- Department of Radiology, The Affiliated Brain Hospital of Nanjing Medical University, Nanjing, China
Background: Stable mild cognitive impairment (sMCI) and progressive mild cognitive impairment (pMCI) represent two distinct subtypes of mild cognitive impairment (MCI). Early and effective diagnosis and accurate differentiation between sMCI and pMCI are crucial for administering targeted early intervention and preventing cognitive decline. This study investigated the intrinsic dysconnectivity patterns in sMCI and pMCI based on degree centrality (DC) and effective connectivity (EC) analyses, with the goal of uncovering shared and distinct neuroimaging mechanisms between subtypes.
Methods: Resting-state functional magnetic resonance imaging combined with DC analysis was used to explore the functional connectivity density in 42 patients with sMCI, 31 patients with pMCI, and 82 healthy control (HC) participants. Granger causality analysis was used to assess changes in EC based on the significant clusters found in DC. Furthermore, correlation analysis was conducted to examine the associations between altered DC/EC values and cognitive function. Receiver operating characteristic curve analysis was performed to determine the accuracy of abnormal DC and EC values in distinguishing sMCI from pMCI.
Results: Compared with the HC group, both pMCI and sMCI groups exhibited increased DC in the left inferior temporal gyrus (ITG), left posterior cerebellum lobe (CPL), and right cerebellum anterior lobe (CAL), along with decreased DC in the left medial frontal gyrus. Moreover, the sMCI group displayed reduced EC from the right CAL to bilateral CPL, left superior temporal gyrus, and bilateral caudate compared with HC. pMCI demonstrated elevated EC from the right CAL to left ITG, which was linked to episodic memory and executive function. Notably, the EC from the right CAL to the right ITG effectively distinguished sMCI from pMCI, with sensitivity, specificity, and accuracy of 0.5806, 0.9512, and 0.828, respectively.
Conclusion: This study uncovered shared and distinct alterations in DC and EC between sMCI and pMCI, highlighting their involvement in cognitive function. Of particular significance are the unidirectional EC disruptions from the cerebellum to the temporal lobe, which serve as a discriminating factor between sMCI and pMCI and provide a new perspective for understanding the temporal-cerebellum. These findings offer novel insights into the neural circuit mechanisms involving the temporal-cerebellum connection in MCI.
Introduction
Mild cognitive impairment (MCI), the preclinical stage of Alzheimer’s disease (AD), is a transitional and intermediary cognitive phase between normal aging and AD (Jessen et al., 2014a; Jessen et al., 2014b). Approximately 10–15% of MCI cases progress to AD annually, compared with only 1–2% of healthy individuals (Jessen et al., 2014b; Petersen et al., 2014). Given AD’s irreversible and progressive neurodegenerative nature, the in-depth study of MCI is of paramount importance. MCI is a heterogeneous condition; not all patients with MCI will inevitably transition to AD during their lifetime, as some may remain relatively stable, and a small subset may even regain normal cognitive function after prolonged follow-up (Mitchell and Shiri-Feshki, 2009; Petersen et al., 2014). According to the outcomes, MCI is classified into stable MCI (sMCI), which maintains MCI status, and progressive MCI (pMCI), which advances into AD (Pereira et al., 2016). The underlying neural mechanisms that govern the progression from MCI to AD remain unclear. Consequently, understanding the neuroimaging mechanisms of sMCI and pMCI is crucial for predicting MCI outcomes and enabling early and timely intervention.
In recent years, resting-state functional magnetic resonance imaging (fMRI) studies have suggested AD as a potential classic disconnection syndrome (Wang et al., 2007; Gu et al., 2020). This means that the changes in brain function in AD are not confined to the changes in a single brain area, but rather involve the changes in the whole brain network (Costumero et al., 2020). Similarly, MCI involves alterations and disruptions in the entire brain network (Xue C. et al., 2019; Xue et al., 2020). The brain connectivity patterns from the metabolic network have demonstrated decreasing inter-and intra-hemispheric connections in both sMCI and pMCI (Huang et al., 2018). Malotaux et al. reported that pMCI involved significantly higher connectivity, particularly within the default mode network (DMN), than sMCI (Malotaux et al., 2023). In pMCI, DMN connectivity increased over time, and the rate of connectivity change was correlated with the rate of cognitive decline. Cai et al. reported that sMCI and pMCI had varying degrees of alterations in the executive control network (Cai et al., 2017). However, to date, few studies have investigated the alterations in whole-brain functional connectivity (FC) patterns or directional connectivity networks associated with sMCI and pMCI.
Degree centrality (DC), a metric derived from graph theory, can assess topological properties in the whole-brain functional network. The DC represents the number of direct connections (or significant suprathreshold correlation weights) of a given voxel within the voxel-linker, measuring the importance of individual nodes. This reflects the “hub” characteristic of brain functional networks (Zuo and Xing, 2014). DC provides an unbiased approach for exploring anomalies across the complete connectivity matrix of the full-brain functional connectome. It allows for the study of functional brain abnormalities at the whole-brain level without prior hypotheses (Zuo et al., 2012). This method is confirmed to have a high level of sensitivity, specificity, and test–retest reliability. It has been utilized to examine the neurobiological mechanisms underlying brain network alterations in various neurological disorders including the AD spectrum and Parkinson’s disease (Lou et al., 2015; Zhou J. et al., 2021; Shan et al., 2023).
Furthermore, FC solely depicts interactions between distinct brain regions, whereas effective connectivity (EC) delves into the direction and intensity of information flow among these regions, providing a deeper understanding of the interaction patterns of different brain regions (Friston et al., 2013; Huang et al., 2021). EC-based findings align more closely with actual brain function mechanisms (Friston et al., 2013). Granger causality analysis (GCA), an approach for assessing EC, models interactions between significantly distinct brain regions (Liao et al., 2010). In recent years, this time-series analysis technique has played a significant role in fMRI causal modeling studies of brain regions using fMRI (Liao et al., 2010; Wang et al., 2017). GCA does not presuppose theoretical assumptions about the existence and direction of influence between any two regions. Xue et al. explored the changes of EC between the hippocampus and other brain regions in MCI and AD, and found abnormalities in the transmission and reception of information in the hippocampus (Xue J. et al., 2019). Yu et al. used GCA to explore the changes of EC of triple networks in aMCI and AD, and found that the EC (excitatory and inhibitory) obtained from GCA could distinguish AD and amnestic MCI (Yu et al., 2019). However, most of the previous studies were based on predetermined assumptions and focused on EC of single brain regions and networks, while EC analysis based on whole brain FC was rare in AD spectrum analysis. Therefore, by combining DC and GCA, an evaluation of abnormally FC in the brain network of sMCI and pMCI can yield a comprehensive and detailed understanding of their brain network changes, providing a more holistic insight into the pathophysiological processes of AD.
In this study, we aimed to delineate the intrinsic dysconnectivity pattern within whole-brain functional networks among patients with sMCI and pMCI. Initially, we employed DC to identify the brain regions manifesting altered FC within the complete brain networks of sMCI and pMCI. Subsequently, we utilized seed-based GCA to analyze EC, thereby comprehending the causal relationships of these alterations. Furthermore, we explored the links between the altered DC and EC indices and cognitive function in individuals with sMCI and pMCI. Our hypothesis posits that the EC attributes of brain nodes, as determined by DC, can evaluate the central neural mechanisms associated with the characteristics of sMCI and pMCI, potentially aiding clinical diagnosis and early intervention.
Materials and methods
Participants
The research data used for our study were sourced from the Alzheimer’s Disease Neuroimaging Initiative (ADNI) database.1 ADNI is a longitudinal multiterm study designed to develop biomarkers for early detection and monitoring of AD, encompassing clinical, imaging, genetic, and biochemical aspects. In the ADNI dataset, there are more than 400 MCI subjects scanned at screening time. After the baseline scan, follow-up scans were acquired every 3, 6 or 12 months for up to 84 months. The present investigation selected a 4-year period as the timeframe for monitoring the transition of MCI. pMCI participants were defined as participants who transitioned from MCI to AD within 4 years, while sMCI participants were defined as participants who maintained their MCI status for at least 4 years (Thung et al., 2016; Chen et al., 2023). Healthy control (HC) participants were included if they maintained HC status for a minimum of 4 years. More detailed inclusion and exclusion criteria for HC and MCI individuals can be found on the ADNI website.2
The current rs-fMRI dataset (n = 170) consisted of 96 HC participants, 42 patients with sMCI, and 32 patients with pMCI. Among them, 16 were excluded because of excessive head motion (cumulative translation or rotation >3.0 mm or 3.00). Finally, the study analyzed 154 participants, including 82 HC, 41 sMCI, and 31 pMCI individuals.
Ethics approval and consent to participate
Ethical approval for the ADNI study was granted by the institutional review committees of all participating institutions. Participants or their authorized representatives provided written informed consent (adni.loni.usc.edu).
Neuropsychological assessment
The episodic memory (EM) and executive function (EF) were calculated according to the model provided by the ADNI website (see text footnote 1). The details regarding EM and EF were provided in Supplementary material.
MRI data acquisition
Detailed scanning information can be obtained from http://adni.loni.usc.edu/wp-content/uploads/2010/05/ADNI2/MRI/Training-Manual-FINAL.pdf and http://adni.loni.usc.edu/wp-content/uploads/2017/07/ADNI3-MRI-protocols.pdf.
Functional data preprocessing
Preprocessing of fMRI data was performed using Data Processing and Analysis for Brain Imaging3 software in MATLAB 2013b.4 The details regarding image preprocessing were provided in Supplementary material.
DC analysis
Voxel-wise DC calculations were performed using DPABI software on preprocessed data to assess network centrality, capturing FC features of neural network nodes. The details regarding DC calculation were provided in Supplementary material.
A one-way analysis of variance (ANOVA) within the gray matter mask was performed using DPABI to compare the differences in DC across the sMCI, pMCI, and HC groups, with age, sex, years of education, and gray matter volume as covariates. Nonparametric permutation testing was performed with 1,000 iterations. The significance threshold was set at a level of p < 0.05, using threshold-free cluster enhancement (TFCE) combined with family-wise error (FWE) correction, and a cluster size of >100 voxels (2,700 mm3). Post-hoc comparisons were conducted using a two-sample t-test with the mask resulting from ANOVA, with age, sex, years of education, and gray matter volume as covariates. The threshold was set at a p < 0.05 with TFCE-FWE correction and a cluster size of >20 voxels (540 mm3).
GCA
To explore directionality effects, GCA was employed to assess changes in EC. Based on the results of the DC analysis, we selected regions of interest (ROIs), that is, the brain regions with significant alterations in the ANOVA. All ROI coordinates were in the MNI space. EC was analyzed using a resting-state hemodynamic response function5 and was used to analyze EC.
In this study, the seed time series of the ROIs was defined as the seed time series x of the ROI, while the time series y represented the whole-brain voxel time series. The linear direct effects of x on y (Fx → y) and y on x (Fy → x) were calculated for each voxel in the brain. Consequently, two Granger causality maps were generated for each ROI based on the impact metric for each participant. The residual-based F was normalized (F′) and converted into Z scores for each voxel (Zy → x and Zx → y). These Z scores were derived by subtracting the global mean F′ values and dividing them by the standard deviation.
For analyzing causal connectivity at a group level, mean values of the Zy → x and Zx → y maps were computed for each group. Thus, a total of six Granger causality maps was obtained, encompassing two maps for each direction (Zy → x and Zx → y) and two for each group (the ROIs included both Zy → x and Zx → y for patients with sMCI or pMCI and HC participants). These Granger causality maps were subsequently entered into DPABI software for group comparisons.
One-way ANOVA within the gray matter mask was conducted using DPABI to evaluate differences in EC across three groups (patients with sMCI and pMCI and HC participants), while considering age, sex, years of education, and gray matter volume as covariates. The significance threshold was set with p < 0.05 (TFCE-FWE corrected), and a cluster size of >100 voxels (2,700 mm3). The two-sample t test was used for post-hoc comparisons with the mask resulting from ANOVA, with age, sex, years of education, and gray matter volume as covariates. The significance level was set with p < 0.05 (TFCE-FWE corrected) and a cluster size of >20 voxels (540 mm3).
Statistical analysis
Statistical Package for the Social Sciences (SPSS) software, version 22.0 (IBM, Armonk, New York, NY, USA) was employed for statistical analysis. ANOVA and the chi-square test were utilized to compare demographic and neurocognitive scales among the three groups: sMCI, pMCI, and HC. Bonferroni correction was applied for post-hoc comparisons, and a p value of <0.05 was considered statistically significant.
Correlation analyses were performed in SPSS, investigating relationships between altered DC and EC and cognitive domains, adjusting for age, sex, and years of education as covariates (Bonferroni corrected, p < 0.05).
Receiver operating characteristic (ROC) curve analysis was carried out using SPSS 25.0 to assess the sensitivity and specificity of the altered DC and EC indexes in differentiating sMCI from pMCI.
Results
Demographic and neurocognitive characteristics
Table 1 presents the data of demographic and neurocognitive characteristics of all participants, including 31 patients with pMCI, 41 patients with sMCI, and 82 HC participants. The HC group exhibited a significant difference in years of education compared with the pMCI and sMCI groups. As anticipated, significant differences existed in cognitive performance, with both the pMCI and sMCI groups demonstrating significantly lower EM and EF scores than the HC group (Bonferroni corrected, p < 0.05).
Degree centrality analysis
ANOVA showed significant alterations in DC among the groups, including the left inferior temporal gyrus (ITG), left cerebellum posterior lobe (CPL), right cerebellum anterior lobe (CAL), and left medial frontal gyrus (MFG). When compared with the HC group, the pMCI group showed increased DC in the left inferior frontal gyrus (IFG), left CPL, and right CAL and decreased DC in the MFG. Meanwhile, sMCI exhibited increased DC in the left ITG, left CPL, and right CPL and decreased DC in the MFG (TFCE-FWE corrected, cluster size of >20, p < 0.05). These results were obtained while accounting for age, sex, years of education, and gray matter volume (Table 2 and Figures 1, 2).
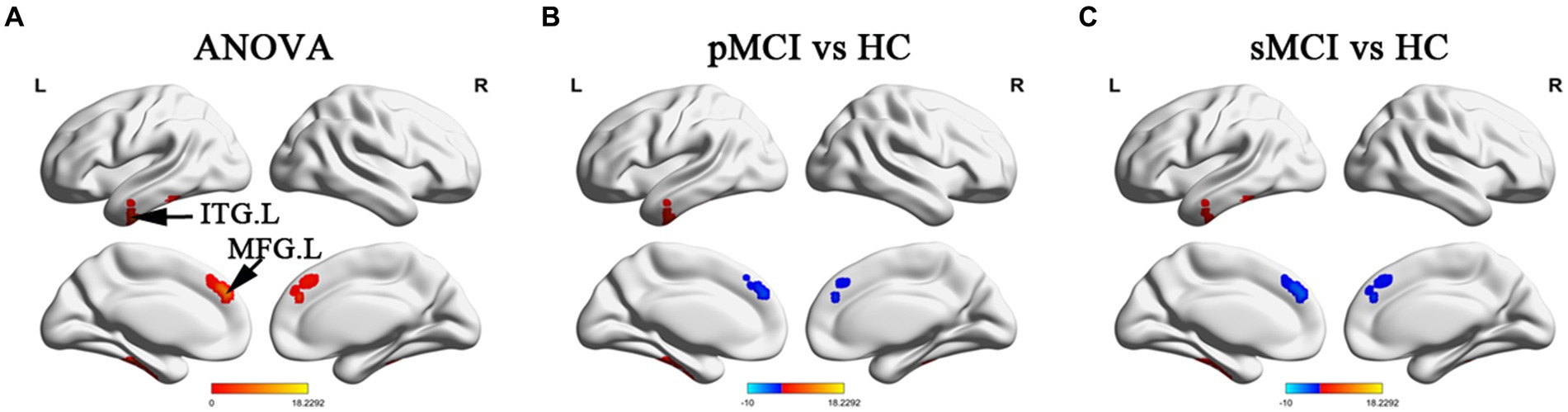
Figure 1. Brain regions exhibiting significant differences in degree centrality within cerebrum. (A) Brain regions within brain hemispheres across three groups, including sMCI, pMCI, and HC (TFCE-FWE corrected, cluster size >100, p < 0.05). (B,C) Results of post-hoc analysis in voxel-wise analysis (TFCE-FWE corrected, cluster size >20, p < 0.05). sMCI, stable mild cognitive impairment; pMCI, progressive mild cognitive impairment; HC, healthy control; ITG. L, left inferior temporal gyrus; MFG. L, left medial frontal gyrus.
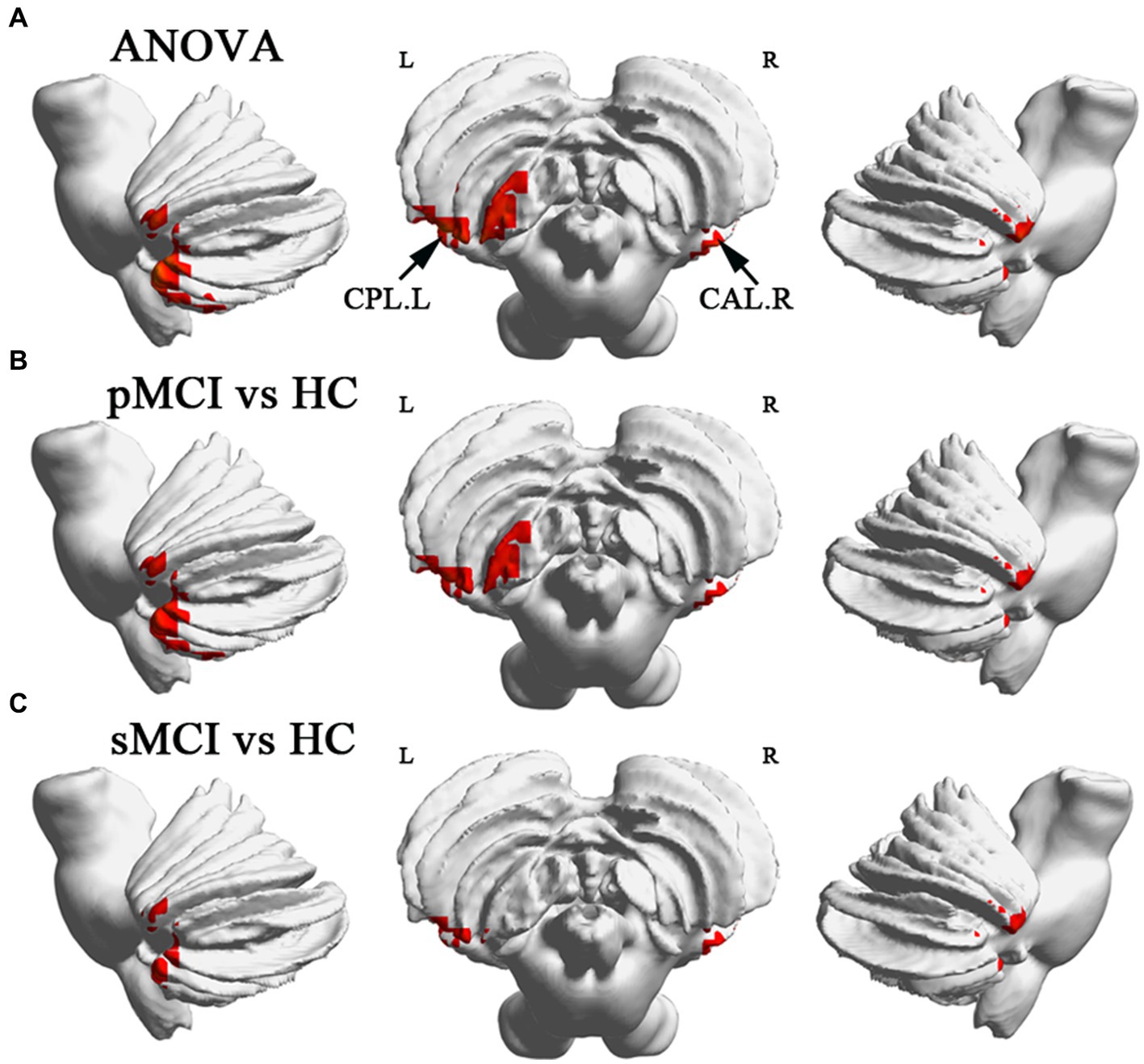
Figure 2. Brain regions exhibiting significant differences in degree centrality within cerebellum. (A) Brain regions within cerebellum across three groups, including sMCI, pMCI, and HC (TFCE-FWE corrected, cluster size >100, p < 0.05). (B,C) Results of post-hoc analysis in voxel-wise analysis (TFCE-FWE corrected, cluster size >20, p < 0.05). sMCI, stable mild cognitive impairment; pMCI, progressive mild cognitive impairment; HC, healthy control; CPL. L, left cerebellum posterior lobule; CAL. R, right cerebellum anterior lobule.
Effective connectivity analysis
Furthermore, ANOVA revealed significant alterations in EC from the right CAL among the groups, encompassing the left bilateral CPL, bilateral superior temporal gyrus (STG), and bilateral caudate. Specifically, when compared with the HC group, sMCI demonstrated decreased EC from the right CAL to the bilateral CPL, bilateral caudate, and left STG. Additionally, when compared with sMCI, pMCI displayed increased EC from the right CAL to the left ITG (TFCE-FWE corrected, cluster size of >20, p < 0.05). These findings were consistent after controlling for age, sex, years of education, and gray matter volumes (Table 3 and Figure 3).
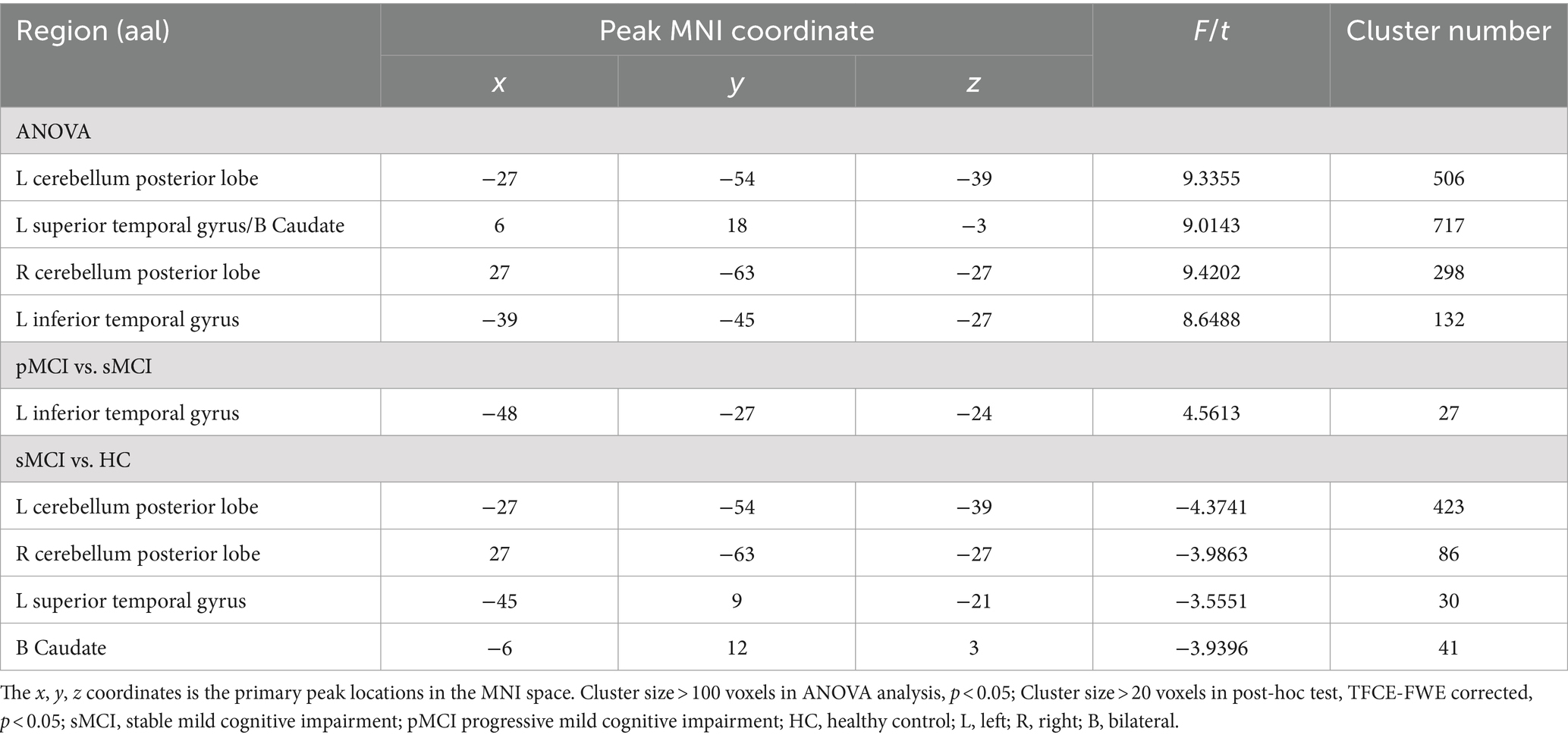
Table 3. The difference of effective connectivity from right CAL to brain regions across three groups.
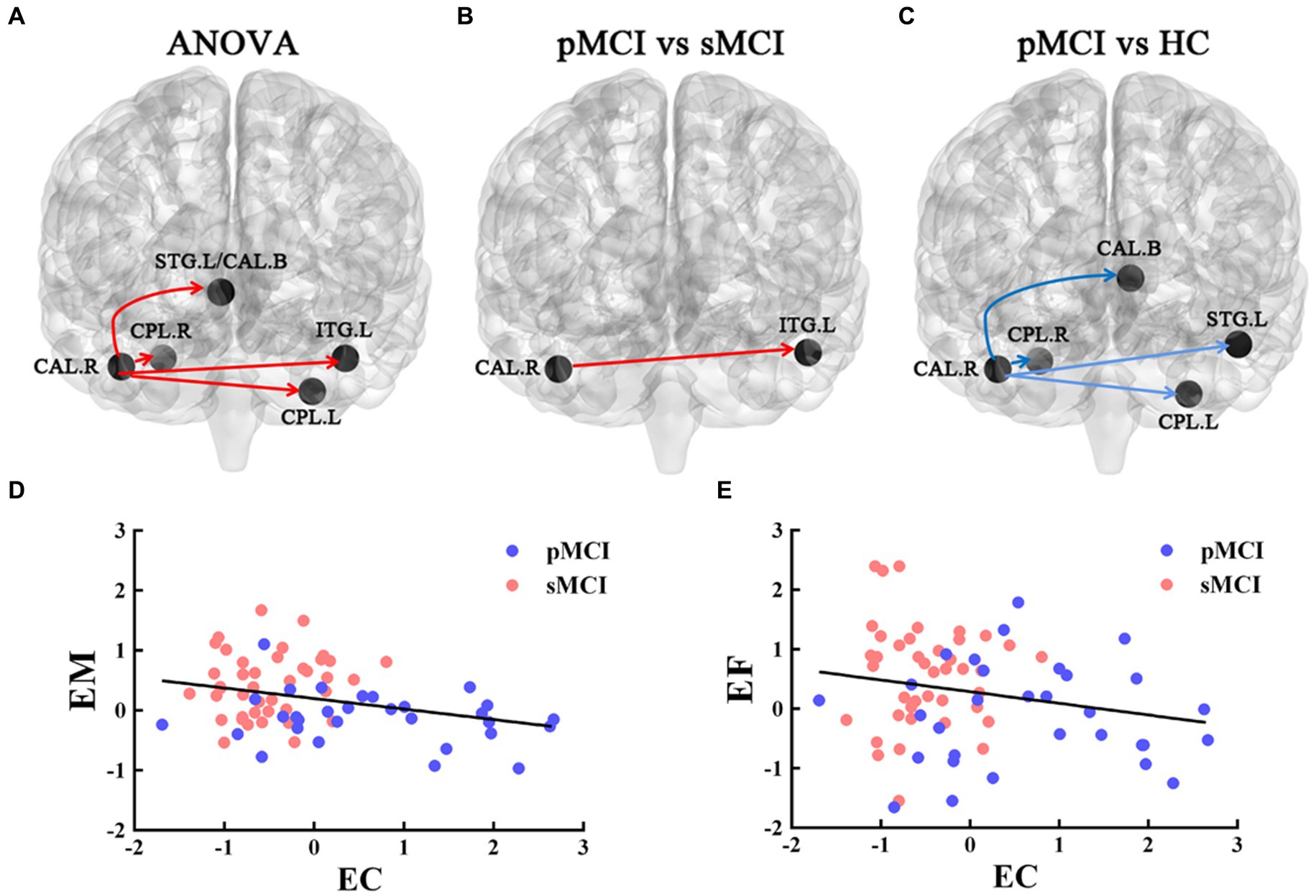
Figure 3. The EC of the right CAL. The red line represents the increased EC from right CAL to the other brain regions; the blue line represents decreased EC from right CAL to the other brain regions. (A) Brain region across three groups, including sMCI, pMCI, and HC (cluster size >100, p < 0.05). (B,C) Results of post-hoc analysis in voxel-wise analysis (TFCE-FWE corrected, cluster size >20, p < 0.05). (D,E) Significant associations between altered EC from right CAL to left ITG and cognitive function. sMCI, stable mild cognitive impairment; pMCI, progressive mild cognitive impairment; HC, healthy control; EC, effective connectivity; CAL, cerebellum anterior lobule; ITG, inferior temporal gyrus; EM, episodic memory; EF, executive function.
Correlation analysis
The EC originating from the right CAL and extending to the left ITG was negatively associated with EM (r = −0.371, p = 0.002) and EF (r = −0.284, p = 0.018; Bonferroni corrected, p < 0.05; Figure 3).
ROC analysis
Most notably, EC from the right CAL to right ITG exhibited the ability to effectively distinguish sMCI from pMCI, with sensitivity, specificity, and accuracy of 0.5806, 0.9512, and 0.828, respectively (Figure 4).
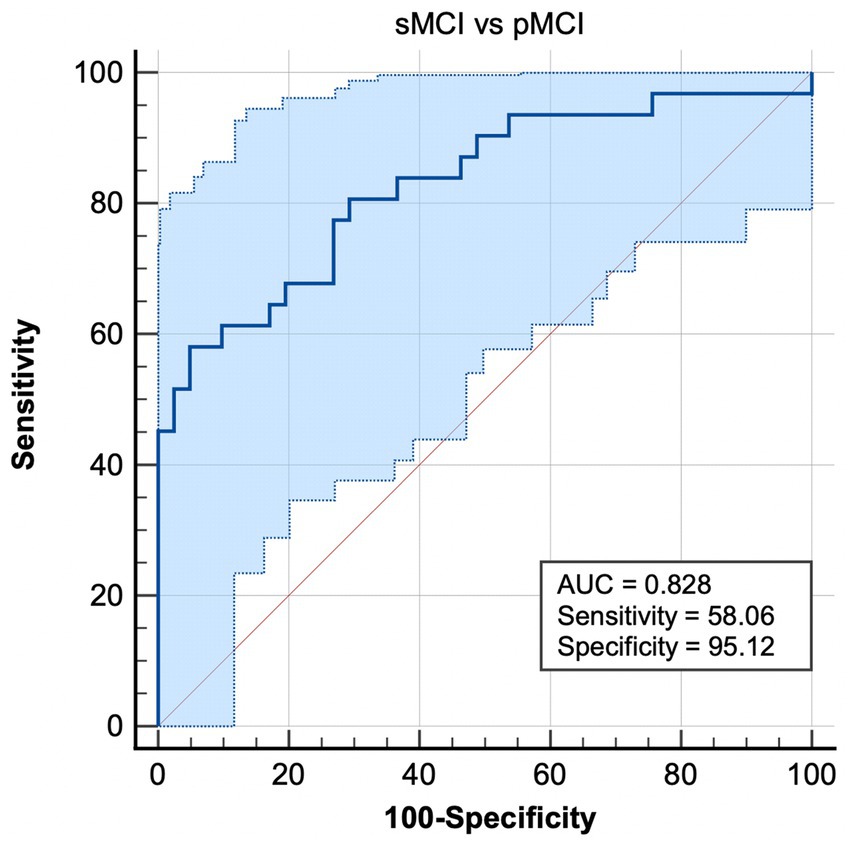
Figure 4. Combination of ROC analysis and EC from right CAL to left ITG differentiated pMCI from sMCI. sMCI, stable mild cognitive impairment; pMCI, progressive mild cognitive impairment; EC, effective connectivity; CAL, cerebellum anterior lobule; ITG, inferior temporal gyrus.
Discussion
To the best of our knowledge, the present study is the first to explore intrinsic brain functional hubs and causal connectivity in individuals with sMCI and pMCI, employing a combination of the DC and GCA methodologies. Using the DC analysis, we identified elevated DC in the left CPL, right CAL, and left ITG, along with reduced DC in the left MFG, in both sMCI and pMCI compared with HCs. Subsequently, the GCA was conducted, leveraging the altered DC values in an ANOVA setup to investigate their causal effect across the whole brain. Specifically, compared with HC participants, pMCI exhibited increased EC originating from the right CAL and extending to the left ITG, a connectivity pattern with a significantly negative association with impairments in EM and EF. Of particular significance, the EC extending from the right CAL to the right ITG demonstrated excellent discriminatory ability between sMCI and pMCI, together with high specificity and accuracy. This study delves into a more profound understanding of the underlying pathological mechanisms characterizing sMCI and pMCI.
The present study showed similar changes in DC values for sMCI and pMCI, shedding light on shared neuroimaging mechanisms between these two conditions. Both groups exhibited increased DC in the left ITG, left CPL, and right CAL, coupled with decreased DC in the left MFG. The coexistence of increased and diminished DC underlines a compensatory mechanism operating in the context of cognitive decline, a phenomenon previously substantiated by existing research (Xue C. et al., 2019; Xue et al., 2021). The left ITG plays a pivotal role in higher-order cognitive functions encompassing visual recognition, language comprehension, decision-making, and emotion regulation (Jackson et al., 2018). Notably, prior research identified the ITG as a hub for intense local β-amyloid/tau interactions, fostering a connectivity profile conducive to accelerated tau propagation (Lee et al., 2022). Moreover, diminished ReHo values in the left ITG in the MCI group were found to be correlated negatively with disease duration (Wu et al., 2022). These findings parallel those of Wang et al., who demonstrated significant alterations in nodal properties (degree and betweenness centrality) within the ITG in AD and MCI, thereby contributing to global topological alterations, consistent with our current study (Wang et al., 2018).
The human cerebellar cortex represents a complex structure characterized by intricate folding, surpassing even the cerebral cortex in this regard (Sereno et al., 2020). Its extensive neural fiber connections with the brain’s cognitive network underscore its pivotal role in shaping behavior and cognition evolution (Sereno et al., 2020). While the cerebellum primarily governs sensorimotor and vestibular functions, it additionally exerts influence over cognitive, emotional, and autonomic domains (Cutando et al., 2022). Notably, age-related declines in cerebellar lobular volumes and cortico-cerebellar FC have been linked to cognitive regression in healthy elderly individuals (Uwisengeyimana et al., 2020; Gellersen et al., 2021). Tang et al. proposed that cortico-cerebellar FC disruptions, particularly within the DMN and fronto-parietal networks, could serve as a novel avenue for early diagnosis and potential early interventions in MCI and AD (Tang et al., 2021). Furthermore, augmented cerebellar activity has been positively correlated with memory enhancement, potentially operating as a compensatory mechanism (McLaren et al., 2012). Hence, heightened connectivity in this context may potentially underpin cerebellar compensatory processes.
The MFG encompasses both caudal and rostral areas, with the latter encompassing portions of the dorsal lateral prefrontal cortex responsible for executive cognitive functions, thinking and perception, memory retrieval, problem-solving, and emotional regulation (Friedman and Robbins, 2022). A meta-analysis confirmed a significant association between reduced gray matter density, cerebral blood flow, hypometabolism in the MFG and increased anosognosia scores in patients with AD (Hallam et al., 2020). Caffarra et al. identified the left anterior cingulate and MFG as mediators of delayed free recall (Caffarra et al., 2016). Furthermore, diminished eigenvector centrality in the MFG was identified in MCI by Lou et al., aligning with the present study’s findings of reduced FC in this region (Lou et al., 2016). Importantly, it becomes evident that the alterations are primarily localized to the DMN, a network pivotal for self-referential psychological processes and social functions (Menon, 2023). It is the most studied network and is thought to be the first network with the risk of damage during AD because it is more susceptible to β-amyloid and tau deposition and glucose hypometabolism, which positions it as an early target in AD pathogenesis (Chiesa et al., 2019; Ingala et al., 2021). Our study corroborates the DMN’s vulnerability as a leading disrupted network in MCI.
Intriguingly, significant differences in DC were not discernible between sMCI and pMCI. However, pronounced distinctions emerged in EC, indicating that EC might offer nuanced neuroimaging insights. Compared with HCs, sMCI displayed a marked EC reduction extending from the right CAL to the bilateral CPL, left STG, and bilateral caudate. The caudate nucleus plays a key role in various higher neural functions, instrumental in executive functions, learning, memory, motivation, and emotion, and it assumes a multifaceted role in higher-order neural functions (Grahn et al., 2008; Yang et al., 2022). The cortical-caudate FC was less distinct in older adults versus younger counterparts, with age-related differences in caudate function associated with memory decline in the context of normal aging (Rieckmann et al., 2018). Task-based fMRI data unveiled notable caudate activity in older adults during virtual navigation tasks, in contrast to hippocampal engagement observed in younger adults, implying an age-related shift in functional demands from the hippocampus to the caudate nucleus during navigation (Konishi et al., 2013). The caudate volume was lower in patients with MCI than in HC participants (Madsen et al., 2010). Gao et al. reported reduced regional homogeneity in the right caudate of patients with MCI relative to HC participants, suggesting that right caudate ReHo can be used as a neuroimaging biomarker of MCI, which can provide objective guidance for the diagnosis and management of MCI in the future (Gao et al., 2022).
Interestingly, no significant difference in EC existed between pMCI and HC, indicating that altered EC could potentially characterize sMCI. Zhou et al. found that sMCI cortical thickness decreased (e.g., ITG and anterior cingulated regions) and node and network efficiency increased, revealing the coexistence of injury and compensation (Zhou and Lui, 2013). The decrease in EC from the right CAL to other regions in sMCI may be a specific network change in sMCI. Notably, pMCI showcased elevated EC extending from the CAL to ITG compared with sMCI. Previous studies underscored compromised FC between the cerebellum and the cerebral cortex (Olivito et al., 2020; Zhou Z. et al., 2021). The results further corroborate the notion that AD/MCI entails disrupted mechanisms influencing synaptic plasticity in long-range interconnections across brain regions (Lou et al., 2016; Sha et al., 2018). Correlation analysis showed that there was a negative correlation between EC from CAL to ITG and EM scores, wherein EC from CAL to ITG increased with the decline of EM, reinforcing the compensatory hypothesis (Gaubert et al., 2019; Bhembre et al., 2023). This phenomenon likely reflects the brain’s endeavor to counteract cognitive decline by augmenting neural activity or connectivity. Of paramount importance, the EC extending from CAL to ITG can effectively discriminate sMCI from pMCI with high specificity and accuracy. Collectively, increased EC originating in the cerebellum and extending to the temporal lobe serves as a promising biomarker for the differentiation and diagnosis of sMCI and pMCI, offering a new perspective for therapeutic target selection.
Limitations
There were three limitations to our study. First, there was a significant difference in years of education across the three groups, which could have potentially confounding our results. However, to mitigate these confounding effects, all statistical analyses were conducted with age, years of education, and gender as covariates. As such, we maintain the credibility of our findings. Secondly, the small sample size of our study may affect the robustness and repeatability of the study. We used strict multiple comparisons to ensure reliability. We will further validate the results when the sample size is expanded in the future. Thirdly, important biomarkers such as APOE-e4 carrier status, Aβ deposition, and tau tangles deposition were not statistically analyzed, which were crucial in the research of the pathological mechanism of MCI. In the following studies, we will explore the value of imaging indicators in predicting MCI disease transformation and pathological features.
Conclusion
In summary, this study uncovers shared and distinctive alterations in DC and EC between sMCI and pMCI, with implications for cognitive function. Importantly, the unidirectional EC disruptions stemming from the cerebellum and extending to the temporal lobe hold considerable potential for effectively distinguishing sMCI from pMCI while also offering novel insights into the neural circuit mechanisms linking the temporal lobe and cerebellum in MCI.
Data availability statement
The datasets presented in this study can be found in online repositories. The names of the repository/repositories and accession number(s) can be found in the article/Supplementary material.
Ethics statement
The studies involving humans were approved by the Institutional Review Committees of all participating institutions. The studies were conducted in accordance with the local legislation and institutional requirements. The participants provided their written informed consent to participate in this study. Written informed consent was obtained from the individual(s) for the publication of any potentially identifiable images or data included in this article.
Author contributions
CX: Data curation, Methodology, Software, Validation, Visualization, Writing – original draft. DZ: Software, Validation, Writing – original draft. YR: Software, Writing – original draft. WG: Software, Writing – original draft. JH: Validation, Writing – original draft, Writing – review & editing.
Group member of the Alzheimer’s Disease Neuroimaging Initiative
Data used in preparation of this article were obtained from the Alzheimer’s Disease Neuroimaging Initiative (ADNI) database (adni.loni.usc.edu). As such, the investigators within the ADNI contributed to the design and implementation of ADNI and/or provided data but did not participate in analysis or writing of this report. A complete listing of ADNI investigators can be found at: http://adni.loni.usc.edu/wp-content/uploads/how_to_apply/ADNI_Acknowledgement_List.pdf.
Funding
The author(s) declare that financial support was received for the research, authorship, and/or publication of this article. Data collection and sharing for this project was funded by the Alzheimer’s Disease Neuroimaging Initiative (ADNI; National Institutes of Health Grant U01 AG024904) and DOD ADNI (Department of Defense award number W81XWH-12-2-0012). ADNI is funded by the National Institute on Aging, the National Institute of Biomedical Imaging and Bioengineering, and through generous contributions from the following: AbbVie; Alzheimer’s Association; Alzheimer’s Drug Discovery Foundation; Araclon Biotech; BioClinica, Inc.; Biogen; Bristol-Myers Squibb Company; CereSpir, Inc.; Cogstate; Eisai Inc.; Elan Pharmaceuticals, Inc.; Eli Lilly and Company; EuroImmun; F. Hoffmann-La Roche Ltd. and its affiliated company Genentech, Inc.; Fujirebio; GE Healthcare; IXICO Ltd.; Janssen Alzheimer Immunotherapy Research & Development, LLC.; Johnson & Johnson Pharmaceutical Research & Development LLC.; Lumosity; Lundbeck; Merck & Co., Inc.; Meso Scale Diagnostics, LLC.; NeuroRx Research; Neurotrack Technologies; Novartis Pharmaceuticals Corporation; Pfizer Inc.; Piramal Imaging; Servier; Takeda Pharmaceutical Company; and Transition Therapeutics. The Canadian Institutes of Health Research is providing funds to support ADNI clinical sites in Canada. Private sector contributions are facilitated by the Foundation for the National Institutes of Health (www.fnih.org). The grantee organization is the Northern California Institute for Research and Education, and the study is coordinated by the Alzheimer’s Therapeutic Research Institute at the University of Southern California. ADNI data are disseminated by the Laboratory for NeuroImaging at the University of Southern California.
Conflict of interest
The authors declare that the research was conducted in the absence of any commercial or financial relationships that could be construed as a potential conflict of interest.
Publisher’s note
All claims expressed in this article are solely those of the authors and do not necessarily represent those of their affiliated organizations, or those of the publisher, the editors and the reviewers. Any product that may be evaluated in this article, or claim that may be made by its manufacturer, is not guaranteed or endorsed by the publisher.
Supplementary material
The Supplementary material for this article can be found online at: https://www.frontiersin.org/articles/10.3389/fnagi.2024.1442721/full#supplementary-material
Footnotes
2. ^ http://adni.loni.usc.edu/data-samples/access-data/
3. ^DPABI, http://rfmri.org/DPABI.
4. ^ http://www.mathworks.com/products/matlab/
5. ^rs-HRF, https://www.nitrc.org/projects/rshrf.
References
Bhembre, N., Bonthron, C., and Opazo, P. (2023). Synaptic compensatory plasticity in Alzheimer’s disease. J. Neurosci. 43, 6833–6840. doi: 10.1523/JNEUROSCI.0379-23.2023
Caffarra, P., Ghetti, C., Ruffini, L., Spallazzi, M., Spotti, A., Barocco, F., et al. (2016). Brain metabolism correlates of the free and cued selective reminding test in mild cognitive impairment. J. Alzheimers Dis. 51, 27–31. doi: 10.3233/JAD-150418
Cai, S., Peng, Y., Chong, T., Zhang, Y., von Deneen, K. M., Huang, L., et al. (2017). Differentiated effective connectivity patterns of the executive control network in progressive MCI: a potential biomarker for predicting AD. Curr. Alzheimer Res. 14, 937–950. doi: 10.2174/1567205014666170309120200
Chen, S., Wu, H., Song, Y., Ge, H., Yan, Z., Xue, C., et al. (2023). Altered structural and functional homotopic connectivity associated with the progression from mild cognitive impairment to Alzheimer’s disease. Psychiatry Res. 319:115000. doi: 10.1016/j.psychres.2022.115000
Chiesa, P. A., Cavedo, E., Vergallo, A., Lista, S., Potier, M. C., Habert, M. O., et al. (2019). Differential default mode network trajectories in asymptomatic individuals at risk for Alzheimer’s disease. Alzheimers Dement. 15, 940–950. doi: 10.1016/j.jalz.2019.03.006
Costumero, V., d'Oleire Uquillas, F., Diez, I., Andorra, M., Basaia, S., Bueicheku, E., et al. (2020). Distance disintegration delineates the brain connectivity failure of Alzheimer’s disease. Neurobiol. Aging 88, 51–60. doi: 10.1016/j.neurobiolaging.2019.12.005
Cutando, L., Puighermanal, E., Castell, L., Tarot, P., Belle, M., Bertaso, F., et al. (2022). Cerebellar dopamine D2 receptors regulate social behaviors. Nat. Neurosci. 25, 900–911. doi: 10.1038/s41593-022-01092-8
Friedman, N. P., and Robbins, T. W. (2022). The role of prefrontal cortex in cognitive control and executive function. Neuropsychopharmacology 47, 72–89. doi: 10.1038/s41386-021-01132-0
Friston, K., Moran, R., and Seth, A. K. (2013). Analysing connectivity with granger causality and dynamic causal modelling. Curr. Opin. Neurobiol. 23, 172–178. doi: 10.1016/j.conb.2012.11.010
Gao, Y., Zhao, X., Huang, J., Wang, S., Chen, X., Li, M., et al. (2022). Abnormal regional homogeneity in right caudate as a potential neuroimaging biomarker for mild cognitive impairment: a resting-state fMRI study and support vector machine analysis. Front. Aging Neurosci. 14:979183. doi: 10.3389/fnagi.2022.979183
Gaubert, S., Raimondo, F., Houot, M., Corsi, M. C., Naccache, L., Diego Sitt, J., et al. (2019). EEG evidence of compensatory mechanisms in preclinical Alzheimer’s disease. Brain 142, 2096–2112. doi: 10.1093/brain/awz150
Gellersen, H. M., Guell, X., and Sami, S. (2021). Differential vulnerability of the cerebellum in healthy ageing and Alzheimer’s disease. Neuroimage Clin. 30:102605. doi: 10.1016/j.nicl.2021.102605
Grahn, J. A., Parkinson, J. A., and Owen, A. M. (2008). The cognitive functions of the caudate nucleus. Prog. Neurobiol. 86, 141–155. doi: 10.1016/j.pneurobio.2008.09.004
Gu, Y., Lin, Y., Huang, L., Ma, J., Zhang, J., Xiao, Y., et al. (2020). Abnormal dynamic functional connectivity in Alzheimer’s disease. CNS Neurosci. Ther. 26, 962–971. doi: 10.1111/cns.13387
Hallam, B., Chan, J., Gonzalez Costafreda, S., Bhome, R., and Huntley, J. (2020). What are the neural correlates of meta-cognition and anosognosia in Alzheimer’s disease? A systematic review. Neurobiol. Aging 94, 250–264. doi: 10.1016/j.neurobiolaging.2020.06.011
Huang, S. Y., Hsu, J. L., Lin, K. J., Liu, H. L., Wey, S. P., Hsiao, I. T., et al. (2018). Characteristic patterns of inter-and intra-hemispheric metabolic connectivity in patients with stable and progressive mild cognitive impairment and Alzheimer’s disease. Sci. Rep. 8:13807. doi: 10.1038/s41598-018-31794-8
Huang, X., Zhang, D., Wang, P., Mao, C., Miao, Z., Liu, C., et al. (2021). Altered amygdala effective connectivity in migraine without aura: evidence from resting-state fMRI with granger causality analysis. J. Headache Pain 22:25. doi: 10.1186/s10194-021-01240-8
Ingala, S., Tomassen, J., Collij, L. E., Prent, N., van 't Ent, D., Ten Kate, M., et al. (2021). Amyloid-driven disruption of default mode network connectivity in cognitively healthy individuals. Brain Commun. 3:fcab201. doi: 10.1093/braincomms/fcab201
Jackson, R. L., Bajada, C. J., Rice, G. E., Cloutman, L. L., and Lambon Ralph, M. A. (2018). An emergent functional parcellation of the temporal cortex. NeuroImage 170, 385–399. doi: 10.1016/j.neuroimage.2017.04.024
Jessen, F., Amariglio, R. E., van Boxtel, M., Breteler, M., Ceccaldi, M., Chetelat, G., et al. (2014a). A conceptual framework for research on subjective cognitive decline in preclinical Alzheimer’s disease. Alzheimers Dement. 10, 844–852. doi: 10.1016/j.jalz.2014.01.001
Jessen, F., Wolfsgruber, S., Wiese, B., Bickel, H., Mosch, E., Kaduszkiewicz, H., et al. (2014b). AD dementia risk in late MCI, in early MCI, and in subjective memory impairment. Alzheimers Dement. 10, 76–83. doi: 10.1016/j.jalz.2012.09.017
Konishi, K., Etchamendy, N., Roy, S., Marighetto, A., Rajah, N., and Bohbot, V. D. (2013). Decreased functional magnetic resonance imaging activity in the hippocampus in favor of the caudate nucleus in older adults tested in a virtual navigation task. Hippocampus 23, 1005–1014. doi: 10.1002/hipo.22181
Lee, W. J., Brown, J. A., Kim, H. R., La Joie, R., Cho, H., Lyoo, C. H., et al. (2022). Regional Abeta-tau interactions promote onset and acceleration of Alzheimer’s disease tau spreading. Neuron 110, 1932–1943.e5. doi: 10.1016/j.neuron.2022.03.034
Liao, W., Mantini, D., Zhang, Z., Pan, Z., Ding, J., Gong, Q., et al. (2010). Evaluating the effective connectivity of resting state networks using conditional granger causality. Biol. Cybern. 102, 57–69. doi: 10.1007/s00422-009-0350-5
Lou, Y., Huang, P., Li, D., Cen, Z., Wang, B., Gao, J., et al. (2015). Altered brain network centrality in depressed Parkinson’s disease patients. Mov. Disord. 30, 1777–1784. doi: 10.1002/mds.26321
Lou, W., Shi, L., Wong, A., Chu, W. C., Mok, V. C., and Wang, D. (2016). Changes of cerebral perfusion and functional brain network organization in patients with mild cognitive impairment. J. Alzheimers Dis. 54, 397–409. doi: 10.3233/JAD-160201
Madsen, S. K., Ho, A. J., Hua, X., Saharan, P. S., Toga, A. W., Jack, C. R. Jr., et al. (2010). 3D maps localize caudate nucleus atrophy in 400 Alzheimer’s disease, mild cognitive impairment, and healthy elderly subjects. Neurobiol. Aging 31, 1312–1325. doi: 10.1016/j.neurobiolaging.2010.05.002
Malotaux, V., Dricot, L., Quenon, L., Lhommel, R., Ivanoiu, A., and Hanseeuw, B. (2023). Default-mode network connectivity changes during the progression toward Alzheimer’s dementia: a longitudinal functional magnetic resonance imaging study. Brain Connect. 13, 287–296. doi: 10.1089/brain.2022.0008
McLaren, D. G., Sreenivasan, A., Diamond, E. L., Mitchell, M. B., Van Dijk, K. R., Deluca, A. N., et al. (2012). Tracking cognitive change over 24 weeks with longitudinal functional magnetic resonance imaging in Alzheimer’s disease. Neurodegener. Dis. 9, 176–186. doi: 10.1159/000335876
Menon, V. (2023). 20 years of the default mode network: a review and synthesis. Neuron 111, 2469–2487. doi: 10.1016/j.neuron.2023.04.023
Mitchell, A. J., and Shiri-Feshki, M. (2009). Rate of progression of mild cognitive impairment to dementia--meta-analysis of 41 robust inception cohort studies. Acta Psychiatr. Scand. 119, 252–265. doi: 10.1111/j.1600-0447.2008.01326.x
Olivito, G., Serra, L., Marra, C., Di Domenico, C., Caltagirone, C., Toniolo, S., et al. (2020). Cerebellar dentate nucleus functional connectivity with cerebral cortex in Alzheimer’s disease and memory: a seed-based approach. Neurobiol. Aging 89, 32–40. doi: 10.1016/j.neurobiolaging.2019.10.026
Pereira, J. B., Mijalkov, M., Kakaei, E., Mecocci, P., Vellas, B., Tsolaki, M., et al. (2016). Disrupted network topology in patients with stable and progressive mild cognitive impairment and Alzheimer’s disease. Cereb. Cortex 26, 3476–3493. doi: 10.1093/cercor/bhw128
Petersen, R. C., Caracciolo, B., Brayne, C., Gauthier, S., Jelic, V., and Fratiglioni, L. (2014). Mild cognitive impairment: a concept in evolution. J. Intern. Med. 275, 214–228. doi: 10.1111/joim.12190
Rieckmann, A., Johnson, K. A., Sperling, R. A., Buckner, R. L., and Hedden, T. (2018). Dedifferentiation of caudate functional connectivity and striatal dopamine transporter density predict memory change in normal aging. Proc. Natl. Acad. Sci. USA 115, 10160–10165. doi: 10.1073/pnas.1804641115
Sereno, M. I., Diedrichsen, J., Tachrount, M., Testa-Silva, G., d'Arceuil, H., and De Zeeuw, C. (2020). The human cerebellum has almost 80% of the surface area of the neocortex. Proc. Natl. Acad. Sci. USA 117, 19538–19543. doi: 10.1073/pnas.2002896117
Sha, Z., Xia, M., Lin, Q., Cao, M., Tang, Y., Xu, K., et al. (2018). Meta-connectomic analysis reveals commonly disrupted functional architectures in network modules and connectors across brain disorders. Cereb. Cortex 28, 4179–4194. doi: 10.1093/cercor/bhx273
Shan, A., Zhang, H., Gao, M., Wang, L., Cao, X., Gan, C., et al. (2023). Aberrant voxel-based degree centrality and functional connectivity in Parkinson’s disease patients with fatigue. CNS Neurosci. Ther. 29, 2680–2689. doi: 10.1111/cns.14212
Tang, F., Zhu, D., Ma, W., Yao, Q., Li, Q., and Shi, J. (2021). Differences changes in cerebellar functional connectivity between mild cognitive impairment and Alzheimer’s disease: a seed-based approach. Front. Neurol. 12:645171. doi: 10.3389/fneur.2021.645171
Thung, K. H., Wee, C. Y., Yap, P. T., and Shen, D. (2016). Identification of progressive mild cognitive impairment patients using incomplete longitudinal MRI scans. Brain Struct. Funct. 221, 3979–3995. doi: 10.1007/s00429-015-1140-6
Uwisengeyimana, J. D., Nguchu, B. A., Wang, Y., Zhang, D., Liu, Y., Qiu, B., et al. (2020). Cognitive function and cerebellar morphometric changes relate to abnormal intra-cerebellar and cerebro-cerebellum functional connectivity in old adults. Exp. Gerontol. 140:111060. doi: 10.1016/j.exger.2020.111060
Wang, K., Liang, M., Wang, L., Tian, L., Zhang, X., Li, K., et al. (2007). Altered functional connectivity in early Alzheimer’s disease: a resting-state fMRI study. Hum. Brain Mapp. 28, 967–978. doi: 10.1002/hbm.20324
Wang, M., Liao, Z., Mao, D., Zhang, Q., Li, Y., Yu, E., et al. (2017). Application of granger causality analysis of the directed functional connection in Alzheimer’s disease and mild cognitive impairment. J. Vis. Exp. 126:56015. doi: 10.3791/56015
Wang, B., Miao, L., Niu, Y., Cao, R., Li, D., Yan, P., et al. (2018). Abnormal functional brain networks in mild cognitive impairment and Alzheimer’s disease: a minimum spanning tree analysis. J. Alzheimers Dis. 65, 1093–1107. doi: 10.3233/JAD-180603
Wu, Y. Q., Wang, Y. N., Zhang, L. J., Liu, L. Q., Pan, Y. C., Su, T., et al. (2022). Regional homogeneity in patients with mild cognitive impairment: a resting-state functional magnetic resonance imaging study. Front. Aging Neurosci. 14:877281. doi: 10.3389/fnagi.2022.877281
Xue, J., Guo, H., Gao, Y., Wang, X., Cui, H., Chen, Z., et al. (2019). Altered directed functional connectivity of the Hippocampus in mild cognitive impairment and Alzheimer’s disease: a resting-state fMRI study. Front. Aging Neurosci. 11:326. doi: 10.3389/fnagi.2019.00326
Xue, C., Sun, H., Hu, G., Qi, W., Yue, Y., Rao, J., et al. (2020). Disrupted patterns of rich-club and diverse-club organizations in subjective cognitive decline and amnestic mild cognitive impairment. Front. Neurosci. 14:575652. doi: 10.3389/fnins.2020.575652
Xue, C., Sun, H., Yue, Y., Wang, S., Qi, W., Hu, G., et al. (2021). Structural and functional disruption of salience network in distinguishing subjective cognitive decline and amnestic mild cognitive impairment. ACS Chem. Neurosci. 12, 1384–1394. doi: 10.1021/acschemneuro.1c00051
Xue, C., Yuan, B., Yue, Y., Xu, J., Wang, S., Wu, M., et al. (2019). Distinct disruptive patterns of default mode subnetwork connectivity across the spectrum of preclinical Alzheimer’s disease. Front. Aging Neurosci. 11:307. doi: 10.3389/fnagi.2019.00307
Yang, Z., Caldwell, J. Z. K., Cummings, J. L., Ritter, A., Kinney, J. W., Cordes, D., et al. (2022). Sex modulates the pathological aging effect on caudate functional connectivity in mild cognitive impairment. Front. Psych. 13:804168. doi: 10.3389/fpsyt.2022.804168
Yu, E., Liao, Z., Tan, Y., Qiu, Y., Zhu, J., Han, Z., et al. (2019). High-sensitivity neuroimaging biomarkers for the identification of amnestic mild cognitive impairment based on resting-state fMRI and a triple network model. Brain Imaging Behav. 13, 1–14. doi: 10.1007/s11682-017-9727-6
Zhou, J., Li, K., Luo, X., Zeng, Q., Jiaerken, Y., Wang, S., et al. (2021). Distinct impaired patterns of intrinsic functional network centrality in patients with early-and late-onset Alzheimer’s disease. Brain Imaging Behav. 15, 2661–2670. doi: 10.1007/s11682-021-00470-3
Zhou, Y., and Lui, Y. W. (2013). Small-world properties in mild cognitive impairment and early Alzheimer’s disease: a cortical thickness MRI study. ISRN Geriatr. 2013, 1–11. doi: 10.1155/2013/542080
Zhou, Z., Zhu, R., Shao, W., Zhang, S. J., Wang, L., Ding, X. J., et al. (2021). Changes in resting-state functional connectivity of cerebellum in amnestic mild cognitive impairment and Alzheimer’s disease: a case-control study. Front. Syst. Neurosci. 15:596221. doi: 10.3389/fnsys.2021.596221
Zuo, X. N., Ehmke, R., Mennes, M., Imperati, D., Castellanos, F. X., Sporns, O., et al. (2012). Network centrality in the human functional connectome. Cereb. Cortex 22, 1862–1875. doi: 10.1093/cercor/bhr269
Keywords: stable mild cognitive impairment, progressive mild cognitive impairment, degree centrality, directed functional connectivity, resting-state functional MRI
Citation: Xue C, Zheng D, Ruan Y, Guo W and Hu J (2024) Alteration in temporal-cerebellar effective connectivity can effectively distinguish stable and progressive mild cognitive impairment. Front. Aging Neurosci. 16:1442721. doi: 10.3389/fnagi.2024.1442721
Edited by:
Ana Lloret, University of Valencia, SpainReviewed by:
Xinglong Yang, The First Affiliated Hospital of Kunming Medical University, ChinaYongxia Zhou, University of Southern California, United States
Copyright © 2024 Xue, Zheng, Ruan, Guo and Hu. This is an open-access article distributed under the terms of the Creative Commons Attribution License (CC BY). The use, distribution or reproduction in other forums is permitted, provided the original author(s) and the copyright owner(s) are credited and that the original publication in this journal is cited, in accordance with accepted academic practice. No use, distribution or reproduction is permitted which does not comply with these terms.
*Correspondence: Jun Hu, bmpua3l5aHVqdW5AMTYzLmNvbQ==