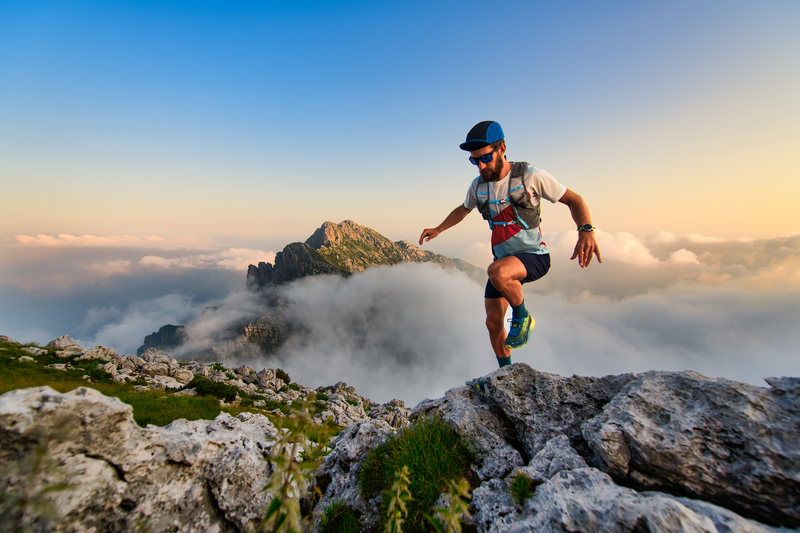
94% of researchers rate our articles as excellent or good
Learn more about the work of our research integrity team to safeguard the quality of each article we publish.
Find out more
MINI REVIEW article
Front. Behav. Neurosci. , 05 February 2019
Sec. Individual and Social Behaviors
Volume 13 - 2019 | https://doi.org/10.3389/fnbeh.2019.00012
This article is part of the Research Topic Fundamentals of 21st Century Neuroscience View all 32 articles
Neuroscience is enjoying a renaissance of discovery due in large part to the implementation of next-generation molecular technologies. The advent of genetically encoded tools has complemented existing methods and provided researchers the opportunity to examine the nervous system with unprecedented precision and to reveal facets of neural function at multiple scales. The weight of these discoveries, however, has been technique-driven from a small number of species amenable to the most advanced gene-editing technologies. To deepen interpretation and build on these breakthroughs, an understanding of nervous system evolution and diversity are critical. Evolutionary change integrates advantageous variants of features into lineages, but is also constrained by pre-existing organization and function. Ultimately, each species’ neural architecture comprises both properties that are species-specific and those that are retained and shared. Understanding the evolutionary history of a nervous system provides interpretive power when examining relationships between brain structure and function. The exceptional diversity of nervous systems and their unique or unusual features can also be leveraged to advance research by providing opportunities to ask new questions and interpret findings that are not accessible in individual species. As new genetic and molecular technologies are added to the experimental toolkits utilized in diverse taxa, the field is at a key juncture to revisit the significance of evolutionary and comparative approaches for next-generation neuroscience as a foundational framework for understanding fundamental principles of neural function.
There are over 1.5 million described, living species of animals; all but a few thousand have nervous systems and nervous system-generated behaviors. Like all characteristics of organisms, nervous systems and behaviors evolve by descent with modification in which selective forces can preserve ancestral traits and amplify freshly generated variation (Figure 1). Selection on nervous system anatomy occurs indirectly, as an intermediate to the genome, where variation originates. It is function—including behavior—rather than structure that is under direct evolutionary selection. Conversely, behaviors are constrained by nervous system architecture, which in turn is determined by a developmental program encoded in genomes (Alexander, 1974; Emlen and Oring, 1977; Agrawal, 2001; Lamichhaney et al., 2015; Session et al., 2016) and phylogenetic history (Ryan et al., 1990; Shaw, 1995; Rosenthal and Evans, 1998; Ng et al., 2014; Odom et al., 2014). Yet despite such constraints imposed on how these systems evolve, plasticity afforded by various processes throughout the nervous system affect how behaviors actually manifest in individuals in response to its unique experiences in the environment (Meyrand et al., 1994; Gross et al., 2010). Ultimately these dynamic relationships have undoubtedly fueled many facets of biological diversity. While the modern experimental toolkit has provided unprecedented glimpses into the intricacies of neural systems, phylogenetic approaches that leverage species differences are pivotal keystones for elucidating the structure and function of neural architecture.
Figure 1. Circular evolutionary tree of representative animal taxa that emerged following the evolution of the nervous and vestibular systems. While junctions of branches represent the degree of phylogenetic relatedness over evolution, distance along the tree does no scale with actual time in natural history. Colored circles indicate last common ancestor for phylogenetic groups that exhibited a particular characteristic of the nervous system which can be used to reconstruct shared, unique and convergent features of nervous systems. The central nervous system emerged early in animal evolution and is homologous across multiple taxa, while granular prefrontal cortex is a unique property of primate brains. Likewise, the independent evolution of centralized brains in vertebrates and multiple invertebrate taxa—insects and cephalopods—represents an example of convergent evolution. The more commonly used “model” organisms in neuroscience are listed in brackets below their taxonomic groups, though this list is not exhaustive.
The arrival of genetically encoded tools to investigate neuronal circuitry has accelerated our rate of discovery in the past decades, but it has come at a cost to the study of species diversity. Ironically, comparative neuroscience that explores a range of species, nervous system organizations, and behaviors has convincingly shown that detailing the nuances of neuronal microcircuitry are critical to understanding behavior (Kepecs and Fishell, 2014; Ben Haim and Rowitch, 2017; Real et al., 2017; Wamsley and Fishell, 2017). However, with an increased reliance on a small number of animal models, we are often left making assumptions about whether a discovered neuronal process reflects a common principle of brain organization and function or is specific to a particular taxon and its biology. Without well-framed, phylogenetically informed species comparisons, the significance of differences between any two species is difficult to understand. Both rodents and primates, for example, have afferent dopaminergic projections from the substantia nigra pars compacta (SNc) to the striatum (nigrostriatal pathways). But whereas in primates the SNc also project to areas of the dorsolateral frontal cortex (nigrocortical pathways), the analogous pathways are essentially absent in rodents (Wiliams and Goldman-Rakic, 1998). Interestingly, the emergence of nigrocortical pathways in primates is correlated with a marked increase of dopamine receptors in frontal cortex (Murray et al., 1994; Düzel et al., 2009). Determining the functional significance of this circuit difference on behavior cannot be ascertained without a more thorough understanding of differences across mammalian taxa more closely related to primates—tree shrews (Scandentia) and flying fox (Dermoptera)—and closer relatives of rodents, such as rabbits (Lagomorpha). Ultimately, these species’ nervous systems comprise some characteristics that are homologous due to common ancestry, some characteristics evolved due to shared selection pressures—reflecting convergence across taxa—and other characteristics that are unique (Figure 1; Kaas, 2013; Karten, 2013; Roth, 2015). Both shared and uniquely adapted characteristics have illuminated our understanding of nervous systems, often in different and complementary ways, but distinguishing between these possibilities can only be accomplished through comparative research. Leveraging the extraordinary resolution afforded by modern molecular technologies within a comparative framework offers a formidable approach to explicating the functional motifs of nervous systems.
The single most powerful method for identifying common principles of neural circuit organization is phylogenetic mapping. Characteristics are mapped onto a well-supported phylogenetic tree that is of appropriate resolution and species richness to the question being addressed (Felsenstein, 1985; Harvey and Krebs, 1991; Harvey and Pagel, 1991; Clark et al., 2001; Krubitzer and Kaas, 2005; Hale, 2014; Striedter et al., 2014; Liebeskind et al., 2016). This approach makes it possible to distinguish the evolutionary origin of a particular property of the brain or nervous system and generate testable hypotheses about its functional significance based on phylogenetic history (Barton et al., 2003; Harrison and Montgomery, 2017; Laubach et al., 2018). In some cases, homologous traits have a long history—such as the hindbrain or spinal column of vertebrates (Hirasawa and Kuratani, 2015)—whereas others only occur in small groups of closely related organisms or single species (Gould, 1976; Catania and Kaas, 1996; Douglas et al., 1998; Shepherd, 2010; Albertin et al., 2015). Characteristics that appear in multiple groups independently are examples of convergent evolution, or homoplasy. Notably, both homologous and homoplasious features have illuminated our understanding of nervous systems. Discoveries of common principles reveal the core building blocks of nervous systems or architectural features that may have biomimetic utility in engineering. Likewise, specialist adaptations in highly niche-adapted species yield critical data on how specific neural circuits evolved to solve key challenges and can serve as powerful heuristics for investigating other species, including humans. Each scenario for nervous system evolution offers the opportunity to better understand neural function and elucidate their dynamical processes. A phylogenetic framework offers a valuable tool to next-generation neuroscience that, when wielded correctly, can drive new frontiers of discovery not only in classic biological disciplines but in fields involving human-engineered systems, such as artificial intelligence and robotics.
Recent studies demonstrate the power of phylogenetic tools in addressing critical questions in neuroscience (Montgomery et al., 2011; Laubach et al., 2018). Gómez-Robles et al. (2017), for example, used evolutionary simulations and a multiple-variance Brownian motion framework to reconstruct hypotheses of ancestral states to examine the classic hypothesis of a relationship of dental reduction to brain size increase in hominins. Their data rejected this idea and indicated different patterns of evolution for tooth reduction and for brain size. Likewise, DeCasien et al. (2017) tested the social brain hypothesis that the large size and complexity of the human brain were driven by increasing social complexity and advantage of a larger, more complex brains in primate ancestors. The researchers used phylogenetic generalized least squares regression of traits with a rigorously derived phylogeny based on the 10KTrees primate resource and other controls. This rigorous analysis showed that sociality is better predicted by diet, specifically frugivory, than it can be explained by other social factors. They suggest that various aspects of foraging, such as retaining complex spatial information, may have benefitted from a larger and more complex brain. These examples illustrate how a phylogenetic framework offers a valuable tool to modern neuroscience, but new approaches make it possible to ask even more precise questions.
Advances in modern molecular neuroscience make it possible to further refine comparative questions about nervous systems by more explicating the relationship between genes and phenotypic expression. This can be accomplished by measuring the strength of evolutionary selection on a gene by calculating a dN/dS ratio (e.g., selection+neutral/neutral). In this approach, a ratio below 1 would indicate negative selection acting on the gene, whereas positive selection would be indicated by a ratio greater than 1. By comparing dN/dS ratios across a large number of species, one can more precisely map positive and negative evolutionary changes within the nervous system (Enard, 2014). For example, primates have notably high encephalization quotients (i.e., brain to body size ratio) than other mammals but questions remain about the evolutionary forces that drove this facet of selection in primates (Preuss, 2007; Dunbar and Shultz, 2017), including the genes that regulated the change. Notably not all primates have large brains, as the overall size of species’ brains within this order varies considerably ranging from large bodied apes on one end of the spectrum, such as humans and gorillas, and Callitrichid monkeys—tamarins and marmosets—on the other end. This latter family of New World monkeys has notably undergone miniaturization during primate evolution (Harris et al., 2014; Miller et al., 2016). To more precisely explore questions of brain size evolution within primates, Mongtomery and colleagues calculated dN/dS ratios for several genes associated with microcephaly across 20 anthropoid monkey species (Montgomery et al., 2011; Montgomery and Mundy, 2012b). While at least three genes revealed positive selection across these primate species, the most compelling case for a genetic correlate of brain size in primates was for ASPM. This gene not only covaried with increased brain size across most primates, but a decrease in brain size in the small bodied Callitrichid monkeys (Montgomery and Mundy, 2012a). While brain size is one broad phenotype in which to perform such comparative analyses, the same phylogenetic approach could be utilized across numerous properties of a nervous systems’ functional architecture and behavior (Krubitzer and Kaas, 2005). When wielded correctly, this powerful comparative method can be implemented to resolve existing debates and drive new frontiers of discovery.
The utilization of phylogenetics in neuroscience has a long and rich history. In mammals, detailed neuroanatomical investigations complementing quantitative studies of behavior across a diversity of species have fueled hypotheses about the functional organization of nervous systems and the mechanisms underlying a diversity of neural processes (Wells, 1978; Young, 1978; Kaas, 1986, 2013; Karten, 1991; Strausfeld, 1995, 2009, 2012; Kotrschal et al., 1998; Strausfeld et al., 1998; Krubitzer, 2000; Rodríguez et al., 2002; Jarvis et al., 2005; Krubitzer and Kaas, 2005; Krubitzer and Seelke, 2012; Striedter et al., 2014). In many respects, the limiting factor to explicating these hypotheses has been the available functional tools to examine the mammalian brain in vivo with the same level of detail available to neuroanatomists. Despite its precise temporal and spatial resolution, neurophysiological recordings are largely blind to the finer details of neural architecture—such as cell types and layers—while the poor spatial and temporal resolution of functional neuroimaging limits its utility to examine key cellular and population-level processes fundamental to nervous system function. Likewise, traditional techniques to functionally manipulate the neural structure, such as electrical microstimulation and pharmacological manipulations, generally impact relatively large populations of neurons. Due to such technological limitations, experimental questions have historically been constrained to broader-scale issues about brain function, such as the role of particular areas or nuclei for a given behavior or task. The development of next-generation molecular technologies opened the door to examine nervous systems with a level of resolution that was not previously possible (Stosiek et al., 2003; Boyden et al., 2005; Mank et al., 2008; Deisseroth, 2015). Perhaps not surprisingly, many of the functional data emerging from the implementation of these molecular methods have supported established anatomical observations and conceptual models suggesting that the fine details of neural circuitry—cell types, patterns of projections, connectivity, et cetera—are pivotal to describing the functional architecture of neural systems that support behavior. Leveraging the power of precision afforded by genetic tool kits in order to explore functional circuitry is a defining feature of modern neuroscience, yet a continued appreciation of species diversity within a phylogenetic framework may be essential to unlock the deepest mysteries of nervous systems (Carlson, 2012; Yartsev, 2017).
A key advantage of a comparative framework in neuroscience is that it provides a powerful tool for testing hypotheses of structure and function in nervous systems. The significance of establishing homology and examining convergent systems is highlighted by work in the motor system of sea slugs where phylogenetically framed studies have shown that what might be classified as a single behavior in this group of organisms has arisen multiple times and with different neural circuit underpinnings (Katz, 2011, 2016). Multiple evolutionary events leading to an association of traits can also support arguments for the relationship between structure and function that might be predicted but not testable by studying one or several individual species without consideration of phylogeny. For example, Aiello et al. (2017) argued an example of the evolutionary tuning of mechanosensation to biomechanical properties of fish fins. They showed that while the basal condition was a very flexible fin consistently across multiple lineages, when stiff fins evolved there was corresponding increase in the sensitivity of mechanosensory afferent (Aiello et al., 2017). In both sea slug and fish examples, access to a group of closely related organisms with a known phylogeny was essential. Such comparative phylogenetic framing would be of limited value among the few traditional genetic model organisms. Ultimately, most species’ neural systems comprise each of these characteristics, reflecting common principles that were inherited and maintained and the evolution of derived mechanisms to support idiosyncratic behaviors of the species. A comparative framework not only allows one to make these distinctions but to determine whether a characteristic is itself an adaptation or the byproduct of other evolutionary forces—a spandrel (Gould and Lewontin, 1979)—with little functional significance.
Consider, for example, the mammalian neocortex. This six-layered brain structure is unique to the taxonomic group and its occurrence in all extant mammals suggesting that it evolved early in mammalian evolution when these synapsids first emerged ~300 mya (Krubitzer and Kaas, 2005). Comparative anatomy and physiology suggest that many characteristics of the avian and reptilian brain—comprised of nuclei and a three-layered cortex—are shared with the mammalian brain (Jarvis et al., 2005; Karten, 2013; Calabrese and Woolley, 2015). Neurons and circuits do not arise de novo as new or altered functions evolve, but rather are adapted from preexisting morphology and developmental programs. The evolutionary history of neurons and circuits and how they differ among taxa provide critical information for interpreting circuit organization in related taxa. Dugas-Ford et al. (2012) used fluorescence in situ hybridization to examine expression of genes to show that cell types within the mammalian neocortical layer IV input and layer V output circuit are homologous with the parallel substrates in the avian brain. Consistent with various evolutionary examples, this suggests that many of the computational foundations of the sauropsid brain were conserved during the evolution of neocortex, presumably because they remained optimal for facets of neural function (Shepherd and Rowe, 2017). We must, however, also ask what computational advantage the derivation of the 6-layered neocortex may have afforded mammals that were constrained by the functional architecture of the avian/reptilian brain (Shepherd, 2011), particularly given the metabolic costs associated with the increased encephalization quotient in neocortex (Isler and van Schaik, 2006). A strategy involving detailed behavioral and neuroanatomical comparisons across species implemented in tandem with modern molecular technologies is ultimately needed to resolve these issues.
The statistician George E. P. Box famously stated that “All models are wrong, but some are useful” (Box, 1979). Revisiting this sentiment is particularly meaningful at this point in time because of our increased reliance on “model” organisms in neuroscience today (Brenowitz and Zakon, 2015; Goldstein and King, 2016; Yartsev, 2017). Whereas anatomical data have historically come from an impressive diversity of species, the weight of work implementing modern molecular approaches in nervous systems has been performed on increasingly fewer animal species. In most cases, these species have been selected for study due to their amenability to transgenic manipulation of their genome, but without clear understanding of the evolutionary origins of the traits being investigated. In some model organisms, for example, the ease of culture and embryo manipulation, limited neuron population size, and accessibility into the nervous system have provided opportunities to investigate neurons and circuits at levels not possible in humans (e.g., C. elegans, White et al., 1986; Venkatachalam et al., 2015; Markert et al., 2016; Jang et al., 2017; Yan et al., 2017), fruit fly (Malsak et al., 2013; Nern et al., 2015; Fushiki et al., 2016), zebrafish (Liu and Fetcho, 1999; Ahrens et al., 2012, 2013; Nauman et al., 2016; Hildebrand et al., 2017), and mice (Glickfeld et al., 2013; Issa et al., 2014; Glickfeld and Olsen, 2017; Guo et al., 2017). By focusing inquiry to these genetic models, we have made considerable discoveries about particular facets of these neural systems. At the same time, the limits of this strategy are increasingly evident. To assume that any single species represents an archetypal brain with unquestioned parallels to humans belies a misunderstanding of evolutionary forces that drive the phylogenetic diversity of nervous systems, particularly given the many known neuroanatomical, physiological and genetic differences across taxa (Bolker, 2012). Superficial similarities may mislead, as brains ultimately should be examined and data interpreted in the context of a species taxonomic lineage. While broad species comparisons can identify gross level similarities, the tactic of leveraging molecular technologies to more precisely explicate shared and derived characteristics of nervous systems across diverse taxa has the potential to be the spine in the next chapters of Neuroscience.
The challenges of utilizing a single model organism—mice—as a model of human disease from a phylogenetic perspective is clearly evident in the context of neuropharmacology. Neuropharmaceuticals identified in mouse-model screens have notoriously failed human clinical trials (Hyman, 2013). Despite the importance of this issue, few failed clinical trials have been investigated retrospectively and the underlying problems remain. This situation necessitates new technologies and phylogenetic approaches to address this fundamental gap. In particular, a revolutionary new technology named Drugs Acutely Restricted by Tethering (DART) offers an unprecedented capacity to selectively deliver clinical drugs to genetically defined cell-types, offering a means to revolutionize our understanding of the circuit mechanisms of neuropharmacological treatments (Shields et al., 2017). Comparative biology will be critical in realizing the full potential of such novel methodologies (Goldstein and King, 2016). For example, while the findings offered by DART in a mouse model of Parkinson’s disease were remarkable, it remains to be tested whether similar effects would occur in primates, including humans, given substantive differences in the basal ganglia between rodents and primates (Petryszyn et al., 2014). These cross-taxa differences include the division of the dorsal striatum into two distinct structures—caudate nucleus and putamen—in primates (Joel and Weiner, 2000). The known circuit differences likely reflect important properties of how the areas of basal ganglia interact with neocortex to support aspects of primate motor behavior that are distinct from those in rodents. It is differences in both functional brain architecture and broader physiology that limit the predictive value of mice as a model of human disease (O’Collins et al., 2006; Sena et al., 2006; Manger et al., 2008; Lin et al., 2014; Grow et al., 2016; Perlman, 2016). By implementing molecular tools within a phylogenetic framework, the functional differences between species could be more precisely examined—at multiple scales of molecular and cellular specificity—to more explicitly test their relationship, identify the key sources of variance and, therefore, increase translational success.
Beyond biomedical implications, a comparison of neural network architecture across taxa in the context of selective behaviors may help design artificial neural networks tailored to artificial intelligence tasks that were previously intractable. Even the simple ideas—such as reinforcement learning—when implemented suitably in the modern context, has yielded automated programs that can defeat humans at the game of Go (Silver et al., 2017). Such a task was previously deemed too difficult for computational approaches. However, the theoretical basis of the improved performance of these artificial neural networks is only beginning to be understood (Marcus, 2018). The comparative approach—and its potential for leading to theoretical understanding—promises to be important for engineering and social applications outside of biomedicine.
Describing the full, synapse by synapse, connectivity of a neural network, dubbed its “connectome,” provides unmatched structural information to inform organizational principles and function and to interpret associated network physiology and behavior. Here use of biodiversity and phylogenetically-informed taxon selection and comparisons would provide exceptional value. Complete reconstructions of processes in the neuropil and synaptic connectivity matrices are being obtained in the nervous systems of invertebrates including the foundational full network model of C. elegans (White et al., 1986), as well as Drosophila (Takemura et al., 2017a,b), Platynereis (Randel et al., 2014), and Hydra (Bosch et al., 2017). These species have significantly smaller nervous systems and fewer neurons and thus are more tractable than vertebrates for comprehensive circuit analysis. One drawback of the electron microscopy (EM) based reconstructions is the lack of information about neurotransmitters and neuromodulators. However, correlative physiological information is now possible to obtain by measuring activity using Ca++ indicators (Bock et al., 2011). This indicates the need to combine data sets across modalities. As yet, the distribution of such EM reconstructions across the phylogeny is sparse. As these data sets grow and the number of species studied broadens, there will be increased opportunities to compare across taxa. For such comparisons of networks a phylogenetic framework will be critical for interpreting variation across taxa (Katz, 2011; Katz and Hale, 2017).
A diverse set of species have laid the foundation for our field (Figure 1). Although we have increasingly relied on a handful of genetic models to push new frontiers of discovery, the stage is set to expand that empirical horizon considerably. As the process of developing new genetically modified organisms becomes easier and cheaper (Sparrow et al., 2000; Sasaki et al., 2009; Takagi et al., 2013; Abe et al., 2015; Okano et al., 2016; Park et al., 2016; Sato et al., 2016; Okano and Kishi, 2018), the potential for the CRISPR/Cas9 system to be applied across many taxa (Niu et al., 2014; Tu et al., 2015) and the increased selectivity afforded by viral approaches (Dimidschtein et al., 2016), the feasibility of applying powerful next-generation molecular tools to a broader diversity of species is increasingly possible (Leclerc et al., 2000; Izpisua Belmonte et al., 2015; Sadakane et al., 2015; Ferenczi et al., 2016; Liberti et al., 2016; MacDougall et al., 2016; Picardo et al., 2016; Roy et al., 2016; Santisakultarm et al., 2016; Kornfeld et al., 2017; Shields et al., 2017). Furthermore, other non-genetic technological advances, such as those involved in systematic mapping of neural architecture at EM and light microscopy (LM) scales (Bohland et al., 2009; Osten and Margrie, 2013; Oh et al., 2014; Kornfeld et al., 2017), as well as associated advanced analytical methods (Helmstaedter and Mitra, 2012), will likely generalize more easily across taxa and offer powerful complementary approaches. With a rapidly expanding toolkit comprised of more traditional and modern techniques available to probe different nervous systems, incredible biological diversity available that has yet to be explored, and phylogenetic tools to interpret neural characteristics within a comparative framework, the coming years are set to be a particularly exciting time to forge new frontiers in our field.
CM and MH were the lead authors on this article, but all contributed significant feedback at various stages of writing.
The genesis of this manuscript was a workshop organized by the authors entitled “Comparative Principles of Brain Architecture and Function” (November 17th & 18th, 2016) that was co-sponsored by the Agency for Medical Research and Development (AMED) of Japan and the National Science Foundation (NSF 1647036) of the United States of America.
The authors declare that the research was conducted in the absence of any commercial or financial relationships that could be construed as a potential conflict of interest.
We thank all the attendees of this workshop for their contributions to the discussions that formed the basis of this manuscript, as well as Kuo-Fen Lee and Michael Tadross for insightful comments on an earlier version of the article. We also thank Abby Miller for creating the circular evolutionary tree shown in Figure 1.
Abe, K., Matsui, S., and Watanabe, D. (2015). Transgenic songbirds with suppressed or enhanced activity of CREB transcription factor. Proc. Natl. Acad. Sci. U S A 112, 7599–7604. doi: 10.1073/pnas.1413484112
Agrawal, A. A. (2001). Phenotypic plasticity in the interactions and evolution of species. Science 294, 321–326. doi: 10.1126/science.1060701
Ahrens, M. B., Li, J. M., Orger, M. B., Robson, D. N., Schier, A. F., Engert, F., et al. (2012). Brain-wide neuronal dynamics during motor adaptation in zebrafish. Nature 485, 471–477. doi: 10.1038/nature11057
Ahrens, M. B., Orger, M. B., Robson, D. N., Li, J. M., and Keller, P. J. (2013). Whole-brain functional imaging at cellular resolution using light-sheet microscopy. Nat. Methods 10, 413–420. doi: 10.1038/nmeth.2434
Aiello, B. R., Westneat, M. W., and Hale, M. E. (2017). Mechanossensation is evolutionarily tuned to locomotor mechanics. Proc. Natl. Acad. Sci. U S A 114, 4459–4464. doi: 10.1073/pnas.1616839114
Albertin, C. B., Simakov, O., Mitros, T., Wang, Z. Y., Pungor, J. R., Edsinger-Gonzales, E., et al. (2015). The octopus genome and the evolution of cephalopod neural and morphological novelties. Nature 524, 220–224. doi: 10.1038/nature14668
Alexander, R. D. (1974). The evolution of social behavior. Annu. Rev. Ecol. Syst. 5, 325–383. doi: 10.1146/annurev.es.05.110174.001545
Barton, R. A., Aggleton, J. P., and Grenyer, R. (2003). Evolutionary coherence of the mammalian amygdala. Proc. R. Soc. Lond. B Biol. Sci. 270, 539–543. doi: 10.1098/rspb.2002.2276
Ben Haim, L., and Rowitch, D. H. (2017). Functional diversity of astrocytes in neural circuit regulation. Nat. Rev. Neurosci. 18, 31–41. doi: 10.1038/nrn.2016.159
Bock, D. D., Lee, W.-C. A., Kerlin, A. M., Andermann, M. L., Hood, G., Wetzel, A. W., et al. (2011). Network anatomy and in vivo physiology of visual cortical neurons. Nature 471, 177–182. doi: 10.1038/nature09802
Bohland, J. W., Wu, C., Barbas, H., Bokil, H., Bota, M., Breiter, H. C., et al. (2009). A proposal for a coordinated effort for the determination of brainwide neuroanatomical connectivity in model organisms at a mesoscopic scale. PLoS Comput. Biol. 5:e1000334. doi: 10.1371/journal.pcbi.1000334
Bolker, J. (2012). There’s more to life than rats and flies. Nature 491, 31–33. doi: 10.1038/491031a
Bosch, T. C. G., Klimovich, A., Domazet-Lošo, T., Gründer, S., Holstein, T. W., Jékely, G., et al. (2017). Back to the basics: cnidarians start to fire. Trends Neurosci. 40, 92–105. doi: 10.1016/j.tins.2016.11.005
Box, G. E. P. (1979). “Robusteness in the strategy of scentific model building,” in Robustness in Statistics, eds R. L. Launer and G. N. Wilkinson (New York, NY: Academic Press), 201–236.
Boyden, E. S., Zhang, F., Bamberg, E., Nagel, G., and Deisseroth, K. (2005). Millisecond-timescale, genetically targeted optical control of neural activity. Nat. Neurosci. 8, 1263–1268. doi: 10.1038/nn1525
Brenowitz, E. A., and Zakon, H. H. (2015). Emerging from the bottleneck: benefits of the comparative approach to modern neuroscience. Trends Neurosci. 38, 273–278. doi: 10.1016/j.tins.2015.02.008
Calabrese, A., and Woolley, S. M. N. (2015). Coding principles of the canonical cortical microcircuit in the avian brain. Proc. Natl. Acad. Sci. U S A 112, 3517–3522. doi: 10.1073/pnas.1408545112
Carlson, B. A. (2012). Diversity matters: the importance of comparative studies and the potential for synergy between neuroscience and evolutionary biology. Arch. Neurol. 69, 987–993. doi: 10.1001/archneurol.2012.77
Catania, K. C., and Kaas, J. H. (1996). The unusual nose and brain of the star-nosed mole. Bioscience 46, 578–586. doi: 10.2307/1312987
Clark, D. A., Mitra, P. P., and Wang, S. S. (2001). Scalable architecuter in mammalian brains. Nature 411, 189–193. doi: 10.1038/35075564
DeCasien, A. R., Williams, S. A., and Higham, J. P. (2017). Primate brain size is predicted by diet but not sociality. Nat. Ecol. Evol. 1:0112. doi: 10.1038/s41559-017-0112
Deisseroth, K. (2015). Optogenetics: 10 years of microbial opsins in neuroscience. Nat. Neurosci. 18, 1213–1225. doi: 10.1038/nn.4091
Dimidschtein, J., Chen, Q., Temblay, R., Rogers, S. L., Saldi, G., Guo, L., et al. (2016). A viral strategy for targeting and manipulating interneurons across vertebrate species. Nat. Neurosci. 19, 1743–1749. doi: 10.1038/nn.4430
Douglas, R. H., Partridge, J. C., Dulai, K., Hunt, D., Mullineaux, C. W., Tauber, A. Y., et al. (1998). Dragon fish see using chlorophyll. Nature 393, 423–424. doi: 10.1038/30871
Dugas-Ford, J., Rowell, J. J., and Ragsdale, C. W. (2012). Cell-type homologies and the origins of the neocortex. Proc. Natl. Acad. Sci. U S A 109, 16974–16979. doi: 10.1073/pnas.1204773109
Dunbar, R. I. M., and Shultz, S. (2017). Why are there so many explanations for primate brain evolution? Philos. Trans. R. Soc. Lond. B Biol. Sci. 372:20160244. doi: 10.1098/rstb.2016.0244
Düzel, E., Bunzek, N., Guitard-Masip, M., Wittmann, B., Schott, B. H., and Tobler, P. N. (2009). Functional imaging of the human dopaminergic midbrain. Trends Neurosci. 32, 321–328. doi: 10.1016/j.tins.2009.02.005
Emlen, S. T., and Oring, L. W. (1977). Ecology, sexual selection, and the evolution of mating systems. Science 197, 215–223. doi: 10.1126/science.327542
Enard, W. (2014). Comparative genomics of brain size evolution. Front. Hum. Neurosci. 8:345. doi: 10.3389/fnhum.2014.00345
Felsenstein, J. (1985). Phylogenies and the comparative method. Am. Nat. 125, 1–15. doi: 10.1086/284325
Ferenczi, E. A., Vierock, J., Atsuta-Tsunoda, K., Tsunoda, S. P., Ramakrishnan, C., Gorini, C., et al. (2016). Optogenetic approaches addressing extracellular modulation of neural excitability. Sci. Rep. 6:23947. doi: 10.1038/srep23947
Fushiki, A., Zwart, M. F., Kohsaka, H., Fetter, R. D., Cardona, A., and Nose, A. (2016). A circuit mechanism for the propagation of waves of muscle contraction in Drosophila. Elife 5:e13253. doi: 10.7554/elife.13253
Glickfeld, L. L., Andermann, M. L., Bonin, V., and Reid, R. C. (2013). Cortico-cortical projections in mouse visual cortex are functionally target specific. Nat. Neurosci. 16, 219–226. doi: 10.1038/nn.3300
Glickfeld, L. L., and Olsen, S. R. (2017). Higer-order areas of the mouse visual cortex. Annu. Rev. Vis. Sci. 3, 251–273. doi: 10.1146/annurev-vision-102016-061331
Goldstein, B., and King, N. (2016). The future of cell biology: emerging model organisms. Trends Cell Biol. 26, 818–824. doi: 10.1016/j.tcb.2016.08.005
Gómez-Robles, A., Smaers, J. B., Holloway, R. L., Polly, P. D., and Wood, B. A. (2017). Brain enlargement and dental reduction were not linked in hominin evolution. Proc. Natl. Acad. Sci. U S A 114, 468–473. doi: 10.1073/pnas.1608798114
Gould, S. J., and Lewontin, R. C. (1979). The spandrels of San Marco and the Panglossian program: a critique of the adaptationist programme. Proc. R. Soc. Lond. B Biol. Sci. 205, 281–288. doi: 10.1098/rspb.1979.0086
Gross, K., Pasinelli, G., and Kunc, H. P. (2010). Behavioral plasticity allows short-term adjustment to a novel environment. Am. Nat. 176, 456–464. doi: 10.1086/655428
Grow, D. A., McCarrey, J. R., and Navara, C. S. (2016). Advantages of nonhuman primates as preclinical models for evaluating stem cell-based therapies for Parkinson’s disease. Stem Cell Res. 17, 352–366. doi: 10.1016/j.scr.2016.08.013
Guo, W., Clause, A. R., Barth-Maron, A., and Polley, D. B. (2017). A corticothalamic circuit for dynamic switching between feature detection and discrimination. Neuron 95, 180.e5–194.e5. doi: 10.1016/j.neuron.2017.05.019
Hale, M. E. (2014). Mapping circuits beyond the models: integrating connectomics and comparative neuroscience. Neuron 83, 1256–1258. doi: 10.1016/j.neuron.2014.08.032
Harris, R. A., Tardif, S. D., Vinar, T., Wildman, D. E., Rutherford, J. N., Rogers, J., et al. (2014). Evolutionary genetics and implications of small size and twinning in callitrichine primates. Proc. Natl. Acad. Sci. U S A 111, 1467–1472. doi: 10.1073/pnas.1316037111
Harrison, P. W., and Montgomery, S. H. (2017). Genetics of cerebellar and neocortical expansion in anthropoid primates: a comparative approach. Brain Behav. Evol. 89, 274–285. doi: 10.1159/000477432
Harvey, P. H., and Krebs, J. R. (1991). Comparing brains. Science 249, 140–146. doi: 10.1126/science.2196673
Harvey, P. H., and Pagel, M. D. (1991). The Comparative Method in Evolutionary Biology. Oxford, England: Oxford University Press.
Helmstaedter, M., and Mitra, P. P. (2012). Computational methods and challenges for large-scale circuit mapping. Curr. Opin. Neurobiol. 22, 162–169. doi: 10.1016/j.conb.2011.11.010
Hildebrand, D. G. C., Cocconet, M., Torres, R. M., Choi, W., Quan, T. M., Moon, J., et al. (2017). Whole-brain serial-section electron microscopy in larval zebrafish. Nature 545, 345–349. doi: 10.1038/nature22356
Hirasawa, T., and Kuratani, S. (2015). Evolution of vertebrate skeleton: morphology, embryology, and development. Zoological Lett. 1:2. doi: 10.1186/s40851-014-0007-7
Hyman, S. E. (2013). Revitalizing psychiatric therapeutics. Neuropsychopharmacology 39, 220–229. doi: 10.1038/npp.2013.181
Isler, K., and van Schaik, C. P. (2006). Metabolic costs of brain size evolution. Biol. Lett. 2, 557–560. doi: 10.1098/rsbl.2006.0538
Issa, J. B., Haeffele, B. D., Agarwal, A., Bergles, D. E., Young, E. D., and Yue, D. T. (2014). Multiscale optical Ca2+ imaging of tonal organization in mouse auditory cortex. Neuron 83, 944–959. doi: 10.1016/j.neuron.2014.07.009
Izpisua Belmonte, J., Callaway, E. M., Caddick, S. J., Churchland, P., Feng, G., Homatics, G. E., et al. (2015). Brains, genes, and primates. Neuron 86, 617–631. doi: 10.1016/j.neuron.2015.03.021
Jang, H., Levy, S., Flavell, S. W., Mende, F., Latham, R., Zimmer, M., et al. (2017). Dissection of neuronal gap junction circuits that regulate social beahvior in Caenorhabditis elegans. Proc. Natl. Acad. Sci. U S A 114, E1263–E1272. doi: 10.1073/pnas.1621274114
Jarvis, E., Güntürkün, O., Bruce, L., Csillag, A., Karten, H., Kuenzel, W., et al. (2005). Avian brains and a new understanding of vertebrate brain evolution. Nat. Rev. Neurosci. 6, 151–159. doi: 10.1038/nrn1606
Joel, D., and Weiner, J. (2000). The connections of the dopaminergic system with the striatum in rats and primates: an analysis with respect to the functional and compartmental oragnization of the striatum. Neuroscience 96, 451–474. doi: 10.1016/s0306-4522(99)00575-8
Kaas, J. H. (1986). “The organization and evolution of neocortex,” in Higher Brain Functions, ed. S. P. Wise (New York, NY: John Wiley and Sons), 347–378.
Kaas, J. H. (2013). The evolution of brains from early mammals to humans. Wiley Interdiscip. Rev. Cogn. Sci. 4, 33–45. doi: 10.1002/wcs.1206
Karten, H. J. (1991). Homology and the evolutionary origins of the ‘neocortex’. Brain Behav. Evol. 38, 264–272. doi: 10.1159/000114393
Karten, H. J. (2013). Neocortical evolution. Neural circuits arise independently of lamination. Curr. Biol. 23, R12–R15. doi: 10.1016/j.cub.2012.11.013
Katz, P. S. (2011). Neural mechanisms underlying the evolvability of behaviour. Philos. Trans. R. Soc. Lond. B Biol. Sci. 366, 2086–2099. doi: 10.1098/rstb.2010.0336
Katz, P. S. (2016). Phylogenetic plasticity in the evolution of molluscan neural circuits. Curr. Opin. Neurobiol. 41, 8–16. doi: 10.1016/j.conb.2016.07.004
Katz, P. S., and Hale, M. E. (2017). “Evolution of motor systems,” in Neurobiology of Motor Control, eds S. L. Hooper and A. Buschges (New York, NY: Wiley and Sons, Inc.), 135–176.
Kepecs, A., and Fishell, G. (2014). Interneuron cell types are a fit to function. Nature 505, 318–326. doi: 10.1038/nature12983
Kornfeld, J., Benezra, S. E., Narayanan, R. T., Svara, F., Egger, R., Oberlaender, M., et al. (2017). EM connectomics reveals axonal target variation in a sequence-generating network. Elife 6:e24364. doi: 10.7554/elife.24364
Kotrschal, K., Van Staaden, M. J., and Huber, R. (1998). Fish brains: evolution and anvironmental relationships. Rev. Fish Biol. Fish. 8, 373–408. doi: 10.1023/A:1008839605380
Krubitzer, L. A. (2000). How does evolution build a complex brain? Novartis Found. Symp. 228, 206–226. doi: 10.1002/0470846631.ch14
Krubitzer, L. A., and Kaas, J. H. (2005). The evolution of the neocortex in mammals: how is phenotypic diversity generated? Curr. Opin. Neurobiol. 15, 444–453. doi: 10.1016/j.conb.2005.07.003
Krubitzer, L. A., and Seelke, A. M. H. (2012). Cortical evolution in mammals: the bane and beauty of phenotypic variability. Proc. Natl. Acad. Sci. U S A 109, 106547–110654. doi: 10.1073/pnas.1201891109
Lamichhaney, S., Berglund, J., Almén, M. S., Maqbool, K., Grabherr, M., Martinez-Barrio, A., et al. (2015). Evolution of Darwin’s finches and their beaks revealed by genome sequencing. Nature 518, 371–375. doi: 10.1038/nature14181
Laubach, M., Amarante, L. M., Swanson, K., and White, S. R. (2018). What, if anything, is rodent prefrontal cortex? eneuro 5:ENEURO.0315–0318.2018. doi: 10.1523/ENEURO.0315-18.2018
Leclerc, C., Webb, S. E., Daguzan, C., Moreau, M., and Miller, A. L. (2000). Imaging patterns of calcium transients during neural induction in Xenopus laevis embryos. J. Cell Sci. 113, 3519–3529.
Liberti, W. A. III., Markowitz, J. E., Perkins, L. N., Liberti, D. C., Leman, D. P., Guitchounts, G., et al. (2016). Unstable neurons underlie a stable learned behavior. Nat. Neurosci. 19, 1665–1671. doi: 10.1038/nn.4405
Liebeskind, B. J., Hillis, D. M., Zakon, H. H., and Hoffmann, H. A. (2016). Complex homology and the evolution of nervous systems. Trends Ecol. Evol. 31, 127–135. doi: 10.1016/j.tree.2015.12.005
Lin, S., Lin, Y., Nery, J. R., Urich, M. A., Breschi, A., Davis, C. A., et al. (2014). Comparison of the transcriptional landscapes between human and mouse tissues. Proc. Natl. Acad. Sci. U S A 111, 17224–17229. doi: 10.1073/pnas.1413624111
Liu, K. S., and Fetcho, J. R. (1999). Laser ablations reveal functional relationships of segmental hindbrain neurons in zebrafish. Neuron 23, 325–335. doi: 10.1016/s0896-6273(00)80783-7
MacDougall, M., Nummela, S. U., Coop, S., Disney, A. A., Mitchell, J. F., and Miller, C. T. (2016). Optogenetic photostimulation of neural circuits in awake marmosets. J. Neurophysiol. 116, 1286–1294. doi: 10.1152/jn.00197.2016
Malsak, M. S., Haag, J., Ammer, G., Serbe, E., Meier, M., Leonardt, A., et al. (2013). A directional tuning map of Drosophila elementary motion detectors. Nature 500, 212–216. doi: 10.1038/nature12320
Manger, P. R., Cort, J., Ebrahim, N., Goodman, A., Henning, J., Karolia, M., et al. (2008). Is 21st century neuroscience too focussed on the rat/mouse model of brain function and dysfunction? Front. Neuroanat. 2:5. doi: 10.3389/neuro.05.005.2008
Mank, M., Santos, A. F., Direnberger, S., Mrsic-Flogel, T. D., Hofer, S. B., Stein, V., et al. (2008). A genetically encoded calcium indicator for chronic in vivo two-photon imaging. Nat. Methods 5, 805–811. doi: 10.1038/nmeth.1243
Marcus, G. F. (2018). Deep learning: a critical appraisal. http://www.arXiv.org
Markert, S. M., Britz, S., Proppert, S., Lang, M., Witvliet, D., Mulcahy, B., et al. (2016). Filling the gap: adding super-resolution to array tomography for correlated ultrastructural and molecular identification of electrical synapses at the C. elegans connectome. Neurophotonics 3:041802. doi: 10.1117/1.nph.3.4.041802
Meyrand, P., Simmers, J., and Moulins, M. (1994). Dynamic construction of a neural network from multiple pattern generators in the lobster stomatogastric nervous system. J. Neurosci. 14, 630–644. doi: 10.1523/JNEUROSCI.14-02-00630.1994
Miller, C. T., Freiwald, W., Leopold, D. A., Mitchell, J. F., Silva, A. C., and Wang, X. (2016). Marmosets: a neuroscientific model of human social behavior. Neuron 90, 219–233. doi: 10.1016/j.neuron.2016.03.018
Montgomery, S. H., Capellini, I., Venditti, C., Barton, R. A., and Mundy, N. I. (2011). Adaptive evolution of four microcephaly genes and the evolution of brain size in anthropoid primates. Mol. Biol Evol. 28, 625–638. doi: 10.1093/molbev/msq237
Montgomery, S. H., and Mundy, N. I. (2012a). Evolution of ASPM is associated with both increases and decreases in brain size in primates. Evolution 66, 927–932. doi: 10.1111/j.1558-5646.2011.01487.x
Montgomery, S. H., and Mundy, N. I. (2012b). Positive selection on NIN, a gene involved in neurogenesis and primate brain evolution. Genes Brain Behav. 11, 903–910. doi: 10.1111/j.1601-183X.2012.00844.x
Murray, A. M., Ryoo, H. L., Gurevich, E., and Joyce, J. N. (1994). Localization of D3 receptors to mesolimbic and D2 receptors to mesostriatal regions of human forebrain. Proc. Natl. Acad. Sci. U S A 91, 11271–11275. doi: 10.1073/pnas.91.23.11271
Nauman, E. A., Fitzgerald, J. E., Dunn, T. W., Rihel, J., Sompolinsky, H., and Engert, F. (2016). From whole-brain data to functional circuit models: the zebrafish optomotor response. Cell 167, 947.e20–960.e20. doi: 10.1016/j.cell.2016.10.019
Nern, A., Pfeiffer, B. D., and Rubin, G. M. (2015). Optimized tools for multicolor stochastic labeling reveal diverse sterotyped cell arrangements in the fly visual system. Proc. Natl. Acad. Sci. U S A 112, E2967–E2976. doi: 10.1073/pnas.1506763112
Ng, S. H., Shankar, S., Shikichi, Y., Akasaka, K., Mori, K., and Yew, J. Y. (2014). Pheromone evolution and sexual behavior in Drosophila are shaped by male sensory exploitation of other males. Proc. Natl. Acad. Sci. U S A 111, 3056’3061. doi: 10.1073/pnas.1313615111
Niu, Y., Shen, B., Cui, Y., Chen, Y., Wang, J., Wang, L., et al. (2014). Generation of gene modified cynomolgus monkey via Cas9/RNA-mediated gene targeting in one-cell embryos. Cell 156, 836–843. doi: 10.1016/j.cell.2014.01.027
O’Collins, V. E., Macleod, M. R., Donnan, G. A., Horky, L. L., van der Worp, B. H., and Howells, D. W. (2006). 1,026 experimental treatments in acute stroke. Ann. Neurol. 59, 467–477. doi: 10.1002/ana.20741
Odom, K. J., Hall, M. L., Riebel, K., Omland, K. E., and Langmore, N. E. (2014). Female song is widespread and ancestral in songbirds. Nat. Commun. 5:3379. doi: 10.1038/ncomms4379
Oh, S. W., Harris, J. A., Ng, L., Winslow, B., Cain, N., Mihalas, S., et al. (2014). A mesoscale connectome of the mouse brain. Nature 508, 207–214. doi: 10.1038/nature13186
Okano, H., and Kishi, N. (2018). Investigation of brain science and neurological/psychiatric disorders using genetically modified nonhuman primates. Curr. Opin. Neurobiol. 50, 1–6. doi: 10.1016/j.conb.2017.10.016
Okano, H., Sasaki, E., Yamamori, T., Iriki, A., Shimogori, T., Yamaguchi, Y., et al. (2016). Brain/MINDS: a japanese national brain project for marmoset neuroscience. Neuron 92, 582–590. doi: 10.1016/j.neuron.2016.10.018
Osten, P., and Margrie, T. W. (2013). Mapping brain circuitry with a light microscope. Nat. Methods 10, 515–523. doi: 10.1038/nmeth.2477
Park, J. E., Zhang, X. F., Choi, S., Okahara, J., Sasaki, E., and Silva, A. C. (2016). Generation of transgenic marmosets expressing genetically encoded calcium indicators. Sci. Rep. 6:34931. doi: 10.1038/srep34931
Perlman, R. L. (2016). Mouse models of human disease. Evol. Med. Public Health 2016, 170–176. doi: 10.1093/emph/eow014
Petryszyn, S., Beaulieu, J. M., Parent, A., and Parent, M. (2014). Distribution and morphological characteristics of striatal interneurons expressing calretinin in mice: a comparison with human and nonhuman primates. J. Chem. Neuroanat. 59, 51–61. doi: 10.1016/j.jchemneu.2014.06.002
Picardo, M. A., Merel, J., Katlowitz, K. A., Vallentin, D., Okobi, D. E., Benezra, S. E., et al. (2016). Population-level representation of temporal sequence underlying song production in the zebra finch. Neuron 90, 866–876. doi: 10.1016/j.neuron.2016.02.016
Preuss, T. M. (2007). “Evolutionary specializations of primate brain systems,” in PRIMATE ORIGINS: Adaptations and Evolution, eds M. J. Ravosa and M. Dagosto (Boston, MA: Springer US), 625–675.
Randel, N., Asadulina, A., Bezares-Calderón, L. A., Verasztó, C., Williams, E. A., Conzelmann, M., et al. (2014). Neuronal connectome of a sensory-motor circuit for visual navigation. Elife 3:e02730. doi: 10.7554/elife.02730
Real, E., Asari, H., Gollisch, T., and Meister, M. (2017). Neural circuit inference from function to struction. Curr. Biol. 27, 189–198. doi: 10.1016/j.cub.2016.11.040
Rodríguez, F., López, J. C., Vargas, J. P., Broglio, C., Gómez, Y., and Salas, C. (2002). Spatial memory and hippocampal pallium through vertebrate evolution: insights from reptiels and teleost fish. Brain Res. Bull. 57, 499–503. doi: 10.1016/s0361-9230(01)00682-7
Rosenthal, G. G., and Evans, C. S. (1998). Female preference for swords in Xiphophorus helleri reflects a bias for large apparent size. Proc. Natl. Acad. Sci. U S A 95, 4431–4436. doi: 10.1073/pnas.95.8.4431
Roth, G. (2015). Convergent evolution of complex brains and high intelligence. Philos. Trans. R. Soc. Lond. B Biol. Sci. 370:20150049. doi: 10.1098/rstb.2015.0049
Roy, A., Osik, J. J., Ritter, N. J., Wang, S., Shaw, J. T., Fiser, J., et al. (2016). Optogenetic spatial and temporal control of cortial circuits on a columnar scale. J. Neurophysiol. 115, 1043–1062. doi: 10.1152/jn.00960.2015
Ryan, M. J., Fox, J. H., Wilczynski, W., and Rand, A. S. (1990). Sexual selection for sensory exploitation in the frog Physalaemus pustulosus. Nature 343, 66–67. doi: 10.1038/343066a0
Sadakane, O., Masamizu, Y., Watakabe, A., Terada, S., Ohtsuka, M., Takaji, M., et al. (2015). Long-term Two-photon Calcium Imaging of neuronal populations with subcellular resolution in adult non-human primates. Cell Rep. 13, 1989–1999. doi: 10.1016/j.celrep.2015.10.050
Santisakultarm, T. P., Kresbergen, C. J., Bandy, D. K., Ide, D. C., Choi, S., and Silva, A. C. (2016). Two-photon imaging of cerebral hemodynamics and neural activity in awake and anesthetized marmosets. J. Neurosci. Methods 271, 55–64. doi: 10.1016/j.jneumeth.2016.07.003
Sasaki, E., Suemizu, H., Shimada, A., Hanazawa, K., Oiwa, R., Kamioka, M., et al. (2009). Generation of transgenic non-human primates with germline transmission. Nature 459, 523–527. doi: 10.1038/nature08090
Sato, K., Oiwa, R., Kumita, W., Henry, R., Sakuma, T., Ryoji, I., et al. (2016). Generation of a nonhuman primate model of severe combined immunodeficiency using highly efficient genome editing. Cell Stem Cell 19, 127–138. doi: 10.1016/j.stem.2016.06.003
Sena, E. S., van der Worp, B. H., Bath, P. M., Howells, D. W., and Macleod, M. R. (2006). Publication bias in reports of animal stroke leads to major overstatement of efficacy. PLoS Biol. 8:e1000344. doi: 10.1371/journal.pbio.1000344
Session, A. M., Uno, Y., Kwon, T., Chapman, J. A., Toyoda, A., Takahashi, S., et al. (2016). Genome evolution in the allotetraploid frog Xenopus laevis. Nature 538, 336–343. doi: 10.1038/nature19840
Shaw, K. (1995). Phylogenetic tests of the sensory exploitation model of sexual selection. Trends Ecol. Evol. Amst. 10, 117–120. doi: 10.1016/s0169-5347(00)89005-9
Shepherd, S. V. (2010). Following gaze: gaze-following behavior as a window into social cognition. Front. Integr. Neurosci. 4:5. doi: 10.3389/fnint.2010.00005
Shepherd, G. M. G. (2011). The microcircuit concept applied to cortical evolution: from three-layer to six-layer cortex. Front. Neuroanat. 5:30. doi: 10.3389/fnana.2011.00030
Shepherd, G. M. G., and Rowe, T. B. (2017). Neocortical lamination: insights from neuron types and evolutionary precursors. Front. Neuroanat. 11:100. doi: 10.3389/fnana.2017.00100
Shields, B. C., Kahupo, E., Kim, C., Apostolides, P. F., Brown, J., Lindo, S., et al. (2017). Deconstructing behavioral neuropharmacology with cellular specificity. Science 356:eaaj2161. doi: 10.1126/science.aaj2161
Silver, D., Schrittwieser, J., Simonyan, K., Antonoglou, I., Huang, A., Guez, A., et al. (2017). Mastering the game of Go without human knowledge. Nature 550, 354–359. doi: 10.1038/nature24270
Sparrow, D. B., Latinkic, B., and Mohun, T. J. (2000). A simplified method of generating transgenic Xenopus. Nucleic Acids Res. 28:E12. doi: 10.1093/nar/28.4.e12
Stosiek, C., Garaschuk, O., Holthoff, K., and Konnerth, A. (2003). In vivo two-photon calcium imaging of neuronal networks. Proc. Natl. Acad. Sci. U S A 100, 7319–7324. doi: 10.1073/pnas.1232232100
Strausfeld, N. J. (1995). Crustacean—insect relationships: the use of brain characters to derive phylogeny amongst segmented invertebrates. Brain Behav. Evol. 52, 186–206. doi: 10.1159/000006563
Strausfeld, N. J. (2009). Brain organization and the origin of insects: an assessment. Proc. R. Soc. Lond. B Biol. Sci. 276, 1929–1937. doi: 10.1098/rspb.2008.1471
Strausfeld, N. J. (2012). Arthropod Brains: Evolution, Functional Elegance and Historical Significance. Cambridge, MA: Harvard University Press.
Strausfeld, N. J., Hansen, L., Li, Y., Gomez, R. S., and Ito, K. (1998). Evolution, discovery, and interpretations of anthropod mushroom bodies. Learn. Mem. 5, 11–37.
Striedter, G., Belgard, T. G., Chen, C. C., Davis, F. P., Finlay, B. L., Güntürkün, O., et al. (2014). NSF workshop report: discovering general principles of nervous system organization by comparing brain maps across species. J. Comp. Neurol. 522, 1445–1453. doi: 10.1002/cne.23568
Takagi, C., Sakamaki, K., Morita, H., Hara, Y., Suzuki, M., Kinoshita, N., et al. (2013). Transgenic Xenopus laevis for live imaging in cell and developmental biology. Dev. Growth Differ. 55, 422–433. doi: 10.1111/dgd.12042
Takemura, S.-Y., Aso, Y., Hige, T., Wong, A., Lu, Z., Xu, C. S., et al. (2017a). A connectome of a learning and memory center in the adult Drosophila brain. Elife 6:e26975. doi: 10.7554/eLife.26975
Takemura, S.-Y., Nern, A., Chklovskii, D. B., Scheffer, L. K., Rubin, G. M., and Meinertzhagen, I. A. (2017b). The comprehensive connectome of a neural substrate for ‘ON’ motion detection in Drosophila. Elife 6:e24394. doi: 10.7554/elife.24394
Tu, Z., Yang, W., Yan, S., Guo, X., and Li, X. J. (2015). CRISPR/Cas9: a powerful genetic engineering tool for establishing large animal models of neurodegenerative diseases. Mol. Neurodegener. 10:35. doi: 10.1186/s13024-015-0031-x
Venkatachalam, V., Ji, N., Wang, X., Clark, C., Mitchell, J., Klein, M., et al. (2015). Pan-neuronal imaging in roaming Caenorhabditis elegans. Proc. Natl. Acad. Sci. U S A 113, E1082–E1088. doi: 10.1073/pnas.1507109113
Wamsley, B., and Fishell, G. (2017). Genetic and activity-dependent mechanisms underlying interneuron diversity. Nat. Rev. Neurosci. 18, 299–309. doi: 10.1038/nrn.2017.30
Wells, M. J. (1978). Octopus: Physiology and Behaviour of An Advanced Invertebrate. London: Chapman and Hall.
White, J. G., Southgate, E., Thomson, J., and Brenner, S. (1986). The structure of the nervous system of the nematode Caenorhabditis elegans. Philos. Trans. R. Soc. Lond. B Biol. Sci. 314, 1–340. doi: 10.1098/rstb.1986.0056
Wiliams, S. M., and Goldman-Rakic, P. S. (1998). Widespread origin of hte primate mesofrontal dopamine system. Cereb. Cortex 8, 321–345. doi: 10.1093/cercor/8.4.321
Yan, G., Vértes, P. E., Towlson, E. K., Chew, Y. L., Walker, D. S., Shafer, W. R., et al. (2017). Network control principles predict neuron function in the Caenorhabditis elegans. Nature 550, 519–523. doi: 10.1038/nature24056
Yartsev, M. M. (2017). The emperor’s new wardrobe: rebalancing diversity of animal models in neuroscience research. Science 358, 466–469. doi: 10.1126/science.aan8865
Keywords: phylogeny, neuroscience, molecular-genetics, evolution, homology (comparative) modeling, brain evolution
Citation: Miller CT, Hale ME, Okano H, Okabe S and Mitra P (2019) Comparative Principles for Next-Generation Neuroscience. Front. Behav. Neurosci. 13:12. doi: 10.3389/fnbeh.2019.00012
Received: 25 September 2018; Accepted: 15 January 2019;
Published: 05 February 2019.
Edited by:
Jimena Andersen, Stanford University, United StatesReviewed by:
Adhil Bhagwandin, University of Cape Town, South AfricaCopyright © 2019 Miller, Hale, Okano, Okabe and Mitra. This is an open-access article distributed under the terms of the Creative Commons Attribution License (CC BY). The use, distribution or reproduction in other forums is permitted, provided the original author(s) and the copyright owner(s) are credited and that the original publication in this journal is cited, in accordance with accepted academic practice. No use, distribution or reproduction is permitted which does not comply with these terms.
*Correspondence: Cory T. Miller, Y29yeW1pbGxlckB1Y3NkLmVkdQ==
† These authors have contributed equally to this work
Disclaimer: All claims expressed in this article are solely those of the authors and do not necessarily represent those of their affiliated organizations, or those of the publisher, the editors and the reviewers. Any product that may be evaluated in this article or claim that may be made by its manufacturer is not guaranteed or endorsed by the publisher.
Research integrity at Frontiers
Learn more about the work of our research integrity team to safeguard the quality of each article we publish.