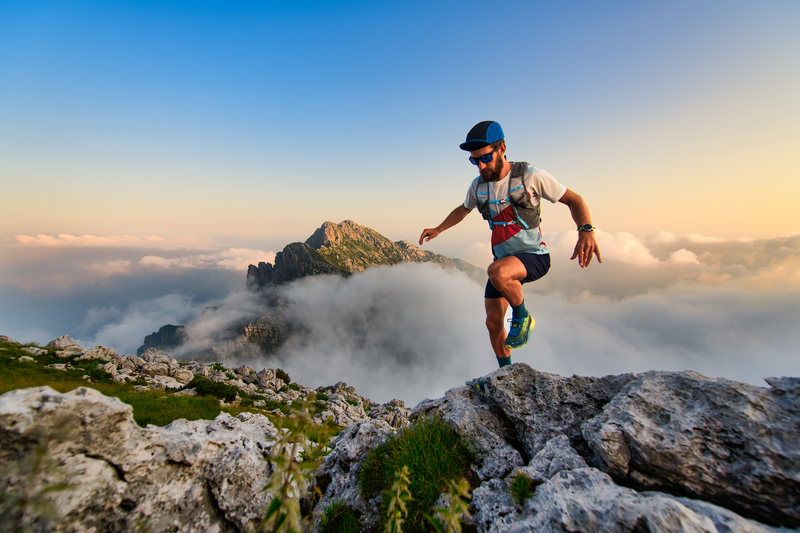
94% of researchers rate our articles as excellent or good
Learn more about the work of our research integrity team to safeguard the quality of each article we publish.
Find out more
EDITORIAL article
Front. Nanotechnol. , 13 June 2024
Sec. Nanodevices
Volume 6 - 2024 | https://doi.org/10.3389/fnano.2024.1431198
This article is part of the Research Topic Nanofluidics: Computational Methods and Applications View all 5 articles
Editorial on the Research Topic
Nanofluidics: computational methods and applications
This Research Topic from Frontiers in Nanotechnology, “Nanofluidics: Computational Methods and Applications”, presents computational, analytical, simulation and experimental techniques aimed at advancing our understanding of nanofluidic phenomena and paving the way for novel applications that can play a leading role in today’s technology. With a Research Topic of four studies, the reader will find i) an interesting paper presenting the novel use of single-layer MoS2 nanopores as sensors for the identification of biomarkers, ii) a seminal paper using various machine learning and deep learning methods to reproduce particle trajectories from molecular dynamics simulations taking into account physical laws, iii) a new paper investigating the mechanisms of heat transfer in a vertical double-passage channel with a permeable fluid and, iv) a significant study on the quantification of hydrophobicity in nanoparticle systems with environmental considerations.
The use of Molecular Dynamics (MD) simulations in science and engineering, and specifically in biological applications, dates back to the 1980s. In the paper presented by Hernandez et al. we have an excellent example of how computational tools and simulations at the nanoscale can lead to the development of novel devices like single-layer MoS2 sensors for the identification of biomarkers. This is the first computational study to explore the use of 2D solid-state nanopores in the identification of proteinogenic amino acids. Results from more than 40 different nano-engineered pores and 20 proteinogenic amino acids are presented and analysed in the study, leading to the direct identification of 16 of them. The combination of classical MD simulations and unsupervised machine learning models showed that the MoS2 nanopore sensors can differentiate positively and negatively charged amino acids from neutral ones. The major finding of this paper is that the methodology presented would allow the identification of proteins from their sequences through fingerprints specific to each amino acid, without the need to detect each amino acid of the polypeptide.
Artificial Intelligence and Machine Learning methods are widely used in science and engineering with multiple and innovative applications. Alexiadis focused on systems consisting of moving particles at the nanoscale and presented a set of criteria for evaluating the ability of artificial neural networks (ANNs) to replicate particle trajectories when the ANNs are trained based on MD data. A wide range of ANNs were tested, including feedforward neural networks, convolutional neural networks, recurrent neural networks, time convolutions, self-attention, graph neural networks, neural ordinary differential equations, and physics-informed machine learning. The author’s intention was to provide a method that would let us identify when to rely on the widely available off-the-shelf models and when to integrate them with physics-based models, beyond the single criterion of accuracy. In total 11 criteria are presented and justified with none of the ANNs studied being able to satisfy all of them individually and independently. A combination of methods within more complex networks may possibly lead to a system capable of satisfying all criteria, however this is beyond the scope of Alexiadis’ paper. His primary objective is to assess each network type independently and present their strengths and weaknesses, so that future studies can make informed decisions in the process of designing more advanced multilayer or multiarchitecture models based on his results and set of criteria.
The paper by Athal et al. investigated the impact of viscous dissipation on fully developed mixed convection in a vertical plate whose walls conduct heat to an external fluid. It is a fact that increasing convective heat transfer in a vertical channel is a major goal due to its practical importance in many engineering systems, such as the Research Topic of alternative energy and the cooling of electronic systems, micropumps, heat exchangers for electronic devices, gas chromatography analyzers, and other micro-electro-mechanical systems. Here, the Brinkman model was employed to extract velocity and temperature values in porous material flows. The impact of material factors such as the porosity parameter, σ, the mixed convection parameter, λ, and the channel length was investigated for its effect on the velocity and temperature distribution near the hot and cold walls. It was found that both properties increase as the viscous dissipation parameter for upward flow increases at each location.
Roncari et al. studied the hydrophobicity of silver nanoparticles (AgNPs), a critical Research Topic directly connected with their environmental behaviour and impact. Through a holistic experimental procedure and data analysis the authors show that sulfidation and adsorption of sulfidised NOM/“lipid-free” (LF-)NOM (organic matter), result in an increased hydrophobic character of AgNPs. The efficiency of the dark-field microscopy method, as a method used for the assessment of the interaction, was also demonstrated. The modelling of the particles as hard spheres interacting with an infinite flat surface, the consideration of the uniform character of the particle surface properties and the physicochemical characteristics of the dye molecules (size, interaction properties), revealed differences in the hydrophobic character. The main goal of the present study was to highlight the differences associated with the nature of the physical mechanisms underlying the interaction effects in NPs and to provide different ways for the design and assessment of their hydrophobic character.
FS: Writing–review and editing, Writing–original draft, Conceptualization. KR: Writing–review and editing, Writing–original draft. AA: Writing–review and editing, Writing–original draft.
We are grateful to all authors who contributed and created an interesting and high-quality Research Topic. We would also like to thank the reviewers who helped us with the process.
The authors declare that the research was conducted in the absence of any commercial or financial relationships that could be construed as a potential conflict of interest.
All claims expressed in this article are solely those of the authors and do not necessarily represent those of their affiliated organizations, or those of the publisher, the editors and the reviewers. Any product that may be evaluated in this article, or claim that may be made by its manufacturer, is not guaranteed or endorsed by the publisher.
Keywords: molecular dynamics, porous flow, physics-based machine learning, heat transfer, nanoparticles, hydrophobicity
Citation: Sofos F, Ritos K and Avramopoulos A (2024) Editorial: Nanofluidics: computational methods and applications. Front. Nanotechnol. 6:1431198. doi: 10.3389/fnano.2024.1431198
Received: 11 May 2024; Accepted: 30 May 2024;
Published: 13 June 2024.
Edited and reviewed by:
Themis Prodromakis, University of Edinburgh, United KingdomCopyright © 2024 Sofos, Ritos and Avramopoulos. This is an open-access article distributed under the terms of the Creative Commons Attribution License (CC BY). The use, distribution or reproduction in other forums is permitted, provided the original author(s) and the copyright owner(s) are credited and that the original publication in this journal is cited, in accordance with accepted academic practice. No use, distribution or reproduction is permitted which does not comply with these terms.
*Correspondence: Filippos Sofos, ZnNvZm9zQHV0aC5ncg==; Konstantinos Ritos, a29ucml0b3NAdXRoLmdy; Aggelos Avramopoulos, YWF2cmFtb3BvdWxvc0B1dGguZ3I=
†These authors have contributed equally to this work
Disclaimer: All claims expressed in this article are solely those of the authors and do not necessarily represent those of their affiliated organizations, or those of the publisher, the editors and the reviewers. Any product that may be evaluated in this article or claim that may be made by its manufacturer is not guaranteed or endorsed by the publisher.
Research integrity at Frontiers
Learn more about the work of our research integrity team to safeguard the quality of each article we publish.