- 1IUSS Cognitive Neuroscience (ICoN) Center, Scuola Universitaria Superiore IUSS, Pavia, Italy
- 2Istituti Clinici Scientifici Maugeri IRCCS, Cognitive Neuroscience Laboratory of Pavia Institute, Pavia, Italy
Introduction: Previous neuroimaging evidence highlighted the role of the insular and dorsal anterior cingulate cortex (dACC) in conflict monitoring and decision-making, thus supporting the translational implications of targeting these regions in neuro-stimulation treatments for clinical purposes. Recent advancements of targeting and modeling procedures for high-definition tDCS (HD-tDCS) provided methodological support for the stimulation of otherwise challenging targets, and a previous study confirmed that cathodal HD-tDCS of the dACC modulates executive control and decision-making metrics in healthy individuals. On the other hand, evidence on the effect of stimulating the insula is still needed.
Methods: We used a modeling/targeting procedure to investigate the effect of stimulating the posterior insula on Flanker and gambling tasks assessing, respectively, executive control and both loss and risk aversion in decision-making. HD-tDCS was applied through 6 small electrodes delivering anodal, cathodal or sham stimulation for 20 min in a within-subject offline design with three separate sessions.
Results: Bayesian statistical analyses on Flanker conflict effect, as well as loss and risk aversion, provided moderate evidence for the null model (i.e., absence of HD-tDCS modulation).
Discussion: These findings suggest that further research on the effect of HD-tDCS on different regions is required to define reliable targets for clinical applications. While modeling and targeting procedures for neuromodulation in clinical research could lead to innovative protocols for stand-alone treatment, or possibly in combination with cognitive training, assessing the effectiveness of insula stimulation might require sensitive metrics other than those investigated here.
Introduction
Previous neuroimaging evidence has consistently shown the role of the insular cortex in conflict monitoring and decision-making (Canessa et al., 2013, 2017; Markett et al., 2016). Several studies have reported the involvement of this region in a wide array of functions, placing it at the interface between interoception, emotion and cognition (Chang et al., 2013; Uddin et al., 2017; Molnar-Szakacs and Uddin, 2022). In particular, insular activity related to interoceptive representation, performance monitoring, as well as awareness of emotional states and body movement, has been interpreted in terms of bodily physiological signals guiding decision-making based on the outcome of behavioral learning processes (Craig, 2009). This hypothesis was supported by studies investigating the neural bases of decision-making under risk and/or uncertainty, showing increased activation of the right insular cortex when making riskier compared to safer choices (Paulus et al., 2003) and higher anticipatory insular response preceding riskless choices (Kuhnen and Knutson, 2005).
Based on anatomical, functional and connectivity features, the insula has been parceled in multiple subregions, and particularly in posterior and anterior sections divided by the central insular sulcus (Wysiadecki et al., 2018). The anterior section, highly connected with frontal and limbic areas, has been mainly related to emotional and cognitive functions, whereas the posterior insula (PI) plays a crucial role in interoceptive processing and behavioral regulation via connections to posterior-temporal and parietal areas, as well as sensorimotor cortices (Cloutman et al., 2012; Centanni et al., 2021). These sectors appear to underpin a posterior-to-anterior flow of neural signals, whereby the progressive integration of sensory information into mechanisms of “salience” detection underpins the modulation of cognitive control and goal-directed behavior by sensory-interoceptive and affective stimuli (Craig, 2009; Centanni et al., 2021). Accordingly, neuroimaging studies have shown the PI role in representing homeostatic states related to the experience of risk, in turn shaping upcoming decisions (Xue et al., 2010). One such experience concerns the mental anticipation and evaluation of choices entailing both positive and negative prospective outcomes, typically associated with the overweighting of potential losses compared with gains, and thus with the individual’s tendency to prefer avoiding losses to acquiring equivalent gains (i.e., loss aversion; Kahneman and Tversky, 1979). Previous neuroimaging evidence shows that individual differences in the extent of loss aversion reflect the strength of activation of multiple brain structures, including the striatal and dorsal anterior cingulate (dACC) sectors of the meso-cortico-limbic pathway (Tom et al., 2007), and the right PI—extending into the supramarginal gyrus—both when making real choices (Canessa et al., 2013) and at rest (Canessa et al., 2017).
Consistent with the role of the insula in loss aversion, clinical evidence shows that operculo-insular resection for drug-resistant epileptic seizures impairs patients’ sensitivity to expected value when facing a potential loss during decision-making under risk (Von Siebenthal et al., 2017). Moreover, insular damage has been associated to a reduction of the typical gambling-related cognitive distortions in tasks simulating real-life gambling (Clark et al., 2014): compared both to controls and patients with lesions in the amygdala or ventromedial prefrontal cortex (vmPFC), those with lesions confined to insula did not show the so-called near-miss effects (i.e., higher motivation to play a gamble after non-wins close to the jackpot) or gambler’s fallacy (i.e., the belief that non-recent outcomes are more likely to happen).
By showing a role of the insula in modulating adaptive behavioral learning via sensory-affective signals, these data suggest its possible involvement in conditions characterized by poor adaptation to changing environments. This view is supported by growing evidence of structural and functional abnormalities, in this region, in patients with major depressive disorder (Herwig et al., 2010; Surguladze et al., 2010; Takahashi et al., 2010; Stratmann et al., 2014), bipolar disorder (Ellison-Wright and Bullmore, 2010; Hulvershorn et al., 2012; Wise et al., 2017), anxiety disorders (Stein et al., 2007; Terasawa et al., 2013), schizophrenia (Wylie and Tregellas, 2010), psychopathy for abnormal socio-emotional processing (Blair, 2013), anorexia nervosa (Kaye et al., 2009), addiction (Ibrahim et al., 2019), as well as different deficits associated with neurological disorders (Löffler et al., 2016; Namkung et al., 2017). Overall, this evidence highlights the importance of assessing insular responsiveness to tasks tapping evaluation and control as a potential translational biomarkers (or “endophenotypes”; Sharp et al., 2012) of adaptive behavioral learning, that might also represent the target of innovative neuromodulation protocols with therapeutical purposes (Downar et al., 2016).
Notably, several studies indeed showed strong functional connectivity between the PI and the dACC (Taylor et al., 2009; Ghaziri et al., 2017), i.e., a key node of the “executive” network (Shenhav et al., 2016), which highlights a possible neural basis of the insula role in cognitive control via salience processing (Dosenbach et al., 2007; Seeley et al., 2007). This notion highlights the potential translational implications of investigating the role of insula and dACC, since both regions map strikingly well with a common core of brain areas involved in different psychiatric conditions (Goodkind et al., 2015; Downar et al., 2016), that might be targeted in innovative treatment protocols. Since this aim can be pursued with non-invasive neurostimulation and neuromodulation techniques, studies investigating their feasibility are required to pave the way to possible clinical applications (Downar et al., 2016). Transcranial direct current stimulation (tDCS) is a relatively inexpensive, portable and well tolerated candidate for this purpose (Brunoni et al., 2012), especially with high-definition montages (HD-tDCS), that are expected to improve the intensity and/or focality of stimulation compared with the “conventional” bipolar approach (Edwards et al., 2013; Huang et al., 2019). It is worth noting that optimization methods for HD-tDCS highlighted a non-trivial trade-off between focality and variability of the effects with different montages (Dmochowski et al., 2011; Mikkonen et al., 2020; Lee et al., 2021). The available evidence from computational models suggests that 4 × 1 ring montages (i.e., one small stimulating electrode surrounded by a ring of four small reference electrodes) grant higher focality, but at the cost of larger inter-individual variability in strength and distribution of electric fields (Mikkonen et al., 2020; Van Hoornweder et al., 2022). On the other hand, model-based HD-tDCS montages produce larger current dispersion, which is however compensated by higher stimulation intensity at the target site (Lee et al., 2021), resulting in an overall optimal trade-off between focality, individual variability, and intensity (Mikkonen et al., 2020).
By using a model-based approach, we previously reported evidence of the significant effect of dACC stimulation, via HD-tDCS, on cognitive control and loss aversion in decision-making under risk (Mattavelli et al., 2022). It is still unknown, instead, whether such modulation might be also elicited by HD-tDCS of the insula. Since this area is a potential key target for the treatment of different neuropsychiatric conditions (Downar et al., 2016), this is a critical gap that should be filled to investigate its feasibility and its effects.
On this basis, we assessed the effects of anodal and cathodal HD-tDCS over the right PI in modulating executive control on the Flanker task, and both loss and risk aversion in well-established tasks of decision-making under risk (Kahneman and Tversky, 1979). We replicated the experimental paradigms and methodological procedures used in our previous study (Mattavelli et al., 2022), in which decreased “Flanker” conflict effect and increased loss/risk aversion suggested an improvement of conflict monitoring—involving both visual-attentional skills and behavioral control—after cathodal HD-tDCS of the dACC. As mentioned above, the dACC and PI are highly connected, and the activity of both regions has been reported to track individual differences in response inhibition (Wager et al., 2005; Fedota et al., 2016) and loss aversion (Canessa et al., 2013). We therefore predicted that active HD-tDCS of the right PI, compared to sham stimulation, might confirm the modulation of behavioral performance in the Flanker and gambling tasks observed in our previous study.
Materials and methods
Participants
Twenty-five healthy right-handed participants (15 females) aged 20–26 years [mean 22.6; standard deviation (SD): 1.87] took part in the study. Three participants were excluded due to inconsistencies in their performance, leading to a total of 22 participants. In detail, the model for estimating loss aversion did not converge for one participant, while two participants showed loss aversion lambda (λ) > 10, suggestive of the tendency to reject all gambles (see the method below). We replicated the experimental design, power analysis and sample size used in our previous study (Mattavelli et al., 2022). Namely, we planned to perform a repeated measures ANOVA on the Flanker conflict effect, by entering in G*Power (Faul et al., 2007) the effect size computed from a study with a similar design (Weiss and Lavidor, 2012; partial η2 = 0.17). This procedure resulted in an estimated sample size of 19 participants assessed in three separate sessions (α = 0.05, 1 − β = 0.9). Notably, the observed effect size from our first experiment was in line with, and even larger than, this previous evidence (partial η2 = 0.197), indicating that a sample of 22 participants should enable finding modulatory effects.
Participants’ eligibility was assessed with a questionnaire considering the following exclusion criteria for brain stimulation studies (Keel et al., 2001): (1) diagnosis of, or familiarity with, epilepsy; (2) susceptibility to, or history of, migraine; (3) history of neurological or psychiatric disorders; (4) history of brain surgery, tumor or intracranial metal implantation (such as hearing aids, pacemakers or metal plates near the face); (5) current use of psychiatric drugs or psychoactive medications; (6) presence of pacemaker or other implanted devices; (7) diagnosis of specific learning disabilities (e.g., dyslexia); (8) current pregnancy. Participants provided their written informed consent to the experimental procedure, previously approved by ICS Maugeri Ethics Committee, that was based on the latest version of the declaration of Helsinki and on tDCS safety guidelines (Poreisz et al., 2007).
Experimental procedure
The experiment was based on a single-blind cross-over design. Participants received three stimulation sessions with either active anodal, active cathodal or sham HD-tDCS, separated by a wash-out period of at least 3 days. Although findings regarding the greater effectiveness of offline vs. online stimulation are still controversial (Martin et al., 2014), the former appears to result in greater modulation of task performance (Friehs and Frings, 2019). Based on this evidence, and in keeping with our previous study (Mattavelli et al., 2022), we used an offline approach to assess the neurostimulation effect of HD-tDCS on the right PI. The order of both stimulation conditions (anodal, cathodal, sham) and experimental tasks (Flanker, Loss aversion, Risk aversion) was randomized to control for potential effects of task order or participants’ fatigue.
During the training phase, participants were informed that their performance on the three tasks would led to increase or decrease an initial monetary endowment. Both stimulation and tasks were then performed in a shielded silent cabin, where participants were seated on a comfortable chair and were asked to remain still and relaxed in front of a high-frequency (148 Hz) LCD screen, placed at a 65 cm viewing distance. Each stimulation session lasted 20 min, during which participants were presented a series of short videoclips previously rated as emotionally neutral (Samson et al., 2016). Since the duration of tDCS aftereffects is known to at least equate the duration of stimulation (Batsikadze et al., 2013; Renzi et al., 2015; To et al., 2018; Mattavelli et al., 2019, 2022), the three tasks (Flanker, Loss Aversion, and Risk Aversion) were started immediately after stimulation, so as to be completed within 20 min. The experimental tasks were presented via Presentation software,1 while responses were recorded throughout a keyboard. At the end of each session, participants were asked to fill a questionnaire addressing potential HD-tDCS cutaneous or adverse effects (Fertonani et al., 2015). Finally, after the last session, they were also asked to report which sessions they believed were real or placebo, and to express the degree of confidence of their judgment on a scale from 1 to 10 (1 = not certain at all; 10 = completely certain).
High-definition transcranial direct current stimulation
The ROAST toolbox (Huang et al., 2016) was used to identify the optimal montage for maximum intensity of stimulation over the insula. Specifically, the montage was set to target the right PI coordinates where activity was previously reported to track individual difference in loss aversion (Canessa et al., 2013, 2017). The montage was optimized by modeling 6 mm radius circular electrodes (DaSilva et al., 2015) together with the indexes of conductivity assigned to different tissues based on a high resolution T1-weighted MRI image (Huang et al., 2016). The resulting optimal solution consisted of 3 anodes and 3 cathodes, placed on CP4-C6-CP6 and FT8-F10-FT10, respectively. This montage was then modeled to check for the current flow location and intensity when placing 9.5 mm radius electrodes and conductive gel (Figure 1). Each anode and cathode electrode acts as a source and sink, for a total delivered current of 3 mA (i.e., 1 mA current intensity each; current density of 0.35 mA/cm2). The electrodes were placed into saline-soaked sponges of the same shape and size, and placed on the participants’ scalp with the help of an EEG brain cap that was used to correctly identify the electrode locations based on the 10–10 system. The HD montage was implemented through 3 triggered battery-driven neurostimulation devices (Brainstim, EMS Italy).
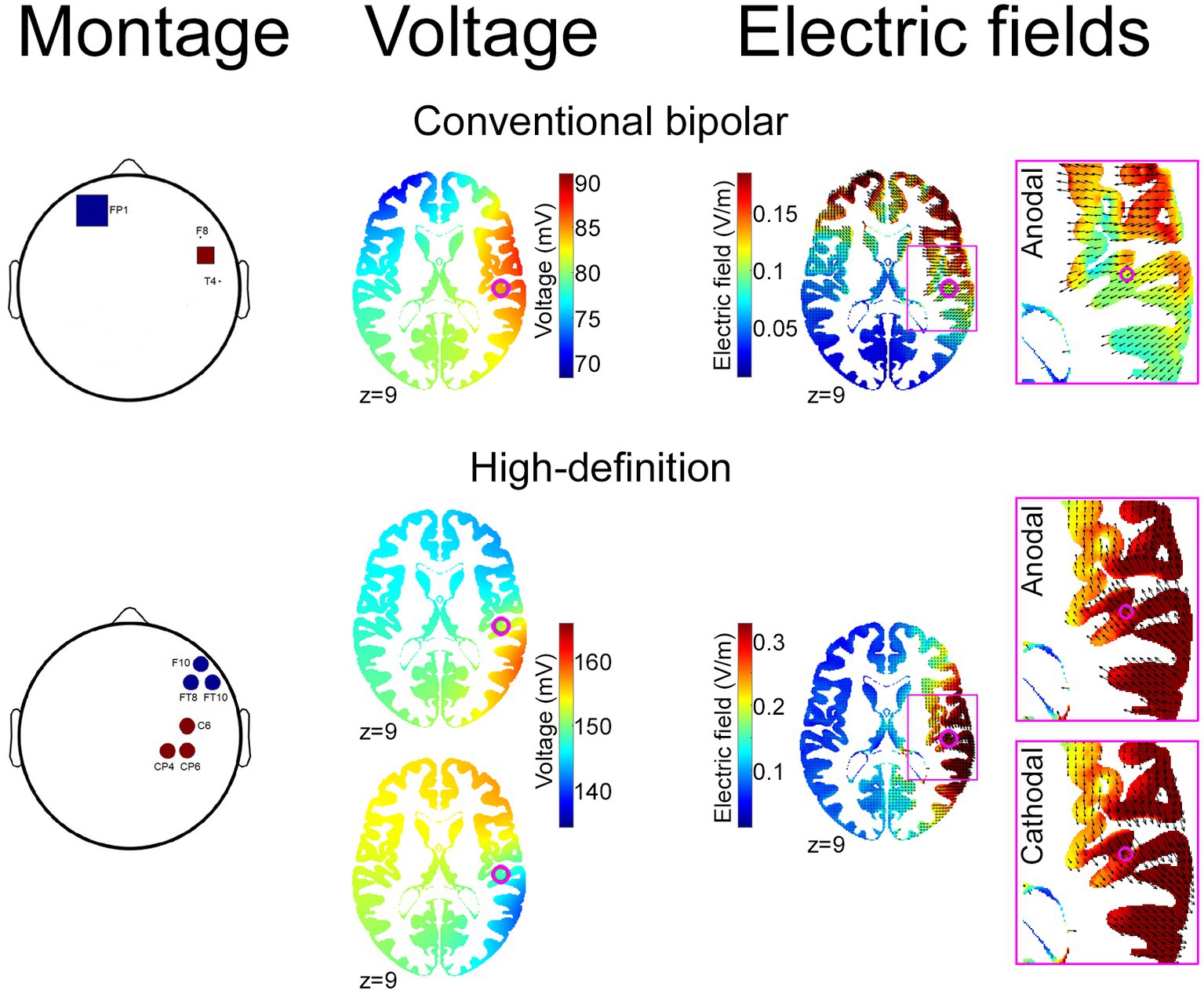
Figure 1. Conventional bipolar, and optimized HD, tDCS montages. The figure depicts tDCS montages aimed to stimulate the right posterior insular cortex using a previously reported conventional bipolar approach (Sagliano et al., 2019; top) and an optimized electrode configuration generated by the ROAST toolbox (Huang et al., 2016; bottom) to provide maximum stimulation intensity in the stereotactic coordinates that have been previously associated with neural loss aversion [xyz: 48 −21 9 (pink dot); Canessa et al., 2013]. For each configuration, the figure depicts the montage [number, shape and size of anode (red) and cathode (blue) electrodes; left], alongside the resulting voltage (middle) and electric field (right) distribution. For HD-tDCS, the right-most panels show the opposite direction of current flows—maximally involving the right posterior insula—for anodal and cathodal montages.
During anodal sessions, stimulation was performed through a constant current of 1 mA for each anode electrode, that was maintained for 20 min, gradually ramping up in intensity for the first 15 s and slowly decreasing down to 0 mA for the last 15 s. The same holds for the cathodal stimulation, but with reversed polarity. These parameters are in line with safety guidelines for transcranial electric stimulation (tDCS) in humans (Nitsche et al., 2003a, 2004; Gandiga et al., 2006). For the sham sessions, intensity gradually ramped up at the beginning of the stimulation, was maintained for the first 30 s, and then slowly decreased to 0 mA; it was then increased again for the final 30 s of the stimulation. This protocol was adopted to ensure participants’ blinding to the stimulation type (Gandiga et al., 2006; Poreisz et al., 2007; Palm et al., 2013), without inducing an after-effect (Nitsche et al., 2003b; Woods et al., 2016).
Tasks and stimuli
Flanker task
Participants were asked to indicate the left or right direction of a central horizontal arrow by pressing the corresponding key on a keyboard as quickly and accurately as possible. Three conditions were presented (32 trials each, for a total of 96 trials) in which arrows could either appear surrounded by (1) horizontal lines with no symbolic value—neutral condition; (2) horizontal arrows with the same direction as the target arrow—congruent condition; (3) horizontal arrows with opposite direction to the target—incongruent condition (Figure 2). To assess executive control, Fan et al.’s (2002) “conflict effect” measure was adopted, by subtracting the mean RT of congruent conditions from the mean RT of incongruent condition. The resulting difference is a measure of participants’ susceptibility to the conflict effect, typically reflecting in slower RTs when responding to incongruent than congruent flankers (Fan et al., 2002). Arrows could either appear above or below the fixation point, with the target arrow being preceded by several possible visual cues: no cue, spatial cue, central cue, double cue. While spatial cues could indicate the position of the target arrow (above or below the fixation point), central and double cues were ambiguous. While this manipulation would enable to calculate alerting and orienting effects (Fan et al., 2002), the limited number of trials—due to time constraints related to the duration of the stimulation—only allowed to focus on the conflict effect. Each trial included a fixation point (400 ms), followed or not by the visual cue (100 ms), another fixation point (400 ms), and then the target stimuli (1,700 ms). Trials were presented in random order and were separated by a blank screen of variable duration (either 1,450, 1,500, or 1,550 ms). The eye-monitor distance and the size of the stimuli were computed to obtain a visual angle of 0.588 for each arrow, and 0.068 for the distance between successive arrows (Fan et al., 2002, 2005; Mattavelli et al., 2022).
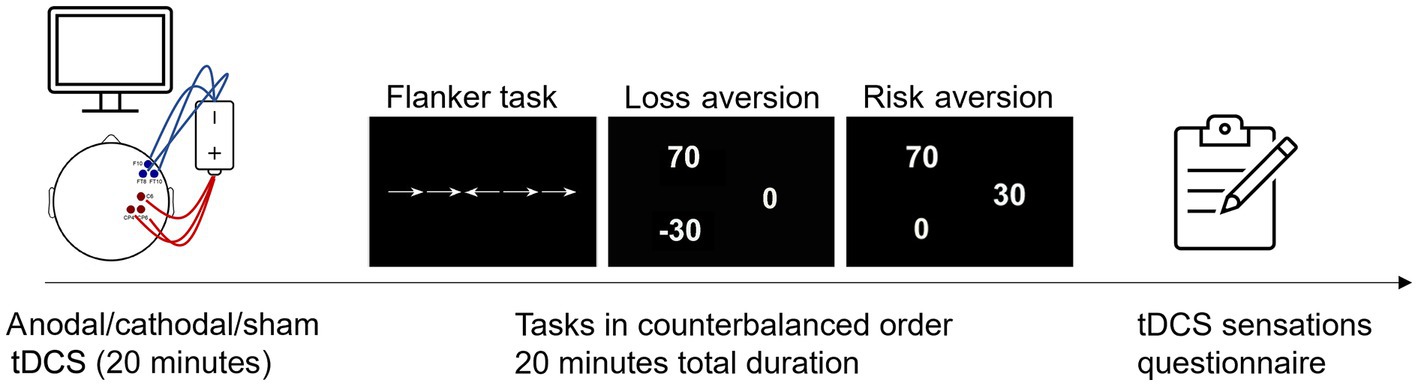
Figure 2. The figure depicts one representative tDCS session, with examples of stimuli from the three experimental tasks (presented in counterbalanced order across participants), i.e., from left to right: an incongruent trial of the Flanker task, and gambling tasks requiring to choose between (a) a certain outcome and a risky mixed-gamble resulting in two equally probable (p = 50%) gain-loss outcomes (loss aversion task), or (b) a gamble with equally probable variable positive or 0 outcomes and a certain but smaller gain (risk aversion task).
Loss and risk aversion tasks
To assess individual differences in loss and risk aversion, and their possible modulation by HD-tDCS, participants were asked to perform two gambling tasks that required evaluating real prospective monetary gains and losses. In each trial of both tasks, participants had to choose between a risky gamble resulting in one of two equally probable (p = 50%) outcomes, and a certain alternative. The Loss Aversion task entailed 50 gain-loss trials during which participants were asked to choose between (a) a certain 0 outcome (i.e., the status quo) and (b) a gamble that might result—with equal probabilities—in either a gain or a loss. The Risk Aversion task included 50 gain-only trials requiring to choose between (a) a gamble with equal probabilities of leading to a gain or 0, and (b) a certain but smaller gain (Figure 2). In both tasks, gambles were presented for 4,500 ms, with the inter-trial-interval ranging between 1,450 and 1,550 ms (Mattavelli et al., 2022). Unbeknownst to participants, the gain-loss ratio in the Loss Aversion tasks, and the gain-certain outcome ratio in the Risk Aversion task, were trial-wise adjusted based on participants’ previous responses. Such adjustment was based on a staircase algorithm (Fleming and Dolan, 2010; Takahashi et al., 2012; Takeuchi et al., 2016; Chen et al., 2020; Mattavelli et al., 2022), through which the initial ratio, set at 2.5, either increased or decreased when the participant rejected or accepted a gamble, respectively. This adjustment had a step-size of 0.5/n, where “n” is the number of trials after the last reversal between accepting or rejecting the gamble. Stimuli were sampled from a symmetric gain-loss matrix ranging between 1 and 100, in steps of 1 (Mattavelli et al., 2022). This procedure was aimed at progressively reducing the range of the subjective indifference point, i.e., the gain-loss (or risky-certain outcome) ratio corresponding to the maximum decisional conflict, associated with a 50% probability of accepting/rejecting the gamble (Mattavelli et al., 2022). To avoid possible learning effects and focus on “decision utility” (i.e., “pure” anticipation without the expectation of outcome delivery; Canessa et al., 2013, 2017; Tom et al., 2007), gambles were not solved immediately. Instead, participants were informed that one among the accepted gambles would be randomly extracted and played by the computer, leading to increase or decrease the monetary reward in case of win or loss, respectively.
The individual degree of loss and risk aversion were estimated, for each subject and stimulation session, by simultaneously fitting both gain-loss and gain-only trials to the following Prospect-theory-inspired model (Sokol-Hessner et al., 2015):
where G is the gain (G > 0), L is the loss (L < 0 for gain-loss gambles and L = 0 for gain-only gambles), B the guaranteed gain (B = 0 for gain-loss gambles and B > 0 for gain-only gambles), pG = 0.5 is the probability of a gain and pL = 1 − pG = 0.5 is the probability of a loss. The free parameters of the model are: (a) the loss aversion lambda (λ), i.e., the multiplicative weight associated with anticipated losses compared with gains; (b) the risk attitude rho (ρ), i.e., the curvature of the value function u(x) = x^ρ that embodies the diminishing sensitivity to increasing outcome; and (c) the choice consistency or “softmax temperature” (μ), i.e., a measure of noisiness vs. systematicity in choices. Since λ is positively skewed, its natural logarithm—ln(λ)—was modeled in the statistical analyses. Exclusion criteria were (a) a lack of convergence after 50,000 iterations, indicating the lack of model fitting and thus representing inconsistent choices; (b) λ >10, suggestive of the tendency to reject all gambles (Arioli et al., 2023).
Results
tDCS tolerability and blinding
Responses to the questionnaire on tDCS-related sensations were analyzed as in our previous study (Mattavelli et al., 2022). Supporting the effectiveness of the blinding procedure, there was no difference across anodal, cathodal or sham sessions concerning (a) the proportion of participants reporting that they received a real stimulation [86%, 86%, and 73%, respectively; χ2(2) = 1.83, p = 0.4], and (b) the confidence in this judgment [F(2, 42) = 2.89, p = 0.07]. Subjective sensations were generally low. Only the burning sensation was significantly different across condition, with higher ratings in the cathodal compared to the sham session (post hoc Bonferroni corrected p = 0.04), while all the other sensations were not rated differently across sessions (see Supplementary Table S1).
Flanker task
The conflict effect was computed as the differential RTs to incongruent and congruent trials (Fan et al., 2005), after excluding for each participants the trials with RTs greater than 2 standard deviations above the individual mean (Figure 3). To assess the evidence for, or against, the presence of HD-tDCS modulatory effects, a Bayesian statistical approach was adopted using JASP software (Version 0.16; Westfall et al., 1997; Rouder et al., 2012; Morey and Rouder, 2015). Analyses with frequentist statistics were also performed, and reported in Supplementary material. In line with the classification scheme from the JASP guidelines (van Doorn et al., 2021), Bayes Factor (BF) between 1 and 3 is considered weak/anecdotal evidence, BF between 3 and 10 is considered moderate evidence, and BF > 10 is considered strong evidence, in favor of the alternative hypothesis. On the other side, BF between 1 and 1/3 indicates weak/anecdotal evidence, BF between 1/3 and 1/10 indicates moderate evidence, and BF < 1/10 indicates strong evidence, in support of the null hypothesis.
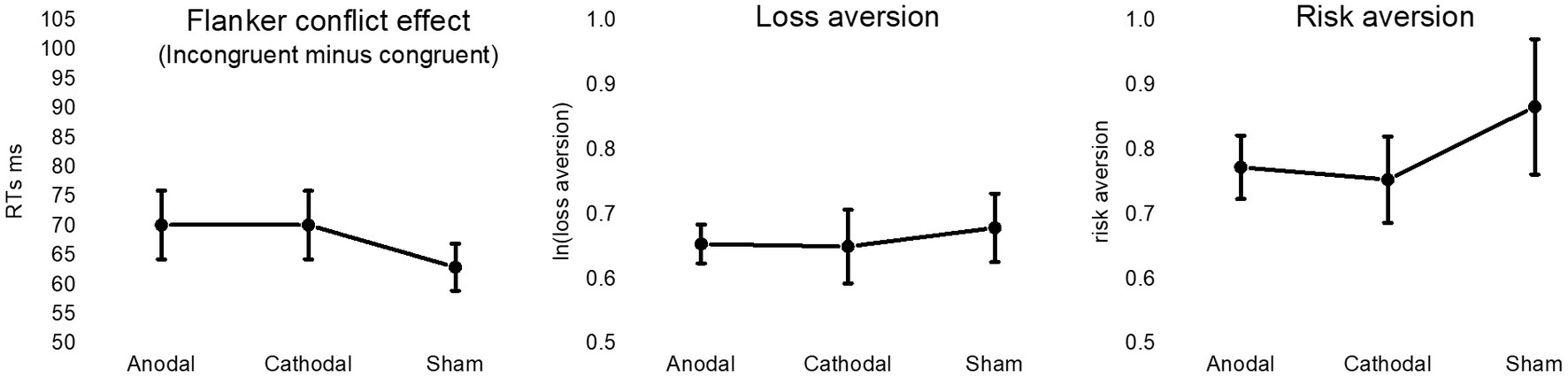
Figure 3. The figure depicts mean values in anodal, cathodal and sham HD-tDCS stimulation session for the conflict effect associated with the differential response time to incongruent and congruent trials of the Flanker task (left panel), the natural logarithm of the degree of loss aversion (central panel), and the degree of risk aversion (right panel). Vertical bars represent standard error of the means.
A Bayesian repeated-measures analysis of variance (ANOVA) with default prior (r scale fixed effects = 0.5; r scale random effects = 1) was used to test the effect of HD-tDCS on executive control, by introducing conflict effect as dependent variable and stimulation condition (anodal, cathodal and sham) as independent variable.
The Mean conflict effect was 70.17 ms (SD = 27.46), 70.17 ms (SD = 27.34), and 62.99 ms (SD = 18.77) in the anodal, cathodal and sham sessions, respectively. Bayesian ANOVA provided moderate support to the null hypothesis (BF10 = 0.28), thus indicating no differential modulation of Flanker performance by stimulation conditions.
For the sake of completeness, we also performed analyses on both (a) RTs to different trial types (congruent, incongruent, neutral), and (b) the effect of the previous trial on congruent/incongruent trials (congruency sequence effects; see Egner, 2007). Their results (reported in Supplementary material) confirmed the absence of HD-tDCS modulation on these outcome variables.
Loss and risk aversion
The mean degree of loss aversion was 1.94 (SD = 0.28), 1.99 (SD = 0.67) and 2.05 (SD = 0.80) following anodal, cathodal and sham stimulation, respectively. Mean risk aversion was 0.77 (SD = 23), 0.75 (SD = 0.31) and 0.86 (SD = 0.49) in the anodal, cathodal and sham sessions, respectively. For both indexes, Bayesian repeated-measures ANOVA with default prior provided moderate support to the null hypothesis, i.e., BF10 = 0.15 for loss aversion and BF10 = 0.21 for risk aversion (Figure 3). The analysis on choice consistency (i.e., the slope of the logistic regression curve) provided anecdotal evidence for the null hypothesis (BF10 = 0.4), being the mean index of uncertainty 1.10 (SD = 1.20), 1.23 (SD = 1.62), and 1.54 (SD = 1.62) in the anodal, cathodal condition and sham sessions, respectively. Results from frequentist statistical analyses are reported in Supplementary material.
Discussion
We aimed to assess the effect of HD-tDCS of the right PI on different facets of cognitive control such as the conflict effect on the Flanker task, and both loss and risk aversion in a decision-making task based on mixed gambles, in healthy individuals.
To the best of our knowledge, this is the first study using a modeling/targeting approach to modulate insular activity with HD-tDCS, that allows to maximize stimulation intensity at the target site, within safety parameters and with good tolerability (Huang et al., 2019). To this purpose, we replicated the experimental procedure adopted in a previous study that proved successful in modulating performance in the same tasks following HD-tDCS of the dACC (Mattavelli et al., 2022). There are both scientific and translational implications of investigating the feasibility and the effects of modulating PI activity: (i) strong connectivity between insula and dACC (Medford and Critchley, 2010; Ghaziri et al., 2017); (ii) previous evidence of an asymmetric anticipatory response of activation for losses and deactivation for gains reflecting behavioral loss aversion (i.e., “neural loss aversion”; Tom et al., 2007; Canessa et al., 2013, 2017); (iii) the translational relevance of targeting the insula, which, alongside the dACC, has been highlighted as a common neural substrate for several neuropsychiatric conditions (Downar et al., 2016).
While cathodal stimulation of the dACC increased behavioral control both in the Flanker and gambling tasks (Mattavelli et al., 2022), no such effect was found, in the present study, when targeting the right PI. Bayesian statistics rather provided moderate evidence in favor of the null model for the Flanker conflict effect and for both loss and risk aversion, along with anecdotal evidence for the null model concerning the “choice consistency” index. Overall, these results are suggestive of no significant modulation of performance in these tasks by HD-tDCS of the right PI.
As mentioned in the Introduction, the modeling/targeting procedure that we applied is expected to maximize the intensity of the stimulation on the target (Huang et al., 2016) and to decrease the individual heterogeneity of tDCS effects (Mikkonen et al., 2020; Lee et al., 2021), although the electric field modeling showed some current dispersion in temporo-parietal regions (Figure 1). This represents a limitation of the model-based HD-tDCS approach, since, in absence of direct measures of the physiological effect of stimulation, we cannot rule out the interpretation that results might reflect a suboptimal modulation of the insula, or counterproductive behavioral effects of electric fields affecting the cortical regions around the target. Our previous findings on the significant effect of dACC stimulation (Mattavelli et al., 2022) however support the effectiveness of targeting regions located in the depth of sulci, and thus suggest that the null present findings might rather indicate that the right PI—despite its strong connectivity with dACC (Ghaziri et al., 2017)—is not an effective target for modulating cognitive control or decision-making. However, this claim needs further investigation to be confirmed, using neurophysiological methods or combined neurostimulation and neuroimaging techniques (e.g., Meinzer et al., 2012; Pisoni et al., 2018; Esmaeilpour et al., 2020; Vergallito et al., 2023), which would allow disentangling the hypotheses that such null findings reflect the lack of impact on these tasks despite an effective insular modulation, or rather the ineffective modulation of the cortical target.
In the case of the Flanker conflict effect, another potential target is the anterior insula, where previous neuroimaging studies identified, both in normal (Trautwein et al., 2016) and pathological (Galandra et al., 2018, 2019, 2021; Crespi et al., 2019; Canessa et al., 2021, 2022) conditions, subregions involved in attentional control and salience processing that might act as a gatekeeper in executive control (see Molnar-Szakacs and Uddin, 2022). Based also on the high connectivity between posterior and anterior insula (Craig, 2009), the latter might be targeted by further studies to investigate a modulation of performance in tasks tapping executive control, including the Flanker conflict effect.
The lack of a significant modulation of participants’ loss and risk aversion is instead more unexpected, based both on our previous evidence of “neural loss aversion” in the right PI (Canessa et al., 2013, 2017), and on other neuroimaging (Xue et al., 2010; Purcell et al., 2021) and lesional (Clark et al., 2014) data showing its involvement in risky decision making. One possible interpretation for this null result concerns the use of a single-session stimulation, that has been questioned by meta-analytic evidence, particularly for cognitive tasks (Horvath et al., 2015; Medina and Cason, 2017). Single sessions are indeed more vulnerable to the unwanted impact of individual differences in susceptibility to stimulation and brain plasticity (Berryhill and Martin, 2018; Vergallito et al., 2022). Since multiple-sessions studies are more robust in this respect (Elmasry et al., 2015; Berryhill, 2017; see Berryhill and Martin, 2018), future studies should investigate whether repeated sessions of HD-tDCS stimulation over the insula allow overcoming individual variability in responsiveness to induce significant modulatory effects. This is not only relevant, but also more common than single-session protocols, in clinical contexts, which might benefit from usual multi-session protocols to improve symptoms in conditions associated with altered insular activity (Downar et al., 2016; Ibrahim et al., 2019). Other types of electric stimulation protocols, such as transcranial alternating current stimulation (tACS) and transcranial random noise stimulation (tRNS), have been reported as effective in modulating cortical activity, but, to the best of our knowledge, there are no studies applying these protocols to target the insula. In particular, theta-tACS seems promising in modulating executive functions (Klink et al., 2020), while there is limited evidence on the modulation of higher cognitive functions by tRNS, that has been mainly tested on motor and perceptual tasks (van der Groen et al., 2022; Brancucci et al., 2023). Thus, both the outcomes of tACS and tRNS on executive control and decision-making, and their effectiveness in targeting the insula, represent open research lines.
On the other hand, the importance of addressing the technical feasibility of insula stimulation is supported by recent multifaceted evidence of its effect on interoceptive accuracy (Sagliano et al., 2019) and compassion motivation when attending to another’s pain (Di Bello et al., 2023). Both these studies used a bipolar montage with electrodes placed over the frontotemporal region, that—as shown by computational modeling (see Figure 1)—induced larger electric fields over the frontal cortex compared to our high-density montage. This finding highlights the non-trivial trade-off between the effects of maximizing the intensity of stimulation via the targeting procedure vs. spreading electric fields in wide portions of the cortex at the cost of further decreased focality when bipolar montages are applied. Other studies targeted the insula with alternative neurostimulation techniques, such as transcranial magnetic stimulation (TMS) with H-coils, that, while generally associated with some discomfort (Levkovitz et al., 2009; Kaster et al., 2018), is considered a safe procedure allowing to reach deep brain regions (Zangen et al., 2005; Roth et al., 2007). Repetitive TMS with H-coil over the insula has been reported to reduce dopamine levels in substantia nigra and striatum (Malik et al., 2018), and to affect functional connectivity with the medial prefrontal cortex (Lee et al., 2020) and the default mode network (Moeller et al., 2022). Another study targeting the insula with H-coils in a sample of patients with anorexia nervosa reported a significant reduction of symptoms related to obsessions and compulsions, as well as depression and anxiety scores (Knyahnytska et al., 2019). In contrast, a study applying a single session in healthy volunteers reported the absence of significant modulations on blink-suppression and risk behavior (Spagnolo et al., 2019). Overall, these findings highlight inconsistent data on the effects of insula stimulation, which should therefore be interpreted with caution because of methodological variability across studies.
In conclusion, we assessed for the first time the modulatory effect of HD-tDCS applied on the right PI based on a modeling/targeting procedure. Apart from the target region, we replicated a methodological procedure that proved effective in decreasing the Flanker conflict effect and increasing both loss and risk aversion after cathodal stimulation of the dACC (Mattavelli et al., 2022). Bayesian statistical analyses provided moderate support for the absence of modulatory effects on executive control and decision-making variables following anodal or cathodal stimulation of the right PI. While confirming the technical feasibility of stimulating this region with a HD-tDCS approach grounded in targeting and modeling procedures, this negative finding suggests that further research is needed to unveil the factors shaping its actual effects and the tasks that are best suited to measure them, to increase the efficacy of translational applications.
Data availability statement
The raw data supporting the conclusions of this article will be made available by the authors, without undue reservation.
Ethics statement
The studies involving humans were approved by Ethics Committee of ICS Maugeri—Pavia Institute. The studies were conducted in accordance with the local legislation and institutional requirements. The participants provided their written informed consent to participate in this study.
Author contributions
GM and NC conceptualized and designed the study. IG and GM collected the data, performed the statistical analysis, and wrote the first draft of the manuscript. IG organized the database. NC wrote sections of the manuscript. All authors contributed to the article and approved the submitted version.
Funding
This research was partially supported by the “Ricerca Corrente” funding scheme of the Italian Ministry of Health.
Acknowledgments
The authors thank Sofia Giovanetti for supporting data collection.
Conflict of interest
The authors declare that the research was conducted in the absence of any commercial or financial relationships that could be construed as a potential conflict of interest.
Publisher’s note
All claims expressed in this article are solely those of the authors and do not necessarily represent those of their affiliated organizations, or those of the publisher, the editors and the reviewers. Any product that may be evaluated in this article, or claim that may be made by its manufacturer, is not guaranteed or endorsed by the publisher.
Supplementary material
The Supplementary material for this article can be found online at: https://www.frontiersin.org/articles/10.3389/fnbeh.2023.1234837/full#supplementary-material
Footnotes
1. ^ www.neurobs.com
References
Arioli, M., Basso, G., Baud-Bovy, G., Mattioni, L., Poggi, P., and Canessa, N. (2023). Neural bases of loss aversion when choosing for oneself versus known or unknown others. Cereb. Cortex 33, 7120–7135. doi: 10.1093/cercor/bhad025
Batsikadze, G., Moliadze, V., Paulus, W., Kuo, M. F., and Nitsche, M. (2013). Partially non-linear stimulation intensity-dependent effects of direct current stimulation on motor cortex excitability in humans. J. Physiol. 591, 1987–2000. doi: 10.1113/jphysiol.2012.249730
Berryhill, M. E. (2017). Longitudinal tDCS: Consistency across working memory training studies. Psychology 4, 71–86. doi: 10.3934/Neuroscience.2017.2.71
Berryhill, M. E., and Martin, D. (2018). Cognitive effects of transcranial direct current stimulation in healthy and clinical populations: an overview. J. ECT 34, e25–e35. doi: 10.1097/YCT.0000000000000534
Blair, R. J. R. (2013). The neurobiology of psychopathic traits in youths. Nat. Rev. Neurosci. 14, 786–799. doi: 10.1038/nrn3577
Brancucci, A., Rivolta, D., Nitsche, M. A., and Manippa, V. (2023). The effects of transcranial random noise stimulation on motor function: A comprehensive review of the literature. Physiol. Behav. 261:114073. doi: 10.1016/j.physbeh.2023.114073
Brunoni, A. R., Nitsche, M. A., Bolognini, N., Bikson, M., Wagner, T., Merabet, L., et al. (2012). Clinical research with transcranial direct current stimulation (tDCS): challenges and future directions. Brain Stimul. 5, 175–195. doi: 10.1016/j.brs.2011.03.002
Canessa, N., Basso, G., Carne, I., Poggi, P., and Gianelli, C. (2021). Increased decision latency in alcohol use disorder reflects altered resting-state synchrony in the anterior salience network. Sci. Rep. 11:19581. doi: 10.1038/s41598-021-99211-1
Canessa, N., Basso, G., Manera, M., Poggi, P., and Gianelli, C. (2022). Functional coherence in intrinsic frontal executive networks predicts cognitive impairments in alcohol use disorder. Brain Sci. 13:45. doi: 10.3390/brainsci13010045
Canessa, N., Crespi, C., Baud-Bovy, G., Dodich, A., Falini, A., Antonellis, G., et al. (2017). Neural markers of loss aversion in resting-state brain activity. Neuroimage 146, 257–265. doi: 10.1016/j.neuroimage.2016.11.050
Canessa, N., Crespi, C., Motterlini, M., Baud-Bovy, G., Chierchia, G., Pantaleo, G., et al. (2013). The functional and structural neural basis of individual differences in loss aversion. J. Neurosci. 33, 14307–14317. doi: 10.1523/JNEUROSCI.0497-13.2013
Centanni, S. W., Janes, A. C., Haggerty, D. L., Atwood, B., and Hopf, F. W. (2021). Better living through understanding the insula: why subregions can make all the difference. Neuropharmacology 198:108765. doi: 10.1016/j.neuropharm.2021.108765
Chang, L. J., Yarkoni, T., Khaw, M. W., and Sanfey, A. G. (2013). Decoding the role of the insula in human cognition: functional parcellation and large-scale reverse inference. Cereb. Cortex 23, 739–749. doi: 10.1093/cercor/bhs065
Chen, X., Voets, S., Jenkinson, N., and Galea, J. M. (2020). Dopamine-dependent loss aversion during efort-based decision-making. J. Neurosci. 40, 661–670. doi: 10.1523/JNEUROSCI.1760-19.2019
Clark, L., Studer, B., Bruss, J., Tranel, D., and Bechara, A. (2014). Damage to insula abolishes cognitive distortions during simulated gambling. Proc. Natl. Acad. Sci. 111, 6098–6103. doi: 10.1073/pnas.1322295111
Cloutman, L. L., Binney, R. J., Drakesmith, M., Parker, G. J., and Ralph, M. A. L. (2012). The variation of function across the human insula mirrors its patterns of structural connectivity: evidence from in vivo probabilistic tractography. Neuroimage 59, 3514–3521. doi: 10.1016/j.neuroimage.2011.11.016
Craig, A. D. (2009). How do you feel—now? The anterior insula and human awareness. Nat. Rev. Neurosci. 10, 59–70. doi: 10.1038/nrn2555
Crespi, C., Galandra, C., Manera, M., Basso, G., Poggi, P., and Canessa, N. (2019). Executive impairment in alcohol use disorder reflects structural changes in large-scale brain networks: a joint independent component analysis on gray-matter and white-matter features. Front. Psychol. 10:2479. doi: 10.3389/fpsyg.2019.02479
DaSilva, A. F., Truong, D. Q., DosSantos, M. F., Toback, R. L., Datta, A., and Bikson, M. (2015). State-of-art neuroanatomical target analysis of high-definition and conventional tDCS montages used for migraine and pain control. Front. Neuroanat. 9:89. doi: 10.3389/fnana.2015.00089
Di Bello, M., Giudetti, F., Palani, S., Petrocchi, N., McIntosh, R., and Ottaviani, C. (2023). Modulatory effects of transcranial direct current stimulation of right insula on compassion motivation. Int. J. Clin. Health Psychol. 23:100362. doi: 10.1016/j.ijchp.2022.100362
Dmochowski, J. P., Datta, A., Bikson, M., Su, Y., and Parra, L. C. (2011). Optimized multi-electrode stimulation increases focality and intensity at target. J. Neural Eng. 8:046011. doi: 10.1088/1741-2560/8/4/046011
Dosenbach, N. U., Fair, D. A., Miezin, F. M., Cohen, A. L., Wenger, K. K., Dosenbach, R. A., et al. (2007). Distinct brain networks for adaptive and stable task control in humans. Proc. Natl. Acad. Sci. 104, 11073–11078. doi: 10.1073/pnas.0704320104
Downar, J., Blumberger, D. M., and Daskalakis, Z. J. (2016). The neural crossroads of psychiatric illness: an emerging target for brain stimulation. Trends Cogn. Sci. 20, 107–120. doi: 10.1016/j.tics.2015.10.007
Edwards, D., Cortes, M., Datta, A., Minhas, P., Wassermann, E. M., and Bikson, M. (2013). Physiological and modeling evidence for focal transcranial electrical brain stimulation in humans: a basis for high-definition tDCS. Neuroimage 74, 266–275. doi: 10.1016/j.neuroimage.2013.01.042
Egner, T. (2007). Congruency sequence effects and cognitive control. Cogn. Affect. Behav. Neurosci. 7, 380–390. doi: 10.3758/CABN.7.4.380
Ellison-Wright, I., and Bullmore, E. (2010). Anatomy of bipolar disorder and schizophrenia: a meta-analysis. Schizophr. Res. 117, 1–12. doi: 10.1016/j.schres.2009.12.022
Elmasry, J., Loo, C., and Martin, D. (2015). A systematic review of transcranial electrical stimulation combined with cognitive training. Restor. Neurol. Neurosci. 33, 263–278. doi: 10.3233/RNN-140473
Esmaeilpour, Z., Shereen, A. D., Ghobadi-Azbari, P., Datta, A., Woods, A. J., Ironside, M., et al. (2020). Methodology for tDCS integration with fMRI. Hum. Brain Mapp. 41, 1950–1967. doi: 10.1002/hbm.24908
Fan, J., McCandliss, B. D., Sommer, T., Raz, A., and Posner, M. I. (2002). Testing the efciency and independence of attentional networks. J. Cogn. Neurosci. 14, 340–347. doi: 10.1162/089892902317361886
Fan, J., McCandliss, B., Fossella, J., Flombaum, J., and Posner, M. (2005). The activation of attentional networks. Neuroimage 26, 471–479. doi: 10.1016/j.neuroimage.2005.02.004
Faul, F., Erdfelder, E., Lang, A. G., and Buchner, A. (2007). G*Power 3: a flexible statistical power analysis program for the social, behavioral, and biomedical sciences. Behav. Res. Methods 39, 175–191. doi: 10.3758/bf03193146
Fedota, J. R., Matous, A. L., Salmeron, B. J., Gu, H., Ross, T. J., and Stein, E. A. (2016). Insula demonstrates a non-linear response to varying demand for cognitive control and weaker resting connectivity with the executive control network in smokers. Neuropsychopharmacology 41, 2557–2565. doi: 10.1038/npp.2016.62
Fertonani, A., Ferrari, C., and Miniussi, C. (2015). What do you feel if I apply transcranial electric stimulation? Safety, sensations and secondary induced effects. Clin. Neurophysiol. 126, 2181–2188. doi: 10.1016/j.clinph.2015.03.015
Fleming, S. M., and Dolan, R. J. (2010). Efects of loss aversion on post-decision wagering: implications for measures of awareness. Conscious. Cogn. 19, 352–363. doi: 10.1016/j.concog.2009.11.002
Friehs, M. A., and Frings, C. (2019). Offline beats online: transcranial direct current stimulation timing influences on working memory. Neuroreport 30, 795–799. doi: 10.1097/WNR.0000000000001272
Galandra, C., Basso, G., Manera, M., Crespi, C., Giorgi, I., Vittadini, G., et al. (2018). Salience network structural integrity predicts executive impairment in alcohol use disorders. Sci. Rep. 8:14481. doi: 10.1038/s41598-018-32828-x
Galandra, C., Basso, G., Manera, M., Crespi, C., Giorgi, I., Vittadini, G., et al. (2019). Abnormal fronto-striatal intrinsic connectivity reflects executive dysfunction in alcohol use disorders. Cortex 115, 27–42. doi: 10.1016/j.cortex.2019.01.004
Galandra, C., Crespi, C., Basso, G., Manera, M. R., Giorgi, I., Poggi, P., et al. (2021). Decreased information processing speed and decision-making performance in alcohol use disorder: combined neurostructural evidence from VBM and TBSS. Brain Imaging Behav. 15, 205–215. doi: 10.1007/s11682-019-00248-8
Gandiga, P. C., Hummel, F. C., and Cohen, L. G. (2006). Transcranial DC stimulation (tDCS): a tool for double-blind sham-controlled clinical studies in brain stimulation. Clin. Neurophysiol. 117, 845–850. doi: 10.1016/j.clinph.2005.12.003
Ghaziri, J., Tucholka, A., Girard, G., Houde, J. C., Boucher, O., Gilbert, G., et al. (2017). The corticocortical structural connectivity of the human insula. Cereb. Cortex 27, 1216–1228. doi: 10.1093/cercor/bhv308
Goodkind, M., Eickhoff, S. B., Oathes, D. J., Jiang, Y., Chang, A., Jones-Hagata, L. B., et al. (2015). Identification of a common neurobiological substrate for mental illness. JAMA Psychiatry 72, 305–315. doi: 10.1001/jamapsychiatry.2014.2206
Herwig, U., Bruehl, A. B., Kaffenberger, T., Baumgartner, T., Boeker, H., and Jaencke, L. (2010). Neural correlates of ‘pessimistic’attitude in depression. Psychol. Med. 40, 789–800. doi: 10.1017/S0033291709991073
Horvath, J. C., Forte, J. D., and Carter, O. (2015). Quantitative review finds no evidence of cognitive effects in healthy populations from single-session transcranial direct current stimulation (tDCS). Brain Stimul. 8, 535–550. doi: 10.1016/j.brs.2015.01.400
Huang, Y., Datta, A., Bikson, M., and Parra, L. C. (2019). Realistic volumetric-approach to simulate transcranial electric stimulation—ROAST—a fully automated open-source pipeline. J. Neural Eng. 16:056006. doi: 10.1088/1741-2552/ab208d
Huang, Y., Parra, L. C., and Haufe, S. (2016). The New York head—a precise standardized volume conductor model for EEG source localization and tES targeting. Neuroimage 140, 150–162. doi: 10.1016/j.neuroimage.2015.12.019
Hulvershorn, L. A., Karne, H., Gunn, A. D., Hartwick, S. L., Wang, Y., Hummer, T. A., et al. (2012). Neural activation during facial emotion processing in unmedicated bipolar depression, euthymia, and mania. Biol. Psychiatry 71, 603–610. doi: 10.1016/j.biopsych.2011.10.038
Ibrahim, C., Rubin-Kahana, D. S., Pushparaj, A., Musiol, M., Blumberger, D. M., Daskalakis, Z., et al. (2019). The insula: a brain stimulation target for the treatment of addiction. Front. Pharmacol. 10, 1–18. doi: 10.3389/fphar.2019.00720
Kahneman, D., and Tversky, A. (1979). Prospect theory: an analysis of decision under risk. Econometrica 47:263. doi: 10.2307/1914185
Kaster, T. S., Daskalakis, Z. J., Noda, Y., Knyahnytska, Y., Downar, J., Rajji, T. K., et al. (2018). Efficacy, tolerability, and cognitive effects of deep transcranial magnetic stimulation for late-life depression: a prospective randomized controlled trial. Neuropsychopharmacology 43, 2231–2238. doi: 10.1038/s41386-018-0121-x
Kaye, W. H., Fudge, J. L., and Paulus, M. (2009). New insights into symptoms and neurocircuit function of anorexia nervosa. Nat. Rev. Neurosci. 10, 573–584. doi: 10.1038/nrn2682
Keel, J. C., Smith, M. J., and Wassermann, E. M. (2001). A safety screening questionnaire for transcranial magnetic stimulation. Clin. Neurophysiol. 112:720. doi: 10.1016/S1388-2457(00)00518-6
Klink, K., Paßmann, S., Kasten, F. H., and Peter, J. (2020). The modulation of cognitive performance with transcranial alternating current stimulation: a systematic review of frequency-specific effects. Brain Sci. 10:932. doi: 10.3390/brainsci10120932
Knyahnytska, Y. O., Blumberger, D. M., Daskalakis, Z. J., Zomorrodi, R., and Kaplan, A. S. (2019). Insula H-coil deep transcranial magnetic stimulation in severe and enduring anorexia nervosa (SE-AN): a pilot study. Neuropsychiatr. Dis. Treat. 15, 2247–2256. doi: 10.2147/NDT.S207630
Kuhnen, C. M., and Knutson, B. (2005). The neural basis of financial risk taking. Neuron 47, 763–770. doi: 10.1016/j.neuron.2005.08.008
Lee, J. S. A., Bestmann, S., and Evans, C. (2021). A future of current flow modelling for transcranial electrical stimulation? Curr. Behav. Neurosci. Rep. 8, 150–159. doi: 10.1007/s40473-021-00238-5
Lee, M. R., Caparelli, E. C., Leff, M., Steele, V. R., Maxwell, A. M., McCullough, K., et al. (2020). Repetitive transcranial magnetic stimulation delivered with an H-coil to the right insula reduces functional connectivity between insula and medial prefrontal cortex. Neuromodulation 23, 384–392. doi: 10.1111/ner.13033
Levkovitz, Y., Harel, E. V., Roth, Y., Braw, Y., Most, D., Katz, L. N., et al. (2009). Deep transcranial magnetic stimulation over the prefrontal cortex: evaluation of antidepressant and cognitive effects in depressive patients. Brain Stimul. 2, 188–200. doi: 10.1016/j.brs.2009.08.002
Löffler, L. A., Radke, S., Morawetz, C., and Derntl, B. (2016). Emotional dysfunctions in neurodegenerative diseases. J. Comp. Neurol. 524, 1727–1743. doi: 10.1002/cne.23816
Malik, S., Jacobs, M., Cho, S. S., Boileau, I., Blumberger, D., Heilig, M., et al. (2018). Deep TMS of the insula using the H-coil modulates dopamine release: a crossover [11C] PHNO-PET pilot trial in healthy humans. Brain Imaging Behav. 12, 1306–1317. doi: 10.1007/s11682-017-9800-1
Markett, S., Heeren, G., Montag, C., Weber, B., and Reuter, M. (2016). Loss aversion is associated with bilateral insula volume. A voxel based morphometry study. Neurosci. Lett. 619, 172–176. doi: 10.1016/j.neulet.2016.03.029
Martin, D. M., Liu, R., Alonzo, A., Green, M., and Loo, C. K. (2014). Use of transcranial direct current stimulation (tDCS) to enhance cognitive training: effect of timing of stimulation. Exp. Brain Res. 232, 3345–3351. doi: 10.1007/s00221-014-4022-x
Mattavelli, G., Gallucci, A., Schiena, G., D'Agostino, A., Sassetti, T., Bonora, S., et al. (2019). Transcranial direct current stimulation modulates implicit attitudes towards food in eating disorders. Int. J. Eat. Disord. 52, 576–581. doi: 10.1002/eat.23046
Mattavelli, G., Lo Presti, S., Tornaghi, D., and Canessa, N. (2022). High-definition transcranial direct current stimulation of the dorsal anterior cingulate cortex modulates decision-making and executive control. Brain Struct. Funct. 227, 1565–1576. doi: 10.1007/s00429-022-02456-3
Medford, N., and Critchley, H. D. (2010). Conjoint activity of anterior insular and anterior cingulate cortex: awareness and response. Brain Struct. Funct. 214, 535–549. doi: 10.1007/s00429-010-0265-x
Medina, J., and Cason, S. (2017). No evidential value in samples of transcranial direct current stimulation (tDCS) studies of cognition and working memory in healthy populations. Cortex 94, 131–141. doi: 10.1016/j.cortex.2017.06.021
Meinzer, M., Antonenko, D., Lindenberg, R., Hetzer, S., Ulm, L., Avirame, K., et al. (2012). Electrical brain stimulation improves cognitive performance by modulating functional connectivity and task-specific activation. J. Neurosci. 32, 1859–1866. doi: 10.1523/JNEUROSCI.4812-11.2012
Mikkonen, M., Laakso, I., Tanaka, S., and Hirata, A. (2020). Cost of focality in TDCS: Interindividual variability in electric fields. Brain Stimul. 13, 117–124. doi: 10.1016/j.brs.2019.09.017
Moeller, S. J., Gil, R., Weinstein, J. J., Baumvoll, T., Wengler, K., Fallon, N., et al. (2022). Deep rTMS of the insula and prefrontal cortex in smokers with schizophrenia: proof-of-concept study. Schizophrenia 8, 1–9. doi: 10.1038/s41537-022-00224-0
Molnar-Szakacs, I., and Uddin, L. Q. (2022). Anterior insula as a gatekeeper of executive control. Neurosci. Biobehav. Rev. 139:104736. doi: 10.1016/j.neubiorev.2022.104736
Namkung, H., Kim, S. H., and Sawa, A. (2017). The insula: an underestimated brain area in clinical neuroscience, psychiatry, and neurology. Trends Neurosci. 40, 200–207. doi: 10.1016/j.tins.2017.02.002
Nitsche, M. A., Fricke, K., Henschke, U., Schlitterlau, A., Liebetanz, D., Lang, N., et al. (2003a). Pharmacological modulation of cortical excitability shifts induced by transcranial direct current stimulation in humans. J. Physiol. 553, 293–301. doi: 10.1113/jphysiol.2003.049916
Nitsche, M. A., Liebetanz, D., Lang, N., Antal, A., Tergau, F., and Paulus, W. (2003b). Safety criteria for transcranial direct current stimulation (tDCS) in humans. Clin. Neurophysiol. 114, 2220–2222. doi: 10.1016/S1388-2457(03)00235-9
Nitsche, M. A., Niehaus, L., Hoffmann, K. T., Hengst, S., Liebetanz, D., Paulus, W., et al. (2004). MRI study of human brain exposed to weak direct current stimulation of the frontal cortex. Clin. Neurophysiol. 115, 2419–2423. doi: 10.1016/j.clinph.2004.05.001
Palm, U., Reisinger, E., Keeser, D., Kuo, M.-F., Pogarell, O., Leicht, G., et al. (2013). Evaluation of sham transcranial direct current stimulation for randomized, placebo-controlled clinical trials. Brain Stimul. 6, 690–695. doi: 10.1016/j.brs.2013.01.005
Paulus, M. P., Rogalsky, C., Simmons, A., Feinstein, J. S., and Stein, M. B. (2003). Increased activation in the right insula during risk-taking decision making is related to harm avoidance and neuroticism. Neuroimage 19, 1439–1448. doi: 10.1016/S1053-8119(03)00251-9
Pisoni, A., Mattavelli, G., Papagno, C., Rosanova, M., Casali, A. G., and Romero Lauro, L. J. (2018). Cognitive enhancement induced by anodal tDCS drives circuit-specific cortical plasticity. Cereb. Cortex 28, 1132–1140. doi: 10.1093/cercor/bhx021
Poreisz, C., Boros, K., Antal, A., and Paulus, W. (2007). Safety aspects of transcranial direct current stimulation concerning healthy subjects and patients. Brain Res. Bull. 72, 208–214. doi: 10.1016/j.brainresbull.2007.01.004
Purcell, J. R., Jahn, A., Fine, J. M., and Brown, J. W. (2021). Neural correlates of visual attention during risky decision evidence integration. Neuroimage 234:117979. doi: 10.1016/j.neuroimage.2021.117979
Renzi, C., Ferrari, C., Schiavi, S., Pisoni, A., Papagno, C., Vecchi, T., et al. (2015). The role of the occipital face area in holistic processing involved in face detection and discrimination: a tDCS study. Neuropsychology 29, 409–416. doi: 10.1037/neu0000127
Roth, Y., Amir, A., Levkovitz, Y., and Zangen, A. (2007). Three-dimensional distribution of the electric field induced in the brain by transcranial magnetic stimulation using figure-8 and deep H-coils. J. Clin. Neurophysiol. 24, 31–38. doi: 10.1097/WNP.0b013e31802fa393
Rouder, J. N., Morey, R. D., Speckman, P. L., and Province, J. M. (2012). Default Bayes factors for ANOVA designs. J. Math. Psychol. 56, 356–374. doi: 10.1016/j.jmp.2012.08.001
Sagliano, L., Magliacano, A., Parazzini, M., Fiocchi, S., Trojano, L., and Grossi, D. (2019). Modulating interoception by insula stimulation: a double-blinded tDCS study. Neurosci. Lett. 696, 108–113. doi: 10.1016/j.neulet.2018.12.022
Samson, A. C., Kreibig, S. D., Soderstrom, B., Wade, A. A., and Gross, J. J. (2016). Eliciting positive, negative and mixed emotional states: a film library for affective scientists. Cognit. Emot. 30, 827–856. doi: 10.1080/02699931.2015.1031089
Seeley, W. W., Menon, V., Schatzberg, A. F., Keller, J., Glover, G. H., Kenna, H., et al. (2007). Dissociable intrinsic connectivity networks for salience processing and executive control. J. Neurosci. 27, 2349–2356. doi: 10.1523/JNEUROSCI.5587-06.2007
Sharp, C., Monterosso, J., and Montague, P. R. (2012). Neuroeconomics: a bridge for translational research. Biol. Psychiatry 72, 87–92. doi: 10.1016/j.biopsych.2012.02.029
Shenhav, A., Cohen, J. D., and Botvinick, M. M. (2016). Dorsal anterior cingulate cortex and the value of control. Nat. Neurosci. 19, 1286–1291. doi: 10.1038/nn.4384
Sokol-Hessner, P., Lackovic, S. F., Tobe, R. H., Camerer, C. F., Leventhal, B. L., and Phelps, E. A. (2015). Determinants of Propranolol’s selective effect on loss aversion. Psychol. Sci. 26, 1123–1130. doi: 10.1177/0956797615582026
Spagnolo, P. A., Wang, H., Srivanitchapoom, P., Schwandt, M., Heilig, M., and Hallett, M. (2019). Lack of target engagement following low-frequency deep transcranial magnetic stimulation of the anterior insula. Neuromodulation 22, 877–883. doi: 10.1111/ner.12875
Stein, M. B., Simmons, A. N., Feinstein, J. S., and Paulus, M. P. (2007). Increased amygdala and insula activation during emotion processing in anxiety-prone subjects. Am. J. Psychiatry 164, 318–327. doi: 10.1176/ajp.2007.164.2.318
Stratmann, M., Konrad, C., Kugel, H., Krug, A., Schöning, S., Ohrmann, P., et al. (2014). Insular and hippocampal gray matter volume reductions in patients with major depressive disorder. PloS One 9:e102692. doi: 10.1371/journal.pone.0102692
Surguladze, S. A., El-Hage, W., Dalgleish, T., Radua, J., Gohier, B., and Phillips, M. L. (2010). Depression is associated with increased sensitivity to signals of disgust: a functional magnetic resonance imaging study. J. Psychiatr. Res. 44, 894–902. doi: 10.1016/j.jpsychires.2010.02.010
Takahashi, H., Fujie, S., Camerer, C., Arakawa, R., Takano, H., Kodaka, F., et al. (2012). Norepinephrine in the brain is associated with aversion to fnancial loss. Mol. Psychiatry 18, 3–4. doi: 10.1038/mp.2012.7
Takahashi, T., Yücel, M., Lorenzetti, V., Tanino, R., Whittle, S., Suzuki, M., et al. (2010). Volumetric MRI study of the insular cortex in individuals with current and past major depression. J. Affect. Disord. 121, 231–238. doi: 10.1016/j.jad.2009.06.003
Takeuchi, H., Kawada, R., Tsurumi, K., Yokoyama, N., Takemura, A., Murao, T., et al. (2016). Heterogeneity of loss aversion in pathological gambling. J. Gambl. Stud. 32, 1143–1154. doi: 10.1007/s10899-015-9587-1
Taylor, K. S., Seminowicz, D. A., and Davis, K. D. (2009). Two systems of resting state connectivity between the insula and cingulate cortex. Hum. Brain Mapp. 30, 2731–2745. doi: 10.1002/hbm.20705
Terasawa, Y., Shibata, M., Moriguchi, Y., and Umeda, S. (2013). Anterior insular cortex mediates bodily sensibility and social anxiety. Soc. Cogn. Affect. Neurosci. 8, 259–266. doi: 10.1093/scan/nss108
Tom, S. M., Fox, C. R., Trepel, C., and Poldrack, R. A. (2007). The neural basis of loss aversion in decision-making under risk. Science 315, 515–518. doi: 10.1126/science.1134239
To, W. T., Eroh, J., Hart, J., and Vanneste, S. (2018). Exploring the effects of anodal and cathodal high-definition transcranial direct current stimulation targeting the dorsal anterior cingulate cortex. Sci. Rep. 8, 1–16. doi: 10.1038/s41598-018-22730-x
Trautwein, F. M., Singer, T., and Kanske, P. (2016). Stimulus-driven reorienting impairs executive control of attention: evidence for a common bottleneck in anterior insula. Cereb. Cortex 26, 4136–4147. doi: 10.1093/cercor/bhw225
Uddin, L. Q., Nomi, J. S., Hébert-Seropian, B., Ghaziri, J., and Boucher, O. (2017). Structure and function of the human insula. J. Clin. Neurophysiol. 34, 300–306. doi: 10.1097/WNP.0000000000000377
van der Groen, O., Potok, W., Wenderoth, N., Edwards, G., Mattingley, J. B., and Edwards, D. (2022). Using noise for the better: The effects of transcranial random noise stimulation on the brain and behavior. Neurosci. Biobehav. Rev. 138:104702. doi: 10.1016/j.neubiorev.2022.104702
van Doorn, J., van den Bergh, D., Böhm, U., Dablander, F., Derks, K., Draws, T., et al. (2021). The JASP guidelines for conducting and reporting a Bayesian analysis. Psychon. Bull. Rev. 28, 813–826. doi: 10.3758/s13423-020-01798-5
Van Hoornweder, S., Caulfield, A. K., Nitsche, M., Thielscher, A., and Meesen, R. L. J. (2022). Addressing transcranial electrical stimulation variability through prospective individualized dosing of electric field strength in 300 participants across two samples: the 2-SPED approach. J. Neural Eng. 19:056045. doi: 10.1088/1741-2552/ac9a78
Vergallito, A., Feroldi, S., Pisoni, A., and Romero Lauro, L. J. (2022). Inter-individual variability in tDCS effects: a narrative review on the contribution of stable, variable, and contextual factors. Brain Sci. 12:522. doi: 10.3390/brainsci12050522
Vergallito, A., Varoli, E., Pisoni, A., Mattavelli, G., Del Mauro, L., Feroldi, S., et al. (2023). State-dependent effectiveness of cathodal transcranial direct current stimulation on cortical excitability. Neuroimage 277:120242. doi: 10.1016/j.neuroimage.2023.120242
Von Siebenthal, Z., Boucher, O., Rouleau, I., Lassonde, M., Lepore, F., and Nguyen, D. K. (2017). Decision-making impairments following insular and medial temporal lobe resection for drug-resistant epilepsy. Soc. Cogn. Affect. Neurosci. 12, 128–137. doi: 10.1093/scan/nsw152
Wager, T. D., Sylvester, C. Y. C., Lacey, S. C., Nee, D. E., Franklin, M., and Jonides, J. (2005). Common and unique components of response inhibition revealed by fMRI. Neuroimage 27, 323–340. doi: 10.1016/j.neuroimage.2005.01.054
Weiss, M., and Lavidor, M. (2012). When less is more: evidence for a facilitative cathodal tDCS effect in attentional abilities. J. Cogn. Neurosci. 24, 1826–1833. doi: 10.1162/jocn_a_00248
Westfall, P. H., Johnson, W. O., and Utts, J. M. (1997). A Bayesian perspective on the Bonferroni adjustment. Biometrika 84, 419–427. doi: 10.1093/biomet/84.2.419
Wise, T., Radua, J., Via, E., Cardoner, N., Abe, O., Adams, T. M., et al. (2017). Common and distinct patterns of grey-matter volume alteration in major depression and bipolar disorder: evidence from voxel-based meta-analysis. Mol. Psychiatry 22, 1455–1463. doi: 10.1038/mp.2016.72
Woods, A. J., Antal, A., Bikson, M., Boggio, P. S., Brunoni, A. R., Celnik, P., et al. (2016). A technical guide to tDCS, and related non-invasive brain stimulation tools. Clin. Neurophysiol. 127, 1031–1048. doi: 10.1016/j.clinph.2015.11.012
Wylie, K. P., and Tregellas, J. R. (2010). The role of the insula in schizophrenia. Schizophr. Res. 123, 93–104. doi: 10.1016/j.schres.2010.08.027
Wysiadecki, G., Małkiewicz, A., Rożniecki, J., Polguj, M., Haładaj, R., Żytkowski, A., et al. (2018). Anatomical variations of the insular gyri: a morphological study and proposal of unified classification. Clin. Anat. 31, 347–356. doi: 10.1002/ca.23060
Xue, G., Lu, Z., Levin, I. P., and Bechara, A. (2010). The impact of prior risk experiences on subsequent risky decision-making: the role of the insula. Neuroimage 50, 709–716. doi: 10.1016/j.neuroimage.2009.12.097
Keywords: high-definition tDCS, insula, executive control, decision-making, loss/risk aversion, treatment
Citation: Gorrino I, Canessa N and Mattavelli G (2023) Testing the effect of high-definition transcranial direct current stimulation of the insular cortex to modulate decision-making and executive control. Front. Behav. Neurosci. 17:1234837. doi: 10.3389/fnbeh.2023.1234837
Edited by:
Yoann Stussi, University of Geneva, SwitzerlandReviewed by:
Davide Gheza, Washington University in St. Louis, United StatesValerio Manippa, University of Bari Aldo Moro, Italy
Copyright © 2023 Gorrino, Canessa and Mattavelli. This is an open-access article distributed under the terms of the Creative Commons Attribution License (CC BY). The use, distribution or reproduction in other forums is permitted, provided the original author(s) and the copyright owner(s) are credited and that the original publication in this journal is cited, in accordance with accepted academic practice. No use, distribution or reproduction is permitted which does not comply with these terms.
*Correspondence: Giulia Mattavelli, giulia.mattavelli@iusspavia.it