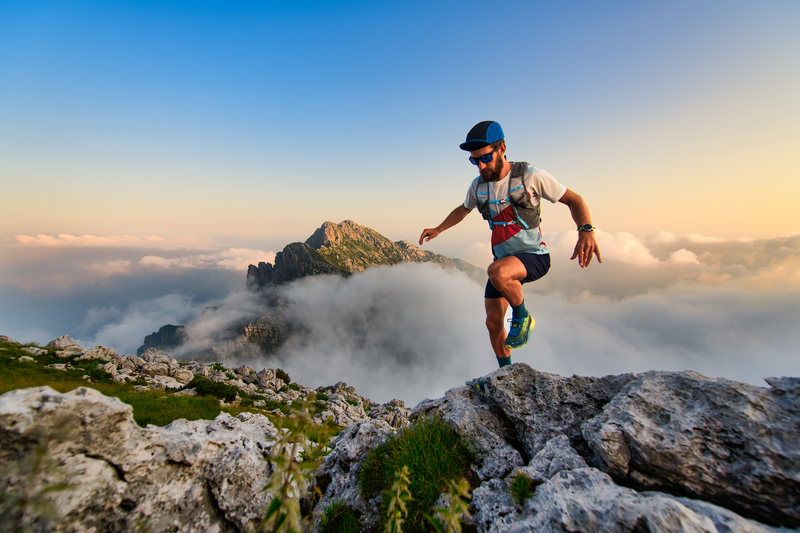
94% of researchers rate our articles as excellent or good
Learn more about the work of our research integrity team to safeguard the quality of each article we publish.
Find out more
ORIGINAL RESEARCH article
Front. Aging Neurosci. , 25 September 2024
Sec. Alzheimer's Disease and Related Dementias
Volume 16 - 2024 | https://doi.org/10.3389/fnagi.2024.1462302
Background: Advances in in vivo MRI techniques enable cerebral barrier transfer rates (Ktrans) measurement in patients with vascular cognitive impairment and dementia (VCID). However, a consensus has not been reached on the dynamic contribution and importance of cerebral barrier abnormalities to the differential diagnosis of dementia subtypes. Our goal was to investigate the dynamics of blood-brain barrier (BBB) and blood-CSF barrier (BCSFB) Ktrans in patients with VCID longitudinally and determine the effect of aging.
Methods: We studied subjects at two time points over two years; they were 65.5 years of age (SD = 15.94, M/F = 24/14) at the first visit. We studied 38 patients, 18 of whom had two visits. We calculated the BBB and BCSFB Ktrans with dynamic contrast-enhanced T1 MR, and we used 1H-MR spectroscopy to measure N-acetylaspartate (NAA) levels in the white matter as a marker of injury. In addition, we measured CSF levels of active-matrix metalloproteinase-3 (MMP3) as an inflammatory biomarker to aid in patient clustering.
Results: Longitudinal BBB measurements revealed variable dynamic behavior: after two years, the BBB Ktrans increased in 55% of patients and decreased in the remaining 45% unpredictably. We did not find a significant linear model of BBB Ktrans versus age for VCID. For healthy controls, the model was Ktrans = 0.0014 + 0.0002 × age, which was significant (p = 0.046). VCID patients showed a reduction in BCSFB Ktrans compared to healthy controls (p = 0.01). Combining NAA, CSF MMP3, and Ktrans in a clustering analysis separated patients into groups.
Conclusion: These results suggest that BBB Ktrans in VCID is dynamic and BCSFB Ktrans reduced by age. By combining inflammatory biomarkers with BBB Ktrans data, it is possible to separate VCID patients into distinct groups with different underlying pathologies.
The prevalence of dementia is increasing with aging populations worldwide. The most common form of dementia is Alzheimer’s disease (AD), but recent studies indicate a prominent role in dementia for vascular disease (Toledo et al., 2013; Schneider et al., 2007). Vascular cognitive impairment and dementia (VCID) is a heterogeneous group of cognitive disorders that share presumed cerebrovascular diseases. When both abnormal proteins and damaged blood vessels are present, an inflammatory response occurs that induces extracellular proteases that disrupt the blood-brain barrier (BBB) by attacking the basal lamina and loosening blood vessel tight junctions (Vercellino et al., 2008). Numerous cerebrovascular diseases affect the widely studied cerebral barriers, the BBB, and the blood-CSF barrier (BCSFB). In particular, BBB function alterations have been reported in both focal and diffuse abnormalities observed in the brain with inflammation, such as multiple sclerosis (Vos et al., 2005) and VCID (Taheri et al., 2011a). Although it is accepted that alterations in these barriers limit their protection against inflammatory mediators and escalate inflammation, the impact of injury to the BBB and CSFB is not fully understood. It is now possible to determine the extent of injury by assessing barrier dynamics, cerebrospinal fluid (CSF) circulation, and inflammation, thanks to advances in quantitative in vivo imaging and the ability to measure the impact of inflammation on barriers noninvasively.
Neurovascular dysfunction and vulnerability to cerebrovascular diseases are associated with arterial aging, as evidenced by increased arterial stiffness and reduced vascular reactivity (Farrall and Wardlaw, 2009). For example, the permeability of the BBB increases with age (Ford et al., 2022; Andjelkovic et al., 2023; Senatorov et al., 2019; Montagne et al., 2015), and CSF production and circulation decrease with age (Rubenstein, 1998; Chiu et al., 2012). In addition, aging may exacerbate BBB and BCSFB disruptions caused by pathological conditions (Ueno et al., 2016). In aging-related diseases such as VCID, increased BBB transfer rate (Ktrans) is believed to be a predictor of injury progression (Hussain et al., 2021).
Therefore, we can use Ktrans as a measure of barrier health and to categorize VCID patients according to the severity of Ktrans. In our studies, we showed that various biomarkers may differentially reflect VCID disease severity (Taheri et al., 2011b; Taheri et al., 2011a; Gasparovic et al., 2013; Candelario-Jalil et al., 2011). In this report, we hypothesized that a multimodal statistical classification method could differentiate VCID patients based on their most likely pathological contributors. An investigation of biomarkers collected at the University of New Mexico was also conducted to test this hypothesis. Various methods of statistically clustering subjects based on biomedical data have been used to classify patients (Jain et al., 1999). Model-based clustering is a technique for estimating group membership based on parametric mixture models (Fraley and Raftery, 2002; Browne and McNicholas, 2012). In the model-based clustering literature, the finite Gaussian mixture model is most commonly used (McNicholas and Murphy, 2010). We then used a multi-parametric mixture modeling approach (Bertoletti et al., 2015; Grun and Leisch, 2008) to blindly cluster VCID patients into related subgroups.
The purpose of this study was to determine the impact of aging on BBB Ktrans and BCSFB Ktrans in a group of VCID patients and compare with healthy controls. Our main hypothesis was that when cerebrovascular injury develops in VCID, the BBB Ktrans increases and the BCSFB Ktrans decreases. To test this hypothesis, we used DCE-MRI to quantify BBB Ktrans (Taheri et al., 2011b) and BCSFB Ktrans (Evans et al., 2020). In addition, we used N-acetylaspartate (NAA) to indicate white matter injury and MMP3 to indicate inflammation. Using the BBB Ktrans value with other biomarkers, we examined whether it is possible to differentiate patients with VCID according to the severity of the disease. As a contribution to the literature, this study highlights the dynamic nature of the BBB Ktrans in patients with VCID. Furthermore, given the dynamic changes in the BBB Ktrans, we propose the importance of a multimodal approach in VCID classification, using a combination of biomarkers.
Patients were recruited from the Center for Memory and Aging Clinic at the University of New Mexico Hospital, and from the Memory Clinic at the Albuquerque Veterans Administration Hospital. The experiments were strictly randomized and blinded. Statistical planning assumed an α-error of 5% and a β-error of 20%. Participants included in this analysis included 18 VCID patients and 15 healthy controls (HC). The HCs had an average age of 51 years (SD = 19, M/F = 10/7), whereas the patients had an average age of 65.5 years (SD = 15.94, M/F = 24/14). Among the 38 patients who participated in the study, we acquired complete data for three biomarkers: NAA compounds, mean BBB Ktrans, and active CSF MMP3. Of these patients, 18 were able to participate in a BBB Ktrans follow-up study. The average age of the follow-up patients enrolled in this study was 62 years (SD = 20.36, M/F = 12/6). The demographic data of patients and controls who participated in this study are summarized in Table 1.
Table 1. Clinical, demographic and neuropathological characteristics of patients and controls participated in the study.
Patients must meet all the following criteria for admission to this study: (1) signed, written informed consent, (2) male or nonpregnant, nonlactating female patients, and (3) had cognitive impairment and had ischemic vascular abnormalities on MRI. The questionnaire included patients with a clinical dementia rating (CDR) scale of 0, 0.5, or 1.0. Patients who met any of the following criteria were excluded from the study: (1) had a history of malignancy (except for basal cell skin carcinoma, for which the patient was eligible only if disease-free for 5 years or more), (2) had any disability acquired from trauma or another illness that, in the opinion of the investigator, could interfere with the evaluation of disability due to AD or mild cognitive impairment (MCI), (3) were unable to undergo MRI with gadolinium administration, (4) had untreated major depressive disorder (MDD), (5) had epileptic seizures that were not adequately controlled by treatment, and (6) had suicidal ideation.
The MRI data were acquired with a 1.5 Tesla Siemens Sonata scanner with a standard eight-channel array head coil (Siemens AG, Erlangen, Germany). As part of the MR protocol, we performed structural imaging, dynamic contrast-enhanced MRI (DCE-MRI) and 1H-MR magnetic resonance spectroscopy (1H-MRS). In a DCE-MRI procedure, a series of T1 map images were acquired before and after an optimized dose of Gadolinium diethylene triamine pentaacetic acid (Gd-DTPA, MW = 938 Da; Bayer Healthcare) was injected as a contrast agent (Taheri et al., 2016).
Data acquisition for calculating BBB transfer rates was based on a series of 8 T1 maps acquired with a fast T1 mapping sequence before and after the Gd-DTPA injection. One T1 map was acquired before Gd-DTPA injection, and the rest were acquired post-injection, resulting in a 2D time series dataset of contrast-enhanced MR images. The T1 mapping sequence used was TAPIR (T1-mapping with partial inversion recovery) (Shah et al., 2001; Neeb et al., 2006). Data were acquired with six 5-mm slices in the axial plane centered above the lateral ventricles and parallel to the anterior-posterior commissure axis (TR/TE/TI = 13 ms/2 ms/30 ms, flip angle = 25 degrees, FOV = 220 mm × 220 mm, slice thickness = 5.0 mm, slice gap = 5 mm, number of slices = 6, number of averages = 1, matrix size = 128 × 128, receiver bandwidth = 50 kHz). This parameter selection results in an in-plane resolution of 0.582 voxels/mm and a sampling interval of approximately three minutes. 1H-MRS was used to investigate the levels of both total creatine (Cr) and NAA in the white matter region above the lateral ventricles. 1H-MRS was performed with a phase-encoded version of a point resolved spectroscopy sequence (PRESS) with FOV = 220 mm × 220 mm and slice thickness = 15 mm. The nominal voxel size was 6.88 × 6.88 × 15 mm3. For more details on MRS data acquisition, see a previous paper (Gasparovic et al., 2013). We ensured that all follow-up imaging sessions were conducted in the same manner, including anatomical locations and MR sequence parameters.
The levels of active MMP3 in the CSF were measured with a fluorometric activity assay and were used to stratify patients according to patient clustering. A description of CSF MMP data collection and processing has been provided previously and will be briefly described (Candelario-Jalil et al., 2011). The activity of MMP-3 (stromelysin-1) in CSF and plasma was measured fluorometrically using a 5-FAM/QXL520 fluorescence resonance energy transfer peptide. With the intact fluorescence resonance energy transfer peptide (5-FAM-Arg-Pro-Lys-Pro-Val-Glu-Nva-Trp-Arg-Lys[QXL520]-NH2), the fluorescence of 5-FAM (5-carboxyfluorescein) is quenched by QXL520. Upon cleavage into 2 separate fragments by MMP3, the fluorescence of 5-FAM is recovered and can be monitored at excitation/emission wavelengths of 490/520 nm. This peptide has been shown to be cleaved by only MMP-3 and MMP-12 but not by other MMPs (Jain et al., 1999).
The details of the Ktrans calculations were described previously (Taheri et al., 2011a; Taheri et al., 2011b). In brief, Ktrans was calculated using Patlak compartmental analysis on dynamic contrast-enhanced T1 maps with Gd-DTPA as a contrast agent. Color-coded Ktrans maps were subsequently generated. The mean BBB Ktrans for WM was calculated from voxel-by-voxel data with a voxel size of 0.34 × 0.34 × 5 mm3 for six consecutive slices with a 5 mm thickness starting from the top of the brain. We used the same codes and software to process the follow-up data.
Three methods were used to interpret elevated BBB Ktrans at the voxel level: (1) mean elevated BBB Ktrans, which includes voxels with values higher than the average normal BBB Ktrans value in healthy control brain (Taheri et al., 2011b); (2) mean elevated BBB Ktrans voxel values in WM; and (3) total elevated BBB Ktrans in WM, which represents the sum of all voxel values in WM. Notably, the difference between the mean elevated BBB Ktrans and the total elevated BBB Ktrans represents the BBB Ktrans intensity.
To measure the choroid plexus (CP) transfer rate, a bolus of contrast agent (Gd-DTPA) was injected intravenously, followed by 25 min of sampling within a region of interest (ROI) placed in one of the lateral ventricles excluding the choroid plexus. A rectangular ROI of 10 × 20 voxels was placed within the ventricular area without covering any tissues. The concentration of Gd-DTPA ([Gd-DTPA]) in the ventricular area was determined using the average of the acquired time series of T1 images in ROI. For quantitative measurements of [Gd-DTPA], a quarter dose of 0.1 mmol/kg allowed us to use a linear approximation between T1 and [Gd-DTPA]. Based on the widely accepted constant-relaxivity relationship between T1 and [Gd-DTPA], [Gd-DTPA] was estimated in the ROI of the T1 map images of the ventricles using the constant-relaxivity relationship. 1/T1 = r1*[Gd-DTPA]. Where r1 is the relaxivity (mM–1s–1; which is reported to be 4.79 for 1.5T) and [Gd-DTPA] is in mmol. The reduction in the T1 value in the ventricular cavity reflects the rate at which [Gd-DTPA] increases in the ventricular cavity through the CP. As Gd-DTPA can easily pass through CP, it is suitable for measuring CP transfer rate. Therefore, we quantified the rate of CP transfer through the BCSFB by measuring the change in [Gd-DTPA]. Of note is that this method enables us to measure the transfer of substances whose molecular weight is lower than or equal to that of Gd-DTPA. The CP transfer rate was then calculated using the same procedure proposed for calculating the BBB Ktrans. In this study, we examined the CP transfer rate of 12 healthy controls and compared it to that of 12 patients with VCID.
Quantitative data was analyzed using the “R” platform (version 4.3.2, R Development Core Team, 2023). All the datasets were tested for normality using the Shapiro–Wilk test, and a subsequent unpaired t-test or Mann–Whitney test was applied based on the presence of a parametric or nonparametric distribution, respectively. We performed the Dixons Q test in R to exclude outlier in the analysis. Parametric statistical comparisons between the datasets were made based on the representation of the mean ± standard deviation (SD). Student’s t-test (for nonparametric data) was used for statistical analyses. Statistical analyses between groups were performed using two-way repeated measurements and analysis of variance (ANOVA) with Tukey’s post-hoc tests for multiple comparisons. A linear regression model (lm in R) was used to fit a line to the data for comparison. R2 was used as a goodness-of-fit. We considered a P-value < 0.05 as indicating statistical significance.
A multi-parametric model-based method was used to blindly classify VCID data using Ktrans, NAA, and active MMP3. A mixed modeling approach assumes data from different sources, and each source is modeled separately. We assumed that the mixture of VCID patients consisted of K clusters, with a specific distribution for each cluster. Patient v of VCID is assigned a probability Pvk to cluster k, where each cluster follows a parametric distribution.
The mixture distribution is given by the weighted sum of the K clusters. The n-dimensional vector x = (x1, …, xn)T contains the values of n variables measured for each of the VCID patients. The mixture density of variable x for patient j, xj is
Where φ is the vector of all unknown parameters of the model, fk is the specific density function of cluster k, and πk is the weight of the kth cluster that can be considered as a prior probability for an observation to come from this cluster. Model parameters were estimated by the expectation maximization (EM) algorithm, where the E-step was used to determine a-posteriori probabilities and the M-step was used to maximize the likelihood function.
This study was conducted using the flexible mixture analysis package (flexmix) (Grun and Leisch, 2008; Lo and Gottardo, 2012) in the R environment provided by the R Core Team (R Development Core Team, 2023). The number of clusters was optimized using the Bayesian information criterion (BIC) and the integrated completed likelihood (ICL). In mixture models, the ICL criteria are commonly used for clustering data by automatically selecting the number of clusters (Bertoletti et al., 2015; Vrieze, 2012). After selecting the number of clusters, we classified VCID patients into different clusters based on their entity values. Next, we provided estimated values for entities in each cluster. These values could be used to assign VCID patients to different clusters.
BBB damage in patients with VCID is dynamic. WM BBB transfer rates (Ktrans) of two patients with VCID are shown in Figure 1. FLAIR anatomical and corresponding WM BBB Ktrans maps of two consecutive axial slices of two patients with VCID acquired by anatomical and DCE-MRI two years apart are shown in this figure. In the left panel of this figure, mean WM BBB leakage increased as it is visible in the bottom row compared with the top row of Ktrans maps. For this patient, the hyperintensity area on the FLAIR image also increased. By contrast, the left panel depicts a patient with a decrease in mean WM Ktrans within two years. For this patient, the hyperintensity in FLAIR did not increase.
Figure 1. An illustration of BBB Ktrans dynamics in two patients for two years. Right panel shows a patient with increasing mean WM BBB Ktrans and the left panel shows a patient with decreasing mean WM BBB Ktrans within two years. In this figure, two consecutive axial slices of the brain of a patient with VCID acquired by structural- and DCE-MRI two years apart are depicted. In the upper rows of each panel, FLAIR anatomical images of the two slices are displayed. Each panel displays Ktrans maps overlaid on FLAIR anatomical images representing white matter in the lower rows. The S1 and S2 slices were matched between the two visits. A Ktrans map was created by color-coding each pixel’s Ktrans. The color bar on the right indicates Ktrans values in mL/(g-min). Based on FLAIR MR imaging, the white matter injury appears to be progressing in the left panel, whereas there is no significant change in the right panel. Within two years, the mean WM BBB damage for the patient depicted in the left panel has become more severe and extensive.
Longitudinal BBB Ktrans measurements do not reveal a pattern for VCID patients. Table 2 shows the longitudinal mean elevated BBB Ktrans for the sampled brain parenchyma of VCID patients at two consecutive times. There is no conclusive pattern in BBB Ktrans progression, as Ktrans increases in 55% of patients and decreases in the remaining 45% in an unpredictable manner. Table 2 shows the mean BBB Ktrans for patients with VCID white matter (WM) was calculated. Similar to Ktrans in the entire brain parenchyma, this figure illustrates that there is no clear pattern for Ktrans changes in WM. In half of the patients, the mean WM Ktrans increases, while in the other half, it decreases.
Supplementary Figure 1A illustrates the total elevated BBB Ktrans dynamics in WM for each patient. The total elevated BBB Ktrans is another measure that can be used to separate cases where there is a small area with high BBB Ktrans versus a larger area with small BBB Ktrans. Even though more than 50% of patients showed an increase in the WM BBB Ktrans, we were unable to identify a longitudinal pattern in the WM BBB Ktrans. We sampled the superior sagittal sinus area as the arterial input function (AIF). To test the rigor of the BBB Ktrans calculation, we compared the AIF at both visits. There was no statistically significant difference in the AIF of VCID patients between the first and second visit (Supplementary Figure 1B).
Older VCID patients had a greater average BBB Ktrans. We observed a greater slope for VCID patients than for control patients when we fit the age distribution of the average BBB Ktrans with linear regression. In Figure 2A, the age distributions of VCID patients and healthy control subjects are shown. The age distribution of these three groups did not differ significantly (NS) [p = 0.062, N controls = 15, N VCID at first time (VCt1) = 18, and N VCID at second time (VCt2) = 18]. Figure 2B represents a comparison of the average BBB Ktrans for VCID patients at two times and healthy controls in the whole brain parenchyma with the exclusion of the CSF compartment. There is a statistically significant difference between the mean BBB Ktrans of controls and VCt1 (p = 0.033). However, we did not find any statistically significant difference between mean BBB Ktrans of VCID at two times. Figure 2C shows the distribution of the mean BBB Ktrans in the whole brain parenchyma, excluding the CSF compartment in healthy controls and VCID patients as a function of age. To find a meaningful relationship between the age of patients and BBB Ktrans, we fitted a linear regression model to both VCID and control data. We did not find a statistically significant linear model of BBB Ktrans versus age for VCID (for both t1 and t2). For controls, the model was Ktrans = 0.0014 + 0.0002 × age, which was statistically significant (p = 0.046). This equation indicates a slight increase in BBB Ktrans with age in healthy controls.
Figure 2. Plots of BBB transfer rate (Ktrans) against age. Panel (A) compares the age distribution of VCID patients and healthy controls used in this study. The age distribution of these three groups did not differ significantly (NS) [p = 0.062, N controls (Ctrl) = 15, N VCID at first time (VCt1) = 18, N VCID at second time (VCt2) = 18]. Panel (B) represents a comparison of the average BBB Ktrans for VCID patients at two times and healthy controls in the whole brain parenchyma with the exclusion of the CSF compartment. There is a statistically significant difference between the mean BBB Ktrans of controls and VCt1 (p = 0.040). However, we did not find any statistically significant difference between mean BBB Ktrans of VCID at two times. Mean BBB Ktrans was calculated voxel-wise with a voxel size of 0.34 × 0.34 × 5 mm3 (sampled with six consecutive slices with a 5 mm thickness). N = 15 controls and 18 VCID subjects were used in this analysis. Panel (C) represents the distribution of mean BBB Ktrans in healthy controls and VCID subjects at two times, respectively, against age. A linear regression model was used to investigate the impact of age on these two groups’ BBB Ktrans. We did not find a statistically significant linear model of BBB Ktrans versus age for VCID. The model for controls was Ktrans = 0.0014 + 0.0002 × age which was statistically significant (p = 0.046).
The BCSFB transfer rate is reduced in VCID patients. We also compared the transfer of contrast agent from blood to CSF between VCID patients and healthy controls by measuring the transfer rate of Gd-DTPA into the lateral ventricles. In patients with VCID, the percentage of Gd-DTPA that passed through BCSFB into the ventricular area was significantly lower than in healthy controls. Furthermore, we observed that Gd-DTPA accumulation in the ventricles occurs at a slower rate than the AIF. After using compartmental modeling to calculate Ktrans, we observed that the average CP transfer rate in VCID patients was significantly lower than that in healthy controls (p-value = 0.0101; Figure 3A). Figure 3B shows the sampling area of a lateral ventricle. A sample [Gd-DTPA] time-activity curve (TAC) in the lateral ventricle of a patient with VCID is shown in Figure 3C. The ventricular TAC of the VCID group showed delayed and gradual accumulation of Gd-DTPA compared to that of the control TAC group. This figure also compares the VCID ventricular TAC with Gd-DTPA TAC of the superior sagittal sinus which is used as an arterial input function (AIF) to calculate Ktrans. The Gd-DTPA TAC shows wash-in and wash-out periods of Gd-DTPA.
Figure 3. Choroid plexus transfer rates in VCID patients are lower than those in healthy controls. The blood cerebrospinal fluid barrier (BCSFB) transfer rates were calculated for twelve healthy controls and twelve VCID age- and sex-matched subjects whose DCE-MRI data covered the ventricular spaces. Patlak compartmental modeling techniques were used to calculate the BCSFB transfer rate. (A) Average BCSFB transfer rate in VCID is significantly reduced when statistically compared with healthy controls, with a p-value of 0.0101. Panel (B) illustrates the sampling area in the lateral ventricular area for BCSFB transfer rate calculation. An illustration of Gd-DTPA concentration ([Gd-DTPA]) time activity curve (TAC) in the ventricular area can be found in Panel (C). This curve shows delayed leakage of Gd-DTPA into the ventricular area in a VCID patient in comparison to sampled arterial input function (AIF) that shows Gd-DTPA wash-in and wash-out periods.
A statistical classification of VCID patients based on the BBB Ktrans. A panel of correlated parameters, such as lesion volume and NAA concentration in WM, did not cluster VCID patients. However, the use of a panel of biomarkers from different sources enables us to cluster patients with VCID successfully. Figure 4 illustrates the use of cerebral metabolites (NAAs) to stratify patients in conjunction with a parameter derived from known CSF biomarkers of inflammation (MMP3) and an imaging biomarker (BBB Ktrans). In this figure, we can see that the BBB Ktrans value provides additional information on NAA and MMP3 levels. The figure illustrates the clustering power of a biomarker regarding the WM measurement area. In this study, the BBB Ktrans was calculated within the WM areas of the covered brain volume. To cluster patients, we compared a panel of BBB Ktrans values in WM with MMP3 to a panel of BBB Ktrans values in WM without MMP3. According to this figure, WM BBB Ktrans (including lesions areas), has greater clustering power if combined with other biomarkers.
Figure 4. The cohort of patients with VCID has been classified statistically into two groups. To determine the optimal number of clusters for patients, an information criterion is used as a first step. Panel (A) illustrates the Bayesian information criterion and the integrated completed likelihood (ICL) optimized for two clusters. An optimal ICL was used to determine the number of clusters. Panels (B–D) illustrate ellipse plots for Gaussian mixtures fitted by FLXMCmvnorm at 50 and 95% confidence levels. This model identified two clusters. A flexible mixture analysis was used to cluster patients based on three parameters: mean Ktrans in WM, NAA in WM, and active-MMP3. Flexible mixture analysis is a model-based mixture analysis method.
In Figure 4, the top left panel depicts the Bayesian information criterion as well as an integrated completed likelihood (ICL) optimized for two clusters. Using the ICL, we could identify two statistically distinct groups of patients (Figure 4A). We identified two clusters using this model. In the remaining panels, ellipse plots are shown for Gaussian mixtures fitted with FLXMCmvnorm at 50 and 95% confidence levels (Figures 4B–D).
The metrics for VCID clustering were determined using the estimated mean values of the selected biomarkers in each cluster. After using a statistical method to cluster VCID patients into two groups automatically, we compared the entity values between the two clusters. This provides a metric for assigning VCID patients to a proper cluster. Figure 5 shows the normalized values of the three biomarkers that we used for VCID patient clustering. As is clear, each cluster has different estimated values for these biomarkers. We could classify VCID patients into one of two clusters by estimating metrics for the selected biomarkers.
Figure 5. Estimated mean values of biomarkers within each cluster. The figure shows the normalized values of three biomarkers used for clustering VCIDs. As it is clear, each cluster has a different estimated value for these parameters. Based on these values, VCID patients could be assigned to one of these clusters. After using a statistical method to automatically cluster VCID patients into two groups, we compare biomarker values between the two clusters. VCID patients can be classified into two clusters using these metrics (which were estimated for selected biomarkers).
Our longitudinal study findings suggest that the in vivo quantified BBB transfer rate (Ktrans) is dynamic in patients with VCID. Even though we found that BBB Ktrans was greater in older patients with VCID, we could not find a linear relationship between BBB Ktrans and age. We could fit a linear model to the mean BBB Ktrans with age in healthy controls. This highlights the difference between an individual longitudinal study and a mean study of BBB Ktrans in VCID patients. On the other hand, BCSFB Ktrans was reduced in this group of patients. Along with other elements, such as active MMP3 and NAA, the BBB Ktrans contributes significantly to VCID classification. A model-based clustering method was used to cluster VCID patients into two clusters. The severity of the underlying pathology in VCID patients can be effectively stratified by using a combination of biomarkers, including the BBB Ktrans.
Several cerebral inflammatory diseases affect the BBB and BCSFB tight junctions (Abbott, 2000; Saul et al., 2020). BBB Ktrans alterations have been reported in both focal and diffuse abnormalities in cerebral diseases, such as MS (Vos et al., 2005) and VCID (Taheri et al., 2011a). It has been shown that BCSFB disruption occurs in a variety of cerebral conditions, including AD (Brkic et al., 2015) and amyotrophic lateral sclerosis (ALS) (Saul et al., 2020). However, to the best of our knowledge, there are no reports of BCSFB impairment in VCID patients. Our data revealed a statistically significant reduction in BCSFB Ktrans in VCID patients compared with healthy controls. It is not clear what causes BCSFB Ktrans reduction in VCID. Mechanisms that impact tight junctions, or thickening of the choroid plexus walls with age, may, however, be related to the reduction in BCSFB Ktrans in VCID (Ott et al., 2018; Kant et al., 2018).
As a result of cerebrospinal fluid (CSF) recycling between the subarachnoid space, the brain, and the ventricles, ISF convection is promoted, benefiting both trophic and excretory functions. Fluid clearance occurs across capillaries facilitated by astrocytic endfeet containing aquaporin 4 (AQP-4) and the arachnoid villi are likely to complement CSF reabsorption via multiple pathways (olfactory and spinal arachnoid bulk flow) when CSF pressure and fluid retention are markedly elevated (Johanson et al., 2008). CSF–ISF homeostasis regulates water and solute fluxes at the blood–CSF and blood–brain interfaces. An impaired BBB adversely affects the interstitial environment of neurons (Obermeier et al., 2013). The average CSF turnover rate is between four and five times per day (Telano and Baker, 2023). There is a marked reduction in this rate in AD due to an increase in ventricular space (Ott et al., 2010). CSF turnover decreases with age, this leads to reduced solute clearance, including Aβ (Erickson and Banks, 2013). Interestingly, researchers have demonstrated that senile plague-bearing transgenic mice show a significant impairment in water influx into CSF, similar to the observations made in AQP-4 knockout mice (Igarashi et al., 2014).
However, the role of the BCSFB in determining CSF turnover through the ventricular space has not been fully explored. In this study, we analyzed the data for impairment in movement across the choroid plexus. We used the contrast agent transfer rate into the lateral ventricles as the transfer rate of the CP. Comparing VCID CP transfer rates with those of healthy controls; we observed that in VCID patients contrast agent transfer via the CP was reduced (Figure 2). Both age and pathological conditions change the extracellular matrix components that modulate the extracellular space (ECS) microenvironment and change ECS diffusion (Sykova and Nicholson, 2008; Han et al., 2014; Postnikov et al., 2022; Tonnesen et al., 2023). The hyperintensity acute reperfusion injury marker (HARM), defined as Gd-DTPA enhancement of CSF on fluid-attenuated inversion recovery (FLAIR), was observed in patients with elevated MMP9 (Barr et al., 2010).
The choroid plexus has a unique structure with fenestrated blood vessels and tight junctions located between the ependymal cells forming the surface of the choroid plexus. Removing the choroid plexus reduces CSF production, but continued formation is because of production by cerebral blood vessels. Possibly, our findings can be attributed to the aging process, resulting in a decrease in CSF circulation (Solar et al., 2020).
The BBB functions as a heterogeneous and dynamic barrier (Villabona-Rueda et al., 2019; O’Keeffe et al., 2020), playing an essential role in fluid homeostasis and waste clearance (Sagare et al., 2012; Sengillo et al., 2013). By longitudinally investigating the degree of BBB impairment, this study shows the dynamic nature of the BBB transfer rate in VCID pathology. This observation has potential implications regarding planning treatment strategies based on the brain barriers’ health for patients with cognitive impairment. However, in vivo evidence does not fully support the idea that normal aging disrupts the BBB, since current imaging techniques cannot detect subtle BBB changes. The imaging techniques described in the literature are mainly suitable for pathological BBB disruptions.
Considering recent advances in quantitative in vivo imaging techniques and the ability to measure inflammation of cerebral barriers noninvasively, quantitative information is now available regarding the focal and distributed effects of diseases on these barriers. Despite this, our study does not answer the following two questions regarding the relationship between barrier impairment and cognitive decline: Does cognitive impairment result from focal impairments of the BBB and BCSFB? And, how does damage to the cerebral barriers affect the onset and course of cognitive impairment?
In this study, we examined both the WM area and the whole brain parenchyma with the exclusion of the CSF compartment for the BBB Ktrans. Notably, the change in BBB Ktrans in lesion areas may differ because the nature of the lesion may evolve over 2 years. The nature of WM lesion evolution over time is still debatable. Although the Wardlaw group described WM lesions as highly dynamic (Wardlaw et al., 2015), recent studies by Sun et al. (2022) have shown that WMLs are relatively stable over 1–2 years. In future work, separating the BBB Ktrans in WMLs from that in normal-appearing WM (NAWM) would be more informative. There was no pattern to explain the changes in BBB Ktrans in either direction. Natural fluctuations in permeability could explain the changes in BBB Ktrans in either direction, similar to what we observe in MS patients’ brains. The variability of the BBB in our patients limits the usefulness of measurements of BBB permeability in clinical trials of therapeutic agents, since the natural history of BBB changes is too variable to determine the impact of treatment. This is unfortunate since BBB opening is important in the pathophysiology of both AD and VCID.
The results of clustering analysis reveal that using multiple biomarkers provides greater accuracy compared to using one biomarker. Combining the inflammatory biomarker, MMP3, with NAA and Ktrans provided a complete separation of VCID patients, confirming the importance of a multimodal approach for patient classification. A broad spectrum of biomarkers has been identified that correlate with BBB breakdown such as MMP3 (Candelario-Jalil et al., 2011; Brkic et al., 2015), MMP9 (Barr et al., 2010), PDGF-β (soluble platelet-derived growth factor receptor β) (Payne et al., 2023), VCAM1 (vascular cell adhesion molecule-1) (Haarmann et al., 2015), and ICAM1 (intercellular adhesion molecule 1) (Dietrich, 2002). In this study, we examined MMP3 and MMP9 for their power in VCID classification. We found that MMP3 has greater power for this cohort of VCID classification. Researchers broadly neglect the study of the role of PDGF-β, VCAM1, and ICAM1 in VCID classification, which merits attention.
Employing statistically optimized panels of biomarkers with different origins may help stratify VCID patients into more specific, cohesive groups for individualized treatment plans. Incorporating multiple biomarkers, such as imaging biomarkers, facilitates a more precise diagnosis of the dominant pathogenesis, also provides the opportunity to explore an optimal combination of biomarkers for VCID classification. Despite the increasing use of advanced in vivo imaging biomarkers for VCID diagnosis and classification, we need to make additional efforts to develop a gold standard panel of biomarkers that includes imaging biomarkers. For example, consider genotypes associated with cognitive impairment. According to recent studies, the APOE4 mutation has been associated with BBB structural impairment and altered hippocampal BBB Ktrans (Halliday et al., 2016; Moon et al., 2021). There is also evidence that BBB breakdown contributes to cognitive decline associated with APOE4 (Montagne et al., 2020).
There is a potential limitation in measuring the true passage rate of CSF through the CP. There is a possibility that Gd-DTPA accumulation in the ventricles is not solely related to CP function. Nevertheless, since the cerebral fluid system under investigation is a closed system, we anticipate that multiple flow data, including data on flow through the aqueduct of Sylvius, will provide a reliable biomarker of CP flow. However, in the current comparative study, any change in CP leakage has informative value. Another limitation of the study is the complexity of the experimental methods, involving MR spectroscopy, complex biochemistry, and Ktrans measurements, which will restrict clinical application. However, diffusion tensor imaging can replace spectroscopy, ultrasensitive fluid measurement instruments can detect inflammation, and cerebrospinal fluid albumin index can measure BBB permeability.
Several studies have demonstrated regional differences in Ktrans (Ha et al., 2021; Ivanidze et al., 2019). Regional analysis of Ktrans dynamics would provide more details on regional cerebral barriers’ vulnerability/resilience in VCID. Studying Ktrans dynamics in anatomical regions relevant to memory and cognition, such as the hippocampus (Montagne et al., 2015; Moon et al., 2021) is of immense value as a continuation of this study.
Longitudinal in vivo studies could significantly increase our knowledge of the pathogenesis variability of VCID classes as well as their evolution. The BBB Ktrans is dynamic in VCID and inflammatory biomarkers with BBB Ktrans can be used to separate VCID into distinct groups that suggest different underlying pathologies. The BCSFB Ktrans showed a decrease in this group of patients with VCID. This highlights the involvement of both CNS barriers in VCID.
The datasets presented in this study can be found in online repositories. The names of the repository/repositories and accession number(s) can be found in this article/Supplementary material.
The studies involving humans were approved by the University of New Mexico Human Research Review Committee. The studies were conducted in accordance with the local legislation and institutional requirements. The participants provided their written informed consent to participate in this study.
ST: Writing – review & editing, Writing – original draft, Software, Methodology, Conceptualization. JP: Writing – review & editing, Methodology, Investigation, Data curation. GR: Writing – review & editing, Writing – original draft, Validation, Supervision, Funding acquisition, Conceptualization.
The authors declare that financial support was received for the research, authorship, and/or publication of this article. The authors gratefully acknowledge financial support from the Alzheimer’s Association (NIRG-12-242467) and the Florida Department of Health (20A18). This study was supported by funding from the NIH (R01NS052305) to GR.
The authors declare that the research was conducted in the absence of any commercial or financial relationships that could be construed as a potential conflict of interest.
All claims expressed in this article are solely those of the authors and do not necessarily represent those of their affiliated organizations, or those of the publisher, the editors and the reviewers. Any product that may be evaluated in this article, or claim that may be made by its manufacturer, is not guaranteed or endorsed by the publisher.
The Supplementary Material for this article can be found online at: https://www.frontiersin.org/articles/10.3389/fnagi.2024.1462302/full#supplementary-material
Supplementary Figure 1 | (A) The total elevated WM BBB Ktrans [in mL/(g-min)] was calculated by summing up the voxel values above the Ktrans threshold in WM. This approach highlights the extend of BBB damage in WM. The same pattern that was observed for the mean BBB Ktrans in WM is visible here. In this figure, the length of the arrow corresponds to the amount of change in BBB Ktrans, and the blue arrow head indicates the direction in which BBB Ktrans has changed. The subject with no change in BBB Ktrans is shown only by the arrow head without the length of the arrow. (B) As part of the BBB Ktrans calculation process, an arterial input function (AIF) needed to represent Gd-DTPA concentration ([Gd-DTPA]) in plasma. We used superior sagittal sinus as AIF. This plot shows T1 value that represents [Gd-DTPA] as samples from superior sagittal sinus (SSS) in the first and second rounds of DCE-MRI, respectively. We did not find any statistically significant differences in the AIFs. This finding supports the rigor of data acquisition for DCE-MRI over a two-year period.
AD, Alzheimer’s diseases; AIF, arterial input function; ALS, amyotrophic lateral sclerosis; ANOVA, analysis of variance; AQP-4, aquaporin 4; BBB, blood-brain barrier; BCSFB, blood-CSF barrier; BIC, Bayesian information criterion; CDR, clinical dementia rating; CNS, central nervous system; CSF, cerebrospinal fluid; CP, choroid plexus; Cr, creatine; DCE-MRI, dynamic contrast-enhanced MRI; ECS, extracellular space; EM, expectation maximization; FLAIR, fluid-attenuated inversion recovery; HARM, hyperintensity acute reperfusion injury marker; HC, healthy controls; Gd-DTPA, gadolinium-DTPA; ICL, integrated completed likelihood; MDD, major depressive disorder; MMP-3, matrix metalloproteinases-3; MRI, magnetic resonance imaging; MS, multiple sclerosis; NAA, N-acetylaspartate; NAWM, normal appearing white matter; PRESS, point resolved spectroscopy sequence; ROI, region of interest; SD, standard deviation; TAC, time-activity curve; TAPIR, T1-mapping with partial inversion recovery; VCID, vascular cognitive impairment and dementia; WM, white matter.
Abbott, N. (2000). Inflammatory mediators and modulation of blood-brain barrier permeability. Cell. Mol. Neurobiol. 20, 131–147.
Andjelkovic, A., Situ, M., Citalan-Madrid, A., Stamatovic, S., Xiang, J., and Keep, R. (2023). Blood-brain barrier dysfunction in normal aging and neurodegeneration: Mechanisms, impact, and treatments. Stroke 54, 661–672. doi: 10.1161/STROKEAHA.122.040578
Barr, T., Latour, L., Lee, K., Schaewe, T., Luby, M., Chang, G., et al. (2010). Blood-brain barrier disruption in humans is independently associated with increased matrix metalloproteinase-9. Stroke 41, e123–e128.
Bertoletti, M., Friel, N., and Rastelli, R. (2015). Choosing the number of clusters in a finite mixture model using an exact integrated completed likelihood criterion. Metron 73, 177–199.
Brkic, M., Balusu, S., Van Wonterghem, E., Gorle, N., Benilova, I., Kremer, A., et al. (2015). Amyloid beta oligomers disrupt blood-CSF barrier integrity by activating matrix metalloproteinases. J. Neurosci. 35, 12766–12778. doi: 10.1523/JNEUROSCI.0006-15.2015
Browne, R., and McNicholas, P. (2012). Model-based clustering, classification, and discriminant analysis of data with mixed type. J. Stat. Plan Infer. 142, 2976–2984.
Candelario-Jalil, E., Thompson, J., Taheri, S., Grossetete, M., Adair, J., Edmonds, E., et al. (2011). Matrix metalloproteinases are associated with increased blood-brain barrier opening in vascular cognitive impairment. Stroke 42, 1345–1350.
Chiu, C., Miller, M., Caralopoulos, I., Worden, M., Brinker, T., Gordon, Z., et al. (2012). Temporal course of cerebrospinal fluid dynamics and amyloid accumulation in the aging rat brain from three to thirty months. Fluids Barriers CNS 9:3. doi: 10.1186/2045-8118-9-3
Dietrich, J. (2002). The adhesion molecule ICAM-1 and its regulation in relation with the blood-brain barrier. J. Neuroimmunol. 128, 58–68.
Erickson, M., and Banks, W. (2013). Blood-brain barrier dysfunction as a cause and consequence of Alzheimer’s disease. J. Cereb. Blood Flow Metab. 33, 1500–1513.
Evans, P., Sokolska, M., Alves, A., Harrison, I., Ohene, Y., Nahavandi, P., et al. (2020). Non-Invasive MRI of Blood-Cerebrospinal Fluid Barrier Function. Nat. Commun. 11:2081.
Farrall, A., and Wardlaw, J. (2009). Blood-brain barrier: Ageing and microvascular disease–systematic review and meta-analysis. Neurobiol. Aging 30, 337–352. doi: 10.1016/j.neurobiolaging.2007.07.015
Ford, J., Zhang, Q., Sweeney, E., Merkler, A., de Leon, M., Gupta, A., et al. (2022). Quantitative water permeability mapping of blood-brain-barrier dysfunction in aging. Front. Aging Neurosci. 14:867452. doi: 10.3389/fnagi.2022.867452
Fraley, C., and Raftery, A. (2002). Model-based clustering, discriminant analysis, and density estimation. J. Am. Stat. Assoc. 97, 611–631.
Gasparovic, C., Prestopnik, J., Thompson, J., Taheri, S., Huisa, B., Schrader, R., et al. (2013). 1H-MR spectroscopy metabolite levels correlate with executive function in vascular cognitive impairment. J. Neurol. Neurosurg. Psychiatry 84, 715–721. doi: 10.1136/jnnp-2012-303878
Grun, B., and Leisch, F. (2008). FlexMix Version 2: Finite mixtures with concomitant variables and varying and constant parameters. J. Stat. Softw. 28, 1–35.
Ha, I., Lim, C., Kim, Y., Moon, Y., Han, S., and Moon, W. (2021). Regional differences in blood-brain barrier permeability in cognitively normal elderly subjects: A dynamic contrast-enhanced MRI-based study. Korean J. Radiol. 22, 1152–1162. doi: 10.3348/kjr.2020.0816
Haarmann, A., Nowak, E., Deiss, A., van der Pol, S., Monoranu, C., Kooij, G., et al. (2015). Soluble VCAM-1 impairs human brain endothelial barrier integrity via integrin alpha-4-transduced outside-in signalling. Acta Neuropathol. 129, 639–652. doi: 10.1007/s00401-015-1417-0
Halliday, M., Rege, S., Ma, Q., Zhao, Z., Miller, C., Winkler, E., et al. (2016). Accelerated pericyte degeneration and blood-brain barrier breakdown in apolipoprotein E4 carriers with Alzheimer’s disease. J. Cereb. Blood Flow Metab. 36, 216–227.
Han, H., Shi, C., Fu, Y., Zuo, L., Lee, K., He, Q., et al. (2014). A novel MRI tracer-based method for measuring water diffusion in the extracellular space of the rat brain. IEEE J. Biomed. Health Inform 18, 978–983. doi: 10.1109/JBHI.2014.2308279
Hussain, B., Fang, C., and Chang, J. (2021). Blood-brain barrier breakdown: An emerging biomarker of cognitive impairment in normal aging and dementia. Front. Neurosci. 15:688090. doi: 10.3389/fnins.2021.688090
Igarashi, H., Suzuki, Y., Kwee, I., and Nakada, T. (2014). Water influx into cerebrospinal fluid is significantly reduced in senile plaque bearing transgenic mice, supporting beta-amyloid clearance hypothesis of Alzheimer’s disease. Neurol. Res. 36, 1094–1098. doi: 10.1179/1743132814Y.0000000434
Ivanidze, J., Mackay, M., Hoang, A., Chi, J., Cheng, K., Aranow, C., et al. (2019). Dynamic contrast-enhanced MRI reveals unique blood-brain barrier permeability characteristics in the hippocampus in the normal brain. AJNR Am. J. Neuroradiol. 40, 408–411. doi: 10.3174/ajnr.A5962
Jain, A., Murty, M., and Flynn, P. (1999). Data clustering: A review. ACM Comput. Surv. 31, 264–323.
Johanson, C., Duncan, J. III, Klinge, P., Brinker, T., Stopa, E., and Silverberg, G. (2008). Multiplicity of cerebrospinal fluid functions: New challenges in health and disease. Cerebrospinal Fluid Res. 5:10. doi: 10.1186/1743-8454-5-10
Kant, S., Stopa, E., Johanson, C., Baird, A., and Silverberg, G. (2018). Choroid plexus genes for CSF production and brain homeostasis are altered in Alzheimer’s disease. Fluids Barriers CNS 15:34.
Lo, K., and Gottardo, R. (2012). Flexible mixture modeling via the multivariate t distribution with the Box-Cox transformation: An alternative to the skew-t distribution. Stat. Comput. 22, 33–52. doi: 10.1007/s11222-010-9204-1
McNicholas, P., and Murphy, T. (2010). Model-based clustering of microarray expression data via latent Gaussian mixture models. Bioinformatics 26, 2705–2712. doi: 10.1093/bioinformatics/btq498
Montagne, A., Barnes, S., Sweeney, M., Halliday, M., Sagare, A., Zhao, Z., et al. (2015). Blood-brain barrier breakdown in the aging human hippocampus. Neuron 85, 296–302.
Montagne, A., Nation, D., Sagare, A., Barisano, G., Sweeney, M., Chakhoyan, A., et al. (2020). APOE4 leads to blood-brain barrier dysfunction predicting cognitive decline. Nature 581, 71–76.
Moon, W., Lim, C., Ha, I., Kim, Y., Moon, Y., Kim, H., et al. (2021). Hippocampal blood-brain barrier permeability is related to the APOE4 mutation status of elderly individuals without dementia. J. Cereb. Blood Flow Metab. 41, 1351–1361. doi: 10.1177/0271678X20952012
Neeb, H., Zilles, K., and Shah, N. J. (2006). A new method for fast quantitative mapping of absolute water content in vivo. Neuroimage 31, 1156–1168.
Obermeier, B., Daneman, R., and Ransohoff, R. (2013). Development, maintenance and disruption of the blood-brain barrier. Nat. Med. 19, 1584–1596.
O’Keeffe, E., Kelly, E., Liu, Y., Giordano, C., Wallace, E., Hynes, M., et al. (2020). Dynamic blood-brain barrier regulation in mild traumatic brain injury. J. Neurotrauma 37, 347–3562.
Ott, B., Cohen, R., Gongvatana, A., Okonkwo, O., Johanson, C., Stopa, E., et al. (2010). Brain ventricular volume and cerebrospinal fluid biomarkers of Alzheimer’s disease. J. Alzheimers Dis. 20, 647–657.
Ott, B., Jones, R., Daiello, L., de la Monte, S. M., Stopa, E. G., Johanson, C. E., et al. (2018). Blood-cerebrospinal fluid barrier gradients in mild cognitive impairment and Alzheimer’s disease: Relationship to inflammatory cytokines and chemokines. Front. Aging Neurosci. 10:245. doi: 10.3389/fnagi.2018.00245
Payne, L., Abdelazim, H., Hoque, M., Barnes, A., Mironovova, Z., Willi, C., et al. (2023). A soluble platelet-derived growth factor receptor-beta originates via pre-mRNA splicing in the healthy brain and is upregulated during hypoxia and aging. Biomolecules 13:3. doi: 10.3390/biom13040711
Postnikov, E., Lavrova, A., and Postnov, D. (2022). Transport in the brain extracellular space: Diffusion, but which kind? Int. J. Mol. Sci. 23:12401.
R Development Core Team (2023). R: A language and environment for statistical computing. Vienna: R Foundation for statistical computing.
Rubenstein, E. (1998). Relationship of senescence of cerebrospinal fluid circulatory system to dementias of the aged. Lancet 351, 283–285.
Sagare, A., Bell, R., and Zlokovic, B. (2012). Neurovascular dysfunction and faulty amyloid beta-peptide clearance in Alzheimer disease. Cold Spring Harb. Perspect. Med. 2:3475405.
Saul, J., Hutchins, E., Reiman, R., Saul, M., Ostrow, L., Harris, B., et al. (2020). Global alterations to the choroid plexus blood-CSF barrier in amyotrophic lateral sclerosis. Acta Neuropathol. Commun. 8:92. doi: 10.1186/s40478-020-00968-9
Schneider, J., Arvanitakis, Z., Bang, W., and Bennett, D. (2007). Mixed brain pathologies account for most dementia cases in community-dwelling older persons. Neurology 69, 2197–2204.
Senatorov, V. Jr., Friedman, A., Milikovsky, D., Ofer, J., Saar-Ashkenazy, R., Charbash, A., et al. (2019). Blood-brain barrier dysfunction in aging induces hyperactivation of TGFbeta signaling and chronic yet reversible neural dysfunction. Sci. Transl. Med. 11:eaaw8283. doi: 10.1126/scitranslmed.aaw8283
Sengillo, J., Winkler, E., Walker, C., Sullivan, J., Johnson, M., and Zlokovic, B. (2013). Deficiency in mural vascular cells coincides with blood-brain barrier disruption in Alzheimer’s disease. Brain Pathol. 23, 303–103628957. doi: 10.1111/bpa.12004
Shah, N., Zaitsev, M., Steinhoff, S., and Zilles, K. (2001). A new method for fast multislice T(1) mapping. Neuroimage 14, 1175–1185.
Solar, P., Zamani, A., Kubickova, L., Dubovy, P., and Joukal, M. (2020). Choroid plexus and the blood-cerebrospinal fluid barrier in disease. Fluids Barriers CNS 17:35.
Sun, Y., Hu, Y., Qiu, Y., Zhang, Y., Jiang, C., Lu, P., et al. (2022). Characterization of white matter over 1-2 years in small vessel disease using MR-based quantitative susceptibility mapping and free-water mapping. Front. Aging Neurosci. 14:998051. doi: 10.3389/fnagi.2022.998051
Sykova, E., and Nicholson, C. (2008). Diffusion in brain extracellular space. Physiol. Rev. 88, 1277–1340.
Taheri, S., Gasparovic, C., Huisa, B., Adair, J., Edmonds, E., Prestopnik, J., et al. (2011a). Blood-brain barrier permeability abnormalities in vascular cognitive impairment. Stroke 42, 2158–2163.
Taheri, S., Gasparovic, C., Shah, N., and Rosenberg, G. (2011b). Quantitative measurement of blood-brain barrier permeability in human using dynamic contrast-enhanced MRI with fast T1 mapping. Magn. Reson. Med. 65, 1036–1042. doi: 10.1002/mrm.22686
Taheri, S., Shah, N., and Rosenberg, G. (2016). Analysis of pharmacokinetics of Gd-DTPA for dynamic contrast-enhanced magnetic resonance imaging. Magn. Reson. Imaging 34, 1034–1040.
Telano, L., and Baker, S. (2023). Physiology, cerebral spinal fluid. Disclosure: Stephen Baker declares no relevant financial relationships with ineligible companies. Treasure Island, FL: StatPearls.
Toledo, J., Arnold, S., Raible, K., Brettschneider, J., Xie, S., Grossman, M., et al. (2013). Contribution of cerebrovascular disease in autopsy confirmed neurodegenerative disease cases in the National Alzheimer’s Coordinating Centre. Brain 136, 2697–2706. doi: 10.1093/brain/awt188
Tonnesen, J., Hrabetova, S., and Soria, F. (2023). Local diffusion in the extracellular space of the brain. Neurobiol. Dis. 177:105981.
Ueno, M., Chiba, Y., Murakami, R., Matsumoto, K., Kawauchi, M., and Fujihara, R. (2016). Blood-brain barrier and blood-cerebrospinal fluid barrier in normal and pathological conditions. Brain Tumor Pathol. 33, 89–96.
Vercellino, M., Votta, B., Condello, C., Piacentino, C., Romagnolo, A., Merola, A., et al. (2008). Involvement of the choroid plexus in multiple sclerosis autoimmune inflammation: A neuropathological study. J. Neuroimmunol. 199, 133–141. doi: 10.1016/j.jneuroim.2008.04.035
Villabona-Rueda, A., Erice, C., Pardo, C., and Stins, M. (2019). The evolving concept of the blood brain barrier (BBB): From a single static barrier to a heterogeneous and dynamic relay center. Front. Cell. Neurosci. 13:405. doi: 10.3389/fncel.2019.00405
Vos, C., Geurts, J., Montagne, L., van Haastert, E., Bo, L., van der Valk, P., et al. (2005). Blood-brain barrier alterations in both focal and diffuse abnormalities on postmortem MRI in multiple sclerosis. Neurobiol. Dis. 20, 953–960. doi: 10.1016/j.nbd.2005.06.012
Vrieze, S. (2012). Model selection and psychological theory: A discussion of the differences between the Akaike information criterion (AIC) and the Bayesian information criterion (BIC). Psychol. Methods 17, 228–243. doi: 10.1037/a0027127
Keywords: blood-brain barrier, vascular cognitive impairment and dementia (VCID), dynamic contrast-enhanced MRI (DCE-MRI), blood-cerebrospinal fluid barrier, MR spectroscopy, inflammation, cluster analysis
Citation: Taheri S, Prestopnik J and Rosenberg GA (2024) Barriers of the CNS transfer rate dynamics in patients with vascular cognitive impairment and dementia. Front. Aging Neurosci. 16:1462302. doi: 10.3389/fnagi.2024.1462302
Received: 09 July 2024; Accepted: 04 September 2024;
Published: 25 September 2024.
Edited by:
Zhen Zhao, University of Southern California, United StatesReviewed by:
Axel Montagne, University of Edinburgh, United KingdomCopyright © 2024 Taheri, Prestopnik and Rosenberg. This is an open-access article distributed under the terms of the Creative Commons Attribution License (CC BY). The use, distribution or reproduction in other forums is permitted, provided the original author(s) and the copyright owner(s) are credited and that the original publication in this journal is cited, in accordance with accepted academic practice. No use, distribution or reproduction is permitted which does not comply with these terms.
*Correspondence: Saeid Taheri, taheris@health.usf.edu
Disclaimer: All claims expressed in this article are solely those of the authors and do not necessarily represent those of their affiliated organizations, or those of the publisher, the editors and the reviewers. Any product that may be evaluated in this article or claim that may be made by its manufacturer is not guaranteed or endorsed by the publisher.
Research integrity at Frontiers
Learn more about the work of our research integrity team to safeguard the quality of each article we publish.