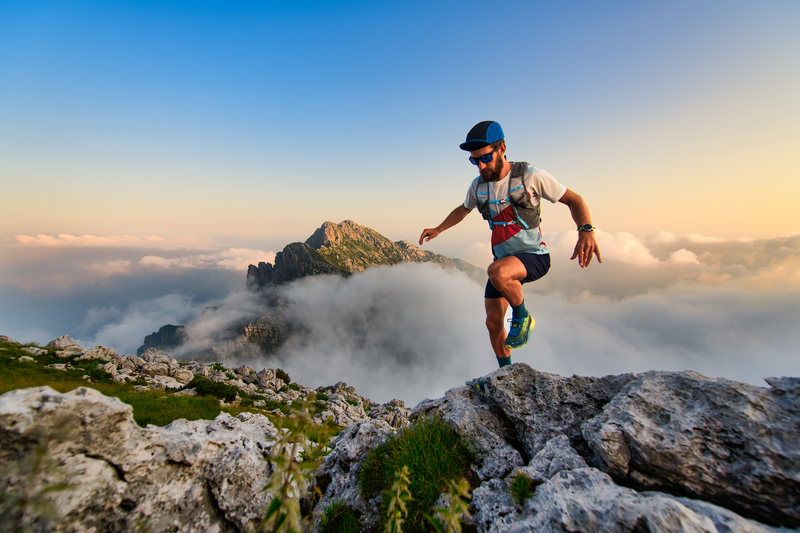
95% of researchers rate our articles as excellent or good
Learn more about the work of our research integrity team to safeguard the quality of each article we publish.
Find out more
ORIGINAL RESEARCH article
Front. Aging Neurosci. , 08 November 2024
Sec. Neurocognitive Aging and Behavior
Volume 16 - 2024 | https://doi.org/10.3389/fnagi.2024.1451968
Introduction: Numerous studies have reported alterations in the composition of gut microbiota in patients with acute ischemic stroke (AIS), with changes becoming more pronounced as the disease progresses. However, the association between the progression of transient ischemic attack (TIA) and AIS remains unclear. This study aims to elucidate the microbial differences among TIA, AIS, and healthy controls (HC) while exploring the associations between disease progression and gut microbiota.
Methods: Fecal samples were collected from acute TIA patients (n = 28), AIS patients (n = 235), and healthy controls (n = 75) and analyzed using 16 s rRNA gene sequencing. We determined characteristic microbiota through linear discriminant analysis effect size and used the receiver operating characteristic (ROC) curve to assess their predictive value as diagnostic biomarkers.
Results: Our results showed significant gut microbial differences among the TIA, AIS, and HC groups. Patients with AIS exhibited higher abundances of Lactobacillus and Streptococcus, along with lower abundances of Butyricicoccaceae and Lachnospiraceae_UCG-004. Further analysis revealed that the abundance of characteristic bacteria, such as Lactobacillus and Streptococcus, was negatively correlated with HDL levels, while Lactobacillus was positively correlated with risk factors such as homocysteine (Hcy). In contrast, the abundance of Lachnospiraceae_UCG-004 was negatively correlated with both Hcy and D-dimer levels. ROC models based on the characteristic bacteria Streptococcus and Lactobacillus effectively distinguished TIA from AIS, yielding areas under the curve of 0.699 and 0.626, respectively.
Conclusion: We identified distinct changes in gut bacteria associated with the progression from TIA to AIS and highlighted specific characteristic bacteria as predictive biomarkers. Overall, our findings may promote the development of microbiome-oriented diagnostic methods for the early detection of AIS.
Acute ischemic stroke (AIS) is a leading cause of death and long-term disability worldwide, driven by complex and multifaceted etiological factors, including metabolic disorders, alterations in gut microbiota, and systemic inflammation. Although these traditional risk factors are crucial, they do not fully account for the variability in AIS occurrence and outcomes. Notably, up to 15–30% of strokes are preceded by transient ischemic attack (TIA) (Rothwell et al., 2006; Rothwell and Warlow, 2005), which is characterized by a series of temporary neurological symptoms and serves as a strong predictor of subsequent stroke. The 90-day stroke risk after a TIA may be as high as 10–20%, with approximately half of these occurring within 2 days of the index event (Johnston et al., 2007; Hill et al., 2004). TIA, characterized by its suddenness, transience, and reversibility, is widely regarded as a high-risk factor for stroke and represents the optimal time window for prevention and intervention.
Due to the lack of precisely predictive biomarkers, a considerable proportion of TIA patients miss the critical window for preventive measures due to the lack of precise predictive biomarkers. Currently, the diagnosis of TIA primarily relies on medical history and imaging examinations. However, the insufficient sensitivity of imaging techniques and the potential for inaccurate recollection of symptoms by TIA patients create challenges for clinical diagnosis (Lima Filho et al., 2016; Amarenco et al., 2012). Therefore, there is an urgent need to explore novel biomarkers that can effectively screen high-risk TIA patients who may experience a stroke and evaluate disease progression from TIA to AIS.
Emerging evidence has begun to reveal the intricate relationship between altered gut microbiota and AIS, highlighting their significant potential to impact both AIS risk and recovery. Recent studies have shown that fecal microbiota transfer (FMT) from healthy microbiota can confer neuroprotective effects after a stroke (Singh et al., 2016; Lee et al., 2020). Additionally, it has been confirmed that stroke can induce dysbiosis in the gut microbiota and compromise epithelial barrier integrity (Peh et al., 2022; Pluta et al., 2021), leading to an exaggerated immune response that contributes to brain injury.
Furthermore, changes in gut microbiota have been widely associated with various brain diseases, especially in patients with stroke, where significant alterations in gut microbiota composition have been observed. Clinical studies have reported a notable decrease in the abundance of Bacteroidetes in individuals with AIS (Plovier et al., 2017). In addition, expansion of Enterobacteriaceae in the gut has been identified in both clinical and animal studies (Xu et al., 2021).
Furthermore, the dysbiosis of gut microbiota can, in turn, affect stroke outcomes, and the depletion of gut microbiota by antibiotic pretreatment exacerbates the prognosis of stroke (Winek et al., 2016; Honarpisheh et al., 2022). In addition, changes in the gut microbiota profile were associated with the diagnosis of AIS patients. Our previous study established a diagnostic model and identified potential microbial biomarkers for AIS patients with H-type hypertension (Yu et al., 2023). Moreover, we revealed the gut microbiota in patients with post-stroke depression (PSD) was characterized by increased genus Streptococcus, Akkermansia, and Barnesiella, which were diagnostic microbial biomarkers of PSD (Yao et al., 2023). Our previous study showed that the abundance of pro-inflammatory bacterial genera, such as Streptococcus, Veillonella, and Acidaminococcus, was increased in lacunar cerebral infarction patients (Ma et al., 2024). Emerging evidence has shown that the abnormal gut microbiota may be a cause or result of disease, suggesting that gut microbiota might provide biomarkers for detecting the risk or progression of AIS. However, previous studies merely focused on the link between the microbiota and TIA or AIS, which remains unexplored as a novel microbiome signature for early detection as a signal for the progression of TIA to AIS.
In this study, we aimed to characterize the alterations in gut microbiota among patients with TIA and AIS patients and further explored the associations between the characteristic microbiota and disease progression. These findings may encourage the development of microbiome-oriented diagnostics for predicting AIS.
This study recruited 235 AIS patients, 28 TIA patients, and 75 healthy controls (HC) who had not experienced cerebrovascular events. The participants were recruited from the Second Hospital of Wenzhou Medical University in China from September 2020 to May 2024. The inclusion criteria were as follows: (1) admission within 72 h after AIS; (2) participants aged ≥18 years; (3) diagnosis of large-artery atherosclerosis confirmed by carotid artery ultrasound or transcranial Doppler sonography (TCD).
The exclusion criteria were as follows: (1) patients who had taken antibiotics or prebiotics that could disturb the gut microbiota within the past 3 months; (2) severe comorbid conditions (e.g., severe gastrointestinal disease, malignancy, respiratory failure, severe liver dysfunction, or renal failure), gastrointestinal surgery, or pregnancy; (3) clear causes of stroke or TIA unrelated to atherosclerosis (e.g., cervical artery dissection, cardiogenic cerebral infarction, perivascular procedural stroke, or other TOAST subtypes); (4) special dietary habits, such as vegetarianism.
AIS and TIA patients met the diagnostic criteria set by the American Heart Association/American Stroke Association (Sacco et al., 2013; Easton et al., 2009). All control participants underwent TCD, color Doppler flow imaging, and echocardiography to assess their cardiovascular health. The National Institutes of Health Stroke Scale (NIHSS) was used to assess the degree of neurological impairment (Zavaglia et al., 2015; Wu et al., 2015). This study was approved by the Medical Ethics Committee of the Second Affiliated Hospital of Wenzhou Medical University, and informed consent was obtained from all participants.
The information on demographics (e.g., age and gender) was collected by trained researchers. Comorbidities like diabetes and hyperlipidemia (HL) were diagnosed by professionals of endocrinology. To determine lifestyle behaviors, we also collected participants’ histories of smoking or drinking. Moreover, laboratory indexes involved fasting glucose (FBG), glycosylated hemoglobin (HbAlc), homocysteine (Hcy), triglycerides (TG), high-density lipoprotein cholesterol (HDL), and D-dimer were measured and recorded.
Each fecal sample (200 mg) was obtained from patients during inpatient or outpatient visits. Control group members provided stool samples voluntarily at the health screening center.
To ensure the quality of fecal samples, we used 2-mL sterile centrifuge tubes, froze them immediately in liquid nitrogen after collection, and stored them at −80°C. DNA was extracted from collected samples by the E.Z.N.A.® Soil DNA Kit (Omega Bio-Tek, Norcross, GA, United States). For further detection, NanoDrop2000 (Thermo Scientific, Wilmington, United States) was used to determine the DNA concentration and purity. Then, the high mutation region of 16S ribosomal RNA (rRNA) was amplified. According to the manufacturer’s protocol, PCR products were recovered using 2% agarose gel, sequenced on the Illumina MiSeq platform (Illumina, San Diego, United States), and spliced using FLASH software.
Alpha diversity was quantified using the Ace and Shannon indices based on the Wilcoxon rank sum test. To further evaluate the overall ecology of the microbiome, β-diversity was calculated and visualized using a principal coordinates analysis (PCoA) diagram. The microbiota health index (MDI) was utilized to evaluate the degree of microbial dysbiosis, while microbiota composition was presented through bar charts at the phylum, family, and genus levels. In addition, linear discriminant analysis (LDA) effect size (LEfSe) was conducted to identify significant species capable of differentiating between groups, with a threshold LDA score set at >2. The correlation between the genus selected by LEfSe and clinical variables was visualized using a heatmap based on the Spearman correlation coefficient.
SPSS 26.0 software (SPSS, Chicago, United States) was used for the statistical analyses, and GraphPad Prism V.9.0.0 (La Jolla, CA, United States) was used for graph creation. PCoA diagram, MDI, and LDA analyses were carried out using R.1 Continuous variables follow a standard normal distribution (as inspected by the Kolmogorov–Smirnov test and the Shapiro–Wilk test) and were expressed by mean ± standard deviation (SD). As for the non-normally continuous variables, we used the non-parametric Mann–Whitney U-test or the Kruskal-Wallis test with Dunn’s correction for multiple comparisons to assess the significance of the differences among groups and expressed the result by the median and quartiles. Chi-square analysis assessed the significance of the differences between the groups for the nominal variables. Categorical variables were reported as the number of cases and percentages (%). A p-value of <0.05 was considered statistically significant.
Eighteen participants were excluded due to the exclusion criteria, while nine individuals dropped out for failing to provide fecal samples or follow-up. The enrolled TIA patients had a median age of 65 years (Q57.5, 73.5) and were 50% female (14 out of 28). The demographic and laboratory data of the recruited participants are shown in Table 1.
The α-diversity indices (Shannon index and Simpson index) did not significantly differ between HC and TIA groups or between HC and AIS (p > 0.05) (Figures 1A,B). However, α-diversity between TIA and AIS groups showed significant differences that suggested the discrepancy in microbial composition (p < 0.05) (Figures 1A,B). The PCoA diagram on the ASV level analyzed the potential principal components affecting the differences in community composition and reflected an obvious separation trend in three groups (p < 0.05) (Figure 1C). The comparison of the MDI exhibited that the HC, TIA, and AIS groups had microbial dysbiosis (Figure 1D). In addition, MDI showed an increasing trend from TIA to AIS groups compared with HC.
Figure 1. Microbial diversity analysis among HC, TIA, and AIS groups. (A,B) Alpha-diversity analysis among three groups, comparing Shannon and Simpson indices (Wilcoxon rank-sum test). (C) PCoA diagram on the ASV level analyzed the potential principal components affecting the differences in community composition. (D) Comparison of the Microbial dysbiosis index (MDI) among three groups. *p < 0.05, ***p < 0.001.
At the phylum level (Figure 2A), the microbiota detected in this study predominantly belong to four phyla: Firmicutes, Proteobacteria, Bacteroidota, and Actinobacteriota. Notably, Proteobacteria exhibited a higher abundance in the TIA group compared to the other groups. According to Figure 2B, at the family level, the taxa Lachnospiraceae, Enterobacteriaceae, Ruminococcaceae, Bacteroidaceae, Streptococcaceae, Lactobacillaceae, Bifidobacteriaceae, and Prevotellaceae collectively comprise approximately 70% of the total microbial composition across the three groups (Figure 2B). In the TIA group, Enterobacteriaceae showed a greater abundance relative to other groups, while Ruminococcaceae had a lower representation.
Figure 2. Microbial composition based on relative abundance among three groups. Circos diagram at the phylum level (A), bar diagram at the family level (B), and the genus level (C). Different colors and heights indicate the abundance ratios of different bacterial groups.
Moreover, the characterization at the genus level was more intricate; 19 genera, including Escherichia-Shigella, Bacteroides, Blautia, Faecalibacterium, Lactobacillus, Streptococcus, Bifidobacterium, and Subdoligranulum, accounted for 60% of the total bacterial population (Figure 2C). Notably, the proportion of Escherichia-Shigella in the TIA group was significantly increased.
The comparisons of differences among the three groups were exhibited at the genus level (Figures 3A–C) and the family level (Figure 3D). At the family level, Butyricicoccaceae (p < 0.05) displayed significant differences among the three groups (Figure 3D). At the genus level, Lactobacillus (p < 0.01), Streptococcus (p < 0.001), and Lachnospiraceae_UCG-004 (p < 0.05) revealed significant differences (Figures 3A–C). In addition, Lactobacillus and Streptococcus showed a progressive increase tendency from HC and TIA to AIS, while Lachnospiraceae_UCG-004 displayed a decrease tendency. LDA discriminant histogram analyzed multi-level differential species and used LDA value to measure the influence of species on the differential effect, suggesting that the species might play a key role in the occurrence and development of diseases. As shown in Figure 3E, the LDA discriminant histogram revealed the most significant taxa representing differences among the three groups from family to genus level. A total of 26 taxa with an LDA score of >2 were selected as characteristic bacteria to distinguish three groups (Figure 3E).
Figure 3. The difference of characteristic bacteria among the three groups. Histogram of bacterial differences based on Wilcoxon rank-sum test at family (D) and genus levels (A–C). (E) Histogram of LDA discrimination showing the phylogenetic relationships of bacteria taxa and LDA scores among three groups, based on LDA scores >2. *p < 0.05, **p < 0.01, ***p < 0.001.
The heatmap showed that the abundance of Lactobacillus displayed an increasing trend across the HC, TIA, and AIS groups. It was significantly positively correlated with the stroke risk factor Hcy (ρ = 0.2235, p < 0.05) and negatively correlated with HDL (ρ = −0.2395, p < 0.05). Similarly, the abundance of Streptococcus exhibited a significant negative correlation with HDL (ρ = −0.1135, p < 0.05).
In contrast, the abundance of Lachnospiraceae_UCG-004, which exhibited a decreasing trend in the HC, TIA, and AIS groups, was significantly negatively correlated with the stroke risk factors D-dimer (ρ = −0.1393, p < 0.05) and Hcy (ρ = −0.1129, p < 0.05). Additionally, among the bacterial flora that increased in AIS patients compared to HC, the abundance of unclassified_c__Bacilli showed a significant positive correlation with D-dimer (ρ = 0.1125, p < 0.05), HbA1c (ρ = 0.1291, p < 0.05), and Hcy (ρ = 0.1243, p < 0.05).
The abundance of Anaerovoracaceae was also positively correlated with D-dimer (ρ = 0.1259, p < 0.05) and HbA1c (ρ = 0.1146, p < 0.05) while demonstrating a significant negative correlation with triglycerides (TG) (ρ = −0.1491, p < 0.05). Finally, the abundance of Synergistaceae exhibited a significant negative correlation with HDL (ρ = −0.1253, p < 0.05) (Figure 4).
Figure 4. Correlation analysis between characteristic bacteria and clinical indicators. The heatmap revealed associations between differential bacteria and clinical factors (based on Spearman). The red color was positively correlated, and blue color was negatively correlated. Deeper colors indicated higher correlation values. *p < 0.05, **p < 0.01, ***p < 0.01.
According to the LDA discriminant histogram and heatmap diagram, we screened out Lactobacillus and Streptococcus as the significant genus to distinguish TIA from AIS. As shown in Figure 5, Lactobacillus achieved an AUC value of 0.626 (p < 0.05), and Streptococcus achieved an AUC value of 0.699 (p < 0.05).
Figure 5. ROC curve analysis of potential microbial biomarkers for prediction of AIS patients. The green line represented Streptococcus, which achieved an AUC value of 0.699 (p < 0.05), and the purple line represented Lactobacillus, which achieved an AUC value of 0.626 (p < 0.05).
In this study, gut microbial diversity in the TIA and AIS groups exhibited significant distinctions compared with the HC group.
AIS patients exhibited an enriched abundance of Lactobacillus and Streptococcus, along with a decreased abundance of Lachnospiraceae_UCG-004. Moreover, further study revealed characteristic bacteria: the abundance of Lactobacillus and Streptococcus was negatively correlated with HDL, and Lactobacillus was positively correlated with risk factors Hcy. In contrast, the abundance of Lachnospiraceae_UCG-004 negatively correlated with Hcy and D-dimer. Notably, ROC models based on the characteristic bacteria Lactobacillus and Streptococcus could effectively distinguish AIS patients from TIA patients. These results indicated that gut bacteria had the potential clinical utility to identify TIA patients who were likely to develop AIS.
Accumulating evidence indicated that gut microbiota played important roles in the occurrence and development of AIS. Our previous studies have demonstrated that gut microbiota composition significantly influences the outcome of AIS (Yu et al., 2023; Yao et al., 2023; Ma et al., 2024; Shi et al., 2023). Furthermore, the microbial composition significantly changes during AIS (Xu et al., 2021). Microbial alterations were also observed in animals subjected to stroke, such as the middle cerebral artery occlusion (MCAO) model (Kim et al., 2020). This study showed that TIA and AIS patients, compared with healthy controls, exhibited distinct microbial diversity, an increased microbial dysbiosis index, and altered bacterial communities. Yin et al. revealed that patients with stroke and TIA showed significant dysbiosis of the gut microbiota (Yin et al., 2015). These findings suggested that altered gut microbiota might be implicated in the initiation and pathogenesis of stroke.
In this study, we characterized the dysbiosis of gut microbiota in the TIA and AIS groups, noting an enriched abundance of genera, such as Streptococcus and Lactobacillus, alongside a decreased abundance of short-chain fatty acids (SCFA)-producing bacteria, specifically Butyricicoccaceae and Lachnospiraceae_UCG-004, compared to the HC group. Notably, Lactobacillus and Streptococcus showed a progressive increase from HC to TIA and then to AIS, while Butyricicoccaceae and Lachnospiraceae_UCG-004 displayed a decreasing trend across the same groups.
Streptococcus is a Gram-positive bacterium (Good, 2020). Consistent with our findings, other studies reported an increased abundance of Streptococcus in stroke patients (Peh et al., 2022; Yu et al., 2023; Sun et al., 2023). It has been suggested that Streptococcus can lead to endocarditis, promoting the occurrence of ischemic stroke (Stöllberger et al., 2003; Cao and Bi, 2019). In addition, Lactobacillus also showed an upward trend across the three groups. Lactobacillus has been extensively studied for its beneficial effects on human health (Avall-Jääskeläinen and Palva, 2005; Slattery et al., 2019). However, the function of Lactobacillus can also vary depending on factors such as the host’s immune status, the specific strain of Lactobacillus, and the presence of underlying diseases (Goldstein et al., 2015). Consistent with our results, other studies have also indicated an increase in Lactobacillus among stroke patients (Yamashiro et al., 2017; Li et al., 2020; Ling et al., 2020).
Additionally, the abundance of Lachnospiraceae_UCG-004 in the AIS group decreased compared to the HC group. Lachnospiraceae_UCG-004 was involved in the production of SCFAs (Jin et al., 2019; Huang et al., 2020). It was reported that SCFAs could relieve symptoms of the diseases by reducing neurotoxicity and neuroinflammation (Alpino et al., 2024; Zhang et al., 2021).
The pathogenesis of AIS has not been fully understood, but inflammation plays a pivotal role in the occurrence and progression of stroke (Chaturvedi and De Marchis, 2024; Kelly et al., 2021; Candelario-Jalil et al., 2022). Intense neuroinflammation, which occurred during the acute phase of stroke, was associated with blood–brain barrier (BBB) breakdown, neuronal injury, and worse neurological outcomes (Simats and Liesz, 2022; Oh and Parikh, 2022). The systemic inflammatory state induced by metabolic disorders, such as higher Hcy, HBA1c, and TG, actively participates in AIS pathogenesis (Ge et al., 2022). Streptococcus was reported to trigger an inflammatory response by leading to systemic induction of interleukin (IL)-1 and profoundly exacerbated (50–90%) ischemic brain injury in rats and mice (Dénes et al., 2014). This is evidenced by the active secretion of a variety of inflammatory mediators by the adipose tissue in obese individuals, which compounds the effects of traditional AIS risk factors like hypertension and hyperlipidemia (Chait and den Hartigh, 2020). Emerging evidence indicated that dysbiosis of the gut microbiota could cause an imbalance of metabolites and thereby affect the progression of stroke. The abundance of Lachnospiraceae_UCG-004, an SCFA-producing bacteria, decreased in the AIS group compared with the HC group. SCFA reduction might have a detrimental role in the whole setting of systemic inflammation. Chen R et al. confirmed that supplementation with butyrate in model rats of AIS could effectively remodel the gut microbiota and intestinal permeability and improve neurological deficits (Chen et al., 2019). SCFAs could alleviate hypertension, mitigate systemic inflammation, and decrease aortic atherosclerotic lesion area, which revealed the important association between SCFA-producing bacteria and vascular diseases (Dalile et al., 2019; Hu et al., 2022; Frampton et al., 2020). Animals subjected to the MCAO model, which mimics ischemic stroke, have been reported to have lower levels of SCFAs (Deleu et al., 2021). SCFAs can decrease microglial activation and modulate the integrity of BBB (Duan et al., 2023). In this study, we elucidated potential increases in inflammatory bacteria and decreases in SCFA-producing bacteria during the progression from TIA to AIS. Consequently, abnormal gut microbiota may influence the occurrence and progression of stroke through inflammatory responses and metabolite production.
In this study, our results showed that several risk factors for AIS, such as elevated D-dimer, HBA1c, Hcy, and TG, were associated with specific bacterial characteristics. A recent study demonstrated that AIS risk factors, such as metabolic disorders, hypertension, diabetes, obesity, and systemic inflammation, have been related to gut microbiota dysbiosis (Battaglini et al., 2020). This dysbiosis subsequently exacerbates the outcomes of AIS. Additionally, we established a diagnostic model for AIS using several abundant bacteria, including Lactobacillus and Streptococcus; the AUC demonstrated satisfactory predictive performance. These findings suggest that AIS patients exhibit more significant differences in gut microbial composition compared to those with transient ischemic attack (TIA), indicating that characteristic bacteria may serve as diagnostic biomarkers for AIS.
However, this study still has several limitations. First, it was a single-center study, limiting the observation of dynamic changes between participants and their gut microbiota. Second, the correlation analysis between the microbiota and AIS did not establish a causal relationship. Further validation of the identified differential bacteria and metabolites is essential to elucidate the underlying mechanisms of their interactions. Finally, individual variations in dietary habits and drug use might influence the composition and function of gut microbiota. Therefore, further analyses are imperative to elucidate the nuanced relationship between gut microbiota and AIS. In addition, addressing these limitations through rigorous experimental design and larger-scale clinical studies will contribute to a more comprehensive understanding of the potential of characteristic bacteria in diagnosing AIS.
In conclusion, this study revealed that the gut microbiota of AIS and TIA patients undergo significant changes. Moreover, Streptococcus and Lactobacillus were microbial biomarkers for AIS, which was worthy of further study on clinical application. These findings assist in predicting AIS in TIA patients and facilitate early warnings for AIS.
The datasets presented in this study can be found in online repositories. The names of the repository/repositories and accession number(s) can be found at: https://www.ncbi.nlm.nih.gov/, PRJNA1140893.
The studies involving humans were approved by the Medical Ethics Committee of the Second Affiliated Hospital of Wenzhou Medical University. The studies were conducted in accordance with the local legislation and institutional requirements. The participants provided their written informed consent to participate in this study.
SY: Writing – original draft. JSh: Investigation, Software, Writing – review & editing. GY: Data curation, Investigation, Writing – review & editing. JX: Investigation, Software, Writing – review & editing. YD: Formal analysis, Investigation, Writing – review & editing. YL: Formal analysis, Investigation, Writing – review & editing. HX: Data curation, Investigation, Writing – review & editing. JL: Formal analysis, Writing – review & editing. JSu: Writing – original draft, Writing – review & editing.
The author(s) declare that financial support was received for the research, authorship, and/or publication of this article. This study was supported by the Clinical Medical Research Project of Zhejiang Medical Association (2022ZYC-D10).
The authors declare that the research was conducted in the absence of any commercial or financial relationships that could be construed as a potential conflict of interest.
All claims expressed in this article are solely those of the authors and do not necessarily represent those of their affiliated organizations, or those of the publisher, the editors and the reviewers. Any product that may be evaluated in this article, or claim that may be made by its manufacturer, is not guaranteed or endorsed by the publisher.
The Supplementary material for this article can be found online at: https://www.frontiersin.org/articles/10.3389/fnagi.2024.1451968/full#supplementary-material
Alpino, G., Pereira-Sol, G. A., Dias, M. M. E., Aguiar, A. S., and Peluzio, M. (2024). Beneficial effects of butyrate on brain functions: a view of epigenetic. Crit. Rev. Food Sci. Nutr. 64, 3961–3970. doi: 10.1080/10408398.2022.2137776
Amarenco, P., Labreuche, J., and Lavallée, P. C. (2012). Patients with transient ischemic attack with ABCD2 <4 can have similar 90-day stroke risk as patients with transient ischemic attack with ABCD2 ≥4. Stroke 43, 863–865. doi: 10.1161/strokeaha.111.636506
Avall-Jääskeläinen, S., and Palva, A. (2005). Lactobacillus surface layers and their applications. FEMS Microbiol. Rev. 29, 511–529. doi: 10.1016/j.fmrre.2005.04.003
Battaglini, D., Pimentel-Coelho, P. M., Robba, C., Dos Santos, C. C., Cruz, F. F., Pelosi, P., et al. (2020). Gut microbiota in acute ischemic stroke: from pathophysiology to therapeutic implications. Front. Neurol. 11:598. doi: 10.3389/fneur.2020.00598
Candelario-Jalil, E., Dijkhuizen, R. M., and Magnus, T. (2022). Neuroinflammation, stroke, blood-brain barrier dysfunction, and imaging modalities. Stroke 53, 1473–1486. doi: 10.1161/strokeaha.122.036946
Cao, G. F., and Bi, Q. (2019). Pediatric infective endocarditis and stroke: a 13-year single-center review. Pediatr. Neurol. 90, 56–60. doi: 10.1016/j.pediatrneurol.2018.07.001
Chait, A., and den Hartigh, L. J. (2020). Adipose tissue distribution, inflammation and its metabolic consequences, including diabetes and cardiovascular disease. Front Cardiovasc Med 7:22. doi: 10.3389/fcvm.2020.00022
Chaturvedi, S., and De Marchis, G. M. (2024). Inflammatory biomarkers and stroke subtype: an important new frontier. Neurology 102:e208098. doi: 10.1212/wnl.0000000000208098
Chen, R., Xu, Y., Wu, P., Zhou, H., Lasanajak, Y., Fang, Y., et al. (2019). Transplantation of fecal microbiota rich in short chain fatty acids and butyric acid treat cerebral ischemic stroke by regulating gut microbiota. Pharmacol. Res. 148:104403. doi: 10.1016/j.phrs.2019.104403
Dalile, B., Van Oudenhove, L., Vervliet, B., and Verbeke, K. (2019). The role of short-chain fatty acids in microbiota-gut-brain communication. Nat. Rev. Gastroenterol. Hepatol. 16, 461–478. doi: 10.1038/s41575-019-0157-3
Deleu, S., Machiels, K., Raes, J., Verbeke, K., and Vermeire, S. (2021). Short chain fatty acids and its producing organisms: an overlooked therapy for IBD? EBioMedicine 66:103293. doi: 10.1016/j.ebiom.2021.103293
Dénes, Á., Pradillo, J. M., Drake, C., Sharp, A., Warn, P., Murray, K. N., et al. (2014). Streptococcus pneumoniae worsens cerebral ischemia via interleukin 1 and platelet glycoprotein Ibα. Ann. Neurol. 75, 670–683. doi: 10.1002/ana.24146
Duan, H., Wang, L., Huangfu, M., and Li, H. (2023). The impact of microbiota-derived short-chain fatty acids on macrophage activities in disease: mechanisms and therapeutic potentials. Biomed. Pharmacother. 165:115276. doi: 10.1016/j.biopha.2023.115276
Easton, J. D., Saver, J. L., Albers, G. W., Alberts, M. J., Chaturvedi, S., Feldmann, E., et al. (2009). Definition and evaluation of transient ischemic attack: a scientific statement for healthcare professionals from the American Heart Association/American Stroke Association stroke council; council on cardiovascular surgery and anesthesia; council on cardiovascular radiology and intervention; council on cardiovascular nursing; and the interdisciplinary council on peripheral vascular disease. The American Academy of Neurology affirms the value of this statement as an educational tool for neurologists. Stroke 40, 2276–2293. doi: 10.1161/strokeaha.108.192218
Frampton, J., Murphy, K. G., Frost, G., and Chambers, E. S. (2020). Short-chain fatty acids as potential regulators of skeletal muscle metabolism and function. Nat. Metab. 2, 840–848. doi: 10.1038/s42255-020-0188-7
Ge, Y., Zadeh, M., Yang, C., Candelario-Jalil, E., and Mohamadzadeh, M. (2022). Ischemic stroke impacts the gut microbiome, ileal epithelial and immune homeostasis. iScience 25:105437. doi: 10.1016/j.isci.2022.105437
Goldstein, E. J., Tyrrell, K. L., and Citron, D. M. (2015). Lactobacillus species: taxonomic complexity and controversial susceptibilities. Clin. Infect. Dis. 60, S98–S107. doi: 10.1093/cid/civ072
Good, M. F. (2020). Streptococcus: an organism causing diseases beyond neglect. PLoS Negl. Trop. Dis. 14:e0008095. doi: 10.1371/journal.pntd.0008095
Hill, M. D., Yiannakoulias, N., Jeerakathil, T., Tu, J. V., Svenson, L. W., and Schopflocher, D. P. (2004). The high risk of stroke immediately after transient ischemic attack: a population-based study. Neurology 62, 2015–2020. doi: 10.1212/01.wnl.0000129482.70315.2f
Honarpisheh, P., Bryan, R. M., and McCullough, L. D. (2022). Aging microbiota-gut-brain Axis in stroke risk and outcome. Circ. Res. 130, 1112–1144. doi: 10.1161/circresaha.122.319983
Hu, T., Wu, Q., Yao, Q., Jiang, K., Yu, J., and Tang, Q. (2022). Short-chain fatty acid metabolism and multiple effects on cardiovascular diseases. Ageing Res. Rev. 81:101706. doi: 10.1016/j.arr.2022.101706
Huang, K., Yu, W., Li, S., Guan, X., Liu, J., Song, H., et al. (2020). Effect of embryo-remaining oat rice on the lipid profile and intestinal microbiota in high-fat diet fed rats. Food Res. Int. 129:108816. doi: 10.1016/j.foodres.2019.108816
Jin, M., Kalainy, S., Baskota, N., Chiang, D., Deehan, E. C., McDougall, C., et al. (2019). Faecal microbiota from patients with cirrhosis has a low capacity to ferment non-digestible carbohydrates into short-chain fatty acids. Liver Int. 39, 1437–1447. doi: 10.1111/liv.14106
Johnston, S. C., Rothwell, P. M., Nguyen-Huynh, M. N., Giles, M. F., Elkins, J. S., Bernstein, A. L., et al. (2007). Validation and refinement of scores to predict very early stroke risk after transient ischaemic attack. Lancet 369, 283–292. doi: 10.1016/s0140-6736(07)60150-0
Kelly, P. J., Lemmens, R., and Tsivgoulis, G. (2021). Inflammation and stroke risk: a new target for prevention. Stroke 52, 2697–2706. doi: 10.1161/strokeaha.121.034388
Kim, K. A., Kim, D., Kim, J. H., Shin, Y. J., Kim, E. S., Akram, M., et al. (2020). Autophagy-mediated occludin degradation contributes to blood-brain barrier disruption during ischemia in bEnd.3 brain endothelial cells and rat ischemic stroke models. Fluids Barriers CNS 17:21. doi: 10.1186/s12987-020-00182-8
Lee, J., d'Aigle, J., Atadja, L., Quaicoe, V., Honarpisheh, P., Ganesh, B. P., et al. (2020). Gut microbiota-derived short-chain fatty acids promote poststroke recovery in aged mice. Circ. Res. 127, 453–465. doi: 10.1161/circresaha.119.316448
Li, H., Zhang, X., Pan, D., Liu, Y., Yan, X., Tang, Y., et al. (2020). Dysbiosis characteristics of gut microbiota in cerebral infarction patients. Transl. Neurosci. 11, 124–133. doi: 10.1515/tnsci-2020-0117
Lima Filho, J. B., de Lima, T. I., Luvizutto, G. J., Pereira Braga, G., and Bazan, R. (2016). ABCD2 score and secondary stroke prevention: meta-analysis and effect per 1,000 patients triaged. Neurology 86:697. doi: 10.1212/wnl.0000000000002411
Ling, Y., Gong, T., Zhang, J., Gu, Q., Gao, X., Weng, X., et al. (2020). Gut microbiome signatures are biomarkers for cognitive impairment in patients with ischemic stroke. Front. Aging Neurosci. 12:511562. doi: 10.3389/fnagi.2020.511562
Ma, J., Xie, H., Yuan, C., Shen, J., Chen, J., Chen, Q., et al. (2024). The gut microbial signatures of patients with lacunar cerebral infarction. Nutr. Neurosci. 27, 620–636. doi: 10.1080/1028415x.2023.2242121
Oh, S. E., and Parikh, N. S. (2022). Recent advances in the impact of infection and inflammation on stroke risk and outcomes. Curr. Neurol. Neurosci. Rep. 22, 161–170. doi: 10.1007/s11910-022-01179-6
Peh, A., O'Donnell, J. A., Broughton, B. R. S., and Marques, F. Z. (2022). Gut microbiota and their metabolites in stroke: a double-edged sword. Stroke 53, 1788–1801. doi: 10.1161/strokeaha.121.036800
Plovier, H., Everard, A., Druart, C., Depommier, C., Van Hul, M., Geurts, L., et al. (2017). A purified membrane protein from Akkermansia muciniphila or the pasteurized bacterium improves metabolism in obese and diabetic mice. Nat. Med. 23, 107–113. doi: 10.1038/nm.4236
Pluta, R., Januszewski, S., and Czuczwar, S. J. (2021). The role of gut microbiota in an ischemic stroke. Int. J. Mol. Sci. 22:915. doi: 10.3390/ijms22020915
Rothwell, P. M., Buchan, A., and Johnston, S. C. (2006). Recent advances in the management of transient ischaemic attacks and minor ischaemic strokes. Lancet Neurol. 5, 323–331. doi: 10.1016/s1474-4422(06)70408-2
Rothwell, P. M., and Warlow, C. P. (2005). Timing of TIAs preceding stroke: time window for prevention is very short. Neurology 64, 817–820. doi: 10.1212/01.Wnl.0000152985.32732.Ee
Sacco, R. L., Kasner, S. E., Broderick, J. P., Caplan, L. R., Connors, J. J., Culebras, A., et al. (2013). An updated definition of stroke for the 21st century: a statement for healthcare professionals from the American Heart Association/American Stroke Association. Stroke 44, 2064–2089. doi: 10.1161/STR.0b013e318296aeca
Shi, J., Zhao, Y., Chen, Q., Liao, X., Chen, J., Xie, H., et al. (2023). Association analysis of gut microbiota and prognosis of patients with acute ischemic stroke in basal ganglia region. Microorganisms 11:2667. doi: 10.3390/microorganisms11112667
Simats, A., and Liesz, A. (2022). Systemic inflammation after stroke: implications for post-stroke comorbidities. EMBO Mol. Med. 14:e16269. doi: 10.15252/emmm.202216269
Singh, V., Roth, S., Llovera, G., Sadler, R., Garzetti, D., Stecher, B., et al. (2016). Microbiota dysbiosis controls the neuroinflammatory response after stroke. J. Neurosci. 36, 7428–7440. doi: 10.1523/jneurosci.1114-16.2016
Slattery, C., Cotter, P. D., and O'Toole, P. W. (2019). Analysis of health benefits conferred by Lactobacillus species from kefir. Nutrients 11:1252. doi: 10.3390/nu11061252
Stöllberger, C., Finsterer, J., Pratter, A., Kopsa, W., Preiser, J., and Valentin, A. (2003). Ischemic stroke and splenic rupture in a case of Streptococcus bovis endocarditis. J. Clin. Microbiol. 41, 2654–2658. doi: 10.1128/jcm.41.6.2654-2658.2003
Sun, W., Huang, S., Yang, X., Luo, Y., Liu, L., and Wu, D. (2023). The oral microbiome of patients with ischemic stroke predicts their severity and prognosis. Front. Immunol. 14:1171898. doi: 10.3389/fimmu.2023.1171898
Winek, K., Engel, O., Koduah, P., Heimesaat, M. M., Fischer, A., Bereswill, S., et al. (2016). Depletion of cultivatable gut microbiota by broad-Spectrum antibiotic pretreatment worsens outcome after murine stroke. Stroke 47, 1354–1363. doi: 10.1161/strokeaha.115.011800
Wu, O., Cloonan, L., Mocking, S. J., Bouts, M. J., Copen, W. A., Cougo-Pinto, P. T., et al. (2015). Role of acute lesion topography in initial ischemic stroke severity and long-term functional outcomes. Stroke 46, 2438–2444. doi: 10.1161/strokeaha.115.009643
Xu, K., Gao, X., Xia, G., Chen, M., Zeng, N., Wang, S., et al. (2021). Rapid gut dysbiosis induced by stroke exacerbates brain infarction in turn. Gut 70, 1486–1494. doi: 10.1136/gutjnl-2020-323263
Xu, D. J., Wang, K. C., Yuan, L. B., Li, H. F., Xu, Y. Y., Wei, L. Y., et al. (2021). Compositional and functional alterations of gut microbiota in patients with stroke. Nutr. Metab. Cardiovasc. Dis. 31, 3434–3448. doi: 10.1016/j.numecd.2021.08.045
Yamashiro, K., Tanaka, R., Urabe, T., Ueno, Y., Yamashiro, Y., Nomoto, K., et al. (2017). Gut dysbiosis is associated with metabolism and systemic inflammation in patients with ischemic stroke. PLoS One 12:e0171521. doi: 10.1371/journal.pone.0171521
Yao, S., Xie, H., Wang, Y., Shen, N., Chen, Q., Zhao, Y., et al. (2023). Predictive microbial feature analysis in patients with depression after acute ischemic stroke. Front. Aging Neurosci. 15:1116065. doi: 10.3389/fnagi.2023.1116065
Yin, J., Liao, S. X., He, Y., Wang, S., Xia, G. H., Liu, F. T., et al. (2015). Dysbiosis of gut microbiota with reduced trimethylamine-N-oxide level in patients with large-artery atherosclerotic stroke or transient ischemic attack. J. Am. Heart Assoc. 4:e002699. doi: 10.1161/jaha.115.002699
Yu, S., Chen, J., Zhao, Y., Liao, X., Chen, Q., Xie, H., et al. (2023). Association analysis of the gut microbiota in predicting outcomes for patients with acute ischemic stroke and H-type hypertension. Front. Neurol. 14:1275460. doi: 10.3389/fneur.2023.1275460
Zavaglia, M., Forkert, N. D., Cheng, B., Gerloff, C., Thomalla, G., and Hilgetag, C. C. (2015). Mapping causal functional contributions derived from the clinical assessment of brain damage after stroke. Neuroimage Clin 9, 83–94. doi: 10.1016/j.nicl.2015.07.009
Keywords: acute ischemic stroke, biomarkers, gut microbiota, predict, transient ischemic attack
Citation: Yu S, Shi J, Yu G, Xu J, Dong Y, Lin Y, Xie H, Liu J and Sun J (2024) Specific gut microbiome signatures predict the risk of acute ischemic stroke. Front. Aging Neurosci. 16:1451968. doi: 10.3389/fnagi.2024.1451968
Received: 20 June 2024; Accepted: 21 October 2024;
Published: 08 November 2024.
Edited by:
Kristy A. Nielson, Marquette University, United StatesReviewed by:
Prabhakar Singh, Sathyabama Institute of Science and Technology, IndiaCopyright © 2024 Yu, Shi, Yu, Xu, Dong, Lin, Xie, Liu and Sun. This is an open-access article distributed under the terms of the Creative Commons Attribution License (CC BY). The use, distribution or reproduction in other forums is permitted, provided the original author(s) and the copyright owner(s) are credited and that the original publication in this journal is cited, in accordance with accepted academic practice. No use, distribution or reproduction is permitted which does not comply with these terms.
*Correspondence: Jiaming Liu, d3pqaWFtaW5nX2xpdUAxNjMuY29t; Jing Sun, c3Vuand6QDEyNi5jb20=
†These authors have contributed equally to this work
Disclaimer: All claims expressed in this article are solely those of the authors and do not necessarily represent those of their affiliated organizations, or those of the publisher, the editors and the reviewers. Any product that may be evaluated in this article or claim that may be made by its manufacturer is not guaranteed or endorsed by the publisher.
Research integrity at Frontiers
Learn more about the work of our research integrity team to safeguard the quality of each article we publish.