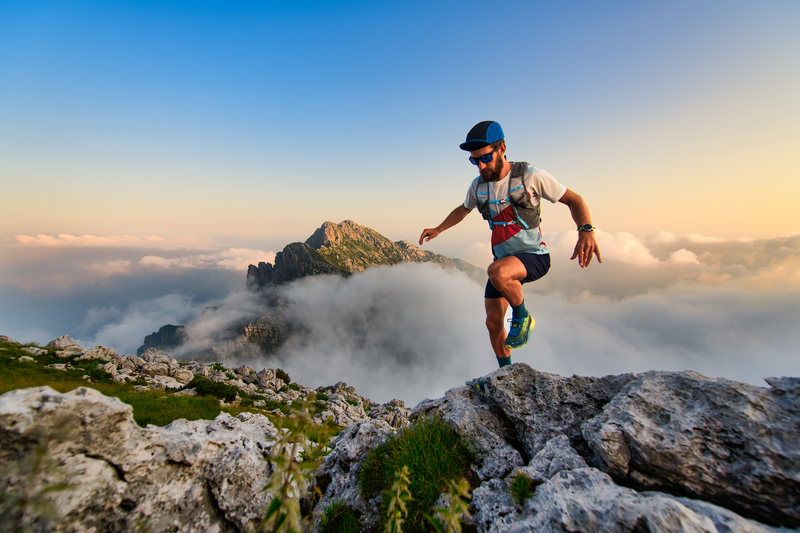
95% of researchers rate our articles as excellent or good
Learn more about the work of our research integrity team to safeguard the quality of each article we publish.
Find out more
BRIEF RESEARCH REPORT article
Front. Behav. Neurosci. , 04 July 2022
Sec. Emotion Regulation and Processing
Volume 16 - 2022 | https://doi.org/10.3389/fnbeh.2022.880641
This article is part of the Research Topic How Does Sleep Help Regulate Negative Emotion? View all 16 articles
Sleep deprivation has in several studies been found to increase anxiety. However, the extent to which this anxiogenic effect depends on one’s underlying trait anxiety has not previously been determined. Using two separate sleep-loss experiments, the current research investigated whether trait anxiety (STAI-T) moderates the increase in state anxiety (STAI-S) following one night of total sleep loss (study 1, N = 182, age 25.3 ± 6.5, 103 women) and two nights of partial sleep restriction (study 2, N = 67, age 26.5 ± 7.4, 38 women). Both studies showed the expected anxiogenic effect of sleep loss, and a clear relationship between trait anxiety and state anxiety. However, the anxiogenic effect of sleep loss was not moderated by trait anxiety, as there was an equal impact regardless of trait anxiety level. These findings indicate that, although sleep loss is related to general anxiety as well as anxiety disorders, for a non-clinical sample the anxiogenic effect of short-term sleep loss is not affected by baseline levels of anxiety.
Sleep is intertwined with daily anxiety, with higher levels of anxiety acting both as a predictor of sleep quality and a consequence of sleep loss (Alvaro et al., 2013; Horváth et al., 2016; Pires et al., 2016). Several studies have focused on the anxiogenic effect of sleep deprivation, suggesting that hypoactivity in the medial prefrontal cortex is a potential mechanism (Ben Simon et al., 2020a) and that gray matter volume may determine vulnerability differently for men and women (Goldstein-Piekarski et al., 2018), indicating that there are individual differences in this anxiogenic effect. Indeed, although the relationship between sleep and anxiety does not seem to depend on the impact of mood (Ben Simon et al., 2020a), the emotional responses to sleep loss of anxious individuals may be amplified compared to those with less trait anxiety (Goldstein et al., 2013; Alfano et al., 2020). This suggests that trait anxiety may predict vulnerability to the anxiogenic effects of sleep deprivation as well, but surprisingly little attention has been devoted to the relationship between trait anxiety and the effects of sleep loss in healthy subjects (Palmer and Alfano, 2020).
In order to understand who is vulnerable or resilient to the anxiogenic effects of sleep loss, more research is needed regarding underlying predictive factors. For example, there are large individual differences in how people respond to sleep deprivation in the cognitive domains (Tkachenko and Dinges, 2018), and these may in part be predicted by trait-level, domain-specific, cognitive abilities (Brieva et al., 2021; Floros et al., 2021). If the same is true for affective domains, those low in trait anxiety may have a smaller anxiogenic effect of sleep deprivation than those high in trait anxiety. For example, in adolescents with generalized anxiety disorder (GAD), natural variations in sleep duration has been shown as a predictor of morning anxiety, whereas for healthy controls it has not (Mullin et al., 2017). Specifically, for the adolescents with GAD, shorter sleep duration was associated with more morning anxiety the following day. However, as disturbed sleep is strongly comorbid with anxiety disorders it is difficult to disentangle cause and effect from observational studies of clinical samples. In other words, it is yet unknown whether there is a moderating effect of subclinical variations in trait anxiety on the anxiogenic effect of experimentally induced sleep loss. The aim of this brief report is thus to elucidate whether interindividual, subclinical variation in trait anxiety predicts the impact of sleep deprivation (study 1) and sleep restriction (study 2) on state anxiety.
One hundred and eighty-two healthy participants were randomly assigned to one of two conditions: one night of total sleep deprivation (TSD; N = 91, average age 25.4 ± 6.21, range 18–45 years; 52 women) or normal 8h-sleep (NS; N = 91, average age 25.3 ± 6.82, range 17–45 years; 51 women). Exclusion criteria during the initial screening included physical or mental health problems, sleep disturbances, a subjective sleep need outside of 7–9 h, shift work in the previous 3 weeks, and addiction to coffee or other drugs (see Holding et al., 2019 for a complete list of exclusion criteria). Participants provided written informed consent and were compensated for their time. The study was approved by the Regional Ethical Review Board in Stockholm (2014/1766-21).
Prior to being informed of their condition, participants filled out the trait version of the State-Trait Anxiety Inventory (STAI; Spielberger et al., 1983; Julian, 2011). This assesses “How you generally feel” with statements such as “I feel safe” and “I am a stable person.” The response options range from “1-almost never” to “4-almost always.” Participants were then instructed to sleep 8–9 h per night (between 22:00 and 08:00) in their home for three nights, with sleep times assessed using actigraphy (Sadeh, 2011) and sleep diaries. After the third night, they were informed of their sleep condition and were either instructed to spend one more night according to this schedule (NS group) and come into the lab at 10:00 the following day (the test day), or arrive at the lab at 22:00 that evening and stay awake until the following day (TSD group). Participants in the sleep-deprivation condition were monitored throughout the night, and not allowed to engage in any strenuous activities. Around 10:30 on the test day (∼26.5 h awake for the TSD group, ∼2.5 h awake for the NS group) they filled out the state version of STAI. This version assesses “How you feel at this moment” with statements such as “I feel safe” and “I feel nervous.” Response options range from “1-not at all” to “4-very much so.”
State-Trait Anxiety Inventory was scored according to the standard instructions, adding up the responses to all items while reverse-scoring anxiety-absent items. This results in a value between 20 and 80 for each scale. All STAI scores were then converted to z-scores, and Bayesian linear modeling was used to test the main effects and interaction of TSD and trait anxiety on state anxiety. The Bayesian models provide a point effect estimate and a probability distribution of plausible alternative values (posterior distribution). To determine uncertainty around our point estimate, we use the 95% Credible Interval (95% CI; 2.5 and 97.5% quantiles of the posterior). The 95% credible interval is analogous to the 95% confidence interval though with greater precision (Gray et al., 2015).
A key benefit of taking a Bayesian approach is the ability to assess the relative strength of evidence for different hypotheses. Therefore, we can state (unlike in classical frequentist analysis which can only be used to reject the null hypothesis and never support it) whether the data supports the alternative hypothesis, the null hypothesis, or doesn’t provide enough information to support either. In other words, we can make clearer conclusions about situations in which no effect is present. In our case, we compare models with and without a coefficient of interest to calculate Bayes factors (BF10). A rule of thumb for interpreting these is that a BF10 greater than 3 suggests that there is evidence for the alternative hypothesis (i.e., that the coefficient improves the model prediction), a BF10 of less than 0.33 provides evidence of the null hypothesis (i.e., that the coefficients does not improve the model prediction), and a BF10 between these values suggests that there is not enough data to support either hypothesis (Dienes, 2014).
Data and analysis code can be found at https://osf.io/tcdne/. STAI values (both state and trait) over 3 SD from the mean were excluded. The data of three participants (all from the normal-sleep condition) were excluded for this reason. One further participant (from the normal-sleep condition) was excluded due to not completing the state STAI and another participant (from the sleep-deprivation condition) was excluded due to not completing the trait STAI.
The analysis was run using in R (version 4.1.0; R Core Team, 2021) with RStudio (version 1.4.1106; RStudio Team, 2021). To run the main analysis we used the brms (version 2.16.1; Bürkner, 2017) package using 8 chains and 40,000 iterations (20,000 warm-up). Priors were set on the coefficients with mean 0 and SD 1. All other parameters used the default non-informative regularizing priors of the brms package. To calculate the relative evidence of adding coefficients of interest (compared to a simpler model without this coefficient, i.e., the null hypothesis), we computed Bayes factors (BF10) using the BayestestR package (version 0.10.0; Makowski et al., 2019). For data preprocessing we used the following packages: dplyr, readxl, sjplot, tidyr, and readr (Wickham and Bryan, 2019; Wickham and Hester, 2020; Lüdecke, 2021; Wickham, 2021; Wickham et al., 2021).
Sixty-seven healthy participants (average age 26.5 ± 7.4, range 18–46 years; 38 women) took part in a within-subject protocol with two conditions: normal sleep and sleep restriction. Exclusion criteria included poor physical or mental health, sleep disturbances, a sleep need outside of 7–9 h, shift work during the previous 3 weeks, and an addiction to coffee or other drugs. Participants provided written informed consent and were compensated for their time. The study was approved by the Regional Ethical Review Board in Stockholm (2010/1506-31).
Participants came to the lab on two occasions, with sleep conditions in a randomized counterbalanced order and a washout period of minimum 1 week between the two. They arrived at the lab in the afternoon (14:30 ± 1 h). The arrival time could vary between 13:00 and 16:00, but was always the same within participant. Upon arrival, they spent 30 min sitting down in a light-controlled room and filling out questionnaires. In the normal-sleep condition (two consecutive nights of 8 h of sleep) they filled out both the state and trait versions of STAI, and in the sleep-restriction condition (two consecutive nights of no more than 4 h of sleep) they filled out only the state version. In order to ensure adherence to the study protocol, participants wore actigraphs during all four nights, filled out sleep diaries, and sent a text message to the experimenter upon bedtime and waking.
Hypotheses and analyses for study 2 were preregistered prior to looking at the data. All STAI scores were converted to z-scores, and Bayesian mixed-effect modeling was used to test the main effects and interaction of TSD and trait anxiety on state anxiety. Preregistration, data, and analysis code can be found at https://osf.io/ysa42 and https://osf.io/tcdne/.
As planned in the preregistration, STAI values (both state and trait) over 3 SD from the mean were excluded. Since the study was a repeated-measures cross-over design, it was possible for individuals to be excluded for a single session only. Two participants’ data were excluded in the sleep-restriction session for this reason, and thus their data is only represented in the normal-sleep session. A further participant did not complete the state STAI for the sleep-restriction session, and thus their data was only included in the normal-sleep session.
The statistical analysis was essentially identical to study 1. The only difference is that due to the repeated-measures design, we included random intercepts for participant ID to account for clustering of variance within these units.
See Table 1 for average sleep times and anxiety scores between groups and conditions. See Supplementary Table 1 for average sleep and wake times.
In both studies, sleep loss led to a clear increase in state anxiety, and trait anxiety predicted higher state anxiety for all groups and conditions (Table 2). However, there was no apparent moderating effect of trait anxiety on the anxiogenic effect of sleep loss (Table 2 and Figure 1). The Bayes factor of the interaction coefficients suggests that the data is approximately 4.6–6.8 times more likely to be generated under a model without a moderating effect of trait anxiety on the effect of sleep loss.
Figure 1. Association between trait anxiety and state anxiety reported before (gray) and after (red) sleep loss. The left-hand graph represents the results from study 1, using between-subjects sleep deprivation. The right-hand graph represents the results from study 2, using within-subjects sleep restriction. Model predictions are based on the models in Table 2. Solid line represents estimated association, with 95% credible intervals. Dots represent raw data points (jittered).
State anxiety when well rested and when sleep restricted were correlated (study 2; estimate = 0.46, 95% CI [0.23, 0.70], BF10 = 110.51 compared to intercept only model), and trait anxiety in a univariate model reliably predicted state anxiety (study 1: estimate = 0.46, 95% CI [0.32, 0.60], BF10 compared to intercept-only model = 42700000; study 2: estimate = 0.52, 95% CI [0.38, 0.66], BF10 compared to intercept-only model = 171000000).
As preregistered robustness checks, we ran the analysis in two further ways. Firstly, we compared participants in both studies who rated higher or lower than 1 SD from the mean trait anxiety score (see Supplementary Figure 1 and Supplementary Table 2). We also conducted the primary analyses without excluding any participants (i.e., not excluding outliers, see Supplementary Figure 2 and Supplementary Table 3). The results of these supplementary analyses follow the same pattern as the primary results presented.
As a non-preregistered robustness check, we also ran the analysis including participant gender as a covariate. The results follow the same pattern as the primary results presented (see Supplementary Table 4).
In the modern 24-society, sleep loss is prevalent (Webb and Agnew, 1975; Knutson et al., 2010; Shochat, 2012; Blom et al., 2020; but see Marshall and Lallukka, 2018 for discussion). Rates of anxiety are also high (Wiegner et al., 2015; Bosman et al., 2019), which studies have shown can have roots in lack of sleep (see Ben Simon et al., 2020b for review). We aimed to understand more about this relationship, by assessing whether the effect of sleep loss on anxiety was stronger in individuals who generally were already reporting high levels of anxiety.
In both studies, we found a clear influence of sleep loss on self-reported state anxiety. Sleep loss led to an increase in anxiety in the range of 0.60–0.65 SD, indicating a medium-to-large effect size (Sawilowsky, 2009). However, any potential moderation by trait anxiety of the impact of insufficient sleep on state anxiety was negligible. Bayes factors from both studies suggest that the data is much more likely if sleep loss has an approximately equal impact on state anxiety, irrespective of trait anxiety level. Additionally, this pattern was robust to two alternative methods of analyzing the data. These results provide important evidence regarding individual differences in the anxiogenic effects of sleep loss; although sleep loss causes an increase in state anxiety across levels of trait anxiety, the effect appears to be no different for those with high or low levels of trait anxiety.
The bidirectionality of sleep and anxiety has not been taken into account in this study. For example, it is possible that higher levels of anxiety following sleep loss is related to more subsequent rumination, in turn resulting in more troubled sleep (e.g., Gordon et al., 2019). This could amplify the effect for those high in trait anxiety across time, laying the foundation for the strong correlational relationship found between the two (e.g., Ben Simon et al., 2020a). Indeed, sleep disturbances in childhood are predictive of anxiety disorders in adulthood, even when controlling for internalizing problems and socioeconomic status (Gregory et al., 2005). For those who are more vulnerable, i.e., have higher trait anxiety, the increase in state anxiety due to sleep loss may thus be more detrimental even though it is not objectively larger. A potential limitation here is the use of a self-report measure of anxiety, which may be affected differently than more objective measures (see, e.g., Egloff and Schmukle, 2004).
Although higher trait anxiety seems to modify the emotional effects of sleep loss (Alfano et al., 2020; Palmer and Alfano, 2020), our results indicate that this is not due to differences in the anxiogenic effect of sleep loss. Rather, the resulting high levels of state anxiety for those higher in trait anxiety may be the explaining factor. It is possible that there is an inflection point at which one’s levels of anxiety relate to stronger emotional reactions to one’s environment.
The results of the two studies do not appear to be due to ceiling effects in state anxiety for those with high trait anxiety. The range of state anxiety was similar between the two groups (study 1) and two conditions (study 2), with no participant getting the maximum possible score in either. Although our exclusion of individuals with state anxiety over 3 SD above the mean may have inadvertently induced such a ceiling effect, especially in those with high trait anxiety, our supplementary analysis where we do not use this exclusion criteria (Supplementary Figure 2 and Supplementary Table 3) suggests that this did not affect the conclusions. Normative values for STAI-S in Sweden (where both studies were conducted) have been estimated at 33.2 ± 9.6, although this was based on a rather small sample (Forsberg and Björvell, 1993). Other reported normative values, in larger, non-Swedish samples were around 33–35.5 (SD ranging from 7.3 to 8.6) for STAI-S and 33–36 (SD ranging from 7.8 to 8.9) for STAI-T (Knight et al., 1983; Crawford et al., 2011). The participants in this study thus seem to represent a fairly normal population in terms of anxiety levels. Although those scoring high in trait anxiety (e.g., above 40 or 53) may be at a higher risk of developing an anxiety disorder (e.g., Dennis et al., 2013; Zingano et al., 2019), this was a subclinical sample and it is likely that individuals with clinical levels of anxiety respond differently to sleep loss, as indicated by previous research (e.g., Mullin et al., 2017).
In conclusion, variations in trait anxiety does not predict the anxiogenic effect of short-term sleep loss in a non-clinical sample. The findings of this study bring us closer to elucidating risk factors and protective mechanisms regarding the negative effects of sleep loss.
The datasets presented in this study can be found in online repositories. The names of the repository/repositories and accession number(s) can be found below: https://osf.io/tcdne/.
The studies involving human participants were reviewed and approved by the Regional Ethical Review Board in Stockholm. The patients/participants provided their written informed consent to participate in this study.
TS and BH conceptualized and designed the study. TS organized the data collections and wrote the first draft on the manuscript. BH performed the statistical analyses. Both authors wrote sections of and revised the manuscript, and approved the submitted version.
This was funded by the Swedish Research Council (HS-2013-18), FORTE (Swedish Research Council for Health, Working Life and Welfare) (2013-01539) and the Swedish Foundation for Humanities and Social Sciences (P13-1159:1).
The authors declare that the research was conducted in the absence of any commercial or financial relationships that could be construed as a potential conflict of interest.
All claims expressed in this article are solely those of the authors and do not necessarily represent those of their affiliated organizations, or those of the publisher, the editors and the reviewers. Any product that may be evaluated in this article, or claim that may be made by its manufacturer, is not guaranteed or endorsed by the publisher.
We would like to thank John Axelsson, Danja Porada, Johan Lundström, Malin Eriksson, Therese Johansson, Sara Gershagen, Lukasz Cybulski, and several students for help with data collection.
The Supplementary Material for this article can be found online at: https://www.frontiersin.org/articles/10.3389/fnbeh.2022.880641/full#supplementary-material
Alfano, C. A., Bower, J. L., Harvey, A. G., Beidel, D. C., Sharp, C., and Palmer, C. A. (2020). Sleep restriction alters children’s positive emotional responses, but effects are moderated by anxiety. J. Child Psychol. Psychiatry 61, 1150–1159. doi: 10.1111/jcpp.13287
Alvaro, P. K., Roberts, R. M., and Harris, J. K. (2013). A systematic review assessing bidirectionality between sleep disturbances, anxiety, and depression. Sleep 36, 1059–1068. doi: 10.5665/sleep.2810
Ben Simon, E., Rossi, A., Harvey, A. G., and Walker, M. P. (2020a). Overanxious and underslept. Nat. Hum. Behav. 4, 100–110. doi: 10.1038/s41562-019-0754-8
Ben Simon, E., Vallat, R., Barnes, C. M., and Walker, M. P. (2020b). Sleep loss and the socio-emotional brain. Trends Cogn. Sci. 24, 435–450. doi: 10.1016/j.tics.2020.02.003
Blom, V., Kallings, L. V., Ekblom, B., Wallin, P., Andersson, G., Hemmingsson, E., et al. (2020). Self-reported general health, overall and work-related stress, loneliness, and sleeping problems in 335,625 swedish adults from 2000 to 2016. Int. J. Environ. Res. Public Health 17:511. doi: 10.3390/ijerph17020511
Bosman, R. C., Ten Have, M., de Graaf, R., Muntingh, A. D., van Balkom, A. J., and Batelaan, N. M. (2019). Prevalence and course of subthreshold anxiety disorder in the general population: a three-year follow-up study. J. Affect. Disord. 247, 105–113. doi: 10.1016/j.jad.2019.01.018
Brieva, T. E., Casale, C. E., Yamazaki, E. M., Antler, C. A., and Goel, N. (2021). Cognitive throughput and working memory raw scores consistently differentiate resilient and vulnerable groups to sleep loss. Sleep 44:zsab197. doi: 10.1093/sleep/zsab197
Bürkner, P.-C. (2017). brms: an R package for bayesian multilevel models using stan. J. Stat. Softw. 80, 1–28.
Crawford, J., Cayley, C., Lovibond, P. F., Wilson, P. H., and Hartley, C. (2011). Percentile norms and accompanying interval estimates from an australian general adult population sample for self-report mood scales (BAI, BDI, CRSD, CES-D, DASS, DASS-21, STAI-X, STAI-Y, SRDS, and SRAS). Aust. Psychol. 46, 3–14. doi: 10.1111/j.1742-9544.2010.00003.x
Dennis, C.-L., Coghlan, M., and Vigod, S. (2013). Can we identify mothers at-risk for postpartum anxiety in the immediate postpartum period using the State-Trait Anxiety Inventory? J. Affect. Disord. 150, 1217–1220. doi: 10.1016/j.jad.2013.05.049
Dienes, Z. (2014). Using bayes to get the most out of non-significant results. Front. Psychol. 5:781. doi: 10.3389/fpsyg.2014.00781
Egloff, B., and Schmukle, S. C. (2004). Gender differences in implicit and explicit anxiety measures. Pers. Individ. Differ. 36, 1807–1815. doi: 10.1016/j.paid.2003.07.002
Floros, O., Axelsson, J., Almeida, R., Tigerström, L., Lekander, M., Sundelin, T., et al. (2021). Vulnerability in executive functions to sleep deprivation is predicted by subclinical attention-deficit/hyperactivity disorder symptoms. Biol. Psychiatry. Cogn. Neurosci. Neuroimaging 6, 290–298. doi: 10.1016/j.bpsc.2020.09.019
Forsberg, C., and Björvell, H. (1993). Swedish population norms for the GHRI, HI and STAI-state. Qual. Life Res. 2, 349–356. doi: 10.1007/BF00449430
Goldstein, A. N., Greer, S. M., Saletin, J. M., Harvey, A. G., Nitschke, J. B., and Walker, M. P. (2013). Tired and apprehensive: anxiety amplifies the impact of sleep loss on aversive brain anticipation. J. Neurosci. 33, 10607–10615. doi: 10.1523/JNEUROSCI.5578-12.2013
Goldstein-Piekarski, A. N., Greer, S. M., Saletin, J. M., Harvey, A. G., Williams, L. M., and Walker, M. P. (2018). Sex, sleep deprivation, and the anxious brain. J. Cogn. Neurosci. 30, 565–578. doi: 10.1162/jocn_a_01225
Gordon, A. M., Del Rosario, K., Flores, A. J., Mendes, W. B., and Prather, A. A. (2019). Bidirectional links between social rejection and sleep. Psychosom. Med. 81, 739–748. doi: 10.1097/PSY.0000000000000669
Gray, K., Hampton, B., Silveti-Falls, T., McConnell, A., and Bausell, C. (2015). Comparison of bayesian credible intervals to frequentist confidence intervals. J. Mod. Appl. Stat. Methods 14, 43–52. doi: 10.22237/jmasm/1430453220
Gregory, A. M., Caspi, A., Eley, T. C., Moffitt, T. E., O’Connor, T. G., and Poulton, R. (2005). Prospective longitudinal associations between persistent sleep problems in childhood and anxiety and depression disorders in adulthood. J. Abnorm. Child Psychol. 33, 157–163. doi: 10.1007/s10802-005-1824-0
Holding, B. C., Sundelin, T., Lekander, M., and Axelsson, J. (2019). Sleep deprivation and its effects on communication during individual and collaborative tasks. Sci. Rep. 9:3131. doi: 10.1038/s41598-019-39271-6
Horváth, A., Montana, X., Lanquart, J.-P., Hubain, P., Szûcs, A., Linkowski, P., et al. (2016). Effects of state and trait anxiety on sleep structure: a polysomnographic study in 1083 subjects. Psychiatry Res. 244, 279–283. doi: 10.1016/j.psychres.2016.03.001
Julian, L. J. (2011). Measures of anxiety: state-trait anxiety inventory (STAI), beck anxiety inventory (BAI), and hospital anxiety and depression scale-anxiety (HADS-A). Arthritis Care Res. 63, S467–S472. doi: 10.1002/acr.20561
Knight, R. G., Waal-Manning, H. J., and Spears, G. F. (1983). Some norms and reliability data for the state-trait anxiety inventory and the zung self-rating depression scale. Br. J. Clin. Psychol. 22, 245–249. doi: 10.1111/j.2044-8260.1983.tb00610.x
Knutson, K. L., Van Cauter, E., Rathouz, P. J., DeLeire, T., and Lauderdale, D. S. (2010). Trends in the prevalence of short sleepers in the USA: 1975–2006. Sleep 33, 37–45. doi: 10.1093/sleep/33.1.37
Lüdecke, D. (2021). sjPlot: Data Visualization for Statistics in Social Science_. R package version 2.8.8. Available online at: https://CRAN.R-project.org/package=sjPlot (accessed May 25, 2021).
Makowski, D., Ben-Shachar, M. S., and Lüdecke, D. (2019). bayestestR: describing effects and their uncertainty, existence and significance within the bayesian framework. J. Open Source Softw. 4:1541. doi: 10.21105/joss.01541
Marshall, N. S., and Lallukka, T. (2018). Sleep pirates–are we really living through a sleep deprivation epidemic and what’s stealing our sleep? Eur. J. Public Health 28, 394–395. doi: 10.1093/eurpub/cky016
Mullin, B. C., Pyle, L., Haraden, D., Riederer, J., Brim, N., Kaplan, D., et al. (2017). A preliminary multimethod comparison of sleep among adolescents with and without generalized anxiety disorder. J. Clin. Child Adolesc. Psychol. 46, 198–210. doi: 10.1080/15374416.2016.1220312
Palmer, C. A., and Alfano, C. A. (2020). Anxiety modifies the emotional effects of sleep loss. Curr. Opin. Psychol. 34, 100–104. doi: 10.1016/j.copsyc.2019.12.001
Pires, G. N., Bezerra, A. G., Tufik, S., and Andersen, M. L. (2016). Effects of acute sleep deprivation on state anxiety levels: a systematic review and meta-analysis. Sleep Med. 24, 109–118. doi: 10.1016/j.sleep.2016.07.019
R Core Team (2021). R: A Language and Environment for Statistical Computing. Vienna: R Foundation for Statistical Computing.
RStudio Team (2021). RStudio: Integrated Development Environment for R. RStudio, PBC. Available online at: http://www.rstudio.com/ (accessed February 22, 2021).
Sadeh, A. (2011). The role and validity of actigraphy in sleep medicine: an update. Sleep Med. Rev. 15, 259–267.
Sawilowsky, S. S. (2009). New effect size rules of thumb. J. Mod. Appl. Stat. Methods 8, 597–599. doi: 10.22237/jmasm/1257035100
Shochat, T. (2012). Impact of lifestyle and technology developments on sleep. Nat. Sci. Sleep 4, 19–31. doi: 10.2147/NSS.S18891
Spielberger, C. D., Gorsuch, R. L., Lushene, R., and Jacobs, G. A. (1983). Manual for the State-Trait Anxiety Inventory. Palo Alto, CA: Consulting Psychologists Press.
Tkachenko, O., and Dinges, D. F. (2018). Interindividual variability in neurobehavioral response to sleep loss: a comprehensive review. Neurosci. Biobehav. Rev. 89, 29–48. doi: 10.1016/j.neubiorev.2018.03.017
Webb, W. B., and Agnew, H. W. (1975). Are we chronically sleep deprived? Bull. Psychon. Soc. 6, 47–48. doi: 10.3758/BF03333140
Wickham, H. (2021). Tidyr: Tidy Messy Data. R package version 1.1.3. Available online at: https://CRAN.R-project.org/package=tidyr (accessed March 3, 2021).
Wickham, H., and Bryan, J. (2019). Readxl: Read Excel Files. R package version 1.3.1. Available online at: https://CRAN.R-project.org/package=readxl (accessed May 5, 2021).
Wickham, H., and Hester, J. (2020). Readr: Read Rectangular Text Data. R package version 1.4.0. Available online at: https://CRAN.R-project.org/package=readr (accessed February 22, 2021).
Wickham, H., François, R., Henry, L., and Müller, K. (2021). Dplyr: A grammar of data manipulation. R package version 1.0.6. Available online at: https://CRAN.R-project.org/package=dplyr (accessed May 5, 2021).
Wiegner, L., Hange, D., Björkelund, C., and Ahlborg, G. (2015). Prevalence of perceived stress and associations to symptoms of exhaustion, depression and anxiety in a working age population seeking primary care—An observational study. BMC Fam. Pract. 16:38. doi: 10.1186/s12875-015-0252-7
Zingano, B., de, L., Guarnieri, R., Diaz, A. P., Schwarzbold, M. L., Wolf, P., et al. (2019). Hospital anxiety and depression scale-anxiety subscale (HADS-A) and the state-trait anxiety inventory (STAI) accuracy for anxiety disorders detection in drug-resistant mesial temporal lobe epilepsy patients. J. Affect. Disord. 246, 452–457. doi: 10.1016/j.jad.2018.12.072
Keywords: anxiety, STAI, sleep loss, individual differences, sleep restriction
Citation: Sundelin T and Holding BC (2022) Trait Anxiety Does Not Predict the Anxiogenic Response to Sleep Deprivation. Front. Behav. Neurosci. 16:880641. doi: 10.3389/fnbeh.2022.880641
Received: 21 February 2022; Accepted: 16 June 2022;
Published: 04 July 2022.
Edited by:
Candice A. Alfano, University of Houston, United StatesReviewed by:
Federico Salfi, University of L’Aquila, ItalyCopyright © 2022 Sundelin and Holding. This is an open-access article distributed under the terms of the Creative Commons Attribution License (CC BY). The use, distribution or reproduction in other forums is permitted, provided the original author(s) and the copyright owner(s) are credited and that the original publication in this journal is cited, in accordance with accepted academic practice. No use, distribution or reproduction is permitted which does not comply with these terms.
*Correspondence: Tina Sundelin, dGluYS5zdW5kZWxpbkBwc3ljaG9sb2d5LnN1LnNl
Disclaimer: All claims expressed in this article are solely those of the authors and do not necessarily represent those of their affiliated organizations, or those of the publisher, the editors and the reviewers. Any product that may be evaluated in this article or claim that may be made by its manufacturer is not guaranteed or endorsed by the publisher.
Research integrity at Frontiers
Learn more about the work of our research integrity team to safeguard the quality of each article we publish.