- 1Research and Academic Affairs Service Line, Mid-Atlantic Mental Illness Research Education and Clinical Center, Salisbury VA Medical Center, Salisbury, NC, United States
- 2Department of Neurobiology and Anatomy, Wake Forest School of Medicine, Winston-Salem, NC, United States
- 3Department of Psychiatry and Behavioral Medicine, Wake Forest School of Medicine, Winston-Salem, NC, United States
- 4Department of Neurology, Wake Forest School of Medicine, Winston-Salem, NC, United States
- 5Department of Physiology and Pharmacology, Wake Forest School of Medicine, Winston-Salem, NC, United States
Purpose: A fundamental question for Alcohol use disorder (AUD) is how and when naïve brain networks are reorganized in response to alcohol consumption. The current study aimed to determine the progression of alcohol’s effect on functional brain networks during transition from the naïve state to chronic consumption.
Procedures: Resting-state brain networks of six female rhesus macaque (Macaca mulatta) monkeys were acquired using magnetoencephalography (MEG) prior to alcohol exposure and after free-access to alcohol using a well-established model of chronic heavy alcohol consumption. Functional brain network metrics were derived at each time point.
Results: The average connection frequency (p < 0.024) and membership of the Rich Club (p < 0.022) changed significantly over time. Metrics describing network topology remained relatively stable from baseline to free-access drinking. The minimum degree of the Rich Club prior to alcohol exposure was significantly predictive of future free-access drinking (r = −0.88, p < 0.001).
Conclusions: Results suggest naïve brain network characteristics may be used to predict future alcohol consumption, and that alcohol consumption alters functional brain networks, shifting hubs and Rich Club membership away from previous regions in a non-systematic manner. Further work to refine these relationships may lead to the identification of a high-risk drinking phenotype.
Introduction
Alcohol use disorder (AUD) constitutes a global problem and is ranked among the top substance abuse problems in the United States, with over 70% of adults that struggle with substance use disorder estimated to abuse alcohol (Substance Abuse and Mental Health Services Administration (SAMHSA), 2020). AUD impacts global brain functional networks including the default mode, executive, attentional, salience and reward networks (Loeber et al., 2009; Park et al., 2010; Chanraud et al., 2011, 2013; Camchong et al., 2013; Sullivan et al., 2013) but the neurocircuitry underlying vulnerability and resilience to AUD is not clearly understood, making it difficult to establish viable, targeted treatment options.
This lack of clarity is due, in part, to the difficulty in capturing an alcohol-naïve baseline in human subjects. Clinical studies are often conducted with long-term drinkers at different drinking phases after changes in brain networks have already manifested. It is clear that AUD is characterized in part by dysfunctional information processing (Sullivan et al., 2000) that occurs in part through altered brain activity during both resting state (RS) and task performance in alcoholics (Rangaswamy and Porjesz, 2008) as compared to other neurological conditions (Georgopoulos et al., 2007). Functional brain networks including the default mode, salience, and executive networks are known to be sensitive to chronic alcohol use (Sullivan and Pfefferbaum, 2005; Sullivan et al., 2013; Weiland et al., 2014; Fede et al., 2019) however, the temporal nature and anatomic directionality of changes that occur remains unclear.
Studies such as IMAGEN have attempted to address this issue by evaluating adolescents prior to initiation of substance use (Maričić et al., 2020). Ivanov et al. (2020) used the IMAGEN study data to demonstrate that expected reductions in impulsivity were associated with lower levels of new onset drinking in an adolescent sample. They also found that blunted medial orbitofrontal activity in response to reward was associated with increased new onset and use. Nees et al. (2012) used the IMAGEN dataset to demonstrate that personality factors (novelty seeking, impulsivity, extraversion, and sensation seeking) were more strongly related to the initiation of drinking behaviors than reward related brain function, but that reward related brain function (particularly ventral striatum) was more strongly related to the development of problematic alcohol use. Heinrich et al. (2016) extended these findings in the IMAGEN study by including genetic factors. Results replicated the strong influence of personality factors on early initiation of drinking behaviors, while genetic factors appeared to become equally important when predicting future alcohol misuse behaviors. Reward related brain function did not contribute significant variance to the model. Similarly, Harper et al. (2019) used data from the Minnesota Twin Family Study Enrichment Sample to demonstrate that P3 amplitude mid-frontal theta power during an oddball task were predictors of new onset alcohol behaviors 3 years later in a sample of 14-year-old adolescents. While these studies offer valuable insight into the factors associated with initiation of use and onset of problematic use, they do not fully alleviate the confounding influence of environmental variables.
Non-human primate (NHP) models are valuable tools to help address the limitations of studies involving human participants (Grant et al., 2008). The model applied in the current study has been used to demonstrate that daily drinking for 15 months causes functional and genomic changes across the brain when contrasted against the alcohol naïve brain (Budygin et al., 2003; Floyd et al., 2004; Alexander et al., 2006; Carden et al., 2006; Anderson et al., 2007; Acosta et al., 2010; Cuzon Carlson et al., 2011; Mohr et al., 2013), as well as reorganization of brain networks measured by fMRI (Telesford et al., 2015) and significantly altered signal power of multiple bandwidths across the brain using magnetoencephalography (MEG; Rowland et al., 2017a). Using the same NHP model, alcohol naïve predictors of future drinking have also been identified. These include low cognitive flexibility (Shnitko et al., 2019), early drinking phenotypes (i.e., gulping vs. sipping; Grant et al., 2008; Baker et al., 2017), age, latency to begin drinking, and the number of “bouts” of drinking (Helms et al., 2014; Baker et al., 2017). No studies have examined alcohol naïve aspects of brain function as predictors of future drinking levels using this model.
The objective of the current study was a longitudinal examination of the trajectory of these changes at an earlier time point (6 months of chronic heavy alcohol intake) than previously examined (15 months chronic heavy alcohol intake) to identify at what point they begin to manifest and if baseline, alcohol-naïve indicators of future drinking can be identified.
Materials and Methods
Animals
Adult female rhesus monkeys (n = 6, 5–7 years old at study start) were subjects in an ongoing ethanol (EtOH) self-administration study. This age group reflects late adolescence to early adulthood in humans. The monkeys were trained on an operant panel to self-administer all fluids and food using a well-established drinking model that parallels levels and patterns of intake observed in alcoholics (Grant et al., 2008; Baker et al., 2014). This process begins with EtOH-naïve monkeys that are induced to drink escalating doses of EtOH (0.5, 1.0 and 1.5 g/kg) for 30 days at each dose (induction phase). This phase introduces the monkeys to the reinforcing properties of alcohol and results in rapid and equal daily alcohol intake without causing taste aversion. All monkeys were maintained at 1.5 g/kg for 20 drinking days while operant panels were serviced and re-programed. Animals were then provided free access to EtOH and water for 22 h per day, 5 days per week for 180 days. Sessions began at 11:00 am each day. MEG recordings were acquired under EtOH naïve conditions (Baseline) and after 180 open access drinking days (Free Access) to determine the impact of chronic, daily intake on RS brain function. The alcohol-naïve baseline served as a within-animal control dataset.
Preparation for MEG Scans
Animals were fasted overnight from food but not EtOH prior to scans. Average time between last drink and sedation for imaging was 344.2 min (SD = 377.9, min = 0.0, max = 977) at the Free Access Scan. These time frames raise the possibility of acute withdrawal (Winger and Woods, 1973); however, symptoms of withdrawal were not observed during similar time frames on non-imaging days (Pieper and Skeen, 1977) and the anesthetic agent (propofol, a GABAA receptor positive allosteric modulator; Shin et al., 2018) helped ensure acute withdrawal symptoms were not present during data acquisition. Previous work has shown that acute withdrawal in this model peaks between 24–72 h (Cuzon Carlson et al., 2011), which is beyond the duration since the last drink present here. Animals were sedated with ketamine (12 mg/kg, i.m.) for transport to the MEG suite. Anesthesia was induced with a bolus injection of 2.0–4.0 mg/kg propofol to allow intubation and was maintained via intravenous continuous infusion of 200 μg/kg/min propofol via syringe pump (Sage, Orion Research Corporation, Cambridge, MA, USA). Animals were placed in a supine position and artificially ventilated. These preparations are consistent with our previous reports (Telesford et al., 2015; Rowland et al., 2017a).
MEG Signal Recordings
Data were acquired using a whole head CTF Systems Inc. MEG 2005 neuromagnetometer system equipped with 275 first-order axial gradiometer coils. Head localization was achieved using a conventional three-point fiducial system (nasion and preauricular points). Each monkey was tattooed at each fiducial location to ensure consistent placement over time. Resting-state recording was conducted with animals lying supine for 5 min. Data were sampled at 1,200 or 2,400 Hz over a DC-300 or DC-600 Hz bandwidth, respectively. MEG data were preprocessed using synthetic 3rd order gradient balancing, whole trial DC offsetting, and band pass filtered from DC-80 Hz with powerline filtering. Data were visually inspected for obvious muscle artifact, and such epochs, if present, were discarded from further analyses. Following initial MEG recording, a T1 weighted MRI image was obtained for each animal for co-registration and localization of MEG signals.
Network Analysis
Network analysis was conducted identically to previous work (Rowland et al., 2017a,b, 2018). Network analysis proceeded by first identifying nodes of the network and quantifying communication among those nodes. The resulting matrices are conducive to the application of graph theory for calculating metrics describing the topology of the network.
Network Creation
Node Identification
For each animal 41 non-adjacent bilateral regions of interest (ROIs, voxel size = 2 × 2 × 2 mm) were identified in native brain space representing the default mode and reward networks. These networks have been previously demonstrated to be affected by chronic heavy alcohol consumption in humans (Chanraud et al., 2011; Müller-Oehring et al., 2015; Zhang and Volkow, 2019) and shown to be present in NHPs (Vincent et al., 2007; Mantini et al., 2011; Belcher et al., 2013). Brain regions included the anterior cingulate, medial and lateral orbital frontal cortex, principle sulcus, nucleus accumbens, caudate head and body, head of the putamen, parietal area, precuneus, lateral and medial amygdala, anterior, medial, and posterior hippocampus, vermis, anterior and posterior lobes of the cerebellum, thalamus, and anterior insula.
Functional Connectivity
Source series representing the unique weighted sum of the output across all MEG sensors for a specific ROI in the brain were calculated using a well-validated beamformer (synthetic aperture magnetometry, SAM; Robinson and Vrba, 1999; Hillebrand et al., 2005). Prior work has demonstrated the sensitivity of MEG beamformers to superficial as well as deeper sources (Stapleton-Kotloski et al., 2014, 2018). The weighted phase lag index (wPLI; Vinck et al., 2011) was calculated between all pairs of source series to establish functional connectivity, filtered between 1 and 80 Hz. The wPLI is a phase-based metric insensitive to fluctuations in source amplitude. A surrogate distribution of 5,000 unique pairs of phase-randomized time series was created for each animal individually (Prichard and Theiler, 1994). Connectivity was operationalized at the frequency with the greatest difference in wPLI value between the real and surrogate data, calculated as standard deviations. This approach allows the frequency at which connections occur to vary from connection to connection, representing a better model of brain activity than restricting connectivity to a specific frequency band (Chen et al., 2008; Hillebrand et al., 2012). To remove connections not different from noise, connections without a real-surrogate difference exceeding 2.5 standard deviations were left unconnected. The resulting networks were then thresholded by satisfying the equation S = log(N)/log(K) where N represents the number of nodes in the network and K the average degree using S = 2.5 (Hayasaka and Laurienti, 2010).
Network Metrics
Network metrics calculated are listed in Table 2. Metrics were selected with a focus on characterizing the topology of the overall network. Clustering Coefficient was selected as an indicator of clustering and subgroup formation within the network. This metric was calculated as defined in Stam and Reijneveld (2007). Modularity was selected as an indicator of well-defined subnetworks within the larger network. This metric was calculated using the Louvain method of community detection (Blondel et al., 2008). The analysis was run 500 times, using the average number of modules (Number Modules) as outcome variables. Assortativity coefficient represents the correlation coefficient of the degree of nodes on each end of a connection. The degree of a node is the number of direct connections that node has to other nodes in the network. A positive coefficient suggests nodes are preferentially connecting to other nodes of similar degree, while a negative coefficient suggests nodes preferentially connect to those of different degree (Newman, 2002). Rich Club was selected as an indicator of the presence of a “network backbone.” The Rich Club is a subset of highly connected and highly interconnected nodes forming the basis of the broader network. Rich Club characteristics (Colizza et al., 2006) were calculated using 500 independently generated random networks. The number of nodes (Rich Club Nodes) within the Rich Club, the minimum degree of those nodes (Rich Club Degree), and interconnectivity among those nodes (Rich Club Coefficient) were used as outcome variables. The Rich Club Coefficient was weighted by the average of the same metric across the 500 random networks, representing the level of increased interconnectivity over a random network. The Mean Connection Frequency was calculated as the average of the frequency at which connections occurred across all connections in the network. The number of connections occurring in each of the canonical frequency bands (e.g., delta, theta, alpha, beta, gamma) was also calculated as outcome variables. Hubs of the network were identified as the 10% of nodes (n = 4) with the highest degree.
Materials
Beamforming and source series construction were completed using software provided by CTF MEG International Services LP (Coquitlam, BC, Canada). Further analyses of source series data and network creation were conducted using Matlab 2016a. Network metrics were calculated using the Brain Connectivity Toolbox (Rubinov and Sporns, 2010). SAS Enterprise Guide 7.1 (SAS Institute Inc., Cary, NC, USA) was used for statistical analysis.
Analyses
Differences across time in network metrics (Baseline to Free Access) were examined using paired samples t-tests. Consistency over time in the distribution of hub and Rich Club members was examined using paired samples t-tests. The data used are presented in Tables 4, 5. The independent variable was time point, and the dependent variable was the distribution of membership across regions. Secondary Chi-Square analyses (McNemara’s test) were run to examine how the hub and Rich Club status of individual brain regions changed over time. Spearman rank correlations were conducted to examine the relationship between network metrics and drinking outcomes (daily average g/kg) during Free Access. Two-tailed tests and alpha of 0.05 were used for significance.
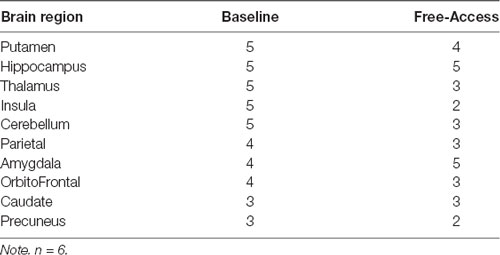
Table 5. The number of subjects for which each brain region was considered a member of the Rich Club.
Results
Daily average g/kg EtOH consumption increased significantly once given free access [mean (SD); Free-Access = 4.7 (1.0), p < 0.01, Cohen’s d = 5.23], as shown in Table 1. Network metrics at Baseline and Free-Access (after 180 days unrestricted access) are shown in Table 2.
Networks Predicting EtOH Consumption
Table 3 illustrates correlations between Baseline (EtOH naïve) network metrics and Free Access consumption. The minimum degree of the Rich Club at baseline was strongly related to Free Access consumption levels (Figure 1).
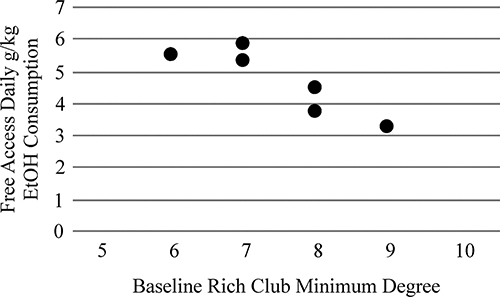
Figure 1. The minimum degree of the Rich Club of the alcohol naïve (Baseline) functional brain network was strongly related to future drinking when animals were provided free-access to alcohol (r = −0.88, p = 0.02).
Effects of EtOH on Networks
Table 2 shows mean and standard deviations of network metrics at Baseline and Free Access. The average connection frequency was significantly higher at Free Access than at Baseline, t(5) = −3.20, p = 0.024, but no other differences in network metrics were observed.
Specific Brain Regions
Table 4 demonstrates areas considered hubs across animals at each time point. For brain structures with multiple aspects, the region was considered a hub if any of the aspects were considered a hub (e.g., if either the posterior, medial, or anterior hippocampus was a hub, then Table 4 indicates the hippocampus as a hub). There was no significant change in brain regions considered hubs from Baseline to Free Access. Table 5 includes brain regions that were members of the Rich Club for at least three animals at any time point, again collapsing within regions. A significant decrease in the commonality of regions in the Rich Club across animals was observed from Baseline to Free Access, t(9) = 2.74, p = 0.022. Tests of change in individual regions were not significant.
Discussion
The current study demonstrates that characteristics of the Rich Club of alcohol-naïve brain networks are related to future drinking behaviors. In addition, following extended chronic drinking the connection strength in the network was altered; however, no effect on network metrics was observed. Finally, while hubs of the network were not observed to change significantly over time, membership in the Rich Club was significantly altered by chronic heavy drinking.
Aspects of alcohol-naïve resting-state functional brain networks were demonstrated to predict future drinking levels. Higher minimum degree of the Rich Club at Baseline (alcohol-naïve) was strongly correlated with decreased alcohol consumption during the future free access period. The Rich Club is a community of nodes within the network that have high degree and interconnectedness, serving as the “spine” of the network (Colizza et al., 2006). These nodes represent hubs within the network and communication among these nodes can often serve as “shortcuts” within the network, increasing efficiency of communication across otherwise distantly connected nodes. As the minimum degree of the Rich Club decreases, the centrality of Rich Club nodes also decreases, meaning fewer aspects of communication are routed through these nodes. Essentially, as the minimum degree decreases, the Rich Club becomes less differentiated from the other nodes in the network. This result suggests that the level of Rich Club differentiation may be predictive of future drinking levels, even when measured prior to alcohol exposure.
Alterations to the Rich Club subnetwork are likely to have broad and sweeping effects on brain communication and information processing (van den Heuvel and Sporns, 2013). Differences in Rich Club characteristics have been observed in many neurodevelopmental disorders, including schizophrenia (Collin et al., 2017), bipolar disorder (Wang et al., 2019), and autism (Hong et al., 2019). As such, the broad differences in Rich Club characteristics observed in this study are unlikely to serve as a direct “neurophenotype” of AUD without further refinement and empirical study. However, these results identify that differences in network topology are important to understanding individuals who might be at risk for future heavy drinking or AUD. Further, these results are consistent with previous work using the same NHP model indicating that premorbid behaviors and those occurring early in the drinking history may be predictive of future consumption levels. These factors include low cognitive flexibility (Shnitko et al., 2019), early drinking phenotypes (i.e., gulping vs. sipping; Grant et al., 2008; Baker et al., 2017), age, latency to begin drinking, and the number of “bouts” of drinking (Helms et al., 2014; Baker et al., 2017). The current results are the first to provide a brain-based factor indicative of future drinking in this model, suggesting that alcohol-naïve differences in Rich Club characteristics of functional brain networks also predict future drinking in this model.
These results extend recent findings in human participants demonstrating that white matter brain networks of individuals with AUD displayed lower Rich Club characteristics compared to their non-abusing siblings, who displayed lower levels compared to control participants (Zorlu et al., 2019). These results are also consistent with recent findings using the same NHP model indicating that chronic heavy drinking inhibits white matter growth (i.e., reduced connectivity) during late adolescence (Shnitko et al., 2019). While Zorlu et al. (2019) suggest potential premorbid differences in white matter network structure may be a marker of risk or susceptibility to AUD, the results of the current study provide direct empirical support for this hypothesis, showing that Rich Club characteristics of premorbid functional brain networks are directly related to future drinking levels. These results are also consistent with those of several longitudinal projects conducted in humans including the IMAGEN and NCANDA studies (Brumback et al., 2016; Maričić et al., 2020; Silveira et al., 2020). However, the current results examine functional RS brain networks and not reward based processing or structural aspects. The current study also did not acquire genetic or behavioral data as was acquired by IMAGEN, limiting further comparisons. Findings from the current study as well as these previous studies demonstrate that brain function and structure measured prior to exposure to alcohol may be able to identify individuals at risk for future heavy alcohol consumption.
Alcohol-induced changes in functional brain networks were observed following a period of exposure and free access to alcohol. The mean connection frequency increased following chronic heavy drinking; however, changes in network metrics were not observed. This suggests the general topology of networks were not altered (e.g., path lengths, clustering, etc.) Significant changes were seen in the membership of the Rich Club. There was much less consistency in Rich Club membership following chronic heavy drinking, suggesting the networks were being altered in an inconsistent manner across animals. It should be noted that the quantity of alcohol consumed during the free access period was fully determined by each animal. These results support the potential for a dose-dependent relationship between patterns of alcohol consumption and the effect on functional brain networks (Correas et al., 2015, 2016; López-Caneda et al., 2017; Perez-Ramirez et al., 2017).
Limitations of the current pilot study include the small sample size, which limits the complexity and sensitivity of analyses that can be conducted. Neuroimaging was conducted under anesthesia, which has known effects on brain function (Boveroux et al., 2010; Xie et al., 2013; Guldenmund et al., 2016). Possible interactions between the anesthetic and alcohol could have occurred, if not directly, then through the indirect development of tolerance. However, anesthesia was maintained at consistent levels and physiological indicators of arousal were monitored continuously, suggesting that levels of sedation were consistent across scans and animals. Additionally, after baseline data was acquired in the alcohol-naïve state, all animals entered the alcohol self-administration paradigm. We are mindful that this within-animal comparison across time limits insight into potential non-alcohol related changes over time or changes as a result of operant manipulations. However, test-retest scan sessions in monkeys 1 year apart and in humans 6 years apart (Stapleton-Kotloski et al., 2014) have established that networks remain stable over time.
Neuroimaging under conscious conditions will be required to completely understand the effects of alcohol on brain function using this model. The interval between ethanol access and MEG scans raises the possibility that some animals may have been experiencing symptoms of withdrawal (Winger and Woods, 1973). However, signs of withdrawal were not observed during the same time periods on non-imaging days (Pieper and Skeen, 1977; Cuzon Carlson et al., 2011). Also, propofol was used as the anesthetic agent, helping to ensure animals were not experiencing withdrawal symptoms during scans (Shin et al., 2018). Finally, animals who ceased alcohol consumption prior to scans did so voluntarily and in a time frame consistent with non-imaging days and were not forcibly fasted.
Conclusions
The current study identified a relationship between functional brain networks in the alcohol-naïve state and future alcohol consumption, consistent with other work using this model demonstrating early behavioral markers of future drinking. This is the first brain-based predictor of future alcohol consumption identified for this model. Additionally, significant alteration in the Rich Club of the network was observed following 180 days of chronic heavy consumption of alcohol, an earlier time point than examined in previous works. Future work will be invaluable in clarifying the changes, and specifying the timing of those changes, that infer risk specific to AUD in humans.
Data Availability Statement
The raw data supporting the conclusions of this article will be made available by the authors, without undue reservation.
Ethics Statement
The animal study was reviewed and approved by Wake Forest School of Medicine ACUC.
Author Contributions
JR, JS-K, AD, DG, and JD contributed to the study design, JR, JS-K, AD, GA, PE, and JD contributed to data acquisition. JR, JS-K, DG, GA, and JD contributed to data analysis and interpretation. JR, JS-K, GA, AD, PE, DG, and JD contributed to manuscript preparation. All authors contributed to the article and approved the submitted version.
Funding
JD was supported by National Institutes of Health (NIH) grant NIAAA R21 AA028007, P01AA021099- S1. GA was supported by NIH grant NIAAA F30 AA 23708-02. DG was supported by NIH grants AA016852, NS116519, and AA026117.
Conflict of Interest
The authors declare that the research was conducted in the absence of any commercial or financial relationships that could be construed as a potential conflict of interest.
Acknowledgments
We thank the Neurosciences Center of Excellence for pilot MEG scans.
References
Acosta, G., Hasenkamp, W., Daunais, J. B., Freidman, D. P., Grant, K. A., and Hemby, S. E. (2010). Ethanol self-administration modulation of NMDA receptor subunit and related synaptic protein mRNA expression in prefrontal cortical fields. Brain Res. 1318, 144–154. doi: 10.1016/j.brainres.2009.12.050
Alexander, G. M., Carden, W. B., Mu, J., Kurukulasuriya, N. C., McCool, B. A., Nordskog, B. K., et al. (2006). The native T-type calcium current in relay neurons of the primate thalamus. Neuroscience 141, 453–461. doi: 10.1016/j.neuroscience.2006.03.042
Anderson, N. J., Daunais, J. B., Friedman, D. P., Grant, K. A., and McCool, B. A. (2007). Long-term ethanol self-administration by the non-human primate, macaca fascicularis, decreases the benzodiazepine sensitivity of amygdala GABAA receptors. Alcohol. Clin. Exp. Res. 31, 1061–1070. doi: 10.1111/j.1530-0277.2007.00394.x
Baker, E. J., Farro, J., Gonzales, S., Helms, C., and Grant, K. A. (2014). Chronic alcohol self-administration in monkeys shows long-term quantity/frequency categorical stability. Alcohol. Clin. Exp. Res. 38, 2835–2843. doi: 10.1111/acer.12547
Baker, E. J., Walter, N. A. R., Salo, A., Rivas Perea, P., Moore, S., Gonzales, S., et al. (2017). Identifying future drinkers: behavioral analysis of monkeys initiating drinking to intoxication is predictive of future drinking classification. Alcohol. Clin. Exp. Res. 41, 626–636. doi: 10.1111/acer.13327
Belcher, A. M., Yen, C. C., Stepp, H., Gu, H., Lu, H., Yang, Y., et al. (2013). Large-scale brain networks in the awake, truly resting marmoset monkey. J. Neurosci. 33, 16796–16804. doi: 10.1523/JNEUROSCI.3146-13.2013
Blondel, V. D., Guillaume, J.-L., Lambiotte, R., and Lefebvre, E. (2008). Fast unfolding of communities in large networks. J. Stat. Mech. 2008:P10008. doi: 10.1103/PhysRevE.77.036114
Boveroux, P., Vanhaudenhuyse, A., Bruno, M.-A., Noirhomme, Q., Lauwick, S., Luxen, A., et al. (2010). Breakdown of within- and between-network resting state functional magnetic resonance imaging connectivity during propofol-induced loss of consciousness. Anesthesiology 113, 1038–1053. doi: 10.1097/ALN.0b013e3181f697f5
Brumback, T., Worley, M., Nguyen-Louie, T. T., Squeglia, L. M., Jacobus, J., and Tapert, S. F. (2016). Neural predictors of alcohol use and psychopathology symptoms in adolescents. Dev. Psychopathol. 28, 1209–1216. doi: 10.1017/S0954579416000766
Budygin, E. A., John, C. E., Mateo, Y., Daunais, J. B., Friedman, D. P., Grant, K. A., et al. (2003). Chronic ethanol exposure alters presynaptic dopamine function in the striatum of monkeys: a preliminary study. Synapse 50, 266–268. doi: 10.1002/syn.10269
Camchong, J., Stenger, A., and Fein, G. (2013). Resting-state synchrony during early alcohol abstinence can predict subsequent relapse. Cereb. Cortex 23, 2086–2099. doi: 10.1093/cercor/bhs190
Carden, W. B., Alexander, G. M., Friedman, D. P., Daunais, J. B., Grant, K. A., Mu, J., et al. (2006). Chronic ethanol drinking reduces native T-type calcium current in the thalamus of nonhuman primates. Brain Res. 1089, 92–100. doi: 10.1016/j.brainres.2006.02.135
Chanraud, S., Pitel, A.-L., Müller-Oehring, E. M., Pfefferbaum, A., and Sullivan, E. V. (2013). Remapping the brain to compensate for impairment in recovering alcoholics. Cereb. Cortex 23, 97–104. doi: 10.1093/cercor/bhr381
Chanraud, S., Pitel, A.-L., Pfefferbaum, A., and Sullivan, E. V. (2011). Disruption of functional connectivity of the default-mode network in alcoholism. Cereb. Cortex 21, 2272–2281. doi: 10.1093/cercor/bhq297
Chen, A. C. N., Feng, W., Zhao, H., Yin, Y., and Wang, P. (2008). EEG default mode network in the human brain: spectral regional field powers. NeuroImage 41, 561–574. doi: 10.1016/j.neuroimage.2007.12.064
Colizza, V., Flammini, A., Serrano, M. A., and Vespignani, A. (2006). Detecting rich-club ordering in complex networks. Nature Phys. 2, 110–115. doi: 10.1038/nphys209
Collin, G., Scholtens, L. H., Kahn, R. S., Hillegers, M. H. J., and van den Heuvel, M. P. (2017). Affected anatomical rich club and structural-functional coupling in young offspring of schizophrenia and bipolar disorder patients. Biol. Psychiatry 82, 746–755. doi: 10.1016/j.biopsych.2017.06.013
Correas, A., Cuesta, P., López-Caneda, E., Rodríguez Holguín, S., García-Moreno, L. M., Pineda-Pardo, J. A., et al. (2016). Functional and structural brain connectivity of young binge drinkers: a follow-up study. Sci. Rep. 6:31293. doi: 10.1038/srep31293
Correas, A., Rodriguez Holguín, S., Cuesta, P., López-Caneda, E., García-Moreno, L. M., Cadaveira, F., et al. (2015). Exploratory analysis of power spectrum and functional connectivity during resting state in young binge drinkers: a MEG study. Int. J. Neural Syst. 25:1550008. doi: 10.1142/S0129065715500082
Cuzon Carlson, V. C., Seabold, G. K., Helms, C. M., Garg, N., Odagiri, M., Rau, A. R., et al. (2011). Synaptic and morphological neuroadaptations in the putamen associated with long-term, relapsing alcohol drinking in primates. Neuropsychopharmacology 36, 2513–2528. doi: 10.1038/npp.2011.140
Fede, S. J., Grodin, E. N., Dean, S. F., Diazgranados, N., and Momenan, R. (2019). Resting state connectivity best predicts alcohol use severity in moderate to heavy alcohol users. Neuroimage Clin. 22:101782. doi: 10.1016/j.nicl.2019.101782
Floyd, D. W., Friedman, D. P., Daunais, J. B., Pierre, P. J., Grant, K. A., and McCool, B. A. (2004). Long-term ethanol self-administration by cynomolgus macaques alters the pharmacology and expression of GABAA receptors in basolateral amygdala. J. Pharmacol. Exp. Ther. 311, 1071–1079. doi: 10.1124/jpet.104.072025
Georgopoulos, A. P., Karageorgiou, E., Leuthold, A. C., Lewis, S. M., Lynch, J. K., Alonso, A. A., et al. (2007). Synchronous neural interactions assessed by magnetoencephalography: a functional biomarker for brain disorders. J. Neural Eng. 4, 349–355. doi: 10.1088/1741-2560/4/4/001
Grant, K. A., Leng, X., Green, H. L., Szeliga, K. T., Rogers, L. S. M., and Gonzales, S. W. (2008). Drinking typography established by scheduled induction predicts chronic heavy drinking in a monkey model of ethanol self-administration. Alcohol. Clin. Exp. Res. 32, 1824–1838. doi: 10.1111/j.1530-0277.2008.00765.x
Guldenmund, P., Gantner, I. S., Baquero, K., Das, T., Demertzi, A., Boveroux, P., et al. (2016). Propofol-induced frontal cortex disconnection: a study of resting-state networks, total brain connectivity and mean BOLD signal oscillation frequencies. Brain Connect. 6, 225–237. doi: 10.1089/brain.2015.0369
Harper, J., Malone, S. M., and Iacono, W. G. (2019). Parietal P3 and midfrontal theta prospectively predict the development of adolescent alcohol use. Psychol. Med. 51, 1–10. doi: 10.1017/S0033291719003258
Hayasaka, S., and Laurienti, P. J. (2010). Comparison of characteristics between region-and voxel-based network analyses in resting-state fMRI data. NeuroImage 50, 499–508. doi: 10.1016/j.neuroimage.2009.12.051
Helms, C. M., Rau, A., Shaw, J., Stull, C., Gonzales, S. W., and Grant, K. A. (2014). The effects of age at the onset of drinking to intoxication and chronic ethanol self-administration in male rhesus macaques. Psychopharmacology 231, 1853–1861. doi: 10.1007/s00213-013-3417-x
Heinrich, A., Müller, K. U., Banaschewski, T., Barker, G. J., Bokde, A. L. W., Bromberg, U., et al. (2016). Prediction of alcohol drinking in adolescents: personality-traits, behavior, brain responses, and genetic variations in the context of reward sensitivity. Biol. Psychol. 118, 79–87. doi: 10.1016/j.biopsycho.2016.05.002
Hillebrand, A., Barnes, G. R., Bosboom, J. L., Berendse, H. W., and Stam, C. J. (2012). Frequency-dependent functional connectivity within resting-state networks: an atlas-based MEG beamformer solution. NeuroImage 59, 3909–3921. doi: 10.1016/j.neuroimage.2011.11.005
Hillebrand, A., Singh, K. D., Holliday, I. E., Furlong, P. L., and Barnes, G. R. (2005). A new approach to neuroimaging with magnetoencephalography. Hum. Brain Mapp. 25, 199–211. doi: 10.1002/hbm.20102
Hong, S.-J., Vos de Wael, R., Bethlehem, R. A. I., Lariviere, S., Paquola, C., Valk, S. L., et al. (2019). Atypical functional connectome hierarchy in autism. Nat. Commun. 10:1022. doi: 10.1038/s41467-019-08944-1
Ivanov, I., Parvaz, M. A., Velthorst, E., Shaik, R. B., Sandin, S., Gan, G., et al. (2020). Substance use initiation, particularly alcohol, in drug naïve adolescents: possible predictors and consequences from a large cohort naturalistic study. J. Am. Acad. Child Adolesc. Psychiatry doi: 10.1016/j.jaac.2020.08.443
Loeber, S., Duka, T., Welzel, H., Nakovics, H., Heinz, A., Flor, H., et al. (2009). Impairment of cognitive abilities and decision making after chronic use of alcohol: the impact of multiple detoxifications. Alcohol Alcohol. 44, 372–381. doi: 10.1093/alcalc/agp030
López-Caneda, E., Cadaveira, F., Correas, A., Crego, A., Maestú, F., and Rodríguez Holguín, S. (2017). The brain of binge drinkers at rest: alterations in theta and beta oscillations in first-year college students with a binge drinking pattern. Front. Behav. Neurosci. 11:168. doi: 10.3389/fnbeh.2017.00168
Mantini, D., Gerits, A., Nelissen, K., Durand, J.-B., Joly, O., Simone, L., et al. (2011). Default mode of brain function in monkeys. J. Neurosci. 31, 12954–12962. doi: 10.1523/JNEUROSCI.2318-11.2011
Maričić, L. M., Walter, H., Rosenthal, A., Ripke, S., Quinlan, E. B., Banaschewski, T., et al. (2020). The IMAGEN study: a decade of imaging genetics in adolescents. Mol. Psychiatry 25, 2648–2671. doi: 10.1038/s41380-020-0822-5
Mohr, C., Kolotushkina, O., Kaplan, J. S., Welsh, J., Daunais, J., Grant, K., et al. (2013). Primate cerebellar granule cells exhibit a tonic GABAAR conductance that is not affected by alcohol: a possible cellular substrate of the low level of response phenotype. Front. Neural Circuits 7:189. doi: 10.3389/fncir.2013.00189
Müller-Oehring, E. M., Jung, Y.-C., Pfefferbaum, A., Sullivan, E. V., and Schulte, T. (2015). The resting brain of alcoholics. Cereb. Cortex 25, 4155–4168. doi: 10.1093/cercor/bhu134
Nees, F., Tzschoppe, J., Patrick, C. J., Vollstídt-Klein, S., Steiner, S., Poustka, L., et al. (2012). Determinants of early alcohol use in healthy adolescents: the differential contribution of neuroimaging and psychological factors. Neuropsychopharmacology 37, 986–995. doi: 10.1038/npp.2011.282
Newman, M. E. J. (2002). Assortative mixing in networks. Phys. Rev. Lett. 89:208701. doi: 10.1103/PhysRevLett.89.208701
Park, S. Q., Kahnt, T., Beck, A., Cohen, M. X., Dolan, R. J., Wrase, J., et al. (2010). Prefrontal cortex fails to learn from reward prediction errors in alcohol dependence. J. Neurosci. 30, 7749–7753. doi: 10.1523/JNEUROSCI.5587-09.2010
Perez-Ramirez, U., Diaz-Parra, A., Ciccocioppo, R., Canals, S., and Moratal, D. (2017). Brain functional connectivity alterations in a rat model of excessive alcohol drinking: a resting-state network analysis. Annu. Int. Conf. IEEE Eng. Med. Biol. Soc. 2017, 3016–3019. doi: 10.1109/EMBC.2017.8037492
Pieper, W. A., and Skeen, M. J. (1977). Alcohol withdrawal reactions in rhesus monkeys. Adv. Exp. Med. Biol. 85B, 42–55. doi: 10.1007/978-1-4615-9038-5_4
Prichard, D., and Theiler, J. (1994). Generating surrogate data for time series with several simultaneously measured variables. Phys. Rev. Lett. 73, 951–954. doi: 10.1103/PhysRevLett.73.951
Rangaswamy, M., and Porjesz, B. (2008). Uncovering genes for cognitive (dys)function and predisposition for alcoholism spectrum disorders: a review of human brain oscillations as effective endophenotypes. Brain Res. 1235, 153–171. doi: 10.1016/j.brainres.2008.06.053
Robinson, S. E., and Vrba, J. (1999). “Functional Neuroimaging by Synthetic Aperture Magnetometry (SAM),” in Recent Advances in Biomagnetism, eds T. Yoshimoto, M. Kotani, S. Kuriki, H. Karibe and N. Nakasato (Sendai, Japan: Tohoku University Press), 302–305.
Rowland, J. A., Stapleton-Kotloski, J. R., Alberto, G. E., Davenport, A. T., Kotloski, R. J., Friedman, D. P., et al. (2017a). Changes in nonhuman primate brain function following chronic alcohol consumption in previously naïve animals. Drug Alcohol Depend. 177, 244–248. doi: 10.1016/j.drugalcdep.2017.03.036
Rowland, J. A., Stapleton-Kotloski, J. R., Alberto, G. E., Rawley, J. A., Kotloski, R. J., Taber, K. H., et al. (2017b). Contrasting effects of posttraumatic stress disorder and mild traumatic brain injury on the whole-brain resting-state network: a magnetoencephalography study. Brain Connect. 7, 45–57. doi: 10.1089/brain.2015.0406
Rowland, J. A., Stapleton-Kotloski, J. R., Dobbins, D. L., Rogers, E., Godwin, D. W., and Taber, K. H. (2018). Increased small-world network topology following deployment-acquired traumatic brain injury associated with the development of post-traumatic stress disorder. Brain Connect. 8, 205–211. doi: 10.1089/brain.2017.0556
Rubinov, M., and Sporns, O. (2010). Complex network measures of brain connectivity: uses and interpretations. NeuroImage 52, 1059–1069. doi: 10.1016/j.neuroimage.2009.10.003
Shin, D. J., Germann, A. L., Johnson, A. D., Forman, S. A., Steinbach, J. H., and Akk, G. (2018). Propofol is an allosteric agonist with multiple binding sites on concatemeric ternary GABAA receptors. Mol. Pharmacol. 93, 178–189. doi: 10.1124/mol.117.110403
Shnitko, T. A., Gonzales, S. W., and Grant, K. A. (2019). Low cognitive flexibility as a risk for heavy alcohol drinking in non-human primates. Alcohol 74, 95–104. doi: 10.1016/j.alcohol.2018.04.007
Silveira, S., Shah, R., Nooner, K. B., Nagel, B. J., Tapert, S. F., de Bellis, M. D., et al. (2020). Impact of childhood trauma on executive function in adolescence-mediating functional brain networks and prediction of high-risk drinking. Biol. Psychiatry Cogn. Neurosci. Neuroimaging 5, 499–509. doi: 10.1016/j.bpsc.2020.01.011
Stam, C. J., and Reijneveld, J. C. (2007). Graph theoretical analysis of complex networks in the brain. Nonlinear Biomed. Phys. 1:3. doi: 10.1186/1753-4631-1-3
Stapleton-Kotloski, J. R., Kotloski, R. J., Boggs, J. A., Popli, G., O’Donovan, C. A., Couture, D. E., et al. (2014). Localization of interictal epileptiform activity using magnetoencephalography with synthetic aperture magnetometry in patients with a vagus nerve stimulator. Front. Neurol. 5:244. doi: 10.3389/fneur.2014.00244
Stapleton-Kotloski, J., Kotloski, R., Popli, G., and Godwin, D. (2018). Magnetoencephalography: clinical and research practices. Brain Sci. 8:157. doi: 10.3390/brainsci8080157
Substance Abuse and Mental Health Services Administration (SAMHSA) (2020). 2019 NSDUH annual national report. Available online at: https://www.samhsa.gov/data/report/2019-nsduh-annual-national-report. Accessed October 1, 2020.
Sullivan, E. V., Müller-Oehring, E., Pitel, A.-L., Chanraud, S., Shankaranarayanan, A., Alsop, D. C., et al. (2013). A selective insular perfusion deficit contributes to compromised salience network connectivity in recovering alcoholic men. Biol. Psychiatry 74, 547–555. doi: 10.1016/j.biopsych.2013.02.026
Sullivan, E. V., and Pfefferbaum, A. (2005). Neurocircuitry in alcoholism: a substrate of disruption and repair. Psychopharmacology 180, 583–594. doi: 10.1007/s00213-005-2267-6
Sullivan, E. V., Rosenbloom, M. J., and Pfefferbaum, A. (2000). Pattern of motor and cognitive deficits in detoxified alcoholic men. Alcohol. Clin. Exp. Res. 24, 611–621. doi: 10.1111/j.1530-0277.2000.tb02032.x
Telesford, Q. K., Laurienti, P. J., Davenport, A. T., Friedman, D. P., Kraft, R. A., and Daunais, J. B. (2015). The effects of chronic alcohol self-administration in nonhuman primate brain networks. Alcohol. Clin. Exp. Res. 39, 659–671. doi: 10.1111/acer.12688
van den Heuvel, M. P., and Sporns, O. (2013). Network hubs in the human brain. Trends Cogn. Sci. 17, 683–696. doi: 10.1016/j.tics.2013.09.012
Vincent, J. L., Patel, G. H., Fox, M. D., Snyder, A. Z., Baker, J. T., Van Essen, D. C., et al. (2007). Intrinsic functional architecture in the anaesthetized monkey brain. Nature 447, 83–86. doi: 10.1038/nature05758
Vinck, M., Oostenveld, R., van Wingerden, M., Battaglia, F., and Pennartz, C. M. A. (2011). An improved index of phase-synchronization for electrophysiological data in the presence of volume-conduction, noise and sample-size bias. NeuroImage 55, 1548–1565. doi: 10.1016/j.neuroimage.2011.01.055
Wang, Y., Deng, F., Jia, Y., Wang, J., Zhong, S., Huang, H., et al. (2019). Disrupted rich club organization and structural brain connectome in unmedicated bipolar disorder. Psychol. Med. 49, 510–518. doi: 10.1017/S0033291718001150
Weiland, B. J., Sabbineni, A., Calhoun, V. D., Welsh, R. C., Bryan, A. D., Jung, R. E., et al. (2014). Reduced left executive network functional connectivity is associated with alcohol use disorders. Alcohol. Clin. Exp. Res. 38, 2445–2453. doi: 10.1111/acer.12505
Winger, G. D., and Woods, J. H. (1973). The reinforcing property of ethanol in the rhesus monkey: I. Initiation, maintenance and termination of intravenous ethanol-reinforced responding. Ann. N Y Acad. Sci. 215, 162–175. doi: 10.1111/j.1749-6632.1973.tb28263.x
Xie, P., Yu, T., Fu, X., Tu, Y., Zou, Y., Lui, S., et al. (2013). Altered functional connectivity in an aged rat model of postoperative cognitive dysfunction: a study using resting-state functional MRI. PLoS One 8:e64820. doi: 10.1371/journal.pone.0064820
Zhang, R., and Volkow, N. D. (2019). Brain default-mode network dysfunction in addiction. NeuroImage 200, 313–331. doi: 10.1016/j.neuroimage.2019.06.036
Keywords: magnetoencephalography, substance use disorder, risk factor, primate, brain function
Citation: Rowland JA, Stapleton-Kotloski JR, Alberto GE, Davenport AT, Epperly PM, Godwin DW and Daunais JB (2021) Rich Club Characteristics of Alcohol-Naïve Functional Brain Networks Predict Future Drinking Phenotypes in Rhesus Macaques. Front. Behav. Neurosci. 15:673151. doi: 10.3389/fnbeh.2021.673151
Received: 26 February 2021; Accepted: 28 April 2021;
Published: 02 June 2021.
Edited by:
Hisao Nishijo, University of Toyama, JapanReviewed by:
Michael Fritz, University of Ulm, GermanyMustapha Muzaimi, Universiti Sains Malaysia Health Campus, Malaysia
Copyright © 2021 Rowland, Stapleton-Kotloski, Alberto, Davenport, Epperly, Godwin and Daunais. This is an open-access article distributed under the terms of the Creative Commons Attribution License (CC BY). The use, distribution or reproduction in other forums is permitted, provided the original author(s) and the copyright owner(s) are credited and that the original publication in this journal is cited, in accordance with accepted academic practice. No use, distribution or reproduction is permitted which does not comply with these terms.
*Correspondence: Jared A. Rowland, amFyZWQucm93bGFuZEB2YS5nb3Y=