- 1Department of Psychiatry, National Clinical Research Center for Mental Disorders, The Second Xiangya Hospital of Central South University, Changsha, China
- 2Department of Radiology, The Second Xiangya Hospital of Central South University, Changsha, China
- 3Department of Radiology, Tianjin Medical University General Hospital, Tianjin, China
- 4Department of Psychiatry, The Third People’s Hospital of Foshan, Foshan, China
- 5Department of Psychiatry, Qiqihar Medical University, Qiqihar, China
Early brain functional changes induced by pharmacotherapy in patients with obsessive–compulsive disorder (OCD) in relation to drugs per se or because of the impact of such drugs on the improvement of OCD remain unclear. Moreover, no neuroimaging biomarkers are available for diagnosis of OCD and prediction of early treatment response. We performed a longitudinal study involving 34 patients with OCD and 36 healthy controls (HCs). Patients with OCD received 5-week treatment with paroxetine (40 mg/d). Resting-state functional magnetic resonance imaging (fMRI), regional homogeneity (ReHo), support vector machine (SVM), and support vector regression (SVR) were applied to acquire and analyze the imaging data. Compared with HCs, patients with OCD had higher ReHo values in the right superior temporal gyrus and bilateral hippocampus/parahippocampus/fusiform gyrus/cerebellum at baseline. ReHo values in the left hippocampus and parahippocampus decreased significantly after treatment. The reduction rate (RR) of ReHo values was positively correlated with the RRs of the scores of Yale–Brown Obsessive–Compulsive Scale (Y-BOCS) and obsession. Abnormal ReHo values at baseline could serve as potential neuroimaging biomarkers for OCD diagnosis and prediction of early therapeutic response. This study highlighted the important role of the hippocampal–cortical system in the neuropsychological mechanism underlying OCD, pharmacological mechanism underlying OCD treatment, and the possibility of building models for diagnosis and prediction of early treatment response based on spontaneous activity in the hippocampal–cortical system.
Introduction
Obsessive–compulsive disorder (OCD) is a severe and chronically disabling clinical condition with a lifetime prevalence rate of 2–3% (Sasson et al., 1997; Zohar, 1999; Ruscio et al., 2010). Increasing evidence suggests that OCD should be considered as a disorder of network connectivity rather than discrete cerebral regions (Hao et al., 2019). Cumulative and consistent evidence suggests that OCD is mediated by abnormal cortico-striato-thalamo-cortical (CSTC) circuits (Beucke et al., 2013). Several cerebral regions outside the CSTC circuits, such as the parietal cortex, insula, hippocampus, parahippocampus, and cerebellum, are also involved in the pathogenesis of OCD (Del Casale et al., 2011; Tang et al., 2015; Chen et al., 2016; Zhao et al., 2017; Yang et al., 2019), suggesting that the networks involved in OCD should be revisited. Recently, abnormal default mode network (DMN), frontal–parietal network, top–down control network, and salience network in OCD have attracted the attention of researchers (Göttlich et al., 2014; Gonçalves et al., 2016). However, outcomes of these studies were heterogeneous. The incomplete understanding of the neurophysiological mechanism underlying OCD has hampered efforts to enhance extant interventions and develop new treatments.
The pharmacological mechanism underlying the treatment for OCD remains unclear. The first-line treatments for OCD are selective serotonin reuptake inhibitors (SSRIs) and cognitive-behavioral therapy (CBT) (Bloch et al., 2010; Olatunji et al., 2013). Nevertheless, 30–40% of patients do not respond to SSRIs, and most patients do not generally achieve full remission (Fineberg et al., 2012, 2013). A recent article reviewed the neuroimaging studies about functional and structural neural changes in OCD after pharmacotherapy and found that drugs could generally affect the brains of patients with OCD (Atmaca, 2019). However, whether treatment-induced early neuroimaging changes are related to drugs per se or to the impact of such drugs on the improvement of OCD are unclear.
Thus far, no diagnostic biomarkers are available for OCD, and its diagnosis relies most on the recognition of characteristic symptoms assessed by clinical interview. Neuroimaging studies [such as resting-state functional magnetic resonance imaging (fMRI), task-based fMRI, positron emission tomography, and electroencephalogram] and electro-physiological studies (Riesel et al., 2011, 2015) have found various brain structural, functional, and metabolic abnormalities in patients with OCD compared with healthy controls (HCs). However, most studies have been conducted at the group level, thus limiting the diagnostic usage in clinical practice. In recent years, new statistical approaches, such as random forest models and multivariate pattern analysis (MVPA) techniques based on the individual level, have been developed, which may fill the research gap. A recent article reviewed the studies that applied MVPA techniques to neuroimaging data for distinguishing patients with OCD from HCs. Results showed that the accuracies ranged from 66 to 100% (Bruin et al., 2019) and indicated the enormous potential of MVPA techniques connected with neuroimaging studies in building models for the diagnosis of OCD.
Prediction of treatment response could optimize the use of medical resources, minimize exposure to ineffective drugs, and increase patient compliance. The prediction of treatment response for OCD has become an important research priority considering the prevalence and disability associated with it and the availability of different effective interventions. Although many studies have recognized demographic and psychometric characteristics correlated with treatment response in OCD, outcomes of predictions are inconsistent (Knopp et al., 2013; Alemany-Navarro et al., 2019). Neuroimaging features are promising for the prediction of treatment response for OCD (Salomoni et al., 2009; Sanematsu et al., 2010; Hoexter et al., 2013; Göttlich et al., 2015; Dunlop et al., 2016; Reggente et al., 2018). However, to the best of our knowledge, no studies have explored the possibility of using resting-state neuroimaging signals combined with machine learning techniques to predict early treatment response for OCD to paroxetine.
Thus, we designed the present longitudinal study, which compared the pre-treatment images with post-treatment images; for the latter, scanning was performed after 5-week treatment with paroxetine (40 mg/d). The 5-week treatment duration as the endpoint for observing early treatment response was appropriate for three reasons. First, a statistically significant benefit of SSRIs compared with placebo was observed within 2 weeks after the initial point of treatment in adults or children with OCD (Issari et al., 2016; Varigonda et al., 2016). Second, the practice guidelines for the treatment of OCD with serotonin reuptake inhibitors consider 8–12 weeks or at least 4–6 weeks at the highest comfortably tolerated dose as an adequate duration for a trial before switching drugs or augmenting therapy with another agent (American Psychiatric Association [APA], 2007). Third, to the best of our knowledge, no studies have measured early imaging changes (treatment duration < 12 weeks) after treatment with SSRIs in OCD. A recent systematic review involving studies performed from 1990 to 2020 to evaluate treatment-induced neuroimaging changes in brains of patients with OCD found that the treatment duration of all included studies varied from 12 weeks to 1 year (Bijanki et al., 2021). We aimed to explore neurophysiological mechanism underlying OCD; pharmacological mechanism of SSRIs on the treatment of OCD, especially for recognition of the pharmacotherapy-induced early functional brain changes that were related to drugs per se or to the impact of such drugs on the alleviation of OCD; and potential neuroimaging biomarkers for diagnosis and prediction of early treatment response.
We applied several techniques, namely, resting-state fMRI, regional homogeneity (ReHo), support vector machine (SVM), and support vector regression (SVR). Resting-state fMRI maps the spatiotemporal distribution of intrinsic spontaneous neural activity in a non-invasive and non-task-related manner. Since its discovery in 1992 (Ogawa et al., 1992), resting-state fMRI has rapidly become a vital tool in neuroscience research. ReHo was an extensively used and highly reliable approach to reveals the local functional connectivity (Zang et al., 2004). As a data-driven method and without any prior hypothesis, ReHo could access a high amount of information throughout the entire brain. SVM, one of the classical machine learning techniques (Meyer et al., 2003), was used to explore the possibility of abnormal ReHo values in patients with OCD to serve as neuroimaging biomarkers for diagnosis. SVM yields good performance in classification compared with classification trees, neural networks, or learning vector quantization (Meyer et al., 2003). SVR, a supervised machine learning technique, could efficiently resolve a non-linear regression problem by projecting the original feature into a kernel space, in which data were linearly discriminated (Ben-Hur et al., 2008). We used SVR to examine whether abnormal ReHo values at baseline could serve as potential neuroimaging biomarkers for the prediction of early treatment response.
This study hypothesized that abnormal ReHo values in the cerebral regions within the CSTC circuits and outside the CSTC circuits would be observed at baseline, and changes in ReHo values after 5 weeks of paroxetine treatment were correlated with the levels of the remission of OCD. We also hypothesized that abnormal ReHo values at baseline could serve as neuroimaging biomarkers for diagnosis and prediction of early treatment response.
Materials and methods
Participants
This study was approved by the Research Ethics Committee of the Second Xiangya Hospital of Central South University in China. The ethical code of the present study was 2018023. A written informed consent was acquired from each participant.
Thirty-six right-handed patients with drug-naive OCD were recruited from the Second Xiangya Hospital, and 36 right-handed HCs were recruited from the local community from January 2019 to September 2021. The diagnosis of OCD was confirmed by using the Structural Clinical Interview for Diagnostic and Statistical Manual of Mental Disorders-Fifth Edition (DSM-5) patient version (American Psychiatric Association [APA], 2013). HCs were screened using the Structured Clinical Interview for DSM-5 Axis I Disorders-Non-patient Edition. All participants were 18–49 years old and had more than 6 years of formal education. All patients with OCD had a Yale–Brown Obsessive–Compulsive Scale (Y-BOCS) score of more than 16 and a 17-item Hamilton Depression Rating Scale (HAMD-17) score of less than 18. All participants had no history of neurological illness or other major physical diseases, no history of any other psychiatric disorders, and no history of substance abuse disorders. Participants were excluded if they had significant medical conditions or were pregnant.
Procedure
At baseline, all participants were scanned using a brain MRI scanner, and their general information was collected. Subsequently, patients with OCD received a paroxetine treatment for 5 weeks (40 mg/day) and were scanned again after the 5-week treatment. No other medicine and/or psychotherapy were involved during the 5-week period. Clinical symptoms of patients with OCD were assessed with Y-BOCS, Hamilton Depression Rating Scale (HAMD), Hamilton Anxiety Rating Scale (HAMA), Social Disability Screening Schedule (SDSS), Simplified Coping Style Questionnaire (CSQ), and Brief Cognitive Assessment Tool for Schizophrenia (B-CATS) at baseline and after treatment.
Measures
Yale–Brown Obsessive–Compulsive Scale, a clinician-administered semi-structured interview, was scored on a five-point Likert scale (0–4, higher scores indicated greater disturbance). The total score was computed from the first 10 items (without items 1b and 6b). Items 1 to 5 measured obsession-related dysfunctions, whereas items 6 to 10 assessed compulsion-related dysfunctions. A validated Chinese version of Y-BOCS was applied in the present study (Zhang et al., 2019). The Chinese version of Y-BOCS had a high internal consistency as a whole (alpha = 0.90), as well as the obsessions (alpha = 0.87) and compulsions (alpha = 0.88) subscales. The test-retest reliability of the Chinese version of Y-BOCS was adequate (intraclass correlation coefficient = 0.63). Convergent validity of the Chinese version of Y-BOCS with the Clinical Global Impression-Severity (r = 0.75) and National Institute of Mental Health Global Obsessive Compulsive Scale was satisfactory (r = 0.71).
The 17-item HAMD (Bagby et al., 2004) was applied to assess depressive symptoms in patients with OCD, and higher scores indicated greater severity in depressive symptoms.
Hamilton Anxiety Rating Scale was used to measure the severity of anxiety symptoms in patients with OCD. HAMA included 14 items, which scored 0–4. A higher score indicated greater severity of anxiety symptoms.
Social Disability Screening Schedule was a 10-item clinician-administered scale to measure the degree of functional impairment. Each item was scored on a three-point Likert scale (0–2). Higher scores indicated greater functional impairment.
Simplified Coping Style Questionnaire (Xie, 1998) was based on the Ways of Coping questionnaire by Folkman and Lazarus (1988). It consisted of 20 items related to different approaches to cope with daily life events, and each item was scored on a four-point Likert scale (0–3). The CSQ contained two dimensions, namely, active coping (12 items) and passive coping (eight items).
Brief Cognitive Assessment Tool for Schizophrenia was an easily administered cognitive battery composed of four parts, namely, Digit Symbol Substitution Test, Trail-Making Test A, Trail-Making Test B, and Animal Fluency (Hurford et al., 2018). The Digit Symbol Substitution Test was scored with the number of correct number-symbol pairs. Trail-Making Test A and B were scored according to the time to completion. Animal Fluency was scored with the number of unique and appropriate answers.
Imaging data acquisition and pre-processing
Functional MRI scans were obtained with a 3.0 T Philips scanner (Philips Achieva; Philips Medical Systems, Best; Netherlands) in the Second Xiangya Hospital. The participants were instructed to remain motionless and keep their eyes closed but remain awake. Soft earplugs and foam pads were used to reduce scanner noise and head motion. The scanning parameters were as follows: repetition time/echo time = 2000/30 ms, 33 slices, 64 × 64 matrix, 90° flip angle, 22 cm FOV, 4 mm-thick slice, no gap, and 240 volumes (8 min). The voxel size was 3.75 mm × 3.75 mm × 4 mm.
Data pre-processing was conducted in MATLAB1 with a data-processing assistant software (DPARSF) (Chao-Gan and Yu-Feng, 2010). The first 10 time points of each participant were removed for the participants to adapt to the scanning environment and signal equilibrium. The remaining 230 volumes underwent slice timing and head motion correction. All participants should not exceed the 2 mm maximum displacement in the x-, y-, or z-axis and the 2° angular motion in any direction to control the impact of head movement. Subsequently, the images were spatially normalized to the standard Montreal Neurological Institute (MNI) space by using the echo-planar imaging (EPI) template and resampled with a resolution of 3 × 3 × 3 mm3. The obtained images were bandpass filtered (0.01–0.08 Hz) and linearly detrended. A Band Pass Filter (BPF) was applied which could transmit all signals of frequencies within a given frequency band radians per second without any distortion and completely block all signals of frequencies outside this given frequency band. The frequency band (e.g., 0.01–0.08 Hz) was called the bandwidth of the BPF. The phase function of a BPF for the distortion less transmission was
where ω was the frequency, td was the time duration. The transfer function of a BPF was
The applied BPF filtered the data in the frequency domain by frequency sampling method, i.e., fast Fourier transform (FFT) transform to frequency domain, then selected the desired frequency band, and finally transformed back to time domain by inverse fast Fourier transform (iFFT).
Regional homogeneity calculation
Regional homogeneity analysis was performed by using the DPARSF software. Individual ReHo maps were generated by calculating Kendall’s coefficient of concordance (KCC) to synchronize the time series of a given voxel with those of its 26 nearest neighbors. The formula for calculating the KCC value was described previously by Zang et al. (2004). The ReHo maps were normalized through the division of the KCC among each voxel by the average KCC of the whole brain for each subject to reduce the influence of individual variations. Subsequently, spatial smoothing was performed on the ReHo maps to improve the signal to noise ratio (SNR) (Mikl et al., 2008). Spatial smoothing was employed by convolving the fMRI signal with a Gaussian function of a specific width. The width was expressed in terms of Full Width at Half Maximum (FWHM). A 4 mm FWHM Gaussian kernel was applied as suggested in a previous study (Mikl et al., 2008).
Statistical analysis
Differences in demographic data between patients with OCD and HCs were analyzed by using two-sample t-tests and a chi-square test as appropriate with the SPSS 19.0 software (SPSS Inc., Chicago, IL, United States). Paired t-tests were performed to compare the clinical symptoms of the patients at baseline with those after treatment. Normality of the difference of variable was assessed prior to using the paired t-tests. The Q-Q plots of the residual signal were provided (Supplementary Figure 1). Statistical significance was determined by p < 0.05. For the imaging data, statistical analyses were conducted with DPARSF. Two-sample t-tests were conducted on the individual normalized ReHo maps to distinguish clusters with abnormal ReHo values in patients with OCD in comparison with HCs. Each participant’s framewise displacement (FD) was calculated with the method in a previous study (Power et al., 2012). The mean FD, gender, age, and years of education were used as covariates of no interest in the two-sample t-tests. The ReHo values of these clusters between pre- and post-treatments in patients with OCD were compared using paired t-tests. The mean FD was used as covariates of no interest in the paired t-tests. In the two-sample and paired t-tests, the significance levels of the p values were corrected for multiple comparisons based on the Gaussian random field (GRF) theory (voxel significance: p < 0.001, cluster significance: p < 0.05) as suggested by a previous study (Chen et al., 2018).
Correlation analysis
Pearson correlation analyses were performed to assess the relationship between abnormal ReHo values and the clinical data in patients with OCD at baseline. Before that, the normality of them was assessed (Supplementary Figure 2). The correlations between the percentage changes in clinical data (scores of Y-BOCS, obsessions, compulsions, HAMA, HAMD, SDSS, active and passive coping, Digit Symbol Substitution Test, Trail-Making Test A, Trail-Making Test B, and Animal Fluency) and the percentage changes in ReHo values of clusters were determined by Pearson correlation analyses.
Support vector machine analysis
Classification analysis was performed to examine whether abnormal ReHo values at baseline could serve as imaging indicators to distinguish patients with OCD from HCs. Classification analysis was conducted using SVM in the LIBSVM software package (Chang and Lin, 2011). A Gaussian radial basis function kernel was applied. The C was the penalty parameter and γ was the free parameter in the radial basis function kernel. A “grid search” on C and γ was conducted using a cross-validation method. The pair of (C, γ) with the best cross-validation accuracy was picked (Liu et al., 2018). The cross-validation accuracy was the percentage of data which was correctly classified (Hsu et al., 2003). We performed permutation tests to assess whether the estimated accuracy differed from what was expected if the classifier was randomly assigning labels. By permuting the data labels and each shuffled instance used to train one SVM, we could estimate the distribution of the accuracy of classification under the null hypothesis (Gaonkar et al., 2015). In the permutation test, the probability of acquiring a given or more extreme of the accuracy of classification could be estimated by dividing the number of times, ni, the accuracy of classification obtained with the permuted data labels was higher or equal to the value of the accuracy of classification estimated with the true data labels, by the times of randomly permuted the data labels (np). Thus, the equation of p values of statistical significance in the permutation test was (Rosa et al., 2015). In the present study, np = 100,000.
Support vector machine analysis
Support vector machine analysis was performed to determine whether abnormal ReHo values at baseline could predict early therapeutic response by using the LIBSVM software package (Chang and Lin, 2011). Actual treatment response to paroxetine was defined as reduction rate (RR) of Y-BOCS total scores, and subscale scores, including obsession and compulsion scores. The RR of Y-BOCS total scores was calculated according to the following formula: RRY–BOCS total = (Y-BOCStotal 0 – Y-BOCStotal 5w)/Y-BOCStotal 0. RRY–BOCS total refers to the RR of Y-BOCS total scores after 5 weeks of treatment. Y-BOCStotal 0 refers to the scores of Y-BOCS at baseline. Y-BOCStotal 5w refers to the scores of Y-BOCS after treatment. The RRs of obsession and compulsion scores were calculated in the same manner. Descriptions of algorithms and training set for SVR were presented in our previous study (Yan et al., 2022). In simple terms, we applied a leave-one-out cross-validation to select the components which maximized predictability of the model. A “grid search” method was used to access parameter optimization. The predicted treatment response was compared with the actual treatment response via the correlation coefficient. We also assessed the regression results with the Bland Altman plots.
Results
Demographic and clinical characteristics
Thirty-six patients and 36 HCs were enrolled in the present study. Two patients were excluded due to excessive head movement. Thirty-four patients and 36 HCs were included in the final analysis. Twenty-four of the 34 patients completed the 5-week follow-up. The main reason for dropping out was the inconvenience of participating in the follow-up during the COVID-19 pandemic. Detailed demographic and clinical data of the participants are shown in Table 1. No significant differences in age, gender, and years of education were observed between the OCD and control groups.
Paroxetine treatment outcome
The clinical characteristics of 24 patients who completed the follow-up are shown in Table 2. The treatment duration of patients who completed the follow-up was 34 ± 5.23 days (from baseline to the second resting-state fMRI scan). After the 5-week treatment, patients with OCD showed significant clinical improvement relative to their baseline assessments. The scores of Y-BOCS (p < 0.001), subscales of Y-BOCS (p < 0.001), HAMD (p < 0.001), HAMA (p < 0.001), SDSS (p < 0.001), active coping (p = 0.019), and subscales of B-CATS, such as Digit symbol substitution (p = 0.002), Trail making test part A (p = 0.002), and Category fluency (p = 0.020), changed significantly. However, the scores of passive coping (p = 0.368) and Trail making test part B did not change significantly (p = 0.170).
Regional homogeneity analysis in pre-treatment patients and healthy controls
Compared with HCs, patients with OCD had higher ReHo values in the right hippocampus/parahippocampus/fusiform gyrus/cerebellum, left hippocampus/parahippocampus/fusi form gyrus/cerebellum, and right superior temporal gyrus (STG) at baseline. Detailed information is presented in Table 3 and Figure 1. No significant difference in mean FD was found between patients and HCs. The signal of the blood-oxygen-level dependent (BOLD) suffers the magnetic susceptibility artifacts, particularly in the STG. These artifacts appear as geometric distortions and signal loss in the T2*-weighted EPI. To assess the detectability of fMRI signals, the temporal SNR (tSNR) of the three clusters with abnormal ReHo values was calculated. The tSNR served as a quantitative indicator of the temporal characteristics of fMRI time-course data which could be used to measure the detectability of fMRI signals (Murphy et al., 2007). The tSNR of a time series xi was defined by:
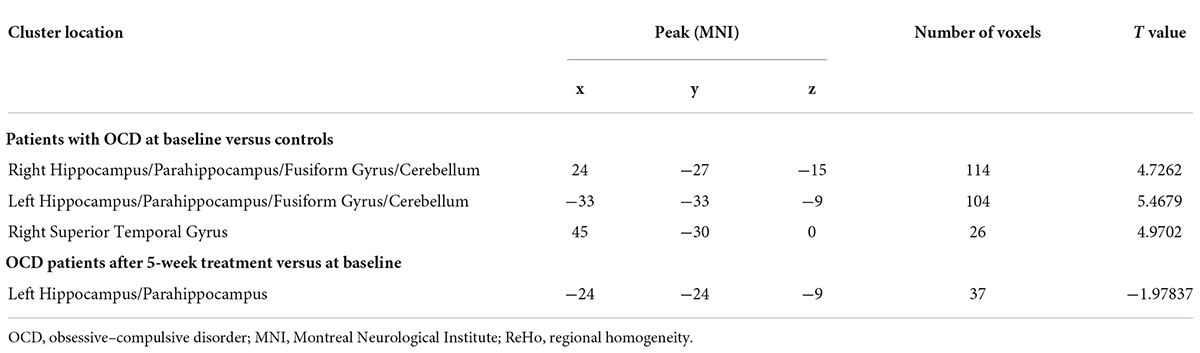
Table 3. Regions with abnormal ReHo values in patients with OCD at baseline and alterations of ReHo values after treatment.
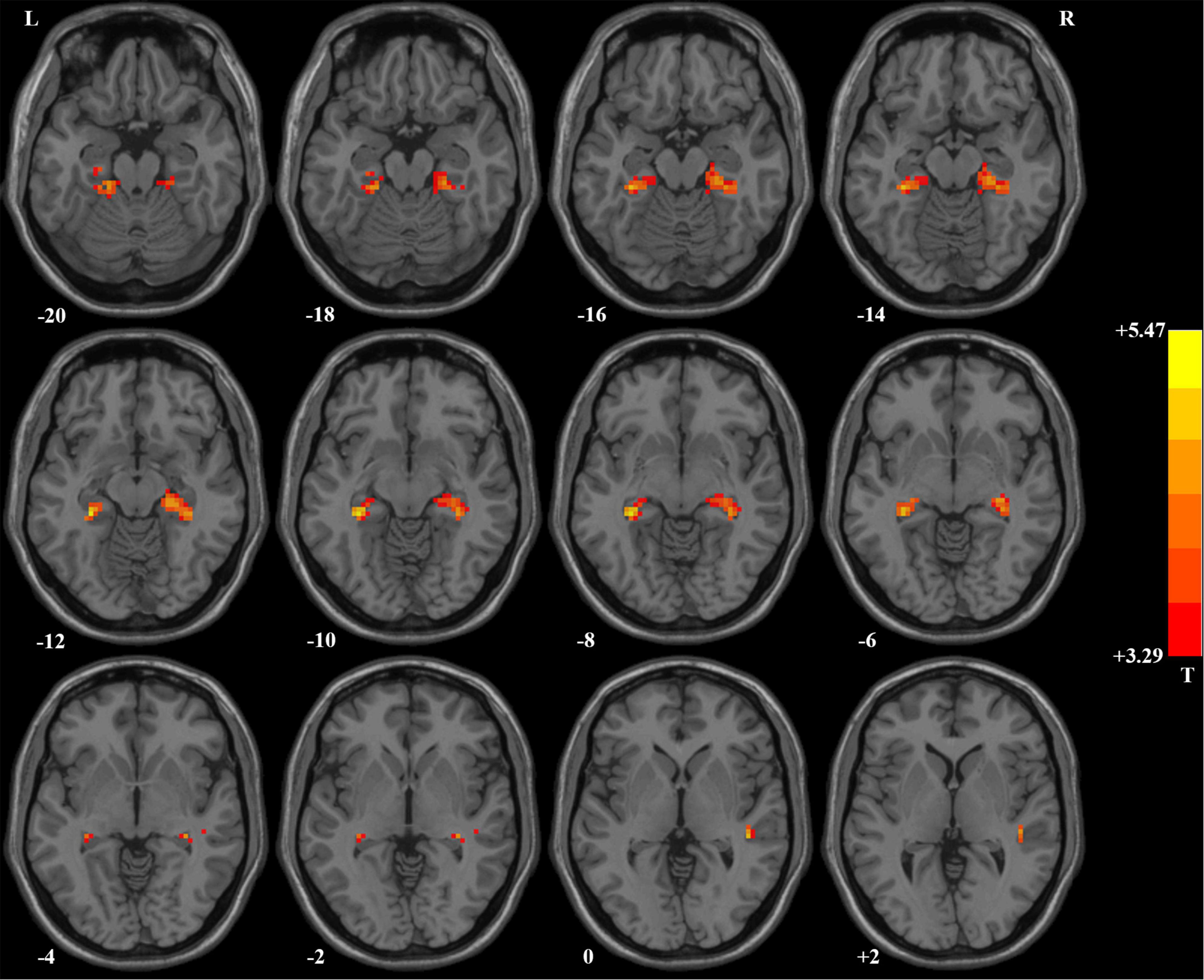
Figure 1. Brain regions with significant difference in ReHo values between patients with OCD and healthy controls. Compared with healthy controls, patients with OCD had higher ReHo values in the right superior temporal gyrus and bilateral hippocampus/parahippocampus/fusiform gyrus/cerebellum. ReHo, regional homogeneity; OCD, obsessive–compulsive disorder.
where μ was the mean of the time series, δ was its standard deviation, and N was the number of time points. In the present study, the number of time points after pre-processing of imaging data was 230. We repeated the two-sample t-tests to assess the impact of possible artifacts on results, in which the mean FD, gender, age, years of education, and tSNR were used as covariates of no interest. The results did not change. The higher ReHo values in the right STG after adding the tSNR as covariate in the two-sample t-test were shown in Supplementary Figure 3.
Support vector machine analysis result
In the combination of ReHo values in the right hippo campus/parahippocampus/fusiform gyrus/cerebellum and left hippocampus/parahippocampus/fusiform gyrus/cerebellum, 58 out of 70 participants were accurately classified with the highest accuracy. This combination was the optimal combination with a sensitivity of 73.53%, a specificity of 91.67%, and an accuracy of 82.86% [Figure 2; confidence interval (CI) 95% = 73.44–92.29%; p < 0.001, a permutation test with 100,000 repetitions]. All the combinations had a sensitivity = 67.64 ± 6.35%, a specificity = 87.30 ± 4.50%, and an accuracy = 77.75 ± 2.72% (Figure 2).
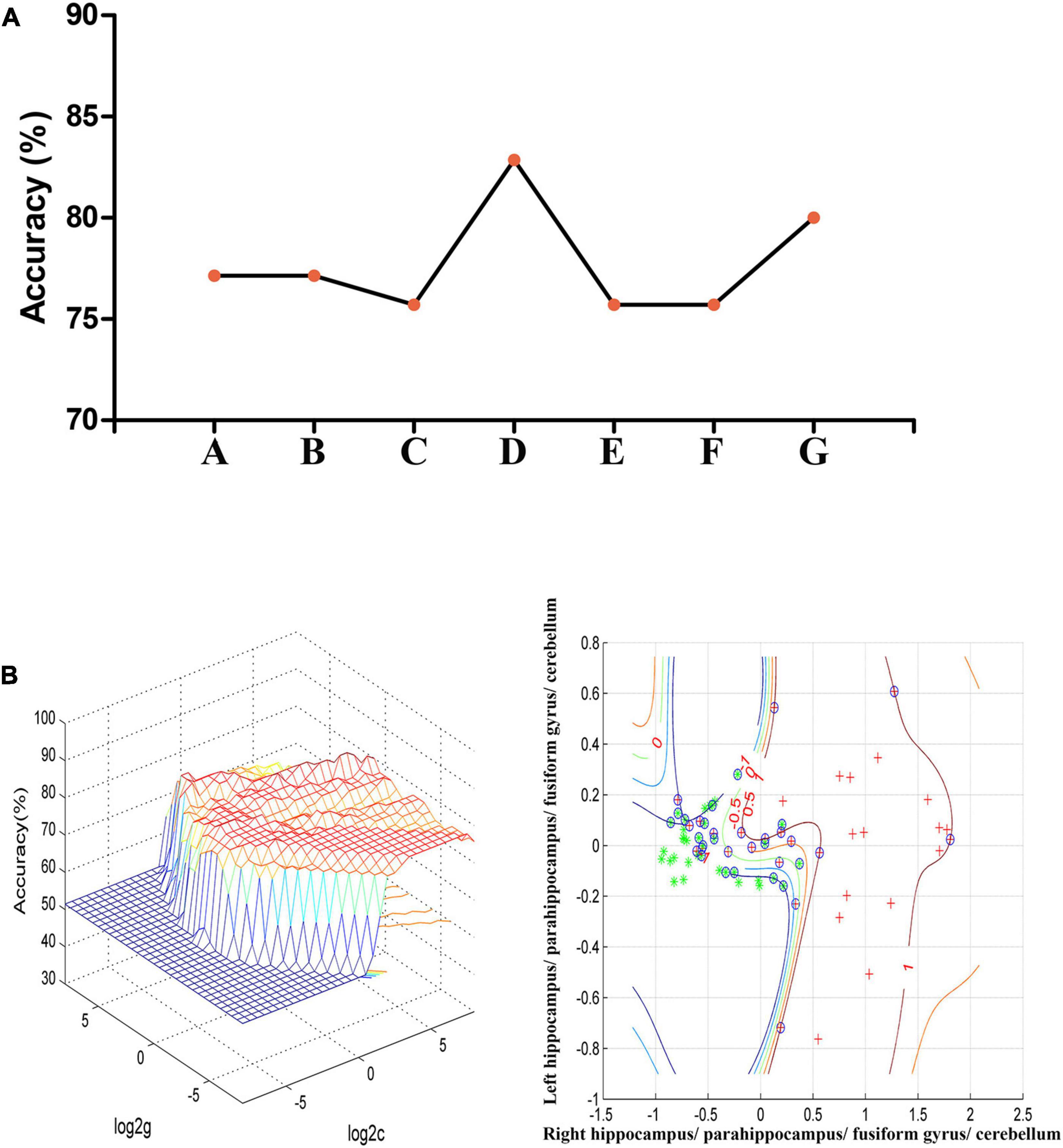
Figure 2. (A) The accuracy of classification of seven combinations of ReHo values. A represents the right hippocampus/parahippocampus/fusiform gyrus/cerebellum; B represents the left hippocampus/parahippocampus/fusiform gyrus/cerebellum; C represents the right superior temporal gyrus; D represents the right hippocampus/parahippocampus/fusiform gyrus/cerebellum and left hippocampus/parahippocampus/fusiform gyrus/cerebellum; E represents the right hippocampus/parahippocampus/fusiform gyrus/cerebellum and right superior temporal gyrus; F represents the left hippocampus/parahippocampus/fusiform gyrus/cerebellum and right superior temporal gyrus; G represents the right hippocampus/parahippocampus/fusiform gyrus/cerebellum, left hippocampus/parahippocampus/fusiform gyrus/cerebellum, and right superior temporal gyrus. (B) SVM analysis of the combination of ReHo values in the right hippocampus/parahippocampus/fusiform gyrus/cerebellum and left hippocampus/parahippocampus/fusiform gyrus/cerebellum. Sensitivity = 73.53%, specificity = 91.67%, and accuracy = 82.86%. SVM, support vector machines; ReHo, regional homogeneity.
Regional homogeneity analysis in pre-treatment and post-treatment patients with obsessive–compulsive disorder
We compared the ReHo values of patients with OCD who completed follow-up in brain regions with abnormal ReHo values at baseline. Compared with baseline data, patients with OCD showed significantly decreased ReHo values after 5-week paroxetine treatment in the left hippocampus and parahippocampus (Table 3 and Figure 3).
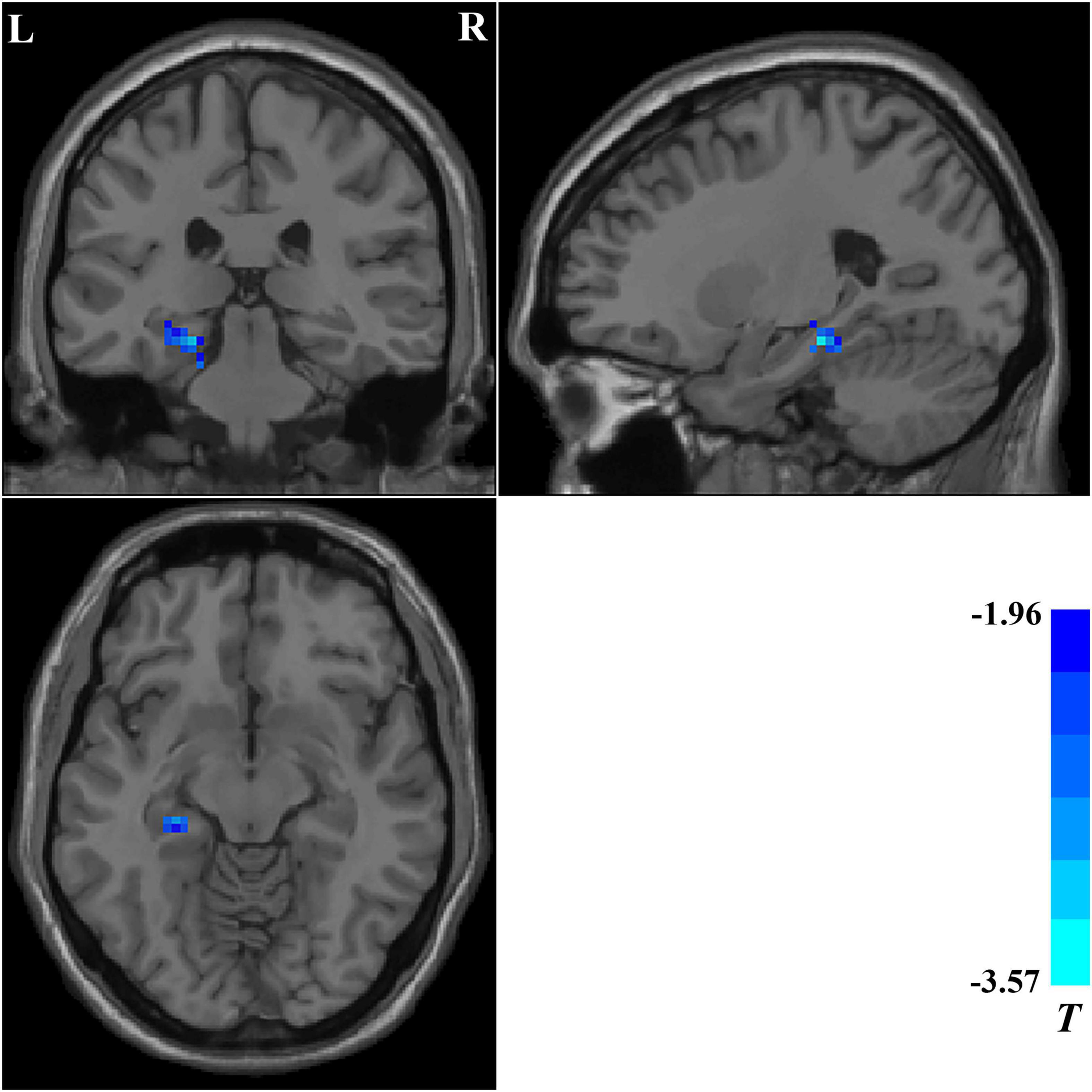
Figure 3. Brain regions showing significant changes in ReHo values after treatment. Compared with the baseline data, patients with OCD showed significantly decreased ReHo values in the left hippocampus and parahippocampus after a 5-week paroxetine treatment. ReHo, regional homogeneity; OCD, obsessive–compulsive disorder.
Support vector regression analysis result
The ReHo values in the left hippocampus/parahippocampus/fusiform gyrus/cerebellum at baseline could be used to predict early therapeutic response. The SVR results showed positive correlations between actual and predicted RRs in the total scores of Y-BOCS (r = 0.590, p = 0.002, Figure 4A), and scores of obsession (r = 0.661, p < 0.001, Figure 4B) and compulsion (r = 0.595, p = 0.002, Figure 4C). The significance threshold was p = 0.017 after Bonferroni correction (0.05/3 for the three types of Y-BOCS scores). The Bland Altman plots of the regression results were shown in the Supplementary Figure 4.
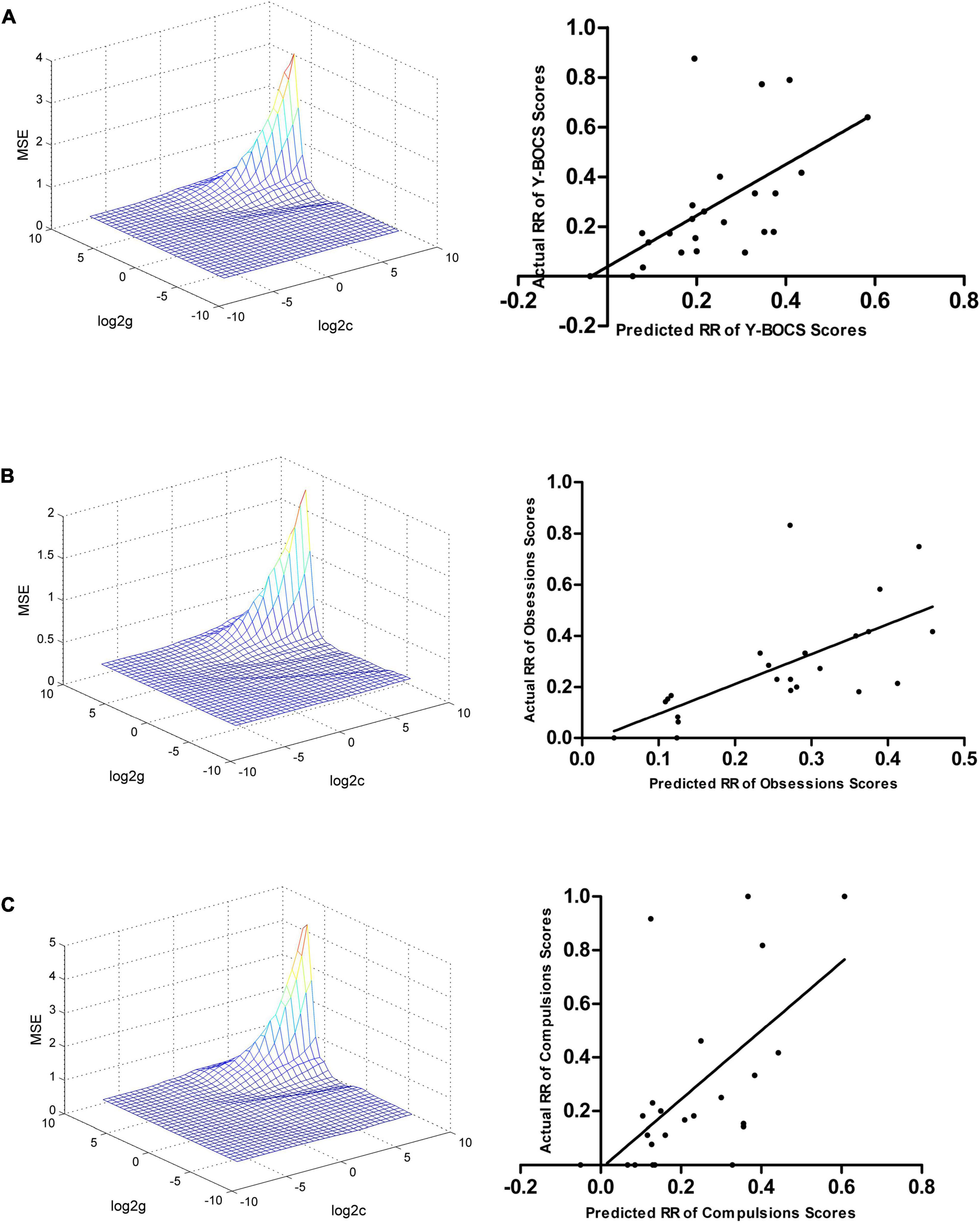
Figure 4. Support vector regression results suggested that the ReHo values in the left hippocampus, parahippocampus, fusiform gyrus, and cerebellum at baseline can be used to predict early therapeutic response. (A) SVR results showed a positive correlation between the actual and predicted RRs in the total scores of Y-BOCS (r = 0.590, p = 0.002). (B) SVR results showed a positive correlation between the actual and predicted RRs in scores of obsession (r = 0.661, p < 0.001). (C) SVR results showed a positive correlation between the actual and predicted RRs in the scores of compulsion (r = 0.595, p = 0.002). SVR, support vector regression; ReHo, regional homogeneity; RR, reduction rate.
Correlation between regional homogeneity values and clinical symptoms of patients with obsessive–compulsive disorder
No significant correlation was found between abnormal ReHo values and clinical symptoms (illness duration, the scores of Y-BOCS, obsessions, compulsions, HAMD, HAMA, SDSS, subscales of CSQ, and subscales of B-CATS) in patients with OCD at baseline.
The RR of the ReHo values in the left hippocampus and parahippocampus, where ReHo values decreased significantly after the 5-week paroxetine treatment, was positively correlated with the RRs of the scores of Y-BOCS (r = 0.407, p = 0.049.) and obsession (r = 0.422, p = 0.040).
Discussion
This longitudinal study found that drug-naive patients with OCD had higher ReHo values in the right STG, and bilateral hippocampus/parahippocampus/fusiform gyrus/cerebellum than HCs. After the 5-week treatment with paroxetine (40 mg/day), patients showed significant clinical improvement in the symptoms of OCD, depressive symptoms, anxiety symptoms, social function, active coping, and cognition compared with their baseline assessment. The elevated ReHo values in the left hippocampus and parahippocampus decreased significantly after treatment, and the RR of ReHo values was positively correlated with the RRs of the scores of Y-BOCS and obsession. The SVR results showed that the ReHo values in the left hippocampus/parahippocampus/fusiform gyrus/cerebellum at baseline could be applied to predict early therapeutic response. The ReHo values in the right hippocampus/parahippocampus/fusiform gyrus/cerebellum and left hippocampus/parahippocampus/fusiform gyrus/cerebellum at baseline could be used to distinguish patients with OCD from HCs with high accuracy, sensitivity, and specificity.
Cumulative evidence suggested that abnormal CSTC circuits were not the only important pathological basis of OCD. Other cerebral regions outside the CSTC circuits were involved in OCD, such as the parietal cortex, insula, cerebellum, hippocampus, parahippocampus, fusiform gyrus, and STG (Del Casale et al., 2011; Zhao et al., 2017; Hao et al., 2019; Xia et al., 2019; Yang et al., 2019). A previous study suggested that a combination of amplitude of low-frequency fluctuation (ALFF) and ReHo which depicted different regional characteristics of resting-state fMRI might yield a more comprehensive pathophysiological framework for mental disorders (Sroubek et al., 2013). Thus, we measured ALFF in the present study. Compared with HCs, patients with OCD had higher ALFF values in the CSTC circuits such as the orbitofrontal cortex, superior medial frontal cortex, and anterior cingulate cortex (Supplementary Figure 5 and Supplementary Table 1). However, abnormal ALFF values in the patients with OCD did not change significantly after treatment (Supplementary Table 1).
Increased regional homogeneity values in the right superior temporal gyrus in patients with obsessive–compulsive disorder at baseline
Increased brain spontaneous activities in the right STG in patients with OCD were found at baseline. Evidence suggested the existence of hemispheric bias in STG, with a preference for the right STG in processing social and emotional stimuli and for the left STG in processing language stimuli (Narumoto et al., 2001; Redcay, 2008). Xia et al. (2019) reported that OCD patients with anhedonia showed significantly decreased ALFF in the right STG compared with OCD patients without anhedonia, and the ALFF values in the right STG were negatively correlated with the severity of social anhedonia. Anatomically, the STG is directly connected with the caudate nucleus, which is one of the central nodes for reward processing (Yeterian and Pandya, 1998). Abnormal functional connectivity between the caudate nucleus and the right STG in patients with OCD has been reported (Chen et al., 2016). McLellan et al. (2018) reported that adolescents with treatment-resistant depression and history of suicide attempt had reduced right STG volume compared with HCs. Pan et al. (2015) also found that patients with major depressive disorder and a history of suicide attempt had lower right STG volume compared with HCs. These findings suggested that the right STG was an important region for emotional regulation. Park et al. (2011) found that the right STG served as a central region for the network nodes responsible for retaining stimulus through gamma activity, suggesting that the right STG had an important role in working memory. Paulus et al. (2005) found that bilateral STG showed increased activity in a decision-making situation, suggesting that STG was involved in information assessment and action selection. In summary, increased brain spontaneous activities in the right STG might be a compensatory mechanism for impaired emotional regulation, working memory, and decision-making in patients with OCD (Abramovitch et al., 2013; Robbins et al., 2019).
Increased regional homogeneity values in the bilateral fusiform gyrus in patients with obsessive–compulsive disorder at baseline
We found increased brain spontaneous activities in the bilateral fusiform gyrus in patients at baseline. Previous studies reported that patients with OCD had abnormal local spontaneous activities (Yang et al., 2010; Hao et al., 2019) and functional connectivity (Ciesielski et al., 2012; Beucke et al., 2014) in the fusiform gyrus. The fusiform gyrus is commonly considered a key structure for high-level vision, such as face perception, reading, and object recognition (Kanwisher et al., 1997; McCandliss et al., 2003; Weiner and Zilles, 2016). Evidence suggested that fusiform gyrus had an important role in visuospatial and visuoperceptual working memory (Druzgal and D’Esposito, 2001; Sala-Llonch et al., 2015), social perception (Schultz, 2005), social cognition (Schultz et al., 2003), and emotional response inhibition (Berlin et al., 2015). OCD might be a consequence of dysfunctional circuits for response inhibition, working memory, cognitive flexibility, error monitoring, and planning (goal-directed behavior) (Abramovitch et al., 2013; Robbins et al., 2019). Our findings implied that the fusiform gyrus was a crucial structure in the neuropsychological mechanism underlying OCD.
Increased regional homogeneity values in the bilateral cerebellum in patients with obsessive–compulsive disorder at baseline
Consistent with previous studies (Ping et al., 2013; Yang et al., 2015; Chen et al., 2016), increased ReHo values in the bilateral cerebellum were found in patients with OCD at baseline. Previous studies revealed the important role of cerebellum in attention (Schweizer et al., 2007; Mannarelli et al., 2019). Increased brain spontaneous activities in the cerebellum might be a compensatory mechanism for impaired attention, especially selective attention, which was identified to be central to the symptomatology of OCD (Clayton et al., 1999; Muller and Roberts, 2005). Previous studies also suggested the function of emotional regulation in the cerebellum (Schutter and van Honk, 2009; Baumann and Mattingley, 2012). The dysfunction of emotional regulation might contribute to the maintenance of distress and anxiety in patients with OCD (Fergus and Bardeen, 2014; Stern et al., 2014). Cerebellum has a crucial role in fear conditioning (Sacchetti et al., 2002, 2005), prediction, especially prediction errors (Schlerf et al., 2012; Ernst et al., 2019), response inhibition (Mostofsky et al., 2003; Wynn et al., 2019), and working memory (Durisko and Fiez, 2010; Ashida et al., 2019). Increased brain spontaneous activities in the cerebellum might be a compensatory mechanism for impaired fear conditioning, response inhibition, and working memory and could be used to explain the obsessive thoughts and repetitive behaviors that originated from excessive error prediction.
Elevated regional homogeneity values in the bilateral hippocampus and parahippocampus decreased significantly after treatment
A previous longitudinal study found that patients with OCD had higher ALFF values in the left hippocampus/parahippocampus than HCs at baseline, and the differences disappeared after a 4-week CBT treatment (Zhao et al., 2017). We found increased ReHo values in the bilateral hippocampus and parahippocampus in patients at baseline. Moreover, after the 5-week treatment with paroxetine, the elevated ReHo values in the left hippocampus and parahippocampus decreased significantly. The hippocampal–cortical system, including the hippocampal region and its widespread cortical targets, might provide the neural building blocks for simulating upcoming events during planning and decision-making (prediction) and when imagining novel scenarios (imagination) (Buckner, 2010; Stachenfeld et al., 2017). In the hippocampal–cortical system, the hippocampal region was connected to the cortical regions through the adjacent entorhinal cortex, which was projected to the parahippocampal and perirhinal cortices. As a result, the parahippocampal and perirhinal cortices were projected to widespread cortical areas. Increased brain spontaneous activities in the hippocampus/parahippocampus might partly explain the excessive worries and anxiety over upcoming events through inappropriate imagination and prediction in OCD (O’Connor and Aardema, 2003). There was other evidence about the increased brain spontaneous activities in the parahippocampus mediated the excessive worries and anxiety over upcoming events in OCD. A task-based fMRI study was performed to test which brain regions contributed to suspicion in a repeated bargaining game and found that activations in the bilateral parahippocampus were associated with suspicion. Furthermore, less credibility appeared when more sensitive parahippocampal activation was observed (Bhatt et al., 2012). Thus, the parahippocampus might contribute to suspicion; excessive suspicion might be the basis for obsessive thoughts and repetitive behaviors and the accompanying excessive worries and anxiety for upcoming events in OCD. In addition, the hippocampus played a crucial role in response inhibition (Davidson and Jarrard, 2004) and working memory (Leszczynski, 2011; Yonelinas, 2013). Task-based fMRI (Luck et al., 2010) and lesion studies (Hasselmo and Stern, 2006; Smits et al., 2009) suggested that the parahippocampus had a critical role in working memory. Increased brain spontaneous activities in the hippocampus/parahippocampus might serve as compensatory mechanisms for impaired response inhibition and working memory. The hippocampus plays an important role in contextual fear conditioning (Anagnostaras et al., 2001; Sanders et al., 2003). Dysfunction of fear acquisition and extinction is a core perspective in the cognitive behavioral model of OCD, which underlies exposure-based CBT (McGuire et al., 2016; Cooper and Dunsmoor, 2021). In a task-based fMRI study performed by Shapira et al., 2003, patients with OCD showed significantly increased activation in the left parahippocampus compared with HCs when subjected to disgust-inducing stimuli. This finding suggested that the parahippocampus contributed to the arousal of disgust, which was an important symptomatology of OCD (Knowles et al., 2018). In summary, our findings highlighted the crucial role of the hippocampal–cortical system in the neuropsychological mechanism of OCD.
Elevated ReHo values in the left hippocampus/parahippocampus decreased significantly after the 5-week treatment with paroxetine, and the RR of the ReHo values in the left hippocampus/parahippocampus was positively correlated with the RRs of the scores of Y-BOCS and obsession. These findings implied the important role of the hippocampus and parahippocampus in the pharmacological mechanism underlying SSRIs in the treatment of OCD. Early brain functional changes induced by pharmacotherapy (normalization in the present study) were related to the effects of drugs on the improvement of OCD (treatment effects) rather than their side effects. Consistent with our findings, Gilbert et al. (2000) reported that the greater thalamic volume in patients with OCD than HCs was reduced significantly after 12-week treatment with paroxetine and the reduction of the thalamic volume was associated with the decrease in symptomatic severity. Rosenberg et al. (2000) found that the higher glutamatergic concentration in the caudate of pediatric patients with OCD than HCs decreased significantly after 12-week treatment with paroxetine. Denormalization in brains of patients with OCD after treatment with SSRIs was also observed in the previous studies. Hansen et al. (2002) reported that regional cerebral glucose metabolic rate in the right caudate nucleus of patients with OCD reduced significantly after 12–20 weeks of treatment with paroxetine. Lázaro et al. (2008) observed that patients with OCD had decreased activation in the left putamen and insula in response to the serial reaction task after 6-month treatment with fluoxetine (activation in the left putamen and insula in response to the serial reaction task in patients with OCD before treatment showed no significant difference related to HCs). These normalization and denormalization in brains of patients with OCD could be explained by treatment effects and side effects of pharmacotherapy, respectively (Abbott et al., 2013).
Furthermore, the ReHo values in the left hippocampus/parahippocampus/fusiform gyrus/cerebellum at baseline can be applied to the prediction of early therapeutic response. Sanematsu et al. (2010) found that during symptom provocation task, patients with OCD showed brain activation in the right cerebellum, and the pre-treatment activation in the right cerebellum could be used to predict the improvement in the Y-BOCS score after the 12-week treatment with fluvoxamine. Although different SSRIs were used in the treatments of OCD, their results were consistent with our findings.
Strengths and limitations
This study was the first to apply resting-state fMRI technique combined with local spontaneous brain activity analysis (ReHo analysis) and methods of machine learning, including SVM and SVR analyses, to explore the neuropsychological mechanism underlying OCD, pharmacological mechanism underlying OCD treatment, and the possibility of building models for the diagnosis and prediction of early treatment response. Drug-naïve patients were recruited, and the age range was from 18 to 49 years old to avoid the impacts of drug, neurodevelopment, and aging on local spontaneous brain activity. However, several limitations were noted. First, this monocentric study with a relatively small sample size and high drop-out rate due to the inconvenience of participation in the follow-up during the COVID-19 pandemic might restrict the possibility of the generalization of these outcomes to other centers. Second, we only performed a 5-week follow-up for the patients, who were treated with paroxetine. Although the patients showed a significant improvement in clinical symptoms after treatment, the insufficient treatment might result in a false negative outcome in the normalization of increased ReHo values. Third, although the tSNR was measured which could be used to assess the detectability of fMRI signals, the possible artifacts could bias the results. Fourth, although the cerebellum was involved in the neurophysiological mechanism underlying OCD, with a slice thickness of 4 mm it might be difficult to infer precise localization within the cerebellum. Fifth, denormalization in brains of patients with OCD related to side effects of SSRIs was reported previously which was opposite to our findings. Besides, other domains of side effects of the SSRIs use were reported before. Sayyah et al. (2016) reported that SSRIs use could cause cognitive dysfunction in patients with OCD or depression in the acute phase of treatment. Skandali et al. (2018) reported that acute escitalopram administration on healthy volunteers impaired functions of cognitive flexibility and learning. Thus, explaining normalization of ReHo values in the left hippocampus/parahippocampus of patients with OCD after 5-week treatment with paroxetine should be cautious. Sixth, previous studies reported numerous abnormalities of distant functional connectivity in patients with OCD (Kaufmann et al., 2013; Beucke et al., 2014). We cannot measure abnormal distant functional connectivity using the ReHo indicator in the present study.
Conclusion
This study highlighted the important role of the hippocampal–cortical system in the neuropsychological mechanisms underlying OCD and the pharmacological mechanism underlying the treatments of OCD, as well as the possibility of building models for the diagnosis and prediction of early treatment response based on the spontaneous activity in the hippocampal–cortical system. This study also revealed that the early functional brain changes induced by pharmacotherapy might be related to the treatment effects rather than the drugs per se or their side effects.
Data Availability Statement
The raw data supporting the conclusions of this article will be made available by the authors, without undue reservation.
Ethics statement
The studies involving human participants were reviewed and approved by Research Ethics Committee of Second Xiangya Hospital of Central South University in China. The patients/participants provided their written informed consent to participate in this study.
Author contributions
HY: methodology, data curation, formal analysis, and writing – review and editing. XS: conceptualization, data curation, and writing – review and editing. HL: data curation. FL: conceptualization and funding acquisition. WG: methodology, data curation, writing – review and editing, and funding acquisition. All authors contributed to the article and approved the submitted version.
Funding
This study was supported by grants from the National Natural Science Foundation of China (Grant no. 82171508), Natural Science Foundation of Hunan (Grant no. 2020JJ4784), Science and Technology Program of Hunan Province (Grant no. 2020SK53413), and Natural Science Foundation of Tianjin (Grant no. 18JCQNJC10900).
Conflict of Interest
The authors declare that the research was conducted in the absence of any commercial or financial relationships that could be construed as a potential conflict of interest.
Publisher’s Note
All claims expressed in this article are solely those of the authors and do not necessarily represent those of their affiliated organizations, or those of the publisher, the editors and the reviewers. Any product that may be evaluated in this article, or claim that may be made by its manufacturer, is not guaranteed or endorsed by the publisher.
Acknowledgments
We thank all the research participants.
Supplementary Material
The Supplementary Material for this article can be found online at: https://www.frontiersin.org/articles/10.3389/fncel.2022.906534/full#supplementary-material
Footnotes
References
Abbott, C. C., Jaramillo, A., Wilcox, C. E., and Hamilton, D. A. (2013). Antipsychotic drug effects in schizophrenia: a review of longitudinal FMRI investigations and neural interpretations. Curr. Med. Chem. 20, 428–437. doi: 10.2174/0929867311320030014
Abramovitch, A., Abramowitz, J. S., and Mittelman, A. (2013). The neuropsychology of adult obsessive-compulsive disorder: a meta-analysis. Clin. Psychol. Rev. 33, 1163–1171. doi: 10.1016/j.cpr.2013.09.004
Alemany-Navarro, M., Costas, J., Real, E., Segalàs, C., Bertolín, S., Domènech, L., et al. (2019). Do polygenic risk and stressful life events predict pharmacological treatment response in obsessive compulsive disorder? A gene-environment interaction approach. Transl. Psychiatry 9:70. doi: 10.1038/s41398-019-0410-0
American Psychiatric Association [APA] (2007). Practice Guideline for the Treatment of Patients with Obsessive–Compulsive Disorder. Arlington, VA: American Psychiatric Association.
American Psychiatric Association [APA] (2013). Diagnostic and Statistical Manual of Mental Disorders, 5th Edn. Arlington, VA: American Psychiatric Association.
Anagnostaras, S. G., Gale, G. D., and Fanselow, M. S. (2001). Hippocampus and contextual fear conditioning: recent controversies and advances. Hippocampus 11, 8–17. doi: 10.1002/1098-1063(2001)11:1<8::AID-HIPO1015>3.0.CO;2-7
Ashida, R., Cerminara, N. L., Edwards, R. J., Apps, R., and Brooks, J. C. W. (2019). Sensorimotor, language, and working memory representation within the human cerebellum. Hum. Brain Mapp. 40, 4732–4747. doi: 10.1002/hbm.24733
Atmaca, M. (2019). Functional and structural neural changes in obsessive-compulsive disorder after pharmacotherapy. Curr. Neuropharmacol. 17, 737–740. doi: 10.2174/1570159x16666180613074059
Bagby, R. M., Ryder, A. G., Schuller, D. R., and Marshall, M. B. (2004). The Hamilton depression rating scale: has the gold standard become a lead weight? Am. J. Psychiatry 161, 2163–2177. doi: 10.1176/appi.ajp.161.12.2163
Baumann, O., and Mattingley, J. B. (2012). Functional topography of primary emotion processing in the human cerebellum. Neuroimage 61, 805–811. doi: 10.1016/j.neuroimage.2012.03.044
Ben-Hur, A., Ong, C. S., Sonnenburg, S., Scholkopf, B., and Ratsch, G. (2008). Support vector machines and kernels for computational biology. PLoS Comput. Biol. 4:e1000173. doi: 10.1371/journal.pcbi.1000173
Berlin, H. A., Schulz, K. P., Zhang, S., Turetzky, R., Rosenthal, D., and Goodman, W. (2015). Neural correlates of emotional response inhibition in obsessive-compulsive disorder: a preliminary study. Psychiatry Res. 234, 259–264. doi: 10.1016/j.pscychresns.2015.09.019
Beucke, J. C., Sepulcre, J., Eldaief, M. C., Sebold, M., Kathmann, N., and Kaufmann, C. (2014). Default mode network subsystem alterations in obsessive-compulsive disorder. Br. J. Psychiatry 205, 376–382. doi: 10.1192/bjp.bp.113.137380
Beucke, J. C., Sepulcre, J., Talukdar, T., Linnman, C., Zschenderlein, K., Endrass, T., et al. (2013). Abnormally high degree connectivity of the orbitofrontal cortex in obsessive-compulsive disorder. JAMA Psychiatry 70, 619–629. doi: 10.1001/jamapsychiatry.2013.173
Bhatt, M. A., Lohrenz, T., Camerer, C. F., and Montague, P. R. (2012). Distinct contributions of the amygdala and parahippocampal gyrus to suspicion in a repeated bargaining game. Proc. Natl. Acad. Sci. U.S.A. 109, 8728–8733. doi: 10.1073/pnas.1200738109
Bijanki, K. R., Pathak, Y. J., Najera, R. A., Storch, E. A., Goodman, W. K., Simpson, H. B., et al. (2021). Defining functional brain networks underlying obsessive-compulsive disorder (OCD) using treatment-induced neuroimaging changes: a systematic review of the literature. J. Neurol. Neurosurg. Psychiatry 92, 776–786. doi: 10.1136/jnnp-2020-324478
Bloch, M. H., McGuire, J., Landeros-Weisenberger, A., Leckman, J. F., and Pittenger, C. (2010). Meta-analysis of the dose-response relationship of SSRI in obsessive-compulsive disorder. Mol. Psychiatry 15, 850–855. doi: 10.1038/mp.2009.50
Bruin, W., Denys, D., and van Wingen, G. (2019). Diagnostic neuroimaging markers of obsessive-compulsive disorder: initial evidence from structural and functional MRI studies. Prog. Neuropsychopharmacol. Biol. Psychiatry 91, 49–59. doi: 10.1016/j.pnpbp.2018.08.005
Buckner, R. L. (2010). The role of the hippocampus in prediction and imagination. Annu. Rev. Psychol. 61, 27–48, c21–c28. doi: 10.1146/annurev.psych.60.110707.163508
Chang, C.-C., and Lin, C.-J. (2011). LIBSVM: a library for support vector machines. ACM Trans. Intell. Syst. Technol. 2, 1–27. doi: 10.1145/1961189.1961199
Chao-Gan, Y., and Yu-Feng, Z. (2010). DPARSF: a MATLAB toolbox for “pipeline” data analysis of resting-state fMRI. Front. Syst. Neurosci. 4:13. doi: 10.3389/fnsys.2010.00013
Chen, X., Lu, B., and Yan, C. G. (2018). Reproducibility of R-fMRI metrics on the impact of different strategies for multiple comparison correction and sample sizes. Hum. Brain Mapp. 39, 300–318. doi: 10.1002/hbm.23843
Chen, Y., Juhás, M., Greenshaw, A. J., Hu, Q., Meng, X., Cui, H., et al. (2016). Abnormal resting-state functional connectivity of the left caudate nucleus in obsessive-compulsive disorder. Neurosci. Lett. 623, 57–62. doi: 10.1016/j.neulet.2016.04.030
Ciesielski, K. T., Rauch, S. L., Ahlfors, S. P., Vangel, M. E., Wilhelm, S., Rosen, B. R., et al. (2012). Role of medial cortical networks for anticipatory processing in obsessive-compulsive disorder. Hum. Brain Mapp. 33, 2125–2134. doi: 10.1002/hbm.21341
Clayton, I. C., Richards, J. C., and Edwards, C. J. (1999). Selective attention in obsessive-compulsive disorder. J. Abnorm. Psychol. 108, 171–175. doi: 10.1037//0021-843x.108.1.171
Cooper, S. E., and Dunsmoor, J. E. (2021). Fear conditioning and extinction in obsessive-compulsive disorder: a systematic review. Neurosci. Biobehav. Rev. 129, 75–94. doi: 10.1016/j.neubiorev.2021.07.026
Davidson, T. L., and Jarrard, L. E. (2004). The hippocampus and inhibitory learning: a ‘Gray’ area? Neurosci. Biobehav. Rev. 28, 261–271. doi: 10.1016/j.neubiorev.2004.02.001
Del Casale, A., Kotzalidis, G. D., Rapinesi, C., Serata, D., Ambrosi, E., Simonetti, A., et al. (2011). Functional neuroimaging in obsessive-compulsive disorder. Neuropsychobiology 64, 61–85. doi: 10.1159/000325223
Druzgal, T. J., and D’Esposito, M. (2001). Activity in fusiform face area modulated as a function of working memory load. Brain Res. Cogn. Brain Res. 10, 355–364. doi: 10.1016/s0926-6410(00)00056-2
Dunlop, K., Woodside, B., Olmsted, M., Colton, P., Giacobbe, P., and Downar, J. (2016). Reductions in cortico-striatal hyperconnectivity accompany successful treatment of obsessive-compulsive disorder with dorsomedial prefrontal rTMS. Neuropsychopharmacology 41, 1395–1403. doi: 10.1038/npp.2015.292
Durisko, C., and Fiez, J. A. (2010). Functional activation in the cerebellum during working memory and simple speech tasks. Cortex 46, 896–906. doi: 10.1016/j.cortex.2009.09.009
Ernst, T. M., Brol, A. E., Gratz, M., Ritter, C., Bingel, U., Schlamann, M., et al. (2019). The cerebellum is involved in processing of predictions and prediction errors in a fear conditioning paradigm. eLife 8:e46831. doi: 10.7554/eLife.46831
Fergus, T. A., and Bardeen, J. R. (2014). Emotion regulation and obsessive–compulsive symptoms: a further examination of associations. J. Obsessive Compuls. Relat. Disord. 3, 243–248. doi: 10.1016/j.jocrd.2014.06.001
Fineberg, N. A., Brown, A., Reghunandanan, S., and Pampaloni, I. (2012). Evidence-based pharmacotherapy of obsessive-compulsive disorder. Int. J. Neuropsychopharmacol. 15, 1173–1191. doi: 10.1017/s1461145711001829
Fineberg, N. A., Reghunandanan, S., Brown, A., and Pampaloni, I. (2013). Pharmacotherapy of obsessive-compulsive disorder: evidence-based treatment and beyond. Aust. N. Z. J. Psychiatry 47, 121–141. doi: 10.1177/0004867412461958
Folkman, S., and Lazarus, R. S. (1988). Coping as a mediator of emotion. J. Pers. Soc. Psychol. 54, 466–475.
Gaonkar, B., Shinohara, T. R., and Davatzikos, C. (2015). Interpreting support vector machine models for multivariate group wise analysis in neuroimaging. Med. Image Anal. 24, 190–204. doi: 10.1016/j.media.2015.06.008
Gilbert, A. R., Moore, G. J., Keshavan, M. S., Paulson, L. A., Narula, V., Mac Master, F. P., et al. (2000). Decrease in thalamic volumes of pediatric patients with obsessive-compulsive disorder who are taking paroxetine. Arch. Gen. Psychiatry 57, 449–456. doi: 10.1001/archpsyc.57.5.449
Gonçalves, Ó. F., Carvalho, S., Leite, J., Fernandes-Gonçalves, A., Carracedo, A., and Sampaio, A. (2016). Cognitive and emotional impairments in obsessive-compulsive disorder: evidence from functional brain alterations. Porto Biomed. J. 1, 92–105. doi: 10.1016/j.pbj.2016.07.005
Göttlich, M., Krämer, U. M., Kordon, A., Hohagen, F., and Zurowski, B. (2014). Decreased limbic and increased fronto-parietal connectivity in unmedicated patients with obsessive-compulsive disorder. Hum. Brain Mapp. 35, 5617–5632. doi: 10.1002/hbm.22574
Göttlich, M., Krämer, U. M., Kordon, A., Hohagen, F., and Zurowski, B. (2015). Resting-state connectivity of the amygdala predicts response to cognitive behavioral therapy in obsessive compulsive disorder. Biol. Psychol. 111, 100–109. doi: 10.1016/j.biopsycho.2015.09.004
Hansen, E. S., Hasselbalch, S., Law, I., and Bolwig, T. G. (2002). The caudate nucleus in obsessive-compulsive disorder. Reduced metabolism following treatment with paroxetine: a PET study. Int. J. Neuropsychopharmacol. 5, 1–10. doi: 10.1017/s1461145701002681
Hao, H., Chen, C., Mao, W., Xia, W., Yi, Z., Zhao, P., et al. (2019). Alterations in resting-state local functional connectivity in obsessive-compulsive disorder. J. Affect. Disord. 245, 113–119. doi: 10.1016/j.jad.2018.10.112
Hasselmo, M. E., and Stern, C. E. (2006). Mechanisms underlying working memory for novel information. Trends Cogn. Sci. 10, 487–493. doi: 10.1016/j.tics.2006.09.005
Hoexter, M. Q., Dougherty, D. D., Shavitt, R. G., D’Alcante, C. C., Duran, F. L., Lopes, A. C., et al. (2013). Differential prefrontal gray matter correlates of treatment response to fluoxetine or cognitive-behavioral therapy in obsessive-compulsive disorder. Eur. Neuropsychopharmacol. 23, 569–580. doi: 10.1016/j.euroneuro.2012.06.014
Hsu, C.-W., Chang, C.-C., and Lin, C.-J. (2003). A Practical Guide to Support Vector Classification. Taipei: National Taiwan University.
Hurford, I. M., Ventura, J., Marder, S. R., Reise, S. P., and Bilder, R. M. (2018). A 10-minute measure of global cognition: validation of the brief cognitive assessment tool for schizophrenia (B-CATS). Schizophr. Res. 195, 327–333. doi: 10.1016/j.schres.2017.08.033
Issari, Y., Jakubovski, E., Bartley, C. A., Pittenger, C., and Bloch, M. H. (2016). Early onset of response with selective serotonin reuptake inhibitors in obsessive-compulsive disorder: a meta-analysis. J. Clin. Psychiatry 77, e605–e611. doi: 10.4088/JCP.14r09758
Kanwisher, N., McDermott, J., and Chun, M. M. (1997). The fusiform face area: a module in human extrastriate cortex specialized for face perception. J. Neurosci. 17, 4302–4311. doi: 10.1523/jneurosci.17-11-04302.1997
Kaufmann, C., Beucke, J. C., Preuße, F., Endrass, T., Schlagenhauf, F., Heinz, A., et al. (2013). Medial prefrontal brain activation to anticipated reward and loss in obsessive-compulsive disorder. Neuroimage Clin. 2, 212–220. doi: 10.1016/j.nicl.2013.01.005
Knopp, J., Knowles, S., Bee, P., Lovell, K., and Bower, P. (2013). A systematic review of predictors and moderators of response to psychological therapies in OCD: do we have enough empirical evidence to target treatment? Clin. Psychol. Rev. 33, 1067–1081. doi: 10.1016/j.cpr.2013.08.008
Knowles, K. A., Jessup, S. C., and Olatunji, B. O. (2018). Disgust in anxiety and obsessive-compulsive disorders: recent findings and future directions. Curr. Psychiatry Rep. 20:68. doi: 10.1007/s11920-018-0936-5
Lázaro, L., Caldú, X., Junqué, C., Bargalló, N., Andrés, S., Morer, A., et al. (2008). Cerebral activation in children and adolescents with obsessive-compulsive disorder before and after treatment: a functional MRI study. J. Psychiatr. Res. 42, 1051–1059. doi: 10.1016/j.jpsychires.2007.12.007
Leszczynski, M. (2011). How does hippocampus contribute to working memory processing? Front. Hum. Neurosci. 5:168. doi: 10.3389/fnhum.2011.00168
Liu, Y., Zhang, Y., Lv, L., Wu, R., Zhao, J., and Guo, W. (2018). Abnormal neural activity as a potential biomarker for drug-naive first-episode adolescent-onset schizophrenia with coherence regional homogeneity and support vector machine analyses. Schizophr. Res. 192, 408–415. doi: 10.1016/j.schres.2017.04.028
Luck, D., Danion, J. M., Marrer, C., Pham, B. T., Gounot, D., and Foucher, J. (2010). The right parahippocampal gyrus contributes to the formation and maintenance of bound information in working memory. Brain Cogn. 72, 255–263. doi: 10.1016/j.bandc.2009.09.009
Mannarelli, D., Pauletti, C., Currà, A., Marinelli, L., Corrado, A., Delle Chiaie, R., et al. (2019). The cerebellum modulates attention network functioning: evidence from a cerebellar transcranial direct current stimulation and attention network test study. Cerebellum 18, 457–468. doi: 10.1007/s12311-019-01014-8
McCandliss, B. D., Cohen, L., and Dehaene, S. (2003). The visual word form area: expertise for reading in the fusiform gyrus. Trends Cogn. Sci. 7, 293–299. doi: 10.1016/s1364-6613(03)00134-7
McGuire, J. F., Orr, S. P., Wu, M. S., Lewin, A. B., Small, B. J., Phares, V., et al. (2016). FEAR CONDITIONING AND EXTINCTION IN YOUTH WITH OBSESSIVE-COMPULSIVE DISORDER. Depress. Anxiety 33, 229–237. doi: 10.1002/da.22468
McLellan, Q., Wilkes, T. C., Swansburg, R., Jaworska, N., Langevin, L. M., and MacMaster, F. P. (2018). History of suicide attempt and right superior temporal gyrus volume in youth with treatment-resistant major depressive disorder. J. Affect. Disord. 239, 291–294. doi: 10.1016/j.jad.2018.07.030
Meyer, D., Leisch, F., and Hornik, K. (2003). The support vector machine under test. Neurocomputing 55, 169–186. doi: 10.1016/s0925-2312(03)00431-4
Mikl, M., Marecek, R., Hlustík, P., Pavlicová, M., Drastich, A., Chlebus, P., et al. (2008). Effects of spatial smoothing on fMRI group inferences. Magn. Reson. Imaging 26, 490–503. doi: 10.1016/j.mri.2007.08.006
Mostofsky, S. H., Schafer, J. G., Abrams, M. T., Goldberg, M. C., Flower, A. A., Boyce, A., et al. (2003). fMRI evidence that the neural basis of response inhibition is task-dependent. Brain Res. Cogn. Brain Res. 17, 419–430. doi: 10.1016/s0926-6410(03)00144-7
Muller, J., and Roberts, J. E. (2005). Memory and attention in obsessive-compulsive disorder: a review. J. Anxiety Disord. 19, 1–28. doi: 10.1016/j.janxdis.2003.12.001
Murphy, K., Bodurka, J., and Bandettini, P. A. (2007). How long to scan? The relationship between fMRI temporal signal to noise ratio and necessary scan duration. Neuroimage 34, 565–574. doi: 10.1016/j.neuroimage.2006.09.032
Narumoto, J., Okada, T., Sadato, N., Fukui, K., and Yonekura, Y. (2001). Attention to emotion modulates fMRI activity in human right superior temporal sulcus. Brain Res. Cogn. Brain Res. 12, 225–231. doi: 10.1016/s0926-6410(01)00053-2
O’Connor, K., and Aardema, F. (2003). Fusion or confusion in obsessive compulsive disorder. Psychol. Rep. 93, 227–232. doi: 10.2466/pr0.2003.93.1.227
Ogawa, S., Tank, D. W., Menon, R., Ellermann, J. M., Kim, S. G., Merkle, H., et al. (1992). Intrinsic signal changes accompanying sensory stimulation: functional brain mapping with magnetic resonance imaging. Proc. Natl. Acad. Sci. U.S.A. 89, 5951–5955. doi: 10.1073/pnas.89.13.5951
Olatunji, B. O., Davis, M. L., Powers, M. B., and Smits, J. A. (2013). Cognitive-behavioral therapy for obsessive-compulsive disorder: a meta-analysis of treatment outcome and moderators. J. Psychiatr. Res. 47, 33–41. doi: 10.1016/j.jpsychires.2012.08.020
Pan, L. A., Ramos, L., Segreti, A., Brent, D. A., and Phillips, M. L. (2015). Right superior temporal gyrus volume in adolescents with a history of suicide attempt. Br. J. Psychiatry 206, 339–340. doi: 10.1192/bjp.bp.114.151316
Park, H., Kang, E., Kang, H., Kim, J. S., Jensen, O., Chung, C. K., et al. (2011). Cross-frequency power correlations reveal the right superior temporal gyrus as a hub region during working memory maintenance. Brain Connect. 1, 460–472. doi: 10.1089/brain.2011.0046
Paulus, M. P., Feinstein, J. S., Leland, D., and Simmons, A. N. (2005). Superior temporal gyrus and insula provide response and outcome-dependent information during assessment and action selection in a decision-making situation. Neuroimage 25, 607–615. doi: 10.1016/j.neuroimage.2004.12.055
Ping, L., Su-Fang, L., Hai-Ying, H., Zhang-Ye, D., Jia, L., Zhi-Hua, G., et al. (2013). Abnormal spontaneous neural activity in obsessive-compulsive disorder: a resting-state functional magnetic resonance imaging study. PLoS One 8:e67262. doi: 10.1371/journal.pone.0067262
Power, J. D., Barnes, K. A., Snyder, A. Z., Schlaggar, B. L., and Petersen, S. E. (2012). Spurious but systematic correlations in functional connectivity MRI networks arise from subject motion. Neuroimage 59, 2142–2154. doi: 10.1016/j.neuroimage.2011.10.018
Redcay, E. (2008). The superior temporal sulcus performs a common function for social and speech perception: implications for the emergence of autism. Neurosci. Biobehav. Rev. 32, 123–142. doi: 10.1016/j.neubiorev.2007.06.004
Reggente, N., Moody, T. D., Morfini, F., Sheen, C., Rissman, J., O’Neill, J., et al. (2018). Multivariate resting-state functional connectivity predicts response to cognitive behavioral therapy in obsessive-compulsive disorder. Proc. Natl. Acad. Sci. U.S.A. 115, 2222–2227. doi: 10.1073/pnas.1716686115
Riesel, A., Endrass, T., Auerbach, L. A., and Kathmann, N. (2015). Overactive performance monitoring as an endophenotype for obsessive-compulsive disorder: evidence from a treatment study. Am. J. Psychiatry 172, 665–673. doi: 10.1176/appi.ajp.2014.14070886
Riesel, A., Endrass, T., Kaufmann, C., and Kathmann, N. (2011). Overactive error-related brain activity as a candidate endophenotype for obsessive-compulsive disorder: evidence from unaffected first-degree relatives. Am. J. Psychiatry 168, 317–324. doi: 10.1176/appi.ajp.2010.10030416
Robbins, T. W., Vaghi, M. M., and Banca, P. (2019). Obsessive-compulsive disorder: puzzles and prospects. Neuron 102, 27–47. doi: 10.1016/j.neuron.2019.01.046
Rosa, M. J., Portugal, L., Hahn, T., Fallgatter, A. J., Garrido, M. I., Shawe-Taylor, J., et al. (2015). Sparse network-based models for patient classification using fMRI. Neuroimage 105, 493–506. doi: 10.1016/j.neuroimage.2014.11.021
Rosenberg, D. R., MacMaster, F. P., Keshavan, M. S., Fitzgerald, K. D., Stewart, C. M., and Moore, G. J. (2000). Decrease in caudate glutamatergic concentrations in pediatric obsessive-compulsive disorder patients taking paroxetine. J. Am. Acad. Child Adolesc. Psychiatry 39, 1096–1103. doi: 10.1097/00004583-200009000-00008
Ruscio, A. M., Stein, D. J., Chiu, W. T., and Kessler, R. C. (2010). The epidemiology of obsessive-compulsive disorder in the national comorbidity survey replication. Mol. Psychiatry 15, 53–63. doi: 10.1038/mp.2008.94
Sacchetti, B., Baldi, E., Lorenzini, C. A., and Bucherelli, C. (2002). Cerebellar role in fear-conditioning consolidation. Proc. Natl. Acad. Sci. U.S.A. 99, 8406–8411.
Sacchetti, B., Scelfo, B., and Strata, P. (2005). The cerebellum: synaptic changes and fear conditioning. Neuroscientist 11, 217–227. doi: 10.1177/1073858405276428
Sala-Llonch, R., Palacios, E. M., Junqué, C., Bargalló, N., and Vendrell, P. (2015). Functional networks and structural connectivity of visuospatial and visuoperceptual working memory. Front. Hum. Neurosci. 9:340. doi: 10.3389/fnhum.2015.00340
Salomoni, G., Grassi, M., Mosini, P., Riva, P., Cavedini, P., and Bellodi, L. (2009). Artificial neural network model for the prediction of obsessive-compulsive disorder treatment response. J. Clin. Psychopharmacol. 29, 343–349. doi: 10.1097/JCP.0b013e3181aba68f
Sanders, M. J., Wiltgen, B. J., and Fanselow, M. S. (2003). The place of the hippocampus in fear conditioning. Eur. J. Pharmacol. 463, 217–223. doi: 10.1016/s0014-2999(03)01283-4
Sanematsu, H., Nakao, T., Yoshiura, T., Nabeyama, M., Togao, O., Tomita, M., et al. (2010). Predictors of treatment response to fluvoxamine in obsessive-compulsive disorder: an fMRI study. J. Psychiatr. Res. 44, 193–200. doi: 10.1016/j.jpsychires.2009.08.007
Sasson, Y., Zohar, J., Chopra, M., Lustig, M., Iancu, I., and Hendler, T. (1997). Epidemiology of obsessive-compulsive disorder: a world view. J. Clin. Psychiatry 58, (Suppl. 12), 7–10.
Sayyah, M., Eslami, K., AlaiShehni, S., and Kouti, L. (2016). Cognitive function before and during treatment with selective serotonin reuptake inhibitors in patients with depression or obsessive-compulsive disorder. Psychiatry J. 2016:5480391. doi: 10.1155/2016/5480391
Schlerf, J., Ivry, R. B., and Diedrichsen, J. (2012). Encoding of sensory prediction errors in the human cerebellum. J. Neurosci. 32, 4913–4922. doi: 10.1523/jneurosci.4504-11.2012
Schultz, R. T. (2005). Developmental deficits in social perception in autism: the role of the amygdala and fusiform face area. Int. J. Dev. Neurosci. 23, 125–141. doi: 10.1016/j.ijdevneu.2004.12.012
Schultz, R. T., Grelotti, D. J., Klin, A., Kleinman, J., Van der Gaag, C., Marois, R., et al. (2003). The role of the fusiform face area in social cognition: implications for the pathobiology of autism. Philos. Trans. R. Soc. Lond. B Biol. Sci. 358, 415–427. doi: 10.1098/rstb.2002.1208
Schutter, D. J., and van Honk, J. (2009). The cerebellum in emotion regulation: a repetitive transcranial magnetic stimulation study. Cerebellum 8, 28–34. doi: 10.1007/s12311-008-0056-6
Schweizer, T. A., Alexander, M. P., Cusimano, M., and Stuss, D. T. (2007). Fast and efficient visuotemporal attention requires the cerebellum. Neuropsychologia 45, 3068–3074. doi: 10.1016/j.neuropsychologia.2007.05.018
Skandali, N., Rowe, J. B., Voon, V., Deakin, J. B., Cardinal, R. N., Cormack, F., et al. (2018). Dissociable effects of acute SSRI (escitalopram) on executive, learning and emotional functions in healthy humans. Neuropsychopharmacology 43, 2645–2651. doi: 10.1038/s41386-018-0229-z
Smits, M., Dippel, D. W., Houston, G. C., Wielopolski, P. A., Koudstaal, P. J., Hunink, M. G., et al. (2009). Postconcussion syndrome after minor head injury: brain activation of working memory and attention. Hum. Brain Mapp. 30, 2789–2803. doi: 10.1002/hbm.20709
Shapira, N. A., Liu, Y., He, A. G., Bradley, M. M., Lessig, M. C., James, G. A., et al. (2003). Brain activation by disgust-inducing pictures in obsessive-compulsive disorder. Biol. Psychiatry 54, 751–756. doi: 10.1016/s0006-3223(03)00003-9
Sroubek, A., Kelly, M., and Li, X. (2013). Inattentiveness in attention-deficit/hyperactivity disorder. Neurosci. Bull. 29, 103–110. doi: 10.1007/s12264-012-1295-6
Stachenfeld, K. L., Botvinick, M. M., and Gershman, S. J. (2017). The hippocampus as a predictive map. Nat. Neurosci. 20, 1643–1653. doi: 10.1038/nn.4650
Stern, M. R., Nota, J. A., Heimberg, R. G., Holaway, R. M., and Coles, M. E. (2014). An initial examination of emotion regulation and obsessive compulsive symptoms. J. Obsessive Compuls. Relat. Disord. 3, 109–114. doi: 10.1016/j.jocrd.2014.02.005
Tang, W., Huang, X., Li, B., Jiang, X., Li, F., Xu, J., et al. (2015). Structural brain abnormalities correlate with clinical features in patients with drug-naïve OCD: a DARTEL-enhanced voxel-based morphometry study. Behav. Brain Res. 294, 72–80. doi: 10.1016/j.bbr.2015.07.061
Varigonda, A. L., Jakubovski, E., and Bloch, M. H. (2016). Systematic review and meta-analysis: early treatment responses of selective serotonin reuptake inhibitors and clomipramine in pediatric obsessive-compulsive disorder. J. Am. Acad. Child Adolesc. Psychiatry 55, 851–859.e2. doi: 10.1016/j.jaac.2016.07.768
Weiner, K. S., and Zilles, K. (2016). The anatomical and functional specialization of the fusiform gyrus. Neuropsychologia 83, 48–62. doi: 10.1016/j.neuropsychologia.2015.06.033
Wynn, S. C., Driessen, J. M. A., Glennon, J. C., Brazil, I. A., and Schutter, D. (2019). Cerebellar transcranial direct current stimulation improves reactive response inhibition in healthy volunteers. Cerebellum 18, 983–988. doi: 10.1007/s12311-019-01047-z
Xia, J., Fan, J., Du, H., Liu, W., Li, S., Zhu, J., et al. (2019). Abnormal spontaneous neural activity in the medial prefrontal cortex and right superior temporal gyrus correlates with anhedonia severity in obsessive-compulsive disorder. J. Affect. Disord. 259, 47–55. doi: 10.1016/j.jad.2019.08.019
Xie, Y. (1998). Reliability and validity of the simplified coping style questionnaire. Chin. J. Clin. Psychol. 6, 114–115. doi: 10.1177/0961203318815595
Yan, H., Shan, X., Li, H., Liu, F., and Guo, W. (2022). Abnormal spontaneous neural activity as a potential predictor of early treatment response in patients with obsessive-compulsive disorder. J. Affect. Disord. 309, 27–36. doi: 10.1016/j.jad.2022.04.125
Yang, T., Cheng, Y., Li, H., Jiang, H., Luo, C., Shan, B., et al. (2010). Abnormal regional homogeneity of drug-naïve obsessive-compulsive patients. Neuroreport 21, 786–790. doi: 10.1097/WNR.0b013e32833cadf0
Yang, X., Luo, J., Zhong, Z., Yang, X., Yao, S., Wang, P., et al. (2019). Abnormal regional homogeneity in patients with obsessive-compulsive disorder and their unaffected siblings: a resting-state fMRI study. Front. Psychiatry 10:452. doi: 10.3389/fpsyt.2019.00452
Yang, X. Y., Sun, J., Luo, J., Zhong, Z. X., Li, P., Yao, S. M., et al. (2015). Regional homogeneity of spontaneous brain activity in adult patients with obsessive-compulsive disorder before and after cognitive behavioural therapy. J. Affect. Disord. 188, 243–251. doi: 10.1016/j.jad.2015.07.048
Yeterian, E. H., and Pandya, D. N. (1998). Corticostriatal connections of the superior temporal region in rhesus monkeys. J. Comp. Neurol. 399, 384–402.
Yonelinas, A. P. (2013). The hippocampus supports high-resolution binding in the service of perception, working memory and long-term memory. Behav. Brain Res. 254, 34–44. doi: 10.1016/j.bbr.2013.05.030
Zang, Y., Jiang, T., Lu, Y., He, Y., and Tian, L. (2004). Regional homogeneity approach to fMRI data analysis. Neuroimage 22, 394–400. doi: 10.1016/j.neuroimage.2003.12.030
Zhang, C. C., Gong, H., Zhang, Y., Jin, H., Yang, Y., Li, B., et al. (2019). Development and psychometric evaluation of the Mandarin Chinese version of the Yale-Brown obsessive-compulsive scale - second edition. Braz. J. Psychiatry 41, 494–498. doi: 10.1590/1516-4446-2018-0126
Zhao, H. Z., Wang, C. H., Gao, Z. Z., Ma, J. D., Huang, P., Li, H. F., et al. (2017). Effectiveness of cognitive-coping therapy and alteration of resting-state brain function in obsessive-compulsive disorder. J. Affect. Disord. 208, 184–190. doi: 10.1016/j.jad.2016.10.015
Keywords: obsessive–compulsive disorder, regional homogeneity, hippocampus, parahippocampus, support vector machine
Citation: Yan H, Shan X, Li H, Liu F and Guo W (2022) Abnormal spontaneous neural activity in hippocampal–cortical system of patients with obsessive–compulsive disorder and its potential for diagnosis and prediction of early treatment response. Front. Cell. Neurosci. 16:906534. doi: 10.3389/fncel.2022.906534
Received: 28 March 2022; Accepted: 30 June 2022;
Published: 15 July 2022.
Edited by:
Hamid Reza Marateb, Universitat Politècnica de Catalunya, SpainReviewed by:
Marjan Mansourian, Universitat Politècnica de Catalunya, SpainChristian Kaufmann, Humboldt University of Berlin, Germany
Copyright © 2022 Yan, Shan, Li, Liu and Guo. This is an open-access article distributed under the terms of the Creative Commons Attribution License (CC BY). The use, distribution or reproduction in other forums is permitted, provided the original author(s) and the copyright owner(s) are credited and that the original publication in this journal is cited, in accordance with accepted academic practice. No use, distribution or reproduction is permitted which does not comply with these terms.
*Correspondence: Wenbin Guo, Z3Vvd2VuYmluNzZAY3N1LmVkdS5jbg==