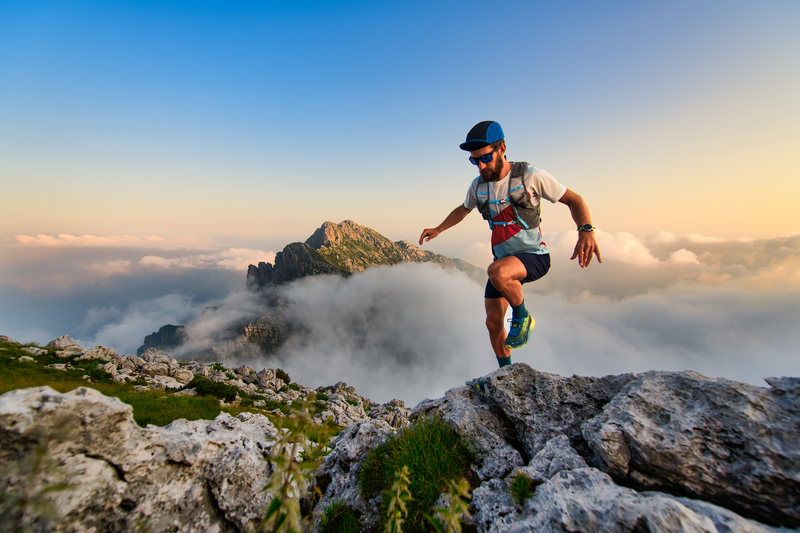
95% of researchers rate our articles as excellent or good
Learn more about the work of our research integrity team to safeguard the quality of each article we publish.
Find out more
REVIEW article
Front. Behav. Neurosci. , 11 January 2023
Sec. Learning and Memory
Volume 16 - 2022 | https://doi.org/10.3389/fnbeh.2022.1068271
This article is part of the Research Topic Dopaminergic Control of Experience Encoding, Memory and Cognition View all 9 articles
Mnemonic functions, supporting rodent behavior in complex tasks, include both long-term and (short-term) working memory components. While working memory is thought to rely on persistent activity states in an active neural network, long-term memory and synaptic plasticity contribute to the formation of the underlying synaptic structure, determining the range of possible states. Whereas, the implication of working memory in executive functions, mediated by the prefrontal cortex (PFC) in primates and rodents, has been extensively studied, the contribution of long-term memory component to these tasks received little attention. This review summarizes available experimental data and theoretical work concerning cellular mechanisms of synaptic plasticity in the medial region of rodent PFC and the link between plasticity, memory and behavior in PFC-dependent tasks. A special attention is devoted to unique properties of dopaminergic modulation of prefrontal synaptic plasticity and its contribution to executive functions.
Any complex purposeful behavior relies on both online control processes and a long-term representation of related previous experience, including its spatio-temporal context and associated goals, strategies or rules. Mnemonic functions supporting these two aspects of executive control can be separated on the basis of relevant time scales and underlying neural mechanisms (Atkinson and Shiffrin, 1971; Cowan, 2008). Online control processes, including, e.g., decision making, conflict monitoring, and active maintenance of goals or rules, rely on working memory, lasting from seconds to minutes and thought to be supported by reverberatory population activity, bistability in single cells or short-term synaptic changes (Durstewitz et al., 2000; Mongillo et al., 2008). In contrast, long-term storage of contextual representations is implemented by synaptic changes that last from tens of minutes to years and involve increases in receptor density, protein synthesis and structural changes at the synaptic site (Davis and Squire, 1984; Bliss and Collingridge, 1993; Yuste and Bonhoeffer, 2001).
PFC is a central structure mediating executive functions (Gilbert and Burgess, 2008) and neuronal mechanisms supporting these functions can also be studied from the processing or representational perspectives (Wood and Grafman, 2003). The discovery of cells with “memory fields” in the primate dorsolateral PFC (Fuster and Alexander, 1971; Goldman-Rakic, 1995), which fired with maximal rates when a stimulus presented at a specific location of the visual field was held in memory during a delay, stimulated an extensive study of the role of working memory in executive functions over the last few decades (Arnsten, 2013). This research led to a considerable progress in our understanding of neuronal mechanisms underlying online control of behavior and their possible implication in related mental disorders (Durstewitz et al., 2000; O'Reilly and Frank, 2006; Rolls et al., 2008). In contrast, the involvement of prefrontal long-term memory in executive functions received relatively little attention. While a general implication of PFC in memory has been demonstrated in different species in various experimental paradigms, in what ways the prefrontal long-term plasticity can be directly involved in the control of behavior is a matter of debate (Blumenfeld and Ranganath, 2007; Euston et al., 2012). The main questions that can be asked in relation to this involvement are:
• What aspects of executive function rely on prefrontal long-term memory?
• What molecular and neuronal mechanisms support such a long-term memory storage?
While the main issue at stake concerns the role of intrinsic prefrontal memory storage mechanisms in human executive functions, a direct experimental study of these questions in primates is difficult. This difficulty stems from the fact that even when PFC neurons are shown to encode task-related information using single cell recordings or neuroimaging methods, it is hard to prove that such information is stored in the PFC and not in other areas with which it is connected. A case in point is the delay-period activity of primate prefrontal cells mentioned above: even though these cells have been studied for several decades now, it is not clear whether their activities are directly associated with remembered stimuli or represent top-down control signals to other (e.g., sensory) cortices that retain stimulus-specific information in memory (Lara and Wallis, 2015). It appears then that rodent models can be of help, since they are readily amenable to electrophysiological, pharmacological and genetic methods in order to test both the memory contents and its storage site. One difficulty of using rodents to understand primate executive functions is that no area in the rodent brain is anatomically homologous to the primate “granular” PFC (including the dorsolateral region), thought to support complex behavioral control (Wise, 2008; Carlén, 2017). However, a large body of research shows that rodent medial PFC (mPFC) mediates many functions attributed to the dorsolateral PFC in primates, suggesting that understanding neuronal mechanisms of rodent executive function, including its long-term memory component, can provide valuable insights into its organization in primates (Brown and Bowman, 2002; Dalley et al., 2004; Chudasama, 2011). In particular, synaptic plasticity research over the last decades provided a considerable amount of data describing in which behavioral tasks and under what experimental conditions prefrontal synapses undergo long-term changes. In parallel, theoretical models of long-term plasticity involvement in behavioral control, in PFC as well as in other areas, and at various levels of physiological detail, suggested ways in which plasticity can be linked with behavior. By unifying available experimental and theoretical evidence, this review attempts to address the above questions from the synaptic plasticity perspective.
The first part of this review describes pharmacological, electrophysiological and behavioral data supporting a direct link between long-term memory and synaptic plasticity in rodents, in experimental paradigms that were shown to depend on the integrity of mPFC. The second part presents a detailed account of prefrontal synaptic plasticity mechanisms, in vivo and in vitro, in mice and rats, demonstrating that neurons in this structure readily exhibit plastic changes in a variety of experimental protocols. A well-known property of neural functioning in mPFC is that it is strongly modulated by the neuromodulator dopamine, to the extent that it is hardly possible to study prefrontal synaptic plasticity and memory without taking into account dopamine involvement (Jay, 2003; Otani, 2004). The third part of the review is therefore devoted to the properties of dopaminergic modulation of prefrontal long-term plasticity. The fourth part compares theoretical models of synaptic plasticity modulated by dopamine in the mPFC, the striatum and the hippocampus, areas that are often co-involved with mPFC in behavioral tasks that tax executive functions (Floresco et al., 1997; Kesner and Rogers, 2004). The review is concluded by attempting to provide answers to the two questions above in the context of executive functions research in rodents.
In an influential review, Wood and Grafman (2003) classified existing models of PFC according to whether they adopt a processing or a representational approach to describe the functional role of this structure in cognition. The processing approach attempts to characterize algorithmic procedures (e.g., selection of goals and rules to achieve them) governing the active control of behavior. These procedures are usually considered to be independent of the nature of the stimuli they operate with, so that knowing what is stored in prefrontal long-term memory is not essential for understanding PFC function from this point of view. In contrast, the representational approach focuses on the type information stored by the PFC, as it is this information that distinguishes it from other cortical structures and defines its functional significance (as, e.g., memory about objects and faces is an essential function of the inferior temporal cortex). The authors argue that the representational approach is more general (since the characterization of the kind of processes that PFC stores fits it), more in line with evolutionary history of PFC and its anatomical properties (see also Wise, 2008) and potentially more fruitful in generating experimentally testable predictions. This work is one of the first to highlight long-term memory as an important issue in prefrontal research. Another line of evidence supporting the important role of PFC, and in particular of its medial region, in long-term memory comes from studies of memory consolidation (see Frankland and Bontempi, 2005, for review). Based on the combined imaging and inactivation methods in an animal model of retrograde amnesia, it is proposed that mPFC plays an essential integrative role in storage and recall of remote memories, complementing the hippocampus that is thought to store primarily recent ones. More recently, Euston et al. (2012) reviewed evidence of mPFC involvement in the processing of both recent and remote memories and proposed that its primary role is to learn associations between the context (including location and events) and adaptive responses.
The direct involvement of mPFC in the storage of recent and remote memories is supported by evidence showing that manipulation of specific molecular targets disrupts memory in this and nearby cortices. In particular, blockade of muscarinic and N-Methyl-D-aspartate (NMDA) receptors in the prelimbic mPFC immediately after learning an odor-reward association induced severe memory impairment 1 or 2 days later (Tronel and Sara, 2003; Carballo-Márquez et al., 2007). Protein synthesis blockade with anisomycin (as well as NMDA receptor disruption) in the same region blocked consolidation of object recognition memory 1 day after learning (Akirav and Maroun, 2006). In the nearby orbitofrontal cortex, disruption of the synapic plasticity cascade mediated by mitogen-activated protein kinases (MAPK) and extracellular signal-regulated kinases (ERK) blocked remote olfactory memory and abolished the late development of cortical structural plasticity (Lesburguères et al., 2011), whereas in the frontal association cortex learning and extinction caused elimination and formation of dendritic spines at the same dendritic branches (Lai et al., 2012). Cortical storage of remote taste memory has been associated with protein kinase M zeta (Shema et al., 2007), whereas remote fear memory was blocked in α-CaMKII+\− mice, in which cortical, but not hippocampal, synaptic plasticity is impaired (Frankland et al., 2001, 2004).
The above data strongly suggest that mPFC, similarly to nearby cortical areas, directly participates in associative long-term memory, at least in rodents. However, in which way this memory supports flexible control of behavior is less clear. Rodent studies of behavioral flexibility, a term that is often used to describe the kind of cognitive functions referred to as “executive” in human and primate literature, can be separated into four main classes according to whether they test strategy selection (or set-shifting), extinction learning, delay-period working memory or decision making (Figure 1). In all these paradigms, (i) Successful performance depends on mPFC integrity and requires task acquisition across hours/days; (ii) Task learning is associated with neuronal activity changes and synaptic plasticity within mPFC; and (iii) Experimental manipulations of synaptic plasticity during or after learning perturbs behavioral performance. These properties make it possible to study which aspects of flexible behavior rely on long-term synaptic plasticity within mPFC.
Figure 1. Typical experimental paradigms in which performance depends on synaptic plasticity in the mPFC. (A) Strategy selection. Rodents are trained to switch between place and response tasks, following a switch of reward contingency (Rich and Shapiro, 2007). In the place task, animals are rewarded if they reach the same arm (e.g., N) starting from any other arm. In the response task, reward is given if animals perform the same body turn at the intersection point. Importantly, the contingency switch is not signalled by any external cue, the animals have to infer it via trial-and-error. In a set-shifting version of the task (Birrell and Brown, 2000), animals switch between decisions based on different types of cue (e.g., odor vs. texture), instead of spatial strategy. (B) Fear extinction. During a conditioning period, animals associate a neutral cue, e.g., a sound tone, with a footshock, so that they acquire a freezing response to the cue (Herry and Garcia, 2002). During an extinction period the cue is repeatedly presented without the footshock, so that animals “learn to forget” the freezing response. In a contextual fear conditioning, the fear response is associated with environmental features (e.g., its visual appearance) rather than a discrete cue (Bouton et al., 2011). (C) Delay-period working memory. In an 8-arm maze, animals retrieve a reward (black dot) from a single open arm (forced choice 1) (Touzani et al., 2007). After a delay, the same arm is open together with an adjacent one and the animal has to choose the non-visited arm to obtain the reward (choice run 1). In a more complicated version of the task, a second forced run is inserted during the delay so that the animal has to keep in memory the two arms and use this information after the corresponding delay. (D) Decision making. During a training phase, animals learn to press on either of the two levers to obtain distinct rewards associated with them (e.g., a food pellet or sucrose solution, Corbit and Balleine, 2003). During the following devaluation phase, the animals are fed to satiety on one of the two reward types. During test, no reward is given and the preponderance of the animals to press on either lever is measured. Goal-directed behavior corresponds to a less frequent use of the lever associated with the devaluated reward. In a more complex version of the task, one employs contingency degradation instead of reward devaluation. In that case, the animal is given a particular reward irrespective of whether it presses on the associated lever or not, leading to a less frequent use of this lever in normal animals.
In the spatial strategy-selection paradigm or its non-spatial version referred to as set-shifting, animals are trained to flexibly switch from one behavioral strategy to another following a sudden change in the stimulus-reward contingency (de Bruin et al., 1994; Ragozzino et al., 1999; Birrell and Brown, 2000; Floresco et al., 2006; Rich and Shapiro, 2007). These paradigms were developed specifically as adaptations of cognitive flexibility studies in primates (such as the Wisconsin Card Sorting Task and its monkey analogs, Berg, 1948; Mansouri et al., 2006) to rodents (Ragozzino et al., 1999; Bissonette et al., 2013). In the spatial version of the task (Figure 1A), animals are initially rewarded when they follow a “place-based” strategy, approaching the same unmarked allocentric location in the maze from different starting positions. Once this strategy is successfully acquired, the experimenter switches (unknown to the animal) the reward contingency so that animals are now rewarded when they follow a different, “response-based” strategy. Here, the animals are rewarded when they make decisions based on immediately perceived cues, for example, “once at the center of the maze, turn left,” or “go to the arm with a yellow landmark at the end.” While learning of the strategies themselves is not impaired by mPFC inactivation, the switching between those strategies is (Ragozzino et al., 1999; White and McDonald, 2002). In set-shifting, animals first learn to choose one of two feeding bowls to dig for food, based on a particular type of perceptual cue (e.g., the odor of the digging medium). After the contingency switch, they have to make choices based on a different type of cue, e.g., the texture of the bowl surface. mPFC lesions selectively impair shifting of the perceptual set, but not its acquisition (Birrell and Brown, 2000). In these tasks, learning is associated with neural activity changes in mPFC (Rich and Shapiro, 2009; Durstewitz et al., 2010; Singh et al., 2019) and with plasticity-related gene expression in mPFC (DeSteno and Schmauss, 2008; Burnham et al., 2010). The main learning components of these tasks are the selection of a new behavioral strategy and inhibition of a previously acquired one, following a contingency switch (Dalley et al., 2004). Different mPFC subregions are differentially involved in mediating these components, depending on the nature of the task (Kesner, 2000; Floresco et al., 2009; Bissonette et al., 2013).
Extinction learning can be considered as a type of behavioral flexibility, in which a previously acquired emotional response (e.g., footshock-induced freezing) to a discrete stimulus (e.g., a sound) or to a contextual cue (e.g., an environment) is learned to be inhibited (Morgan and LeDoux, 1995; Quirk and Mueller, 2008; Bouton et al., 2011; Gass et al., 2014) (Figure 1B). In primates, fear extinction studies are motivated by the general question of emotional regulation of behavior and, in particular, by the role of stress in the development of many psychiatric disorders, including post-traumatic stress disorder (Sotres-Bayon et al., 2006; Maren and Holmes, 2016). In early studies of the role of mnemonic processes in fear conditioning, synaptic changes in hippocampal-mPFC synapses were shown to occur during the acquisition of an associative task using tone-shock pairings (Doyère et al., 1993). Moreover, an increase in plasticity-related immediate-early gene expression (c-fos) in mPFC during a similar task was reported, while its suppression was found to produce learning deficits (Morrow et al., 1999). However, subsequent investigations revealed the key role of the basolateral amygdala in associating sensory and shock-related inputs (Quirk and Mueller, 2008) and a hippocampal involvement in the reinstatement of extinguished fear (Frohardt et al., 2000; Short et al., 2022), whereas mPFC was shown to mediate primarily the consolidation of extinction. More specifically, the learning to inhibit a fear response was shown to be associated with synaptic changes in mPFC, as tested by an electric stimulation of medio-dorsal thalamus or ventral hippocampus synapses to mPFC neurons (Milad and Quirk, 2002; Quirk et al., 2006; Hugues and Garcia, 2007). Moreover, behavioral extinction learning was sped up or slowed down by an electric stimulation of monosynaptic thalamus-mPFC projections (Herry and Garcia, 2002). Finally, local pharmacological block of NMDA receptors, MAPK/ERK pathway or protein synthesis prevented long-term extinction (Runyan, 2004; Santini, 2004; Hugues et al., 2006; Burgos-Robles et al., 2007; Mamiya et al., 2009) and inhibitory avoidance (Zhang et al., 2011). Potentially different mPFC subregions learn different components of fear response (i.e., expression of fear response vs. inhibition of fear response) similarly to what has been proposed for set-shifting (Quirk and Mueller, 2008).
In a delay-period working memory paradigm, adapted from the behavioral paradigm of the same name in primates (Arnsten, 2013), rodents retain in memory a trial-unique spatial information from a training phase. During a test phase, that starts after a delay, they are required to retrieve this information and use it to solve the task (Floresco et al., 1997; Horst and Laubach, 2009). The delay duration varies from 3 s to 30 min in different experiments in rodents, while in primate studies it is at most several seconds. While some lesion studies have shown that intact mPFC is necessary for successful performance in delay-period tasks (Horst and Laubach, 2009), others reported that mPFC is not needed for information storage during the delay and it is only required during spatial information retrieval and use for guiding prospective action (Floresco et al., 1997; Seamans et al., 1998; Gisquet-Verrier and Delatour, 2006). Neuronal activity dynamics in mPFC were shown to reflect learning during a delayed alternation task (see, e.g., Baeg et al., 2003) and in one study, optogenetic suppression of enhanced activity of pyramidal neurons in mouse mPFC impaired learning (Liu et al., 2014). It is not clear whether long-term plasticity within mPFC is involved in simple delay-period memory tasks, but in one study it was shown that a day-to-day improvement in delayed non-matching to sample task with retroactive interference, performance improvement required protein synthesis in mPFC (Touzani et al., 2007 see Figure 1C, see also Marighetto et al., 2008). In a related reference-memory experimental paradigm, early studies have shown that learning was associated with plasticity-related gene expression in hippocampal-prefrontal synapses (Davis et al., 1996; Laroche et al., 2000).
Finally, decision making is often tested in rodents using a reward devaluation or contingency degradation experimental paradigms (Corbit and Balleine, 2003; Killcross and Coutureau, 2003). These paradigms are based on the distinction between goal-directed and habitual behaviors, the former of which is mediated by mPFC in rodents and primates (Balleine and O'Doherty, 2010; Dolan and Dayan, 2013). During a goal-directed behavior, goal information is directly associated with actions (or chains of actions) leading to it, such that a change in the goal value or in the action-reward contingency induces changes in the corresponding behavior. In contrast, habitual behaviors prescribe action choices that are linked to the context or a cue associated with the goal, regardless of the internal motivation for the goal. Thus, if an animal solves a task in a goal-directed manner, reward devaluation (e.g., by feeding to satiety) should lead to a less probable choice of specific actions leading to it, reflecting the flexibility in the choice of actions (Figure 1D). However, if responses are habitual, rather than goal-directed, the actions leading to the now devaluated goal will be automatically repeated. Lesion studies support the implication of (prelimbic) mPFC in goal-directed behavior by showing a strong bias of lesioned animals toward habitual actions (Corbit and Balleine, 2003). Moreover, learning in decision making tasks occurs across several days (Killcross and Coutureau, 2003) and is associated with learning-related changes in response patterns of prefrontal neurons (Mulder et al., 2003). Lastly, the implication of synaptic plasticity mechanisms, intrinsic to mPFC, in goal-directed decision-making tasks was demonstrated by showing an increased expression of MAPK/ERK in mPFC following learning, as well as by the fact that local inhibition of this pathway prevented learning (Hart and Balleine, 2016). Moreover, augmentation of dendritic spine plasticity using cytoskeletal regulatory factor Rho kinase inhibitor stimulated goal-directed behavior in mice compared to control animals who expressed stimulus-response habits (Hart and Balleine, 2016; Swanson et al., 2017; Woon et al., 2020). In spatial goal-directed tasks, a long-term goal representation in mPFC neurons has been observed (Poucet et al., 2004; Hok et al., 2005), whereas inhibition of the MAPK plasticity pathway in the mPFC immediately after training impaired performance in a version of the Morris watermaze task (Leon et al., 2010).
The experimental data above support the proposal that rodent mPFC is directly involved in a behaviorally important long-term memory storage. In relation to the first question asked in the Introduction, the primary candidate options for executive long-term storage in rodents are high-level representations of behavioral strategies (Ragozzino et al., 1999; Rich and Shapiro, 2009) and rules for switching between them (Durstewitz et al., 2010; Hyman et al., 2012), necessary prerequisites of behavioral flexibility (Granon and Floresco, 2009). In spatial navigation tasks, neuronal representations of different strategies correspond to strategy-selective populations of neurons that are activated when memory systems, encoding these strategies, are engaged in the current behavioral task (White and McDonald, 2002; Arleo and Rondi-Reig, 2007; Rich and Shapiro, 2009). In fear conditioning, both the expression of learned fear response and the expression of learned extinction are stored in the distributed network of interconnected structures, including mPFC, which exerts control over other structures such as amygdala (Quirk and Mueller, 2008). The data showing that long-term memory in mPFC is required for complex working-memory tasks suggest that it can keep a memory trace of a mental strategy (Otani et al., 2002), instrumental to “working with memory” (Seamans and Yang, 2004).
Whether a contextual information, either spatial (i.e., related to a representation of surrounding environment during a particular task) or non-spatial (i.e., related to temporal or other aspects of the task), is also stored in the mPFC, may depend on the nature and complexity of the task (Euston et al., 2012; Hyman et al., 2012). In complex spatial tasks or in non-spatial tasks, mPFC has been repeatedly implicated in supporting a high-level hierarchical representation of the environment or the abstract task model (Tanji and Hoshi, 2001; Botvinick, 2008), which can be considered as a more complex form of contextual task representation. In the analysis of reward devaluation experiments, a distinction between goal-directed and habitual actions, is often considered in terms of the distinction between “model-based” and “model-free” algorithms in the reinforcement learning literature (Dayan and Niv, 2008). Thus, in addition to a representation of rules or strategy switches, it is likely that during complex tasks prefrontal memory circuits store a high-level “task model,” e.g., as a topological graph of the environment (Hasselmo, 2005; Martinet et al., 2011) or as a tree-like decision structure (Daw et al., 2005), see Section 5. This proposal is supported by experimental studies showing that mPFC is involved in the memory of spatial goals (Hok et al., 2005) and of a temporal order of spatial information in complex tasks (Kesner, 2000).
Assuming, based on the considerations above, that long-term memories are indeed stored in mPFC and support its role in flexible control of behavior, the second question asked in the Introduction addresses neuronal mechanisms underlying the formation of such memories, i.e., long-term depression (LTD) and long-term potentiation (LTP) (Bliss and Collingridge, 1993). As in many other cortical areas, neurons in mPFC readily exhibit long-lasting changes in synaptic strength under a variety of different experimental protocols, thus providing mechanistic support for the hypothesis of long-term memory storage in this brain structure.
In general, synaptic stimulation at low and high frequencies is expected to result in LTD and LTP, respectively, in agreement with the standard synaptic plasticity model described by the Bienenstock-Cooper-Munro (BCM) theory of cortical plasticity (Bienenstock et al., 1982; Lisman, 1989; Cooper et al., 2004). A prominent feature of this theory is the adaptive threshold between LTD and LTP, the value of which determines the direction (i.e., depression or potentiation) of plasticity at a given stimulation strength (or, alternatively, at a given level of stimulation-induced intra-synaptic calcium concentration). The LTD-LTP pattern of dependence on stimulation frequency has also been observed in rodent mPFC, although with a number of differences between rats and mice in terms of synaptic stimulation protocols, required to induce plasticity, and underlying molecular mechanisms. Studies reviewed below are summarized in Table 1, according to the species, type of plasticity and the corresponding induction protocol.
In vivo. In anesthetized rats, a tetanic stimulation at frequencies 50–250 Hz induced LTP of projections from the hippocampus, the visual cortex, the amygdala and the contralateral cortex to the prelimbic subregion of the mPFC. In particular, in synaptic contacts from hippocampal afferents originating in the CA1 or subiculum, LTP was induced by tetanic stimulation at 250 Hz (Laroche et al., 1990; Jay et al., 1996, 1998; Mulder et al., 1997). This LTP required NMDA receptor activity and protein kinase A (PKA). As shown below, the implication of NMDA-PKA molecular cascade, also participating in the classical hippocampal LTP (Malenka and Bear, 2004), is a common feature of prefrontal LTP induced at high frequencies in rats and mice. In projections from the contralateral prelimbic cortex, LTP was induced at frequencies 10–200 Hz (Gemmell and O'Mara, 2000), and in those from the visual cortex at 50 Hz (Kim et al., 2003). In synaptic contacts from the amygdala, LTP was induced by theta burst stimulation (Maroun and Richter-Levin, 2003).
Standard low-frequency tetanus protocols have initially failed to induce LTD in the synapses from the ventral hippocampal CA1 to the prelimbic cortex (Burette et al., 1997). However, Takita et al. (1999) determined a reliable LTD-inducing low-frequency (1 Hz) burst protocol by varying the burst duration, thus supporting the LTD-LTP plasticity pattern (see also Izaki et al., 2002, 2003).
It is interesting to note here that even though Burette et al. (1997) did not observe LTD following a number of low-frequency protocols, one of them (2-pulse 5 ms bursts delivered at 1 hz) resulted in a depotentiation of a previously induced LTP. This depotentiation decreased synaptic strength to the baseline, but not below it, even after the same stimulation was repeated several times. Depotentiation was initially observed in the hippocampus (Malenka and Bear, 2004) and is considered as a possible forgetting, or extinction, mechanism (Kim et al., 2007). Even though it is largely accepted that extinction constitutes new learning rather than erasure of the old one (Bouton et al., 2011), a recent study has shown that opposite changes at the same dendritic branches in the frontal cortex are associated with fear learning and forgetting (Lai et al., 2012).
In vitro. Layer V pyramidal neurons in rat mPFC slices were recorded either intracellularly (Hirsch and Crepel, 1990; Vickery et al., 1997; Otani et al., 1998; Caruana et al., 2011) or extracellularly (Huang et al., 2004), while an electric stimulation was delivered by extracellular current pulses to layers I–II or layers II–III, respectively. These layers contain synapses of cortico-cortical projections (from neighboring neurons, contralateral mPFC neurons, and from other cortical areas), as well as afferent fibers from a wide variety of subcortical brain structures (including the hippocampus, Kuroda et al., 1998; Hoover and Vertes, 2007).
Similarly to the in vivo studies cited earlier, a high-frequency stimulation (300 Hz) induced NMDA-dependent LTP in these synapses, and pharmacological block of PKA or protein synthesis impaired this LTP (Huang et al., 2004). Lowering stimulation frequencies to 50–100 Hz resulted in high neuron-to-neuron variability in terms of the sign and amplitude of plasticity, as different neurons expressed either LTP, LTD or no change under this protocol (Hirsch and Crepel, 1990; Law-Tho et al., 1995; Vickery et al., 1997; Auclair et al., 2000). While calcium elevation was necessary for both LTD and LTP at these intermediate frequencies (Hirsch et al., 1992), a pharmacological NMDA receptor blockade masked LTP and resulted in LTD instead (Hirsch and Crepel, 1991). In a series of studies, in which tetanic stimulation at a fixed frequency of 50 Hz was used, either no change (when the stimulation included 4 tetanic trains) or NMDA-independent LTD (with 6 tetanic trains) was observed (Otani et al., 1998, 1999; Kolomiets et al., 2009). This variability of plastic changes at the intermediate stimulation frequencies can be interpreted as a consequence of proximity of synaptic stimulation (or of stimulation-induced synaptic state) to the LTD/LTP threshold. Finally, synaptic stimulation at still lower frequencies reliably resulted in LTD (Huang and Hsu, 2010; Caruana et al., 2011; Bai et al., 2014). In particular, two different forms of activity-dependent LTD were observed: one that required NMDA receptor activation, and the other that did not. The first, NMDA-dependent form of LTD, was reported when plasticity was induced by a tetanic stimulation at 3 Hz (Bai et al., 2014). This form of LTD involved phospholipase C-protein kinase C (PLC-PKC) molecular cascade and MAP kinase activity. The second, NMDA-independent form of LTD, was observed following a paired-pulse stimulation at 1 Hz (Huang and Hsu, 2010; Caruana et al., 2011), and required an activation of metabotropic glutamate receptors (mGluRs), muscarinic acetylcholine receptors and PKC.
In the mouse in vivo, a high-frequency (250 Hz) stimulation of mediodorsal thalamic afferents resulted in LTP of stimulation-evoked response approximately 30 min after the stimulation (Herry and Garcia, 2002), while a low-frequency (2 Hz) stimulation of mediodorsal thalamus afferents resulted in LTD or LTP in different mice (Herry et al., 1999; Herry and Garcia, 2002). However, when the same stimulation was combined with fear conditioning, a reliable LTD was observed (probably due to a neuromodulatory effects on plasticity, see below).
In the mouse in vitro, a 100–300 Hz tetanic and theta-burst stimulation delivered to mPFC layer II-III, as well as a pairing protocol for the same synapses also resulted in LTP (Huang et al., 2004; Xu et al., 2009), which was NMDA-dependent (Cui et al., 2011). At low stimulation frequencies (3 and 10 Hz), LTD was observed in the same synapses that did not require NMDA activation, but was instead mGluR-dependent and required endocannabinoid receptor activation (Huang et al., 2004; Lafourcade et al., 2007).
Within the framework of the BCM theory of synaptic plasticity mentioned earlier, the above data can be interpreted in terms of plasticity curves that schematically represent changes in synaptic efficacy as a function of presynaptic stimulation strength (or, equivalently, as a function of stimulation-induced post-synaptic depolarization or calcium elevation, Bear et al., 1987; Lisman, 1989; Kirkwood et al., 1996), see Figure 2A. Thus, a high-frequency stimulation invariably results in LTP, while a low-frequency stimulation leads to LTD, with the LTD/LTP threshold somewhere in between these two regimes. The experimentally observed neuron-to-neuron variability with respect to plasticity amplitude and direction at intermediate frequencies (i.e., in the range of 50–100 Hz in vitro and 3–10 Hz in vivo) may thus reflect proximity of stimulation-induced activation of molecular plasticity processes to the intrinsic neuronal LTD/LTP threshold. This interpretation suggests the LTD/LTP threshold in vivo is shifted toward lower synaptic activity levels and/or calcium concentrations. In addition, the difficulty of choosing the right protocol for LTD induction in vivo can be interpreted as corresponding to a narrower LTD window.
Figure 2. A schematic summary of synaptic plasticity experiments using BCM curves. (A) Plasticity curves corresponding to in vivo (green line) vs. in vitro (black line) data. The LTD/LTP threshold in vivo has a lower value and the LTD window is narrower. (B) Plasticity curves corresponding to low (blue line) and high (red line) tonic DA concentrations, for the in vitro condition. The black curve in (B) is a copy of the curve in (A). The blue and red arrows illustrate D1 facilitation of LTP and D2 facilitation of LTD by low and high DA concentration, respectively, near plasticity threshold. In both plots, the vertical axis corresponds to a change in average synaptic weight of a neuron, measured by the amplitude of somatic EPSP. The horizontal axis represents stimulation frequencies on a logarithmic scale. The black arrows correspond to a stimulation at low (3 Hz), intermediate (50 Hz) and high (300 Hz) frequencies. Insets: the same curves as in the main plots, shown on a linear scale.
Via the mesocortical dopaminergic pathway, mPFC receives direct projections from dopaminergic neurons residing in the ventral tegmental area (VTA) and, to a lesser extent, in the substantia nigra pars compacta (Thierry et al., 1973; Björklund and Dunnett, 2007). An effective dopamine (DA) concentration in mPFC is mainly determined by three processes: release of the neuromodulator by dopaminergic axon terminals, reuptake by DA (DAT) or norepinephrine (NET) transporter membrane proteins in these terminals and metabolism via catechol-O-methyltransferase (COMT), an enzyme located in post synaptic neurons or glial cells (Garris and Wightman, 1994; Mundorf et al., 2001; Yavich et al., 2007; Bai et al., 2014). In wild-type mice, pharmacological DAT inhibition had a negligible effect on extracellular prefrontal DA levels, whereas NAT inhibition increased DA levels 2- to 4-fold (Käenmäki et al., 2010), suggesting that DA uptake in this cortex depends primarily on the NET, in contrast to the striatum where it primarily mediated by DAT. In NET- and COMT-knockout mice, prefrontal DA levels were increased by 55 and 60%, respectively (Morón et al., 2002; Käenmäki et al., 2010). The data from DAT-knockout mice are controversial as either no effect (Shen et al., 2004) or a 3.6-fold increase of DA levels (Xu et al., 2009) were observed.
The release profile of DA is determined by the activity of dopaminergic neurons, thought to occur in vivo in two distinct firing regimes: “tonic” and “phasic” (Floresco et al., 2003; Grace et al., 2007). The tonic regime corresponds to a regular spontaneous activity of a subset of dopaminergic neurons and is thought to provide a stable baseline DA concentration in target structures, including mPFC. The phasic regime corresponds to burst firing of these neurons, providing a neuronal basis for reward-based learning in the brain (Hollerman and Schultz, 1998). It has been argued that due to differences between striatal and cortical dopaminergic projection systems, the latter is more suited to detect relatively slow (i.e., on the time scale of seconds to minutes) changes in tonic DA concentration and is not sensitive to short (i.e., about 200 ms) phasic dopaminergic signals (Seamans and Yang, 2004; Lavin et al., 2005). This distinction is supported by the data showing that mPFC expresses much slower clearance rates for DA (Garris and Wightman, 1994), leading to a prolonged DA response to short activation bursts of DA neurons. Moreover, prefrontal DA appears to be released by a specific subpopulation of VTA dopaminergic neurons that are molecularly and functionally distinct from “conventional” neurons thought to signal reward-related activity, at least in mice (Lammel et al., 2008). This subpopulation is characterized by the ability to sustain tonic firing at high rates for prolonged periods of time, and by a lack of D2 autoreceptors that inhibit DA release by feedback control. Finally, synaptic plasticity experiments have repeatedly shown that long-term (in the range of tens of minutes), but not short-term, changes in background DA concentration determine the amplitude and direction of synaptic plasticity in the mPFC (Matsuda et al., 2006; Kolomiets et al., 2009). This is in contrast to the striatum, where a short DA pulse concurrently with afferent stimulation can induce a change in the direction of plasticity (Wickens et al., 1996).
On the post-synaptic side, there are two main dopaminergic receptor types, D1 (including D1 and D5 receptors) and D2 (including D2, D3, and D4 receptors), both of which are expressed in prefrontal neurons (Vincent et al., 1993; Gaspar et al., 1995; Santana et al., 2009; Zhang et al., 2010) and are important for normal PFC-dependent behaviors (Seamans et al., 1998; Setlow and McGaugh, 2000; Floresco et al., 2006; St Onge et al., 2011). Santana et al. (2009) performed a quantitative analysis of D1- and D2- receptor expression in the rat prelimbic mPFC, showing that these receptors are present in excitatory pyramidal neurons and, to a lesser extent, in inhibitory γ-Aminobutyric acid (GABA) interneurons in all layers. Much larger proportion of pyramidal neurons express DA receptors in deeper (V-VI) than in superficial (II-III) layers. As to the receptor types, a similar proportion of pyramidal cells expressed D1 and D2 receptors in layer V, while in other layers D1-expressing cells were more abundant (3- to 4-fold) than D2-expressing ones. In mice, D1 receptors are more abundant in deeper layers, while D2 receptors in superficial ones, with D1:D2 ratio varying between 1.5 and 2.5 depending on age (Wei et al., 2018; Cullity et al., 2019; Bjerke et al., 2022). Co-localization studies show that only about 25% of prelimbic cells express both receptor types in rats and mice, suggesting a partial segregation of D1- and D2-expressing neurons in the mPFC (Gaspar et al., 1995; Vincent et al., 1995; Wei et al., 2018). Interestingly however, in several electrophysiological studies the effects of pharmacological manipulations of both D1 and D2 receptors were observed in single pyramidal mPFC neurons (e.g., Tseng and O'Donnell, 2004; Matsuda et al., 2006; Xu et al., 2009). In these studies GABAergic transmission was blocked, excluding the possibility that D2 receptors acted on neuronal activity via interneurons (Xu and Yao, 2010).
D1 and D2 receptors are coupled to G proteins stimulating (Gαs/olf) and inhibiting (Gαi/o) second messenger cAMP, respectively (Missale et al., 1998; Beaulieu and Gainetdinov, 2011). Consequently, via their main transduction cascades these receptors exert opposite effects on the classical cAMP - PKA plasticity pathway (Malenka and Bear, 2004). Specifically to the PFC, the D1-mediated facilitatory effect on LTP can be mediated by such mechanisms as stimulation of surface expression of AMPA receptors (Sun et al., 2005), neuronal excitability increase via protein kinase C-phospholipase C cascade (Tseng and O'Donnell, 2004; Chen et al., 2007), and potentiation of NMDA receptor responses (Zheng et al., 1999; Li et al., 2009). The D1-mediated activation of cAMP-PKA pathway has been shown to be necessary for LTP in hippocampal (Jay et al., 1998; Gurden et al., 1999) and callosal (Coppa-Hopman et al., 2009) mPFC synapses in vivo. Some of these D1-mediated actions are mirrored by antagonistic D2-activated cellular pathways. In particular, D2 receptors decrease excitability, downregulate AMPA trafficking, and suppress NMDA receptor activity (Zheng et al., 1999; Wang et al., 2003; Tseng and O'Donnell, 2004; Sun et al., 2005). In addition, D2 receptors modulate redistribution of NMDA receptors away from the synapse and inhibit CaMKII, preventing LTP (Xu et al., 2009). Cooperative effects of these two receptor classes have also been reported. For example, coactivation of D1/D2 receptors leads to an increase in intracellular calcium levels in cell cultures (Lee et al., 2004) and to the activation of the MAPK/ERK, required for both LTD and LTP in in vitro (Otani et al., 1999; Kolomiets et al., 2009).
A given DA concentration will thus activate both D1 and D2 receptors in the local circuit, such that their dynamic balance will determine the type of resulting plasticity (or its absence), depending on differential sensitivities of downstream molecular cascades to DA (Nomura et al., 2014). A certain controversy exists concerning the relative activation of prefrontal D1 and D2 receptors as a function of prefrontal DA levels. A widely held hypothesis is that lower DA concentrations predominantly activate D2 receptors, while higher DA concentrations result in a stronger net D1 activity (Goto and Grace, 2005; Shen et al., 2008). This hypothesis is based on earlier studies suggesting that a larger proportion of D2 receptors are in a high affinity state, and, conversely, a larger proportion of D1 receptors are in low affinity state in the rat striatum (Creese et al., 1983; Richfield et al., 1989). However, this issue is far from clear in relation to PFC. In fact, prefrontal studies have repeatedly reported D1-mediated effects at a lower DA concentrations and D2-mediated effects at a higher one (Zheng et al., 1999; Trantham-Davidson et al., 2004; Li et al., 2009), contrary to the striatum-based data. This can occur due to several reasons: (i) the density of D1 receptor distribution in rodent PFC is higher than that of D2 receptors (see above); (ii) differences in the localization of DA receptors (synaptic for D2 vs. extra-synaptic for D1) may contribute to receptor sensitivities to DA (Seamans and Yang, 2004); (iii) the distribution of affinity states of these receptors may differ between the striatum and PFC (no studies, to our knowledge directly addressed this question so far). Affinity states may change receptor sensitivities to DA by several orders of magnitude (Richfield et al., 1989).
While in primates the study of the direct influence of DA on long-term memory and synaptic plasticity is hard and only correlative evidence exists (see Puig et al., 2014, for review), experiments in rodents have clearly demonstrated that this neuromodulator is strongly involved in executive behavioral control (Floresco et al., 2006), while also strongly influencing the amplitude and direction of prefrontal synaptic plasticity (Jay, 2003; Otani et al., 2003). Elucidating the role of DA in plasticity may thus help to understand its role in mPFC-dependent memory and therefore in executive behavior. However, it has proved difficult to provide a coherent interpretation of results of synaptic plasticity experiments, since it is complicated by the fact that DA effects on plasticity depend on the stimulation length and frequency, as well as on the length and time of application. One of the objectives of this review is to provide such a unifying interpretation by means of plasticity curves introduced in the previous section. The next sections provide an overview of experimental studies, including those from our group, that address DA modulation of prefrontal synaptic plasticity in rats and mice (see Table 2).
The only in vivo data on DA modulation of prefrontal plasticity comes from studies in anesthetized rats, in which an electric stimulation of VTA dopaminergic afferents to mPFC, concurrent with tetanic plasticity induction protocol at 250 Hz, enhanced NMDA-dependent LTP in hippocampal-medial prefrontal synapses (Gurden et al., 1999). An electrolytic lesion of VTA impaired this LTP, while saturation of LTP by stimulation at a higher frequency (300 Hz) eliminated the DA-induced enhancement effect, suggesting that DA acts on plasticity in a limited window of stimulation frequencies. The enhancement of LTP was mediated by D1 receptor activation via cAMP-PKA cascade, while manipulation of D2-receptor activation by agonists or antagonists had no effect (Gurden et al., 2000).
In vitro, LTP induced by 300 Hz stimulation was strongly reduced by D1 receptor antagonism or PKA inhibition, while D2 receptor blockade had no influence (Huang et al., 2004), in a good agreement with the in vivo data above. In the range of lower stimulation frequencies (50–100 Hz), a short and strong DA application (100 μM for 5 min during stimulation) shut down LTP and favored NMDA-independent LTD (Law-Tho et al., 1995; Otani et al., 1998). This LTD required combined activity of D1, D2 receptors and mGluRs, and also involved MAP kinases (Otani et al., 1998, 1999). In a stark contrast to the LTD facilitation by a short-term high-concentration DA bath, a long-term application of low-concentration DA (3 μM for 15–40 min) resulted in an opposite effect, namely LTP facilitation and conversion from LTD to LTP (Matsuda et al., 2006; Kolomiets et al., 2009). Moreover, in the LTP regime, the amplitude of potentiation was highest for an optimal DA concentration, such that too low or too high concentration of DA abolished LTP (Kolomiets et al., 2009). Molecular mechanisms implicated in the DA-facilitated LTP include co-activation of D1, D2 receptors and MAP kinases, similarly to DA-induced LTD above, but also require NMDA receptor activity.
Out of the two different forms of LTD induced by low-frequency stimulation in vitro, NMDA-dependent (Bai et al., 2014) and NMDA-independent (Caruana et al., 2011), only the former was tested in different DA conditions. In that study we have shown that the NMDA-dependent LTD required a co-activation of D1 and D2 receptors, since the antagonism of either receptor blocked plasticity (Bai et al., 2014). Moreover, when endogenous DA activity was augmented by DAT inhibition during stimulation, the LTD was blocked by overstimulation of D1 receptors. Since in these experiments too weak or too strong DA receptor activation impaired LTD, these results can be interpreted in terms of an inverted-U shape profile, as has been done for LTP results above (Bai et al., 2014; Otani et al., 2015). Molecular mechanisms underlying this NMDA-dependent form of LTD involved PLC, PKC and MAP kinases.
With the help of the plasticity curves introduced earlier, DA modulation of plasticity in rats can be interpreted in the following way (see Figure 2B). First, in the regime of high stimulation frequencies (≥200 Hz), in which only LTP can be induced, DA modulates this LTP via D1 receptors acting through cAMP-PKA cascade. Available evidence suggests that D2 receptors are not involved in the LTP induced at these high frequencies, neither in vivo nor in vitro. Second, near plasticity threshold (around 50 Hz in vitro), DA via both D1 and D2 receptors exerts strong bidirectional effect on plasticity. In particular, high DA elevation induces a rightward shift of the LTD/LTP threshold toward higher synaptic activities (high DA, conversion from LTP to LTD, Figure 2B), while low DA elevation induces a leftward shift (low DA, conversion from LTD to LTP). It follows that progressive elevation of DA concentration near threshold should peak at some optimal DA concentration exhibiting the “inverted-U” LTP profile, in agreement with the data (Kolomiets et al., 2009). Finally, still lower stimulation frequencies enter into LTD-only regime, in which DA controls LTD in a bidirectional manner: either DA decrease or DA elevation can shut down LTD via cooperative action on D1 and D2 receptors to affect plasticity. In this regime, the interpretation of experimental results within the proposed framework is complicated by our ignorance about the stimulation-induced synaptic state relative to the LTD/LTP threshold. For example, if the synaptic state is far from the threshold (i.e., close to the baseline synaptic activity), then progressive DA elevation will shut down LTD (as in the study by Bai et al., 2014). On the other hand, if the synaptic state is close to the LTD/LTP threshold, then DA elevation will enhance LTD (as is the case with mouse LTD, Huang et al., 2004, see below). Thus, our hypothesis of DA modulation is neither supported nor disproved by these opposite experimental results on LTD. However, the hypothesis predicts that for a given synaptic state, progressive elevation of DA concentration will result in a particular response profile of synaptic efficacy change, depending on how close the synaptic state is to the plasticity threshold.
It can further be speculated that the same pattern of DA plasticity modulation occurs in vivo, since the same molecular mechanisms are likely to be at play. However, in this condition the strongest dopaminergic modulation of plasticity is predicted to occur at much lower stimulation frequencies (corresponding to a lower LTD/LTP threshold in vivo) and in a narrower frequency window (see Figure 2A), a prediction that is yet to be tested.
While in vivo mouse data testing DA modulation of prefrontal plasticity is currently absent (to our knowledge), in vitro, D1 agonists facilitated LTP induced at 300 Hz (Huang et al., 2004), in agreement with the role of D1 receptors in this form of LTP in rats (without agonist application, a stable but weak LTP was observed in this study).
A synaptic stimulation at an intermediate strength, in particular, a tetanic stimulation (at 100 Hz) or a theta-burst stimulation, induced LTP in wild-type mouse slices (Xu et al., 2009; Xu and Yao, 2010). This LTP could not be induced in slices from DAT-knockout mice by these same protocols. This LTP impairment in the mutant mice has been shown to result from DAT-induced elevated tonic DA concentration, which acted via D2 receptors to activate protein phosphatase 1 and block LTP. This effect was confirmed in experiments with wild-type mice, since when these mice were injected amphetamine or DAT inhibitor (both of which induce elevated DA levels in the mPFC) 30 min before killing and slice preparation, LTP was blocked also in these mPFC slices (Xu et al., 2009). Thus, in mice, as in rats, an elevated tonic DA concentration shuts down LTP, induced at frequencies around 50–100 Hz, although the underlying molecular mechanisms could be different in the two species. In the framework of the proposed hypothesis, the LTP block is explained by a DA-induced shift of the plasticity threshold toward higher synaptic activities (see Figure 2B).
In the low-frequency regime, LTD induced at 3 Hz was blocked by antagonists of D1 and D2 receptors or by PKA inhibition, but enhanced by a high-concentration DA bath via D1 receptor activation (Huang et al., 2004). As discussed earlier, this might be an indication that the stimulation-induced synaptic state in this study was closer to the LTD/LTP threshold, rather than to the baseline synaptic state. Contrary to the observed DA modulation of the LTD at 3 Hz, blockade of DA receptors did not affect LTD induced at 10 Hz (Lafourcade et al., 2007). If confirmed by further research, these data would support the existence of DA-dependent and DA-independent forms of low-frequency induced LTD in mice, as in rats.
In contrast to classic LTD/LTP induction protocols considered earlier, spike-timing dependent plasticity (STDP) protocols induce synaptic changes by pairing a pre-synaptic stimulation (usually an extracellular current pulse delivered to the layer where synaptic connections of the recorded neuron reside), with a post-synaptic spike evoked by an intracellular current injection. Such a paired stimulation is repeated 50-100 times at a low frequency (about 0.1 Hz). The polarity of plasticity depends on whether the pre-synaptic stimulation comes before (leading to LTP in hippocampal cultured neurons and neocortical slices) or after (leading to LTD) the post-synaptic spike (Markram et al., 1997; Bi and Poo, 1998). In STDP experiments targeting mPFC, pyramidal neurons in layers V are usually recorded, with the presynaptic stimulation applied to layer II-III. In what follows, the timing difference Δt between the presynaptic stimulation and the post-synaptic spike is denoted as positive for pre-post pairing, and negative for post-pre pairing. To distinguish between the classical LTD/LTP and that induced by the STDP protocol, we will denote the latter “t-LTD/t-LTP.”
A number of studies applied STDP protocols to study prefrontal long-term plasticity in rats and mice, including its modulation by DA, as well as by other neuromodulators (Couey et al., 2007; Xu and Yao, 2010; Goriounova and Mansvelder, 2012; Zaitsev and Anwyl, 2012; Ruan et al., 2014; Louth et al., 2021). These experiments have shown that regulation of plasticity by pre-post timings in mPFC is different from that in the classical experiments, since in the absence of exogenous DA and with blocked inhibitory (GABA) receptors, LTP was induced by pre-post stimulation at Δt = +5, +10 ms, whereas no plasticity could be induced either by Δt = +30 ms or Δt = −30 ms. An application of a high DA concentration (20–100 μM) during pairing extended t-LTP portion of STDP to all the tested time intervals (Xu and Yao, 2010; Ruan et al., 2014). This DA-facilitated t-LTP was D1-cAMP-PKA dependent and required NMDA activation. Pharmacological modulation of D2 receptor activity did not affect plasticity directly, but only indirectly via inhibition of GABA release by interneurons (Chiu et al., 2010; Xu and Yao, 2010). In the presence of GABA, t-LTD or no change have been observed at almost all tested intervals (in both young adult and mature mice, Louth et al., 2021). Optogenetic activation of VTA DA fibers blocked t-LTD and this modulatory effect was abolished in the presence of the D2 receptor antagonist, but was not affected by the D1 receptor antagonist (Louth et al., 2021). The blockade of t-LTD by D2 receptors is consistent with the evidence of D2-mediated GABA suppression by DA acting by inhibiting its presynaptic release (Xu and Yao, 2010).
A comparison of the STDP data with results from classical induction protocols reviewed earlier suggests that the observed t-LTP corresponds to D1-NMDA-PKA-dependent (and D2-independent) classical high-frequency LTP regime. This suggestion is further supported by the data showing that a high concentration of applied DA in mouse slices was shown to enhance a weak classical LTP (Huang et al., 2004).
Other neuromodulators present in mPFC, such as noradrenalin (NA), acetylcholine, and serotonin, have been reported to act separately or in concert with DA to affect synaptic plasticity.
Dendritic spines of cortical pyramidal neurons appear to be a common target of both DA and NA inputs, and both receptor types share similar signaling cascades, which can modulate excitatory as well as inhibitory synaptic transmission (see Xing et al., 2016, for review). In vivo, locus coeruleus stimulation concurrently with a high-frequency stimulation of hippocampal-to-PFC inputs enhanced LTP in rats (Lim et al., 2010), similarly to the enhancement of LTP by VTA stimulation (Gurden et al., 1999). In vitro, an elevated concentration of NA induced NMDA-dependent LTD in layer I-II to layer V fibers via postsynaptic α-adrenoceptors and molecular cascade involving PKC and MAP kinase (Marzo et al., 2010). In slices from amphetamine-treated mice, in which extracellular levels of monoamines (DA, NA and serotonin) are increased, compared to wild-type controls, a low amphetamine dose enhanced, while a high amphetamine dose abolished, an LTP induced by theta-burst stimulation (Xu and Yao, 2010). This effect is similar to that observed with high and low levels of DA (Otani et al., 1998; Kolomiets et al., 2009). The low-dose LTP facilitation depended on D1 and β-adrenoceptor activation via cAMP-PKA cascade. In contrast, the high-dose LTP blockade was mediated by D2 receptors. In layer III rat pyramidal neurons, β2 adrenoceptor agonist increased the amplitude of t-LTP (Δt = +10 ms) via both postsynaptic signaling by PKA and presynaptic suppression of GABAergic inhibition (Zhou et al., 2013). This is somewhat reminiscent of t-LTP enhancement by both post- and pre-synaptic mechanisms by DA in mice (Xu and Yao, 2010). These data suggest cooperative action of DA and NA on synaptic plasticity.
Concerning the role of prefrontal acetylcholine receptors, it has been shown that an activation of nicotinic receptors (nAChR) on several interneuron types in mouse mPFC can increase GABAergic signaling and prevent t-LTP (Δt = 5 ms) in pyramidal layer V neurons (Couey et al., 2007; Goriounova and Mansvelder, 2012). On the other hand, an activation of muscarinic acetylcholine receptors (mAChR) in rat pyramidal neurons can induce an activity-independent LTD via PLC-PKC cascade (Huang and Hsu, 2010) and convert transient to permanent LTD (induced by low frequency stimulation at about 1 Hz, Caruana et al., 2011). Therefore, endogenous acetylcholine, that stimulates both receptor types, can potentially contribute to LTD induced at low stimulation frequencies around 1 Hz, in both rats and mice.
Lastly, serotonin depletion was shown to result in a LTP enhancement in the hippocampal-prefrontal pathway in rats in vivo (Ohashi et al., 2003). Serotonin application in vitro, together with tetanic stimulation at 50 Hz, facilitated NMDA-independent LTD via mGluR activation and MAP kinase (Zhong et al., 2008). These data suggest that serotonin may also contribute to prefrontal LTD.
In addition to neuromodulatory systems, retrograde signaling was shown to play an important role in prefrontal LTD. Consistent with a general involvement of endocannabinoids in retrograde signaling and cortical LTD (Heifets and Castillo, 2009), they have been implicated in both activity-independent and tetanus-induced LTD in rodent mPFC. In particular, a sole application of cannabinoid agonists and antagonists induced LTD and LTP, respectively, in rat layer V pyramidal neurons (albeit only in a subset of tested cells, Auclair et al., 2000). Consequently, when these pharmacological agents were applied together with high-frequency tetanic stimulation (at 100 Hz), they biased the tetanus-induced plasticity toward LTD or LTP. In mice, a low-frequency stimulation at 10 Hz induced LTD that was completely blocked by endocannabinoid antagonists acting via mGluR-PLC cascade (Lafourcade et al., 2007). Cannabinoid receptors (CB1) were also shown to be involved in controlling prefrontal inhibition. In mice, these receptors co-localize with D2 receptors at about 30% of inhibitory synaptic terminals in the mPFC, so that agonists of either receptor suppress inhibitory transmission (Chiu et al., 2010). Moreover, endocannabinoids may not be the only retrograde messengers in the mPFC, as the study by Huang and Hsu (2010) reported the implication of nitric oxide as a retrograde messenger during activity-independent LTD (see Caruana et al., 2011).
Finally, regulation of long-term plasticity by inhibitory GABAergic transmission has been demonstrated in a number of studies (Couey et al., 2007; Huang et al., 2007; Chiu et al., 2010; Xu and Yao, 2010; Louth et al., 2021). Often the same neuromodulator acts in a cooperative manner on both glutamatergic and GABAergic transmission to influence plasticity (Couey et al., 2007; Xu and Yao, 2010). In most of the synaptic plasticity studies reviewed earlier, GABAergic transmission was routinely blocked to separate a direct influence of neuromodulators on inhibition (which is rather complex, see Seamans and Yang, 2004, for review) from its influence on the plasticity mechanisms per se.
A general conclusion that can be made concerning the cellular mechanisms of long-term plasticity in the mPFC and its modulation by DA is that distinct plasticity mechanisms are at play depending on the level of stimulation-induced synaptic activity. In particular, the experimental data consistently show, in vitro and in vivo, in rats and mice, an involvement of D1 and NMDA receptors, as well as PKA and protein synthesis, in LTP induced at high stimulation frequencies (≥200 Hz). This form of LTP corresponds to the common form of hippocampal LTP (Huang and Kandel, 1995; Navakkode et al., 2007).
In contrast, competing molecular cascades for LTD and LTP mediate plasticity at lower stimulation frequencies around 50–100 Hz in vitro and near 3–5 Hz in vivo. In particular, at these frequencies LTP enters into competition with NMDA-independent LTD. While both types of plasticity near threshold activate MAP kinases, LTP requires NMDA-receptor activation, whereas LTD depends on mGluR activity. This is consistent with the proposal that these two receptors represent “coincidence detectors” for LTP and LTD, respectively (Karmarkar and Buonomano, 2002; Bender et al., 2006). It is likely that the mGluR-dependent form of LTD acts via the retrograde endocannabinoid signaling and is expressed presynaptically as in the somatosensory cortex (Auclair et al., 2000; Bender et al., 2006).
At still lower frequencies (in the range of 1–3 Hz) LTD takes over LTP, and, moreover, new forms of LTD, likely different in rats and mice, start to play a role. In rats, one form of LTD depends on NMDA activity and a cooperative action of D1 and D2 receptors (Bai et al., 2014). The second form of LTD requires mGluR and mAchR activation, but does not depend on either postsynaptic calcium or NMDA receptor activity (Huang and Hsu, 2010; Caruana et al., 2011). It is not known whether this latter form of LTD is under influence of DA. In the study of Bai et al. (2014), no residual LTD was observed at 3 Hz when DA receptors were blocked, suggesting that these two forms of LTD may be successively activated at progressively lower synaptic stimulation levels. More research however is required to support this conclusion, as the activity of acetylcholine receptors was not controlled during this study. At these low frequencies in mice, only NMDA-independent forms of LTD were observed so far (Huang et al., 2004; Lafourcade et al., 2007), in contrast to rats. Both forms of LTD require mGluR activation and endocannabinoid signaling, whereas only 3-Hz LTD was DA-dependent (Huang et al., 2004).
The experimental data strongly suggests that plasticity near threshold is regulated in a bidirectional manner by tonic dopamine via both D1 and D2 receptor subtypes. Although other neuromodulators, such as NA and serotonin, may also be involved in different aspects of this plasticity (especially in LTD Zhong et al., 2008; Marzo et al., 2010), only DA has been shown to directly influence the direction of plasticity to our knowledge (Matsuda et al., 2006; Kolomiets et al., 2009; Zhang et al., 2009). A more complex scenario can not be however excluded, in which a particular combination of several neuromodulators (including DA acting via D1 receptors) favors LTP, while a different combination (including DA acting via D2 receptors) favors LTD (Seol et al., 2007). Such an assumption is hypothetical at present as there are not enough data to support it. In mice, the available evidence suggests that an elevation of tonic DA can shut down LTP induced at 100 Hz via D2 receptors (Xu et al., 2009). While there is no direct evidence that tonic DA can change the direction of plasticity in mice, it was shown that DA facilitates LTP induced at higher stimulation frequencies, while it facilitates LTD at low frequencies, as discussed earlier. Therefore, it is reasonable to suggest that DA effect on plasticity switches sign at some intermediate stimulation strength (likely in the range of stimulation frequencies between 10 and 100 Hz in vitro).
The processing vs. representational dichotomy adopted by Wood and Grafman (2003) to classify high-level models of PFC function can also be applied to describe a large variety of computational models of this structure in the way they rely on long-term memory storage. Processing-type models primarily focus on the ability of PFC neurons to show elevated persistent activity in delay-period tasks, considered to be a neural implementation of working memory (Durstewitz et al., 2000; O'Reilly, 2006). A wide array of such models differ in neuronal mechanisms that are proposed to support persistent activity, but they all share the property that such activity can maintain in the working memory any information relevant for the task. The unique role of PFC is therefore characterized by the operation it can perform, rather than by the nature of the stimuli it operates with. In simple working memory tasks, the persistent network state may represent the direction of eye movement to a remembered visual cue (Compte et al., 2000), whereas it can represent a high-level contextual cue in a hierarchical goal structure or an abstract behavioral rule (Rougier et al., 2005; O'Reilly and Frank, 2006). In these models, the role of long-term plasticity is often implicit and is constrained by the requirement to ensure persistent activity states. For example, an item can be held in working memory by recurrent excitation of neurons interconnected with high synaptic weights, forming a point attractor (Durstewitz et al., 2000). In this case it is assumed that PFC learns slowly over time all the items it can represent, such that the corresponding attractor state could be activated in working memory when needed (Amit and Brunel, 1997). Alternatively, a synaptic matrix can be constructed so as to enable continuous attractor dynamics, in which case the attractor represents the location of a remembered object rather than the objects itself (Camperi and Wang, 1998).
The role of dopamine in processing-type models has been modeled in relation to working, but not long-term, memory. In particular, in a detailed biophysical model (Durstewitz et al., 2010) proposed that an elevated DA by acting primarily on D1 receptors, enhanced the stability of persistent states and their resistance to distractors via combined action on GABA and NMDA currents. This state corresponds to active maintenance of important information in working memory, e.g., during a behavioral task. In low DA conditions, D2 receptors dominate and produce opposing destabilizing effects, allowing the contents of working memory to be rapidly updated (Dreher and Burnod, 2002; Seamans and Yang, 2004). This model, together with an earlier model of gain modulation by DA (Servan-Schreiber et al., 1990), made sense of a multitude of DA effects on neural activity and provided a solid biophysical foundation to more abstract models of working memory (O'Reilly, 2006).
In contrast to processing-type models above, representational models can be distinguished by the type of information that is proposed to be stored by PFC. Models of human cognition focused on behavioral planning (Dehaene and Changeux, 2000), learning hierarchical behavioral structures (Cooper and Shallice, 2006; Botvinick, 2008; Holroyd and Mcclure, 2015) and associations between stimuli, rewards, actions and their outcomes (Alexander and Brown, 2011; Soltani and Koechlin, 2022). Computational models of rodent behavior focused on strategy selection (Dollé et al., 2010; Sheynikhovich and Arleo, 2010), goal-directed behavior and action planning (Hasselmo, 2005; Martinet et al., 2011). In the latter models PFC neurons learned either a representation of a behavioral strategy (e.g., response- vs. place-based) or the structure of a goal-directed task. These high-level models are not specific about neuronal mechanisms supporting information storage and usually assume a combination of associative Hebbian and reinforcement learning rules. The role of DA is either not considered at all or is assumed to signal reward-prediction error in the context of standard reinforcement learning algorithms. Given the wealth of evidence, reviewed earlier, that DA is a powerful modulator or long-term synaptic plasticity in mPFC, we attempted to unify available data in a simple computational model, in which DA shifts the position of the LTD/LTP plasticity threshold and therefore controls the induction of plasticity (Sheynikhovich et al., 2011, 2013).
The model extends the classical calcium-based plasticity rule (Lisman, 1989; Shouval et al., 2002) by implementing the threshold modulation via opponent activation of D1- and D2-receptor pathways. In a first, calcium-dependent component of the model, the amplitude of synaptic efficacy change in the model neuron (Figure 3A) follows a BCM-like dependence, meaning that (i) calcium elevation is necessary for any plasticity to take place; (ii) moderate calcium levels result in a decrease of synaptic strength; (iii) high calcium levels result in an increase of synaptic strength; and (iv) there is a threshold calcium concentration at which LTD is converted to LTP. The postsynaptic calcium concentration in the model is controlled by the calcium influx via NMDA channels and via high-voltage-activated calcium channels, as observed in real mPFC neurons (Seamans et al., 1997). The second, DA-dependent, component of the model describes how an extracellular tonic DA concentration can modulate the calcium-based plasticity rule. In agreement with the analysis of the in vitro experimental data (see Figure 2B), an activation of a D1-mediated molecular cascade facilitates LTP and shifts the threshold to lower calcium concentrations, whereas that of a D2-mediated cascade facilitates LTD and induces a shift in the opposite direction. While in this model we assumed that both D1 and D2 receptors are expressed in single pyramidal neurons, it does not exclude the possibility that the two receptor classes act via segregated circuits (Xu et al., 2009), as long as they exert opposing effect on plasticity in target neurons. The effective plasticity modulation by DA is then proportional to the difference between the activities of the two molecular cascades. Because of distinct affinities of D1 and D2 receptors in the model (Figure 3B), as in real mPFC neurons (Zheng et al., 1999; Trantham-Davidson et al., 2004; Li et al., 2009), this difference has an inverted-U-shape dependence on DA concentration (Figure 3C). As a result of such modulation, lower DA concentrations lead to a leftward shift of LTD/LTP threshold and a net facilitation of LTP (corresponding to the downward deflection in Figure 3C), whereas higher DA concentrations leads to a rightward shift of the threshold and a net facilitation of LTD (corresponding to the upward deflection in Figure 3C). To show the effect of various DA conditions on synaptic plasticity, many neurons with slightly different neuronal parameters (i.e., compartment sizes and properties of ionic currents) were simulated to reflect differences between real neurons in a slice. These neurons were then tested under conditions mimicking in vitro experimental protocols reviewed in Section 4. Modeling results show that for stimulations at high (300 Hz), intermediate (50 Hz, near LTD/LTDP threshold) and low (3 Hz) frequencies all model neurons exhibited LTP, no change, or LTD, respectively (Figure 3D), similarly to standard plasticity models (Shouval et al., 2002; Clopath et al., 2008). At the high and low frequencies, changes in the stimulation protocols (stimulating at the same frequency for a longer or shorter period of time) could only saturate or abolish plasticity (not shown). However, near threshold, where standard protocols did not induce any plasticity, increasing the stimulation length produced either LTP, LTD or no change in different neurons (“No DA” condition in Figure 3E). We hypothesize that this variability in the induced plasticity in the model neurons reflects the variability of synaptic plasticity near this frequency in real neurons in vitro, observed experimentally (Hirsch and Crepel, 1990; Law-Tho et al., 1995; Otani et al., 1998; Kolomiets et al., 2009).
Figure 3. A model of DA-modulated synaptic plasticity and simulation results. (A) A schematic representation of a multicompartment model of pyramidal neurons with AMPA, NMDA, D1 and D2 receptors. (B) A molecular cascade for LTP is facilitated at lower DA concentrations by D1 receptors (shown by the full black line). A molecular cascade for LTD is facilitated at higher DA concentrations by D2 receptors (dashed line). (C) The plasticity threshold between LTD and LTP shifts as a function of a difference between the activities of the two molecular cascades in (B), resulting in a U-shaped net effect of DA concentration on synaptic plasticity. A negative threshold change corresponds to its shift to lower stimulation frequencies in Figure 2B and facilitation of LTP. Conversely, a positive change facilitates LTD. (D) Synaptic plasticity in the model neurons (measured by the amplitude of somatic EPSP±SD) as a result of tetanic stimulation at low (3 Hz, shown by downward triangles), intermediate (50 Hz, circles) and high (300 Hz, upward triangles) stimulation frequencies. Inset: mean EPSP amplitude 40 min after stimulation in the three cases. (E) Dopaminergic modulation of synaptic plasticity near LTD/LTP threshold. The bars show the proportion of simulated neurons that underwent LTD (in gray), no change (in white) or LTP (in black), depending on DA conditions. (F) An inverted-U-shape dependence of the LTP amplitude for different concentrations of DA, as observed experimentally by Kolomiets et al. (2009). The plots are adapted with permission from Sheynikhovich et al. (2013).
In agreement with the key results of plasticity experiments in the mPFC, DA strongly influenced both the sign and amplitude of plasticity near threshold in the model. In particular, LTD was facilitated by a short-term high-concentration DA application in most of the simulated neurons, as in real neurons (Law-Tho et al., 1995; Otani et al., 1998), because this high DA concentration shifted the threshold to higher calcium concentrations on the timescale of several minutes (“High DA” condition in Figure 3E). In contrast, LTP was facilitated by a prolonged low-concentration DA bath (Kolomiets et al., 2009), because lower concentrations induced a slow (on the time scales of tens of minutes) shift of the plasticity threshold to lower calcium levels (“Low DA” condition in Figure 3E). Third, the same model provides an explanation of DA priming effect, showing that a short DA application 40 min before stimulation changes the direction of plasticity in mPFC neurons (“Priming” condition in Figure 3E, Matsuda et al., 2006). This is explained by the adaptive threshold dynamics as a function of DA concentration during washout: initially high DA concentration facilitated LTD, while at a later time the concentration decreased and entered the LTP facilitation regime. Finally, the model is also consistent with the experimentally observed inverted-U dependence of LTP amplitude as a function of DA concentration (Figure 3F; Kolomiets et al., 2009). This form of dependence in the model is a direct consequence of the U-shaped dependence of the threshold position on DA concentration (see Figure 3C).
While the model described above is the only one, to our knowledge, addressing the role of DA in prefrontal plasticity, many previous models considered its role in learning in other brain areas, such as the striatum and the hippocampus. It is therefore of interest to compare the key properties of these models.
On a single neuron level, the role of phasic DA signals in reward-based plasticity, thought to occur in the striatum, has been addressed in a number of phenomenological models of reward-based STDP (reviewed in Frémaux and Gerstner, 2015). In these models, synaptic weight change is proportional to the product of the phasic DA signal (relative to baseline) and a Hebbian STDP term, consisting of a potentiation part (for pre-post pairs of spikes) and a depression part (for post-pre pairs). Thus, a positive DA deflection from baseline during a short period of phasic activity should facilitate plasticity, while a negative DA deflection should inverse the sign of plasticity at these synapses. Both of these effects are in principle compatible with what have been observed in experiments in striatal slices, although the sign flipping effect is controversial (Fino et al., 2005; Pawlak and Kerr, 2008; Shen et al., 2008). A common underlying assumption in reward-based models is that a phasic reward signal arriving some time after activation of a synapse (induced by execution of an action) gates permanent changes at this particular synapse. A memory trace of the activated synapses is proposed to reside in a synapse-specific “eligibility trace” or a “synaptic tag” (Frey and Morris, 1997; Gerstner et al., 2018). These properties are not compatible with prefrontal plasticity model described above, mainly because the timescale of DA action on prefrontal plasticity does not suit stringent timing requirements of reward-based learning (Seamans and Yang, 2004). Experimentally, both positive events, such as novelty or food delivery, and negative events, such as stress, increase mPFC DA levels for prolonged periods (Yoshioka et al., 1996; Lavin et al., 2005) and a prolonged application of low DA concentration (15–40 min) is required to switch the sign of plasticity in the model and experiments (Kolomiets et al., 2009). In agreement with these observations, a disruption of phasic firing of DA neurons (leaving tonic firing intact) did not impair performance in a mPFC-dependent working memory task, but did impair acquisition of reward-depended behaviors attributed to the striatum (Zweifel et al., 2009). Moreover, phasic optogenetic phasic stimulation of VTA-PFC fibers time-locked to the reward delivery failed to improve performance in a place preference task (Ellwood et al., 2017).
In the hippocampus, the role of DA in plasticity differs in three principal ways from that in the striatum. First, a pharmacological block of phasic DA activity (leaving tonic DA firing intact), critically important for striatal learning, does not impair long-term spatial memory, nor novelty-induced exploration, two tasks that are thought to depend on the hippocampal DA (Zweifel et al., 2009). Second, presentation of a novel stimulus that preceded (or followed) reward learning by tens of minutes, improved hippocampal long-term memory via DA-dependent pathway (Wang et al., 2010). This is in contrast to striatal reward-based learning paradigms, in which correct stimulus-actions associations are stamped-in by a brief phasic elevation of DA after, but not before, action learning. Third, STDP experiments in brain slices and cultured hippocampal neurons have shown that DA expands the effective time window for synaptic potentiation to negative pre-post pairings via the activation of D1 receptors and shuts down t-LTD (Zhang et al., 2009; Brzosko et al., 2015). This is in contrast to striatum, where D1 was shown to be necessary for both t-LTP (for positive pre-post timings) and t-LTD (for negative ones, Pawlak and Kerr, 2008). Synaptic tagging and capture hypothesis (Frey and Morris, 1997), positing that DA is involved in the synthesis of plasticity-related proteins, accounted for much of these data, together with earlier evidence that DA is important only for late but not early LTP (Huang and Kandel, 1995; Navakkode et al., 2007; Lisman et al., 2011). It has also inspired computational models of spike-based learning (in either single-neuron setting or in populations of neurons) that successfully reproduced a large array of phenomena observed in electrophysiological and behavioral experiments (Clopath et al., 2008; Ziegler et al., 2015; Gerstner et al., 2018). In these models, the amount of DA directly facilitates synthesis of plasticity-related proteins, which in turn gates the entry of a synapse into a stable state corresponding to late LTP or LTD. Plasticity-related proteins in these models act to switch off a putative “write protection” mechanism, shared by both types of plasticity. This results in a stabilization of transient synaptic changes induced by a weak stimulation, in the presence of novelty-induced elevation of DA. In these models, the amplitude of plasticity (but not its sign) depends on DA, as changes in DA levels control the maintenance, but not the induction, of plasticity, in contrast to the reward-based learning in the striatum.
Given the comparable time scale of DA influence on plasticity in PFC and the hippocampus as well as their apparent insensitivity to phasic DA inputs, the question arises whether the experimental data from mPFC can be accounted for by the synaptic tagging and capture hypothesis of hippocampal learning and related computational models. As mentioned above, in these models DA “stamps in” the state of a synapse that has been tagged for LTD or LTP, whereas the induction process is DA-independent (Wise, 2004; Lisman et al., 2011; Frémaux and Gerstner, 2015). Therefore, higher or lower DA concentrations lead to a higher of lower number of consolidated synapses. It is not clear then, how such a mechanism could explain the bidirectional control of synaptic plasticity, induced by an increase in DA concentration, as has been observed in rodent mPFC (Matsuda et al., 2006; Kolomiets et al., 2009; Xu et al., 2009; Bai et al., 2010).
The experimental data and theoretical models reviewed above support the conclusion that rodent mPFC is directly involved in a behaviorally important long-term memory storage, that synaptic machinery in this structure is well adapted to support such storage, and that the properties of dopaminergic modulation of these plasticity mechanisms are rather unique. Electrophysiological studies suggest that this modulation is strongest within biologically plausible stimulation regimes and DA concentrations. At the same time behavioral studies demonstrate dopaminergic involvement in the same experimental paradigms in which plasticity was tested (Pezze and Feldon, 2004; Floresco et al., 2006; Hitchcott et al., 2007). Less data are available concerning the influence of other neuromodulators on plasticity. At present, it appears that NA shares many properties with DA in terms of its influence on plasticity, due to common intracellular signaling pathways (Xing et al., 2016), although direct evidence for the bidirectional influence on plasticity is scarce.
We argued that the available data on dopaminergic influence in prefrontal plasticity is consistent with the idea that tonic DA levels determine the position of the threshold between LTD and LTP, on the time scale from minutes to tens of minutes. This proposed role of DA is distinct from, but not contradictory to, the other hypotheses of dopaminergic function, namely, DA enabling the late phase of plasticity (in the hippocampus, Lisman et al., 2011) and as a reward signal for stimulus-response learning and strategy selection (in the striatum, Goto and Grace, 2005; Collins and Frank, 2014). First, the proposed DA role in the control of the prefrontal plasticity threshold is functionally independent from its role in controlling plasticity-related protein synthesis and late-phase LTP. Moreover, given that in the hippocampus a low DA concentration switches LTD to LTP (Zhang et al., 2009), it is possible that DA controls the plasticity threshold in the hippocampus, as well as in mPFC. One of possible predictions from this hypothesis is that for a correctly chosen plasticity induction protocol (within either classical or STDP frameworks), the switch in the sign of synaptic plasticity following DA application could be observed in the hippocampus. If true, this would extend the functional role of DA in this area compared to hippocampal models. Second, although reward-based coding in the mPFC can not be completely excluded (especially in its anterior cingulate subregion, Holroyd and Mcclure, 2015), available data suggest that, at least in the prelimbic cortex, properties of DA kinetics and its documented influence on plasticity do not suit well for striatum-like reward-based learning paradigms. It could nevertheless be speculated that short phasic DA events, riding on top of slow elevations of DA during behavioral task, transmit reward-based information that could be used for reward-based learning (Frémaux and Gerstner, 2015). Alternatively, it has been argued that DA neurons could transmit reward prediction errors by phasic co-release of glutamate, in parallel to DA (Lavin et al., 2005). Third, it is possible that slow changes in tonic DA, that have a strong influence on prefrontal plasticity, may switch neuronal learning pathways in the mPFC, as in the dorsal (Shen et al., 2008) and ventral (Goto and Grace, 2005) striatum, although at a much slower time scale. However, what these pathways are and whether they are anatomical or functional, is not clear. Anatomically distinct neuronal populations with different DA sensitivities (as in the dorsal striatal MSNs) have not been observed so far (see Vincent et al., 1993, for such a hypothesis). A more likely situation in our point of view is a functional, activity- or frequency-dependent, separation. It has been shown that neocortical neuronal processing occurs in different frequency bands (Sirota et al., 2008) and DA has been implicated in increasing neuronal coherence in theta band (Benchenane et al., 2010). Based on experimental and computational data reviewed above, we proposed that DA exerts the strongest influence on plasticity near threshold, i.e., in theta-gamma frequency range, depending on previous activity (Kirkwood et al., 1996). DA modulation could then switch learning between neurons synchronized at different frequencies (Fries, 2005).
The reviewed data shows that the direction and amplitude of plasticity in mPFC depends in complex ways on the relative activation of D1 and D2 receptors. More specifically, whereas these receptors facilitate opposite plasticity cascades, at low DA concentrations they cooperatively participate in LTD. There is evidence that some molecular cascades mediating synaptic plasticity are activated by a combined action of the two receptor types (Lee et al., 2004). In agreement with this, a number of studies of behavioral flexibility have shown either cooperative or antagonist effects of these receptors on behavior. In particular, it was shown that blockade of either D1 or D2 receptors impaired switches from one strategy to another in a cross maze, leading to an increased number of perseverative errors (Ragozzino, 2002; Mehta et al., 2004; Floresco et al., 2006). This pattern of results suggests that these receptors cooperatively regulate learning to inhibit a previously learned response. The latter study has also shown that D4 receptor blockade improved learning, exerting an effect opposite to the other receptors (Floresco et al., 2006). In fear extinction studies, both D1 and D2 receptor antagonists infused in mPFC prevented extinction learning in adult rats (Hikind and Maroun, 2008; Mueller et al., 2010), and D2 agonist quinpirole improved long-term extinction in adolescent rats (Zbukvic et al., 2017). In a simple decision making task where animals had to switch lever pressing from one to another following changes in rewards contingency, both D1 and D2 receptor antagonism increased the number of perseverative lever presses (Winter et al., 2009), suggesting a cooperative action of the two receptors. However, in a more complicated task, in which rats were choosing between a small and sure reward vs. a large and risky one, opposite effect of D1 and D2 receptors was observed (St Onge et al., 2011; Jenni et al., 2017). A similar opposite effect was observed in an instrumental tasks that tested a shift from habitual to goal-directed behavior, thought to be controlled by the mPFC (Barker et al., 2013; Nelson and Killcross, 2013). In particular, in rats and mice overtrained to acquire habitual responses (that were therefore insensitive to contingency degradation and goal devaluation procedures, respectively), D1 antagonist or D2 agonist restored goal-directed behavior, whereas D2 antagonists facilitated habitual responding. A first conclusion that may be derived from the above data is that the role of D1 and D2 receptors in behavioral flexibility differs from their role in working memory, where opposite effects of these receptors on performance have never been observed (El-Ghundi et al., 2007). We therefore propose that antagonistic action of the two receptor classes on behavior is a hallmark of the implication of long-term memory in this behavior. A second conclusion is that the antagonism of the two receptor classes has been mostly observed in tasks where the link between actions and rewards is learned, rather than inhibition of the previously acquired response. The latter can be more dependent on the cooperative action of the two receptor classes, suggesting an important role of LTD.
To conclude, mPFC is critically involved in many cognitive processes, components of executive functions. Most of these processes at some stage rely on long-term memory and synaptic plasticity in PFC neurons, as a large number of experimental studies have demonstrated empirically and most theoretical models have (usually tacitly) assumed. Despite this ubiquitous dependence of executive functions on neuronal mechanisms underlying long-term storage of information, their role is underestimated in current PFC research. It is important to study them, because disturbances in the long-term memory component of executive functions may cause long-term consequences of PFC-dependent mental or age-related disorders. Given a wealth of theoretical models addressing the role of dopaminergic modulation of working, as opposed to long-term, memory, as well as its interactions with striatal control of actions, a challenge for future computational theories is to link these models with prefrontal cortical machinery for storage of long-term memories.
DS wrote the manuscript with input from SO and JB. AA provided critical feedback and helped shape the research. All authors contributed to the article and approved the submitted version.
This study was supported by the ANR–Essilor SilverSight Chair (ANR-14-CHIN-0001 and ANR-18-CHIN-0002), LabEx LIFESENSES (ANR-10-LABX-65), and IHU FOReSIGHT (ANR-18-IAHU-01).
The authors declare that the research was conducted in the absence of any commercial or financial relationships that could be construed as a potential conflict of interest.
All claims expressed in this article are solely those of the authors and do not necessarily represent those of their affiliated organizations, or those of the publisher, the editors and the reviewers. Any product that may be evaluated in this article, or claim that may be made by its manufacturer, is not guaranteed or endorsed by the publisher.
Akirav, I., and Maroun, M. (2006). Ventromedial prefrontal cortex is obligatory for consolidation and reconsolidation of object recognition memory. Cereb. Cortex 16, 1759–1765. doi: 10.1093/cercor/bhj114
Alexander, W. H., and Brown, J. W. (2011). Medial prefrontal cortex as an action-outcome predictor. Nat. Neurosci. 14, 1338–1344. doi: 10.1038/nn.2921
Amit, D. J., and Brunel, N. (1997). Model of global spontaneous activity and local structured activity during delay periods in the cerebral cortex. Cereb. Cortex 7, 237–252. doi: 10.1093/cercor/7.3.237
Arleo, A., and Rondi-Reig, L. (2007). Multimodal sensory integration and concurrent navigation strategies for spatial cognition in real and artificial organisms. J. Integr. Neurosci. 6, 327–366. doi: 10.1142/S0219635207001593
Arnsten, A. F. T. (2013). The neurobiology of thought: the groundbreaking discoveries of Patricia Goldman-Rakic 1937-2003. Cereb. Cortex 23, 2269–2281. doi: 10.1093/cercor/bht195
Atkinson, R. C., and Shiffrin, R. M. (1971). The control processes of short-term memory. Technical report, Stahford University, Stanford, CA.
Auclair, N., Otani, S., Soubrie, P., and Crepel, F. (2000). Cannabinoids modulate synaptic strength and plasticity at glutamatergic synapses of rat prefrontal cortex pyramidal neurons. J. Neurophysiol. 83, 3287–3293. doi: 10.1152/jn.2000.83.6.3287
Baeg, E., Kim, Y., Huh, K., Mook-Jung, I., Kim, H., and Jung, M. (2003). Dynamics of population code for working memory in the prefrontal cortex. Neuron 40, 177–188. doi: 10.1016/S0896-6273(03)00597-X
Bai, J., Blot, K., Tzavara, E., Nosten-Bertrand, M., Giros, B., and Otani, S. (2014). Inhibition of dopamine transporter activity impairs synaptic depression in rat prefrontal cortex through over-stimulation of D1 receptors. Cereb. Cortex 24, 945–955. doi: 10.1093/cercor/bhs376
Bai, J., Gorgievski, V., Tzavara, E., Nosten-Bertrand, M., Giros, B., and Otani, S. (2010). Dopamine transporter (DAT) activity regulates the inductionof synaptic plasticity in rodent prefrontal cortex. Schizophr. Res. 117, 507. doi: 10.1016/j.schres.2010.02.968
Balleine, B. W., and O'Doherty, J. P. (2010). Human and rodent homologies in action control: corticostriatal determinants of goal-directed and habitual action. Neuropsychopharmacology 35, 48–69. doi: 10.1038/npp.2009.131
Barker, J. M., Torregrossa, M. M., and Taylor, J. R. (2013). Bidirectional modulation of infralimbic dopamine D1 and D2 receptor activity regulates flexible reward seeking. Front. Neurosci. 7, 126. doi: 10.3389/fnins.2013.00126
Bear, M., Cooper, L., and Ebner, F. (1987). A physiological basis for a theory of synapse modification. Science 237, 42–48. doi: 10.1126/science.3037696
Beaulieu, J.-M., and Gainetdinov, R. R. (2011). The physiology, signaling, and pharmacology of dopamine receptors. Pharmacol Rev. 63, 182–217. doi: 10.1124/pr.110.002642
Benchenane, K., Peyrache, A., Khamassi, M., Tierney, P. L., Gioanni, Y., Battaglia, F. P., et al. (2010). Coherent theta oscillations and reorganization of spike timing in the hippocampal-prefrontal network upon learning. Neuron 66, 921–936. doi: 10.1016/j.neuron.2010.05.013
Bender, V. A., Bender, K. J., Brasier, D. J., and Feldman, D. E. (2006). Two coincidence detectors for spike timing-dependent plasticity in somatosensory cortex. J. Neurosci. 26, 4166–4177. doi: 10.1523/JNEUROSCI.0176-06.2006
Berg, E. A. (1948). A simple objective technique for measuring flexibility in thinking. J. Gen. Psychol. 39, 15–22. doi: 10.1080/00221309.1948.9918159
Bi, G. -Q., and Poo, M. -M. (1998). Synaptic modifications in cultured hippocampal neurons: dependence on spike timing, synaptic strength, and postsynaptic cell type. J. Neurosci. 18, 10464–10472. doi: 10.1523/JNEUROSCI.18-24-10464.1998
Bienenstock, E. L., Cooper, L. N., and Munro, P. W. (1982). Theory for the development of neuron selectivity: orientation specificity and binocular interaction in visual cortex. J. Neurosci. 2, 32–48. doi: 10.1523/JNEUROSCI.02-01-00032.1982
Birrell, J. M., and Brown, V. J. (2000). Medial frontal cortex mediates perceptual attentional set shifting in the rat. J. Neurosci. 20, 4320–4324. doi: 10.1523/JNEUROSCI.20-11-04320.2000
Bissonette, G. B., Powell, E. M., and Roesch, M. R. (2013). Neural structures underlying set-shifting: roles of medial prefrontal cortex and anterior cingulate cortex. Behav. Brain Res. 250, 91–101. doi: 10.1016/j.bbr.2013.04.037
Bjerke, I. E., Cullity, E. R., Kjelsberg, K., Charan, K. M., Leergaard, T. B., and Kim, J. H. (2022). DOPAMAP, high-resolution images of dopamine 1 and 2 receptor expression in developing and adult mouse brains. Scientific Data 9, 175. doi: 10.1038/s41597-022-01268-8
Björklund, A., and Dunnett, S. B. (2007). Dopamine neuron systems in the brain: an update. Trends Neurosci. 30, 194–202. doi: 10.1016/j.tins.2007.03.006
Bliss, T. V., and Collingridge, G. L. (1993). A synaptic model of memory: long-term potentiation in the hippocampus. Nature 361, 31–39. doi: 10.1038/361031a0
Blumenfeld, R. S., and Ranganath, C. (2007). Prefrontal cortex and long-term memory encoding: an integrative review of findings from neuropsychology and neuroimaging. Neuroscientist 13, 280–291. doi: 10.1177/1073858407299290
Botvinick, M. M. (2008). Hierarchical models of behavior and prefrontal function. Trends Cogn. Sci. 12, 201–208. doi: 10.1016/j.tics.2008.02.009
Bouton, M. E., Todd, T. P., Vurbic, D., and Winterbauer, N. E. (2011). Renewal after the extinction of free operant behavior. Learn. Behav. 39, 57–67. doi: 10.3758/s13420-011-0018-6
Brown, V. J., and Bowman, E. M. (2002). Rodent models of prefrontal cortical function. Trends Neurosci. 25, 340–343. doi: 10.1016/S0166-2236(02)02164-1
Brzosko, Z., Schultz, W., and Paulsen, O. (2015). Retroactive modulation of spike timing-dependent plasticity by dopamine. eLife 4, e09685. doi: 10.7554/eLife.09685.017
Burette, F., Jay, T. M., and Laroche, S. (1997). Reversal of LTP in the hippocampal afferent fiber system to the prefrontal cortex in vivo with low-frequency patterns of stimulation that do not produce LTD. J. Neurophysiol. 78, 1155–1160. doi: 10.1152/jn.1997.78.2.1155
Burgos-Robles, A., Vidal-Gonzalez, I., Santini, E., and Quirk, G. J. (2007). Consolidation of fear extinction requires NMDA receptor-dependent bursting in the ventromedial prefrontal cortex. Neuron 53, 871–880. doi: 10.1016/j.neuron.2007.02.021
Burnham, K., Bannerman, D., Dawson, L., Southam, E., Sharp, T., and Baxter, M. (2010). Fos expression in the brains of rats performing an attentional set-shifting task. Neuroscience 171, 485–495. doi: 10.1016/j.neuroscience.2010.09.008
Camperi, M., and Wang, X. J. (1998). A model of visuospatial working memory in prefrontal cortex: recurrent network and cellular bistability. J. Comput. Neurosci. 5, 383–405. doi: 10.1023/A:1008837311948
Carballo-Márquez, A., Vale-Martínez, A., Guillazo-Blanch, G., Torras-Garcia, M., Boix-Trelis, N., and Martí-Nicolovius, M. (2007). Differential effects of muscarinic receptor blockade in prelimbic cortex on acquisition and memory formation of an odor-reward task. Learn. Mem. 14, 616–624. doi: 10.1101/lm.597507
Carlén, M. (2017). What constitutes the prefrontal cortex? Science 358, 478–482. doi: 10.1126/science.aan8868
Caruana, D. A., Warburton, E. C., and Bashir, Z. I. (2011). Induction of activity-dependent LTD requires muscarinic receptor activation in medial prefrontal cortex. J. Neurosci. 31, 18464–18478. doi: 10.1523/JNEUROSCI.4719-11.2011
Chen, L., Bohanick, J. D., Nishihara, M., Seamans, J. K., and Yang, C. R. (2007). Dopamine D1/5 receptor-mediated long-term potentiation of intrinsic excitability in rat prefrontal cortical neurons: Ca2+-dependent intracellular signaling. J. Neurophysiol. 97, 2448–2464. doi: 10.1152/jn.00317.2006
Chiu, C. Q., Puente, N., Grandes, P., and Castillo, P. E. (2010). Dopaminergic modulation of endocannabinoid-mediated plasticity at GABAergic synapses in the prefrontal cortex. J. Neurosci. 30, 7236–7248. doi: 10.1523/JNEUROSCI.0736-10.2010
Chudasama, Y. (2011). Animal models of prefrontal-executive function. Behav. Neurosci. 125, 327–343. doi: 10.1037/a0023766
Clopath, C., Ziegler, L., Vasilaki, E., Büsing, L., and Gerstner, W. (2008). Tag-trigger-consolidation: a model of early and late long-term-potentiation and depression. PLoS Comput. Biol. 4, e1000248. doi: 10.1371/journal.pcbi.1000248
Collins, A. G. E., and Frank, M. J. (2014). Opponent actor learning (OpAL): Modeling interactive effects of striatal dopamine on reinforcement learning and choice incentive. Psychol. Rev. 121, 337–366. doi: 10.1037/a0037015
Compte, A., Brunet, N., Goldman-Rakic, P. S., and Wang, X.-J. (2000). Synaptic mechanisms and network dynamics underlying spatial working memory in a cortical network model. Cereb. Cortex 10, 910–923. doi: 10.1093/cercor/10.9.910
Cooper, L. N., Intrator, N., Blais, B. S., and Shouval, H. Z. (2004). Theory of Cortical Plasticity. Signapore: World Scientific.
Cooper, R. P., and Shallice, T. (2006). Hierarchical schemas and goals in the control of sequential behavior. Psychol. Rev. 113, 887–916. doi: 10.1037/0033-295X.113.4.887
Coppa-Hopman, R., Galle, J., and Pimkine, D. (2009). D1 receptor antagonist-induced long-term depression in the medial prefrontal cortex of rat, in vivo: an animal model of psychiatric hypofrontality. J. Psychopharmacol. 23, 672–685. doi: 10.1177/0269881108091256
Corbit, L. H., and Balleine, B. W. (2003). The role of prelimbic cortex in instrumental conditioning. Behav. Brain Res. 146, 145–157. doi: 10.1016/j.bbr.2003.09.023
Couey, J. J., Meredith, R. M., Spijker, S., Poorthuis, R. B., Smit, A. B., Brussaard, A. B., et al. (2007). Distributed network actions by nicotine increase the threshold for spike-timing-dependent plasticity in prefrontal cortex. Neuron 54, 73–87. doi: 10.1016/j.neuron.2007.03.006
Cowan, N. (2008). Chapter 20 What are the differences between long-term, short-term, and working memory? Prog. Brain Res. 169, 323–338. doi: 10.1016/S0079-6123(07)00020-9
Creese, I., Sibley, D. R., Hamblin, M. W., and Leff, S. E. (1983). The classification of dopamine receptors: relationship to radioligand binding. Annu. Rev. Neurosci. 6, 43–71. doi: 10.1146/annurev.ne.06.030183.000355
Cui, Y., Jin, J., Zhang, X., Xu, H., Yang, L., Du, D., et al. (2011). Forebrain NR2B overexpression facilitating the prefrontal cortex long-term potentiation and enhancing working memory function in mice. PLoS ONE 6, e20312. doi: 10.1371/journal.pone.0020312
Cullity, E. R., Madsen, H. B., Perry, C. J., and Kim, J. H. (2019). Postnatal developmental trajectory of dopamine receptor 1 and 2 expression in cortical and striatal brain regions. J. Compar. Neurol. 527, 1039–1055. doi: 10.1002/cne.24574
Dalley, J. W., Cardinal, R. N., and Robbins, T. W. (2004). Prefrontal executive and cognitive functions in rodents: neural and neurochemical substrates. Neurosci. Biobehav. Rev. 28, 771–784. doi: 10.1016/j.neubiorev.2004.09.006
Davis, H. P., and Squire, L. R. (1984). Protein synthesis and memory: a review. Psychol. Bull. 96, 518–559. doi: 10.1037/0033-2909.96.3.518
Davis, S., Rodger, J., Hicks, A., Mallet, J., and Laroche, S. (1996). Brain structure and task-specific increase in expression of the gene encoding syntaxin 1B during learning in the rat: a potential molecular marker for learning-induced synaptic plasticity in neural networks. Eur. J. Neurosci. 8, 2068–2074. doi: 10.1111/j.1460-9568.1996.tb00727.x
Daw, N. D., Niv, Y., and Dayan, P. (2005). Uncertainty-based competition between prefrontal and dorsolateral striatal systems for behavioral control. Nat. Neurosci. 8, 1704–1711. doi: 10.1038/nn1560
Dayan, P., and Niv, Y. (2008). Reinforcement learning: the good, the bad and the ugly. Curr. Opin. Neurobiol. 18, 185–196. doi: 10.1016/j.conb.2008.08.003
de Bruin, J. P., Sanchez-Santed, F., Heinsbroek, R. P., Donker, A., and Postmes, P. (1994). A behavioural analysis of rats with damage to the medial prefrontal cortex using the morris water maze: evidence for behavioural flexibility, but not for impaired spatial navigation. Brain Res. 652, 323–333. doi: 10.1016/0006-8993(94)90243-7
Dehaene, S., and Changeux, J. P. (2000). Reward-dependent learning in neuronal networks for planning and decision making. Prog. Brain Res. 126, 217–229. doi: 10.1016/S0079-6123(00)26016-0
DeSteno, D., and Schmauss, C. (2008). Induction of early growth response gene 2 expression in the forebrain of mice performing an attention-set-shifting task. Neuroscience 152, 417–428. doi: 10.1016/j.neuroscience.2008.01.012
Dolan, R. J., and Dayan, P. (2013). Goals and Habits in the Brain. Neuron 80, 312–325. doi: 10.1016/j.neuron.2013.09.007
Dollé, L., Sheynikhovich, D., Girard, B., Chavarriaga, R., and Guillot, A. (2010). Path planning versus cue responding: a bio-inspired model of switching between navigation strategies. Biol. Cybern. 103, 299–317. doi: 10.1007/s00422-010-0400-z
Doyère, V., Burette, F., Rédini-Del Negro, C., and Laroche, S. (1993). Long-term potentiation of hippocampal afferents and efferents to prefrontal cortex: implications for associative learning. Neuropsychologia 31, 1031–1053. doi: 10.1016/0028-3932(93)90031-T
Dreher, J.-C., and Burnod, Y. (2002). An integrative theory of the phasic and tonic modes of dopamine modulation in the prefrontal cortex. Neural Netw. 15, 583–602. doi: 10.1016/S0893-6080(02)00051-5
Durstewitz, D., Seamans, J. K., and Sejnowski, T. J. (2000). Neurocomputational models of working memory. Nat. Neurosci. 3, 1184–1191. doi: 10.1038/81460
Durstewitz, D., Vittoz, N. M., Floresco, S. B., and Seamans, J. K. (2010). Abrupt transitions between prefrontal neural ensemble states accompany behavioral transitions during rule learning. Neuron 66, 438–448. doi: 10.1016/j.neuron.2010.03.029
El-Ghundi, M., O'Dowd, B., and George, S. (2007). Insights into the role of dopamine receptor systems in learning and memory. Rev. Neurosci. 18, 37–66. doi: 10.1515/REVNEURO.2007.18.1.37
Ellwood, I. T., Patel, T., Wadia, V., Lee, A. T., Liptak, A. T., Bender, K. J., et al. (2017). Tonic or phasic stimulation of dopaminergic projections to prefrontal cortex causes mice to maintain or deviate from previously learned behavioral strategies. J. Neurosci. 37, 8315–8329. doi: 10.1523/JNEUROSCI.1221-17.2017
Euston, D. R., Gruber, A. J., and McNaughton, B. L. (2012). The role of medial prefrontal cortex in memory and decision making. Neuron 76, 1057–1070. doi: 10.1016/j.neuron.2012.12.002
Fino, E., Glowinski, J., and Venance, L. (2005). Bidirectional activity-dependent plasticity at corticostriatal synapses. J. Neurosci. 25, 11279–11287. doi: 10.1523/JNEUROSCI.4476-05.2005
Floresco, S. B., Magyar, O., Ghods-Sharifi, S., Vexelman, C., and Tse, M. T. L. (2006). Multiple dopamine receptor subtypes in the medial prefrontal cortex of the rat regulate set-shifting. Neuropsychopharmacology 31, 297–309. doi: 10.1038/sj.npp.1300825
Floresco, S. B., Seamans, J. K., and Phillips, A. G. (1997). Selective roles for hippocampal, prefrontal cortical, and ventral striatal circuits in radial-arm maze tasks with or without a delay. J. Neurosci. 17, 1880–1890. doi: 10.1523/JNEUROSCI.17-05-01880.1997
Floresco, S. B., West, A. R., Ash, B., Moore, H., and Grace, A. A. (2003). Afferent modulation of dopamine neuron firing differentially regulates tonic and phasic dopamine transmission. Nat. Neurosci. 6, 968–973. doi: 10.1038/nn1103
Floresco, S. B., Zhang, Y., and Enomoto, T. (2009). Neural circuits subserving behavioral flexibility and their relevance to schizophrenia. Behav. Brain Res. 204, 396–409. doi: 10.1016/j.bbr.2008.12.001
Frankland, P. W., and Bontempi, B. (2005). The organization of recent and remote memories. Nat. Rev. Neurosci. 6, 119–130. doi: 10.1038/nrn1607
Frankland, P. W., Bontempi, B., Talton, L. E., Kaczmarek, L., and Silva, A. J. (2004). The involvement of the anterior cingulate cortex in remote contextual fear memory. Science 304, 881–883. doi: 10.1126/science.1094804
Frankland, P. W., O'Brien, C., Ohno, M., Kirkwood, A., and Silva, A. J. (2001). Alpha-CaMKII-dependent plasticity in the cortex is required for permanent memory. Nature 411, 309–313. doi: 10.1038/35077089
Frémaux, N., and Gerstner, W. (2015). Neuromodulated spike-timing-dependent plasticity, and theory of three-factor learning rules. Front. Neural Circ. 9, 85. doi: 10.3389/fncir.2015.00085
Frey, U., and Morris, R. G. (1997). Synaptic tagging and long-term potentiation. Nature 385, 533–536. doi: 10.1038/385533a0
Fries, P. (2005). A mechanism for cognitive dynamics: neuronal communication through neuronal coherence. Trends Cogn. Sci. 9, 474–480. doi: 10.1016/j.tics.2005.08.011
Frohardt, R. J., Guarraci, F. A., and Bouton, M. E. (2000). The effects of neurotoxic hippocampal lesions on two effects of context after fear extinction. Behav. Neurosci. 114, 227–240. doi: 10.1037/0735-7044.114.2.227
Fuster, J. M., and Alexander, G. E. (1971). Neuron activity related to short-term memory. Science 173, 652–654. doi: 10.1126/science.173.3997.652
Garris, P., and Wightman, R. (1994). Different kinetics govern dopaminergic transmission in the amygdala, prefrontal cortex, and striatum: an in vivo voltammetric study. J. Neurosci. 14, 442–450. doi: 10.1523/JNEUROSCI.14-01-00442.1994
Gaspar, P., Bloch, B., and Moine, C. (1995). D1 and D2 receptor gene expression in the rat frontal cortex: cellular localization in different classes of efferent neurons. Eur. J. Neurosci. 7, 1050–1063. doi: 10.1111/j.1460-9568.1995.tb01092.x
Gass, J. T., Glen, W. B., McGonigal, J. T., Trantham-Davidson, H., Lopez, M. F., Randall, P. K., et al. (2014). Adolescent alcohol exposure reduces behavioral flexibility, promotes disinhibition, and increases resistance to extinction of ethanol self-administration in adulthood. Neuropsychopharmacology 39, 2570–2583. doi: 10.1038/npp.2014.109
Gemmell, C., and O'Mara, S. M. (2000). Long-term potentiation and paired-pulse facilitation in the prelimbic cortex of the rat following stimulation in the contralateral hemisphere in vivo. Exp. Brain Res. 132, 223–229. doi: 10.1007/s002210000334
Gerstner, W., Lehmann, M., Liakoni, V., Corneil, D., and Brea, J. (2018). Eligibility traces and plasticity on behavioral time scales: experimental support of neohebbian three-factor learning rules. Front. Neural Circ. 12, 53. doi: 10.3389/fncir.2018.00053
Gilbert, S. J., and Burgess, P. W. (2008). Executive function. Curr. Biol. 18, R110-R114. doi: 10.1016/j.cub.2007.12.014
Gisquet-Verrier, P., and Delatour, B. (2006). The role of the rat prelimbic/infralimbic cortex in working memory: not involved in the short-term maintenance but in monitoring and processing functions. Neuroscience 141, 585–596. doi: 10.1016/j.neuroscience.2006.04.009
Goldman-Rakic, P. S. (1995). Cellular basis of working memory. Neuron 14, 477–485. doi: 10.1016/0896-6273(95)90304-6
Goriounova, N. A., and Mansvelder, H. D. (2012). Nicotine exposure during adolescence leads to short- and long-term changes in spike timing-dependent plasticity in rat prefrontal cortex. J. Neurosci. 32, 10484–10493. doi: 10.1523/JNEUROSCI.5502-11.2012
Goto, Y., and Grace, A. A. (2005). Dopaminergic modulation of limbic and cortical drive of nucleus accumbens in goal-directed behavior. Nat. Neurosci. 8, 805–812. doi: 10.1038/nn1471
Grace, A. A., Floresco, S. B., Goto, Y., and Lodge, D. J. (2007). Regulation of firing of dopaminergic neurons and control of goal-directed behaviors. Trends Neurosci. 30, 220–227. doi: 10.1016/j.tins.2007.03.003
Granon, S., and Floresco, S. (2009). “Functional neuroanatomy of flexible behaviors in mice and rats,” in Endophenotypes of Psychiatric and Neurodegenerative Disorders in Rodent Models, ed S. Granon (Kerala: Transworld Research Network), 83–103.
Gurden, H., Takita, M., and Jay, T. M. (2000). Essential role of D1 But Not D2 Receptors in the NMDA receptor-dependent long-term potentiation at hippocampal-prefrontal cortex synapses in vivo. J. Neurosci. 20, RC106. doi: 10.1523/JNEUROSCI.20-22-j0003.2000
Gurden, H., Tassin, J. P., and Jay, T. M. (1999). Integrity of the mesocortical dopaminergic system is necessary for complete expression of in vivo hippocampal-prefrontal cortex long-term potentiation. Neuroscience. 94, 1019–1027. doi: 10.1016/S0306-4522(99)00395-4
Hart, G., and Balleine, B. W. (2016). Consolidation of goal-directed action depends on MAPK/ERK signaling in rodent prelimbic cortex. J. Neurosci. 36, 11974–11986. doi: 10.1523/JNEUROSCI.1772-16.2016
Hasselmo, M. E. (2005). A model of prefrontal cortical mechanisms for goal-directed behavior. J. Cogn. Neurosci. 17, 1115–1129. doi: 10.1162/0898929054475190
Heifets, B. D., and Castillo, P. E. (2009). Endocannabinoid signaling and long-term synaptic plasticity. Annu. Rev. Physiol. 71, 283–306. doi: 10.1146/annurev.physiol.010908.163149
Herry, C., and Garcia, R. (2002). Prefrontal Cortex Long-term potentiation, but not long-term depression, is associated with the maintenance of extinction of learned fear in mice. J. Neurosci. 22, 577–583. doi: 10.1523/JNEUROSCI.22-02-00577.2002
Herry, C., Vouimba, R.-M., and Garcia, R. (1999). Plasticity in the mediodorsal thalamo-prefrontal cortical transmission in behaving mice. J. Neurophysiol. 82, 2827–2832. doi: 10.1152/jn.1999.82.5.2827
Hikind, N., and Maroun, M. (2008). Microinfusion of the D1 receptor antagonist, SCH23390 into the IL but not the BLA impairs consolidation of extinction of auditory fear conditioning. Neurobiol. Learn. Mem. 90, 217–222. doi: 10.1016/j.nlm.2008.03.003
Hirsch, J. C., and Crepel, F. (1990). Use-dependent changes in synaptic efficacy in rat prefrontal neurons in vitro. J. Physiol. 427, 31–49. doi: 10.1113/jphysiol.1990.sp018159
Hirsch, J. C., and Crepel, F. (1991). Blockade of NMDA receptors unmasks a long-term depression in synaptic efficacy in rat prefrontal neurons in vitro. Exp. Brain Res. 85, 621–624. doi: 10.1007/BF00231747
Hirsch, J. C., Crépel, F., and Crepel, F. (1992). Postsynaptic calcium is necessary for the induction of LTP and LTD of monosynaptic EPSPs in prefrontal neurons : an in vitro study in the rat. Synapse 10, 173–175. doi: 10.1002/syn.890100211
Hitchcott, P. K., Quinn, J. J., and Taylor, J. R. (2007). Bidirectional modulation of goal-directed actions by prefrontal cortical dopamine. Cereb. Cortex 17, 2820–2827. doi: 10.1093/cercor/bhm010
Hok, V., Save, E., Lenck-Santini, P.-P. P., and Poucet, B. (2005). Coding for spatial goals in the prelimbic/infralimbic area of the rat frontal cortex. Proc. Natl. Acad. Sci. U.S.A. 102, 4602–4607. doi: 10.1073/pnas.0407332102
Hollerman, J. R., and Schultz, W. (1998). Dopamine neurons report an error in the temporal prediction of reward during learning. Nat. Neurosci. 1, 304–309. doi: 10.1038/1124
Holroyd, C. B., and Mcclure, S. M. (2015). Hierarchical control over effortful behavior by rodent medial frontal cortex. Psychol. Rev. 122, 54–83. doi: 10.1037/a0038339
Hoover, W. B., and Vertes, R. P. (2007). Anatomical analysis of afferent projections to the medial prefrontal cortex in the rat. Brain Struct. Funct. 212, 149–179. doi: 10.1007/s00429-007-0150-4
Horst, N., and Laubach, M. (2009). The role of rat dorsomedial prefrontal cortex in spatial working memory. Neuroscience 164, 444–456. doi: 10.1016/j.neuroscience.2009.08.004
Huang, C.-C., and Hsu, K.-S. (2010). Activation of Muscarinic acetylcholine receptors induces a nitric oxide-dependent long-term depression in rat medial prefrontal cortex. Cereb. Cortex 20, 982–996. doi: 10.1093/cercor/bhp161
Huang, C.-C., Lin, H.-J., and Hsu, K.-S. (2007). Repeated cocaine administration promotes long-term potentiation induction in rat medial prefrontal cortex. Cereb. Cortex 17, 1877–1888. doi: 10.1093/cercor/bhl096
Huang, Y.-Y., Simpson, E., Kellendonk, C., and Kandel, E. R. (2004). Genetic evidence for the bidirectional modulation of synaptic plasticity in the prefrontal cortex by D1 receptors. Proc. Natl. Acad. Sci. U.S.A. 101, 3236–3241. doi: 10.1073/pnas.0308280101
Huang, Y. Y., and Kandel, E. R. (1995). D1/D5 receptor agonists induce a protein synthesis-dependent late potentiation in the CA1 region of the hippocampus. Proc. Natl. Acad. Sci. U.S.A. 92, 2446–2450. doi: 10.1073/pnas.92.7.2446
Hugues, S., Chessel, A., Lena, I., Marsault, R., and Garcia, R. (2006). Prefrontal infusion of PD098059 immediately after fear extinction training blocks extinction-associated prefrontal synaptic plasticity and decreases prefrontal ERK2 phosphorylation. Synapse 60, 280–287. doi: 10.1002/syn.20291
Hugues, S., and Garcia, R. (2007). Reorganization of learning-associated prefrontal synaptic plasticity between the recall of recent and remote fear extinction memory. Learn. Mem. 14, 520–524. doi: 10.1101/lm.625407
Hyman, J. M., Ma, L., Balaguer-Ballester, E., Durstewitz, D., and Seamans, J. K. (2012). Contextual encoding by ensembles of medial prefrontal cortex neurons. Proc. Natl. Acad. Sci. U.S.A. 109, 5086–5091. doi: 10.1073/pnas.1114415109
Izaki, Y., Hori, K., and Nomura, M. (2000). Disturbance of rat lever-press learning by hippocampo-prefrontal disconnection. Brain Res. 860, 199–202. doi: 10.1016/S0006-8993(00)02039-4
Izaki, Y., Takita, M., and Akema, T. (2003). Compatibility of bidirectional synaptic plasticity on hippocampo-prefrontal cortex pathway in rats. Neurosci. Lett. 345, 69–71. doi: 10.1016/S0304-3940(03)00492-0
Izaki, Y., Takita, M., and Nomura, M. (2002). Local properties of CA1 region in hippocampo-prefrontal synaptic plasticity in rats. Neuroreport 13, 469–472. doi: 10.1097/00001756-200203250-00022
Jay, T. (2003). Dopamine: a potential substrate for synaptic plasticity and memory mechanisms. Progress in Neurobiology 69, 375–390. doi: 10.1016/S0301-0082(03)00085-6
Jay, T., Burette, F., and Laroche, S. (1996). Plasticity of the hippocampal-prefrontal cortex synapses. J. Physiol. Paris 90, 361–366. doi: 10.1016/S0928-4257(97)87920-X
Jay, T. M., Gurden, H., and Yamaguchi, T. (1998). Rapid increase in PKA activity during long-term potentiation in the hippocampal afferent fibre system to the prefrontal cortex in vivo. Eur. J. Neurosci. 10, 3302–3306. doi: 10.1046/j.1460-9568.1998.00389.x
Jenni, N. L., Larkin, J. D., and Floresco, S. B. (2017). Prefrontal dopamine D1 and D2 receptors regulate dissociable aspects of decision making via distinct ventral striatal and amygdalar circuits. J. Neurosci. 37, 6200–6213. doi: 10.1523/JNEUROSCI.0030-17.2017
Käenmäki, M., Tammimäki, A., Myöhänen, T., Pakarinen, K., Amberg, C., Karayiorgou, M., et al. (2010). Quantitative role of COMT in dopamine clearance in the prefrontal cortex of freely moving mice. J. Neurochem. 114, 1745–1755. doi: 10.1111/j.1471-4159.2010.06889.x
Karmarkar, U. R., and Buonomano, D. V. (2002). A model of spike-timing dependent plasticity: one or two coincidence detectors? J. Neurophysiol. 88, 507–513. doi: 10.1152/jn.2002.88.1.507
Kesner, R. P. (2000). Subregional analysis of mnemonic functions of the prefrontal cortex in the rat. Psychology 28, 219–228. doi: 10.3758/BF03331980
Kesner, R. P., and Rogers, J. (2004). An analysis of independence and interactions of brain substrates that subserve multiple attributes, memory systems, and underlying processes. Neurobiol. Learn. Mem. 82, 199–215. doi: 10.1016/j.nlm.2004.05.007
Killcross, S., and Coutureau, E. (2003). Coordination of actions and habits in the medial prefrontal cortex of rats. Cereb. Cortex 13, 400–408. doi: 10.1093/cercor/13.4.400
Kim, J., Lee, S., Park, K., Hong, I., Song, B., Son, G., et al. (2007). Amygdala depotentiation and fear extinction. Proc. Natl. Acad. Sci. U.S.A. 104, 20955–20960. doi: 10.1073/pnas.0710548105
Kim, M., Chun, S.-K., Kim, Y., Mook-Jung, I., and Jung, M. (2003). Long-term potentiation in visual cortical projections to the medial prefrontal cortex of the rat. Neuroscience 120, 283–289. doi: 10.1016/S0306-4522(03)00021-6
Kirkwood, A., Rioult, M. G., and Bear, M. F. (1996). Experience-dependent modification of synaptic plasticity in visual cortex. Nature 381, 526–528. doi: 10.1038/381526a0
Kolomiets, B., Marzo, A., Caboche, J., Vanhoutte, P., and Otani, S. (2009). Background dopamine concentration dependently facilitates long-term potentiation in rat prefrontal cortex through postsynaptic activation of extracellular signal-regulated kinases. Cereb. Cortex 19, 2708–2718. doi: 10.1093/cercor/bhp047
Kuroda, M., Yokofujita, J., and Murakami, K. (1998). An ultrastructural study of the neural circuit between the prefrontal cortex and the mediodorsal nucleus of the thalamus. Progr. Neurobiol. 54, 417–458. doi: 10.1016/S0301-0082(97)00070-1
Lafourcade, M., Elezgarai, I., Mato, S., Bakiri, Y., Grandes, P., and Manzoni, O. J. (2007). Molecular components and functions of the endocannabinoid system in mouse prefrontal cortex. PLoS ONE 2, e709. doi: 10.1371/journal.pone.0000709
Lai, C. S. W., Franke, T. F., and Gan, W.-B. (2012). Opposite effects of fear conditioning and extinction on dendritic spine remodelling. Nature. 483, 87–91. doi: 10.1038/nature10792
Lammel, S., Hetzel, A., Häckel, O., Jones, I., Liss, B., and Roeper, J. (2008). Unique properties of mesoprefrontal neurons within a dual mesocorticolimbic dopamine system. Neuron 57, 760–773. doi: 10.1016/j.neuron.2008.01.022
Lara, A. H., and Wallis, J. D. (2015). The role of prefrontal cortex in working memory: a mini review. Front. Syst. Neurosci. 9, 173. doi: 10.3389/fnsys.2015.00173
Laroche, S., Davis, S., and Jay, T. M. (2000). Plasticity at hippocampal to prefrontal cortex synapses: dual roles in working memory and consolidation. Hippocampus 10, 438–446. doi: 10.1002/1098-1063(2000)10:4andlt;438::AID-HIPO10andgt;3.0.CO;2-3
Laroche, S., Jay, T. M., and Thierry, A.-,m. (1990). Long-term potentiation in the prefrontal cortex following stimulation of the hippocampal CA1/subicular region. Neurosci. Lett. 114, 184–190. doi: 10.1016/0304-3940(90)90069-L
Lavin, A., Nogueira, L., Lapish, C. C., Wightman, R. M., Phillips, P. E. M., and Seamans, J. K. (2005). Mesocortical dopamine neurons operate in distinct temporal domains using multimodal signaling. J. Neurosci. 25, 5013–5023. doi: 10.1523/JNEUROSCI.0557-05.2005
Law-Tho, D., Desce, J. M., and Crepel, F. (1995). Dopamine favours the emergence of long-term depression versus long-term potentiation in slices of rat prefrontal cortex. Neurosci. Lett. 188, 125–128. doi: 10.1016/0304-3940(95)11414-R
Lee, S. P., So, C. H., Rashid, A. J., Varghese, G., Cheng, R., Lança, A,. J., et al. (2004). Dopamine D1 and D2 receptor Co-activation generates a novel phospholipase C-mediated calcium signal. J. Biol. Chem. 279, 35671–35678. doi: 10.1074/jbc.M401923200
Leon, W. C., Bruno, M. A., Allard, S., Nader, K., and Cuello, A. C. (2010). Engagement of the PFC in consolidation and recall of recent spatial memory. Learn. Mem. 17, 297–305. doi: 10.1101/lm.1804410
Lesburguères, E., Gobbo, O. L., Alaux-Cantin, S., Hambucken, A., Trifilieff, P., and Bontempi, B. (2011). Early tagging of cortical networks is required for the formation of enduring associative memory. Science 331, 924–928. doi: 10.1126/science.1196164
Li, Y.-C., Xi, D., Roman, J., Huang, Y.-Q., and Gao, W.-J. (2009). Activation of glycogen synthase kinase-3 beta is required for hyperdopamine and D2 receptor-mediated inhibition of synaptic NMDA receptor function in the rat prefrontal cortex. J. Neurosci. 29, 15551–15563. doi: 10.1523/JNEUROSCI.3336-09.2009
Lim, E. P., Tan, C. H., Jay, T. M., and Dawe, G. S. (2010). Locus coeruleus stimulation and noradrenergic modulation of hippocampo-prefrontal cortex long-term potentiation. Int. J. Neuropsychopharmacol. 13, 1219–1231. doi: 10.1017/S1461145709991131
Lisman, J., Grace, A. A., and Duzel, E. (2011). A neoHebbian framework for episodic memory; role of dopamine-dependent late LTP. Trends Neurosci. 34, 536–547. doi: 10.1016/j.tins.2011.07.006
Lisman, J. E. (1989). A mechanism for the Hebb and the anti-Hebb processes underlying learning and memory. Proc. Natl. Acad. Sci. U.S.A. 86, 9574–9578. doi: 10.1073/pnas.86.23.9574
Liu, D., Gu, X., Zhu, J., Zhang, X., Han, Z., Yan, W., et al. (2014). Medial prefrontal activity during delay period contributes to learning of a working memory task. Science 346, 458–463. doi: 10.1126/science.1256573
Louth, E. L., Jørgensen, R. L., Korshoej, A. R., Sørensen, J. C. H., and Capogna, M. (2021). Dopaminergic neuromodulation of spike timing dependent plasticity in mature adult rodent and human cortical neurons. Front. Cell Neurosci. 15, 135. doi: 10.3389/fncel.2021.668980
Malenka, R. C., and Bear, M. F. (2004). LTP and LTD: an embarrassment of riches. Neuron 44, 5–21. doi: 10.1016/j.neuron.2004.09.012
Mamiya, N., Fukushima, H., Suzuki, A., Matsuyama, Z., Homma, S., Frankland, P. W., et al. (2009). Brain region-specific gene expression activation required for reconsolidation and extinction of contextual fear memory. J. Neurosci. 29, 402–413. doi: 10.1523/JNEUROSCI.4639-08.2009
Mansouri, F. A., Matsumoto, K., and Tanaka, K. (2006). Prefrontal cell activities related to monkeys' success and failure in adapting to rule changes in a wisconsin card sorting test analog. J. Neurosci. 26, 2745–2756. doi: 10.1523/JNEUROSCI.5238-05.2006
Maren, S., and Holmes, A. (2016). Stress and fear extinction. Neuropsychopharmacology 41, 58–79. doi: 10.1038/npp.2015.180
Marighetto, A., Valerio, S., Jaffard, R., Mormede, C., Mu noz, C., Bernard, K., et al. (2008). The AMPA modulator S 18986 improves declarative and working memory performances in aged mice. Behav. Pharmacol. 19, 235–244. doi: 10.1097/FBP.0b013e3282feb0c1
Markram, H., Lübke, J., Frotscher, M., and Sakmann, B. (1997). Regulation of synaptic efficacy by coincidence of postsynaptic APs and EPSPs. Science 275, 213–215. doi: 10.1126/science.275.5297.213
Maroun, M., and Richter-Levin, G. (2003). Exposure to acute stress blocks the induction of long-term potentiation of the amygdala-prefrontal cortex pathway in vivo. J. Neurosci. 23, 4406–4409. doi: 10.1523/JNEUROSCI.23-11-04406.2003
Martinet, L.-E., Sheynikhovich, D., Benchenane, K., and Arleo, A. (2011). Spatial learning and action planning in a prefrontal cortical network model. PLoS Comput. Biol. 7, e1002045. doi: 10.1371/journal.pcbi.1002045
Marzo, A., Bai, J., Caboche, J., Vanhoutte, P., and Otani, S. (2010). Cellular mechanisms of long-term depression induced by noradrenaline in rat prefrontal neurons. Neuroscience 169, 74–86. doi: 10.1016/j.neuroscience.2010.04.046
Matsuda, Y., Marzo, A., and Otani, S. (2006). The presence of background dopamine signal converts long-term synaptic depression to potentiation in rat prefrontal cortex. J. Neurosci. 26, 4803–4810. doi: 10.1523/JNEUROSCI.5312-05.2006
Mehta, M. A., Manes, F. F., Magnolfi, G., Sahakian, B. J., and Robbins, T. W. (2004). Impaired set-shifting and dissociable effects on tests of spatial working memory following the dopamine D2 receptor antagonist sulpiride in human volunteers. Psychopharmacology 176, 331–342. doi: 10.1007/s00213-004-1899-2
Milad, M. R., and Quirk, G. J. (2002). Neurons in medial prefrontal cortex signal memory for fear extinction. Nature 420, 70–74. doi: 10.1038/nature01138
Missale, C., Nash, S. R., Robinson, S. W., Jaber, M., and Caron, M. G. (1998). Dopamine receptors : from structure to function. Physiol. Rev. 78, 189–226. doi: 10.1152/physrev.1998.78.1.189
Mongillo, G., Barak, O., and Tsodyks, M. (2008). Synaptic theory of working memory. Science 319, 1543–1546. doi: 10.1126/science.1150769
Morgan, M. A., and LeDoux, J. E. (1995). Differential contribution of dorsal and ventral medial prefrontal cortex to the acquisition and extinction of conditioned fear in rats. Behav. Neurosci. 109, 681–688. doi: 10.1037/0735-7044.109.4.681
Morón, J. A., Brockington, A., Wise, R. A., Rocha, B. A., and Hope, B. T. (2002). Dopamine uptake through the norepinephrine transporter in brain regions with low levels of the dopamine transporter: evidence from knock-out mouse lines. J. Neurosci. 22, 389–395. doi: 10.1523/JNEUROSCI.22-02-00389.2002
Morrow, B. A., Elsworth, J. D., Inglis, F. M., and Roth, R. H. (1999). An antisense oligonucleotide reverses the footshock-induced expression of fos in the rat medial prefrontal cortex and the subsequent expression of conditioned fear-induced immobility. J. Neurosci. 19, 5666–5673. doi: 10.1523/JNEUROSCI.19-13-05666.1999
Mueller, D., Bravo-Rivera, C., and Quirk, G. J. (2010). Infralimbic D2 receptors are necessary for fear extinction and extinction-related tone responses. Biol. Psychiatry. 68, 1055–1060. doi: 10.1016/j.biopsych.2010.08.014
Mulder, A. B., Arts, M. P. M., and Silva, F. H. L. (1997). Short- and long-term plasticity of the hippocampus to nucleus accumbens and prefrontal cortex pathways in the rat, in vivo. Eur. J. Neurosci. 9, 1603–1611. doi: 10.1111/j.1460-9568.1997.tb01518.x
Mulder, A. B., Nordquist, R. E., Örgüt, O., and Pennartz, C. M. (2003). Learning-related changes in response patterns of prefrontal neurons during instrumental conditioning. Behav. Brain Res. 146, 77–88. doi: 10.1016/j.bbr.2003.09.016
Mundorf, M. L., Joseph, J. D., Austin, C. M., Caron, M. G., and Wightman, R. M. (2001). Catecholamine release and uptake in the mouse prefrontal cortex. J. Neurochem. 79, 130–142. doi: 10.1046/j.1471-4159.2001.00554.x
Navakkode, S., Sajikumar, S., and Frey, J. U. (2007). Synergistic requirements for the induction of dopaminergic D1/D5-receptor-mediated LTP in hippocampal slices of rat CA1 in vitro. Neuropharmacology 52, 1547–1554. doi: 10.1016/j.neuropharm.2007.02.010
Nelson, A. J., and Killcross, S. (2013). Accelerated habit formation following amphetamine exposure is reversed by D1, but enhanced by D2, receptor antagonists. Front. Neurosci. 7, 76. doi: 10.3389/fnins.2013.00076
Nomura, S., Bouhadana, M., Morel, C., Faure, P., Cauli, B., Lambolez, B., et al. (2014). Noradrenalin and dopamine receptors both control cAMP-PKA signaling throughout the cerebral cortex. Front. Cell Neurosci. 8, 247. doi: 10.3389/fncel.2014.00247
Ohashi, S., Matsumoto, M., Togashi, H., Ueno, K.-,i., and Yoshioka, M. (2003). The serotonergic modulation of synaptic plasticity in the rat hippocampo-medial prefrontal cortex pathway. Neurosci. Lett. 342, 179–182. doi: 10.1016/S0304-3940(03)00293-3
O'Reilly, R. C. (2006). Biologically based computational models of high-level cognition. Science 314, 91–94. doi: 10.1126/science.1127242
O'Reilly, R. C., and Frank, M. J. (2006). Making Working memory work: a computational model of learning in the prefrontal cortex and basal ganglia. Neural Comput. 18, 283–328. doi: 10.1162/089976606775093909
Otani, S., (Ed.). (2004). Prefrontal Cortex: From Synaptic Plasticity to Cognition. Boston, MA: Springer US.
Otani, S., Auclair, N., Desce, J. M., Roisin, M. P., and Crépel, F. (1999). Dopamine receptors and groups and mGluRs cooperate for long-term depression induction in rat prefrontal cortex through converging postsynaptic activation of kinases. J. Neurosci. 19, 9788–9802. doi: 10.1523/JNEUROSCI.19-22-09788.1999
Otani, S., Bai, J., and Blot, K. (2015). Dopaminergic modulation of synaptic plasticity in rat prefrontal neurons. Neurosci. Bull. 31, 183–190. doi: 10.1007/s12264-014-1507-3
Otani, S., Blond, O., Desce, J. M., and Crépel, F. (1998). Dopamine facilitates long-term depression of glutamatergic transmission in rat prefrontal cortex. Neuroscience 85, 669–676. doi: 10.1016/S0306-4522(97)00677-5
Otani, S., Daniel, H., Takita, M., and Crepel, F. (2002). Long-term depression induced by postsynaptic group II metabotropic glutamate receptors linked to phospholipase C and intracellular calcium rises in rat prefrontal cortex. J. Neurosci. 22, 3434–3444. doi: 10.1523/JNEUROSCI.22-09-03434.2002
Otani, S., Hérve, D., Roisin, M. P., and Crepel, F. (2003). Dopaminergic modulation of long-term synaptic plasticity in rat prefrontal neurons. Cereb. Cortex 13, 1251–1256. doi: 10.1093/cercor/bhg092
Pawlak, V., and Kerr, J. N. D. (2008). Dopamine receptor activation is required for corticostriatal spike-timing-dependent plasticity. J. Neurosci. 28, 2435–2446. doi: 10.1523/JNEUROSCI.4402-07.2008
Pezze, M. A., and Feldon, J. (2004). Mesolimbic dopaminergic pathways in fear conditioning. Prog. Neurobiol. 74, 301–320. doi: 10.1016/j.pneurobio.2004.09.004
Poucet, B., Lenck-Santini, P.-P., Hok, V., Save, E., Banquet, J., Gaussier, P., et al. (2004). Spatial navigation and hippocampal place cell firing: the problem of goal encoding. Rev. Neurosci. 15, 89–107. doi: 10.1515/REVNEURO.2004.15.2.89
Puig, M. V., Rose, J., Schmidt, R., and Freund, N. (2014). Dopamine modulation of learning and memory in the prefrontal cortex: insights from studies in primates, rodents, and birds. Front. Neural Circ. 8, 93. doi: 10.3389/fncir.2014.00093
Quirk, G. J., Garcia, R., and González-Lima, F. (2006). Prefrontal mechanisms in extinction of conditioned fear. Biol. Psychiatry 60, 337–343. doi: 10.1016/j.biopsych.2006.03.010
Quirk, G. J., and Mueller, D. (2008). Neural Mechanisms of extinction learning and retrieval. Neuropsychopharmacology 33, 56–72. doi: 10.1038/sj.npp.1301555
Ragozzino, M. E. (2002). The effects of dopamine D1 receptor blockade in the prelimbic - infralimbic areas on behavioral flexibility. Learn. Mem. 9, 18–28. doi: 10.1101/lm.45802
Ragozzino, M. E., Detrick, S., and Kesner, R. P. (1999). Involvement of the prelimbic-infralimbic areas of the rodent prefrontal cortex in behavioral flexibility for place and response learning. J. Neurosci. 19, 4585–4594. doi: 10.1523/JNEUROSCI.19-11-04585.1999
Rich, E. L., and Shapiro, M. (2009). Rat prefrontal cortical neurons selectively code strategy switches. J. Neurosci. 29, 7208–7219. doi: 10.1523/JNEUROSCI.6068-08.2009
Rich, E. L., and Shapiro, M. L. (2007). Prelimbic/infralimbic inactivation impairs memory for multiple task switches, but not flexible selection of familiar tasks. J. Neurosci. 27, 4747–4755. doi: 10.1523/JNEUROSCI.0369-07.2007
Richfield, E. K., Penney, J. B., and Young, A. B. (1989). Anatomical and affinity state comparisons between dopamine D1 and D2 receptors in the rat central nervous system. Neuroscience. 30, 767–777. doi: 10.1016/0306-4522(89)90168-1
Rolls, E. T., Loh, M., Deco, G., and Winterer, G. (2008). Computational models of schizophrenia and dopamine modulation in the prefrontal cortex. Nat. Rev. Neurosci. 9, 696–709. doi: 10.1038/nrn2462
Rougier, N. P., Noelle, D. C., Braver, T. S., Cohen, J. D., and O'Reilly, R. C. (2005). Prefrontal cortex and flexible cognitive control: rules without symbols. Proc. Natl. Acad. Sci. U.S.A. 102, 7338–7343. doi: 10.1073/pnas.0502455102
Ruan, H., Saur, T., and Yao, W.-D. (2014). Dopamine-enabled anti-Hebbian timing-dependent plasticity in prefrontal circuitry. Front. Neural Circ. 8, 38. doi: 10.3389/fncir.2014.00038
Runyan, J. D. (2004). A role for prefrontal cortex in memory storage for trace fear conditioning. J. Neurosci. 24, 1288–1295. doi: 10.1523/JNEUROSCI.4880-03.2004
Santana, N., Mengod, G., and Artigas, F. (2009). Quantitative analysis of the expression of dopamine D1 and D2 receptors in pyramidal and GABAergic neurons of the rat prefrontal cortex. Cereb. Cortex 19, 849–860. doi: 10.1093/cercor/bhn134
Santini, E. (2004). Consolidation of fear extinction requires protein synthesis in the medial prefrontal cortex. J. Neurosci. 24, 5704–5710. doi: 10.1523/JNEUROSCI.0786-04.2004
Seamans, J. K., Floresco, S. B., and Phillips, A. G. (1998). D1 receptor modulation of hippocampal-prefrontal cortical circuits integrating spatial memory with executive functions in the rat. J. Neurosci. 18, 1613–1621. doi: 10.1523/JNEUROSCI.18-04-01613.1998
Seamans, J. K., Gorelova, N. A., and Yang, C. R. (1997). Contributions of voltage-gated Ca2+ channels in the proximal versus distal dendrites to synaptic integration in prefrontal cortical neurons. J. Neurosci. 17, 5936–5948. doi: 10.1523/JNEUROSCI.17-15-05936.1997
Seamans, J. K., and Yang, C. R. (2004). The principal features and mechanisms of dopamine modulation in the prefrontal cortex. Progr. Neurobiol. 74, 1–58. doi: 10.1016/j.pneurobio.2004.05.006
Seol, G. H., Ziburkus, J., Huang, S., Song, L., Kim, I. T., Takamiya, K., et al. (2007). Neuromodulators control the polarity of spike-timing-dependent synaptic plasticity. Neuron. 55, 919–929. doi: 10.1016/j.neuron.2007.08.013
Servan-Schreiber, D., Printz, H., and Cohen, J. D. (1990). A network model of catecholamine effects: gain, signal-to-noise ratio, and behavior. Science 249, 892–895. doi: 10.1126/science.2392679
Setlow, B., and McGaugh, J. L. (2000). D2 Dopamine receptor blockade immediately post-training enhances retention in hidden and visible platform versions of the water maze. Learn. Mem. 7, 187–191. doi: 10.1101/lm.7.3.187
Shema, R., Sacktor, T. C., and Dudai, Y. (2007). Rapid erasure of long-term memory associations in the cortex by an inhibitor of PKMζ. Science 317, 951–953. doi: 10.1126/science.1144334
Shen, H.-,w., Hagino, Y., Kobayashi, H., Shinohara-Tanaka, K., Ikeda, K., Yamamoto, H., et al. (2004). Regional differences in extracellular dopamine and serotonin assessed by in vivo microdialysis in mice lacking dopamine and/or serotonin transporters. Neuropsychopharmacology 29, 1790–1799. doi: 10.1038/sj.npp.1300476
Shen, W., Flajolet, M., Greengard, P., and Surmeier, D. J. (2008). Dichotomous dopaminergic control of striatal synaptic plasticity. Science 321, 848–851. doi: 10.1126/science.1160575
Sheynikhovich, D., and Arleo, A. (2010). A reinforcement learning approach to model interactions between landmarks and geometric cues during spatial learning. Brain Res. 1365, 35–46. doi: 10.1016/j.brainres.2010.09.091
Sheynikhovich, D., Otani, S., and Arleo, A. (2011). The role of tonic and phasic dopamine for long-term synaptic plasticity in the prefrontal cortex: a computational model. J. Physiol. Paris 105, 45–52. doi: 10.1016/j.jphysparis.2011.08.001
Sheynikhovich, D., Otani, S., and Arleo, A. (2013). Dopaminergic control of long-term depression/long-term potentiation threshold in prefrontal cortex. J. Neurosci. 33, 13914–13926. doi: 10.1523/JNEUROSCI.0466-13.2013
Short, A. K., Bui, V., Zbukvic, I. C., Hannan, A. J., Pang, T. Y., and Kim, J. H. (2022). Sex-dependent effects of chronic exercise on cognitive flexibility but not hippocampal Bdnf in aging mice. Neuronal Signaling 6, 1–14. doi: 10.1042/NS20210053
Shouval, H. Z., Bear, M. F., and Cooper, L. N. (2002). A unified model of NMDA receptor-dependent bidirectional synaptic plasticity. Proc. Natl. Acad. Sci. U.S.A. 99, 10831–10836. doi: 10.1073/pnas.152343099
Singh, A., Peyrache, A., and Humphries, M. D. (2019). Medial prefrontal cortex population activity is plastic irrespective of learning. J. Neurosci. 39, 3470–3483. doi: 10.1523/JNEUROSCI.1370-17.2019
Sirota, A., Montgomery, S., Fujisawa, S., Isomura, Y., Zugaro, M., and Buzsáki, G. (2008). Entrainment of neocortical neurons and gamma oscillations by the hippocampal theta rhythm. Neuron 60, 683–697. doi: 10.1016/j.neuron.2008.09.014
Soltani, A., and Koechlin, E. (2022). Computational models of adaptive behavior and prefrontal cortex. Neuropsychopharmacology 47, 58–71. doi: 10.1038/s41386-021-01123-1
Sotres-Bayon, F., Cain, C. K., and LeDoux, J. E. (2006). Brain mechanisms of fear extinction: historical perspectives on the contribution of prefrontal cortex. Biol. Psychiatry 60, 329–336. doi: 10.1016/j.biopsych.2005.10.012
St Onge, J. R., Abhari, H., and Floresco, S. B. (2011). Dissociable contributions by prefrontal D1 and D2 receptors to risk-based decision making. J. Neurosci. 31, 8625–8633. doi: 10.1523/JNEUROSCI.1020-11.2011
Sun, X., Zhao, Y., and Wolf, M. E. (2005). Dopamine Receptor stimulation modulates AMPA receptor synaptic insertion in prefrontal cortex neurons. J. Neurosci. 25, 7342–7351. doi: 10.1523/JNEUROSCI.4603-04.2005
Swanson, A. M., Depoy, L. M., and Gourley, S. L. (2017). Inhibiting Rho kinase promotes goal-directed decision making and blocks habitual responding for cocaine. Nat. Commun. 8, 1–12. doi: 10.1038/s41467-017-01915-4
Takita, M., Izaki, Y., Jay, T. M., Kaneko, H., and Suzuki, S. S. (1999). Induction of stable long-term depression in vivo in the hippocampal-prefrontal cortex pathway. Eur. J. Neurosci. 11, 4145–4148. doi: 10.1046/j.1460-9568.1999.00870.x
Tanji, J., and Hoshi, E. (2001). Behavioral planning in the prefrontal cortex. Curr. Opin. Neurobiol. 11, 164–170. doi: 10.1016/S0959-4388(00)00192-6
Thierry, A. M., Blanc, G., Sobel, A., Stinus, L., and Glowinski, J. (1973). Dopaminergic terminals in the rat cortex. Science 182, 499–501. doi: 10.1126/science.182.4111.499
Touzani, K., Puthanveettil, S. V., and Kandel, E. R. (2007). Consolidation of learning strategies during spatial working memory task requires protein synthesis in the prefrontal cortex. Proc. Natl. Acad. Sci. U.S.A. 104, 5632–5637. doi: 10.1073/pnas.0611554104
Trantham-Davidson, H., Neely, L. C., Lavin, A., and Seamans, J. K. (2004). Mechanisms underlying differential D1 versus D2 dopamine receptor regulation of inhibition in prefrontal cortex. J. Neurosci. 24, 10652–10659. doi: 10.1523/JNEUROSCI.3179-04.2004
Tronel, S., and Sara, S. J. (2003). Blockade of NMDA Receptors in prelimbic cortex induces an enduring amnesia for odor-reward associative learning. J. Neurosci. 23, 5472–5476. doi: 10.1523/JNEUROSCI.23-13-05472.2003
Tseng, K. Y., and O'Donnell, P. (2004). Dopamine-glutamate interactions controlling prefrontal cortical pyramidal cell excitability involve multiple signaling mechanisms. J. Neurosci. 24, 5131–5139. doi: 10.1523/JNEUROSCI.1021-04.2004
Vickery, R. M., Morris, S. H., and Bindman, L. L. J. (1997). Metabotropic glutamate receptors are involved in long-term potentiation in isolated slices of rat medial frontal cortex. J. Neurophysiol. 78, 3039–3046. doi: 10.1152/jn.1997.78.6.3039
Vincent, S. L., Khan, Y., and Benes, F. M. (1993). Cellular Distribution of dopamine D1 and D2 receptors in rat medial prefrontal cortex. J. Neurosci. 13, 2551–2554. doi: 10.1523/JNEUROSCI.13-06-02551.1993
Vincent, S. L., Khan, Y., and Benes, F. M. (1995). Cellular colocalization of dopamine D1 and D2 receptors in rat medial prefrontal cortex. Synapse 19, 112–120. doi: 10.1002/syn.890190207
Wang, S.-H., Redondo, R. L., and Morris, R. G. M. (2010). Relevance of synaptic tagging and capture to the persistence of long-term potentiation and everyday spatial memory. Proc. Natl. Acad. Sci. U.S.A. 107, 19537–19542. doi: 10.1073/pnas.1008638107
Wang, X., Zhong, P., Gu, Z., and Yan, Z. (2003). Regulation of NMDA receptors by dopamine D 4 signaling in prefrontal cortex. J. Neurosci. 23, 9852–9861. doi: 10.1523/JNEUROSCI.23-30-09852.2003
Wei, X., Ma, T., Cheng, Y., Huang, C. C., Wang, X., Lu, J., et al. (2018). Dopamine D1 or D2 receptor-expressing neurons in the central nervous system. Addict. Biol. 23, 569–584. doi: 10.1111/adb.12512
White, N. M., and McDonald, R. J. (2002). Multiple parallel memory systems in the brain of the rat. Neurobiol. Learn. Mem. 77, 125–184. doi: 10.1006/nlme.2001.4008
Wickens, J., Begg, A., and Arbuthnott, G. (1996). Dopamine reverses the depression of rat corticostriatal synapses which normally follows high-frequency stimulation of cortex in vitro. Neuroscience 70, 1–5. doi: 10.1016/0306-4522(95)00436-M
Winter, S., Dieckmann, M., and Schwabe, K. (2009). Dopamine in the prefrontal cortex regulates rats behavioral flexibility to changing reward value. Behav. Brain Res. 198, 206–213. doi: 10.1016/j.bbr.2008.10.040
Wise, R. A. (2004). Dopamine, learning and motivation. Nat. Rev. Neurosci. 5, 483–494. doi: 10.1038/nrn1406
Wise, S. P. (2008). Forward frontal fields: phylogeny and fundamental function. Trends Neurosci. 31, 599–608. doi: 10.1016/j.tins.2008.08.008
Wood, J. N., and Grafman, J. (2003). Human prefrontal cortex: processing and representational perspectives. Nat. Rev. Neurosci. 4, 139–147. doi: 10.1038/nrn1033
Woon, E. P., Sequeira, M. K., Barbee, B. R., and Gourley, S. L. (2020). Involvement of the rodent prelimbic and medial orbitofrontal cortices in goal-directed action: a brief review. J. Neurosci. Res. 98, 1020–1030. doi: 10.1002/jnr.24567
Xing, B., Li, Y. C., and Gao, W. J. (2016). Norepinephrine versus dopamine and their interaction in modulating synaptic function in the prefrontal cortex. Brain Res. 1641, 217–233. doi: 10.1016/j.brainres.2016.01.005
Xu, T.-X., Sotnikova, T. D., Liang, C., Zhang, J., Jung, J. U., Spealman, R. D., et al. (2009). Hyperdopaminergic tone erodes prefrontal long-term potential via a D2 receptor-operated protein phosphatase gate. J. Neurosci. 29, 14086–14099. doi: 10.1523/JNEUROSCI.0974-09.2009
Xu, T.-X., and Yao, W.-D. (2010). D1 and D2 dopamine receptors in separate circuits cooperate to drive associative long-term potentiation in the prefrontal cortex. Proc. Natl. Acad. Sci. U.S.A. 107, 16366–16371. doi: 10.1073/pnas.1004108107
Yavich, L., Forsberg, M. M., Karayiorgou, M., Gogos, J. A., and Mannisto, P. T. (2007). Site-specific role of catechol-O-methyltransferase in dopamine overflow within prefrontal cortex and dorsal striatum. J. Neurosci. 27, 10196–10209. doi: 10.1523/JNEUROSCI.0665-07.2007
Yoshioka, M., Matsumoto, M., Togashi, H., and Saito, H. (1996). Effect of conditioned fear stress on dopamine release in the rat prefrontal cortex. Neurosci. Lett. 209, 201–203. doi: 10.1016/0304-3940(96)12631-8
Yuste, R., and Bonhoeffer, T. (2001). Morphological changes in dendritic spines associated with long-term synaptic plasticity. Annu. Rev. Neurosci. 24, 1071–1089. doi: 10.1146/annurev.neuro.24.1.1071
Zaitsev, A. V., and Anwyl, R. (2012). Inhibition of the slow afterhyperpolarization restores the classical spike timing-dependent plasticity rule obeyed in layer 2/3 pyramidal cells of the prefrontal cortex. J. Neurophysiol. 107, 205–215. doi: 10.1152/jn.00452.2011
Zbukvic, I. C., Park, C. H. J., Ganella, D. E., Lawrence, A. J., and Kim, J. H. (2017). Prefrontal dopaminergic mechanisms of extinction in adolescence compared to adulthood in rats. Front. Behav. Neurosci. 11, 32. doi: 10.3389/fnbeh.2017.00032
Zhang, J.-C., Lau, P.-M., and Bi, G.-Q. (2009). Gain in sensitivity and loss in temporal contrast of STDP by dopaminergic modulation at hippocampal synapses. Proc. Natl. Acad. Sci. U.S.A. 106, 13028–13033. doi: 10.1073/pnas.0900546106
Zhang, Y., Fukushima, H., and Kida, S. (2011). Induction and requirement of gene expression in the anterior cingulate cortex and medial prefrontal cortex for the consolidation of inhibitory avoidance memory. Mol. Brain 4, 4. doi: 10.1186/1756-6606-4-4
Zhang, Z.-W., Burke, M. W., Calakos, N., Beaulieu, J.-M., and Vaucher, E. (2010). Confocal analysis of cholinergic and dopaminergic inputs onto pyramidal cells in the prefrontal cortex of rodents. Front. Neuroanat. 4, 21. doi: 10.3389/fnana.2010.00021
Zheng, P., Zhang, X.-X., Bunney, B. S., and Shi, W.-X. (1999). Opposite modulation of cortical N-methyl-D-aspartate receptor-mediated responses by low and high concentrations of dopamine. Neuroscience 91, 527–535. doi: 10.1016/S0306-4522(98)00604-6
Zhong, P., Liu, W., Gu, Z., and Yan, Z. (2008). Serotonin facilitates long-term depression induction in prefrontal cortex via p38 MAPK/Rab5-mediated enhancement of AMPA receptor internalization. J. Physiol. 586, 4465–4479. doi: 10.1113/jphysiol.2008.155143
Zhou, H.-C., Sun, Y.-Y., Cai, W., He, X.-T., Yi, F., Li, B.-M., et al. (2013). Activation of 2-adrenoceptor enhances synaptic potentiation and behavioral memory via cAMP-PKA signaling in the medial prefrontal cortex of rats. Learn. Mem. 20, 274–284. doi: 10.1101/lm.030411.113
Ziegler, L., Zenke, F., Kastner, D. B., and Gerstner, W. (2015). Synaptic consolidation: from synapses to behavioral modeling. J. Neurosci. 35, 1319–1334. doi: 10.1523/JNEUROSCI.3989-14.2015
Zweifel, L. S., Parker, J. G., Lobb, C. J., Rainwater, A., Wall, V. Z., Fadok, J. P., et al. (2009). Disruption of NMDAR-dependent burst firing by dopamine neurons provides selective assessment of phasic dopamine-dependent behavior. Proc. Natl. Acad. Sci. U.S.A. 106, 7281–7288. doi: 10.1073/pnas.0813415106
Keywords: synaptic plasticity, long-term memory, dopamine, prefrontal cortex, neuromodulation, executive functions, behavioral flexibility, computational models
Citation: Sheynikhovich D, Otani S, Bai J and Arleo A (2023) Long-term memory, synaptic plasticity and dopamine in rodent medial prefrontal cortex: Role in executive functions. Front. Behav. Neurosci. 16:1068271. doi: 10.3389/fnbeh.2022.1068271
Received: 12 October 2022; Accepted: 26 December 2022;
Published: 11 January 2023.
Edited by:
Denise Manahan-Vaughan, Ruhr University Bochum, GermanyReviewed by:
Jee Hyun Kim, Deakin University, AustraliaCopyright © 2023 Sheynikhovich, Otani, Bai and Arleo. This is an open-access article distributed under the terms of the Creative Commons Attribution License (CC BY). The use, distribution or reproduction in other forums is permitted, provided the original author(s) and the copyright owner(s) are credited and that the original publication in this journal is cited, in accordance with accepted academic practice. No use, distribution or reproduction is permitted which does not comply with these terms.
*Correspondence: Denis Sheynikhovich, ZGVuaXMuc2hleW5pa2hvdmljaEBzb3Jib25uZS11bml2ZXJzaXRlLmZy
Disclaimer: All claims expressed in this article are solely those of the authors and do not necessarily represent those of their affiliated organizations, or those of the publisher, the editors and the reviewers. Any product that may be evaluated in this article or claim that may be made by its manufacturer is not guaranteed or endorsed by the publisher.
Research integrity at Frontiers
Learn more about the work of our research integrity team to safeguard the quality of each article we publish.