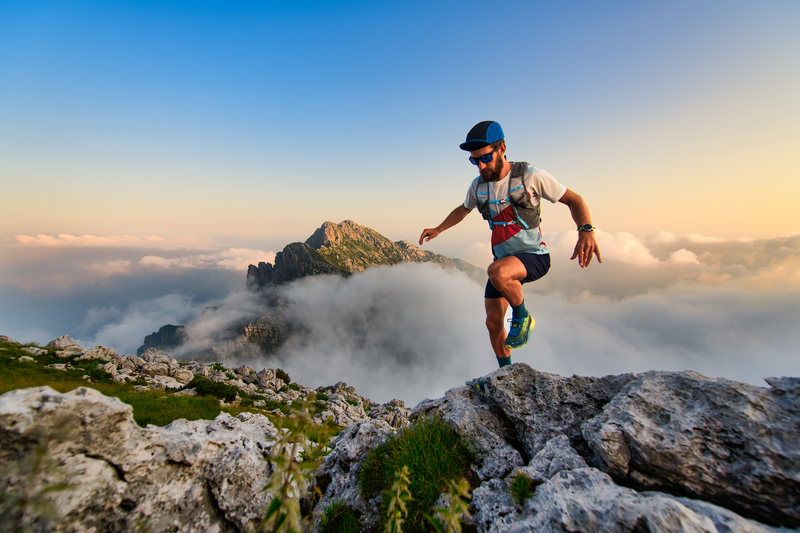
95% of researchers rate our articles as excellent or good
Learn more about the work of our research integrity team to safeguard the quality of each article we publish.
Find out more
ORIGINAL RESEARCH article
Front. Behav. Neurosci. , 02 June 2022
Sec. Behavioral Endocrinology
Volume 16 - 2022 | https://doi.org/10.3389/fnbeh.2022.856276
This article is part of the Research Topic Sex Hormone Fluctuations Across the Female Lifespan: Mechanisms of Action on Brain Structure, Function, and Behavior View all 12 articles
Recent decades have witnessed increased research efforts to clarify how the menstrual cycle influence females’ cognitive and emotional functions. Despite noticeable progress, the research field faces the challenges of inconsistency and low generalizability of research findings. Females of reproductive ages are a heterogeneous population. Generalizing the results of female undergraduates to women in the workplace might be problematic. Furthermore, the critical cognitive processes for daily life and work deserve additional research efforts for improved ecological validity. Thus, this study investigates cognitive performance across the menstrual cycle using a sample of young nurses with similar duties. We developed a mini-computerized cognitive battery to assess four mental skills critical for nursing work: cognitive flexibility, divided attention, response inhibition, and working memory. Participants completed the cognitive battery at menses, late-follicular, and mid-luteal phases. In addition, they were classified into low- and high workload groups according to their subjective workload ratings. Our results demonstrate a general mid-luteal cognitive advantage. Besides, this study reveals preliminary evidence that workload modulates the menstrual cycle effect on cognition. Only females of low workload manifest the mid-luteal cognitive advantage on divided attention and response inhibition, implying that a suitable workload threshold might be necessary for regular neuro-steroid interactions. Thus, this study advocates the significance of research focusing on the cycling brain under workloads.
Ovarian hormones, such as estradiol and progesterone, fluctuate during the menstrual cycle in healthy females of reproductive age. The estradiol levels gradually increase after the menses phase, peaking in the late follicular phase and then dropping after ovulation and rising again in the mid-luteal phase to moderate levels. The progesterone levels increase after ovulation and peak in the middle of the luteal phase. Then, the two ovarian hormones drop to the lowest levels before the onset of the next menses. Thus, the menstrual cycle is a convenient and ecological model of ovarian hormones. Recent years have witnessed an explosion of research on how sex hormones and the menstrual cycle shape female brains (Barth et al., 2015; Ycaza Herrera et al., 2019; Beltz and Moser, 2020; Le et al., 2020; Dubol et al., 2021; Hidalgo-Lopez and Pletzer, 2021).
Estradiol and progesterone have neuroactive effects. The hypothalamic-pituitary-gonadal (HPG) axis regulates the reproductive processes and modulates cognitive and emotional functions through direct or indirect projection to the prefrontal cortex, hippocampus, thalamus, and brainstem (Morrison et al., 2006; Le et al., 2020). It has long been hypothesized that cognitive performance across the menstrual cycle might vary due to the fluctuation of ovarian hormones. The effect of the menstrual cycle has been found on social preference (Durante et al., 2014; Zhuang and Wang, 2014; Wang and Chen, 2020; Wang et al., 2021), cognitive ability (Hussain et al., 2016; Hidalgo-Lopez and Pletzer, 2017; Leeners et al., 2017; Pletzer et al., 2017; Scheuringer and Pletzer, 2017), motor learning (Ikarashi et al., 2020), cortical structures (Lisofsky et al., 2015; Catenaccio et al., 2016; Pletzer et al., 2018), and brain functions (Barth et al., 2016; Diekhof and Ratnayake, 2016; Hidalgo-Lopez and Pletzer, 2019, 2021; Pletzer et al., 2019; Wang et al., 2020a). Moreover, the late follicular or luteal phase advantage on cognition has always been advocated because of high neuroprotective steroids (Sundstrom-Poromaa and Gingnell, 2014; Zhuang et al., 2020; Wang et al., 2021). For example, previous studies suggest that females show superior social cognitive performance during their luteal than menses or follicular phase (Wang and Chen, 2020; Wang et al., 2020a,b, 2021). Although the neuroprotective role of estradiol has been advocated, the role of progesterone remains ambiguous (Baudry et al., 2013). Some studies suggest that progesterone may antagonize rather than synergize estradiol effects (Rosario et al., 2006; Carroll et al., 2008). It is hard to separate the effects of progesterone and estradiol across the menstrual cycle because both hormone levels are high in the mid-luteal phase. Thus, studying the three different phases across the menstrual cycle is necessary to clarify the relationship between hormones.
Despite the appealing association between the menstrual cycle and cognition, the empirical evidence is far from consistent (Sundstrom-Poromaa and Gingnell, 2014; Sundstrom-Poromaa, 2018; Beltz and Moser, 2020; Le et al., 2020). The evolutionary hypothesis implicated that women might show visuospatial ability advantage during their early follicular phase (low ovarian steroids) and verbal ability advantage in the luteal phase (high ovarian steroids). However, Sundstrom-Poromaa and Gingnell (2014) summarized that the supporting evidence is insufficient in the literature. In addition, their following review further suggests that the menstrual cycle might influence emotion, but has a limited effect on cognitive function (Sundstrom-Poromaa, 2018). However, recent neuroimaging studies have revealed consistent evidence that the menstrual cycle modulates the structure, functional activation, and connectivity of brain regions that are responsible for cognitive control (e.g., prefrontal cortex) and memory (e.g., hippocampus) (Beltz and Moser, 2020; Dubol et al., 2021). Thus, it might be too soon to reject the menstrual cycle’s potential effect on “cold” cognition.
The inconsistency might be because the effect of the menstrual cycle is too transient to be captured by behavioral assessment or noise due to methodological flaws (Le et al., 2020). However, it has been long overlooked in the field that healthy females of reproductive age are a heterogeneous population with huge variability in their social-economic status, years of education, social support, occupation, and work pressure. Many studies recruited undergraduate or graduated female students from the campus or females in nearby communities of different professions. These findings might not generalize seamlessly to some specific populations. Only a few studies have employed homogenous samples within a particular workplace, such as nurses (Hatta and Nagaya, 2009). Investigating the cycling brain in specific workplaces is, thus, a valuable research direction.
Furthermore, the inconsistency might be due to the menstrual cycle’s interaction with other factors (Bernal and Paolieri, 2022). It has been proposed that estrogen and progesterone interact with cognition-related neurotransmitter systems, including serotoninergic, dopaminergic, gamma-aminobutyric-acid (GABA)-ergic, and glutamatergic pathways, with profound effects on brain structure and function (Barth et al., 2015). For example, recent evidence shows that the effect of estradiol status on working memory function depends on the baseline dopamine levels (Jacobs and D’Esposito, 2011). Using the eye blink rate (EBR), an indicator of striatal dopamine levels, one following study reveals that females with lower EBR showed superior Stroop performance during their luteal phase and vice versa (Hidalgo-Lopez and Pletzer, 2017). Hidalgo-Lopez and Pletzer (2019) recent work also suggests that baseline performance modulates the menstrual cycle effect on the inhibitory control ability. Besides the factors mentioned above, there might be many contextual and individual factors deserving increasing research attention.
Female nurses account for 90% of the global nursing workforce and play irreplaceable roles in public health (World Health Organization, 2020). Meanwhile, female nurses undertake noticeable workloads. For example, in China, on average, a nurse in a general hospital takes care of 8 patients in the daytime and 23 patients at night (Shen et al., 2020). Compared with other careers, the nursing job characterizes by mental pressures induced by multitasking and attentional interferences. Potter et al. (2005) use a cognitive task analysis methodology to reveal that a nurse must hold 11 activities in mind in the acute care work setting. The nursing job is also full of interruptions associated with procedure failures and clinical errors (Westbrook et al., 2010). Thus, an efficient nurse needs to switch flexibly among tasks (cognitive flexibility), attend to patients and clinical signals simultaneously (divided attention), inhibit automatic, habitual but inappropriate actions (response inhibition), and store necessary information in mind (working memory). Research focusing on the female nurse population is, thus, valuable for promoting their occupational health.
Previous studies have suggested that hormones from the hypothalamic-pituitary-adrenal (HPA) axis regulate the HPG axis (Oyola and Handa, 2017). The HPA axis is the coordinator of the brain’s fight-or-flight response, which increases cortisol production to deal with stressful events. Previous studies have also demonstrated an inverted U-shaped relationship between workload and task performance (Ma et al., 2020). However, it is still ambiguous whether workload would interact with the menstrual cycle to affect cognitive performance. This study investigates whether workload modulates the cycling brain using a homogenous nurse sample. The workload here refers to the cognitive, emotional, and physiological resources expended to complete the task requirement (Alghamdi, 2016). We chose four representative cognitive paradigms (task-switching, divided attention, spatial Stroop, and multiple change detection) to target core mental skills necessary for nursing work. In addition, a self-report measure, namely the National Aeronautics and Space Administration Task Load Index (NASA-TLX), quantifies the nursing workload, which can tease apart six sources of work pressures (Hart, 2016). Although the workload can be evaluated physiologically (Borghini et al., 2014), self-report measures are helpful to provide a convenient, inexpensive, reliable, and valid sampling (Wickens, 2008). We hypothesized that female nurses perform better during their mid-luteal phase. In addition, workload might be a potential modulatory factor of the menstrual cycle effect.
We recruited 96 healthy right-handed female registered nurses in a local hospital. All of them had a regular menstrual cycle of 24–35 days (Le et al., 2020) and variability between cycles of less than 7 days in the past 3 months, with normal or corrected-to-normal vision, had not taken oral contraceptive or other hormonal medications within the previous 3 months, no history of nicotine or alcohol abuse, no sleep disorders, and no neurological, psychiatric, or endocrine disorders, including premenstrual dysphoric disorder (PMDD) and premenstrual syndrome (PMS). Ten participants were excluded due to their actual cycle phase falling out of the normal range during the experiment session according to their follow-up report on the onset of the next cycle. Seven participants dropped out for personal reasons, leaving a final sample of 79 nurses (M = 25.52 years, SD = 4.33 years) with a mean cycle length of 29.42 days (SD = 1.69). The study was approved by the local ethics committee and was conducted following the Declaration of Helsinki. All participants gave written informed consent and received monetary compensation.
Participants completed an online screening questionnaire to determine whether they were eligible to participate in the study. Eligible participants enrolled in the test session were required to record their menses’ start date and duration for at least 3 months to double-check whether their menstrual cycle is regular. The first author (MX) interviewed them privately to survey their subjective nursing workload and check their menstrual cycle information and calculate their cycle phase for those participants. The menstrual cycle mapping was determined using the backward counting procedure widely used in the literature (Zhuang and Wang, 2014; Hidalgo-Lopez and Pletzer, 2017; Schaumberg et al., 2017; Wang et al., 2021). Specifically, we defined the menses phase (low estradiol levels, low progesterone levels) as the 1–4 days after the onset of menstruation; the late-follicular phase (estradiol levels peak and low progesterone levels) as the 3 days before the predicted ovulation; and the mid-luteal phase (moderate estradiol levels and high progesterone levels) as 3 days after the expected ovulation to 3 days before the next onset of the menstruation. We calculated the predicted ovulation by subtracting 14 days from the expected next menstruation onset, determined using each participant’s average cycle length in the last 3 months.
The study was a within-subject, longitudinal design. Thus, participants attended three behavior test sessions during the menses, late-follicular, and mid-luteal phases. These cycle phases were set apart by at least 6 days. The starting session was counterbalanced among participants. About one-third of participants started their first session in the menses, one-third in the late-follicular, and one-third in the mid-luteal phase. For each test session, participants first rated their negative emotions of the past week. Then, they completed a ∼50-min mini-computerized cognitive battery, including inhibitory control, cognitive flexibility, divided attention, and working memory. The experiment environment was a quiet room. A well-trained graduate student (MX) instructed and monitored tests of all participants. After the third test session, participants were tracked for their subsequent menses to validate their predicted cycle phase falling into the normal range. Those who violated were excluded from analysis even after completing the study.
The NASA-TLX measured the subjective workload using six subscales: mental demand, physical demand, temporal demand, performance, effort, and frustration levels (Tubbs-Cooley et al., 2018). Each subscale is rated on a 20-point scale (0 = low, 20 = high), but for the performance scale (0 = good, 20 = poor). Higher scores indicate increased workloads.
The Depression Anxiety Stress Scale-21 (DASS-21) measured negative emotions in the past week, including depression, anxiety, and stress subscale. Each subscale contains seven items. Each item was scored on a 4-point scale ranging from 0 to 3. The final score was the summed score multiplied by two (Henry and Crawford, 2005). Higher scores indicate higher depression, anxiety, or stress levels, respectively.
The mini-computerized cognitive battery was developed using GNU Octave and Psychtoolbox 3.16 (Brainard, 1997; Pelli, 1997) under the UBUNTU 18.04 system on a Thinkpad T61 laptop (12-inch, 1024 × 768 pixels, 50 Hz refresh rate). Participants completed the battery sitting about 50 cm in front of the laptop screen in a quiet room. The battery included four tasks measuring inhibitory control, cognitive flexibility, divided attention, and working memory capacity.
The spatial Stroop task is a paradigm measuring inhibitory control (Aidman et al., 2019). A typical trial starts with a fixation in the screen center for 600 ms. After a blank screen of 200 ms, an arrow pointing leftward or rightward appeared on the left or the right side of the screen. Participants needed to report the arrow direction by pressing corresponding keys as soon as possible with accuracy ensured. The primary interest variable was the congruency between the arrow direction (leftward, rightward) and their position (left side, right side) (Figure 1A). There were 10 practice trials and 60 test trials, including 24 congruent and 36 incongruent trials. The error rate for each condition was summarized. We also calculated the mean reaction time after removal of error trials and trials too fast (< 150 ms) or too slow (> 1,500 ms).
Figure 1. The mini-computerized cognitive battery. (A) Spatial Stroop task. A typical trial starts with a fixation (600 ms). After a blank screen (200 ms), an arrow appears on the left or the right side of the screen. Participants needed to report the arrow direction by pressing corresponding keys as soon as possible with the accuracy ensured. (B) Task-switch task. On each trial, a number (randomly selected from 1, 2, 3, 4, 6, 7, 8, 9) appeared in a cell of a 2 × 2 grid. If the number appeared in the upper row, participants needed to respond whether it was greater or less than 5. If the number appeared in the lower row, they answered whether it was even or odd. The first number appeared in the right-top cell and changed location clock-wisely in the subsequent trials. (C) Divided attention task. A square appears at regular intervals on the screen, and at the same time, participants listen to a sound. Every trial appeared every 1 s and lasted 1 s each. Participants needed to detect changes in either the visual sequence or the audio sequence. Whenever the square gets noticeably lighter, or the sound gets noticeably higher pitch twice in a row, they need to press the space bar as soon as possible. (D) Working memory task. A typical trial starts with a fixation (500 ms), followed by an encoding array (5 colored squares). The colored squares appeared on an imaginary circle (500 ms). A test array appeared after a 1,000 ms delay. The test array could have 0, 1, 2, or 5 changed items with equal probability. Participants had to indicate whether the test array and the encoding array differed.
The task-switching paradigm measures cognitive flexibility (Monsell, 2003; Aidman et al., 2019). A number randomly chosen from 1 to 9 appeared in one cell of a 2 × 2 grid for each trial. If the number appeared in the upper row, participants needed to respond whether it was greater or less than 5. If the number appeared in the lower row, they answered whether it was even or odd. The first number appeared in the right-top cell and changed location clockwise in the subsequent trials. The trial was in repeat condition (same task rule) if the number appeared on the top-right and bottom-left cell; other trials were in switch condition (change of task rule) (Figure 1B). There were 12 practice trials and 60 test trials, including 29 repeat trials (with the first trial discarded) and 30 switching trials. We calculated error rates and mean reaction time for each condition. Error trials and trials too fast (< 150 ms) or low (> 1,500 ms) were excluded from reaction time analysis.
The audiovisual cross-modal monitoring task measures divided attention ability (Himi et al., 2019). A square appears at regular intervals on the screen center, and, at the same time, participants listen to a sound. Participants needed to detect changes in either the visual sequence or the audio sequence. Sometimes the square gets noticeably lighter, and sometimes the sound gets a noticeably higher pitch. Whenever the square gets noticeably lighter or the sound gets noticeably higher pitch twice in a row, they need to press the space bar as soon as possible (Figure 1C). Thirty-two practice trials were followed by the test trials, which consisted of 200 trials. Every trial appeared every 1 s and lasted 1 s each. The index of the task was the sensitivity calculated according to signal detection theory using the non-parametric sensitivity measure (A′) (Stanislaw and Todorov, 1999) and the mean reaction time of correct responses.
The multiple change detection paradigm estimates working memory capacity (Gold et al., 2019). A typical trial starts with a fixation in the screen center for 500 ms, followed by an encoding array (5 colored squares). The colored squares appeared on an imaginary circle with a radius of 120 pixels centered on the center of the screen for 500 ms. A test array appeared after a 1,000-ms delay. The test array could have 0, 1, 2, or 5 changed items with equal probability. Participants had to indicate whether the test array and the encoding array differed (Figure 1D). There were 240 trials with 60 trials for each change type. The working memory capacity (K) was estimated according to a computational model (Feuerstahler et al., 2019) and used R (R Core Team, 2021) and the est_KAG function.1
Participants were classified into high and low workload groups using the mean value of NASA TLX total scores of all participants as the cut-off criterion. The group difference was examined using the independent-samples t-test and chi-square test. We conducted an omnibus mixed factorial analysis of variance (ANOVA) first for each emotion and task measure and performed post hoc comparisons using the LSD method if necessary. The p-value was adjusted using the Greenhouse–Geisser procedure in case of violation of the sphericity hypothesis. We translate p-values in the language of evidence to avoid the black-or-white null-hypothesis testing with an arbitrary p-value cut-off (Muff et al., 2022). The statistical analysis software was IBM SPSS Statistics for Windows (Version 22.0. Armonk, NY, United States: IBM). To exclude the practice effect and potential confounding effect of age, we also conducted linear mixed model analyses by controlling the effect of the session and participants’ age. The supplementary analysis, in the form of an Rnotebook, is available online (see Data Availability Statement). The linear mixed-effect model was conducted using R (version 4.1.1) (R Core Team, 2021), afex (Singmann et al., 2021), and lme4 (Bates et al., 2015) package.
We divided participants into low (n = 41) and high (n = 38) workload groups according to the mean NASA-TLX score (M = 69.65, SD = 14.81). Table 1 compares the low and high workloads groups on demographic information and mental health measures.
A mixed factorial ANOVA of 2 (group: low, high workload) × 3 (cycle phase: menses, late follicular, mid-luteal) was conducted for depression, anxiety, and stress scores, respectively. The results revealed no evidence that the high and the low workload group differed on each emotion subscale (all ps > 0.29, see Supplementary Table 1 for detailed information). There was no evidence of the effect of the cycle phase, no matter in terms of main effect or interaction effect, on the depression and stress scores (all ps > 0.1). However, there was weak evidence of the main effect of the cycle phase on the anxiety score (p = 0.071).
A mixed factorial ANOVA of 2 (group: low, high workload) × 3 (cycle phase: menses, late follicular, mid-luteal) × 2 (congruency: congruent, incongruent) was conducted on error rate and reaction time, respectively. See Supplementary Table 2 for a summary of the ANOVA analysis.
On the measure of error rate, there was moderate evidence for a main effect of congruency [F(1, 77) = 4.873, p = 0.03, η2 = 0.06], indicating generally more errors in the incongruent (M = 0.020, SE = 0.003) than the congruent condition (M = 0.014, SE = 0.002). The statistical evidence supporting the main effect of group and cycle phase was little or no (all ps > 0.2). Besides, we found little or no evidence for the two-way interactions (all ps > 0.05). However, there was moderate evidence for the interaction effect among the three factors [F(2, 154) = 3.885, p = 0.023, η2 = 0.048]. A repeated-measures ANOVA of 3 (cycle phase: menses, late follicular, and mid-luteal) × 2 (congruency: congruent, incongruent) was then performed for the low workload group and the high workload group, respectively.
There was only weak evidence for the low workload group for the main effect of the cycle phase [F(1.56, 62.3) = 2.87, p = 0.076, η2 = 0.067]. However, there was strong evidence for the main effect of congruency [F(1, 40) = 7.76, p = 0.008, η2 = 0.163], and moderate evidence for the interaction effect between the cycle phase and the congruency [F(2, 80) = 4.56, p = 0.013, η2 = 0.102]. Follow-up analyses found no evidence that performance on the congruent condition varied among the cycle phase [F(2, 39) = 0.39, p = 0.68, η2 = 0.02]. However, on the error rate of the incongruent condition, there was strong evidence for the main effect of the cycle phase [F(2, 39) = 5.76, p = 0.006, η2 = 0.23]. Error rates of the mid-luteal phase were lower than the menses (p = 0.003) and the late follicular phase (p = 0.037). There was little or no evidence for the difference between the menses and the late follicular phase on the incongruent condition (p = 0.183). We found little or no evidence for the main effect and interaction term involving the cycle phase in the high workload group [cycle: F(2, 74) = 0.17, p = 0.846, η2 = 0.005, cycle × congruency: F(2, 74) = 1.18, p = 0.312, η2 = 0.031]. See Figure 2 for an illustration.
Figure 2. The interactive effect of workload and the menstrual cycle on the error rate measure of the spatial Stroop task. Error bars represent the standard error of the mean. * and ** indicates p < 0.05 and p < 0.01, correspondingly.
On the measure of reaction time, there was strong evidence for the main effect of congruency [F(1, 77) = 12.759, p = 0.001, η2 = 0.142], indicating faster responses in the congruent condition (M = 487 ms, SE = 8 ms) than the incongruent condition (M = 499 ms, SE = 8 ms). We also found moderate evidence for the main effect of group [F(1, 77) = 5.353, p = 0.023, η2 = 0.065], suggesting that the high workload group (M = 476 ms, SD = 11 ms) responded faster than the low workload group (M = 511 ms, SD = 11 ms) in general. However, we found little or no evidence for the main effect or interaction effect involving cycle phase (all ps > 0.05).
A mixed 2 (group: low, high workload) × 3 (cycle phase: menses, late follicular, mid-luteal) × 2 (condition: repeat, switch) factorial ANOVA was conducted on error rate and reaction time, respectively. See Supplementary Table 3 for a summary of the ANOVA analysis.
On the measure of error rate, there was very strong evidence for the main effect of condition [F(1, 77) = 18.058, p < 0.001, η2 = 0.19], suggesting more errors in the switch condition (M = 0.028, SE = 0.003) than the repeat condition (M = 0.016, SE = 0.002). We also found strong evidence for the main effect of cycle phase [F(1.703, 131.121) = 5.271, p = 0.009, η2 = 0.064], indicating fewer errors in the mid-luteal phase than the menses phase (p = 0.006) and late follicular phase (p = 0.007). However, there was little or no evidence that the menses and the late follicular phase differ (p = 0.317). See Figure 3 for an illustration. The results revealed little or no evidence for the main effect of group [F(1, 77) = 0.628, p = 0.431, η2 = 0.008]. In addition, we found little or no evidence for the two-way and three-way interactions (all ps > 0.2, see Supplementary Table 3).
Figure 3. The main effect of the menstrual cycle on the error rate measure of the task-switching task. Error bars represent the standard error of the mean. ** indicates p < 0.01.
On the measure of reaction time, there was a strong evidence for the main effect of condition [F(1, 77) = 445.941, p < 0.001, η2 = 0.853], indicating slower responses in the switch (M = 987 ms, SE = 15 ms) than the repeat condition (M = 825 ms, SE = 15 ms). However, we found little or no evidence for the main effect of the group and cycle phase and the two-way and three-way interactions (all ps > 0.45, see Supplementary Table 3).
A mixed 2 (group: low, high workload) × 3 (cycle phase: menses, late follicular, mid-luteal) factorial ANOVA was conducted on the sensitivity measure (A′) and reaction time, respectively. See Supplementary Table 4 for a summary of the ANOVA analysis.
The ANOVA on the sensitivity measure (A′) revealed moderate evidence for the main effect of cycle phase [F(2, 154) = 3.29, p = 0.035, η2 = 0.042]. Post hoc analysis indicated higher A’ in the mid-luteal phase than in the menses phase (p = 0.021) and late follicular phase (p = 0.030). However, there was no evidence of the difference between the menses and the late follicular phase (p = 0.780) (Figure 4). We also found little or no evidence for the main effect and interaction terms involving the group (all ps > 0.17, see Supplementary Table 4).
Figure 4. The main effect of the menstrual cycle on the sensitivity measure of the divided attention task. * indicates p < 0.05.
On the measure of reaction time, there was little or no evidence for the main effect of cycle phase [F(2, 154) = 0.74, p = 0.479, η2 = 0.01] or group [F(1, 77) = 0.473, p = 0.494, η2 = 0.006]. However, the results revealed moderate evidence for the interaction between the group and cycle phase [F(2, 154) = 3.21, p = 0.043, η2 = 0.04]. Follow-up analyses revealed that moderate evidence that participants of low workload responded faster during the mid-luteal phase than the menses (p = 0.037) and little or no evidence for other comparisons (mid-L vs. late-F: p = 0.12; late-F vs. menses: p = 0.635). However, there was no evidence for the cycle effect in the high workload group (all ps > 0.11) (Figure 5).
Figure 5. The interactive effect of workload and the menstrual cycle on the reaction time measure of the divided attention task. Error bars represent the standard error of the mean. * indicates p < 0.05.
A mixed 2 (group: low, high workload) × 3 (cycle phase: menses, late follicular, mid-luteal) factorial ANOVA on the working memory capacity estimation (K) was conducted. See Supplementary Table 5 for a summary of ANOVA results. However, there was no evidence for the main effects [group: F(1, 77) = 0.563, p = 0.455, η2 = 0.007; cycle phase: F(2, 154) = 0.705, p = 0.496, η2 = 0.009] and the interaction effect [F(2, 154) = 0.009, p = 0.991, η2 < 0.001]. See Supplementary Table 5 for detailed information.
The present study investigates cognitive performance across the menstrual cycle using a sample of nurses with similar duties. As summarized in Table 2, our results demonstrate evidence for the general cognitive advantage of the mid-luteal phase, as manifested by the main effect of the menstrual cycle on the error rate measure of the task-switch task and the sensitivity measure of the divided attention task. Moreover, the present study demonstrates that workload might be a modulatory factor of the menstrual cycle effect, with preliminary evidence that the cycle phase effect on the reaction time measure of divided attention task and the error rate measure of response inhibition task is only manifested in the low workload group.
Our results demonstrate a mid-luteal phase advantage on the error rate measure of task-switching. The task-switching paradigm adopted in the current research is a classical measure of cognitive flexibility (Monsell, 2003). Our results replicate a typical switch-cost phenomenon: people make slower responses and more errors when the task rule is switched compared with repeat. Using the Wisconsin Card Sorting Task, Solis-Ortiz et al. (2004) reported a similar finding that females show superior cognitive flexibility during their luteal phase. A recent study using a similar task-switching task in functional MRI (fMRI) demonstrated that enhanced prefrontal activations after hormone therapy (sequential estradiol-plus-progesterone) were associated with improved task-switching performance in a sample of early menopausal women (Girard et al., 2017). However, Girard et al. (2017) did not observe beneficial effects on behavioral measures. Their failure to detect behavioral effects might be due to the task design tailored for fMRI or the small sample size.
It should be noteworthy that we found no evidence for the interaction between the menstrual cycle and the switch condition. In other words, the menstrual cycle modulates the general task performance, but not the switching cost in our study. Thus, the performance improvement in the mid-luteal phase might not specifically suggest the ovarian hormone’s effect on the task-switching process. The general task performance improvement in the task-switch task is likely due to an attentional augment mechanism. Female participants in our study might show relatively good skills in neglecting task-irrelevant and focusing on task-relevant information during their mid-luteal phase. This hypothesis is consistent with our finding on the divided attention task.
On the divided attention task, female nurses in our research show superior sensitivity in detecting the visual and auditory changes during their mid-luteal phase. We adopted a cross-modal monitoring task to assess participants’ ability to simultaneously attend to visual and auditory modalities (Himi et al., 2019). The effect of hormones or the menstrual cycle on different facets of attention has been explored, such as sustained attention (Solis-Ortiz and Corsi-Cabrera, 2008), selective attention (Thimm et al., 2014; Brotzner et al., 2015; Wang and Chen, 2020), and divided attention (Leeners et al., 2017; Pletzer et al., 2017). A seminal work from Pletzer et al. (2017) systematically examined the sex and menstrual cycle effect on three aspects of attention, which reports a follicular phase advantage on the accuracy measure of divided and sustained attention. Unlike us, Pletzer et al. (2017) use paper-pencil tests to assess selective and divided attention. Moreover, Pletzer et al. (2017) only compared the luteal and follicular phases, making a direct comparison with us impossible. Leeners et al. (2017) adopted a similar bimodal attention task as us, but they failed to detect any association between hormone levels and divided attention (Leeners et al., 2017). However, Leeners et al. (2017) used a very heterogeneous sample, including both endocrine disorders and healthy females, making a direct comparison with us impossible.
The general cognitive advantage of the mid-luteal phase, manifested in the task switch and divided attention task, is consistent with recent evidence of the progesterone effect on prefrontal function (Dubol et al., 2021). The prefrontal cortex plays essential roles in cognitive control, influencing attention, impulse inhibition, prospective memory, and cognitive flexibility. A recent systematic review of multimodal neuroimaging studies suggests that enhanced prefrontal activations in the middle luteal phase are a convergent finding in the literature (Dubol et al., 2021). For example, Pletzer et al. (2019) investigated brain activations and functional connectivity changes when women perform a spatial navigation task and a verbal fluency task during the menstrual cycle. Intriguingly their study reveals that progesterone increases the BOLD responses of the dorsal prefrontal cortex and caudate during the luteal cycle phase irrespective of the task (Pletzer et al., 2019). Whether the main effect of the menstrual cycle on task switching and divided attention performance was driven by progesterone’s impact on the prefrontal cortex requires additional research efforts. Future studies might use fMRI to clarify this issue.
This study reveals intriguing interactions between the error rate measure of the spatial Stroop task and the reaction time measure of the divided attention task. Analysis of the two tasks reveals a similar finding that only low workload groups performed better during their mid-luteal phases. However, the mid-luteal cognitive advantage disappeared in high workload groups.
In the spatial Stroop task, participants make a speeded response to the arrow direction and inhibit the dominant tendency to respond with the ipsilateral hand matching the arrow position when direction and position information conflict. Cognitive control is necessary to focus on the task-relevant information (selective attention) and inhibit the dominant response tendency (response inhibition) (Pires et al., 2018). Unlike us, previous studies mainly used the color Stroop task, and the results were inconsistent (Hatta and Nagaya, 2009; Hidalgo-Lopez and Pletzer, 2017). For example, a study reported that females performed worse during their luteal phase than during the menses phase (Hatta and Nagaya, 2009). In contrast, we did not find evidence for the main effect but evidence for the interactive effect of the menstrual cycle. There was also moderate evidence for the interaction effect on the reaction time measure of the divided attention task.
Our findings parallel recent studies on modulatory factors (Jacobs and D’Esposito, 2011; Hidalgo-Lopez and Pletzer, 2017, 2019; Bernal and Paolieri, 2022). A recent study demonstrates that the menstrual cycle effect on color Stroop task performance is modulated by the baseline dopamine levels (Hidalgo-Lopez and Pletzer, 2017). Their following research used the stop-signal fMRI task to measure inhibitory control and associated brain activity, indicating the baseline inhibitory control might also be a potential modulating variable (Hidalgo-Lopez and Pletzer, 2019). In addition, the recent review proposes that it is crucial to consider modulating factors to avoid confounding findings (Bernal and Paolieri, 2022). Those pieces of evidence, along with us, advocate research attention to potential modulating factors that might change the direction or strength of the menstrual cycle effect.
It is noteworthy that the mid-luteal phase advantage was eliminated in the high workload group on both the error rate measure of the spatial Stroop task and the reaction time measure of the divided attention task. These preliminary findings imply that work-related stress might offset the protective effect of ovarian steroids. Previous studies have suggested that hormones from the HPA axis are involved in regulating the HPG axis at different levels (Oyola and Handa, 2017). The HPA axis is the coordinator of the brain’s fight-or-flight response, which increases cortisol production to deal with stressful events. A recent study indicates that the hair cortisol concertation predicts work-related stress only in the high workload condition but not in the normal workload condition (van der Meij et al., 2018). The increased perceived workload in the high workload group did not affect emotion yet as we did not find the workload effect on the DASS scores. We failed to detect the workload effect on emotion, but “cold” prefrontal-mediated tasks might be due to the rating scale’s insensitivity. Another explanation is a complex interaction among the HPA axis, HPG axis, and the prefrontal network underneath women’s cycling cognitive and affective performance. Our preliminary findings advocate future research efforts to tease apart the potential dynamics among the “cold”/“hot” brain systems and the neuroendocrine system.
Contrary to our expectations, we did not find an effect of workload and menstrual cycle on working memory. Visual working memory is essential for cognitive performance (Luck and Vogel, 2013). The present study estimates participants’ visual working memory capacity (K) for each test session using a multiple-change detection paradigm (Gold et al., 2019) and a computational model (Feuerstahler et al., 2019). However, this study did not reveal the workload or the menstrual cycle’s effect on the K index, consistent with a recent study using a single probe change detection paradigm (Wassell et al., 2015). Although Wassell et al. (2015) revealed that progesterone levels in the mid-luteal phase modulate mental imagery ability, but they failed to find any association between cycle phase, hormone concentration, and working memory performance.
Previous studies on the menstrual cycle effect primarily used verbal working memory tasks (Joseph et al., 2012; Hidalgo-Lopez and Pletzer, 2021). A recent study found enhanced frontal activity and disinhibition of the salience brain network and striatum in a verbal working memory task (letter N-back) during the luteal phase (Hidalgo-Lopez and Pletzer, 2021). Hampson and Morley (2013) suggest that estradiol, but not progesterone levels, is associated with spatial working memory performance using a sample of women of reproductive age. Their study implies that females might perform best during their follicular phase when the estradiol levels are high. However, using a working memory task for emotional expressions, Gasbarri et al. (2008) indicated that working memory is impaired in the follicular phase.
The inconsistent findings in the literature might be due to methodological differences. Another potential explanation might be complex interactions among the HPA axis, HPG axis, and neurotransmitter systems, such as the dopaminergic system. Previous studies have suggested an inverted U-shape relationship between dopamine concentration and prefrontal cortex mediated cognitive function, such as working memory and cognitive control (Cools and D’Esposito, 2011). Recent studies have found that the mid-luteal phase and progesterone levels drive the effects of dopamine and cycle interactions on cognitive control (Hidalgo-Lopez and Pletzer, 2017). The picture gets increasingly complex by considering another inverted U-shape association between workload and task performance (Ma et al., 2020). It is possible that complex interactions among progesterone, dopamine, and workload obscure the findings of this study. Alternatively, it may be due to other mechanisms, such as functional compensation in the brain. Although this study provides insights on potential intriguing modulating mechanisms, clarifying the exact mechanism is far from our reach. Increasing research efforts are necessary for the future.
This study used a validated backward-counting procedure to determine the late-follicular and mid-luteal phases. To further minimize the impact of menstrual cycle mapping error, we increase the sample sizes. As far as we know, few studies have a sample size bigger than us (n = 79) if they used the longitudinal design with a homogenous sample like us. In addition, we double-checked and excluded participants if their actual menses onset deviated from the normal range during the experiment. Despite this, we admit that it might comprise a potential limitation without saliva, urine, or blood test to verify the hormone levels.
Although our results indicate workload as a modulatory factor on the menstrual cycle’s effect on cognition, caution should be made that the evidence is preliminary. Future research is still necessary to replicate the role of workload with samples of females in other workplace settings. In addition, the workload is a too complex construct that might confound many other concepts. Moreover, participants in the present study rated their generally experienced workload in the past 3 months, not their workload at the moment. Thus, the current findings might not answer how acute work stress impacts the menstrual cycling effect. Future studies might use new research methodology, such as experience sampling (Bos et al., 2015) and wearable neurophysiological recordings (Yokota et al., 2017), to provide an objective and immediate measure of workload.
This study contributes a mini-computerized cognitive battery specifically designed to evaluate four cognitive skills critical for nursing performance. We make it publicly available to make replicative and collaborative research works possible. However, we need to emphasize that the tasks in the battery are only a tiny subset of cognitive assessment and may not capture the cognitive performance at work. We suggest that it is valuable to assess cognitive performance by tracking operational errors when nurses perform routine tasks in their workplace.
How the menstrual cycle impacts the cognitive performance of females in the workplace is less understood. The present study employed a sample of nurses with similar duties and tracked their cognitive performance during their menses, late-follicular, and mid-luteal phases. Our results demonstrate a general mid-luteal advantage in error rate measure of task-switching and sensitivity measure of divided attention. Moreover, the present study reveals preliminary evidence that workload modulates the menstrual cycle effect on cognition. Only females with low workload manifest the mid-luteal cognitive advantage on the reaction time measure of divided attention and the error rate measure of response inhibition, implying that a suitable workload threshold might be necessary for regular neuro-steroid interactions. Thus, this study advocates the significance of research focused on the brain cycle under workloads.
The datasets presented in this study can be found in online repositories. The names of the repository/repositories and accession number(s) can be found below: https://www.doi.org/10.11922/sciencedb.o00068.00001.
The studies involving human participants were reviewed and approved by the Hefei Cancer Hospital, Chinese Academy of Sciences. The patients/participants provided their written informed consent to participate in this study.
L-ZY, MX, HW, and HL designed and made the concept of the study and performed the analysis and interpretation of the data. L-ZY, HW, and HL contributed research tools. MX and DC were responsible for data acquisition. MX and L-ZY drafted the manuscript, which was critically revised and approved by all authors. All authors agreed to be accountable for all aspects of the work in ensuring that questions related to the accuracy or integrity of any part of the work are appropriately investigated and resolved.
This work was supported by the Natural Science Fund of Anhui Province (2008085MC69), the Natural Science Fund of Hefei City (2021033), the General scientific research project of Anhui Provincial Health Commission (AHWJ2021b150), Collaborative Innovation Program of Hefei Science Center, CAS (2020HSC-CIP001 and 2021HSC-CIP013), and Anhui Province Key Laboratory of Medical Physics and Technology (LMPT201904).
The authors declare that the research was conducted in the absence of any commercial or financial relationships that could be construed as a potential conflict of interest.
All claims expressed in this article are solely those of the authors and do not necessarily represent those of their affiliated organizations, or those of the publisher, the editors and the reviewers. Any product that may be evaluated in this article, or claim that may be made by its manufacturer, is not guaranteed or endorsed by the publisher.
We appreciate our participants very much for their patience and kindness during the research. We appreciate Liu-Yang Kuang and Fei-Yan Chen for their generous help in data acquisition. We also acknowledge Jin-Wei Lang, Zheng-Lin Zhang, Jin Liu, and Zheng-Kang Zhang for their invaluable technique support in developing the mini-computerized cognitive battery.
The Supplementary Material for this article can be found online at: https://www.frontiersin.org/articles/10.3389/fnbeh.2022.856276/full#supplementary-material
Aidman, E., Jackson, S. A., and Kleitman, S. (2019). Effects of sleep deprivation on executive functioning, cognitive abilities, metacognitive confidence, and decision making. Appl. Cogn. Psych. 33, 188–200. doi: 10.1002/acp.3463
Alghamdi, M. G. (2016). Nursing workload: a concept analysis. J. Nurs. Manag. 24, 449–457. doi: 10.1111/jonm.12354
Barth, C., Steele, C. J., Mueller, K., Rekkas, V. P., Arelin, K., Pampel, A., et al. (2016). In-vivo dynamics of the human hippocampus across the menstrual cycle. Sci. Rep. 6:32833. doi: 10.1038/srep32833
Barth, C., Villringer, A., and Sacher, J. (2015). Sex hormones affect neurotransmitters and shape the adult female brain during hormonal transition periods. Front. Neurosci. 9:37. doi: 10.3389/fnins.2015.00037
Bates, D., Machler, M., Bolker, B. M., and Walker, S. C. (2015). Fitting linear mixed-effects models using lme4. J. Stat. Softw. 67, 1–48. doi: 10.18637/jss.v067.i01
Baudry, M., Bi, X., and Aguirre, C. (2013). Progesterone-estrogen interactions in synaptic plasticity and neuroprotection. Neuroscience 239, 280–294. doi: 10.1016/j.neuroscience.2012.10.051
Beltz, A. M., and Moser, J. S. (2020). Ovarian hormones: a long overlooked but critical contributor to cognitive brain structures and function. Ann. N. Y. Acad. Sci. 1464, 156–180. doi: 10.1111/nyas.14255
Bernal, A., and Paolieri, D. (2022). The influence of estradiol and progesterone on neurocognition during three phases of the menstrual cycle: modulating factors. Behav. Brain Res. 417:113593. doi: 10.1016/j.bbr.2021.113593
Borghini, G., Astolfi, L., Vecchiato, G., Mattia, D., and Babiloni, F. (2014). Measuring neurophysiological signals in aircraft pilots and car drivers for the assessment of mental workload, fatigue and drowsiness. Neurosci. Biobehav. Rev. 44, 58–75. doi: 10.1016/j.neubiorev.2012.10.003
Bos, F. M., Schoevers, R. A., and Aan het Rot, M. (2015). Experience sampling and ecological momentary assessment studies in psychopharmacology: a systematic review. Eur. Neuropsychopharmacol. 25, 1853–1864. doi: 10.1016/j.euroneuro.2015.08.008
Brotzner, C. P., Klimesch, W., and Kerschbaum, H. H. (2015). Progesterone-associated increase in ERP amplitude correlates with an improvement in performance in a spatial attention paradigm. Brain Res. 1595, 74–83. doi: 10.1016/j.brainres.2014.11.004
Carroll, J. C., Rosario, E. R., and Pike, C. J. (2008). Progesterone blocks estrogen neuroprotection from kainate in middle-aged female rats. Neurosci. Lett. 445, 229–232. doi: 10.1016/j.neulet.2008.09.010
Catenaccio, E., Mu, W., and Lipton, M. L. (2016). Estrogen- and progesterone-mediated structural neuroplasticity in women: evidence from neuroimaging. Brain Struct. Funct. 221, 3845–3867. doi: 10.1007/s00429-016-1197-x
Cools, R., and D’Esposito, M. (2011). Inverted-U-shaped dopamine actions on human working memory and cognitive control. Biol. Psychiatry 69, e113–e125. doi: 10.1016/j.biopsych.2011.03.028
Diekhof, E. K., and Ratnayake, M. (2016). Menstrual cycle phase modulates reward sensitivity and performance monitoring in young women: preliminary fMRI evidence. Neuropsychologia 84, 70–80. doi: 10.1016/j.neuropsychologia.2015.10.016
Dubol, M., Epperson, C. N., Sacher, J., Pletzer, B., Derntl, B., Lanzenberger, R., et al. (2021). Neuroimaging the menstrual cycle: a multimodal systematic review. Front. Neuroendocrinol. 60:100878. doi: 10.1016/j.yfrne.2020.100878
Durante, K. M., Griskevicius, V., Cantú, S. M., and Simpson, J. A. (2014). Money, status, and the ovulatory cycle. J. Market. Res. 51, 27–39. doi: 10.1509/jmr.11.0327
Feuerstahler, L. M., Luck, S. J., MacDonald, A. III, and Waller, N. G. (2019). A note on the identification of change detection task models to measure storage capacity and attention in visual working memory. Behav. Res. Methods 51, 1360–1370. doi: 10.3758/s13428-018-1082-z
Gasbarri, A., Pompili, A., d’Onofrio, A., Cifariello, A., Tavares, M. C., and Tomaz, C. (2008). Working memory for emotional facial expressions: role of the estrogen in young women. Psychoneuroendocrinology 33, 964–972. doi: 10.1016/j.psyneuen.2008.04.007
Girard, R., Metereau, E., Thomas, J., Pugeat, M., Qu, C., and Dreher, J. C. (2017). Hormone therapy at early post-menopause increases cognitive control-related prefrontal activity. Sci. Rep. 7:44917. doi: 10.1038/srep44917
Gold, J. M., Barch, D. M., Feuerstahler, L. M., Carter, C. S., MacDonald, A. W., Ragland, J. D., et al. (2019). Working memory impairment across psychotic disorders. Schizophr. Bull. 45, 804–812. doi: 10.1093/schbul/sby134
Hampson, E., and Morley, E. E. (2013). Estradiol concentrations and working memory performance in women of reproductive age. Psychoneuroendocrinology 38, 2897–2904. doi: 10.1016/j.psyneuen.2013.07.020
Hart, S. G. (2016). Nasa-Task load index (NASA-TLX); 20 years later. Proceed. Hum. Fact. Ergon. Soc. Annu. Meeting 50, 904–908. doi: 10.1177/154193120605000909
Hatta, T., and Nagaya, K. (2009). Menstrual cycle phase effects on memory and Stroop task performance. Arch. Sex Behav. 38, 821–827. doi: 10.1007/s10508-008-9445-7
Henry, J. D., and Crawford, J. R. (2005). The short-form version of the Depression Anxiety Stress Scales (DASS-21): construct validity and normative data in a large non-clinical sample. Br. J. Clin. Psychol. 44(Pt 2), 227–239. doi: 10.1348/014466505X29657
Hidalgo-Lopez, E., and Pletzer, B. (2017). Interactive effects of dopamine baseline levels and cycle phase on executive functions: the role of progesterone. Front. Neurosci. 11:403. doi: 10.3389/fnins.2017.00403
Hidalgo-Lopez, E., and Pletzer, B. (2019). Individual differences in the effect of menstrual cycle on basal ganglia inhibitory control. Sci. Rep. 9:11063. doi: 10.1038/s41598-019-47426-8
Hidalgo-Lopez, E., and Pletzer, B. (2021). Fronto-striatal changes along the menstrual cycle during working memory: effect of sex hormones on activation and connectivity patterns. Psychoneuroendocrinology 125:105108. doi: 10.1016/j.psyneuen.2020.105108
Himi, S. A., Buhner, M., Schwaighofer, M., Klapetek, A., and Hilbert, S. (2019). Multitasking behavior and its related constructs: executive functions, working memory capacity, relational integration, and divided attention. Cognition 189, 275–298. doi: 10.1016/j.cognition.2019.04.010
Hussain, D., Hanafi, S., Konishi, K., Brake, W. G., and Bohbot, V. D. (2016). Modulation of spatial and response strategies by phase of the menstrual cycle in women tested in a virtual navigation task. Psychoneuroendocrinology 70, 108–117. doi: 10.1016/j.psyneuen.2016.05.008
Ikarashi, K., Sato, D., Iguchi, K., Baba, Y., and Yamashiro, K. (2020). Menstrual cycle modulates motor learning and memory consolidation in humans. Brain Sci. 10:696. doi: 10.3390/brainsci10100696
Jacobs, E., and D’Esposito, M. (2011). Estrogen shapes dopamine-dependent cognitive processes: implications for women’s health. J. Neurosci. 31, 5286–5293. doi: 10.1523/JNEUROSCI.6394-10.2011
Joseph, J. E., Swearingen, J. E., Corbly, C. R., Curry, T. E. Jr., and Kelly, T. H. (2012). Influence of estradiol on functional brain organization for working memory. Neuroimage 59, 2923–2931. doi: 10.1016/j.neuroimage.2011.09.067
Le, J., Thomas, N., and Gurvich, C. (2020). Cognition, the menstrual cycle, and premenstrual disorders: a review. Brain Sci. 10:198. doi: 10.3390/brainsci10040198
Leeners, B., Kruger, T. H. C., Geraedts, K., Tronci, E., Mancini, T., Ille, F., et al. (2017). Lack of associations between female hormone levels and visuospatial working memory. divided attention and cognitive bias across two consecutive menstrual cycles. Front. Behav. Neurosci. 11:120. doi: 10.3389/fnbeh.2017.00120
Lisofsky, N., Martensson, J., Eckert, A., Lindenberger, U., Gallinat, J., and Kuhn, S. (2015). Hippocampal volume and functional connectivity changes during the female menstrual cycle. Neuroimage 118, 154–162. doi: 10.1016/j.neuroimage.2015.06.012
Luck, S. J., and Vogel, E. K. (2013). Visual working memory capacity: from psychophysics and neurobiology to individual differences. Trends Cogn. Sci. 17, 391–400. doi: 10.1016/j.tics.2013.06.006
Ma, S., Zhang, W., Yang, Z., Kang, C., Wu, C., Chai, C., et al. (2020). Promote or inhibit: an inverted U-shaped effect of workload on driver takeover performance. Traffic Inj. Prev. 21, 482–487. doi: 10.1080/15389588.2020.1804060
Monsell, S. (2003). Task switching. Trends Cogn. Sci. 7, 134–140. doi: 10.1016/s1364-6613(03)00028-7
Morrison, J. H., Brinton, R. D., Schmidt, P. J., and Gore, A. C. (2006). Estrogen, menopause, and the aging brain: how basic neuroscience can inform hormone therapy in women. J. Neurosci. 26, 10332–10348. doi: 10.1523/JNEUROSCI.3369-06.2006
Muff, S., Nilsen, E. B., O’Hara, R. B., and Nater, C. R. (2022). Rewriting results sections in the language of evidence. Trends Ecol. Evol. 37, 203–210. doi: 10.1016/j.tree.2021.10.009
Oyola, M. G., and Handa, R. J. (2017). Hypothalamic-pituitary-adrenal and hypothalamic-pituitary-gonadal axes: sex differences in regulation of stress responsivity. Stress 20, 476–494. doi: 10.1080/10253890.2017.1369523
Pelli, D. G. (1997). The VideoToolbox software for visual psychophysics: transforming numbers into movies. Spat .Vis. 10, 437–442.
Pires, L., Leitao, J., Guerrini, C., and Simoes, M. R. (2018). Cognitive control during a spatial Stroop task: comparing conflict monitoring and prediction of response-outcome theories. Acta Psychol. 189, 63–75. doi: 10.1016/j.actpsy.2017.06.009
Pletzer, B., Harris, T. A., and Ortner, T. (2017). Sex and menstrual cycle influences on three aspects of attention. Physiol. Behav. 179, 384–390. doi: 10.1016/j.physbeh.2017.07.012
Pletzer, B., Harris, T. A., Scheuringer, A., and Hidalgo-Lopez, E. (2019). The cycling brain: menstrual cycle related fluctuations in hippocampal and fronto-striatal activation and connectivity during cognitive tasks. Neuropsychopharmacology 44, 1867–1875. doi: 10.1038/s41386-019-0435-3
Pletzer, B., Harris, T., and Hidalgo-Lopez, E. (2018). Subcortical structural changes along the menstrual cycle: beyond the hippocampus. Sci. Rep. 8:16042. doi: 10.1038/s41598-018-34247-4
Potter, P., Wolf, L., Boxerman, S., Grayson, D., Sledge, J., Dunagan, C., et al. (2005). Understanding the cognitive work of nursing in the acute care environment. J. Nurs. Adm. 35, 327–335.
R Core Team (2021). R: A Language And Environment For Statisticalcomputing. Vienna: R Foundation for Statistical Computing.
Rosario, E. R., Ramsden, M., and Pike, C. J. (2006). Progestins inhibit the neuroprotective effects of estrogen in rat hippocampus. Brain Res. 1099, 206–210. doi: 10.1016/j.brainres.2006.03.127
Schaumberg, M. A., Jenkins, D. G., Janse de Jonge, X. A. K., Emmerton, L. M., and Skinner, T. L. (2017). Three-step method for menstrual and oral contraceptive cycle verification. J. Sci. Med. Sport 20, 965–969. doi: 10.1016/j.jsams.2016.08.013
Scheuringer, A., and Pletzer, B. (2017). Sex differences and menstrual cycle dependent changes in cognitive strategies during spatial navigation and verbal fluency. Front. Psychol. 8:381. doi: 10.3389/fpsyg.2017.00381
Shen, Y., Jian, W., Zhu, Q., Li, W., Shang, W., and Yao, L. (2020). Nurse staffing in large general hospitals in China: an observational study. Hum. Resour. Health 18:3. doi: 10.1186/s12960-020-0446-5
Singmann, H., Bolker, B., Westfall, J., and Aust, F. (2021). Afex: Analysis of Factorial Experiments”. R Package Version 1.0.
Solis-Ortiz, S., and Corsi-Cabrera, M. (2008). Sustained attention is favored by progesterone during early luteal phase and visuo-spatial memory by estrogens during ovulatory phase in young women. Psychoneuroendocrinology 33, 989–998. doi: 10.1016/j.psyneuen.2008.04.003
Solis-Ortiz, S., Guevara, M. A., and Corsi-Cabrera, M. (2004). Performance in a test demanding prefrontal functions is favored by early luteal phase progesterone: an electroencephalographic study. Psychoneuroendocrinology 29, 1047–1057. doi: 10.1016/j.psyneuen.2003.10.007
Stanislaw, H., and Todorov, N. (1999). Calculation of signal detection theory measures. Behav. Res. Methods Instrum. Comput. 31, 137–149. doi: 10.3758/bf03207704
Sundstrom-Poromaa, I. (2018). The menstrual cycle influences emotion but has limited effect on cognitive function. Vitam Horm. 107, 349–376. doi: 10.1016/bs.vh.2018.01.016
Sundstrom-Poromaa, I., and Gingnell, M. (2014). Menstrual cycle influence on cognitive function and emotion processing-from a reproductive perspective. Front. Neurosci. 8:380. doi: 10.3389/fnins.2014.00380
Thimm, M., Weis, S., Hausmann, M., and Sturm, W. (2014). Menstrual cycle effects on selective attention and its underlying cortical networks. Neuroscience 258, 307–317. doi: 10.1016/j.neuroscience.2013.11.010
Tubbs-Cooley, H. L., Mara, C. A., Carle, A. C., and Gurses, A. P. (2018). The NASA Task Load Index as a measure of overall workload among neonatal, paediatric and adult intensive care nurses. Intensive Crit. Care Nurs. 46, 64–69. doi: 10.1016/j.iccn.2018.01.004
van der Meij, L., Gubbels, N., Schaveling, J., Almela, M., and van Vugt, M. (2018). Hair cortisol and work stress: importance of workload and stress model (JDCS or ERI). Psychoneuroendocrinology 89, 78–85. doi: 10.1016/j.psyneuen.2017.12.020
Wang, J. X., Zhuang, J. Y., Fu, L., and Lei, Q. (2021). Social orientation in the luteal phase: increased social feedback sensitivity. Inhibitory response, interpersonal anxiety and cooperation preference. Evol. Psychol. 19:1474704920986866. doi: 10.1177/1474704920986866
Wang, J. X., Zhuang, J. Y., Fu, L., Lei, Q., Fan, M., and Zhang, W. (2020a). How ovarian hormones influence the behavioral activation and inhibition system through the dopamine pathway. PLoS One 15:e0237032. doi: 10.1371/journal.pone.0237032
Wang, J. X., Zhuang, J. Y., Fu, L., Lei, Q., and Zhang, W. (2020b). Association of ovarian hormones with mapping concept of self and others in the brain’s default mode network. Neuroreport 31, 717–723. doi: 10.1097/WNR.0000000000001477
Wang, J., and Chen, A. (2020). High progesterone levels facilitate women’s social information processing by optimizing attention allocation. Psychoneuroendocrinology 122:104882. doi: 10.1016/j.psyneuen.2020.104882
Wassell, J., Rogers, S. L., Felmingam, K. L., Bryant, R. A., and Pearson, J. (2015). Sex hormones predict the sensory strength and vividness of mental imagery. Biol. Psychol. 107, 61–68. doi: 10.1016/j.biopsycho.2015.02.003
Westbrook, J. I., Woods, A., Rob, M. I., Dunsmuir, W. T., and Day, R. O. (2010). Association of interruptions with an increased risk and severity of medication administration errors. Arch. Intern. Med. 170, 683–690. doi: 10.1001/archinternmed.2010.65
Wickens, C. D. (2008). Multiple resources and mental workload. Hum. Fact. 50, 449–455. doi: 10.1518/001872008X288394
World Health Organization (2020). The State of The World’s Nursing 2020: Investing In Education, Jobs And Leadership. Available online at: https://www.who.int/publications/i/item/9789240003279 (accessed April 6, 2020).
Ycaza Herrera, A., Wang, J., and Mather, M. (2019). The gist and details of sex differences in cognition and the brain: how parallels in sex differences across domains are shaped by the locus coeruleus and catecholamine systems. Prog. Neurobiol. 176, 120–133. doi: 10.1016/j.pneurobio.2018.05.005
Yokota, Y., Tanaka, S., Miyamoto, A., and Naruse, Y. (2017). Estimation of human workload from the auditory steady-state response recorded via a wearable electroencephalography system during walking. Front. Hum. Neurosci. 11:314. doi: 10.3389/fnhum.2017.00314
Zhuang, J. Y., and Wang, J. X. (2014). Women ornament themselves for intrasexual competition near ovulation, but for intersexual attraction in luteal phase. PLoS One 9:e106407. doi: 10.1371/journal.pone.0106407
Keywords: menstrual cycle, workload, cognitive flexibility, inhibitory control, divided attention
Citation: Xu M, Chen D, Li H, Wang H and Yang L-Z (2022) The Cycling Brain in the Workplace: Does Workload Modulate the Menstrual Cycle Effect on Cognition? Front. Behav. Neurosci. 16:856276. doi: 10.3389/fnbeh.2022.856276
Received: 17 January 2022; Accepted: 06 May 2022;
Published: 02 June 2022.
Edited by:
Jaclyn M. Schwarz, University of Delaware, United StatesReviewed by:
Caroline Gurvich, Monash University, AustraliaCopyright © 2022 Xu, Chen, Li, Wang and Yang. This is an open-access article distributed under the terms of the Creative Commons Attribution License (CC BY). The use, distribution or reproduction in other forums is permitted, provided the original author(s) and the copyright owner(s) are credited and that the original publication in this journal is cited, in accordance with accepted academic practice. No use, distribution or reproduction is permitted which does not comply with these terms.
*Correspondence: Hongzhi Wang, d2FuZ2h6QGhmY2FzLmFjLmNu; Li-Zhuang Yang, bHp5YW5nQHVzdGMuZWR1LmNu
Disclaimer: All claims expressed in this article are solely those of the authors and do not necessarily represent those of their affiliated organizations, or those of the publisher, the editors and the reviewers. Any product that may be evaluated in this article or claim that may be made by its manufacturer is not guaranteed or endorsed by the publisher.
Research integrity at Frontiers
Learn more about the work of our research integrity team to safeguard the quality of each article we publish.