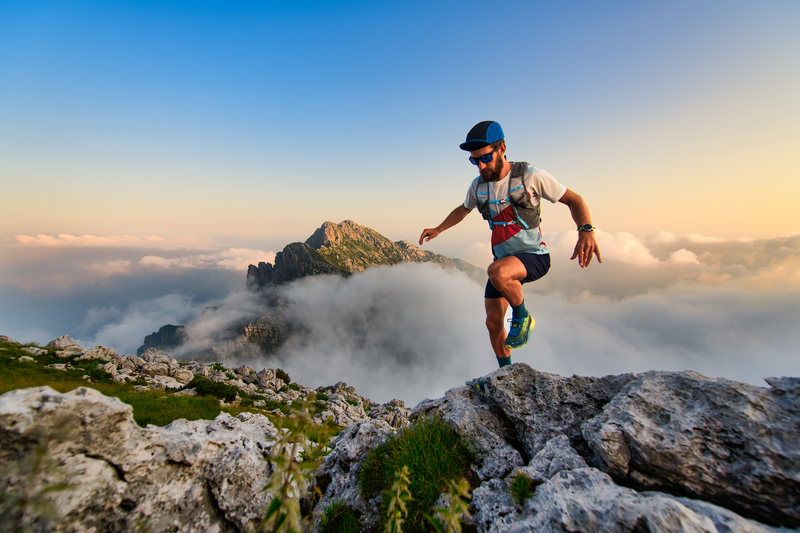
94% of researchers rate our articles as excellent or good
Learn more about the work of our research integrity team to safeguard the quality of each article we publish.
Find out more
BRIEF RESEARCH REPORT article
Front. Behav. Neurosci. , 27 August 2020
Sec. Emotion Regulation and Processing
Volume 14 - 2020 | https://doi.org/10.3389/fnbeh.2020.00154
This article is part of the Research Topic Exploring Brain Connectivity to Understand Behavior View all 10 articles
Autonomous sensory meridian response (ASMR) is a sensory phenomenon in which audio-visual stimuli evoke a tingling sensation and is accompanied by a feeling of calm and relaxation. Therefore, there has been an increasing interest in using stimuli that elicit ASMR in cognitive and clinical neuroscience studies. However, neurophysiological basis of sensory-emotional experiences evoked by ASMR remain largely unexplored. In this study, we investigated how functional connectivity is changed while watching ASMR video, compared to resting state, and assessed its potential association with affective state induced by ASMR. 28 subjects participated in fMRI experiment consisting of 2 sessions (resting-state and task of viewing ASMR-eliciting video). Using a seed-based correlation analysis, we found that functional connections between the posterior cingulate cortex, and superior/middle temporal gyri, cuneus, and lingual gyrus were significantly increased during ASMR compared to resting state. In addition, we found that with the pregenual anterior cingulate cortex seed region, functional connectivity of the medial prefrontal cortex was increased during ASMR condition, relative to resting state. These results imply that ASMR can be elicited and maintained by ongoing interaction between regional activity that are mainly involved in the mentalizing and self-referential processing. We also found that ASMR-induced affective state changes (high activation negative and high activation positive state) were negatively correlated with functional connectivity involved in visual information processing, suggesting that visual information processing in response to high arousal states can be weakened by ASMR-eliciting stimuli.
Stress is common in everyday life, and is believed to affect individual health and happiness (Segerstrom and Miller, 2004; Cohen et al., 2007). As a result, the development of stress management approaches has become an important endeavor of preventing stress-related health problems and accomplishing psychological well-being. In recent years, the autonomous sensory meridian response (ASMR) videos have been widely used in the management of stress, by inducing relaxation and sleep (Barratt and Davis, 2015; Lee et al., 2019). Specifically, ASMR is a sensory phenomenon in which individuals experience a tingling in the head and neck, in response to specific triggering audio and visual stimuli (Barratt and Davis, 2015). The ASMR triggers lead to response of psychologically pleasant effects such as feeling of relaxation, reduction in anxiety, and sleep induction (Barratt et al., 2017; Cash et al., 2018; Poerio et al., 2018).
Several studies have explored the neurophysiological basis of ASMR using functional magnetic resonance imaging (fMRI) (Smith et al., 2017, 2019; Lochte et al., 2018). Specifically, Lochte et al. (2018) examined the brain activation during ASMR, and observed significant activation in regions of the medial prefrontal cortex (mPFC), dorsal anterior cingulate cortex, supplementary motor area, and insular cortex during ASMR condition, compared to the brain activity during resting state.
Smith et al. (2017, 2019) investigated the differences of resting-state network between ASMR experienced and non-ASMR experienced individuals. Using an independent component analysis (Beckmann et al., 2005), they found that participants with ASMR had less connections of the precuneus with other regions of the default mode network (DMN) than controls. These previous studies demonstrated the associations of ASMR with the changes in regional activity and networks of resting state. However, it is still unclear how connections among brain regions are explicitly modulated by ASMR.
To address this issue, this paper focuses on the investigation of ASMR condition-specific functional connectivity changes in a brain network, compared to the resting-state functional connectivity, using 3T functional magnetic resonance imaging (fMRI). Functional connectivity was assessed using a seed-based correlation approach (Biswal et al., 1995; Whitfield-Gabrieli and Nieto-Castanon, 2012). We hypothesized that ASMR condition would change the functional connectivity within the brain network involved in mentalization and self-referential processing as a meditation effect of ASMR. This is based on a previous study (Barratt and Davis, 2015) reporting that sitting quietly while watching relaxed scenes to arouse ASMR for a certain period of time could be regarded as a form of mindfulness. Mindfulness meditation can arouse relaxed and calm states by developing a level of mentalization that controls emotion using a capacity for resilience in the face of distressed conditions (Sharp et al., 2011; Bateman and Fonagy, 2013). Also, the meditation has been known to induce positive emotion using self- and other-referential processing (Logie and Frewen, 2015). The previous study (Logie and Frewen, 2015) has shown that participants who experienced mindfulness meditation had self-positive bias that led to positively affective responses during experimental self- and other-referential processing. Therefore, based on an association of ASMR and meditation conditions, we tested our hypothesis by investigating the ASMR condition-specific connectivity changes in the DMN that are involved in the mentalizing (Lombardo et al., 2010; Mars et al., 2012), and the self- and other-networks that are associated with self- and other-referential processing (Northoff et al., 2006; Murray et al., 2015). The self-network has a function of self-specific processing, indicating non-self-/self-distinction to comprehend self in domain of perception, emotion, and cognition (Northoff et al., 2006). The other-network has a function of other-specific processing that represents other-/self-distinction in understanding others’ mental and emotional states across the domains of perception, emotion, and cognition (Murray et al., 2015).
In addition, since the ASMR triggers have been known to induce a tingling sensation as a secondary phenomenon resulting from intensely positive emotion (Barratt and Davis, 2015), we explored the changes in the functional connectivity of the affective touch network while watching the ASMR stimuli (Morrison, 2016). We selected the seed regions for the default mode, affective touch, and self-/other-networks as follows. The posterior cingulate cortex (PCC), mPFC, and left/right lateral parietal cortex (lLPC, rLPC) were used as the seed regions for the DMN, because these regions are recognized as central hubs within the network (Greicius et al., 2003). For the affective touch network, we used the right posterior insular cortex (Ig2) as a seed region based on a previous meta-analysis study (Morrison, 2016). Morrison (2016) reported a higher activation of Ig2 in response to affective touch compared with discriminative touch. Using this seed region of Ig2, they observed an affective touch network composed of bilateral clusters, including posterior and anterior insular cortex, postcentral primary, and secondary somatosensory regions. For the self- and other-networks, we used the pregenual anterior cingulate cortex (pACC) and posterior cingulate cortex/precuneus (PCC/PC) regions as seed ROIs, because these two seed regions have been reliably shown to be involved in conceptual self- and conceptual other-processing, respectively (Murray et al., 2012). The self-network consisted of the pACC and anterior insular cortex, whereas the other-network consisted of the PCC/PC and angular gyrus/temporoparietal junction (Murray et al., 2015).
Finally, using the functional connectivity estimates, we further investigated the potential association of condition-specific connectivity changes with affective state changes while watching ASMR stimuli. Our hypothesis was that the changes in functional connectivity during ASMR would be closely associated with the changes in pleasant/unpleasant emotion and arousal states during ASMR. We assessed the affective outcomes of watching ASMR video clips using the Multi-Affect Indicator (Warr, 1990; Warr et al., 2014) and then performed a correlation analysis between the functional connectivity strengths and individual scores for affective state induced by ASMR.
Twenty-eight healthy subjects (13 females, 15 males; mean age: 26.39 ± 3.77 years) participated in this study. No subjects had any history of neurological disorders. The study was approved by the Institutional Review Board (IRB) of Korea Basic Science Institute, and the experiment was performed with the understanding and written consent of each participant, according to IRB guidelines.
The experiment consisted of two sessions. In the first session, which served as a control experiment, participants underwent a 5-min resting-state fMRI scan. During this scan, participants were instructed to stare at a fixation point in the center of the screen and remain awake. The scan duration of 5 min was based on previous studies showing that estimates of resting-state functional connectivity stabilized with this acquisition time (Van Dijk et al., 2010). We also determined the specific instructions for resting-state condition (eyes closed, eyes open, or eyes fixated on a cross), based on Patriat et al. (2013). It was found that reliability in the default mode, attention, and auditory networks was the highest when subjects kept their eyes fixated on a cross.
In the second session, participants underwent ASMR task in the MRI scanner. During the scan, participants were instructed to view ASMR-eliciting video for 5 min. This video was trimmed to a length of 5 min from the full-length version of the YouTube video, which comprised repetitive and slow movements with a scratching sound (i.e., scratching of a sand table). The web address is as follows: https://youtu.be/bCFALoEfBGw. While standards for ASMR videos have not yet been extensively examined, several studies (Barratt and Davis, 2015; Fredborg et al., 2017) have established the common stimuli that elicit an intense ASMR experience, including whispering, scratching sound, and slow/repetitive movements. Therefore, we selected the content of the video clips based on these criteria. The length of ASMR video clips was set to be consistent with that of the resting-state condition because the scan length has been known to affect the reliability of fMRI connectivity estimates (Birn et al., 2013).
After completing fMRI experiments, outside the scanner, participants responded to questionnaires for assessing the changes in affective states while watching ASMR video clips (see the Behavior Data Analysis section for more details). Overall, this study consisted of three phases: the first session for resting-state experiment in the MRI scanner (5 min), the second session for ASMR experiment in the MRI scanner (5 min), and behavioral data collection outside the scanner.
All images were acquired using a 3T Philips Achieva scanner (Philips Medical Systems, Best, The Netherlands). Structural images were acquired using a three-dimensional T1-weighted sequence [repetition time (TR) = 6.6 ms; echo time (TE) = 3.1 ms; flip angle = 9°; voxel size = 1.0 × 1.0 × 1.2 mm3; field of view (FOV) = 240 mm; 170 slices]. Blood oxygenation level dependent (BOLD) images were obtained using a T2∗-weighted gradient echo-planar imaging (EPI) sequence (TR = 2000 ms; TE = 35 ms; flip angle = 79°; voxel size = 3.0 × 3.0 × 3.0 mm3, FOV = 195 mm, 34 interleaved slices without slice gap).
The functional connectivity toolbox (CONN toolbox, Whitfield-Gabrieli and Nieto-Castanon, 2012) with the statistical parametric mapping software package (SPM12, Friston et al., 2007) was used for pre-processing of the functional and structural images, and functional connectivity analysis.
The effects of head movement between scans were corrected by realigning all scans to the first image using a six-parameter affine spatial transformation; the geometric distortion was corrected by the unwarp function. The ensuing realignment parameters were saved for modeling residual head motion effects in the BOLD time series. To further mitigate motion-related BOLD effects, including spikes, we used artifact detection tools (ART, https://www.nitrc.org/projects/artifact_detect) interoperable with CONN toolbox. Specifically, outlying volumes in BOLD time series (scan “scrubbing”) were identified based on normalized global mean intensity values (>Z = 5) and motion parameters (>1 mm translational movement in the x, y, or z planes or >0.02 rotation in yaw, pitch, or roll). The matrices of outliers and realignment parameters were then entered as first-level covariates (i.e., nuisance variables). To compensate for slice-acquisition delays, the signal in each slice was realigned temporally to a reference middle slice using sinc interpolation. The structural image was co-registered with functional images and segmented into gray matter (GM), white matter (WM), and cerebrospinal fluid (CSF). All images were spatially normalized to the Montreal Neurological Institute (MNI) space. Spatial smoothing with a 6 mm full-width at half-maximum (FWHM) Gaussian kernel was applied to the normalized images.
Systemic physiological confounds arising from cardiac and respiration have been known to cause spurious correlation structures throughout the brain (Birn et al., 2006; Chang and Glover, 2009; Murphy et al., 2013). We therefore reduced systemic physiological noise using the anatomical component-based noise correction method (aCompCor) (Behzadi et al., 2007). The method has also been shown to be effective in the suppression of motion-related artifacts (Muschelli et al., 2014). Assuming that the physiological noise contribution is globally distributed, and neuronal activity-related signals are low in the WM and CSF, the signals within the WM and CSF were used as sources that primarily reflect physiological noise. The top three components obtained from each of the WM and CSF using principal component analysis were included as the nuisance regressors in the first-level analysis. In addition, to remove spurious task-induced co-activation effects, we constructed a condition-specific regressor and included it as additional temporal confounding factors by convolving a canonical hemodynamic response function with a condition (either ASMR or resting-state) spanning the entire scanner acquisition length (Fair et al., 2007; Whitfield-Gabrieli and Nieto-Castanon, 2012). Prior to the first-level connectivity analysis, these temporal confounding factors (consisting of subject movement, cardiac/respiration, and spurious parameters related to task effects) were regressed out from BOLD time series at each voxel. The resulting residual time series were then band-pass filtered in the range of 0.01–0.1 Hz to constrain the low-frequency BOLD fluctuations presumed to be related to spontaneous neural activity (Biswal et al., 1995; Leopold et al., 2003).
First-level functional connectivity maps were generated by computing Pearson’s correlation coefficients between average BOLD time series calculated across all the voxels of a given seed region and the time series of all other voxels in the brain (Biswal et al., 1995; Fox et al., 2005). The resulting correlation coefficients were converted to Z-scores using Fisher transformation (Fisher, 1915) to improve the normality assumptions of the subsequent second-level general linear model (GLM) analysis. Functional connectivity considered in our analysis was associated with (a) the DMN (Greicius et al., 2003), (b) affective touch network (Morrison, 2016), and (c) the self-/other-networks (Northoff et al., 2006; Murray et al., 2015). As seeds of the DMN, we used the PCC centered at MNI coordinates [1, −61, 38], mPFC (MNI: [1 55 −3]), and l/rLPC (lLPC, MNI: [−55 −12 29], rLPC, MNI: [56 −10 29]). The seed regions of interest (ROIs) were defined using a standardized CONN toolbox atlas (networks.nii) that was originally derived from group-level independent component analysis (ICA) of the human connectome project dataset (Calhoun et al., 2001; Whitfield-Gabrieli and Nieto-Castanon, 2012; Van Essen et al., 2013). For an affective touch network, we used the Ig2 as a seed ROI that comprised all voxels within a sphere of 6 mm radius, centered on the MNI coordinates [42, −14, 8]. Finally, for the self- and other-networks, we used the pACC and PCC/PC regions as seed ROIs (spheres of 6 mm radius, centered on MNI coordinates: [−2, 38, 16] and [2, −61, 26]).
Following the computation for the first-level functional connectivity maps, the resulting voxel-specific Z-scores between a seed area and every other voxel for each subject were entered into a second-level GLM analysis. Specifically, we performed a one-sample t-test at the second level to test the statistical significance of each functional connectivity map in a group of subjects that was generated during resting-state or ASMR conditions (ASMR). We then tested our hypothesis that functional connectivity related to mentalizing and self-referential processing within the default mode, affective touch, and self-/other-networks would be greater during an ASMR condition than the resting-state, using a two-tailed paired sample t-test with a contrast “ASMR > resting-state” at the second-level. This analysis enabled us to compare the functional connectivity patterns between two conditions, including a resting-state and an ASMR condition, and assess their statistical significance in a sample. For false positive control in the whole-brain seed-to-voxel connectivity analysis, we applied a cluster-forming threshold using a height threshold of uncorrected p-value < 0.001 and a cluster-extent threshold of false discovery rate (FDR)-corrected p-value < 0.05 (Friston et al., 1994; Whitfield-Gabrieli and Nieto-Castanon, 2012). We used a semi-automated search for finding local maxima (peaks) and their MNI coordinates within the cluster-corrected thresholded map, to identify regions within the significant functional connectivity maps. Their anatomical labels were determined using xjView toolbox (https://www.alivelearn.net/xjview), and the Brodmann area labels were identified using the Brodmann atlas, which is included in the MRIcron software (https://www.nitrc.org/projects/mricron). Functional connectivity maps were overlaid on a cortical surface atlas using the CONN toolbox (Whitfield-Gabrieli and Nieto-Castanon, 2012).
To investigate the potential association of functional connectivity estimates with the psychological changes of ASMR, we measured the affective outcomes of watching ASMR video clips using the Multi-Affect Indicator (Warr, 1990; Warr et al., 2014). This multi-affect indicator has been designed to specify different kinds of feelings in terms of two dimensions, including the conventional negative-to-positive continuum (from unpleasant to pleasant state) and low-to-high mental activation (arousal) that defines one’s state of readiness for action or energy expenditure (Russell, 2003). Particular feelings were then categorized into four affective states: low-activation positive (LAP, which corresponds to comfort and calmness), high-activation positive (HAP, related to enthusiasm and excitement), low-activation negative (LAN, related to depression and sadness), and high-activation negative states (HAN, related to anxiety and stress). In this study, we used 12 items to measure these affective states (Warr, 1990; Poerio et al., 2018): “calm,” “relaxed,” and “at ease” for LAP; “enthusiastic,” “joyful,” and “excited” for HAP; “depressed,” “dejected,” and “hopeless” for LAN; and “anxious,” “nervous,” and “tense” for HAN. After completing the fMRI experiments, the participants were asked to rate each item in the range of 1 (much less) to 7 (much more) by responding to the question: How did you feel while watching ASMR video clip during the MRI scan, compared to before you watched the video?
We then performed two-tailed paired samples t-tests to compare the means of two affective states that were selected from LAP, HAP, LAN, and HAN, and determined whether there was a significant difference between the two states that can be observed from ASMR stimuli. In addition, we performed a correlation analysis to investigate the associations of these affective state changes with ASMR condition-specific functional connectivity changes. Specifically, for each brain network, we identified clusters that had a significantly higher functional connectivity from a seed region for ASMR condition than the resting-state condition (a height threshold of uncorrected p-value < 0.001 and a cluster-extent threshold of FDR-corrected p-value < 0.05). Then, we extracted the functional connectivity values (z-score) of peak coordinates (i.e., the local maxima of the cluster) for all subjects, and calculated Pearson’s correlation coefficients between these functional connectivity strengths and individual scores for each affective state. We decided that the computed correlation value is significantly different from zero if the p-value is less than 0.05.
Figure 1 shows the group-level functional connectivity of the t-statistic in the default mode network generated during either ASMR or resting-state conditions. Statistical significance of clusters and their peak coordinates for ASMR and resting-state conditions are summarized in Tables 1, 2, respectively. While the global maxima of the functional connectivity was located in the seed cluster, in both conditions of resting-state and ASMR, the significant hubs (local maxima of the functional connectivity within the cluster) were reliably positioned in the PCC, mPFC, lLPC, rLPC, and superior/middle/inferior temporal gyri, and superior/inferior frontal gyri. For seed regions of the PCC and rLPC, the negative functional connectivity was observed in the insular cortex.
Figure 1. Group-level functional connectivity of the t-statistic in the default mode network during resting-state, and in response to ASMR effects. Functional connectivity strengths in terms of t-statistics were thresholded at a significance level of false discovery rate (FDR)-corrected p < 0.05, and overlaid on a cortical surface atlas. Functional connectivity of the posterior cingulate cortex (PCC) seed region in response to ASMR (A), and in resting-state (B). Functional connectivity of the medial prefrontal cortex (mPFC) seed region in response to ASMR (C), and in resting-state (D). Functional connectivity of the left lateral parietal cortex (lLPC) seed region in response to ASMR (E), and in resting-state (F). Functional connectivity of the right lateral parietal cortex (rLPC) seed region in response to ASMR (G), and in resting-state (H).
Table 1. Statistical significance of the group-level functional connectivity generated during ASMR condition.
Table 2. Statistical significance of the group-level functional connectivity generated during resting-state condition.
Figure 2 shows the group-level functional connectivity of the t-statistic in the affective touch, self-, and other-networks generated during either ASMR or resting-state conditions. For the affective touch network with Ig2 seed region, the significant clusters were estimated in the insular cortex and postcentral gyrus in both conditions of resting-state and ASMR. In the self-network with the pACC seed region, we found the positive functional connectivity of the anterior cingulate cortex. In other-network with the PCC/PC seed region, the positive functional connectivity was observed in the angular gyrus, precuneus, and frontal regions extending orbitofrontal and medial prefrontal cortices.
Figure 2. Group-level functional connectivity of the t-statistic in the other networks during resting-state, and in response to ASMR effects. Functional connectivity strengths in terms of t-statistics were thresholded at a significance level of false discovery rate (FDR)-corrected p < 0.05, and overlaid on a cortical surface atlas. Functional connectivity of the right posterior insular cortex seed (Ig2) region in response to ASMR (A), and in resting-state (B). Functional connectivity of the pregenual anterior cingulate cortex (pACC) seed region in response to ASMR (C), and in resting-state (D). Functional connectivity of the posterior cingulate cortex/precuneus (PCC/PC) seed regions in response to ASMR (E), and in resting state (F).
Figure 3 shows the group-level functional connectivity of the t-statistic obtained by the “ASMR > resting-state” contrast. Table 3 summarizes statistical significance of clusters functionally connected to the seed regions of the PCC, l/rLPC, pACC, and Ig2, and their peak coordinates. There were no significant clusters in the DMN with the mPFC seed region and the other-network with the PCC/PC seed region. In the DMN with the PCC seed region, 5 clusters having positive functional connectivity were significantly detected in peaks in the cuneus, superior/middle temporal gyri, and lingual gyrus. In addition, 6 clusters having negative functional connectivity were significantly detected in peaks in the superior/middle frontal gyri, middle occipital lobe, precuneus, and visual area. In the DMN with the lLPC seed region, 2 positive and 1 negative clusters were observed in peaks in the superior temporal gyrus and visual area (calcarine sulcus), and precuneus, respectively. In the DMN with the rLPC seed region, 2 positive clusters were generated in peaks in the cuneus and lingual gyrus. In the self-network with the pACC seed region, a positive cluster was detected in peaks in the middle frontal lobe. In the affective touch network with the the Ig2 seed region, one cluster having positive functional connectivity was observed in peaks in the cuneus.
Figure 3. Group-level functional connectivity of the t-statistic obtained by the “ASMR > resting-state” contrast. The default mode networks with seed regions of (A) the posterior cingulate cortex (PCC), (B) left lateral parietal cortex (lLPC), and (C) right lateral parietal cortex (rLPC). (D) The self-network with the pregenual anterior cingulate cortex (pACC) seed region. (E) Affective touch network with the posterior insular cortex (Ig2) seed region. There were no significant clusters in the default mode network with the mPFC seed region and the other-network with the PCC/PC seed region.
Table 3. Statistical significance of the group-level functional connectivity obtained by the “ASMR > resting-state” contrast.
The beta-values of the group-level functional connectivity for ASMR, resting-state, and ASMR > resting-state contrast are provided in Supplementary Figures.
There was a significant overall main effect on the affective response while watching ASMR video clips. As shown in Figure 4, participants had the most increase in low-activation positive state during the ASMR condition among four affective states that we have considered: LAP (group mean ± standard deviation: 3.94 ± 1.46), HAP (1.51 ± 0.63), LAN (1.45 ± 0.64), and HAN (1.38 ± 0.78). Statistical significance of the comparison between two selected states are as follows: LAP > HAP [beta = 2.429, t = 8.349, p = 5.86 × 10–9, df = 27, 95% confidence interval of the mean = (1.832–3.025); LAP > LAN (beta = 2.488, t = 8.471, p = 4.39 × 10–9, df = 27, 95% confidence interval of the mean = (1.885–3.091); LAP > HAN (beta = 2.560, t = 7.638, p = 3.25 × 10–8, df = 27, 95% confidence interval of the mean = (1.872–3.247)]. Table 4 summarizes the statistical significance of affective states in response to ASMR.
Figure 4. Summary of the results showing changes in affect state after viewing ASMR, relative to before watching ASMR. Bar graphs represent group mean scores for affective state assessed using the Multi-Affect Indicator (Warr, 1990). All variables range from 1 to 7. For self-reported changes in affect, 1 = much less; 7 = much more. The participants had the most increase in low-activation positive state during the ASMR condition among four affective states: low-activation positive state (group mean ± standard deviation: 3.94 ± 1.46), high-activation positive state (1.51 ± 0.63), low-activation negative state (1.45 ± 0.64), and high-activation negative state (1.38 ± 0.78). Statistical significance was determined by a p-value of less than 0.05.
Correlation coefficients between each of the four affective states and ASMR condition-specific connectivity changes are summarized in Table 5. In the DMN with the PCC seed region, significantly negative correlation was estimated between HAN and clusters with peaks in the lingual gyrus. Associations of HAP with clusters of the cuneus and lingual gyrus were also negatively correlated. In the affective touch and self-/other-networks, there were no significant correlation between the affective state scores and the ASMR-condition specific connectivity changes.
Table 5. Statistical results of correlation coefficients between each of the four affective states and ASMR condition-specific connectivity changes.
In this study, we sought to test whether changes in functional connectivity within specific networks, including the DMN, affective touch network, and self-/other-networks occurred during ASMR. As a result, relative to connectivity in the resting-state, significantly altered connectivity of seed regions during viewing of ASMR-eliciting stimulus was found in the main hub composing each network. Furthermore, we confirmed that the strength of connectivity in involved in visual information processing was negatively correlated with the behavior score, including the HAN, and HAP states. We now discuss the implications of these results in more detail.
Our results showed that in the DMN, functional connectivity between the PCC seed region and the superior/middle temporal gyri, cuneus, and lingual gyrus were significantly increased during ASMR condition, compared to the resting-state. Previous functional imaging studies (Carrington and Bailey, 2009; Spreng et al., 2009) have found that the PCC and superior temporal gyrus (STG) are involved in the “mentalizing,” also known as “theory of mind” that is an ability to make inferences about other people’s mental states [i.e., an understanding that the behaviors of others is determined by their desires, attitude, and beliefs (Frith and Frith, 2003)]. Specifically, Castelli et al. (2000) revealed that the superior temporal region was activated while watching silent or computer-presented animations, and this process was related to the attribution of mental states. Fletcher et al. (1995) reported significantly increased cerebral blood flow in the PCC during the condition necessitating the attribution of mental task. Therefore, the increased functional connectivity between the STG and PCC during ASMR condition can be associated with the increased covariance of the STG and the PCC activities compared to the resting-state, which may be interpreted as activation of mentalizing process to infer others’ mental and emotional states by observing objects and perceiving intended actions and using ourselves to simulate their experience to understand them (Blakemore and Decety, 2001; Allen et al., 2003; Frith and Frith, 2006; Liew et al., 2011; Riekki et al., 2018).
We also found the reduced connectivity between the dorsolateral prefrontal cortex (dlPFC) and the PCC during ASMR condition, compared with the resting-state. Lévesque et al. (2003) reported that the dlPFC was involved in inhibition processing such as voluntary suppression of a negative emotion (sadness) while the participants suppressed their emotional reaction to the sad stimuli. For the PCC, this region has been known to be a part of network for emotion evaluation (Lee and Siegle, 2012), including an automatic perception for the emotion salience of stimulus (Maddock, 1999). Thus, compared to the resting state, the decreased functional connectivity between the dlPFC and PCC during ASMR condition can be interpreted as the decrease in voluntary suppression of negative emotion. This process may occur due to the nature of ASMR triggers that often lead to response of psychologically pleasant effects (Poerio et al., 2018).
With the DMN of the bilateral LPC seed regions, we found that the functional connectivity between the l/rLPC seeds and the visual areas of the cuneus and calcarine sulcus was significantly higher during the ASMR condition than during the resting-state. The cuneus is involved in visual information processing that interacts with the primary visual cortex (Vanni et al., 2001) and is known to integrate somatosensory information with other sensory stimuli (Price, 2000). In addition, the LPC is involved in receiving a visual input from the occipital regions, which belong to the dorsal stream of visual processing (Rizzolatti and Matelli, 2003). In terms of the visual stimuli, in our experiment, ASMR-eliciting video clips were much richer in visual information than the instruction for resting-state condition (with eyes fixated on a cross). Therefore, greater functional connectivity of the cuneus and calcarine sulcus within the DMN may reflect the increased visual input and processing from ASMR-eliciting stimuli through functional connectivity, compared to the resting-state condition.
This study showed significant connectivity differences not only in the DMN but also in other network areas, including affective touch network, and self-network. In terms of the affective touch network, we found a greater connectivity between the Ig2 and the cuneus of the occipital region during the ASMR condition than the resting-state. The cuneus is a part of the visual areas and engages in processing of visual input (Waberski et al., 2008) and the insular cortex integrates information from multiple modalities, including visual and auditory sensory modalities (Bamiou et al., 2003). Thus, the increased connection between Ig2 and cuneus indicates the higher visuoauditory influence of ASMR stimulus.
In terms of the self-network involved in the reflection of one’s own experiences against other stimuli (Northoff et al., 2006), we found an increased connectivity between the pACC and the mPFC during ASMR condition, compared to resting-state. Murray et al. (2012) revealed that the mPFC and dorsal anterior cingulate cortex were activated in the self-referencing processing state rather than the other-relevant processing, and Gusnard et al. (2001) showed that these regions were particularly involved in self-referential processing in emotion domain. In addition, Northoff et al. (2006) reported that cortical midline structures including the mPFC and pACC mediate self-referential processing in psychological or physical domain such as autobiographical, emotional, and motor stimuli. Therefore, the increased connectivity between the pACC and the mPFC during ASMR may reflect the self-referential processing triggered by ASMR stimulus.
Although the major focus of this study is the connectivity on which the effects of ASMR are neural underpinnings, a correlation analysis was performed to investigate how these changed connections relate to the feelings felt during ASMR. As a result, in the PCC region, significantly negative correlation was estimated between clusters with peaks in the lingual gyrus and HAN. For rLPC seed region, connectivities in clusters of the lingual gyrus and cuneus were also negatively correlated in HAP. The PCC receives visual information from visual systems (Vogt et al., 2006) and the LPC also accepts visual input through dorsal stream (Rizzolatti and Matelli, 2003). The ASMR stimulus contains audio-visual stimuli that lead to a positive emotional response to calmness and a tingling sensation that emerges from a positive emotion (Barratt and Davis, 2015). Thus, these results imply that visual information processing in response to high arousal states can be weakened by ASMR-eliciting stimuli.
As a limitation of this finding, we did not explicitly measure the affective outcomes of resting state using the behavioral questionnaire [e.g., the Multi-Affect Indicator (Warr, 1990; Warr et al., 2014)]. As described in the Behavioral Data Analysis section, the participants were instructed to indicate how they felt while watching the ASMR video clip during the MRI scan, compared to before they watched the video. Therefore, individual behavioral scores that we measured may reflect relative affective states of ASMR condition to resting state. However, a control acquisition of the behavioral questionnaire after the resting state session would be required to compare the affective state changes between resting-state and ASMR conditions more explicitly. Thus, caution should be exercized when interpreting the correlation coefficient between functional connectivity estimates and behavioral scores used in this study.
In conclusion, using fMRI functional connectivity estimates, we explored the ASMR-condition specific connectivity changes in the DMN, self-/other-networks, and the affective touch network. Compared with the resting-state functional connectivity, we found that several connections within the selected networks were significantly altered while watching ASMR video. In particular, the connections between the PCC and the superior temporal gyrus, between the pACC and the mPFC, and between the Ig2 and the cuneus were significantly greater during ASMR condition than resting state. These results suggest that ASMR process can be associated with ongoing interaction between regional activity that are involved in the integration of visual and auditory information followed by the mentalizing and self-referential processing. In terms of the relationship between connectivity and affective state changes, we found that ASMR-induced affective states (i.e., high activation negative and high activation positive state) were significantly negatively correlated with functional connectivity involved in visual information processing. These results imply that high arousal states can be attenuated in the process of perception of ASMR-eliciting stimuli. Our findings have implications for neurophysiological mechanisms of an ASMR effects in relation to functional connectivity changes.
The fMRI data that support the findings of this study are available from the corresponding author on request.
The studies involving human participants were reviewed and approved by the Institutional Review Board of Korea Basic Science Institute. The patients/participants provided their written informed consent to participate in this study.
SL designed the study. SL and JK conducted the experiment and performed the fMRI data acquisition. SL and ST performed the data analysis, discussed the study idea, analysis, and results, and wrote the manuscript. All authors reviewed the manuscript.
This work was supported in part by the grants from the Korea Basic Science Institute (T38609 and C030130) and the National Research Foundation of Korea (NRF) grant funded by the Korea Government (MIST) (2019R1C1C1011281).
The authors declare that the research was conducted in the absence of any commercial or financial relationships that could be construed as a potential conflict of interest.
The Supplementary Material for this article can be found online at: https://www.frontiersin.org/articles/10.3389/fnbeh.2020.00154/full#supplementary-material
FIGURE S1 | Group-level functional connectivity of the beta-value in the default mode network during resting-state, and in response to ASMR effects. Functional connectivity of the posterior cingulate cortex seed region in response to (A) ASMR, and (B) resting-state. Functional connectivity of the medial prefrontal cortex seed region in response to (C) ASMR, and (D) resting-state. Functional connectivity of the left lateral parietal cortex seed region in response to (E) ASMR, and (F) resting-state. Functional connectivity of the right lateral parietal cortex seed region in response to (G) ASMR, and (H) resting-state.
FIGURE S2 | Group-level functional connectivity of the beta-value in the affective touch, self-, and other-networks during resting-state, and in response to ASMR effects. Functional connectivity of the right posterior insular cortex seed region in response to (A) ASMR, and (B) resting-state. Functional connectivity of the pregenual anterior cingulate cortex seed region (C) in response to ASMR, and (D) resting-state. Functional connectivity of the posterior cingulate cortex/precuneus seed region in response to (E) ASMR, and (F) resting-state.
FIGURE S3 | Group-level functional connectivity of the beta-value for ASMR > resting-state contrast. Default mode networks with seed regions of (A) the posterior cingulate cortex, (B) medial prefrontal cortex, (C) left lateral parietal cortex, and (D) right lateral parietal cortex. (E) Self-network with the pregenual anterior cingulate cortex seed region. (F) Other-network with the posterior cingulate cortex/precuneus seed region. (G) Affective touch network with the posterior insular cortex seed region.
Allen, J. G., Bleiberg, E., and Haslam-Hopwood, T. (2003). Mentalizing as a compass for treatment. Bull. Menninger Clin. 67, 1–11.
Bamiou, D., Musiek, F. E., and Luxon, L. M. (2003). The insula (Island of Reil) and its role in auditory processing: literature review. Brain Res. Rev. 42, 143–154. doi: 10.1016/s0165-0173(03)00172-3
Barratt, E. L., and Davis, N. J. (2015). Autonomous Sensory Meridian Response (ASMR): a flow-like mental state. PeerJ 3:e851. doi: 10.7717/peerj.851
Barratt, E. L., Spence, C., and Davis, N. J. (2017). Sensory determinants of the autonomous sensory meridian response (ASMR): understanding the triggers. PeerJ 5:e3846. doi: 10.7717/peerj.3846
Beckmann, C. F., DeLuca, M., Devlin, J. T., and Smith, S. M. (2005). Investigations into resting-state connectivity using independent component analysis. Philos. Trans. R. Soc. B 360, 1001–1013. doi: 10.1098/rstb.2005.1634
Behzadi, Y., Restom, K., Liau, J., and Liu, T. T. (2007). A component based noise correction method (CompCor) for BOLD and perfusion based fMRI. Neuroimage 37, 90–101. doi: 10.1016/j.neuroimage.2007.04.042
Birn, R. M., Diamond, J. B., Smith, M. A., and Bandettini, P. A. (2006). Separating respiratory-variation-related fluctuations from neuronal-activity-related fluctuations in fMRI. Neuroimage 31, 1536–1548. doi: 10.1016/j.neuroimage.2006.02.048
Birn, R. M., Molloy, E. K., Patriat, R., Parker, T., Meier, T. B., Kirk, G. R., et al. (2013). The effect of scan length on the reliability of resting-state fMRI connectivity estimates. Neuroimage 83, 550–558. doi: 10.1016/j.neuroimage.2013.05.099
Biswal, B., Zerrin Yetkin, F., Haughton, V. M., and Hyde, J. S. (1995). Functional connectivity in the motor cortex of resting human brain using echo-planar MRI. Magn. Reson. Med. 34, 537–541.
Blakemore, S. J., and Decety, J. (2001). From the perception of action to the understanding of intention. Nat. Rev. Neurosci. 2, 561–567. doi: 10.1038/35086023
Calhoun, V. D., Adali, T., Pearlson, G. D., and Pekar, J. J. (2001). A method for making group inferences from functional MRI data using independent component analysis. Hum. Brain Mapp. 14, 140–151. doi: 10.1002/hbm.1048
Carrington, S. J., and Bailey, A. J. (2009). Are there theory of mind regions in the brain? A review of the neuroimaging literature. Hum. Brain Mapp. 30, 2313–2335
Cash, D. K., Heisick, L. L., and Papesh, M. H. (2018). Expectancy effects in the autonomous sensory meridian response. PeerJ 6:e5229. doi: 10.7717/peerj.5229
Castelli, F., Happé, F., Frith, U., and Frith, C. (2000). Movement and mind: a functional imaging study of perception and interpretation of complex intentional movement patterns. Neuroimage 12, 314–325. doi: 10.1006/nimg.2000.0612
Chang, C., and Glover, G. H. (2009). Relationship between respiration, end-tidal CO2, and BOLD signals in resting-state fMRI. Neuroimage 47, 1381–1393. doi: 10.1016/j.neuroimage.2009.04.048
Cohen, S., Janicki-Deverts, D., and Miller, G. E. (2007). Psychological stress and disease. JAMA 298, 1685–1687.
Fair, D. A., Schlaggar, B. L., Cohen, A. L., Miezin, F. M., Dosenbach, N. U., Wenger, K. K., et al. (2007). A method for using blocked and event-related fMRI data to study “resting state” functional connectivity. Neuroimage 35, 396–405. doi: 10.1016/j.neuroimage.2006.11.051
Fisher, R. A. (1915). Frequency distribution of the values of the correlation coefficient in samples from an indefinitely large population. Biometrika 10, 507–521. doi: 10.1093/biomet/10.4.507
Fletcher, P. C., Happe, F., Frith, U., Baker, S. C., Dolan, R. J., Frackowiak, R. S., et al. (1995). Other minds in the brain: a functional imaging study of” theory of mind” in story comprehension. Cognition 57, 109–128. doi: 10.1016/0010-0277(95)00692-r
Fox, M. D., Snyder, A. Z., Vincent, J. L., Corbetta, M., Van Essen, D. C., and Raichle, M. E. (2005). The human brain is intrinsically organized into dynamic, anticorrelated functional networks. Proc. Natl. Acad. Sci. U.S.A. 102, 9673–9678. doi: 10.1073/pnas.0504136102
Fredborg, B., Clark, J., and Smith, S. D. (2017). An examination of personality traits associated with autonomous sensory meridian response (ASMR). Front. Psychol. 8:247.
Friston, K., Ashburner, J., Kiebel, S., Nichols, T., and Penny, W. (2007). Statistical Parametric Mapping: The Analysis of Functional Brain Images. Boston: Academic Press.
Friston, K. J., Worsley, K. J., Frackowiak, R. S., Mazziotta, J. C., and Evans, A. C. (1994). Assessing the significance of focal activations using their spatial extent. Hum. Brain Mapp. 1, 210–220. doi: 10.1002/hbm.460010306
Frith, C. D., and Frith, U. (2006). The neural basis of mentalizing. Neuron 50, 531–534. doi: 10.1016/j.neuron.2006.05.001
Frith, U., and Frith, C. D. (2003). Development and neurophysiology of mentalizing. Philos. Trans. R. Soc. Lond. Ser. B Biol. Sci. 358, 459–473.
Greicius, M. D., Krasnow, B., Reiss, A. L., and Menon, V. (2003). Functional connectivity in the resting brain: a network analysis of the default mode hypothesis. Proc. Natl. Acad. Sci. U.S.A. 100, 253–258. doi: 10.1073/pnas.0135058100
Gusnard, D. A., Akbudak, E., Shulman, G. L., and Raichle, M. E. (2001). Medial prefrontal cortex and self-referential mental activity: relation to a default mode of brain function. Proc. Natl. Acad. Sci. U.S.A. 98, 4259–4264. doi: 10.1073/pnas.071043098
Lee, K. H., and Siegle, G. J. (2012). Common and distinct brain networks underlying explicit emotional evaluation: a meta-analytic study. Soc. Cogn. Affect. Neurosci. 7, 521–534. doi: 10.1093/scan/nsp001
Lee, M., Song, C. -B., Shin, G. -H., and Lee, S. −W. (2019). Possible effect of binaural beat combined with autonomous sensory meridian response for inducing sleep. Front. Hum. Neurosci. 13:425.
Leopold, D. A., Murayama, Y., and Logothetis, N. K. (2003). Very slow activity fluctuations in monkey visual cortex: implications for functional brain imaging. Cereb. Cortex 13, 422–433. doi: 10.1093/cercor/13.4.422
Lévesque, J., Eugène, F., Joanette, Y., Paquette, V., Mensour, B., Beaudoin, G., et al. (2003). Neural circuitry underlying voluntary suppression of sadness. Biol. Psychiatry 53, 502–510. doi: 10.1016/s0006-3223(02)01817-6
Liew, S. L., Han, S., and Aziz-Zadeh, L. (2011). Familiarity modulates mirror neuron and mentalizing regions during intention understanding. Hum. Brain Mapp. 32, 1986–1997. doi: 10.1002/hbm.21164
Lochte, B. C., Guillory, S. A., Richard, C. A., and Kelley, W. M. (2018). An fMRI investigation of the neural correlates underlying the autonomous sensory meridian response (ASMR). Bioimpacts 8:295. doi: 10.15171/bi.2018.32
Logie, K., and Frewen, P. (2015). Self/other referential processing following mindfulness and loving-kindness meditation. Mindfulness 6, 778–787. doi: 10.1007/s12671-014-0317-z
Lombardo, M. V., Chakrabarti, B., Bullmore, E. T., and Wheelwright, S. J. (2010). Shared neural circuits for mentalizing about the self and others. J. Cogn. Neurosci. 22, 1623–1635. doi: 10.1162/jocn.2009.21287
Maddock, R. J. (1999). The retrosplenial cortex and emotion: new insights from functional neuroimaging of the human brain. Trends Neurosci. 22, 310–316. doi: 10.1016/s0166-2236(98)01374-5
Mars, R. B., Neubert, F. X., Noonan, M. P., Sallet, J., Toni, I., and Rushworth, M. F. (2012). On the relationship between the “default mode network” and the “social brain”. Front. Hum. Neurosci. 6:189.
Morrison, I. (2016). ALE meta-analysis reveals dissociable networks for affective and discriminative aspects of touch. Hum. Brain Mapp. 37, 1308–1320. doi: 10.1002/hbm.23103
Murphy, K., Birn, R. M., and Bandettini, P. A. (2013). Resting-state fMRI confounds and cleanup. Neuroimage 80, 349–359. doi: 10.1016/j.neuroimage.2013.04.001
Murray, R. J., Debbané, M., Fox, P. T., Bzdok, D., and Eickhoff, S. B. (2015). Functional connectivity mapping of regions associated with self-and other-processing. Hum. Brain Mapp. 36, 1304–1324. doi: 10.1002/hbm.22703
Murray, R. J., Schaer, M., and Debbané, M. (2012). Degrees of separation: a quantitative neuroimaging meta-analysis investigating self-specificity and shared neural activation between self-and other-reflection. Neurosci. Biobehav. Rev. 36, 1043–1059. doi: 10.1016/j.neubiorev.2011.12.013
Muschelli, J., Nebel, M. B., Caffo, B. S., Barber, A. D., Pekar, J. J., and Mostofsky, S. H. (2014). Reduction of motion-related artifacts in resting state fMRI using aCompCor. Neuroimage 96, 22–35. doi: 10.1016/j.neuroimage.2014.03.028
Northoff, G., Heinzel, A., de Greck, M., Bermpohl, F., Dobrowolny, H., and Panksepp, J. (2006). Self-referential processing in our brain: a meta-analysis of imaging studies on the self. Neuroimage 31, 440–457 doi: 10.1016/j.neuroimage.2005.12.002
Patriat, R., Molloy, E.K., Meier, T.B., Kirk, G.R., Nair, V.A., Meyerand, M.E., Prabhakaran, V., and Birn, R.M. (2013). The effect of resting condition on resting-state fMRI reliability and consistency: a comparison between resting state with eyes open, closed, and fixated. Neuroimage 78, 463–473.
Poerio, G. L., Blakey, E., Hostler, T. J., and Veltri, T. (2018). More than a feeling: autonomous sensory meridian response (ASMR) is characterized by reliable changes in affect and physiology. PLoS One 13:e0196645. doi: 10.1371/journal.pone.0196645
Price, D. D. (2000). Psychological and neural mechanisms of the affective dimension of pain. Science 288, 1769–1772. doi: 10.1126/science.288.5472.1769
Riekki, T., Svedholm-Häkkinen, A. M., and Lindeman, M. (2018). Empathizers and systemizers process social information differently. Soc. Neurosci. 13, 616–627. doi: 10.1080/17470919.2017.1368700
Rizzolatti, G., and Matelli, M. (2003). Two different streams form the dorsal visual system: anatomy and functions. Exp. Brain Res. 153, 146–157. doi: 10.1007/s00221-003-1588-0
Russell, J. A. (2003). Core affect and the psychological construction of emotion. Psychol. Rev. 110, 145–172.
Segerstrom, S. C., and Miller, G. E. (2004). Psychological stress and the human immune system: a meta-analytic study of 30 years of inquiry. Psychol. Bull. 130, 601–630. doi: 10.1037/0033-2909.130.4.601
Sharp, C., Pane, H., Ha, C., Venta, A., Patel, A. B., Sturek, J., et al. (2011). Theory of mind and emotion regulation difficulties in adolescents with borderline traits. J. Am. Acad. Child. Adolesc. Psychiatry 50, 563–573.
Smith, S. D., Fredborg, B. K., and Kornelsen, J. (2019). Atypical functional connectivity associated with autonomous sensory meridian response: an examination of five resting-state networks. Brain 9, 508–518
Smith, S. D., Katherine Fredborg, B., and Kornelsen, J. (2017). An examination of the default mode network in individuals with autonomous sensory meridian response (ASMR). Soc. Neurosci. 1, 361–365. doi: 10.1080/17470919.2016.1188851
Spreng, R. N., Mar, R. A., and Kim, A. S. (2009). The common neural basis of autobiographical memory, prospection, navigation, theory of mind, and the default mode: a quantitative meta-analysis. J. Cogn, Neurosci. 21, 489–510. doi: 10.1162/jocn.2008.21029
Van Dijk, K. R., Hedden, T., Venkataraman, A., Evans, K. C., Lazar, S. W., and Buckner, R. L. (2010). Intrinsic functional connectivity as a tool for human connectomics: theory, properties, and optimization. J. Neurophysiol. 103, 297–321.
Van Essen, D. C., Smith, S. M., Barch, D. M., Behrens, T. E., Yacoub, E., Ugurbil, K., et al. (2013). The WU-Minn human connectome project: an overview. Neuroimage 80, 62–79.
Vanni, S., Tanskanen, T., Seppä, M., Uutela, K., and Hari, R. (2001). Coinciding early activation of the human primary visual cortex and anteromedial cuneus. Proc. Natl. Acad. Sci. U.S.A. 98, 2776–2780.
Vogt, B. A., Vogt, L., and Laureys, S. (2006). Cytology and functionally correlated circuits of human posterior cingulate areas. Neuroimage 29, 452–466.
Waberski, T. D., Gobbelé, R., Lamberty, K., Buchner, H., Marshall, J. C., and Fink, G. R. (2008). Timing of visuo-spatial information processing: electrical source imaging related to line bisection judgements. Neuropsychologia 46, 1201–1210.
Warr, P. (1990). The measurement of well-being and other aspects of mental health. J. Occup. Psychol. 63, 193–210.
Warr, P., Bindl, U. K., Parker, S. K., and Inceoglu, I. (2014). Four-quadrant investigation of job-related affects and behaviours. Eur. J. Work Org. Psychol. 23, 342–363.
Keywords: autonomous sensory meridian response, functional connectivity, functional magnetic resonance imaging, default mode network, affective touch network, self-network
Citation: Lee S, Kim J and Tak S (2020) Effects of Autonomous Sensory Meridian Response on the Functional Connectivity as Measured by Functional Magnetic Resonance Imaging. Front. Behav. Neurosci. 14:154. doi: 10.3389/fnbeh.2020.00154
Received: 24 March 2020; Accepted: 04 August 2020;
Published: 27 August 2020.
Edited by:
India Morrison, Linköping University, SwedenReviewed by:
Simone Di Plinio, University of Studies “G. d’Annunzio” Chieti-Pescara, ItalyCopyright © 2020 Lee, Kim and Tak. This is an open-access article distributed under the terms of the Creative Commons Attribution License (CC BY). The use, distribution or reproduction in other forums is permitted, provided the original author(s) and the copyright owner(s) are credited and that the original publication in this journal is cited, in accordance with accepted academic practice. No use, distribution or reproduction is permitted which does not comply with these terms.
*Correspondence: Sungho Tak, c3Rha0BrYnNpLnJlLmty
Disclaimer: All claims expressed in this article are solely those of the authors and do not necessarily represent those of their affiliated organizations, or those of the publisher, the editors and the reviewers. Any product that may be evaluated in this article or claim that may be made by its manufacturer is not guaranteed or endorsed by the publisher.
Research integrity at Frontiers
Learn more about the work of our research integrity team to safeguard the quality of each article we publish.