opsins. Upon photon absorption, opsins undergo a conformational change that allows for ion transport across the plasma membrane, either depolarizing or hyperpolarizing neurons (Foster and Bellingham, 2002). As described in Table 7, a plethora of opsins has been engineered that allow great flexibility in their experimental use. These opsins have attributes that differ in terms of effects on membrane potential, kinetics, and absorption spectra (Yizhar et al., 2011; Deisseroth, 2015). Furthermore, more than one type of opsin can be expressed by cells. For example, an excitatory opsin activated by blue light (e.g., ChR2) can be co-expressed with an inhibitory opsin activated by yellow light (e.g., halorhodopsin; Han and Boyden, 2007). This allows for either gain- or loss-of-function experimentation within the same animal. Another consideration in the design of an optogenetic experiment is the location of the light source, either at the soma or axon terminals of the opsin-expressing neural population. If placed at the soma, those cells and all of their projection targets will be affected (Figure 6A). If placed at the axon terminals, only that projection site will be affected (Yizhar et al., 2011; Figure 6B). The latter approach allows for greater dissection of the exact circuitry involved in a particular phenotype (Steinberg et al., 2014). Interestingly, stimulating somata versus terminal fields can result in disparate phenotypes. For example, one study used opsins to stimulate or inhibit basolateral amygdala (BLA) projections to the central amygdala, which altered anxiety-related behaviors, while stimulating BLA cell bodies had no effect. This was perhaps due to downstream projections operating in opposition to each other (Tye et al., 2011). Thus, terminal field stimulation is very useful for dissecting circuit function, as cells may have diverse collateralizations.
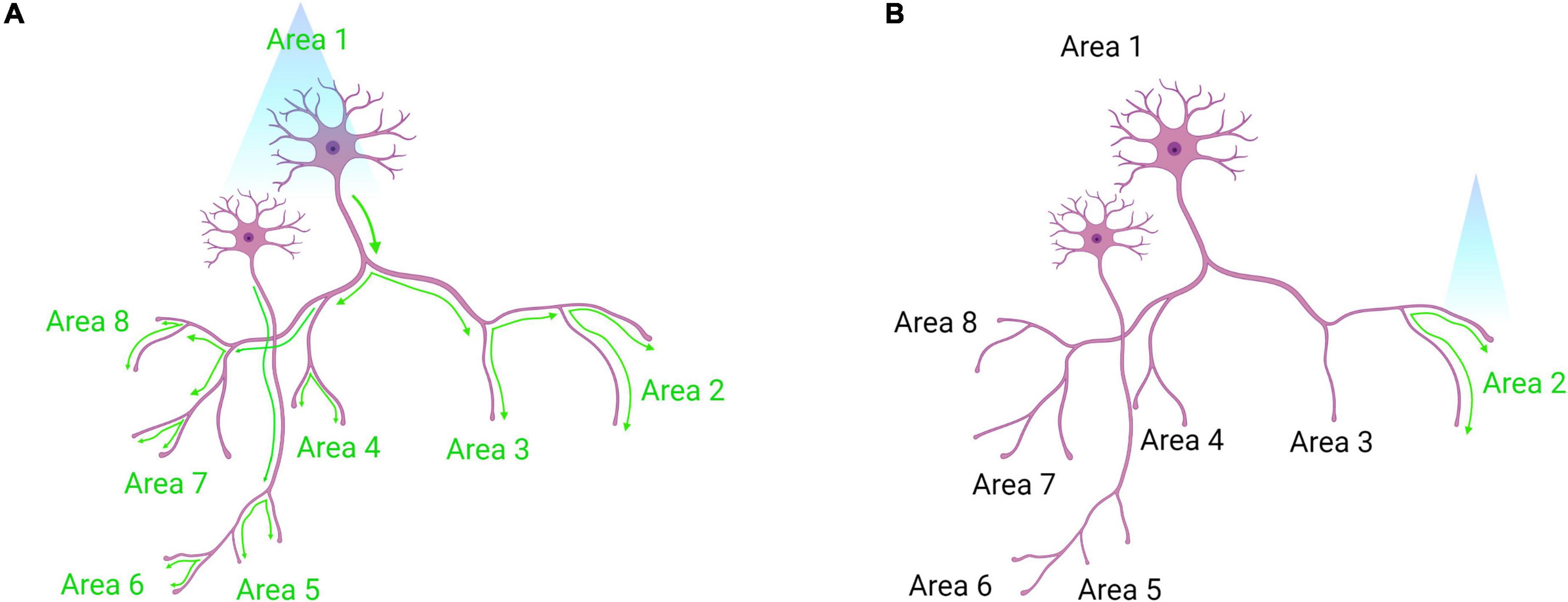
Figure 6. Somatic optogenetic stimulation versus terminal field optogenetic stimulation. (A) Optogenetic stimulation of a population of neurons at their soma will lead to downstream activation of all the projection targets of that population (as indicated by green activation arrows). (B) Terminal field stimulation using optogenetics. Here, an investigator may be interested in the function of Area 1 neuron projections to Area 2 specifically. By placing the laser directly above Area 2, the investigator will activate only those projection terminals to dissect the neural circuit function more precisely. Created with Biorender.com.
However, it must be considered that the nature of optogenetics frequently necessitates a level of invasiveness that may be unacceptable. For example, very deep brain regions with functions essential to survival may not be compatible with external light delivery (Chen et al., 2021). Further, the tethering required by most light-delivery systems may compromise the validity of certain behavioral experiments (Won et al., 2020). Finally, light delivery may lead to tissue toxicity, for example, due to heating or ischemia (Chen et al., 2017a). Therefore, at times less invasive means may be needed to manipulate neural circuits transiently, which is possible through chemogenetics.
Chemogenetics
Chemogenetics refers to a set of techniques whereby genetically engineered receptors expressed on cell surfaces bind to exogenously delivered ligands. Importantly, these ligands are not endogenously produced. Early examples of chemogenetic technologies came in 1998 with Receptors Activated Solely by a Synthetic Ligand (RASSLs; Conklin et al., 2008). Currently, the most commonly used receptors for manipulations are coupled to G proteins known as DREADDs: Designer Receptors Exclusively Activated by Designer Drugs. DREADDs are activated by the ligand Clozapine-N-Oxide (CNO; Whissell et al., 2016; Table 8). Depending on the receptor’s associated downstream signaling cascade, the ligand may be excitatory (Gq, Gs) and increase cell firing, or inhibitory (Gi) and decrease cell firing. While CNO-activated DREADDs are one of the most widely used tools for chemogenetic neural manipulation, this technology has some major limitations. It has been shown that CNO does not cross the blood-brain barrier, but rather its parent metabolite, clozapine (a known antipsychotic drug), exerts effects in the brain (Gomez et al., 2017). Additionally, clozapine has been shown to bind to endogenous receptors throughout the body at high doses and with known effects on animal behavior (Desloovere et al., 2019). However, these findings remain controversial, as other studies report opposing findings in mice (Guettier et al., 2009; Jendryka et al., 2019). To overcome such limitations, studies have shown that at low doses of clozapine, these off-target effects are reduced (Manvich et al., 2018; Matsui et al., 2018). Additionally, novel ligands like olanzapine, JHU37160/152, and deschloroclozapine are being used for DREADD-based manipulations as they have a minimal conversion to other compounds and less off-target effects (Bonaventura et al., 2019; Weston et al., 2019; Nagai et al., 2020).
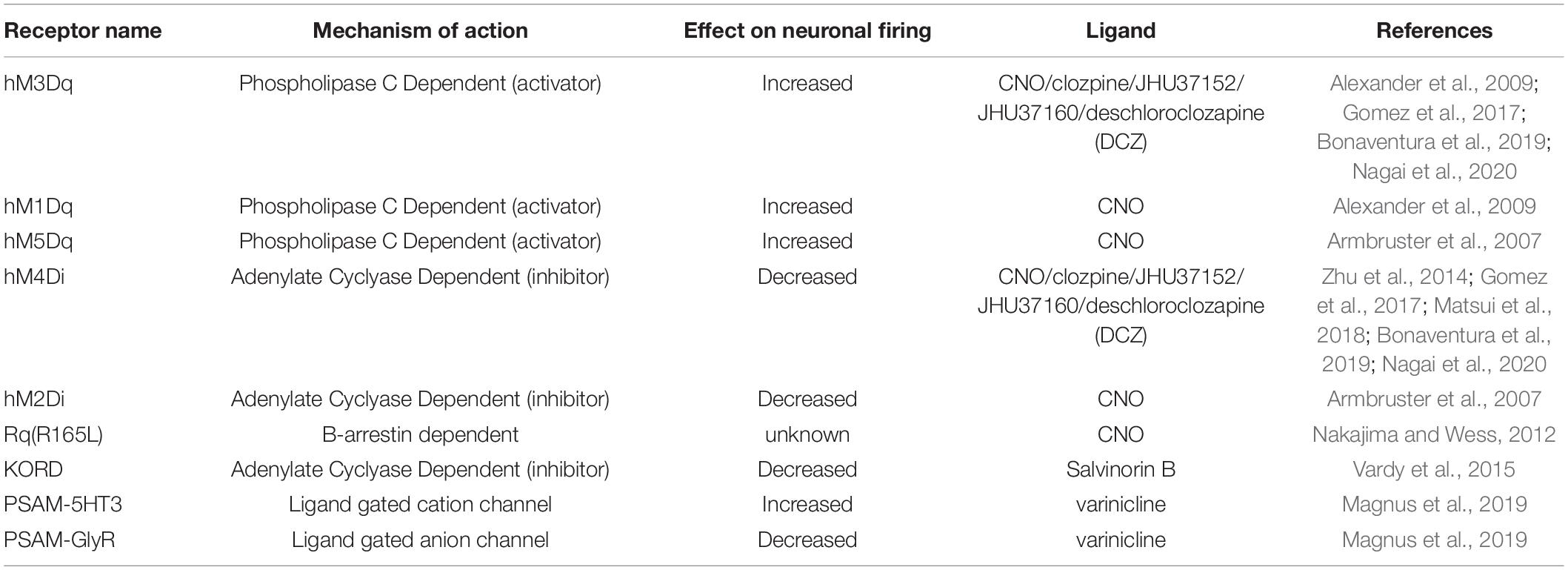
Table 8. Common chemogenetic receptors and ligands with their associated properties and effects on neuronal activity.
Some experiments require one to manipulate several neural populations at once or to bidirectionally control the activity of one neural population. Through a recently developed technology labeled DREADD KORD(R), chemogenetics is afforded the possibility of multiplexing. DREADD KORD(R)s are sensitive to a distinct exogenously delivered ligand known as Salvinorin B and completely insensitive to CNO (Vardy et al., 2015). The non-overlapping pharmacologic activation of DREADD KORD(R) and DREADDs provides superior bidirectional manipulation relative to optogenetics, since opsins may absorb photons at overlapping ranges, potentially confounding results. However, the temporal resolution of DREADD action is less precise than optogenetics and operates on the timescale of minutes to hours (Whissell et al., 2016). This is because it takes time for the synthetic ligand (e.g., CNO) to distribute to areas that express the DREADD, and also because GCPRs have slower kinetics than ion channels. This can be beneficial when requiring more chronic forms of manipulation, but less useful for applications where faster or more temporally-precise manipulations are desired.
While chemogenetics is limited by less precise temporal and spatial resolution, newer technologies are emerging. For example, magnetically-sensitive lipid nanoparticles carrying CNO ensure the steady release of CNO to targeted circuits (Rao et al., 2019). Additionally, it is possible to employ both opsins and DREADDs for bidirectional control of neural activity (Han et al., 2017; Siemian et al., 2021). Alternatively, the development of Pharmacologically Selective Activator Modules (PSAMs) which are activated by Pharmacologically Selective Effector Molecules (PSEMs) increases temporal resolution (Shapiro et al., 2012). Unlike DREADDs which alter neuronal activity through the modulation of GPCR-biased signaling cascades, PSAMs affect neuronal activity through modulation of ligand-gated ion channels. Most recent iterations of the technology use the agonist varenicline, an FDA-approved drug. Although varenicline binds to nicotinic receptors (ligand-gated ion channels), scientists have generated a PSAM that binds to varenicline at a low enough concentration that it will not bind to endogenously produced receptors (Magnus et al., 2019).
Both optogenetic and chemogenetic manipulations allow for controlled activation or inhibition of circuitry in a genetically-defined manner. While useful, these technologies are limited by the need to consistently deliver an actuator (e.g., light through fiber optics for optogenetics or ligands for chemogenetics). Thus, using opto- or chemogenetics for studies in which a circuit would need to be activated/inhibited for more than several hours each day (for example, body weight or metabolism studies) is not optimal. For these studies, it is desirable to chronically activate neural activity, as with the overexpression of exogenous ion channels (Johns et al., 1999; Ren et al., 2001; Lin et al., 2010; Patel et al., 2019; Zhu et al., 2020).
Ion Channel Manipulations: Kir and NaChBac
Neuronal excitability corresponds to a neuron’s likelihood of triggering an action potential. This likelihood is dependent on ion channel expression and functionality. Given the same stimuli, neurons with greater excitability will fire more frequently than neurons with lesser excitability (Hille, 2001). Scientists can bias excitability by manipulating the types and amounts of ion channels expressed on the neuronal plasma membrane so as to shift resting membrane potential (Johns et al., 1999; Lin et al., 2010; Zhu et al., 2020). Since these methods do not require drug administration or photostimulation, they are less invasive measures for studying neural function. However, these methods lack precise on/off control compared to optogenetics or chemogenetics.
One ion channel used to manipulate neural activity chronically is Kir2.1, an inward rectifying potassium channel that makes action potential generation more difficult (Johns et al., 1999). In 2017, the targeted expression of Kir2.1 helped to elucidate a circuit node responsible for integrating social input and modulating aggressive behavioral output (Watanabe et al., 2017). In 2020, the technology was used to understand the arcuate neuronal contribution to feeding behaviors (Zhu et al., 2020). To increase the temporal flexibility of this technology, methods have been devised to temporally control the expression of the channels using ligands or light, or both. For example, SPARK (Synthetic Photoisomerizable Azobene Regulation K + channels) has a genetically engineered K + channel that is sensitive to an exogenously delivered, light-sensitive ligand (Banghart et al., 2004). In the absence of the ligand, the channel remains open and hyperpolarizes the cell. In the presence of the ligand, and the absence of UV light, the K + channel closes. Finally, in the presence of both the ligand and UV light, the channel opens. This affords the flexibility of choosing when to hyperpolarize the cell or allow resting neuron conditions.
Other exogenous ion channels may be used to increase cell excitability and provide gain-of-function approaches. One such channel is the voltage-gated Sodium Bacterial Channel (NaChBac; Ren et al., 2001; Lin et al., 2010). Most importantly, NaChBac opens at more negative voltages relative to eukaryotic sodium channels. Consequently, subthreshold stimuli open these channels, thereby conferring both a greater depolarizing stimulus and an increased probability of action potential generation. Secondly, upon opening, they enable long-lasting depolarizing stimuli that can drive further action potentials. NaChBacs have been used to understand the roles of both corticotropins-releasing hormone (Zhu et al., 2020) and glutamate (Herman et al., 2016; Patel et al., 2019) in modulating feeding behaviors.
Mapping and Monitoring Through Manipulation
Several experimental approaches combine diverse manipulation methods, such as optogenetics, with viral tracing or circuit monitoring techniques. An elegant intersection between circuit mapping, monitoring, and manipulation is the technique known as Channelrhodopsin Assisted Circuit Mapping (CRACM; Petreanu et al., 2007). This technology is a gold standard in the generation of reliable, functional circuit diagrams. Here, opsins and reporters are expressed on a pre-synaptic cell of interest. Then, by taking whole-cell recordings of a putative post-synaptic cell while stimulating the pre-synapse, one may identify synaptic currents on the post-synaptic cell. The temporal coincidence between light stimulation and post-synaptic currents, combined with the application of pharmacological agents that abolish this response, provides strong evidence for a functional synaptic connection between pre- and post-synaptic cells (Petreanu et al., 2009). While CRACM is useful, depending on the circumstance, it can have drawbacks. For example, depending on the expression profile of the opsin, it can be difficult to determine which side of the pre-or post-synapse is being excited by light when an electrophysiological event is being recorded in the post-synaptic cell. This can be mitigated by conditionally expressing the opsin in a neuronal subtype distinct from the post synaptic neuron. Alternatively, one can use an opsin whose expression is spatially restricted to the soma/proximal dendrites (Baker et al., 2016). One other limitation is the challenge of performing CRACM with peptidergic cell types. This is because neuropeptides often signal via volume transmission, which occurs over large distances and with potentially slower effects compared to fast neurotransmitters (like glutamate and GABA; Fuxe et al., 2013; Nederpelt et al., 2016).
Without sophisticated techniques like Patch-Seq, electrophysiology lacks the ability to identify the exact cells being recorded. Combining electrophysiology with optogenetics allows in vivo targeting of genetically labeled cells for electrophysiological recordings through a “sonar”-like method (Lima et al., 2009; Hangya et al., 2015). The development of small implantable optrodes, or electrode(s) juxtaposed to a fiber optic for light delivery, locates conditional ChR2-expressing neurons via optogenetic stimulation in vivo. Once ChR2-expressing cells of interest are located (as indicated by reliable light-induced single units), extracellular recordings assess cellular electrical activity (Figure 7). The “opto-sonar” technique provides a dependable method to genetically identify and target circuits within a live, freely behaving animal. Indeed, with the integration of optogenetics and implantable MEAs, it is now commonplace to not only monitor the activity of genetically defined circuits but manipulate and assess their activity and temporal dynamics in vivo (Kim C. K. et al., 2017).
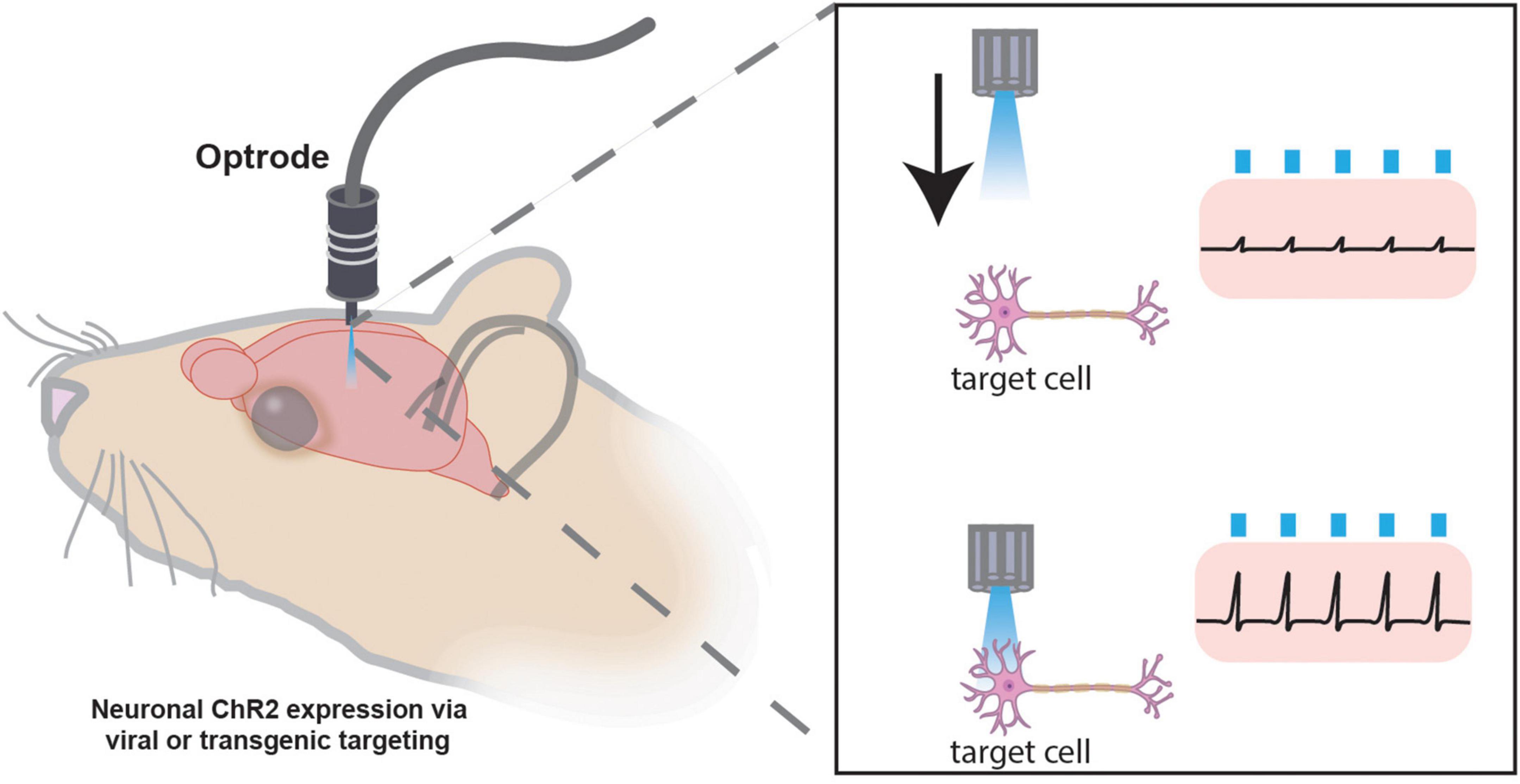
Figure 7. Optogenetic “sonar” method for targeting specific cellular populations for in vivo electrophysiology. Photostimulation “sonar” guides optrode to genetically targeted neurons expressing channelrhodopsin (ChR2) for extracellular electrophysiology recordings. Once the electrode is juxtaposed to a ChR2-expressing cell of interest, electrophysiological recordings may be performed. The optrode may have one or many channels for recording numerous cells simultaneously.
Another emergent multiplexed technology is known as holographic imaging. Holograms are light fields that form any simple and complex shape through wavefront interference. By combining imaging technologies with principles of holography, it has become possible to map, monitor, and manipulate populations of neurons in multiple regions of interest (in different focal planes) with an unprecedented combination of spatial and temporal resolution (Quirin et al., 2014; Yang et al., 2015). In a 2018 paper, Yang and colleagues simultaneously photostimulated 80 neurons within the mouse visual cortex while also monitoring the neighboring neuronal activity (Yang et al., 2018). This technology has since been leveraged to understand how neural ensembles within cortical circuitry can drive recall in a complex behavioral task (Carrillo-Reid et al., 2019). While certain technical limitations to the technology exist (e.g., limited field of view, lag in modulating projected holographic patterns, etc.), more profound challenges present themselves in how the output data is analyzed and interpreted (Carrillo-Reid et al., 2017; Yang and Yuste, 2018). To better use this emergent technology, creating methods to analyze these huge datasets must be a major focus moving forward.
Discussion
The advent of genetic technologies has provided much insight into the complexities of how neural circuits are anatomically and functionally connected. However, dissecting circuits even at the level of molecularly defined groups of neurons may soon be too general. Thus, we expect the future circuit analyses will be done at the level of an individual cell, leveraging omics-based high-throughput approaches to map, monitor, and manipulate neural circuits. For example, while numerous cell-type-specific Cre and Flp drivers have been developed in recent years, continued advances in single-cell sequencing to generate novel drivers, and the use of intersectional and activity-driven genetic labeling techniques may afford the ability to isolate neural subpopulations with greater specificity. Additionally, multiplexed viral tracing techniques strategies that contribute toward maps with greater definition will provide rich anatomical datasets compared to traditional viral tracing or tract labeling methods. Such multiplexed approaches combined with tissue contemporary tissue clearing methods will facilitate the exquisite ability to map individual cells within complex circuits and in three dimensions. Collectively, these methods will rapidly advance the building of comprehensive circuit wiring diagrams with greater resolution.
While electrophysiology will remain a standard for evaluating synaptic connectivity and measuring the electrical properties of individual neurons, novel high-throughput electrophysiology approaches that enable simultaneous recording of hundreds of neurons at once, as with Neuropixels, greatly surpasses current electrophysiology limitations. Additionally, optical approaches for monitoring circuit dynamics will continue to evolve and expand. For example, genetically encoded calcium and voltage indicators, as well as neurotransmitter and neuropeptide sensors, will most likely steadily improve in signal-to-noise, photostability, and kinetics, thus expanding their utility greatly. When used in tandem with GRIN lenses or 2-photon imaging capabilities, genetically encoded imaging experiments can provide a clear picture of the signaling dynamics of individual neurons within dynamic networks. Indeed, the toolbox for circuit activity manipulations will continue to expand as well, with continued advances in optogenetics, chemogenetics, and genetically engineered ion channels with improved temporal, spatial, and cell type precision. With the help of in silico and directed evolution protein engineering methodologies, this will allow a cornucopia of different approaches toward mapping and manipulating neural circuits within living brain tissue.
In the future, multiplexing of a variety of approaches to map, monitor, and manipulate circuits simultaneously will provide more precise experimentation than current methods. However, the major limitations we currently face with these multiplexed, omics-based, and high-throughput methodologies is how these huge datasets will be processed and analyzed in order to make meaningful conclusions about the brain. This will require computation scientists, bioinformaticians, and neuroscientists to work together toward solving these unprecedented problems. Ultimately, better-informed circuit diagrams that link anatomy with the function will provide novel therapeutic targets for numerous neurological diseases, which may include obesity/eating disorders, psychiatric disorders, and neurodegenerative diseases.
Author Contributions
JS, P-SC, JR, and SS: conceptualization, writing, editing, and figure and table generation. PH: writing, figure and table generation. JO-G: editing, figure and table generation. BA: conceptualization and editing. All authors contributed to the article and approved the submitted version.
Funding
We would like to thank numerous funding sources that support the BA’s laboratory and its members in the development of the manuscript: Eunice Kennedy Shriver National Institute of Child Health and Human Development (5P50HD103555-02), National Institute of Neurological Disorders and Stroke (5R01NS078294-08 and 1UF1NS111692-01), National Institute of Diabetes and Digestive and Kidney Diseases (3R01DK109934-05S1 and 2R01DK109934-05), and United States Department of Agriculture (158-3092-0-001).
Conflict of Interest
The authors declare that the research was conducted in the absence of any commercial or financial relationships that could be construed as a potential conflict of interest.
Publisher’s Note
All claims expressed in this article are solely those of the authors and do not necessarily represent those of their affiliated organizations, or those of the publisher, the editors and the reviewers. Any product that may be evaluated in this article, or claim that may be made by its manufacturer, is not guaranteed or endorsed by the publisher.
Acknowledgments
Special thanks to the members of the BA’s laboratory for helping with editing the manuscript and providing valuable feedback.
References
Abdelfattah, A. S., Farhi, S. L., Zhao, Y., Brinks, D., Zou, P., Ruangkittisakul, A., et al. (2016). A bright and fast red fluorescent protein voltage indicator that reports neuronal activity in organotypic brain slices. J. Neurosci. 36, 2458–2472. doi: 10.1523/JNEUROSCI.3484-15.2016
Abdelfattah, A. S., Kawashima, T., Singh, A., Novak, O., Liu, H., Shuai, Y., et al. (2019). Bright and photostable chemigenetic indicators for extended in vivo voltage imaging. Science 365, 699–704. doi: 10.1126/science.aav6416
Abdelfattah, A. S., Valenti, R., Zheng, J., Wong, A., et al. Genie Project, Team (2020). A general approach to engineer positive-going eFRET voltage indicators. Nat. Commun. 11:3444. doi: 10.1038/s41467-020-17322-1
Adam, Y., Kim, J. J., Lou, S., Zhao, Y., Xie, M. E., Brinks, D., et al. (2019). Voltage imaging and optogenetics reveal behaviour-dependent changes in hippocampal dynamics. Nature 569, 413–417. doi: 10.1038/s41586-019-1166-7
Akemann, W., Mutoh, H., Perron, A., Park, Y. K., Iwamoto, Y., and Knöpfel, T. (2012). Imaging neural circuit dynamics with a voltage-sensitive fluorescent protein. J. Neurophysiol. 108, 2323–2337. doi: 10.1152/jn.00452.2012
Akemann, W., Mutoh, H., Perron, A., Rossier, J., and Knöpfel, T. (2010). Imaging brain electric signals with genetically targeted voltage-sensitive fluorescent proteins. Nat. Methods 7, 643–649. doi: 10.1038/nmeth.1479
Akerboom, J., Carreras Calderón, N., Tian, L., Wabnig, S., Prigge, M., Tolö, J., et al. (2013). Genetically encoded calcium indicators for multi-color neural activity imaging and combination with optogenetics. Front. Mol. Neurosci. 6:2. doi: 10.3389/fnmol.2013.00002
Akerboom, J., Chen, T.-W., Wardill, T. J., Tian, L., Marvin, J. S., Mutlu, S., et al. (2012). Optimization of a GCaMP calcium indicator for neural activity imaging. J. Neurosci. 32, 13819–13840. doi: 10.1523/JNEUROSCI.2601-12.2012
Akerboom, J., Rivera, J. D. V., Guilbe, M. M. R., Malavé, E. C. A., Hernandez, H. H., Tian, L., et al. (2009). Crystal structures of the GCaMP calcium sensor reveal the mechanism of fluorescence signal change and aid rational design. J. Biol. Chem. 284, 6455–6464. doi: 10.1074/jbc.M807657200
Alexander, G. M., Rogan, S. C., Abbas, A. I., Armbruster, B. N., Pei, Y., Allen, J. A., et al. (2009). Remote control of neuronal activity in transgenic mice expressing evolved G protein-coupled receptors. Neuron 63, 27–39. doi: 10.1016/j.neuron.2009.06.014
Al-Hasani, R., McCall, J. G., Shin, G., Gomez, A. M., Schmitz, G. P., Bernardi, J. M., et al. (2015). Distinct subpopulations of nucleus accumbens dynorphin neurons drive aversion and reward. Neuron 87, 1063–1077. doi: 10.1016/j.neuron.2015.08.019
Anastassiadis, K., Fu, J., Patsch, C., Hu, S., Weidlich, S., Duerschke, K., et al. (2009). Dre recombinase, like Cre, is a highly efficient site-specific recombinase in E. coli, mammalian cells and mice. Dis. Model. Mech. 2, 508–515. doi: 10.1242/dmm.003087
Aponte, Y., Atasoy, D., and Sternson, S. M. (2011). AGRP neurons are sufficient to orchestrate feeding behavior rapidly and without training. Nat. Neurosci. 14, 351–355. doi: 10.1038/nn.2739
Arenkiel, B. R. (2011). Genetic approaches to reveal the connectivity of adult-born neurons. Front. Neurosci. 5:48. doi: 10.3389/fnins.2011.00048
Armbruster, B. N., Li, X., Pausch, M. H., Herlitze, S., and Roth, B. L. (2007). Evolving the lock to fit the key to create a family of G protein-coupled receptors potently activated by an inert ligand. Proc. Natl. Acad. Sci. U.S.A. 104, 5163–5168. doi: 10.1073/pnas.0700293104
Ataka, K., and Pieribone, V. A. (2002). A genetically targetable fluorescent probe of channel gating with rapid kinetics. Biophys. J. 82, 509–516. doi: 10.1016/S0006-3495(02)75415-5
Atasoy, D., Aponte, Y., Su, H. H., and Sternson, S. M. (2008). A FLEX switch targets Channelrhodopsin-2 to multiple cell types for imaging and long-range circuit mapping. J. Neurosci. 28, 7025–7030. doi: 10.1523/JNEUROSCI.1954-08.2008
Atasoy, D., Betley, J. N., Su, H. H., and Sternson, S. M. (2012). Deconstruction of a neural circuit for hunger. Nature 488, 172–177. doi: 10.1038/nature11270
Awatramani, R., Soriano, P., Rodriguez, C., Mai, J. J., and Dymecki, S. M. (2003). Cryptic boundaries in roof plate and choroid plexus identified by intersectional gene activation. Nat. Genet. 35, 70–75. doi: 10.1038/ng1228
Baird, G. S., Zacharias, D. A., and Tsien, R. Y. (1999). Circular permutation and receptor insertion within green fluorescent proteins. Proc. Natl. Acad. Sci. U.S.A. 96, 11241–11246. doi: 10.1073/pnas.96.20.11241
Baker, C. A., Elyada, Y. M., Parra, A., and Bolton, M. M. (2016). Cellular resolution circuit mapping with temporal-focused excitation of soma-targeted channelrhodopsin. eLife 5:e14193. doi: 10.7554/eLife.14193
Banghart, M., Borges, K., Isacoff, E., Trauner, D., and Kramer, R. H. (2004). Light-activated ion channels for remote control of neuronal firing. Nat. Neurosci. 7, 1381–1386. doi: 10.1038/nn1356
Bardy, C., van den Hurk, M., Kakaradov, B., Erwin, J. A., Jaeger, B. N., Hernandez, R. V., et al. (2016). Predicting the functional states of human iPSC-derived neurons with single-cell RNA-seq and electrophysiology. Mol. Psychiatr. 21, 1573–1588. doi: 10.1038/mp.2016.158
Barretto, R. P. J., Messerschmidt, B., and Schnitzer, M. J. (2009). In vivo fluorescence imaging with high-resolution microlenses. Nat. Methods 6, 511–512. doi: 10.1038/nmeth.1339
Barth, A. L. (2007). Visualizing circuits and systems using transgenic reporters of neural activity. Curr. Opin. Neurobiol. 17, 567–571. doi: 10.1016/j.conb.2007.10.003
Baumgardt, M., Miguel-Aliaga, I., Karlsson, D., Ekman, H., and Thor, S. (2007). Specification of neuronal identities by feedforward combinatorial coding. PLoS Biol. 5:e37. doi: 10.1371/journal.pbio.0050037
Beck, C., and Gong, Y. (2019). A high-speed, bright, red fluorescent voltage sensor to detect neural activity. Sci. Rep. 9:15878. doi: 10.1038/s41598-019-52370-8
Berndt, A., Lee, S. Y., Ramakrishnan, C., and Deisseroth, K. (2014). Structure-guided transformation of channelrhodopsin into a light-activated chloride channel. Science 344, 420–424. doi: 10.1126/science.1252367
Berndt, A., Schoenenberger, P., Mattis, J., Tye, K. M., Deisseroth, K., Hegemann, P., et al. (2011). High-efficiency channelrhodopsins for fast neuronal stimulation at low light levels. Proc. Natl. Acad. Sci. U.S.A. 108, 7595–7600. doi: 10.1073/pnas.1017210108
Berndt, A., Yizhar, O., Gunaydin, L. A., Hegemann, P., and Deisseroth, K. (2009). Bi-stable neural state switches. Nat. Neurosci. 12, 229–234. doi: 10.1038/nn.2247
Bonaventura, J., Eldridge, M. A. G., Hu, F., Gomez, J. L., Sanchez-Soto, M., Abramyan, A. M., et al. (2019). High-potency ligands for DREADD imaging and activation in rodents and monkeys. Nat. Commun. 10:4627. doi: 10.1038/s41467-019-12236-z
Bond, C. W., Trinko, R., Foscue, E., Furman, K., Groman, S. M., Taylor, J. R., et al. (2020). Medial nucleus accumbens projections to the ventral tegmental area control food consumption. J. Neurosci. 40, 4727–4738. doi: 10.1523/JNEUROSCI.3054-18.2020
Bootman, M. D., and Berridge, M. J. (1995). The elemental principles of calcium signaling. Cell 83, 675–678. doi: 10.1016/0092-8674(95)90179-5
Borden, P. M., Zhang, P., Shivange, A. V., Marvin, J. S., Cichon, J., Dan, C., et al. (2020). A fast genetically encoded fluorescent sensor for faithful in vivo acetylcholine detection in mice, fish, worms and flies. BioRxiv [preprint] doi: 10.1101/2020.02.07.939504
Borden, P. Y., Ortiz, A. D., Waiblinger, C., Sederberg, A. J., Morrissette, A. E., Forest, C. R., et al. (2017). Genetically expressed voltage sensor ArcLight for imaging large scale cortical activity in the anesthetized and awake mouse. Neurophotonics 4:031212. doi: 10.1117/1.NPh.4.3.031212
Boyden, E. S., Zhang, F., Bamberg, E., Nagel, G., and Deisseroth, K. (2005). Millisecond-timescale, genetically targeted optical control of neural activity. Nat. Neurosci. 8, 1263–1268. doi: 10.1038/nn1525
Branda, C. S., and Dymecki, S. M. (2004). Talking about a revolution: The impact of site-specific recombinases on genetic analyses in mice. Dev. Cell 6, 7–28. doi: 10.1016/s1534-5807(03)00399-x
Breitman, M. L., Clapoff, S., Rossant, J., Tsui, L. C., Glode, L. M., Maxwell, I. H., et al. (1987). Genetic ablation: targeted expression of a toxin gene causes microphthalmia in transgenic mice. Science 238, 1563–1565. doi: 10.1126/science.3685993
Brobeck, J. R. (1946). Mechanism of the development of obesity in animals with hypothalamic lesions. Physiol. Rev. 26, 541–559. doi: 10.1152/physrev.1946.26.4.541
Broca, P. (1861). Remarques sur le siège de la faculté du langage articulé, suivies d’une observation d’aphémie (perte de la parole). Bull Memoires Soc. anatomique Paris 6, 330–357.
Brooks, C. M., Lockwood, R. A., and Wiggins, M. L. (1946). A study of the effect of hypothalamic lesions on the eating habits of the albino rat. Am. J. Physiol. 147, 735–741. doi: 10.1152/ajplegacy.1946.147.4.735
Bruschetta, G., Jin, S., Liu, Z.-W., Kim, J. D., and Diano, S. (2020). MC4R signaling in dorsal raphe nucleus controls feeding, anxiety, and depression. Cell Rep. 33:108267. doi: 10.1016/j.celrep.2020.108267
Buzsáki, G. (2004). Large-scale recording of neuronal ensembles. Nat. Neurosci. 7, 446–451. doi: 10.1038/nn1233
Cadwell, C. R., Palasantza, A., Jiang, X., Berens, P., Deng, Q., Yilmaz, M., et al. (2016). Electrophysiological, transcriptomic and morphologic profiling of single neurons using Patch-seq. Nat. Biotechnol. 34, 199–203. doi: 10.1038/nbt.3445
Campos, C. A., Bowen, A. J., Schwartz, M. W., and Palmiter, R. D. (2016). Parabrachial CGRP neurons control meal termination. Cell Metab. 23, 811–820. doi: 10.1016/j.cmet.2016.04.006
Cao, G., Platisa, J., Pieribone, V. A., Raccuglia, D., Kunst, M., and Nitabach, M. N. (2013). Genetically targeted optical electrophysiology in intact neural circuits. Cell 154, 904–913. doi: 10.1016/j.cell.2013.07.027
Carlezon, W. A., Boundy, V. A., Haile, C. N., Lane, S. B., Kalb, R. G., Neve, R. L., et al. (1997). Sensitization to morphine induced by viral-mediated gene transfer. Science 277, 812–814. doi: 10.1126/science.277.5327.812
Carrillo-Reid, L., Han, S., Yang, W., Akrouh, A., and Yuste, R. (2019). Controlling visually guided behavior by holographic recalling of cortical ensembles. Cell 178, 447.e–457.e. doi: 10.1016/j.cell.2019.05.045
Carrillo-Reid, L., Yang, W., Kang Miller, J.-E., Peterka, D. S., and Yuste, R. (2017). Imaging and optically manipulating neuronal ensembles. Annu. Rev. Biophys. 46, 271–293. doi: 10.1146/annurev-biophys-070816-033647
Chai, Y., Jiang, X., Ito, Y., Bringas, P., Han, J., Rowitch, D. H., et al. (2000). Fate of the mammalian cranial neural crest during tooth and mandibular morphogenesis. Development 127, 1671–1679. doi: 10.1242/dev.127.8.1671
Chamberland, S., Yang, H. H., Pan, M. M., Evans, S. W., Guan, S., Chavarha, M., et al. (2017). Fast two-photon imaging of subcellular voltage dynamics in neuronal tissue with genetically encoded indicators. eLife 6:e25690. doi: 10.7554/eLife.25690
Chanda, B., Blunck, R., Faria, L. C., Schweizer, F. E., Mody, I., and Bezanilla, F. (2005). A hybrid approach to measuring electrical activity in genetically specified neurons. Nat. Neurosci. 8, 1619–1626. doi: 10.1038/nn1558
Chen, R., Canales, A., and Anikeeva, P. (2017a). Neural recording and modulation technologies. Nat. Rev. Mater. 2:16093. doi: 10.1038/natrevmats.2016.93
Chen, R., Wu, X., Jiang, L., and Zhang, Y. (2017b). Single-Cell RNA-Seq Reveals Hypothalamic Cell Diversity. Cell Rep. 18, 3227–3241. doi: 10.1016/j.celrep.2017.03.004
Chen, R., Gore, F., Nguyen, Q.-A., Ramakrishnan, C., Patel, S., Kim, S. H., et al. (2021). Deep brain optogenetics without intracranial surgery. Nat. Biotechnol. 39, 161–164. doi: 10.1038/s41587-020-0679-9
Chen, T.-W., Wardill, T. J., Sun, Y., Pulver, S. R., Renninger, S. L., Baohan, A., et al. (2013). Ultrasensitive fluorescent proteins for imaging neuronal activity. Nature 499, 295–300. doi: 10.1038/nature12354
Chen, X., Sun, Y.-C., Church, G. M., Lee, J. H., and Zador, A. M. (2018). Efficient in situ barcode sequencing using padlock probe-based BaristaSeq. Nucleic Acids Res. 46:e22. doi: 10.1093/nar/gkx1206
Chen, X., Sun, Y.-C., Zhan, H., Kebschull, J. M., Fischer, S., Matho, K., et al. (2019). High-Throughput Mapping of Long-Range Neuronal Projection Using In Situ Sequencing. Cell 179, 772.e–786.e. doi: 10.1016/j.cell.2019.09.023
Chen, X., Zhang, K., Zhou, L., Gao, X., Wang, J., Yao, Y., et al. (2016). Coupled electrophysiological recording and single cell transcriptome analyses revealed molecular mechanisms underlying neuronal maturation. Protein Cell 7, 175–186. doi: 10.1007/s13238-016-0247-8
Chung, K., Wallace, J., Kim, S.-Y., Kalyanasundaram, S., Andalman, A. S., Davidson, T. J., et al. (2013). Structural and molecular interrogation of intact biological systems. Nature 497, 332–337. doi: 10.1038/nature12107
Chuong, A. S., Miri, M. L., Busskamp, V., Matthews, G. A. C., Acker, L. C., Sørensen, A. T., et al. (2014). Noninvasive optical inhibition with a red-shifted microbial rhodopsin. Nat. Neurosci. 17, 1123–1129. doi: 10.1038/nn.3752
Clark, I. C., Gutiérrez-Vázquez, C., Wheeler, M. A., Li, Z., Rothhammer, V., Linnerbauer, M., et al. (2021). Barcoded viral tracing of single-cell interactions in central nervous system inflammation. Science 372:eabf1230. doi: 10.1126/science.abf1230
Collingridge, G. L., Kehl, S. J., and McLennan, H. (1983). Excitatory amino acids in synaptic transmission in the Schaffer collateral-commissural pathway of the rat hippocampus. J. Physiol. 334, 33–46. doi: 10.1113/jphysiol.1983.sp014478
Colosimo, M. E., Brown, A., Mukhopadhyay, S., Gabel, C., Lanjuin, A. E., Samuel, A. D. T., et al. (2004). Identification of thermosensory and olfactory neuron-specific genes via expression profiling of single neuron types. Curr. Biol. 14, 2245–2251. doi: 10.1016/j.cub.2004.12.030
Conklin, B. R., Hsiao, E. C., Claeysen, S., Dumuis, A., Srinivasan, S., Forsayeth, J. R., et al. (2008). Engineering GPCR signaling pathways with RASSLs. Nat. Methods 5, 673–678. doi: 10.1038/nmeth.1232
Corcoran, K. A., and Quirk, G. J. (2007). Activity in prelimbic cortex is necessary for the expression of learned, but not innate, fears. J. Neurosci. 27, 840–844. doi: 10.1523/JNEUROSCI.5327-06.2007
Csicsvari, J., Henze, D. A., Jamieson, B., Harris, K. D., Sirota, A., Barthó, P., et al. (2003). Massively parallel recording of unit and local field potentials with silicon-based electrodes. J. Neurophysiol. 90, 1314–1323. doi: 10.1152/jn.00116.2003
Cushing, H. (1932). Papers Relating to the Pituitary Body, Hypothalamus and Parasympathetic Nervous System. Springfield, Ill.: C. C. Thomas.
Dana, H., Mohar, B., Sun, Y., Narayan, S., Gordus, A., Hasseman, J. P., et al. (2016). Sensitive red protein calcium indicators for imaging neural activity. eLife 5:e12727. doi: 10.7554/eLife.12727
Dana, H., Sun, Y., Mohar, B., Hulse, B. K., Kerlin, A. M., Hasseman, J. P., et al. (2019). High-performance calcium sensors for imaging activity in neuronal populations and microcompartments. Nat. Methods 16, 649–657. doi: 10.1038/s41592-019-0435-6
Deisseroth, K. (2015). Optogenetics: 10 years of microbial opsins in neuroscience. Nat. Neurosci. 18, 1213–1225. doi: 10.1038/nn.4091
DeNardo, L., and Luo, L. (2017). Genetic strategies to access activated neurons. Curr. Opin. Neurobiol. 45, 121–129. doi: 10.1016/j.conb.2017.05.014
Desloovere, J., Boon, P., Larsen, L. E., Merckx, C., Goossens, M.-G., Van den Haute, C., et al. (2019). Long-term chemogenetic suppression of spontaneous seizures in a mouse model for temporal lobe epilepsy. Epilepsia 60, 2314–2324. doi: 10.1111/epi.16368
Dietrich, M. O., Zimmer, M. R., Bober, J., and Horvath, T. L. (2015). Hypothalamic Agrp neurons drive stereotypic behaviors beyond feeding. Cell 160, 1222–1232. doi: 10.1016/j.cell.2015.02.024
Dimitrov, D., He, Y., Mutoh, H., Baker, B. J., Cohen, L., Akemann, W., et al. (2007). Engineering and characterization of an enhanced fluorescent protein voltage sensor. PLoS One 2:e440. doi: 10.1371/journal.pone.0000440
Dix, R. D., McKendall, R. R., and Baringer, J. R. (1983). Comparative neurovirulence of herpes simplex virus type 1 strains after peripheral or intracerebral inoculation of BALB/c mice. Infect. Immun. 40, 103–112. doi: 10.1128/iai.40.1.103-112.1983
Do, J. P., Xu, M., Lee, S.-H., Chang, W.-C., Zhang, S., Chung, S., et al. (2016). Cell type-specific long-range connections of basal forebrain circuit. eLife 5, e13214. doi: 10.7554/eLife.13214
Duffet, L., Kosar, S., Panniello, M., Viberti, B., Bracey, E., Zych, A. D., et al. (2022). A genetically encoded sensor for in vivo imaging of orexin neuropeptides. Nat. Methods 19, 231–241. doi: 10.1038/s41592-021-01390-2
Dymecki, S. M. (1996). A modular set of Flp, FRT and lacZ fusion vectors for manipulating genes by site-specific recombination. Gene 171, 197–201. doi: 10.1016/0378-1119(96)00035-2
Dymecki, S. M., Rodriguez, C. I., and Awatramani, R. B. (2002). Switching on lineage tracers using site-specific recombination. Methods Mol. Biol. 185, 309–334. doi: 10.1385/1-59259-241-4:309
Dymecki, S. M., and Tomasiewicz, H. (1998). Using Flp-recombinase to characterize expansion of Wnt1-expressing neural progenitors in the mouse. Dev. Biol. 201, 57–65. doi: 10.1006/dbio.1998.8971
Einevoll, G. T., Kayser, C., Logothetis, N. K., and Panzeri, S. (2013). Modelling and analysis of local field potentials for studying the function of cortical circuits. Nat. Rev. Neurosci. 14, 770–785. doi: 10.1038/nrn3599
Enquist, L. W. (2002). Exploiting circuit-specific spread of pseudorabies virus in the central nervous system: insights to pathogenesis and circuit tracers. J. Infect. Dis. 186, S209–S214. doi: 10.1086/344278
Etessami, R., Conzelmann, K.-K., Fadai-Ghotbi, B., Natelson, B., Tsiang, H., and Ceccaldi, P.-E. (2000). Spread and pathogenic characteristics of a G-deficient rabies virus recombinant: an in vitro and in vivo study. J. Gen. Virol. 81, 2147–2153. doi: 10.1099/0022-1317-81-9-2147
Feinberg, E. H., Vanhoven, M. K., Bendesky, A., Wang, G., Fetter, R. D., Shen, K., et al. (2008). GFP Reconstitution Across Synaptic Partners (GRASP) defines cell contacts and synapses in living nervous systems. Neuron 57, 353–363. doi: 10.1016/j.neuron.2007.11.030
Feng, J., Zhang, C., Lischinsky, J. E., Jing, M., Zhou, J., Wang, H., et al. (2019). A genetically encoded fluorescent sensor for rapid and specific in vivo detection of norepinephrine. Neuron 102, 745.e–761.e. doi: 10.1016/j.neuron.2019.02.037
Fenno, L. E., Mattis, J., Ramakrishnan, C., Hyun, M., Lee, S. Y., He, M., et al. (2014). Targeting cells with single vectors using multiple-feature Boolean logic. Nat. Methods 11, 763–772. doi: 10.1038/nmeth.2996
Fenno, L. E., Ramakrishnan, C., Kim, Y. S., Evans, K. E., Lo, M., Vesuna, S., et al. (2020). Comprehensive Dual- and Triple-Feature Intersectional Single-Vector Delivery of Diverse Functional Payloads to Cells of Behaving Mammals. Neuron 107, 836.e–853.e. doi: 10.1016/j.neuron.2020.06.003
Flames, N., and Hobert, O. (2009). Gene regulatory logic of dopamine neuron differentiation. Nature 458, 885–889. doi: 10.1038/nature07929
Földy, C., Darmanis, S., Aoto, J., Malenka, R. C., Quake, S. R., and Südhof, T. C. (2016). Single-cell RNAseq reveals cell adhesion molecule profiles in electrophysiologically defined neurons. Proc. Natl. Acad. Sci. U.S.A. 113, E5222–E5231. doi: 10.1073/pnas.1610155113
Fosque, B. F., Sun, Y., Dana, H., Yang, C.-T., Ohyama, T., Tadross, M. R., et al. (2015). Neural circuits. Labeling of active neural circuits in vivo with designed calcium integrators. Science 347, 755–760. doi: 10.1126/science.1260922
Foster, J. A., Bezin, L., Groc, L., Christopherson, P. L., and Levine, R. A. (2003). Kainic acid lesion-induced nigral neuronal death. J. Chem. Neuroanat. 26, 65–73. doi: 10.1016/S0891-0618(03)00040-1
Foster, R., and Bellingham, J. (2002). Opsins and melanopsins. Curr. Biol. 12, R543–R544. doi: 10.1016/s0960-9822(02)01047-3
Fuxe, K., Borroto-Escuela, D. O., Romero-Fernandez, W., Zhang, W.-B., and Agnati, L. F. (2013). Volume transmission and its different forms in the central nervous system. Chin. J. Integr. Med. 19, 323–329. doi: 10.1007/s11655-013-1455-1
Fuzik, J., Zeisel, A., Máté, Z., Calvigioni, D., Yanagawa, Y., Szabó, G., et al. (2016). Integration of electrophysiological recordings with single-cell RNA-seq data identifies neuronal subtypes. Nat. Biotechnol. 34, 175–183. doi: 10.1038/nbt.3443
Gautam, S. G., Perron, A., Mutoh, H., and Knöpfel, T. (2009). Exploration of fluorescent protein voltage probes based on circularly permuted fluorescent proteins. Front. Neuroeng. 2:14. doi: 10.3389/neuro.16.014.2009
Gerfen, C. R., and Sawchenko, P. E. (1984). An anterograde neuroanatomical tracing method that shows the detailed morphology of neurons, their axons and terminals: immunohistochemical localization of an axonally transported plant lectin, Phaseolus vulgaris leucoagglutinin (PHA-L). Brain Res. 290, 219–238. doi: 10.1016/0006-8993(84)90940-5
Gergues, M. M., Han, K. J., Choi, H. S., Brown, B., Clausing, K. J., Turner, V. S., et al. (2020). Circuit and molecular architecture of a ventral hippocampal network. Nat. Neurosci. 23, 1444–1452. doi: 10.1038/s41593-020-0705-8
Ghosh, K. K., Burns, L. D., Cocker, E. D., Nimmerjahn, A., Ziv, Y., Gamal, A. E., et al. (2011). Miniaturized integration of a fluorescence microscope. Nat. Methods 8, 871–878. doi: 10.1038/nmeth.1694
Gimlich, R. L., and Braun, J. (1985). Improved fluorescent compounds for tracing cell lineage. Dev. Biol. 109, 509–514. doi: 10.1016/0012-1606(85)90476-2
Gokce, O., Stanley, G. M., Treutlein, B., Neff, N. F., Camp, J. G., Malenka, R. C., et al. (2016). Cellular Taxonomy of the Mouse Striatum as Revealed by Single-Cell RNA-Seq. Cell Rep. 16, 1126–1137. doi: 10.1016/j.celrep.2016.06.059
Golgi, C. (1886). Sulla Fina Anatomia Degli Organi Centrali del Sistema Nervoso. Italy: Hoepli, 1886.
Gomez, J. L., Bonaventura, J., Lesniak, W., Mathews, W. B., Sysa-Shah, P., Rodriguez, L. A., et al. (2017). Chemogenetics revealed: DREADD occupancy and activation via converted clozapine. Science 357, 503–507. doi: 10.1126/science.aan2475
Gong, S., Doughty, M., Harbaugh, C. R., Cummins, A., Hatten, M. E., Heintz, N., et al. (2007). Targeting Cre recombinase to specific neuron populations with bacterial artificial chromosome constructs. J. Neurosci. 27, 9817–9823. doi: 10.1523/JNEUROSCI.2707-07.2007
Gong, Y. (2015). The evolving capabilities of rhodopsin-based genetically encoded voltage indicators. Curr. Opin. Chem. Biol. 27, 84–89. doi: 10.1016/j.cbpa.2015.05.006
Gong, Y., Huang, C., Li, J. Z., Grewe, B. F., Zhang, Y., Eismann, S., et al. (2015). High-speed recording of neural spikes in awake mice and flies with a fluorescent voltage sensor. Science 350, 1361–1366. doi: 10.1126/science.aab0810
Gong, Y., Wagner, M. J., Zhong, Li, J., and Schnitzer, M. J. (2014). Imaging neural spiking in brain tissue using FRET-opsin protein voltage sensors. Nat. Commun. 5:3674. doi: 10.1038/ncomms4674
Gouwens, N. W., Sorensen, S. A., Baftizadeh, F., Budzillo, A., Lee, B. R., Jarsky, T., et al. (2020). Integrated Morphoelectric and Transcriptomic Classification of Cortical GABAergic Cells. Cell 183, 935.e–953.e. doi: 10.1016/j.cell.2020.09.057
Govorunova, E. G., Sineshchekov, O. A., Janz, R., Liu, X., and Spudich, J. L. (2015). Natural light-gated anion channels: A family of microbial rhodopsins for advanced optogenetics. Science 349, 647–650. doi: 10.1126/science.aaa7484
Govorunova, E. G., Sineshchekov, O. A., Rodarte, E. M., Janz, R., Morelle, O., Melkonian, M., et al. (2017). The expanding family of natural anion channelrhodopsins reveals large variations in kinetics, conductance, and spectral sensitivity. Sci. Rep. 7:43358. doi: 10.1038/srep43358
Gradinaru, V., Zhang, F., Ramakrishnan, C., Mattis, J., Prakash, R., Diester, I., et al. (2010). Molecular and cellular approaches for diversifying and extending optogenetics. Cell 141, 154–165. doi: 10.1016/j.cell.2010.02.037
Gray, E. G. (1959). Axo-somatic and axo-dendritic synapses of the cerebral cortex: an electron microscope study. J. Anat. 93, 420–433.
Gray, P. A., Fu, H., Luo, P., Zhao, Q., Yu, J., Ferrari, A., et al. (2004). Mouse brain organization revealed through direct genome-scale TF expression analysis. Science 306, 2255–2257. doi: 10.1126/science.1104935
Greenberg, M. E., and Ziff, E. B. (1984). Stimulation of 3T3 cells induces transcription of the c-fos proto-oncogene. Nature 311, 433–438. doi: 10.1038/311433a0
Grenier, V., Daws, B. R., Liu, P., and Miller, E. W. (2019). Spying on Neuronal Membrane Potential with Genetically Targetable Voltage Indicators. J. Am. Chem. Soc. 141, 1349–1358. doi: 10.1021/jacs.8b11997
Grewe, B. F., Langer, D., Kasper, H., Kampa, B. M., and Helmchen, F. (2010). High-speed in vivo calcium imaging reveals neuronal network activity with near-millisecond precision. Nat. Methods 7, 399–405. doi: 10.1038/nmeth.1453
Griesbeck, O., Baird, G. S., Campbell, R. E., Zacharias, D. A., and Tsien, R. Y. (2001). Reducing the environmental sensitivity of yellow fluorescent protein. Mechanism and applications. J. Biol. Chem. 276, 29188–29194. doi: 10.1074/jbc.M102815200
Grimm, J. B., English, B. P., Chen, J., Slaughter, J. P., Zhang, Z., Revyakin, A., et al. (2015). A general method to improve fluorophores for live-cell and single-molecule microscopy. Nat. Methods 12, 244–250. 3 p following 250. doi: 10.1038/nmeth.3256
Guenthner, C. J., Miyamichi, K., Yang, H. H., Heller, H. C., and Luo, L. (2013). Permanent genetic access to transiently active neurons via TRAP: targeted recombination in active populations. Neuron 78, 773–784. doi: 10.1016/j.neuron.2013.03.025
Guettier, J.-M., Gautam, D., Scarselli, M., Ruiz, de Azua, I., Li, J. H., et al. (2009). A chemical-genetic approach to study G protein regulation of beta cell function in vivo. Proc. Natl. Acad. Sci. U.S.A. 106, 19197–19202. doi: 10.1073/pnas.0906593106
Haggerty, D. L., Grecco, G. G., Reeves, K. C., and Atwood, B. (2020). Adeno-Associated Viral Vectors in Neuroscience Research. Mol. Ther. Methods Clin. Dev. 17, 69–82. doi: 10.1016/j.omtm.2019.11.012
Hama, H., Hioki, H., Namiki, K., Hoshida, T., Kurokawa, H., Ishidate, F., et al. (2015). ScaleS: an optical clearing palette for biological imaging. Nat. Neurosci. 18, 1518–1529. doi: 10.1038/nn.4107
Hama, H., Kurokawa, H., Kawano, H., Ando, R., Shimogori, T., Noda, H., et al. (2011). Scale: a chemical approach for fluorescence imaging and reconstruction of transparent mouse brain. Nat. Neurosci. 14, 1481–1488. doi: 10.1038/nn.2928
Han, W., Tellez, L. A., Rangel, M. J., Motta, S. C., Zhang, X., Perez, I. O., et al. (2017). Integrated control of predatory hunting by the central nucleus of the amygdala. Cell 168, 311–324.e18. doi: 10.1016/j.cell.2016.12.027
Han, X., and Boyden, E. S. (2007). Multiple-color optical activation, silencing, and desynchronization of neural activity, with single-spike temporal resolution. PLoS One 2:e299. doi: 10.1371/journal.pone.0000299
Han, Y., Kebschull, J. M., Campbell, R. A. A., Cowan, D., Imhof, F., Zador, A. M., et al. (2018). The logic of single-cell projections from visual cortex. Nature 556, 51–56. doi: 10.1038/nature26159
Hanchate, N. K., Lee, E. J., Ellis, A., Kondoh, K., Kuang, D., Basom, R., et al. (2020). Connect-seq to superimpose molecular on anatomical neural circuit maps. Proc. Natl. Acad. Sci. U.S.A. 117, 4375–4384. doi: 10.1073/pnas.1912176117
Hangya, B., Ranade, S. P., Lorenc, M., and Kepecs, A. (2015). Central cholinergic neurons are rapidly recruited by reinforcement feedback. Cell 162, 1155–1168. doi: 10.1016/j.cell.2015.07.057
Hashikawa, Y., Hashikawa, K., Rossi, M. A., Basiri, M. L., Liu, Y., Johnston, N. L., et al. (2020). Transcriptional and spatial resolution of cell types in the mammalian habenula. Neuron 106, 743.e–758.e. doi: 10.1016/j.neuron.2020.03.011
Helassa, N., Dürst, C. D., Coates, C., Kerruth, S., Arif, U., Schulze, C., et al. (2018). Ultrafast glutamate sensors resolve high-frequency release at Schaffer collateral synapses. Proc. Natl. Acad. Sci. U.S.A. 115, 5594–5599. doi: 10.1073/pnas.1720648115
Helassa, N., Zhang, X., Conte, I., Scaringi, J., Esposito, E., Bradley, J., et al. (2015). Fast-Response Calmodulin-Based Fluorescent Indicators Reveal Rapid Intracellular Calcium Dynamics. Sci. Rep. 5:15978. doi: 10.1038/srep15978
Helmchen, F., Fee, M. S., Tank, D. W., and Denk, W. (2001). A miniature head-mounted two-photon microscope. high-resolution brain imaging in freely moving animals. Neuron 31, 903–912. doi: 10.1016/s0896-6273(01)00421-4
Hendel, T., Mank, M., Schnell, B., Griesbeck, O., Borst, A., and Reiff, D. F. (2008). Fluorescence changes of genetic calcium indicators and OGB-1 correlated with neural activity and calcium in vivo and in vitro. J. Neurosci. 28, 7399–7411. doi: 10.1523/JNEUROSCI.1038-08.2008
Herman, A. M., Ortiz-Guzman, J., Kochukov, M., Herman, I., Quast, K. B., Patel, J. M., et al. (2016). A cholinergic basal forebrain feeding circuit modulates appetite suppression. Nature 538, 253–256. doi: 10.1038/nature19789
Hill, C. L., and Stephens, G. J. (2021). An introduction to patch clamp recording. Methods Mol. Biol. 2188, 1–19. doi: 10.1007/978-1-0716-0818-0_1
Hochbaum, D. R., Zhao, Y., Farhi, S. L., Klapoetke, N., Werley, C. A., Kapoor, V., et al. (2014). All-optical electrophysiology in mammalian neurons using engineered microbial rhodopsins. Nat. Methods 11, 825–833. doi: 10.1038/nmeth.3000
Hoogland, T. M., Kuhn, B., Göbel, W., Huang, W., Nakai, J., Helmchen, F., et al. (2009). Radially expanding transglial calcium waves in the intact cerebellum. Proc. Natl. Acad. Sci. U.S.A. 106, 3496–3501. doi: 10.1073/pnas.0809269106
Huang, H., Lee, S. H., Ye, C., Lima, I. S., Oh, B.-C., Lowell, B. B., et al. (2013). ROCK1 in AgRP neurons regulates energy expenditure and locomotor activity in male mice. Endocrinology 154, 3660–3670. doi: 10.1210/en.2013-1343
Huang, L., Kebschull, J. M., Fürth, D., Musall, S., Kaufman, M. T., Churchland, A. K., et al. (2020). BRICseq Bridges Brain-wide Interregional Connectivity to Neural Activity and Gene Expression in Single Animals. Cell 182, 177.e–188.e. doi: 10.1016/j.cell.2020.05.029
Inagaki, S., Agetsuma, M., Ohara, S., Iijima, T., Yokota, H., Wazawa, T., et al. (2019). Imaging local brain activity of multiple freely moving mice sharing the same environment. Sci. Rep. 9:7460. doi: 10.1038/s41598-019-43897-x
Inagaki, S., Tsutsui, H., Suzuki, K., Agetsuma, M., Arai, Y., Jinno, Y., et al. (2017). Genetically encoded bioluminescent voltage indicator for multi-purpose use in wide range of bioimaging. Sci. Rep. 7:42398. doi: 10.1038/srep42398
Ino, D., Hibino, H., and Nishiyama, M. (2021). A fluorescent sensor for the real-time measurement of extracellular oxytocin dynamics in the brain. BioRxiv [preprint] doi: 10.1101/2021.07.30.454450
Inoue, M., Takeuchi, A., Horigane, S., Ohkura, M., Gengyo-Ando, K., Fujii, H., et al. (2015). Rational design of a high-affinity, fast, red calcium indicator R-CaMP2. Nat. Methods 12, 64–70. doi: 10.1038/nmeth.3185
Inoue, M., Takeuchi, A., Manita, S., Horigane, S.-I., Sakamoto, M., Kawakami, R., et al. (2019). Rational engineering of xcamps, a multicolor GECI suite for in vivo imaging of complex brain circuit dynamics. Cell 177, 1346.e–1360.e. doi: 10.1016/j.cell.2019.04.007
Isobe, T., Tomita, M., Matsumoto, J., Itoh, T., and Fujita, T. (1983). Hematologic and immunologic aberrations in patients under diphenylhydantoin administration. Nippon Ketsueki Gakkai Zasshi 46, 1–5.
Jendryka, M., Palchaudhuri, M., Ursu, D., van der Veen, B., Liss, B., Kätzel, D., et al. (2019). Pharmacokinetic and pharmacodynamic actions of clozapine-N-oxide, clozapine, and compound 21 in DREADD-based chemogenetics in mice. Sci. Rep. 9:4522. doi: 10.1038/s41598-019-41088-2
Jin, L., Baker, B., Mealer, R., Cohen, L., Pieribone, V., Pralle, A., et al. (2011). Random insertion of split-cans of the fluorescent protein venus into Shaker channels yields voltage sensitive probes with improved membrane localization in mammalian cells. J. Neurosci. Methods 199, 1–9. doi: 10.1016/j.jneumeth.2011.03.028
Jing, M., Li, Y., Zeng, J., Huang, P., Skirzewski, M., Kljakic, O., et al. (2020). An optimized acetylcholine sensor for monitoring in vivo cholinergic activity. Nat. Methods 17, 1139–1146. doi: 10.1038/s41592-020-0953-2
Jing, M., Zhang, P., Wang, G., Feng, J., Mesik, L., Zeng, J., et al. (2018). A genetically encoded fluorescent acetylcholine indicator for in vitro and in vivo studies. Nat. Biotechnol. 36, 726–737. doi: 10.1038/nbt.4184
Johns, D. C., Marx, R., Mains, R. E., O’Rourke, B., and Marbán, E. (1999). Inducible genetic suppression of neuronal excitability. J. Neurosci. 19, 1691–1697. doi: 10.1523/JNEUROSCI.19-05-01691.1999
Jun, J. J., Steinmetz, N. A., Siegle, J. H., Denman, D. J., Bauza, M., Barbarits, B., et al. (2017). Fully integrated silicon probes for high-density recording of neural activity. Nature 551, 232–236. doi: 10.1038/nature24636
Kakava-Georgiadou, N., Zwartkruis, M. M., Bullich-Vilarrubias, C., Luijendijk, M. C. M., Garner, K. M., van der Plasse, G., et al. (2019). An Intersectional Approach to Target Neural Circuits With Cell- and Projection-Type Specificity: Validation in the Mesolimbic Dopamine System. Front. Mol. Neurosci. 12:49. doi: 10.3389/fnmol.2019.00049
Kannan, M., Vasan, G., Huang, C., Haziza, S., Li, J. Z., Inan, H., et al. (2018). Fast, in vivo voltage imaging using a red fluorescent indicator. Nat. Methods 15, 1108–1116. doi: 10.1038/s41592-018-0188-7
Kannan, M., Vasan, G., and Pieribone, V. A. (2019). Optimizing strategies for developing genetically encoded voltage indicators. Front. Cell. Neurosci. 13:53. doi: 10.3389/fncel.2019.00053
Kato, H. E., Kamiya, M., Sugo, S., Ito, J., Taniguchi, R., Orito, A., et al. (2015). Atomistic design of microbial opsin-based blue-shifted optogenetics tools. Nat. Commun. 6:7177. doi: 10.1038/ncomms8177
Katz, L. C., Burkhalter, A., and Dreyer, W. J. (1984). Fluorescent latex microspheres as a retrograde neuronal marker for in vivo and in vitro studies of visual cortex. Nature 310, 498–500. doi: 10.1038/310498a0
Katz, L. C., and Larovici, D. M. (1990). Green fluorescent latex microspheres: a new retrograde tracer. Neuroscience 34, 511–520. doi: 10.1016/0306-4522(90)90159-2
Ke, M.-T., Nakai, Y., Fujimoto, S., Takayama, R., Yoshida, S., Kitajima, T. S., et al. (2016). Super-Resolution Mapping of Neuronal Circuitry With an Index-Optimized Clearing Agent. Cell Rep. 14, 2718–2732. doi: 10.1016/j.celrep.2016.02.057
Kebschull, J. M., Garcia, da Silva, P., Reid, A. P., Peikon, I. D., Albeanu, D. F., et al. (2016). High-Throughput Mapping of Single-Neuron Projections by Sequencing of Barcoded RNA. Neuron 91, 975–987. doi: 10.1016/j.neuron.2016.07.036
Kim, C. K., Adhikari, A., and Deisseroth, K. (2017). Integration of optogenetics with complementary methodologies in systems neuroscience. Nat. Rev. Neurosci. 18, 222–235. doi: 10.1038/nrn.2017.15
Kim, J., Zhang, X., Muralidhar, S., LeBlanc, S. A., and Tonegawa, S. (2017). Basolateral to central amygdala neural circuits for appetitive behaviors. Neuron 93, 1464.e–1479.e. doi: 10.1016/j.neuron.2017.02.034
Kim, C. K., Sanchez, M. I., Hoerbelt, P., Fenno, L. E., Malenka, R. C., Deisseroth, K., et al. (2020). A molecular calcium integrator reveals a striatal cell type driving aversion. Cell 183, 2003.e–2019.e. doi: 10.1016/j.cell.2020.11.015
Kim, J., Zhao, T., Petralia, R. S., Yu, Y., Peng, H., Myers, E., et al. (2011). mGRASP enables mapping mammalian synaptic connectivity with light microscopy. Nat. Methods 9, 96–102. doi: 10.1038/nmeth.1784
King, B. M. (2006). The rise, fall, and resurrection of the ventromedial hypothalamus in the regulation of feeding behavior and body weight. Physiol. Behav. 87, 221–244. doi: 10.1016/j.physbeh.2005.10.007
Klapoetke, N. C., Murata, Y., Kim, S. S., Pulver, S. R., Birdsey-Benson, A., Cho, Y. K., et al. (2014). Independent optical excitation of distinct neural populations. Nat. Methods 11, 338–346. doi: 10.1038/nmeth.2836
Knöpfel, T., and Song, C. (2019). Optical voltage imaging in neurons: moving from technology development to practical tool. Nat. Rev. Neurosci. 20, 719–727. doi: 10.1038/s41583-019-0231-4
Köbbert, C., Apps, R., Bechmann, I., Lanciego, J. L., Mey, J., and Thanos, S. (2000). Current concepts in neuroanatomical tracing. Prog. Neurobiol. 62, 327–351. doi: 10.1016/S0301-0082(00)00019-8
Kostyuk, A. I., Demidovich, A. D., Kotova, D. A., Belousov, V. V., and Bilan, D. S. (2019). Circularly Permuted Fluorescent Protein-Based Indicators: History, Principles, and Classification. Int. J. Mol. Sci 20:4200. doi: 10.3390/ijms20174200
Kralj, J. M., Douglass, A. D., Hochbaum, D. R., Maclaurin, D., and Cohen, A. E. (2011). Optical recording of action potentials in mammalian neurons using a microbial rhodopsin. Nat. Methods 9, 90–95. doi: 10.1038/nmeth.1782
Kristensson, K. (1977). Retrograde Axonal Transport of Horseradish Peroxidase. Acta Neuropathol. 38, 143–147.
Kristensson, K., and Olsson, Y. (1971). Uptake and Retrograde Axonal Transport of Peroxidase in Hypoglossal Neurones. Acta Neuropathol 19, 1–9.
Kuhlman, S. J., and Huang, Z. J. (2008). High-resolution labeling and functional manipulation of specific neuron types in mouse brain by Cre-activated viral gene expression. PLoS One 3:e2005. doi: 10.1371/journal.pone.0002005
Kuypers, H. G., and Ugolini, G. (1990). Viruses as transneuronal tracers. Trends Neurosci. 13, 71–75. doi: 10.1016/0166-2236(90)90071-h
Lam, A. J., St-Pierre, F., Gong, Y., Marshall, J. D., Cranfill, P. J., Baird, M. A., et al. (2012). Improving FRET dynamic range with bright green and red fluorescent proteins. Nat. Methods 9, 1005–1012. doi: 10.1038/nmeth.2171
Lanciego, J. L., and Wouterlood, F. G. (2011). A half century of experimental neuroanatomical tracing. J. Chem. Neuroanat. 42, 157–183. doi: 10.1016/j.jchemneu.2011.07.001
Lavoie, A., and Liu, B.-H. (2020). Canine adenovirus 2: A natural choice for brain circuit dissection. Front. Mol. Neurosci. 13:9. doi: 10.3389/fnmol.2020.00009
Lavond, D. G., and Steinmetz, J. E. (2003). “Lesion techniques for behavioral experiments,” in Handbook of Classical Conditioning. E. S. Murphy and F. K. McSweeney (Boston, MA: Springer US), 249–276. doi: 10.1007/978-1-4615-0263-0_8
Li, L., Tasic, B., Micheva, K. D., Ivanov, V. M., Spletter, M. L., Smith, S. J., et al. (2010). Visualizing the distribution of synapses from individual neurons in the mouse brain. PLoS One 5:e11503. doi: 10.1371/journal.pone.0011503
Lilley, C. E., Groutsi, F., Han, Z., Palmer, J. A., Anderson, P. N., Latchman, D. S., et al. (2001). Multiple immediate-early gene-deficient herpes simplex virus vectors allowing efficient gene delivery to neurons in culture and widespread gene delivery to the central nervous system in vivo. J. Virol. 75, 4343–4356. doi: 10.1128/JVI.75.9.4343-4356.2001
Lima, S. Q., Hromádka, T., Znamenskiy, P., and Zador, A. M. (2009). PINP: a new method of tagging neuronal populations for identification during in vivo electrophysiological recording. PLoS One 4:e6099. doi: 10.1371/journal.pone.0006099
Lin, C.-W., Sim, S., Ainsworth, A., Okada, M., Kelsch, W., and Lois, C. (2010). Genetically increased cell-intrinsic excitability enhances neuronal integration into adult brain circuits. Neuron 65, 32–39. doi: 10.1016/j.neuron.2009.12.001
Lin, J. Y. (2011). A user’s guide to channelrhodopsin variants: features, limitations and future developments. Exp. Physiol. 96, 19–25. doi: 10.1113/expphysiol.2009.051961
Lin, J. Y., Knutsen, P. M., Muller, A., Kleinfeld, D., and Tsien, R. Y. (2013). ReaChR: a red-shifted variant of channelrhodopsin enables deep transcranial optogenetic excitation. Nat. Neurosci. 16, 1499–1508. doi: 10.1038/nn.3502
Lin, J. Y., Lin, M. Z., Steinbach, P., and Tsien, R. Y. (2009). Characterization of engineered channelrhodopsin variants with improved properties and kinetics. Biophys. J. 96, 1803–1814. doi: 10.1016/j.bpj.2008.11.034
Lipovsek, M., Bardy, C., Cadwell, C. R., Hadley, K., Kobak, D., and Tripathy, S. J. (2021). Patch-seq: Past. Present, and Future. J. Neurosci. 41, 937–946. doi: 10.1523/JNEUROSCI.1653-20.2020
Lo, L., and Anderson, D. J. (2011). A Cre-dependent, anterograde transsynaptic viral tracer for mapping output pathways of genetically marked neurons. Neuron 72, 938–950. doi: 10.1016/j.neuron.2011.12.002
Lobas, M. A., Tao, R., Nagai, J., Kronschläger, M. T., Borden, P. M., Marvin, J. S., et al. (2019). A genetically encoded single-wavelength sensor for imaging cytosolic and cell surface ATP. Nat. Commun. 10:711. doi: 10.1038/s41467-019-08441-5
Lohse, M., Bajo, V. M., King, A. J., and Willmore, B. D. B. (2020). Neural circuits underlying auditory contrast gain control and their perceptual implications. Nat. Commun. 11:324. doi: 10.1038/s41467-019-14163-5
Lundby, A., Mutoh, H., Dimitrov, D., Akemann, W., and Knöpfel, T. (2008). Engineering of a genetically encodable fluorescent voltage sensor exploiting fast Ci-VSP voltage-sensing movements. PLoS One 3:e2514. doi: 10.1371/journal.pone.0002514
Luquet, S., Perez, F. A., Hnasko, T. S., and Palmiter, R. D. (2005). NPY/AgRP neurons are essential for feeding in adult mice but can be ablated in neonates. Science 310, 683–685. doi: 10.1126/science.1115524
Lutas, A., Fernando, K., Zhang, S. X., Sambangi, A., and Andermann, M. L. (2022). History-dependent dopamine release increases cAMP levels in most basal amygdala glutamatergic neurons to control learning. Cell Rep. 38:110297. doi: 10.1016/j.celrep.2022.110297
Lütcke, H., Murayama, M., Hahn, T., Margolis, D. J., Astori, S., and Zum Alten, et al. (2010). Optical recording of neuronal activity with a genetically-encoded calcium indicator in anesthetized and freely moving mice. Front. Neural Circuits 4:9. doi: 10.3389/fncir.2010.00009
Lyford, G. L., Yamagata, K., Kaufmann, W. E., Barnes, C. A., Sanders, L. K., Copeland, N. G., et al. (1995). Arc, a growth factor and activity-regulated gene, encodes a novel cytoskeleton-associated protein that is enriched in neuronal dendrites. Neuron 14, 433–445. doi: 10.1016/0896-6273(95)90299-6
Madden, C. J., and Morrison, S. F. (2019). Central nervous system circuits that control body temperature. Neurosci. Lett. 696, 225–232. doi: 10.1016/j.neulet.2018.11.027
Magnus, C. J., Lee, P. H., Bonaventura, J., Zemla, R., Gomez, J. L., Ramirez, M. H., et al. (2019). Ultrapotent chemogenetics for research and potential clinical applications. Science 364:eaav5282. doi: 10.1126/science.aav5282
Manvich, D. F., Webster, K. A., Foster, S. L., Farrell, M. S., Ritchie, J. C., Porter, J. H., et al. (2018). The DREADD agonist clozapine N-oxide (CNO) is reverse-metabolized to clozapine and produces clozapine-like interoceptive stimulus effects in rats and mice. Sci. Rep. 8:3840. doi: 10.1038/s41598-018-22116-z
Martin, X., and Dolivo, M. (1983). Neuronal and transneuronal tracing in the trigeminal system of the rat using the herpes virus suis. Brain Res. 273, 253–276. doi: 10.1016/0006-8993(83)90850-8
Marvin, J. S., Borghuis, B. G., Tian, L., Cichon, J., Harnett, M. T., Akerboom, J., et al. (2013). An optimized fluorescent probe for visualizing glutamate neurotransmission. Nat. Methods 10, 162–170. doi: 10.1038/nmeth.2333
Marvin, J. S., Scholl, B., Wilson, D. E., Podgorski, K., Kazemipour, A., Müller, J. A., et al. (2018). Stability, affinity, and chromatic variants of the glutamate sensor iGluSnFR. Nat. Methods 15, 936–939. doi: 10.1038/s41592-018-0171-3
Marvin, J. S., Shimoda, Y., Magloire, V., Leite, M., Kawashima, T., Jensen, T. P., et al. (2019). A genetically encoded fluorescent sensor for in vivo imaging of GABA. Nat. Methods 16, 763–770. doi: 10.1038/s41592-019-0471-2
Massengill, C. I., Day-Cooney, J., Mao, T., and Zhong, H. (2021). Genetically encoded sensors towards imaging cAMP and PKA activity in vivo. J. Neurosci. Methods 362:109298. doi: 10.1016/j.jneumeth.2021.109298
Matsui, S., Sasaki, T., Kohno, D., Yaku, K., Inutsuka, A., Yokota-Hashimoto, H., et al. (2018). Neuronal SIRT1 regulates macronutrient-based diet selection through FGF21 and oxytocin signalling in mice. Nat. Commun. 9:4604. doi: 10.1038/s41467-018-07033-z
Matsumoto, K., Mitani, T. T., Horiguchi, S. A., Kaneshiro, J., Murakami, T. C., Mano, T., et al. (2019). Advanced CUBIC tissue clearing for whole-organ cell profiling. Nat. Protoc. 14, 3506–3537. doi: 10.1038/s41596-019-0240-9
Mattis, J., Tye, K. M., Ferenczi, E. A., Ramakrishnan, C., O’Shea, D. J., Prakash, R., et al. (2011). Principles for applying optogenetic tools derived from direct comparative analysis of microbial opsins. Nat. Methods 9, 159–172. doi: 10.1038/nmeth.1808
McCulloch, W. S., and Pitts, W. (1943). A logical calculus of the ideas immanent in nervous activity. Bull. Math. Biophys. 5, 115–133. doi: 10.1007/BF02478259
Meinke, G., Bohm, A., Hauber, J., Pisabarro, M. T., and Buchholz, F. (2016). Cre recombinase and other tyrosine recombinases. Chem. Rev. 116, 12785–12820. doi: 10.1021/acs.chemrev.6b00077
Mishina, Y., Mutoh, H., Song, C., and Knöpfel, T. (2014). Exploration of genetically encoded voltage indicators based on a chimeric voltage sensing domain. Front. Mol. Neurosci. 7:78. doi: 10.3389/fnmol.2014.00078
Miyawaki, A., Llopis, J., Heim, R., McCaffery, J. M., Adams, J. A., Ikura, M., et al. (1997). Fluorescent indicators for Ca2+ based on green fluorescent proteins and calmodulin. Nature 388, 882–887. doi: 10.1038/42264
Mollinedo-Gajate, I., Song, C., and Knöpfel, T. (2021). Genetically encoded voltage indicators. Adv. Exp. Med. Biol. 1293, 209–224. doi: 10.1007/978-981-15-8763-4_12
Muñoz-Manchado, A. B., Bengtsson Gonzales, C., Zeisel, A., Munguba, H., Bekkouche, B., Skene, N. G., et al. (2018). Diversity of Interneurons in the Dorsal Striatum Revealed by Single-Cell RNA Sequencing and PatchSeq. Cell Rep. 24, 2179.e–2190.e. doi: 10.1016/j.celrep.2018.07.053
Murakami, T. C., Mano, T., Saikawa, S., Horiguchi, S. A., Shigeta, D., Baba, K., et al. (2018). A three-dimensional single-cell-resolution whole-brain atlas using CUBIC-X expansion microscopy and tissue clearing. Nat. Neurosci. 21, 625–637. doi: 10.1038/s41593-018-0109-1
Mutoh, H., Perron, A., Dimitrov, D., Iwamoto, Y., Akemann, W., Chudakov, D. M., et al. (2009). Spectrally-resolved response properties of the three most advanced FRET based fluorescent protein voltage probes. PLoS One 4:e4555. doi: 10.1371/journal.pone.0004555
Nagai, Y., Miyakawa, N., Takuwa, H., Hori, Y., Oyama, K., Ji, B., et al. (2020). Deschloroclozapine, a potent and selective chemogenetic actuator enables rapid neuronal and behavioral modulations in mice and monkeys. Nat. Neurosci. 23, 1157–1167. doi: 10.1038/s41593-020-0661-3
Nagel, G., Szellas, T., Huhn, W., Kateriya, S., Adeishvili, N., Berthold, P., et al. (2003). Channelrhodopsin-2, a directly light-gated cation-selective membrane channel. Proc. Natl. Acad. Sci. U.S.A. 100, 13940–13945. doi: 10.1073/pnas.1936192100
Nakai, J., Ohkura, M., and Imoto, K. (2001). A high signal-to-noise Ca(2+) probe composed of a single green fluorescent protein. Nat. Biotechnol. 19, 137–141. doi: 10.1038/84397
Nakajima, K., and Wess, J. (2012). Design and functional characterization of a novel, arrestin-biased designer G protein-coupled receptor. Mol. Pharmacol. 82, 575–582. doi: 10.1124/mol.112.080358
Narahashi, T., Moore, J. W., and Scott, W. R. (1964). Tetrodotoxin blockage of sodium conductance increase in lobster giant axons. J. Gen. Physiol. 47, 965–974. doi: 10.1085/jgp.47.5.965
Nectow, A. R., and Nestler, E. J. (2020). Viral tools for neuroscience. Nat. Rev. Neurosci. 21, 669–681. doi: 10.1038/s41583-020-00382-z
Nederpelt, I., Bunnik, J., IJzerman, A. P., and Heitman, L. H. (2016). Kinetic Profile of Neuropeptide-Receptor Interactions. Trends Neurosci. 39, 830–839. doi: 10.1016/j.tins.2016.09.008
Neher, E., and Sakmann, B. (1976). Noise analysis of drug induced voltage clamp currents in denervated frog muscle fibres. J. Physiol. 258, 705–729. doi: 10.1113/jphysiol.1976.sp011442
Newmaster, K. T., Kronman, F. A., Wu, Y.-T., and Kim, Y. (2021). Seeing the forest and its trees together: implementing 3D light microscopy pipelines for cell type mapping in the mouse brain. Front. Neuroanat. 15:787601. doi: 10.3389/fnana.2021.787601
Oe, Y., Wang, X., Patriarchi, T., Konno, A., Ozawa, K., Yahagi, K., et al. (2020). Author Correction: Distinct temporal integration of noradrenaline signaling by astrocytic second messengers during vigilance. Nat. Commun. 11:3447. doi: 10.1038/s41467-020-17059-x
Oh, J., Lee, C., and Kaang, B.-K. (2019). Imaging and analysis of genetically encoded calcium indicators linking neural circuits and behaviors. Korean J. Physiol. Pharmacol. 23, 237–249. doi: 10.4196/kjpp.2019.23.4.237
Ohkura, M., Matsuzaki, M., Kasai, H., Imoto, K., and Nakai, J. (2005). Genetically encoded bright Ca2+ probe applicable for dynamic Ca2+ imaging of dendritic spines. Anal. Chem. 77, 5861–5869. doi: 10.1021/ac0506837
Ohkura, M., Sasaki, T., Kobayashi, C., Ikegaya, Y., and Nakai, J. (2012a). An improved genetically encoded red fluorescent Ca2+ indicator for detecting optically evoked action potentials. PLoS One 7:e39933. doi: 10.1371/journal.pone.0039933
Ohkura, M., Sasaki, T., Sadakari, J., Gengyo-Ando, K., Kagawa-Nagamura, Y., Kobayashi, C., et al. (2012b). Genetically encoded green fluorescent Ca2+ indicators with improved detectability for neuronal Ca2+ signals. PLoS One 7:e51286. doi: 10.1371/journal.pone.0051286
Patel, J. M., Swanson, J., Ung, K., Herman, A., Hanson, E., Ortiz-Guzman, J., et al. (2019). Sensory perception drives food avoidance through excitatory basal forebrain circuits. eLife 8:e44548. doi: 10.7554/eLife.44548
Patriarchi, T., Cho, J. R., Merten, K., Howe, M. W., Marley, A., Xiong, W.-H., et al. (2018). Ultrafast neuronal imaging of dopamine dynamics with designed genetically encoded sensors. Science 360:eaat4422. doi: 10.1126/science.aat4422
Patriarchi, T., Mohebi, A., Sun, J., Marley, A., Liang, R., Dong, C., et al. (2020). An expanded palette of dopamine sensors for multiplex imaging in vivo. Nat. Methods 17, 1147–1155. doi: 10.1038/s41592-020-0936-3
Pérez Koldenkova, V., and Nagai, T. (2013). Genetically encoded Ca(2+) indicators: properties and evaluation. Biochim. Biophys. Acta 1833, 1787–1797. doi: 10.1016/j.bbamcr.2013.01.011
Perron, A., Mutoh, H., Akemann, W., Gautam, S. G., Dimitrov, D., Iwamoto, Y., et al. (2009). Second and third generation voltage-sensitive fluorescent proteins for monitoring membrane potential. Front. Mol. Neurosci. 2:5. doi: 10.3389/neuro.02.005.2009
Petreanu, L., Huber, D., Sobczyk, A., and Svoboda, K. (2007). Channelrhodopsin-2-assisted circuit mapping of long-range callosal projections. Nat. Neurosci. 10, 663–668. doi: 10.1038/nn1891
Petreanu, L., Mao, T., Sternson, S. M., and Svoboda, K. (2009). The subcellular organization of neocortical excitatory connections. Nature 457, 1142–1145. doi: 10.1038/nature07709
Piao, H. H., Rajakumar, D., Kang, B. E., Kim, E. H., and Baker, B. J. (2015). Combinatorial mutagenesis of the voltage-sensing domain enables the optical resolution of action potentials firing at 60 Hz by a genetically encoded fluorescent sensor of membrane potential. J. Neurosci. 35, 372–385. doi: 10.1523/JNEUROSCI.3008-14.2015
Piatkevich, K. D., Bensussen, S., Tseng, H.-A., Shroff, S. N., Lopez-Huerta, V. G., Park, D., et al. (2019). Population imaging of neural activity in awake behaving mice. Nature 574, 413–417. doi: 10.1038/s41586-019-1641-1
Piatkevich, K. D., Jung, E. E., Straub, C., Linghu, C., Park, D., Suk, H.-J., et al. (2018). A robotic multidimensional directed evolution approach applied to fluorescent voltage reporters. Nat. Chem. Biol. 14, 352–360. doi: 10.1038/s41589-018-0004-9
Piccolino, M. (1997). Luigi Galvani and animal electricity: two centuries after the foundation of electrophysiology. Trends Neurosci. 20, 443–448. doi: 10.1016/s0166-2236(97)01101-6
Piccolino, M. (1998). Animal electricity and the birth of electrophysiology: the legacy of Luigi Galvani. Brain Res. Bull. 46, 381–407. doi: 10.1016/S0361-9230(98)00026-4
Porumb, T., Yau, P., Harvey, T. S., and Ikura, M. (1994). A calmodulin-target peptide hybrid molecule with unique calcium-binding properties. Protein Eng. 7, 109–115. doi: 10.1093/protein/7.1.109
Qian, T., Wang, H., Wang, P., Geng, L., Mei, L., Osakada, T., et al. (2022). Compartmental neuropeptide release measured using a new oxytocin sensor. BioRxiv [preprint] doi: 10.1101/2022.02.10.480016
Qian, Y., Cosio, D. M. O., Piatkevich, K. D., Aufmkolk, S., Su, W.-C., Celiker, O. T., et al. (2020). Improved genetically encoded near-infrared fluorescent calcium ion indicators for in vivo imaging. PLoS Biol. 18:e3000965. doi: 10.1371/journal.pbio.3000965
Quirin, S., Jackson, J., Peterka, D. S., and Yuste, R. (2014). Simultaneous imaging of neural activity in three dimensions. Front. Neural Circuits 8:29. doi: 10.3389/fncir.2014.00029
Rao, S., Chen, R., LaRocca, A. A., Christiansen, M. G., Senko, A. W., Shi, C. H., et al. (2019). Remotely controlled chemomagnetic modulation of targeted neural circuits. Nat. Nanotechnol. 14, 967–973. doi: 10.1038/s41565-019-0521-z
Redolfi, N., García-Casas, P., Fornetto, C., Sonda, S., Pizzo, P., and Pendin, D. (2021). Lighting up ca2+ dynamics in animal models. Cells 10:2133. doi: 10.3390/cells10082133
Reiff, D. F., Ihring, A., Guerrero, G., Isacoff, E. Y., Joesch, M., Nakai, J., et al. (2005). In vivo performance of genetically encoded indicators of neural activity in flies. J. Neurosci. 25, 4766–4778. doi: 10.1523/JNEUROSCI.4900-04.2005
Reijmers, L. G., Perkins, B. L., Matsuo, N., and Mayford, M. (2007). Localization of a stable neural correlate of associative memory. Science 317, 1230–1233. doi: 10.1126/science.1143839
Ren, D., Navarro, B., Xu, H., Yue, L., Shi, Q., and Clapham, D. E. (2001). A prokaryotic voltage-gated sodium channel. Science 294, 2372–2375. doi: 10.1126/science.1065635
Reynolds, S. M., and Berridge, K. C. (2002). Positive and negative motivation in nucleus accumbens shell: bivalent rostrocaudal gradients for GABA-elicited eating, taste “liking”/”disliking” reactions, place preference/avoidance, and fear. J. Neurosci. 22, 7308–7320. doi:20026734. doi: 10.1523/JNEUROSCI.22-16-07308.2002
Root, D. H., Mejias-Aponte, C. A., Qi, J., and Morales, M. (2014). Role of glutamatergic projections from ventral tegmental area to lateral habenula in aversive conditioning. J. Neurosci. 34, 13906–13910. doi: 10.1523/JNEUROSCI.2029-14.2014
Rose, T., Goltstein, P. M., Portugues, R., and Griesbeck, O. (2014). Putting a finishing touch on GECIs. Front. Mol. Neurosci. 7:88. doi: 10.3389/fnmol.2014.00088
Rossi, M. A., Basiri, M. L., Liu, Y., Hashikawa, Y., Hashikawa, K., Fenno, L. E., et al. (2021). Transcriptional and functional divergence in lateral hypothalamic glutamate neurons projecting to the lateral habenula and ventral tegmental area. Neuron 109, 3823.e–3837.e. doi: 10.1016/j.neuron.2021.09.020
Rossi, M. A., Basiri, M. L., McHenry, J. A., Kosyk, O., Otis, J. M., van den Munkhof, H. E., et al. (2019). Obesity remodels activity and transcriptional state of a lateral hypothalamic brake on feeding. Science 364, 1271–1274. doi: 10.1126/science.aax1184
Rubaiy, H. N. (2017). A short guide to electrophysiology and ion channels. J. Pharm. Pharm. Sci. 20, 48–67. doi: 10.18433/J32P6R
Ruder, L., Schina, R., Kanodia, H., Valencia-Garcia, S., Pivetta, C., and Arber, S. (2021). A functional map for diverse forelimb actions within brainstem circuitry. Nature 590, 445–450. doi: 10.1038/s41586-020-03080-z
Sabatini, B. L., and Tian, L. (2020). Imaging Neurotransmitter and Neuromodulator Dynamics In Vivo with Genetically Encoded Indicators. Neuron 108, 17–32. doi: 10.1016/j.neuron.2020.09.036
Saito, M., Iwawaki, T., Taya, C., Yonekawa, H., Noda, M., Inui, Y., et al. (2001). Diphtheria toxin receptor-mediated conditional and targeted cell ablation in transgenic mice. Nat. Biotechnol. 19, 746–750. doi: 10.1038/90795
Sakai, R., Repunte-Canonigo, V., Raj, C. D., and Knöpfel, T. (2001). Design and characterization of a DNA-encoded, voltage-sensitive fluorescent protein. Eur. J. Neurosci. 13, 2314–2318. doi: 10.1046/j.0953-816x.2001.01617.x
Sakurai, K., Zhao, S., Takatoh, J., Rodriguez, E., Lu, J., Leavitt, A. D., et al. (2016). Capturing and Manipulating Activated Neuronal Ensembles with CANE Delineates a Hypothalamic Social-Fear Circuit. Neuron 92, 739–753. doi: 10.1016/j.neuron.2016.10.015
Saleeba, C., Dempsey, B., Le, S., Goodchild, A., and McMullan, S. (2019). A student’s guide to neural circuit tracing. Front. Neurosci. 13:897. doi: 10.3389/fnins.2019.00897
Saunders, A., Macosko, E. Z., Wysoker, A., Goldman, M., Krienen, F. M., de Rivera, H., et al. (2018). Molecular Diversity and Specializations among the Cells of the Adult Mouse Brain. Cell 174, 1015.e–1030.e. doi: 10.1016/j.cell.2018.07.028
Scala, F., Kobak, D., Bernabucci, M., Bernaerts, Y., Cadwell, C. R., Castro, J. R., et al. (2021). Phenotypic variation of transcriptomic cell types in mouse motor cortex. Nature 598, 144–150. doi: 10.1038/s41586-020-2907-3
Schwarz, L. A., Miyamichi, K., Gao, X. J., Beier, K. T., Weissbourd, B., DeLoach, K. E., et al. (2015). Viral-genetic tracing of the input-output organization of a central noradrenaline circuit. Nature 524, 88–92. doi: 10.1038/nature14600
Scoville, W. B., and Milner, B. (1957). Loss of recent memory after bilateral hippocampal lesions. J. Neurol. Neurosurg. Psychiatr. 20, 11–21. doi: 10.1136/jnnp.20.1.11
Shapiro, M. G., Frazier, S. J., and Lester, H. A. (2012). Unparalleled control of neural activity using orthogonal pharmacogenetics. ACS Chem. Neurosci. 3, 619–629. doi: 10.1021/cn300053q
Sharpe, M. J., Batchelor, H. M., Mueller, L. E., Gardner, M. P. H., and Schoenbaum, G. (2021). Past experience shapes the neural circuits recruited for future learning. Nat. Neurosci. 24, 391–400. doi: 10.1038/s41593-020-00791-4
Shimomura, O., Johnson, F. H., and Saiga, Y. (1962). Extraction, purification and properties of aequorin, a bioluminescent protein from the luminous hydromedusan. Aequorea. J. Cell. Comp. Physiol. 59, 223–239. doi: 10.1002/jcp.1030590302
Shivange, A. V., Borden, P. M., Muthusamy, A. K., Nichols, A. L., Bera, K., Bao, H., et al. (2019). Determining the pharmacokinetics of nicotinic drugs in the endoplasmic reticulum using biosensors. J. Gen. Physiol. 151, 738–757. doi: 10.1085/jgp.201812201
Shobe, J. L., Claar, L. D., Parhami, S., Bakhurin, K. I., and Masmanidis, S. C. (2015). Brain activity mapping at multiple scales with silicon microprobes containing 1,024 electrodes. J. Neurophysiol. 114, 2043–2052. doi: 10.1152/jn.00464.2015
Siegel, M. S., and Isacoff, E. Y. (1997). A genetically encoded optical probe of membrane voltage. Neuron 19, 735–741. doi: 10.1016/s0896-6273(00)80955-1
Siemian, J. N., Arenivar, M. A., Sarsfield, S., Borja, C. B., Russell, C. N., and Aponte, Y. (2021). Lateral hypothalamic LEPR neurons drive appetitive but not consummatory behaviors. Cell Rep. 36, 109615. doi: 10.1016/j.celrep.2021.109615
Sike, Á, Wengenroth, J., Upīte, J., Brüning, T., Eiriz, I., Sántha, P., et al. (2017). Improved method for cannula fixation for long-term intracerebral brain infusion. J. Neurosci. Methods 290, 145–150. doi: 10.1016/j.jneumeth.2017.07.026
Sohn, J.-W. (2014). Network of Hypothalamic Neurons that Control Appetite. BMB Rep. 48, 229–233. doi: 10.5483/BMBRep.2015.48.4.272
Song, J. H., Lucaci, D., Calangiu, I., Brown, M. T. C., Park, J. S., Kim, J., et al. (2018). Combining mGRASP and Optogenetics Enables High-Resolution Functional Mapping of Descending Cortical Projections. Cell Rep. 24, 1071–1080. doi: 10.1016/j.celrep.2018.06.076
Soudais, C., Laplace-Builhe, C., Kissa, K., and Kremer, E. J. (2001). Preferential transduction of neurons by canine adenovirus vectors and their efficient retrograde transport in vivo. FASEB J. 15, 2283–2285. doi: 10.1096/fj.01-0321fje
Srinivasan, P., Griffin, N. M., Joshi, P., Thakur, D., Nguyen-Le, A., McCotter, S., et al. (2019). An Autonomous Molecular Bioluminescent Reporter (AMBER) for voltage imaging in freely moving animals. BioRxiv [preprint] doi: 10.1101/845198
Steinberg, E. E., Boivin, J. R., Saunders, B. T., Witten, I. B., Deisseroth, K., and Janak, P. H. (2014). Positive reinforcement mediated by midbrain dopamine neurons requires D1 and D2 receptor activation in the nucleus accumbens. PLoS One 9:e94771. doi: 10.1371/journal.pone.0094771
Stellar, S. (1953). a history of neurological surgery. A. earl walker. Q. Rev. Biol. 28, 162–162. doi: 10.1086/399528
Sternson, S. M., and Atasoy, D. (2014). Agouti-related protein neuron circuits that regulate appetite. Neuroendocrinology 100, 95–102. doi: 10.1159/000369072
Stoeckel, K., Schwab, M., and Thoenen, H. (1977). Role of gangliosides in the uptake and retrograde axonal transport of cholera and tetanus toxin as compared to nerve growth factor and wheat germ agglutinin. Brain Res. 132, 273–285. doi: 10.1016/0006-8993(77)90421-8
St-Pierre, F., Marshall, J. D., Yang, Y., Gong, Y., Schnitzer, M. J., and Lin, M. Z. (2014). High-fidelity optical reporting of neuronal electrical activity with an ultrafast fluorescent voltage sensor. Nat. Neurosci. 17, 884–889. doi: 10.1038/nn.3709
Sun, F., Zeng, J., Jing, M., Zhou, J., Feng, J., Owen, S. F., et al. (2018). A genetically encoded fluorescent sensor enables rapid and specific detection of dopamine in flies, fish, and mice. Cell 174, 481.e–496.e. doi: 10.1016/j.cell.2018.06.042
Sun, Y.-C., Chen, X., Fischer, S., Lu, S., Zhan, H., Gillis, J., et al. (2021). Integrating barcoded neuroanatomy with spatial transcriptional profiling enables identification of gene correlates of projections. Nat. Neurosci. 24, 873–885. doi: 10.1038/s41593-021-00842-4
Sundukova, M., Prifti, E., Bucci, A., Kirillova, K., Serrao, J., Reymond, L., et al. (2019). A chemogenetic approach for the optical monitoring of voltage in neurons. Angew. Chem. Int. Ed. Engl. 58, 2341–2344. doi: 10.1002/anie.201812967
Sung, U., Sepehri-Rad, M., Piao, H. H., Jin, L., Hughes, T., Cohen, L. B., et al. (2015). Developing fast fluorescent protein voltage sensors by optimizing FRET interactions. PLoS One 10:e0141585. doi: 10.1371/journal.pone.0141585
Susaki, E. A., Tainaka, K., Perrin, D., Kishino, F., Tawara, T., Watanabe, T. M., et al. (2014). Whole-brain imaging with single-cell resolution using chemical cocktails and computational analysis. Cell 157, 726–739. doi: 10.1016/j.cell.2014.03.042
Suzuki, E., and Nakayama, M. (2011). VCre/VloxP and SCre/SloxP: new site-specific recombination systems for genome engineering. Nucleic Acids Res. 39:e49. doi: 10.1093/nar/gkq1280
Sweeney, S. T., Broadie, K., Keane, J., Niemann, H., and O’Kane, C. J. (1995). Targeted expression of tetanus toxin light chain in Drosophila specifically eliminates synaptic transmission and causes behavioral defects. Neuron 14, 341–351. doi: 10.1016/0896-6273(95)90290-2
Tallini, Y. N., Ohkura, M., Choi, B.-R., Ji, G., Imoto, K., Doran, R., et al. (2006). Imaging cellular signals in the heart in vivo: Cardiac expression of the high-signal Ca2+ indicator GCaMP2. Proc. Natl. Acad. Sci. U.S.A. 103, 4753–4758. doi: 10.1073/pnas.0509378103
Tepe, B., Hill, M. C., Pekarek, B. T., Hunt, P. J., Martin, T. J., Martin, J. F., et al. (2018). Single-Cell RNA-Seq of Mouse Olfactory Bulb Reveals Cellular Heterogeneity and Activity-Dependent Molecular Census of Adult-Born Neurons. Cell Rep. 25, 2689.e–2703.e. doi: 10.1016/j.celrep.2018.11.034
Tervo, D. G. R., Hwang, B.-Y., Viswanathan, S., Gaj, T., Lavzin, M., Ritola, K. D., et al. (2016). A designer AAV variant permits efficient retrograde access to projection neurons. Neuron 92, 372–382. doi: 10.1016/j.neuron.2016.09.021
Tewson, P. H., Martinka, S., Shaner, N. C., Hughes, T. E., and Quinn, A. M. (2016). New DAG and cAMP Sensors Optimized for Live-Cell Assays in Automated Laboratories. J. Biomol. Screen. 21, 298–305. doi: 10.1177/1087057115618608
Tian, L., Hires, S. A., Mao, T., Huber, D., Chiappe, M. E., Chalasani, S. H., et al. (2009). Imaging neural activity in worms, flies and mice with improved GCaMP calcium indicators. Nat. Methods 6, 875–881. doi: 10.1038/nmeth.1398
Tomer, R., Ye, L., Hsueh, B., and Deisseroth, K. (2014). Advanced CLARITY for rapid and high-resolution imaging of intact tissues. Nat. Protoc. 9, 1682–1697. doi: 10.1038/nprot.2014.123
Tye, K. M., Prakash, R., Kim, S.-Y., Fenno, L. E., Grosenick, L., Zarabi, H., et al. (2011). Amygdala circuitry mediating reversible and bidirectional control of anxiety. Nature 471, 358–362. doi: 10.1038/nature09820
Ugolini, G. (1995). Specificity of Rabies Virus as a Transneuronal Tracer. J. Comp. Neurol. 356, 457–480. doi: 10.1002/cne.903560312
Ugolini, G. (2010). Advances in viral transneuronal tracing. J. Neurosci. Methods 194, 2–20. doi: 10.1016/j.jneumeth.2009.12.001
Unger, E. K., Keller, J. P., Altermatt, M., Liang, R., Matsui, A., Dong, C., et al. (2020). Directed evolution of a selective and sensitive serotonin sensor via machine learning. Cell 183, 1986.e–2002.e. doi: 10.1016/j.cell.2020.11.040
Vardy, E., Robinson, J. E., Li, C., Olsen, R. H. J., DiBerto, J. F., Giguere, P. M., et al. (2015). A new DREADD facilitates the multiplexed chemogenetic interrogation of behavior. Neuron 86, 936–946. doi: 10.1016/j.neuron.2015.03.065
Vercelli, A., Repici, M., Garbossa, D., and Grimaldi, A. (2000). Recent techniques for tracing pathways in the central nervous system of developing and adult mammals. Brain Res. Bull. 51, 11–28. doi: 10.1016/S0361-9230(99)00229-4
Verderio, C., Coco, S., Bacci, A., Rossetto, O., De Camilli, P., Montecucco, C., et al. (1999). Tetanus toxin blocks the exocytosis of synaptic vesicles clustered at synapses but not of synaptic vesicles in isolated axons. J. Neurosci. 19, 6723–6732. doi: 10.1523/JNEUROSCI.19-16-06723.1999
Vierock, J., Rodriguez-Rozada, S., Dieter, A., Pieper, F., Sims, R., Tenedini, F., et al. (2021). BiPOLES is an optogenetic tool developed for bidirectional dual-color control of neurons. Nat. Commun. 12:4527. doi: 10.1038/s41467-021-24759-5
Wallace, M. L., Huang, K. W., Hochbaum, D., Hyun, M., Radeljic, G., and Sabatini, B. L. (2020). Anatomical and single-cell transcriptional profiling of the murine habenular complex. eLife 9:e51271. doi: 10.7554/eLife.51271
Wan, J., Peng, W., Li, X., Qian, T., Song, K., Zeng, J., et al. (2021). A genetically encoded sensor for measuring serotonin dynamics. Nat. Neurosci. 24, 746–752. doi: 10.1038/s41593-021-00823-7
Wang, D., He, X., Zhao, Z., Feng, Q., Lin, R., Sun, Y., et al. (2015). Whole-brain mapping of the direct inputs and axonal projections of POMC and AgRP neurons. Front. Neuroanat. 9:40. doi: 10.3389/fnana.2015.00040
Wang, D., Zhang, Z., Chanda, B., and Jackson, M. B. (2010). Improved probes for hybrid voltage sensor imaging. Biophys. J. 99, 2355–2365. doi: 10.1016/j.bpj.2010.07.037
Watanabe, K., Chiu, H., Pfeiffer, B. D., Wong, A. M., Hoopfer, E. D., Rubin, G. M., et al. (2017). A Circuit Node that Integrates Convergent Input from Neuromodulatory and Social Behavior-Promoting Neurons to Control Aggression in Drosophila. Neuron 95, 1112.e–1128.e. doi: 10.1016/j.neuron.2017.08.017
Weston, M., Kaserer, T., Wu, A., Mouravlev, A., Carpenter, J. C., Snowball, A., et al. (2019). Olanzapine: A potent agonist at the hM4D(Gi) DREADD amenable to clinical translation of chemogenetics. Sci. Adv. 5:eaaw1567. doi: 10.1126/sciadv.aaw1567
Whissell, P. D., Tohyama, S., and Martin, L. J. (2016). The use of dreadds to deconstruct behavior. Front. Genet. 7:70. doi: 10.3389/fgene.2016.00070
Wickersham, I. R., Finke, S., Conzelmann, K.-K., and Callaway, E. M. (2007). Retrograde neuronal tracing with a deletion-mutant rabies virus. Nat. Methods 4, 47–49. doi: 10.1038/nmeth999
Wietek, J., Beltramo, R., Scanziani, M., Hegemann, P., Oertner, T. G., and Wiegert, J. S. (2015). An improved chloride-conducting channelrhodopsin for light-induced inhibition of neuronal activity in vivo. Sci. Rep. 5:14807. doi: 10.1038/srep14807
Wietek, J., Rodriguez-Rozada, S., Tutas, J., Tenedini, F., Grimm, C., Oertner, T. G., et al. (2017). Anion-conducting channelrhodopsins with tuned spectra and modified kinetics engineered for optogenetic manipulation of behavior. Sci. Rep. 7:14957. doi: 10.1038/s41598-017-14330-y
Winden, K. D., Oldham, M. C., Mirnics, K., Ebert, P. J., Swan, C. H., Levitt, P., et al. (2009). The organization of the transcriptional network in specific neuronal classes. Mol. Syst. Biol. 5:291. doi: 10.1038/msb.2009.46
Wojaczynski, G. J., Engel, E. A., Steren, K. E., Enquist, L. W., and Patrick Card, J. (2015). The neuroinvasive profiles of H129 (herpes simplex virus type 1) recombinants with putative anterograde-only transneuronal spread properties. Brain Struct. Funct. 220, 1395–1420. doi: 10.1007/s00429-014-0733-9
Won, S. M., Song, E., Reeder, J. T., and Rogers, J. A. (2020). Emerging modalities and implantable technologies for neuromodulation. Cell 181, 115–135. doi: 10.1016/j.cell.2020.02.054
Wu, J., Abdelfattah, A. S., Zhou, H., Ruangkittisakul, A., Qian, Y., Ballanyi, K., et al. (2018). Genetically Encoded Glutamate Indicators with Altered Color and Topology. ACS Chem. Biol. 13, 1832–1837. doi: 10.1021/acschembio.7b01085
Xu, X., Holmes, T. C., Luo, M.-H., Beier, K. T., Horwitz, G. D., Zhao, F., et al. (2020). Viral Vectors for Neural Circuit Mapping and Recent Advances in Trans-synaptic Anterograde Tracers. Neuron 107, 1029–1047. doi: 10.1016/j.neuron.2020.07.010
Yang, H. H., and St-Pierre, F. (2016). Genetically encoded voltage indicators: opportunities and challenges. J. Neurosci. 36, 9977–9989. doi: 10.1523/JNEUROSCI.1095-16.2016
Yang, S. J., Allen, W. E., Kauvar, I., Andalman, A. S., Young, N. P., Kim, C. K., et al. (2015). Extended field-of-view and increased-signal 3D holographic illumination with time-division multiplexing. Opt. Express 23, 32573–32581. doi: 10.1364/OE.23.032573
Yang, W., Carrillo-Reid, L., Bando, Y., Peterka, D. S., and Yuste, R. (2018). Simultaneous two-photon imaging and two-photon optogenetics of cortical circuits in three dimensions. eLife 7:e32671. doi: 10.7554/eLife.32671
Yang, W., and Yuste, R. (2018). Holographic imaging and photostimulation of neural activity. Curr. Opin. Neurobiol. 50, 211–221.
Ye, H., Feng, B., Wang, C., Saito, K., Yang, Y., Ibrahimi, L., et al. (2022). An estrogen-sensitive hypothalamus-midbrain neural circuit controls thermogenesis and physical activity. Sci. Adv. 8:eabk0185. doi: 10.1126/sciadv.abk0185
Yizhar, O., Fenno, L. E., Davidson, T. J., Mogri, M., and Deisseroth, K. (2011). Optogenetics in neural systems. Neuron 71, 9–34. doi: 10.1016/j.neuron.2011.06.004
Zeisel, A., Muñoz-Manchado, A. B., Codeluppi, S., Lönnerberg, P., La Manno, G., Juréus, A., et al. (2015). Brain structure. Cell types in the mouse cortex and hippocampus revealed by single-cell RNA-seq. Science 347, 1138–1142. doi: 10.1126/science.aaa1934
Zemanick, M. C., Strick, P. L., and Dix, R. D. (1991). Direction of transneuronal transport of herpes simplex virus 1 in the primate motor system is strain-dependent. Proc. Natl. Acad. Sci. U.S.A. 88, 8048–8051. doi: 10.1073/pnas.88.18.8048
Zeng, W.-B., Jiang, H.-F., Gang, Y.-D., Song, Y.-G., Shen, Z.-Z., Yang, H., et al. (2017). Anterograde monosynaptic transneuronal tracers derived from herpes simplex virus 1 strain H129. Mol. Neurodegener. 12:38. doi: 10.1186/s13024-017-0179-7
Zhan, C., Zhou, J., Feng, Q., Zhang, J.-E., Lin, S., Bao, J., et al. (2013). Acute and long-term suppression of feeding behavior by POMC neurons in the brainstem and hypothalamus, respectively. J. Neurosci. 33, 3624–3632. doi: 10.1523/JNEUROSCI.2742-12.2013
Zhang, L., Liang, B., Barbera, G., Hawes, S., Zhang, Y., Stump, K., et al. (2019). Miniscope GRIN Lens System for Calcium Imaging of Neuronal Activity from Deep Brain Structures in Behaving Animals. Curr. Protoc. Neurosci. 86:e56. doi: 10.1002/cpns.56
Zhao, Y., Araki, S., Wu, J., Teramoto, T., Chang, Y.-F., Nakano, M., et al. (2011). An expanded palette of genetically encoded Ca2+ indicators. Science 333, 1888–1891. doi: 10.1126/science.1208592
Zhou, P., Reimer, J., Zhou, D., Pasarkar, A., Kinsella, I., Froudarakis, E., et al. (2020). EASE: EM-Assisted Source Extraction from calcium imaging data. BioRxiv [preprint] doi: 10.1101/2020.03.25.007468
Zhu, C., Jiang, Z., Xu, Y., Cai, Z.-L., Jiang, Q., Xu, Y., et al. (2020). Profound and redundant functions of arcuate neurons in obesity development. Nat. Metab. 2, 763–774. doi: 10.1038/s42255-020-0229-2
Zhu, H., Pleil, K. E., Urban, D. J., Moy, S. S., Kash, T. L., and Roth, B. L. (2014). Chemogenetic inactivation of ventral hippocampal glutamatergic neurons disrupts consolidation of contextual fear memory. Neuropsychopharmacology 39, 1880–1892. doi: 10.1038/npp.2014.35
Zingg, B., Peng, B., Huang, J., Tao, H. W., and Zhang, L. I. (2020). Synaptic specificity and application of anterograde transsynaptic AAV for probing neural circuitry. J. Neurosci. 40, 3250–3267. doi: 10.1523/JNEUROSCI.2158-19.2020
Zong, W., Wu, R., Li, M., Hu, Y., Li, Y., Li, J., et al. (2017). Fast high-resolution miniature two-photon microscopy for brain imaging in freely behaving mice. Nat. Methods 14, 713–719. doi: 10.1038/nmeth.4305
Keywords: neural circuit tracing, cell type-specific, viral genetic technology, calcium/voltage indicator imaging, neurotransmitter/biosensors, chemogenetics, optogenetics, targeted ablation
Citation: Swanson JL, Chin P-S, Romero JM, Srivastava S, Ortiz-Guzman J, Hunt PJ and Arenkiel BR (2022) Advancements in the Quest to Map, Monitor, and Manipulate Neural Circuitry. Front. Neural Circuits 16:886302. doi: 10.3389/fncir.2022.886302
Received: 28 February 2022; Accepted: 27 April 2022;
Published: 26 May 2022.
Edited by:
Petr Tvrdik, University of Virginia, United StatesReviewed by:
Ayumu Inutsuka, Jichi Medical University, JapanTommaso Patriarchi, University of Zurich, Switzerland
Copyright © 2022 Swanson, Chin, Romero, Srivastava, Ortiz-Guzman, Hunt and Arenkiel. This is an open-access article distributed under the terms of the Creative Commons Attribution License (CC BY). The use, distribution or reproduction in other forums is permitted, provided the original author(s) and the copyright owner(s) are credited and that the original publication in this journal is cited, in accordance with accepted academic practice. No use, distribution or reproduction is permitted which does not comply with these terms.
*Correspondence: Benjamin R. Arenkiel, arenkiel@bcm.edu
†These authors have contributed equally to this work