- 1Department of Pathology and Immunology, Baylor College of Medicine, Houston, TX, United States
- 2Program in Developmental Biology, Baylor College of Medicine, Houston, TX, United States
- 3Jan and Dan Duncan Neurological Research Institute of Texas Children’s Hospital, Houston, TX, United States
- 4Department of Neuroscience, Baylor College of Medicine, Houston, TX, United States
- 5Department of Anatomy and Neurobiology, University of Tennessee Health Science Center, Memphis, TN, United States
The brain contains a large diversity of unique cell types that use specific genetic programs to control development and instruct the intricate wiring of sensory, motor, and cognitive brain regions. In addition to their cellular diversity and specialized connectivity maps, each region’s dedicated function is also expressed in their characteristic gross external morphologies. The folds on the surface of the cerebral cortex and cerebellum are classic examples. But, to what extent does structure relate to function and at what spatial scale? We discuss the mechanisms that sculpt functional brain maps and external morphologies. We also contrast the cryptic structural defects in conditions such as autism spectrum disorders to the overt microcephaly after Zika infections, taking into consideration that both diseases disrupt proper cognitive development. The data indicate that dynamic processes shape all brain areas to fit into jigsaw-like patterns. The patterns in each region reflect circuit connectivity, which ultimately supports local signal processing and accomplishes multi-areal integration of information processing to optimize brain functions.
Introduction
The brain is responsible for a seemingly endless number of behaviors that require cognition, sensation, and action. How the brain processes information from its environment, develops thoughts, weighs emotions, and drives the repertoire of responsive behaviors depends on a precisely coordinated interaction of different structures, each with its own specialized functions. The cerebral cortex, subcortical structures, and the cerebellum broadly plan behaviors by controlling emotions, organizing and interpreting external and internal sensory information, forming memories, maintaining homeostasis, coordinating appropriate muscle activity, and instructing language. Brain functions are clearly diverse; therefore, it makes sense that none of the brain regions have morphologies that look alike from the outside and that their networks are comprised of distinct layers and cell types. However, there are many genetic, cellular, molecular, and developmental processes that are shared between brain regions. Given that these similarities are superimposed upon a multitude of differences, can structure tell us anything about function?
While the answers to these questions might, at first glance, seem to be an overwhelming “yes,” there is not always clear linkage between structure and function for many brain areas. To address this problem, one must consider the developmental mechanisms that generate the brain. The central nervous system arises from a simple neuroepitehlium that is initially unremarkable in its specificity along its rostral-caudal axis. Gene function during embryogenesis transforms the neuroepithelium into distinct domains that will form particular brain regions. It is at this stage of development that one may ask how structures are uniquely shaped to acquire their final function. Cells in each region begin to proliferate according to a specific spatial and temporal timetable. At least two classes are produced: neurons and glia. The glia serve as a lineage source for neurons, but they also form the cellular substrate for neuronal migration to occur. Therefore, depending on the classes of neurons and glia and their organization, it has been argued that the suggested ratio of 90% glia to 10% neurons in the mammalian brain could provide clues as to how the brain acquires its intricate morphologies. But, this ratio has been challenged recently, suggesting a ratio closer to 50:50 (Herculano-Houzel, 2014). Moreover, although relatively simple in form, the drosophila melanogaster brain only has 10% glia (Kremer et al., 2017), yet there is still considerable complexity in the fly brain that includes intricate folds and specialized functions. But, why fold in the first place? It may be argued that massive regions such as the cerebral cortex must fold in order to accommodate the sheer quantity of critical circuits. However, the cerebellum contains fewer types of circuits (certainly at the basic anatomical level, although this could be challenged based on molecular complexity, as discussed later), yet it has more neurons than any other part of the brain. Based on these problems, we then face the question, what drives the folding, and are there equivalent mechanisms in different parts of the brain? In this review, we address these issues and take into account that each brain region contains an array of distinct cell types with unique morphologies, densities, and functions, and we also consider how neurons migrate and how their axons are guided into precise locations to form brain networks. We ask, what physical forces assemble these network components into a brain region (Garcia et al., 2018)? We discuss how functionality is assembled across brain regions and how neural circuits link up into previously unappreciated wiring diagrams that are critical for behavior. Our attempt is not to solve every question and conundrum in the field of cerebellar and cerebral cortical folding. Instead, our efforts are to take a wholistic view of how the brain is packaged from the outside to its inside, and to stimulate a discussion about how one level of complexity, be it cellular or molecular, feeds into the next, in developing and adult circuits. This view might also teach us about brain function.
Main Text
Multiple Levels of Heterogeneity in the Brain
Regional Variation and Specificity
Historically, the cerebral cortex and cerebellum have been extensively studied for their structures and functions. For example, Brodmann (1909) identified 43 areas in the human brain based on the general cytoarchitecture of the cerebral cortex. After taking into account regional cellular composition and density, Penfield (1968) considered the functional contributions of the cerebral cortex, elucidating the areas responsible for processing somatosensory, visual, auditory, and motor information through producing an exhaustive functional map based on responses to stimulation techniques that he pioneered for therapy in epilepsy. Thereafter, impairments to these cortical subdivisions have been linked to a large number of diverse developmental and pathological features of brain disease.
Cerebellar studies also have a rich and fascinating history (Manto, 2008), with suggestions of functional topography dating back to the early 1900s (Bolk, 1906). The cerebellar cortex, which is often described as having a uniform cellular composition, is in fact heterogeneous in its molecular properties. As we will discuss later, the cerebellar cortex is divided into an array of parasagittal patterns that segment all of its cell classes. Importantly, however, the molecular patterning is accompanied by anatomical divisions, both in the cerebellar cortex (Ozol and Hawkes, 1997) and the white matter (Voogd and Ruigrok, 1997). Indeed, much like the cerebral cortex, the different domains within the cerebellum reflect developmental (Sillitoe and Joyner, 2007; Apps and Hawkes, 2009; Apps et al., 2018), functional (Cerminara et al., 2015), and pathological features (Sarna and Hawkes, 2003). Strikingly, each of these medial-lateral patterning properties is superimposed upon a broader anatomical plan that is also segmented upon the same axis. From medial to lateral the vermis, paravermis, and hemispheres occupy distinct locations, have specific folding architectures, contain specific circuits, and contribute to largely different behaviors.
Findings in the cerebral cortex and cerebellum have been complemented by studies into understanding the unique structures and functions characteristic to other areas such as the hippocampus. We will not review the thousands of studies devoted to hippocampal functional specificity, but suffice it to say that a large body of work has revealed an intricate and remarkable segmentation of its functions (Hitti and Siegelbaum, 2014; Kohara et al., 2014; Okuyama et al., 2016). Support for these modern animal model studies, like many sectors of neuroscience, came from older studies from human patients and the field of psychiatry. The concept that the cerebral cortex segments and shuttles information into distinct brain regions and that this process can be compromised in disease was found to be shared with the hippocampus. For example, the resulting amnesia after lesioning the hippocampus revealed its critical roles in memory formation (Scoville and Milner, 1957). The main issue to consider, as a starting point, is that each unique brain structure appears to come equipped with a unique set of capabilities. The question we ask is how do these capabilities arise, and are there common mechanistic themes for how they arise?
Cell Layering and Connectivity
One common feature between the cerebral cortex, cerebellum, and hippocampus is that they all have an exquisite layering of cells. Importantly, the layering in all three structures is disrupted in mice that lack REELIN and related proteins (Howell et al., 1997). These data indicate a common requirement for specific genetic programs and at least some shared dependence on developmental processes such as neuronal migration (Wasser and Herz, 2017). Still, each structure must use these “common” cues to assemble unique circuits. The layering and interactions of cells between the layers of the cerebellar cortex are a prime example. To appreciate these ideas, it is useful to recall that connectivity within the cerebellum is understood at a considerable level of detail, with each cell type forming stereotypical connections with its neighbors. The cerebellum has three distinct layers, and for comparison, the much more complicated cerebral cortex has six main layers. The most superficial cerebellar layer contains inhibitory interneurons and excitatory parallel and climbing fibers. Both project onto Purkinje cells, which make up the middle layer called the Purkinje cell layer. The Purkinje cells perform the main computations in the cerebellum. The deepest layer is called the granular layer and it contains millions of excitatory neurons called granule cells as well as mossy fibers that deliver sensory signals to the Purkinje cells. Below the three layers is a dense network of fiber tracks. Embedded in this network are the cerebellar nuclei. The cerebellar nuclei are specialized neurons that transmit the final output of the cerebellum. They link the cerebellum to the rest of the brain and spinal cord. Early studies of the cerebellum revealed an incredible level of structural and functional variation in the circuitry as was found in the cortex (Fox and Snider, 1967). Work from Marr (1969) and Albus (1971) used this structural map of the cerebellum, and at that time the quickly emerging details of its functional connectivity (Eccles, 1965), to postulate theories on its computational power over motor control. Given that the cerebellum has one neuronal population responsible for the output of its cortex, the Purkinje cell, and that this cell type is innervated by inputs in a predictable, reproducible pattern, the cerebellum is thought to execute a multitude of motor behaviors by modulating Purkinje cell spiking and the downstream consequences on cerebellar nuclear neuron firing (Marr, 1969; Gilbert, 1974; Ruigrok, 2011). It is also important to note that Purkinje cells project to distinct cerebellar output nuclei as revealed by the pattern of axonal projections, compartmental expression of molecules, and functional designation. These combined features further subdivide the cerebellum and potentially add complexity to its computational capabilities (Ruigrok, 2011; Apps et al., 2018; Miterko et al., 2018). In a similar manner, but for different behavioral consequences, the hippocampus is separated into distinct areas [cornu ammonis (CA) fields, dentate gyrus, and subiculum] and layers that are conceptually reminiscent of those in the cerebellum, where cells are organized in a predictable, spatial pattern (Arbib et al., 1998). This particular organization is thought to promote information processing and neuronal coupling (Arbib et al., 1998) to support different non-motor and motor behaviors.
Functional Specializations
The heterogeneity of the brain, as exemplified by these three examples—the cerebral cortex, cerebellum, and hippocampus—spans a great number of structures and their associated functions. Among the anatomical differences, there are also physiological and chemical differences that affect circuit formation and function. Nonetheless, what each of these three brain regions have are subdivisions, which is theorized to support their functions. Comparative analyses upheld this belief, adding to it that as mammals evolved, so did brain structure to accommodate higher order functions. This manifested in a trend in toward a greater subdivision of the cerebral cortex into functionally distinct areas, where early mammals likely had on the order of 20 distinct cortical areas while humans may have more than 200 distinct cortical areas (Kaas, 2013). Neuroimaging of the human cerebellar cortex also reveals functional topography spanning not only motor control but also specific higher order limbic and cognitive tasks, including lateralization of language-related activity (Stoodley and Schmahmann, 2009).
Modules and Maps
How should we determine whether structural features of the brain constitute functionally separate areas or nuclei, without under-dividing or over-dividing? Kaas (1982) proposed five criteria to test for this problem: that distinct cortical areas and nuclei should have differences in cytoarchitecture, a relatively complete single representation of the sensory surface, unique inputs and outputs, different response properties, and dissociable behavioral impairments after lesions. In the cerebellum, the repeating Purkinje cell circuit is subdivided into olivocorticonuclear modules, which also contain micromodules that could either represent distinct or combinations of functional entities (Apps et al., 2018). For instance, lesions made into distinct olivary sub-nuclei, key components of the cerebellar modules, result in specific behavioral deficits during movement (Horn et al., 2010). Moreover, in vivo recordings in mice and rats demonstrate different Purkinje cell firing properties that are dependent on location within the cerebellar cortical patterns (Xiao et al., 2014; Zhou et al., 2014). However, a more fundamental feature that has been a useful and reliable landmark for locating distinct areas is the overlying brain morphology. Most notably, the folds overlying the cerebral cortex and cerebellum. The relationship between external morphology and functional organization has also been essential for studying the brain functions of extinct species, as we are only able to examine skull endocasts. The cerebral cortex contains a series of gyri that are separated by sulci, whereas the cerebellum has lobules separated by fissures (Figure 1). Cortical folds are consistent across individuals (and between some mammalian species), whereas cerebellar folds are highly conserved as the major pattern across species, from birds through mammals (Larsell, 1970). In primates, larger brains preserve neuronal packing density and generally have more and deeper folds (Herculano-Houzel et al., 2007), which are especially evident in the massive and proportional expansion of the human neocortex and cerebellar cortex. There has been a long-standing debate as to how folding is accomplished, and how it might relate to brain function (Mota and Herculano-Houzel, 2015). We next discuss and debate some of the theories and experimental evidence.
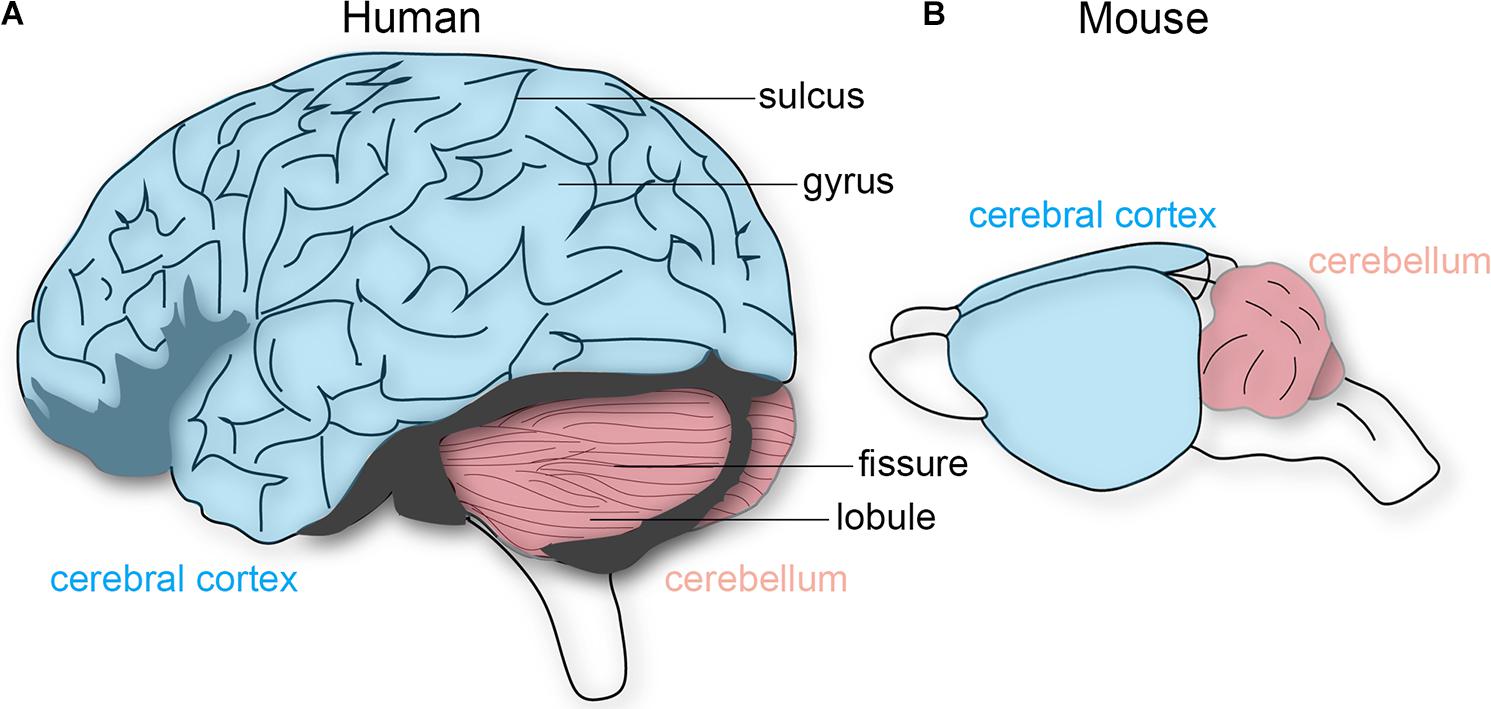
FIGURE 1. Schematic depiction of the human and mouse brain. (A) The human cerebral cortex (blue) and cerebellum (pink) both have characteristic folding patterns. The cerebral cortex contains gyri (peaks) that are interrupted by sulci (valleys). An equivalent architecture is observed in the cerebellum, which is comprised of lobules and fissures. (B) The mouse cerebellum is folded whereas the cerebral cortex is not. The brains are not drawn to scale.
Mechanics of Folding a Neural Sheet
In recent years, much focus has been placed on testing the tension theory proposed by Van Essen (1997). The general tenet of his theory is that cortico-cortical axons physically pull on regions of the cortex and result in gyri formation, and almost by default, the sulci form as well. Other prominent theories, such as the radial gradient hypothesis, propose that an increase in the expansion of supragranular cortical layers relative to the infra-granular layers causes buckling of adjacent regions (Richman et al., 1975). In the differential tangential expansion hypothesis, it is proposed that tangential expansion of the cortex causes an increase in tangential pressure, and as a consequence buckling acts to reduce the pressure (Le Gros Clark, 1945; Ronan et al., 2013). In this case, the theory leans on Brodmann’s findings on cytoarchitectural differences, which allow for pattern-specific folding. Following this theory, the stability of folding patterns reflects the stability of expansion forces in a given region. It is worth mentioning that these theories share the idea that cortical folding upon itself is necessary for packing the large brain into the small skull, an idea that could be challenged by experimental evidence almost 70 years ago (Barron, 1950).
Moreover, empirical studies of the cerebral cortex argue against features of these theories, and an emerging counter argument is that the timing of developmental events simply could not facilitate tension or buckling (Ronan and Fletcher, 2015). We could make similar arguments for the cerebellum. Van Essen (1997) posits that tension along parallel fibers, the axons of granule cells, could explain why the cerebellar cortex is highly elongated but also folded like an accordion into lobules. Arguing against Van Essen’s tension-based mechanism is the fact that cerebellar cortical folding starts embryonically whereas most parallel fibers form postnatally, and, despite the number of fiber tracts entering, no one class could drive the folding because afferents enter the cerebellum starting from mid-embryogenesis and continue to sequentially invade the structure throughout postnatal development (White and Sillitoe, 2013). Moreover, the several theories above do not take into account the highly dynamic nature of circuit formation as fibers are also pruned away, and the target cells—not the axons—are key regulators of wiring processes that should also impact tension and buckling based on these theories (Uesaka et al., 2014). One attractive idea, based on the original hypothesis of Altman and Bayer (1997), is that the shape of folds is driven by the precise timing of the appearance of specialized “anchoring centers” at the future base of each cerebellar fissure and the subsequent coordinated proliferation and migration of granule cells down Bergmann glia astrocytes (Sudarov and Joyner, 2007). But, the fact that cerebellar folds are well conserved and reliably patterned in every animal points to genetic mechanisms that support what might be a functional necessity. Indeed, sonic hedgehog (SHH) morphogen signaling and engrailed transcription factor function, among other pathways (Ryan et al., 2017), could play integrated roles in shaping the cerebellum (Sudarov and Joyner, 2007; Blaess et al., 2008).
The idea of neuronal proliferation driving folding extends to the cerebral cortex as well (Hevner, 2006; Ronan and Fletcher, 2015; Sun and Hevner, 2016). However, it is not just the progenitors that exhibit spatial organization. It was postulated that perhaps folds encompass units with functional restriction (Welker, 1990). Genetic clonal analysis uncovered a modular mode of development for cerebellar granule cells, with lineages restricted within folds (Legue et al., 2015). In the cerebral cortex, neurons born from a common lineage form local clusters that migrate into distinct cortical, hippocampal, and striatal regions (Sultan et al., 2016); although this idea is challenged by Mayer et al. (2016). These early patterns are reminiscent of protomaps (Rakic et al., 2009). More importantly, developmental clusters produce the maps required for adult function and behavior. In the cerebellum, clusters might not directly determine function, but we know they are part of a framework for establishing topography, which is important for function. There is also a possibility that clusters, and the genes that are differentially expressed within each subset, instruct the cellular and molecular properties that eventually produce the diversity of cerebellar-related behaviors.
Still, there are many features of the theories put forth by Van Essen et al. (2018) that could beautifully explain a number of processes required for cerebral cortical and cerebellar folding, especially from the view of evolution. It is likely that these and other ideas (Na(V), Smith et al., 2018; extracellular matrix, Long et al., 2018) will merge to unveil the full explanation for how neural tissue accomplishes its folding (Meng et al., 2018). In addition, perhaps it is time to consider new model systems (Matsumoto et al., 2017). Recent work used human microcephaly as a starting point for understanding cortical size and complexity. Knowing that mutations in the abnormal spindle-like microcephaly-associated (Aspm) gene are common in the human disease but have surprisingly little impact on mouse cortex, Johnson and colleagues developed an Aspm knockout ferret (Johnson et al., 2018). The rationale for choosing this model was the more complicated gryrification of the cerebral cortex compared to rodents and the greater diversity of neuronal progenitors. In the Aspm model, the approach proved fruitful as they uncovered essential roles for radial glia in controlling cortical expansion. It would be interesting to examine the role of Aspm in the ferret cerebellum, considering the milder, but evident cerebellar defects (Johnson et al., 2018).
Topography Relates to Function
Folds roughly correlate to functional domains. In the cerebral cortex, the four principle lobes each have a predominant set of functions. Different sensory, motor, and cognitive behaviors are mapped to distinct regions (a famous correlate was the removal of the hippocampi in patient HM and the resulting specific inability to form new memories), and in some cases, each with specific cytoarchitectures. A remarkable evolutionary adaptation reflecting the structure-function relationship is seen in star-nosed moles, which have 22 fleshy foraging appendages that ring their nostrils. Each appendage is represented in the somatosensory cortex, where they form a “cortical star” that mimics the structure (Catania, 2012). An equivalent topographical mapping of sensory representations onto the cortical surface is revealed in ocular dominance columns in the visual cortex (Hubel and Wiesel, 1979), barrels in the somatosensory cortex (Li and Crair, 2011), patch matrix compartments in the striatum (Bloem et al., 2017), and odorant maps in the olfactory system (Bozza et al., 2002). One of the best-studied examples of brain organization is the Purkinje cell zonal map (Figure 2; Miterko et al., 2018). The topographic inputs and outputs of Purkinje cell zones form functional modules (Ruigrok, 2011). Gene expression reveals patterns of stripes that demarcate the modules (cerebellar stripe patterns are obvious in Purkinje cells; Figure 2). Modules are derived from lineage patterning mechanisms that instruct specific classes of sensory afferents to target particular regions of the cerebellum (and to some extent particular folds). Modules are therefore comprised of neurons, glia, and terminals that are wired together with a specific topography. It is proposed that modules are organized into patterns that provide an efficient packaging framework for circuits to encode behaviors and that could allow parallel processing of information during complex behaviors (Horn et al., 2010). From a developmental perspective, one could ask what are the embryonic origins of two adjacent circuits that perform complementary functions during a given behavior? For instance, the hindlimb and forelimb cooperate during locomotion, and it is not a coincidence that limb sensory axons are placed side by side in the module map (Gebre et al., 2012). The wiring of the cerebellar map is genetically determined (Sillitoe et al., 2010) and sculpted to precision by activity (White et al., 2014), and the same is true for retinotopic maps in the visual cortex (Cang et al., 2008). The topographic structure of the input and output pathways as well as their internal maps (e.g., Purkinje cell patterns) is therefore intimately linked to circuit function and the behaviors that the circuits support.
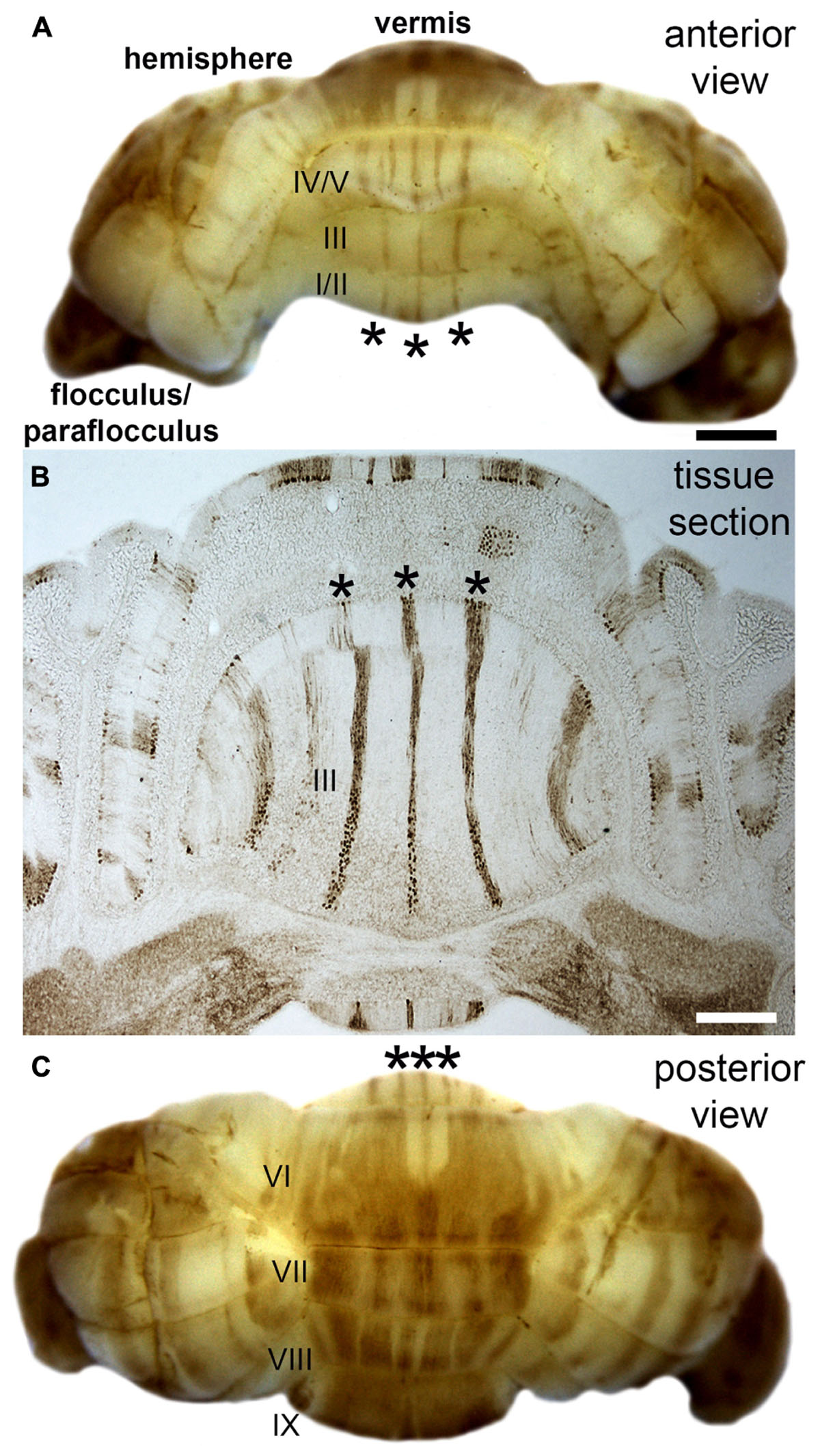
FIGURE 2. Purkinje cell patterning in the adult mouse cerebellum. (A) Anterior view of the mouse cerebellum stained in wholemount with a monoclonal antibody to zebrin II/aldolaseC. (B) Coronal tissue section cut through the anterior cerebellum illustrating the pattern of zebrin II stripes in the anterior lobules (asterisks in all panels). Although the intervening stripes are not immunoreactive for zebrin II, they do express other markers that define this complementary set of stripes. (C) Posterior view of the mouse cerebellum stained in wholemount with zebrin II. The lobules in the vermis are labeled with Roman numerals according to the nomenclature of Larsell (Larsell, 1970). Scale bar in A = 1 mm (applies to C), and the scale bar in B = 500 μm. The images were modified with permission from White et al. (2012).
Taking Shape in Space and Time
Besides folds, brain and region size is another important feature established during development that is thought to influence global and local function. As folds became increasingly more complex, and potentially co-evolving with the necessity for higher order brain functions, the need for larger mammalian brains possibly arose. Accompanying this increase in brain size is an increase in the number of neurons and glia, and an increase in the diversity within each cell class. More specifically, it is thought that the increase in the number of astrocytes is to preserve its roles of synaptogenesis and regulating metabolic demand in a more complex and robust neural environment (Nedergaard et al., 2003; Oberheim et al., 2006).
Individual brain regions also enlarged as a result of adaptation. For example, the neocortex in primates exponentially increased in size (Finlay and Darlington, 1995) and the hippocampus of foraging birds enlarged as the need for improved social interaction and spatial memory mounted (Sherry et al., 1992). Epigenetic events during development, such as loss of a sensory organ (Kahn and Krubitzer, 2002), have been shown to influence the size and function of cortical areas, though within limits established by genetic programs. The observation that magnification has accompanied the acquisition of complex behaviors raises the question of how regions expand during development, and with this process again comes the obligatory issue of folding. Genetic programs during development create balance between proliferation and cell death, and when genes such as LIS1, DCX, ASPM, EMX2, FGF, and Gpr56 affect the normal processes of neuron and glia formation and migration, a host of conditions including lissencephaly and polymicrogyria ensue (Ronan and Fletcher, 2015). Interestingly, defective SHH signaling, one of the most powerful morphogens in mammalian development and an essential component of cerebellar folding during late embryogenesis and early postnatal development in mice (Corrales, 2006), also controls cerebral cortical growth and folding by modulating cell proliferation (Wang L. et al., 2016; Wang Y. et al., 2016). It should therefore not be surprising that the outcome of conditions with defective SHH signaling can be extremely severe, as is the case when the catalytic subunit of phosphoinositide 3-kinase (PIK3CA) is mutated causing brain and body overgrowth. In the brain, PIK3CA-induced overgrowth causes bilateral dysplastic megalencephaly, hemimegalencephaly, and focal cortical dysplasia, the most common cause of intractable pediatric epilepsy (Roy et al., 2015).
Clear behavioral symptoms also result from the reversed problem, i.e., when reduced growth is triggered, as in the case of reeler mutations, the result is a small brain with no folds. In this scenario, the organized layering of the cerebral cortex, hippocampus, and cerebellum is also disrupted (Sudarov et al., 2011; Guy and Staiger, 2017). A recent epidemic with similar consequences is Zika virus infection, which causes microcephaly with cognitive disability. The Zika virus impairs Musashi-1 function in neural progenitors, leading to decreased neurogenesis in both the cortex and the dentate gyrus and therefore an overall decrease in size (Chavali et al., 2017; Christian et al., 2018). Moreover, the Zika virus disorganizes the granule cell layer and strata, in addition to predisposes infants to brain calcifications (Christian et al., 2018). Accompanying these structural deficits to the brain are insults to neural circuit development. The Zika virus promotes gliosis, impairs myelination, disrupts synaptogenesis, and alters the levels of SHH and FGF (Christian et al., 2018; Thawani et al., 2018). But, during normal development it should be noted that even before secreted molecules such as SHH, FGF, and REELIN impact cell placement, lineage history and birthdate initiate a spatial and temporal order of cellular invasion of a territory (Sudarov et al., 2011). In addition, in the cerebral cortex, arealization maps the areas of invasion by a transcription factor-mediated process (O’Leary et al., 2007) that can also involve repurposed axon guidance cues in rodents and primates (Homman-Ludiye and Bourne, 2014).
Axon guidance itself plays a multitude of roles in shaping structures for function. For instance, functional systems are determined by how effective pioneer tracts are in setting up circuits, how guidepost cells mediate the proper trajectory of axons, and how chemoaffinity cues such EPH-EPHRIN and cadherin signaling establish circuit maps through axon-target recognition. Interestingly, progenitors not only drive proliferation for regional expansion, but recent evidence indicates that they also produce the NETRIN guidance cue for proper long-range targeting of axons (Dominici et al., 2017; Varadarajan et al., 2017). Remarkably, yet an additional role for SHH is to mediate axon-axon guidance to shape structure into the optic chiasm crossing (Peng et al., 2018), a necessary step in forming the precise retinotectal topography that underlies vision.
It is interesting to speculate that changes in these molecular processes and axon routing could account for the subtle but significant variations in the sizes of brain regions observed in different strains of inbred mice (Hikishima et al., 2017). To solve how this occurs, dissecting the impact of a large number of intersecting genetic pathways in vivo would be impractical. However, one could consider highlighting the relationship between structure and function, even within the context of folding, in a disease-relevant model using organoids. The cellular, molecular, and structural features of Zika, and the more basic mechanisms of brain folding, were rapidly worked out with organoids (Cugola et al., 2016; Garcez et al., 2016; Qian et al., 2017; Karzbrun et al., 2018). Perhaps human organoids could also be helpful in developmental conditions in which the structural defects are unclear yet function is perturbed. One example is hydrocephalus. Hydrocephalus is a severe developmental disorder characterized by an excessive build-up of CSF, which causes pressure on the surrounding tissue. In rare cases, the hydrocephalus may be spontaneously corrected such that brain scans no longer show the ventricular enlargement (Dreazen et al., 1989). This is surprising because hydrocephalus alters hindbrain shape, and if shape is no longer defective, then endogenous repair mechanisms could be at play. This is intriguing because recent work shows that a specific progenitor population in the cerebellum can rescue granule cells post-injury (Wojcinski et al., 2017).
More complicated are conditions such as autism with overt behavioral deficits and covert structural deficits. While a reduction in gray matter volume has been found in the cerebellum, hippocampus, amygdala, and parietal lobe of children with autism spectrum disorder (ASD), others have reported an overall increase in brain volume as a hallmark feature of ASD (Carlisi et al., 2017; Riddle et al., 2017). Studies on the underlying genes associated with ASD pathogenesis suggest that a range of functions are disrupted such as synaptic maintenance and motor control (Antoine et al., 2018; Golden et al., 2018; Tatavarty et al., 2018). However, inconsistent structural findings and the seemingly endless number or combination of genetic causes have complicated the process of studying function in autism and other diseases. As a result, the development of new therapeutic approaches has been modest. Recent methodological advances could hold promise.
Organoids and iPSC approaches could be tailor-made to address the mechanism of diseases with either structural or circuit-based defects. In their remarkable study, Orly Reiner and colleagues were inspired by physics and tackled the problem of cortical folding using organoids. They used “wrinkling” as a proxy for folding and uncovered that the process is driven by mechanical instability (Karzbrun et al., 2018). Furthermore, they showed in their organoid system that a LIS1+/− mutation reduced wrinkling, and at the cellular level, the defects arose through a combination of defects in nuclear motion, extracellular matrix, and cytoskeleton. A major question to answer—and model—is whether circuit function could be fully restored if the structure of the developing brain is rescued in time. In addressing this question, it could be that the regional differences in stem cell and progenitor population number and capabilities have to be considered. For instance, radial glia-like cells in the outer subventricular zone could promote cortical folding complexity (Fietz et al., 2010; Hansen et al., 2010; Wang et al., 2011). It would be interesting if the inner versus outer granule cell progenitors in the external granular layer have differential effects on cerebellar lobulation. Moreover, there may be a great number of genes, such as ARHGAP11A (which encodes a Rho guanosine triphosphatase-activating protein), that control the species-specific complexity of folding (Florio et al., 2015), not only in cortex but other regions as well. To disentangle whether function could be rescued if folding is corrected, the timing of when the activity of such progenitors or genes is initiated is critical.
Structure-Function Reciprocity
Evidently, injury to the brain and more specifically to regions such as the cortex and cerebellum has severe consequences on function (Dahlem et al., 2016). In addition to the direct consequences of missing an area, one has to consider that compensation for loss of function, even in long-range circuits such as the brain to spinal cord connectivity or cerebro-cerebellar connectivity, adds considerable complexity to the structure-function relationship (Hollis et al., 2016). In stroke, the functional improvements are dependent on structural plasticity that induces changes at the synapse (Cooperrider et al., 2014). Indeed, in the cerebellum, topographic molecular mechanisms determine spatial patterns of synaptic plasticity (Wadiche and Jahr, 2005). Synaptic connectivity also follow an architectural heterogeneity of the targets. For example, despite the textbook view of a canonical Purkinje cell, these cells vary in their form and function depending on where in the cerebellum they are located (Cerminara et al., 2015). Purkinje cells have different dendritic structures depending on where they are in a fold. And, at the action potential level, subsets of Purkinje cells have very unique functional (firing) properties that align almost perfectly with the modular stripe topography of the cerebellar cortex (Xiao et al., 2014; Zhou et al., 2014).
Similarly, one can imagine that that cellular diversity and the dense connectivity plan between cerebral cortical cells should impact computations (Jiang et al., 2015), especially when synaptic structural variations, such as size and neurotransmitter phenotype, are taken into account (Larramendi et al., 1967). We can also no longer ignore that glial diversity will shape synaptic output (John Lin et al., 2017). In the cerebellum, loss of AMPA receptors in Bergmann glia results in motor coordination defects (Saab et al., 2012). Bergmann glia are patterned according to Purkinje cell stripes (Reeber et al., 2018) and are critical for foliation (Ma et al., 2012; Li et al., 2014). It would therefore be interesting to consider whether the three-dimensional plan of the cerebellum with its Cartesian coordinate-like map (Sillitoe and Joyner, 2007) uses Bergmann glia heterogeneity and their influence over neuronal activity to help sculpt the folding and shape of the cerebellum. Could the anchor centers at the base of each fold be defined by particular stripe and zone features? And, are these features unique to each fold? Perhaps the glia-neuron heterogeneity plays a role in differentiating the vermis from hemisphere folds, which require additional curvature even seen in the mouse cerebellum. Certainly, in addition to the differences in functional roles of the vermis compared to the hemispheres, there exists developmental (Govek et al., 2018) as well as disease related distinctions (Tan et al., 2018) that drive shape. It is intriguing to speculate that the initiation and growth of the additional folia and sub-folia in higher order mammals could be facilitated by cellular and molecular heterogeneity in the highly conserved cerebellar map (Sillitoe et al., 2005; Marzban and Hawkes, 2011), and perhaps other classes of glia such as oligodendrocytes and NG2+ cells could in theory promote specific aspects of folding over time. Might there be equivalent features of heterogeneity in the cerebral cortex and hippocampus to support their folding? There is certainly enough heterogeneity for this to be possible.
Behavioral Implications
Considering the above findings together, we are reminded that cellular and structural heterogeneity, even in structures with as high a regional diversity as the cerebral cortex, map to a fundamental functional plan (Mountcastle, 1957). But there is a problem raised by this heterogeneity in that how do these different circuits work together to ultimately drive behavior? This problem is in part solved by neuronal synchrony. Whether it is in the cerebral cortex, cerebellum, or another area, circuits that are linked by cell lineage or connectivity can be synchronously activated in a manner that provides flexibility in controlling behavior (Welsh et al., 2002). But, does long-range connectivity between brain areas with different canonical circuit structures allow for different types of plasticity to work together to influence behavior (Caligore et al., 2017)? Such a model would provide one mechanism for increasing functional flexibility.
Also, localized cellular diversity in each cortical column or cerebellar module could add computational diversity (Figure 3). These discrete functional features are built into the brain, but some degree of structure has to be in place first. Though, it is intriguing that blocking neuronal function in the embryonic cerebellum causes lifelong changes in circuit function and behavior without changing structure (White and Sillitoe, 2017). This is consistent with findings that the wiring of topographic circuits–a proxy for arising function–is not strictly linked to the morphology of overlying folds (Sillitoe et al., 2008). Still, the intricate folding (cerebral cortex and cerebellum) and layering (cerebral cortex, cerebellum, hippocampus, and other regions; Figure 3) in the different regions of the brain are necessary developmental steps in establishing circuits.
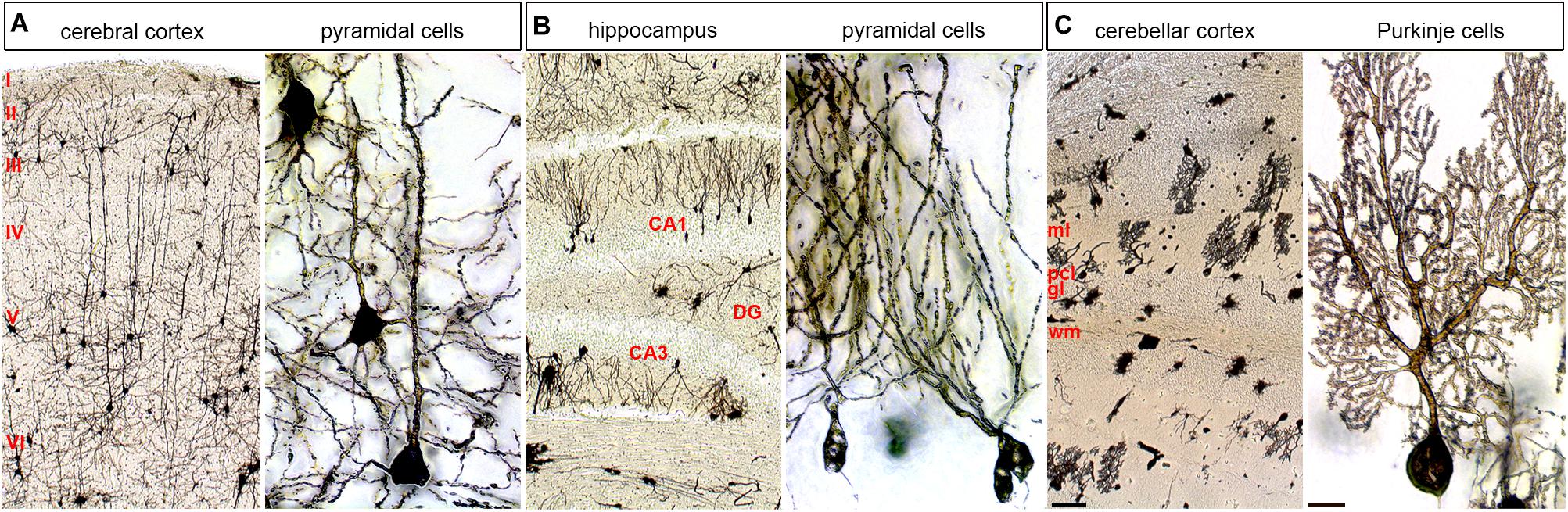
FIGURE 3. Cellular diversity and specificity in different layered brain structures. The adult mouse brain was stained using a modified Golgi–Cox method. (A) Cerebral cortex shown at low and high power. (B) Hippocampus shown at low and high power shown at low and high power. (C) Cerebellum shown at low and high power shown at low and high power. Pyramidal cells are shown at high power for the cerebral cortex and hippocampus and Purkinje cells are shown for the cerebellum. ml, molecular layer; pcl, Purkinje cell layer; gl, granular layer; wm, white matter; DG, dentate gyrus. The six main layers of the cerebral cortex are labeled with Roman numerals. The scale bar in C (cerebellar cortex) = 100 μm and applies to all three low power images. The scale bar in C (Purkinje cells) = 20 μm and applies to all three high power images.
Summary
Regional differences in proliferation set up the structural flexibility that folds and shapes the brain. Genetic cascades control the process, but they also initiate the patterned wiring of circuits. Establishment of structure-function relationships is therefore dependent on topographic circuits, which define the connectivity patterns of networks of neurons with specific physiological properties, which jointly control particular behavioral outcomes. Cues such as SHH play multiple roles throughout development, tying several aspects of brain growth, patterning, wiring, and behavior together. But there is a still a major gap in our understanding of how the precise structure formed in one region relates to that in connected regions. For simplicity, we have discussed somewhat independently the cerebral cortex and cerebellum as models of two areas that are structurally vastly different but are massively interconnected (Bostan et al., 2013) with widely overlapping functional involvements. Moreover, conditions such as ASDs could involve long-range functional interactions from the cerebellum to cortex (Stoodley et al., 2017). Could coordinated inter-regional growth perform critical functions, with common spatial and temporal developmental features? And how would this get processed to impact behavior? The synchronous neuronal activity within structurally defined local circuits could be integrated with circuits of similar design in the connected regions. Proper network structures allow neural computations to actively harness the power of “communication through coherence” as a means of integrating multiple brain systems (Fries, 2015). Indeed, there is growing evidence that this may be how the cerebellum communicates with the hippocampus and the cerebral cortex during non-motor function (McAfee et al., 2017). To further resolve this, future studies could exploit the phenotypes of rare human diseases (Wangler et al., 2017) as a means to systematically define how developmental programs drive the coordinated assembly of structure and function in the brain.
Ethics Statement
Animal studies were performed under an approved Institutional Animal Care and Use Committee (IACUC) protocol at Baylor College of Medicine.
Author Contributions
LM and RS wrote the first draft. LM, EL, DH, and RS edited the manuscript and finalized the paper.
Funding
RS was supported by funds from Baylor College of Medicine (BCM) and Texas Children’s Hospital, the Hamill Foundation, BCM IDDRC Grant U54HD083092 from the Eunice Kennedy Shriver National Institute of Child Health and Human Development (The IDDRC Neuropathology Sub-Core contributed to the tissue staining), and the National Institutes of Neurological Disorders and Stroke (NINDS) R01NS089664 and R01NS100874. DH and RS were supported by National Institutes of Mental Health (NIMH) R01MH112143.
Conflict of Interest Statement
The authors declare that the research was conducted in the absence of any commercial or financial relationships that could be construed as a potential conflict of interest.
References
Albus, J. S. (1971). A theory of cerebellar function. Math. Biosci. 10, 25–61. doi: 10.1016/0025-5564(71)90051-4
Altman, J., and Bayer, S. (1997). Development of the Cerebellar System in Relation to its Evolution, Structure, and Function. Boca Raton, FL: CRC Press.
Antoine, M. W., Schnepel, P., Langberg, T., and Feldman, D. E. (2018). Increased excitation-inhibition ratio stabilizes synapse and circuit excitability in four autism mouse models. bioRxiv [Preprint]. doi: 10.1101/317693
Apps, R., and Hawkes, R. (2009). Cerebellar cortical organization: a one-map hypothesis. Nat. Rev. Neurosci. 10, 670–681.
Apps, R., Hawkes, R., Aoki, S., Bengtsson, F., Brown, A. M., Chen, G., et al. (2018). Cerebellar modules and their role as operational cerebellar processing units. Cerebellum doi: 10.1007/s12311-018-0952-3 [Epub ahead of print].
Arbib, M. A., Érdi, P., and Szentágothai, J. (1998). Neural Organization: Structure, Function, and Dynamics. Cambridge, MA: The MIT Press.
Barron, D. H. (1950). An experimental analysis of some factors involved in the development of the fissure pattern of the cerebral cortex. J. Exp. Zool. 113, 553–581. doi: 10.1002/jez.140113034
Blaess, S., Stephen, D., and Joyner, A. L. (2008). Gli3 coordinates three-dimensional patterning and growth of the tectum and cerebellum by integrating Shh and Fgf8 signaling. Development 135, 2093–2103. doi: 10.1242/dev.015990
Bloem, B., Huda, R., Sur, M., and Graybiel, A. M. (2017). Two-photon imaging in mice shows striosomes and matrix have overlapping but differential reinforcement-related responses. Elife 6:e32353. doi: 10.7554/eLife.32353
Bostan, A. C., Dum, R. P., and Strick, P. L. (2013). Cerebellar networks with the cerebral cortex and basal ganglia. Trends Cogn. Sci. 17, 241–254. doi: 10.1016/j.tics.2013.03.003
Bozza, T., Feinstein, P., Zheng, C., and Mombaerts, P. (2002). Odorant receptor expression defines functional units in the mouse olfactory system. J. Neurosci. 22, 3033–3043. doi: 10.1523/JNEUROSCI.22-08-03033.2002
Brodmann, K. (1909). Vergleichende Lokalisationslehre der Grosshirnrinde in ihren Prinzipien dargestellt auf Grund des Zellenbaues. Leipzig: J. A. Barth.
Caligore, D., Pezzulo, G., Baldassarre, G., Bostan, A. C., Strick, P. L., Doya, K., et al. (2017). Consensus paper: towards a systems-level view of cerebellar function: the interplay between cerebellum, basal ganglia, and cortex. Cerebellum 16, 203–229. doi: 10.1007/s12311-016-0763-3
Cang, J., Niell, C. M., Liu, X., Pfeiffenberger, C., Feldheim, D. A., and Stryker, M. P. (2008). Selective disruption of one cartesian axis of cortical maps and receptive fields by deficiency in ephrin-As and structured activity. Neuron 57, 511–523. doi: 10.1016/j.neuron.2007.12.025
Carlisi, C. O., Norman, L. J., Lukito, S. S., Radua, J., Mataix-Cols, D., and Rubia, K. (2017). Comparative multimodal meta-analysis of structural and functional brain abnormalities in autism spectrum disorder and obsessive-compulsive disorder. Biol. Pyschiatry 82, 83–102. doi: 10.1016/j.biopsych.2016.10.006
Catania, K. C. (2012). Tactile sensing in specialized predators: from behavior to the brain. Curr. Opin. Neurobiol. 22, 251–258. doi: 10.1016/j.conb.2011.11.014
Cerminara, N. L., Lang, E. J., Sillitoe, R. V., and Apps, R. (2015). Redefining the cerebellar cortex as an assembly of non-uniform Purkinje cell microcircuits. Nat. Rev. Neurosci. 16, 79–93. doi: 10.1038/nrn3886
Chavali, P. L., Stojic, L., Meredith, L. W., Joseph, N., Nahorski, M. S., Sanford, T. J., et al. (2017). Neurodevelopmental protein Musashi-1 interacts with the Zika genome and promotes viral replication. Science 357, 83–88. doi: 10.1126/science.aam9243
Christian, K. M., Song, H., and Ming, G.-L. (2018). A previously undetected pathology of Zika virus infection. Nat. Med. 24, 258–259. doi: 10.1038/nm.4510
Cooperrider, J., Furmaga, H., Plow, E., Park, H. J., Chen, Z., Kidd, G., et al. (2014). Chronic deep cerebellar stimulation promotes long-term potentiation, microstructural plasticity, and reorganization of perilesional cortical representation in a rodent model. J. Neurosci. 34, 9040–9050. doi: 10.1523/JNEUROSCI.0953-14.2014
Corrales, J. D. (2006). The level of sonic hedgehog signaling regulates the complexity of cerebellar foliation. Development 133, 1811–1821. doi: 10.1242/dev.02351
Cugola, F. R., Fernandes, I. R., Russo, F. B., Freitas, B. C., Dias, J. L. M., Guimarães, K. P., et al. (2016). The Brazilian Zika virus strain causes birth defects in experimental models. Nature 534, 267–271. doi: 10.1038/nature18296
Dahlem, K., Valko, Y., Schmahmann, J. D., and Lewis, R. F. (2016). Cerebellar contributions to self-motion perception: evidence from patients with congenital cerebellar agenesis. J. Neurophysiol. 115, 2280–2285. doi: 10.1152/jn.00763.2015
Dominici, C., Moreno-Bravo, J. A., Puiggros, S. R., Rappeneau, Q., Rama, N., Vieugue, P., et al. (2017). Floor-plate-derived netrin-1 is dispensable for commissural axon guidance. Nature 545, 349–354. doi: 10.1038/nature22331
Dreazen, E., Tessler, F., Sarti, D., and Crandall, B. F. (1989). Spontaneous resolution of fetal hydrocephalus. J. Ultrasound Med. 8, 155–157. doi: 10.7863/jum.1989.8.3.155
Eccles, J. (1965). Functional meaning of the patterns of synaptic connections in the cerebellum. Perspect. Biol. Med. 8, 289–310. doi: 10.1353/pbm.1965.0041
Fietz, S. A., Kelava, I., Vogt, J., Wilsch-Brauninger, M., Stenzel, D., Fish, J. L., et al. (2010). OSVZ progenitors of human and ferret neocortex are epithelial-like and expand by integrin signaling. Nat. Neurosci. 13, 690–699. doi: 10.1038/nn2553
Finlay, D., and Darlington, R. (1995). Linked regularities in the development and evolution of mammalian brains. Science 268, 1578–1584. doi: 10.1126/science.7777856
Florio, M., Albert, M., Taverna, E., Namba, T., Brandi, H., Lewitus, E., et al. (2015). Human-specific gene ARHGAP11B promotes basal progenitor amplification and neocortex expansion. Science 347, 1465–1470. doi: 10.1126/science.aaa1975
Fries, P. (2015). Rhythms for cognition: communication through coherence. Neuron 88, 220–235. doi: 10.1016/j.neuron.2015.09.034
Garcez, P. P., Loiola, E. C., Da Costa, R. M., Higa, L. M., Trindade, P., Delvecchio, R., et al. (2016). Zika virus: Zika virus impairs growth in human neurospheres and brain organoids. Science 352, 816–818. doi: 10.1038/srep40780
Garcia, M. A., Nelson, J., and Chavez, N. (2018). Cell-cell junctions organize structural and signaling networks. Cold Spring Harb. Perspect. Biol. 10:a029181. doi: 10.1101/cshperspect.a029181
Gebre, S. A., Reeber, S. L., and Sillitoe, R. V. (2012). Parasagittal compartmentation of cerebellar mossy fibers as revealed by the patterned expression of vesicular glutamate transporters VGLUT1 and VGLUT2. Brain Struct. Funct. 217, 165–180. doi: 10.1007/s00429-011-0339-4
Gilbert, P. F. (1974). A theory of memory that explains the function and structure of the cerebellum. Brain Res. 70, 1–18. doi: 10.1016/0006-8993(74)90208-X
Golden, C. E., Buxbaum, J. D., and De Rubeis, S. (2018). Disrupted circuits in mouse models of autism spectrum disorder and intellectual disability. Curr. Opin. Neurobiol. 48, 106–112. doi: 10.1016/j.conb.2017.11.006
Govek, E.-E., Wu, Z., Acehan, D., Molina, H., Rivera, K., Zhu, X., et al. (2018). Cdc42 regulates neuronal polarity during cerebellar axon formation and glial-guided migration. iScience 1, 35–48. doi: 10.1016/j.isci.2018.01.004
Guy, J., and Staiger, T. F. (2017). The functioning of a cortex without layers. Front. Neuroanat. 11:54. doi: 10.3389/fnana.2017.00054
Hansen, D. V., Lui, J. H., Parker, P. R., and Kriegstein, A. R. (2010). Neurogenic radial glia in the outer subventricular zone of human neocortex. Nature 464, 554–561. doi: 10.1038/nature08845
Herculano-Houzel, S. (2014). The glia/neuron ratio: how it varies uniformly across brain structures and species and what that means for brain physiology and evolution. Glia 62, 1377–1391. doi: 10.1002/glia.22683
Herculano-Houzel, S., Collins, C. E., Wong, P., and Kaas, J. H. (2007). Cellular scaling for primate brains. Proc. Natl. Acad. Sci. U.S.A. 104, 3562–3567. doi: 10.1073/pnas.0611396104
Hevner, R. F. (2006). From radial glia to pyramidal-projection neuron: transcription factor cascades in cerebral cortex development. Mol. Neurobiol. 33, 33–50. doi: 10.1385/MN:33:1:033
Hikishima, K., Komaki, Y., Seki, F., Ohnishi, Y., Okano, H. J., and Okano, H. (2017). In vivo microscopic voxel-based morphometry with a brain template to characterize strain specific structures in the mouse brain. Sci. Rep. 7:85. doi: 10.1038/s41598-017-00148-1
Hitti, F. L., and Siegelbaum, S. A. (2014). The hippocampal CA2 region is essential for social memory. Nature 508, 88–92. doi: 10.1038/nature13028
Hollis, E. R., Ishiko, N., Yu, T., Lu, C. C., Haimovich, A., Tolentino, K., et al. (2016). Ryk controls remapping of motor cortex during functional recovery after spinal cord injury. Nat. Neurosci. 19, 697–705. doi: 10.1038/nn.4282
Homman-Ludiye, J., and Bourne, J. A. (2014). Mapping arealisation of the visual cortex of non-primate species: lessons for development and evolution. Front. Neural Circuits 8:79. doi: 10.3389/fncir.2014.00079
Horn, K. M., Pong, M., and Gibson, A. R. (2010). Functional relations of cerebellar modules of the cat. J. Neurosci. 30, 9411–9423. doi: 10.1523/JNEUROSCI.0440-10.2010
Howell, B. W., Hawkes, R., Soriano, P., and Cooper, J. A. (1997). Neuronal position in the developing brain is regulated by mouse disabled-1. Nature 389, 733–737. doi: 10.1038/39607
Hubel, D. H., and Wiesel, T. N. (1979). Brain mechanisms of vision. Sci. Am. 241, 150–162. doi: 10.1038/scientificamerican0979-150
Jiang, X., Shen, S., Cadwell, C. R., Berens, P., Sinz, F., Ecker, A. S., et al. (2015). Principles of connectivity among morphologically defined cell types in adult neocortex. Science 350:aac9462. doi: 10.1126/science.aac9462
John Lin, C. C., Yu, K., Hatcher, A., Huang, T. W., Lee, H. K., Carlson, J., et al. (2017). Identification of diverse astrocyte populations and their malignant analogs. Nat. Neurosci. 20, 396–405. doi: 10.1038/nn.4493
Johnson, M. B., Sun, X., Kodani, A., Borges-Monroy, R., Girskis, K. M., Ryu, S. C., et al. (2018). Aspm knockout ferret reveals an evolutionary mechanism governing cerebral cortical size. Nature 556, 370–375.
Kaas, J. H. (1982). “The segregation of function in the nervous system: why do sensory systems have so many subdivisions?,” in Contributions to Sensory Physiology, Vol. 7, ed. W. P. Neff (New York, NY: Academic Press), 201–240.
Kaas, J. H. (2013). The evolution of brains from early mammals to humans. Wiley Interdiscip. Rev. Cogn. Sci. 4, 33–45. doi: 10.1002/wcs.1206
Kahn, D. M., and Krubitzer, L. (2002). Massive cross-modal cortical plasticity and the emergence of a new cortical area in developmentally blind mammals. Proc. Natl. Acad. Sci. U.S.A. 99, 11429–11434. doi: 10.1073/pnas.162342799
Karzbrun, E., Kshirsagar, A., Cohen, S. R., Hanna, J. H., and Reiner, O. (2018). Human brain organoids on a chip reveal the physics of folding. Nat. Phys. 14, 515–522. doi: 10.1038/s41567-018-0046-7
Kohara, K., Pignatelli, M., Rivest, A. J., Jung, H. Y., Kitamura, T., Suh, J., et al. (2014). Cell type-specific genetic and optogenetic tools reveal hippocampal CA2 circuits. Nat. Neurosci. 17, 269–279. doi: 10.1038/nn.3614
Kremer, M. C., Jung, C., Batelli, S., Rubin, G. M., and Gaul, U. (2017). The glia of the adult Drosophila nervous system. Glia 65, 606–638. doi: 10.1002/glia.23115
Larramendi, L. M., Fickenscher, N., and Lemkey-Johnston, N. (1967). Synaptic vesicles of inhibitory and excitatory terminals in the cerebellum. Science 156, 967–969. doi: 10.1126/science.156.3777.967
Larsell, O. (1970). The Comparative Anatomy and Histology of the Cerebellum from Monotremes through Apes. Minneapolis, MN: University of Minnesota Press.
Le Gros Clark, W. (1945). Deformation Patterns on the Cerebral Cortex: Essays on Growth and Form. London: Oxford University Press.
Legue, E., Riedel, E., and Joyner, A. L. (2015). Clonal analysis reveals granule cell behaviors and compartmentalization that determine the folded morphology of the cerebellum. Development 142, 1661–1671. doi: 10.1242/dev.120287
Li, H., and Crair, M. C. (2011). How do barrels form in somatosensory cortex? Ann. N. Y. Acad. Sci. 1225, 119–129. doi: 10.1111/j.1749-6632.2011.06024.x
Li, K., Leung, A. W., Guo, Q., Yang, W., and Li, J. Y. (2014). Shp2-dependent ERK signaling is essential for induction of Bergmann glia and foliation of the cerebellum. J. Neurosci. 34, 922–931. doi: 10.1523/JNEUROSCI.3476-13.2014
Long, K. R., Newland, B., Florio, M., Kalebic, N., Langen, B., Kolterer, A., et al. (2018). Extracellular matrix components HAPLN1, lumican, and collagen I cause hyaluronic acid-dependent folding of the developing human neocortex. Neuron 99, 702–719.e7. doi: 10.1016/j.neuron.2018.07.013
Ma, S., Kwon, H. J., and Huang, Z. (2012). Ric-8a, a guanine nucleotide exchange factor for heterotrimeric G proteins, regulates Bergmann glia-basement membrane adhesion during cerebellar foliation. J. Neurosci. 32, 14979–14993. doi: 10.1523/JNEUROSCI.1282-12.2012
Manto, M. (2008). The cerebellum, cerebellar disorders, and cerebellar research—two centuries of discoveries. Cerebellum 7, 505–516. doi: 10.1007/s12311-008-0063-7
Marr, D. (1969). A theory of cerebellar cortex. J. Physiol. 202, 437–470. doi: 10.1113/jphysiol.1969.sp008820
Marzban, H., and Hawkes, R. (2011). On the architecture of the posterior zone of the cerebellum. Cerebellum 10, 422–434. doi: 10.1007/s12311-010-0208-3
Matsumoto, N., Shinmyo, Y., Ichikawa, Y., and Kawasaki, H. (2017). Gyrification of the cerebral cortex requires FGF signaling in the mammalian brain. Elife 6:e29285. doi: 10.7554/eLife.29285
Mayer, C., Bandler, R. C., and Fishell, G. (2016). Lineage is a poor predictor of interneuron positioning within the forebrain. Neuron 92, 45–51. doi: 10.1016/j.neuron.2016.09.035
McAfee, S. S., Liu, Y., Sillitoe, R. V., and Heck, D. H. (2017). Cerebellar Purkinje cell simple spike activity in awake mice represents phase differences between oscillations in medial prefrontal cortex and hippocampus. bioRxiv [Preprint]. doi: 10.1101/173849
Meng, Y., Li, G., Wang, L., Lin, W., Gilmore, J. H., and Shen, D. (2018). Discovering cortical sulcal folding patterns in neonates using large-scale dataset. Hum. Brain Mapp. doi: 10.1002/hbm.24199 [Epub ahead of print].
Miterko, L. N., Sillitoe, R. V., and Hawkes, R. (2018). “Zones and stripes: development of cerebellar topography,” in Handbook of the Cerebellum and Cerebellar Disorders, 2nd Edn, eds M. Manto, J. D. Schmahmann, F. Rossi, D. L. Gruol, and N. Koibuchi (Dordrecht: Springer), 43–59.
Mota, B., and Herculano-Houzel, S. (2015). BRAIN STRUCTURE. Cortical folding scales universally with surface area and thickness, not number of neurons. Science 349, 74–77. doi: 10.1126/science.aaa9101
Mountcastle, V. B. (1957). Modality and topographic properties of single neurons of cat’s somatic sensory cortex. J. Neurophysiol. 20, 408–434. doi: 10.1152/jn.1957.20.4.408
Nedergaard, M., Ransom, B., and Goldman, S. A. (2003). New roles for astrocytes: redefining the functional architecture of the brain. Trends Neurosci. 26, 523–530. doi: 10.1016/j.tins.2003.08.008
Oberheim, N. A., Wang, X., Goldman, S., and Nedergaard, M. (2006). Astrocytic complexity distinguishes the human brain. Trends Neurosci. 29, 547–553. doi: 10.1016/j.tins.2006.08.004
Okuyama, T., Kitamura, T., Roy, D. S., Itohara, S., and Tonegawa, S. (2016). Ventral CA1 neurons store social memory. Science 353, 1536–1541. doi: 10.1126/science.aaf7003
O’Leary, D. D., Chou, S. J., and Sahara, S. (2007). Area patterning of the mammalian cortex. Neuron 56, 252–269. doi: 10.1016/j.neuron.2007.10.010
Ozol, K. O., and Hawkes, R. (1997). Compartmentation of the granular layer of the cerebellum. Histol. Histopathol. 12, 171–184.
Penfield, W. (1968). Engrams in the human brain: mechanisms of memory. Proc. R. Soc. Med. 61, 831–840.
Peng, J., Fabre, P. J., Dolique, T., Swikert, S. M., Kermasson, L., Shimogori, T., et al. (2018). Sonic hedgehog is a remotely produced cue that controls axon guidance trans-axonally at a midline choice point. Neuron 97, 326–340. doi: 10.1016/j.neuron.2017.12.028
Qian, X., Nguyen, H. N., Jacob, F., Song, H., and Ming, G. (2017). Using brain organoids to understand Zika virus-induced microcephaly. Development 144, 952–957. doi: 10.1242/dev.14707
Rakic, P., Ayoub, A. E., Breunig, J. J., and Dominguez, M. H. (2009). Decision by division: making cortical maps. Trends Neurosci. 32, 291–301. doi: 10.1016/j.tins.2009.01.007
Reeber, S. L., Arancillo, M., and Sillitoe, R. V. (2018). Bergmann glia are patterned into topographic molecular zones in the developing and adult mouse cerebellum. Cerebellum 17, 392–403. doi: 10.1007/s12311-014-0571-6
Richman, D., Stewart, R., Hutchinson, J., and Caviness, V. (1975). Mechanical model of brain convolutional development. Science 189, 18–21. doi: 10.1126/science.1135626
Riddle, K., Cascio, C. J., and Woodward, N. D. (2017). Brain structure in autism: a voxel-based morphometry analysis of the Autism Brain Imaging Database Exchange (ABIDE). Brain Imaging Behav. 11, 541–551. doi: 10.1007/s11682-016-9534-5
Ronan, L., and Fletcher, P. C. (2015). From genes to folds: a review of cortical gyrification theor. Brain Struct. Funct. 220, 2475–2483. doi: 10.1007/s00429-014-0961-z
Ronan, L., Voets, N., Rua, C., Alexander-Bloch, A., Hough, M., Mackay, C., et al. (2013). Differential tangential expansion as a mechanism for cortical gyrification. Cereb. Cortex 24, 2219–2228. doi: 10.1093/cercor.bht082
Roy, A., Skibo, J., Kalume, F., Ni, J., Rankin, S., Lu, Y., et al. (2015). Mouse models of human PIK3CA-related brain overgrowth have acutely treatable epilepsy. eLife 4:e12703. doi: 10.7554/eLife.12703
Ruigrok, T. J. H. (2011). Ins and outs of cerebellar modules. Cerebellum 10, 464–474. doi: 10.1007/s12311-010-0164-y
Ryan, K. E., Kim, P. S., Fleming, J. T., Brignola, E., Cheng, F. Y., Litingtung, Y., et al. (2017). Lkb1 regulates granule cell migration and cortical folding of the cerebellar cortex. Dev. Biol. 432, 165–177. doi: 10.1016/j.ydbio.2017.09.036
Saab, A. S., Neumeyer, A., Jahn, H. M., Cupido, A., Simek, A. A. M., Boele, H.-J., et al. (2012). Bergmann glial AMPA receptors are required for fine motor coordination. Science 337, 749–753. doi: 10.1126/science.1221140
Sarna, J. R., and Hawkes, R. (2003). Patterned Purkinje cell death in the cerebellum. Prog. Neurobiol. 70, 473–507. doi: 10.1016/S0301-0082(03)00114-X
Scoville, W. B., and Milner, B. (1957). Loss of recent memory after bilateral hippocampal lesions. J. Neurol. Neurosurg. Psychiatry 20, 11–21. doi: 10.1136/jnnp.20.1.11
Sherry, D. F., Jacobs, L. F., and Gaulin, S. J. C. (1992). Spatial memory and adaptive specialization of the hippocampus. Trends Neurosci. 15, 298–303. doi: 10.1016/0166-2236(92)90080-R
Sillitoe, R. V., and Joyner, A. L. (2007). Morphology, molecular codes, and circuitry produce the three-dimensional complexity of the cerebellum. Annu. Rev. Cell Dev. Biol. 23, 549–577. doi: 10.1146/annurev.cellbio.23.090506.123237
Sillitoe, R. V., Marzban, H., Larouche, M., Zahedi, S., Affanni, J., and Hawkes, R. (2005). Conservation of the architecture of the anterior lobe vermis of the cerebellum across mammalian species. Prog. Brain Res. 148, 283–297. doi: 10.1016/S0079-6123(04)48022-4
Sillitoe, R. V., Stephen, D., Lao, Z., and Joyner, A. L. (2008). Engrailed homeobox genes determine the organization of Purkinje cell sagittal stripe gene expression in the adult cerebellum. J. Neurosci. 28, 12150–12162. doi: 10.1523/JNEUROSCI.2059-08.2008
Sillitoe, R. V., Vogel, M. W., and Joyner, A. L. (2010). Engrailed homeobox genes regulate establishment of the cerebellar afferent circuit map. J. Neurosci. 30, 10015–10024. doi: 10.1523/JNEUROSCI.0653-10-2010
Smith, R. S., Kenny, C. J., Ganesh, V., Jang, A., Borges-Monroy, R., Partlow, J. N., et al. (2018). Sodium channel SCN3A (NaV1.3) regulation of human cerebral cortical folding and oral motor development. Neuron 99, 905–913.e7. doi: 10.1016/j.neuron.2018.07.052
Stoodley, C. J., D’Mello, A. M., Ellegood, J., Jakkamsetti, V., Liu, P., Nebel, M. B., et al. (2017). Altered cerebellar connectivity in autism and cerebellar-mediated rescue of autism-related behaviors in mice. Nat. Neurosci. 20, 1744–1751. doi: 10.1038/s41593-017-0004-1
Stoodley, C. J., and Schmahmann, J. D. (2009). Functional topography in the human cerebellum: a meta-analysis of neuroimaging studies. Neuroimage 44, 489–501. doi: 10.1016/j.neuroimage.2008.08.039
Sudarov, A., and Joyner, A. L. (2007). Cerebellum morphogenesis: the foliation pattern is orchestrated by multi-cellular anchoring centers. Neural Dev. 2:26. doi: 10.1186/1749-8104-2-26
Sudarov, A., Turnbull, R. K., Kin, E. J., Lebel-Potter, M., Guillemot, F., and Joyner, A. L. (2011). Ascl1 genetics reveals insights into cerebellum local circuit assembly. J. Neurosci. 31, 11055–11069. doi: 10.1523/JNEUROSCI.0479-11.2011
Sultan, K., Han, Z., Zhang, X. J., Xianyu, A., Li, Z., Huang, K., et al. (2016). Clonally related GABAergic interneurons do no randomly disperse but frequently form local clusters in the forebrain. Neuron 92, 31–44. doi: 10.1016/j.neuron.2016.09.033
Sun, T., and Hevner, R. F. (2016). Growth and folding of the mammalian cerebral cortex: from molecules to malformations. Nat. Rev. Neurosci. 15, 217–232. doi: 10.1038/nrn3707
Tan, G. H., Liu, Y. Y., Wang, L., Li, K., Zhang, Z. Q., Li, H. F., et al. (2018). PRRT2 deficiency induces paroxysmal kinesigenic dyskinesia by regulating synaptic transmission in cerebellum. Cell Res. 28, 90–110. doi: 10.1038/cr.2017.128
Tatavarty, V., Pacheco, A. T., Lin, H., Miska, N. J., Hengen, K. B., Wagner, F. F., et al. (2018). Autism-associated Shank3 is essential for homeostatic plasticity and neuronal circuit stability. bioRxiv [Preprint]. doi: 10.1101/365445
Thawani, A., Sirohi, D., Kuhn, R. J., and Fekete, D. M. (2018). Zika virus can strongly infect and disrupt secondary organizers in the ventricular zones of the embryonic chicken brain. Cell 23, 692–700. doi: 10.1016/j.celrep.2018.03.080
Uesaka, N., Uchigashima, M., Mikuni, T., Nakazawa, T., Nakao, H., Hirai, H., et al. (2014). Retrograde semaphoring signaling regulates synapse elimination in the developing mouse brain. Science 344, 1020–1023. doi: 10.1016/j.neuron.2018.01.018
Van Essen, D. C. (1997). A tension-based theory of morphogenesis and compact wiring in the central nervous system. Nature 385, 313–318. doi: 10.1038/385313a0
Van Essen, D. C., Donahue, C. J., and Glasser, M. F. (2018). Development and evolution of cerebral and cerebellar cortex. Brain Behav. Evol. 91, 158–169. doi: 10.1159/000489943
Varadarajan, S. G., Kong, J. H., Phan, K. D., Kao, T. J., Panaitof, S. C., Cardin, J., et al. (2017). Netrin1 produced by neural progenitors, not floor plate cells, is required for axon guidance in the spinal cord. Neuron 94, 790–799. doi: 10.1016/j.ydbio.2017.08.001
Voogd, J., and Ruigrok, T. J. (1997). Transverse and longitudinal patterns in the mammalian cerebellum. Prog. Brain Res. 114, 21–37. doi: 10.1016/S0079-6123(08)63356-7
Wadiche, J. I., and Jahr, C. E. (2005). Patterned expression of Purkinje cell glutamate transporters controls synaptic plasticity. Nat. Neurosci. 8, 1329–1334. doi: 10.1038/nn1539
Wang, L., Hou, S., and Han, Y. G. (2016). Hedgehog signaling promotes basal progenitor expansion and the growth and folding of the neocortex. Nat. Neurosci. 19, 888–896. doi: 10.1038/nn.4307
Wang, X., Tsai, J.-W., LaMonica, B., and Kriegstein, A. R. (2011). A new subtype of progenitor cell in the mouse embryonic neocortex. Nat. Neurosci. 14, 555–561. doi: 10.1038/nn.2807
Wang, Y., Wu, Q., Yang, P., Wang, C., Liu, J., Ding, W., et al. (2016). LSD1 co-repressor Rcor2 orchestrates neurogenesis in the developing mouse brain. Nat. Commun. 7:10481. doi: 10.1038/ncomms10481
Wangler, M. F., Yamamoto, S., Chao, H. T., Posey, J. E., Westerfield, J., Postlethwait, J., et al. (2017). Model organisms facilitate rare disease diagnosis and therapeutic research. Genetics 207, 9–27. doi: 10.1534/genetics.117.203067
Wasser, C. R., and Herz, J. (2017). Reelin: neurodevelopmental architect and homeostatic regulator of excitatory synapses. J. Biol. Chem. 292, 1330–1338. doi: 10.1074/jbc.R116.766782
Welker, W. (1990). “Why does cerebral cortex fissure and fold?,” in Cerebral Cortex, Vol. 8B, eds E. G. Jones and A. Peters (Boston, MA: Springer), 3–136.
Welsh, J. P., Yuen, G., Placantonakis, D. G., Vu, T. Q., Haiss, F., O’Hearn, E., et al. (2002). Why do Purkinje cells die so easily after global brain ischemia? Aldolase C, EAAT4, and the cerebellar contribution to posthypoxic myoclonus. Adv. Neurol. 89, 331–359.
White, J. J., Arancillo, M., Stay, T. L., George-Jones, N. A., Levy, S. L., Heck, D. H., et al. (2014). Cerebellar zonal patterning relies on Purkinje cell neurotransmission. J. Neurosci. 34, 8231–8245. doi: 10.1523/JNEUROSCI.0122-14.2014
White, J. J., Reeber, S. L., Hawkes, R., and Sillitoe, R. V. (2012). Wholemount immunohistochemistry for revealing complex brain topography. J. Vis. Exp. 5:e4042. doi: 10.3791/4042
White, J. J., and Sillitoe, R. V. (2013). Development of the cerebellum: from gene expression patterns to circuit maps. Wiley Interdiscip. Rev. Dev. Biol. 2, 149–164. doi: 10.1002/wdev.65
White, J. J., and Sillitoe, R. V. (2017). Genetic silencing of olivocerebellar synapses causes dystonia-like behaviour in mice. Nat. Commun. 8:14912. doi: 10.1038/ncomms14912
Wojcinski, A., Lawton, A. K., Bayin, N. S., Lao, Z., Stephen, D. N., and Joyner, A. L. (2017). Cerebellar granule cell replenishment postinjury by adaptive reprogramming of Nestin + progenitors. Nat. Neurosci. 20, 1361–1370. doi: 10.1038/nn.4621
Xiao, J., Cerminara, N. L., Kotsurovskyy, Y., Aoki, H., Burroughs, A., Wise, A. K., et al. (2014). Systematic regional variations in Purkinje cell spiking patterns. PLoS One 9:e105633. doi: 10.1371/journal.pone.0105633
Keywords: neuron, glia, folding, layering, connectivity, topography, patterning
Citation: Miterko LN, Lackey EP, Heck DH and Sillitoe RV (2018) Shaping Diversity Into the Brain’s Form and Function. Front. Neural Circuits 12:83. doi: 10.3389/fncir.2018.00083
Received: 22 May 2018; Accepted: 18 September 2018;
Published: 10 October 2018.
Edited by:
Jing-Ning Zhu, Nanjing University, ChinaReviewed by:
Jiayi Zhang, Fudan University, ChinaAnnalisa Buffo, Università degli Studi di Torino, Italy
Copyright © 2018 Miterko, Lackey, Heck and Sillitoe. This is an open-access article distributed under the terms of the Creative Commons Attribution License (CC BY). The use, distribution or reproduction in other forums is permitted, provided the original author(s) and the copyright owner(s) are credited and that the original publication in this journal is cited, in accordance with accepted academic practice. No use, distribution or reproduction is permitted which does not comply with these terms.
*Correspondence: Roy V. Sillitoe, c2lsbGl0b2VAYmNtLmVkdQ==