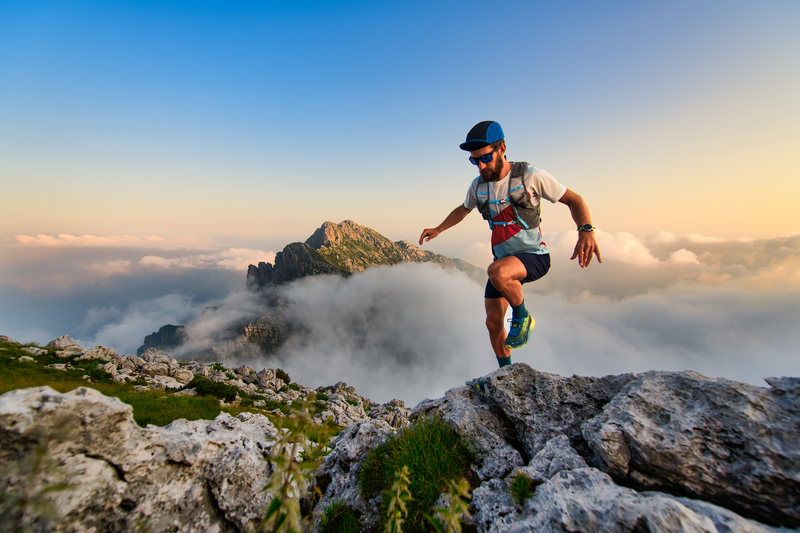
94% of researchers rate our articles as excellent or good
Learn more about the work of our research integrity team to safeguard the quality of each article we publish.
Find out more
EDITORIAL article
Front. Neurorobot. , 23 October 2023
Volume 17 - 2023 | https://doi.org/10.3389/fnbot.2023.1306042
This article is part of the Research Topic Engineering Applications of Neurocomputing Volume II View all 6 articles
Editorial on the Research Topic
Engineering applications of neurocomputing, volume II
Inspired by how the human brain works, neurocomputing algorithms, including deep learning, reinforcement learning, and neurodynamic optimization, have achieved tremendous success in various applications across many domains, e.g., visual object tracking, speech recognition, human-level control, text understanding, and real-time optimization.
Various types of intelligent equipment and hardware devices are developed to implement the neurocomputing models for engineering systems. Deep learning has been employed for industrial robotic applications, including stereo reconstruction, object pose recognition, and product quality check. With the advent of Internet of Things and edge computing devices, predictive maintenance of engineering equipment is gaining popularity using deep reinforcement learning. Embedded convolutional neural networks are widely utilized for autonomous vehicle control. The success of applying neurocomputing approaches and related hardware implementations in different engineering domains, such as intelligent manufacturing, energy internet and smart healthcare, has proved the potential of employing neurocomputing for solving real problems in various engineering fields.
Nowadays, advances in sensor and data storage technologies have enabled cumulation of a large amount of data from engineering systems. Driven by big data generated from engineering systems, neurocomputing and its hardware implementation will continually transform engineering systems to more intelligent forms.
This Research Topic aims to provide a forum for researchers to present the latest research on applications of neurocomputing algorithms and neurocomputing-based hardware in engineering systems. The Volume II of this Research Topic collected another 5 high quality papers reporting the latest applications of neurocomputing in wind energy, nuclear power, and autonomous driving.
The paper titled “Wind speed interval prediction based on the hybrid ensemble model with biased convex cost function” by Long et al. proposed a combination interval prediction based hybrid ensemble model for short-term wind speed prediction. The combination interval prediction model employed the extreme learning machine as the predictor with a biased convex cost function. With the benefit of the biased convex cost function and ensemble technique, the high computational efficiency and stable performance of the proposed prediction model was guaranteed simultaneously. The superiority of the proposed interval prediction model was verified based on comprehensive experiments.
The paper titled “Neural network aided flexible joint optimization with design of experiment method for nuclear power plant inspection robot” by Wang G. et al. developed a neural network aided flexible joint structure optimization method with the Design of Experiment method for the nuclear power plant inspection robot. With this method, the joint's dual-spiral flexible coupler was optimized regarding the minimum mean square error of the stiffness. The optimal flexible coupler was demonstrated and tested via experiments.
The paper titled “Soft-masks guided faster region-based convolutional neural network for domain adaptation in wind turbine detection” by Xu et al. reported a synthetic generation method trying to simulate the real wind turbines from the aspects of style and content. Meanwhile, a novel soft-masks guided faster region-based convolutional neural network was developed for domain adaptation in wind turbine detection. The effectiveness of the proposed approach was evaluated by comparing with other representative models on the real dataset.
The paper titled “Real-time depth completion based on LiDAR-stereo for autonomous driving” by Wei et al. introduced a real-time LiDAR-stereo depth completion network without 3D convolution to fuse point clouds and binocular images using injection guidance. At the same time, a kernel-connected spatial propagation network was utilized to refine the depth. The output of dense 3D information was more accurate for autonomous driving. Experimental results on the KITTI dataset showed that the proposed method used real-time techniques effectively.
The paper titled “A semantic segmentation scheme for night driving improved by irregular convolution” by Xuantao et al. investigated a fuzzy information complementation strategy based on generative models and a network that fuses different intermediate layer outputs to complement spatial semantics. The experimental results demonstrated that the proposed method can effectively cope with various problems faced by night driving and enhance the model's perception.
This topic reported various applications of neurocomputing in the engineering domain. These neurocomputing-based approaches demonstrate great potential in solving real problems. With the innovative improvements from the algorithmic side, more successful applications of neurocomputing will appear in future engineering systems.
LW: Writing—original draft. ZS: Writing—review and editing. ZZ: Writing—review and editing. CH: Writing—review and editing.
The author(s) declare financial support was received for the research, authorship, and/or publication of this article. This work was supported in part by the National Natural Science Foundation of China under Grant 62202044, in part by the Guangdong Basic and Applied Basic Research Foundation under Grants 2020A1515110431 and 2022A1515240044, in part by the Fundamental Research Funds for the Central Universities and the Youth Teacher International Exchange and Growth Program under Grant QNXM20220040, and in part by the Interdisciplinary Research Project for Young Teachers of USTB (Fundamental Research Funds for the Central Universities) under Grant FRF-IDRY-21-003.
The authors declare that the research was conducted in the absence of any commercial or financial relationships that could be construed as a potential conflict of interest.
All claims expressed in this article are solely those of the authors and do not necessarily represent those of their affiliated organizations, or those of the publisher, the editors and the reviewers. Any product that may be evaluated in this article, or claim that may be made by its manufacturer, is not guaranteed or endorsed by the publisher.
Keywords: neurocomputing, engineering systems, neural networks, manufacturing, healthcare
Citation: Wang L, Song Z, Zhang Z and Huang C (2023) Editorial: Engineering applications of neurocomputing, volume II. Front. Neurorobot. 17:1306042. doi: 10.3389/fnbot.2023.1306042
Received: 03 October 2023; Accepted: 10 October 2023;
Published: 23 October 2023.
Edited and reviewed by: Tinghui Ouyang, National Institute of Advanced Industrial Science and Technology (AIST), Japan
Copyright © 2023 Wang, Song, Zhang and Huang. This is an open-access article distributed under the terms of the Creative Commons Attribution License (CC BY). The use, distribution or reproduction in other forums is permitted, provided the original author(s) and the copyright owner(s) are credited and that the original publication in this journal is cited, in accordance with accepted academic practice. No use, distribution or reproduction is permitted which does not comply with these terms.
*Correspondence: Chao Huang, Y2hhby5odWFuZ0BteS5jaXR5dS5lZHUuaGs=
Disclaimer: All claims expressed in this article are solely those of the authors and do not necessarily represent those of their affiliated organizations, or those of the publisher, the editors and the reviewers. Any product that may be evaluated in this article or claim that may be made by its manufacturer is not guaranteed or endorsed by the publisher.
Research integrity at Frontiers
Learn more about the work of our research integrity team to safeguard the quality of each article we publish.