- 1Department of Neurology, The Second Xiangya Hospital, Central South University, Changsha, China
- 2Department of Medical Genetics, The Second Xiangya Hospital, Central South University, Changsha, China
- 3Key Laboratory of Hunan Province in Neurodegenerative Disorders, Central South University, Changsha, China
- 4Department of Geriatric Surgery, The Second Xiangya Hospital, Central South University, Changsha, China
Background: Observational studies have shown that oxidative stress (OS) is associated with Parkinson’s disease (PD). However, whether such observations reflect cause–effect remains largely unknown. To test this, we performed a two-sample bidirectional Mendelian randomization (MR) analysis to investigate the causal-effects between OS biomarkers and PD.
Methods: We selected summary statistics data for single-nucleotide polymorphisms (SNPs) associated with catalase (n = 13), glutathione peroxidases (n = 12), superoxide dismutase (n = 13), vitamin A (n = 7), vitamin C (n = 10), vitamin E (n = 12), vitamin B12 (n = 8), folate (n = 14), copper (n = 6), Zinc (n = 7), and iron (n = 23) levels, and the corresponding data for PD from the International Parkinson Disease Genomics Consortium (IPDGC, 33,674 cases and 449,056 controls). Inverse-variance weighted (IVW) MR analyses were conducted to estimate associations of OS with PD. Reverse MR analysis was further performed to predict the causal effects of PD on the above OS biomarkers.
Results: As for PD, the IVW method suggested that the Zinc (Zn) levels was significantly associated with PD (OR = 1.107, 95% CI 1.013–1.211; p = 0.025), which is consistent with results from the weighted median analyses. Moreover, the results remained consistent and robust in the sensitivity analysis. However, there were no significant associations of catalase, glutathione peroxidases, superoxide dismutase, vitamin A, vitamin C, vitamin E, vitamin B12, folate, copper, or iron with PD. As for OS, our reverse MR analysis also did not support a causal effect of liability to PD on OS.
Conclusion: The MR study supported the causal effect of Zn on PD. These findings may inform prevention strategies and interventions directed toward OS and PD.
1 Introduction
Parkinson’s disease (PD), the most common neurodegenerative disease worldwide, is characterized by a progressive loss of substantia nigra (SN) dopaminergic neurons and accumulation of α-synuclein in the SN (Poewe et al., 2017). The main clinical manifestations of PD are motor symptoms (i.e., resting tremor, bradykinesia, rigidity, and postural instability) and nonmotor symptoms (i.e., depression, constipation, olfactory deficits, and cognitive impairment), which seriously affect the quality of life of patients and the economic burden of their family (Kalia and Lang, 2015). At present, the etiology and mechanism of PD remain elusive (Kalia and Lang, 2015). Consequently, the treatment for PD is primarily symptomatic, focusing on alleviating symptoms rather than slowing or halting disease progression (Bloem et al., 2021). Notably, with the discovery and studies of PD-related pathogenic genes (Blauwendraat et al., 2020), i.e., SNCA, PARKIN, PINK1, LRRK2, and DJ-1…, it has been revealed that oxidative stress (OS) is considered a key modulator in the occurrence and development of PD (Maiti et al., 2017). Mechanistically, OS refers to the overproduction of reactive oxygen species (ROS) and insufficient endogenous antioxidants, ultimately leading to cell dysfunction and death (Khan and Ali, 2018).
Substantial observational and experimental studies suggest that altered OS homeostasis may be involved in the etiology and pathogenesis of PD (Puspita et al., 2017; Chang and Chen, 2020; Jimenez-Moreno and Lane, 2020). It has been established that low levels of antioxidants, incapable of controlling ROS production, lead to neurodegeneration in PD (Medeiros et al., 2016). Oxidized lipids, proteins, and DNA can be seen in the substantia nigra of PD patients, which are all evidence of OS involvement (Abdelhamid and Nagano, 2023). LRRK2 mutant IPSC-derived DA neurons showed increased expression of genes involved in OS regulation and increased susceptibility to OS (Nguyen et al., 2011). Notably, studies have found that the concentration of oxidized proteins in the SN of healthy individuals is twice that of the caudate nucleus, putamen nucleus, and frontal cortex, suggesting a susceptibility of the SN to OS (Floor and Wetzel, 1998). These results suggest that OS plays an important role in PD.
Therefore, it is speculated that antioxidant treatment to regulate OS may be a strategy to treat PD. Although many antioxidants, such as vitamin E, vitamin C, and desferrioxamine, have been tested in clinical trials, none of them have been convincingly shown to improve neurodegeneration in PD patients (Fahn, 1992; Group, 1993, 1998; Devos et al., 2014). OS can be measured by the biomarkers of OS. Natural antioxidants are a very large diversified family of molecules classified by activity (enzymatic or nonenzymatic), and chemical structure (e.g., vitamins, trace elements, etc.). Catalase (CAT), glutathione peroxidases (GPx), superoxide dismutase (SOD), vitamin A, vitamin C, vitamin E, vitamin B12, folate, copper (Cu), zinc (Zn), and iron (Fe) are important antioxidants in the body. Impairments in antioxidant enzymes or non-enzymatic antioxidant networks, along with imbalance of redox-active metals, can induce the formation of toxic hydroxyl radicals and increase OS, leading to protein oxidation, misfolding, and ultimately cell death in PD (Umeno et al., 2017; Ben-Shushan and Miller, 2021). Furthermore, studies have also proven that many of the abovementioned antioxidants are altered in PD patients (Pichler et al., 2013; Medeiros et al., 2016; Anandhan et al., 2017), but not all studies agree with this view (Vinish et al., 2011). Moreover, it remains controversial as to whether such an OS injury is a cause or a downstream effect of PD. Observational research faces challenges in establishing causality regarding the causal relationship between OS and PD.
Mendelian randomization (MR) overcomes such confounders of observational studies by using genetic variants (SNPs) as instrumental variables (IVs) to infer the causal effect of an exposure on an outcome. MR can also reduce bias from reverse causation, because genetic phenotypes are postnatally unchanged through a lifetime. Therefore, in this study, we aimed to assess the bidirectional causal relationship between PD and OS. Understanding the role of OS in PD can lead to the development of targeted therapies aimed at mitigating its impact and potentially slowing down the progression of the disease.
2 Methods
2.1 Study design
In this study, the bidirectional causal relationship between OS-related biomarkers and the risk of PD was explored using a bidirectional MR design. In the present research, we only extracted summarized data from the consortia. Ethical approval was not required because the study was based on existing publications and public databases. Figure 1 presents the MR analysis flow using the TwoSampleMR R package. To distinguish between a true negative result and a lack of validity of the MR studies, multiple sensitivity analyses were applied to ensure that the three MR assumptions were satisfied: (1) Relevance: The chosen genetic variants are associated with the exposure of interest. (2) Independence: The genetic variants are independent of confounding factors that might influence the exposure-outcome relationship. (3) Exclusivity: The genetic variants affect the outcome solely through their influence on the exposure, not through any alternative pathways.
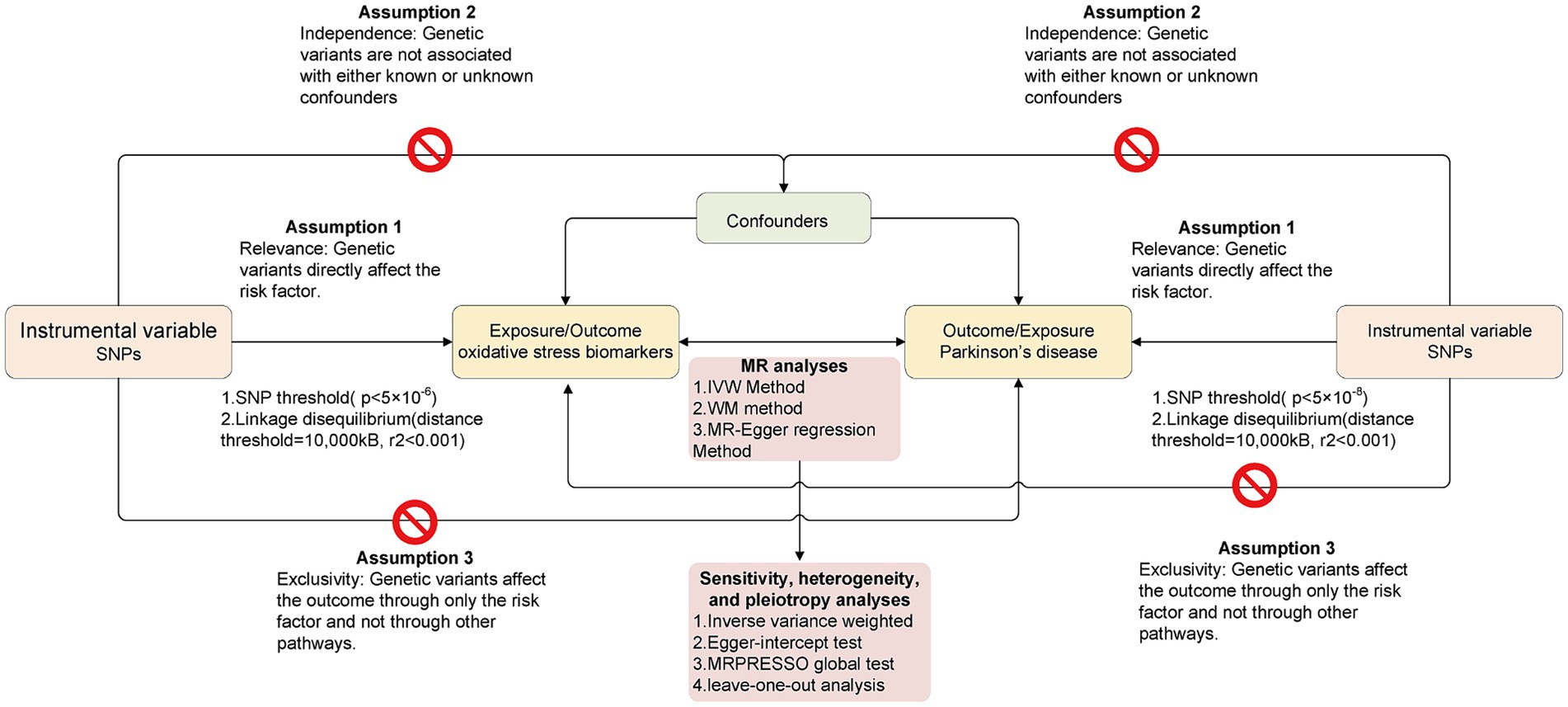
Figure 1. The overall flow chart of bidirectional MR study. The “” means that genetic variants are not associated with confounders or cannot be directly involved in outcome but via the exposure pathway.
2.2 GWAS data sources
We retrieved the genetic data of PD and OS biomarkers from the online platform of the Integrative Epidemiology Unit (IEU) open genome-wide association study (GWAS) project (https://gwas.mrcieu.ac.uk/, accessed on 20 March 2023). In this study, 11 biomarkers of OS were included, namely, CAT (Sun et al., 2018), G-Px (Sun et al., 2018), SOD (Sun et al., 2018), vitamin A (data were from the United Kingdom Biobank), vitamin C (Shin et al., 2014), vitamin E (data was from the United Kingdom Biobank), vitamin B12 (data were from the United Kingdom Biobank), folate (data were from the United Kingdom Biobank), Cu (Evans et al., 2013), Zn (Evans et al., 2013), and Fe (data were from the United Kingdom Biobank and Benyamin et al., 2014). The summary data of PD were obtained from a GWAS including 33,674 PD cases and 449,056 controls of the International Parkinson Disease Genomics Consortium (IPDGC) (Nalls et al., 2019). As mentioned, only the European population summarized data were adopted to avoid population heterogeneity. All datasets are publicly available online upon request. Detailed information on the GWAS datasets is described in Table 1.
2.3 Mendelian randomization
2.3.1 Selection of IVs
We used the default settings in the R package TwoSampleMR, and extracted SNPs showing a significant association with each of the OS biomarkers at the conventional GWAS threshold (p < 5 × 10−6) for lack of significant SNPs. Similarly, IVs of PD were genome-wide significant SNPs (p < 5 × 10−8). To ensure the independence of IVs, all SNPs were clumped with a 10,000-kB window to a threshold of r2 < 0.001 to ascertain independence between genetic variants. Then, the proportion of variance of the exposures explained by the SNPs (R2) and F-statistics were calculated to estimate the strength of IVs to satisfy the first MR assumption (F > 10 for MR analyses; Palmer et al., 2011). The same approach was taken for the reverse MR; as such, we performed 22 bidirectional MR studies, where OS biomarkers and PD were regarded either as exposure or as the outcome.
2.3.2 Mendelian randomization analyses
After clumping IVs, we first performed Steiger filtering to exclude SNPs explaining more variance in the outcome than in the exposure. Next, we harmonized the exposure and outcome data to produce data for MR analysis. In the main analysis, the inverse-variance weighted (IVW) method with random–effects was applied to combine the effect of different IVs. The IVW method is a widely used approach in MR because it provides a straightforward and interpretable summary estimate of the causal effect while leveraging genetic information. The odds ratio (OR) and 95% confidence intervals (CIs) were calculated for each SNP using IVW to assess the risk of exposure to outcome. In addition, we also performed the simple median method, weighted median (WM) method and MR–Egger regression method. The WM method is a median of the weighted estimates and provides a consistent effect even if 50% of IVs are pleiotropic. This means that even if a substantial proportion (up to 50%) of the IVs used in the analysis are pleiotropic, the WM method can still provide consistent and unbiased causal effect estimates. The MR–Egger regression method is used to detect possible violations of instrumental variable assumptions due to directional pleiotropy. It does this by allowing for an intercept term in the regression model, which accounts for any bias introduced by pleiotropic effects. Additionally, the Mendelian Randomization Pleiotropy RESidual Sum and Outlier (MRPRESSO) global test is used to detect outlier SNPs that may be biasing estimates through horizontal pleiotropy (i.e., SNPs with p < 0.05) and adjust for these. For heterogeneity, we applied Cochran’s Q statistic in the inverse variance weighting (IVW) and MR Egger regression methods. Finally, a leave-one-out analysis (LOO) was performed to detect whether any single SNP was disproportionately responsible for the result of each MR study. The results of the present study are shown as ORs (95% CIs) per genetically predicted increase in each lifestyle factor. For result visualization, we constructed scatter plots, forest diagrams, and funnel diagrams using the TwoSampleMR package and MRPRESSO package in the statistical program R. A p value less than 0.05 was considered statistically significant evidence of a causal association.
3 Results
3.1 Instrumental variables for Mendelian randomization
The number of IVs and the phenotypic variances they accounted for by the IVs are shown in Table 2. All of the variables were associated with OS at genome-wide significance. The variance in the exposures explained by their respective set of SNPs ranged from 0.2 to 20.1% (Tables 2, 3). Other than vitamin C, all instruments had an F-statistics of >21, which is above the standard cut-of (> 10) indicating sufficient instrumental strength (Bowden et al., 2018; Burgess et al., 2019).
3.2 Causal effect of oxidative stress biomarkers on PD
We investigated the association of OS with PD. The IVW method provided the results about the causal relationship between PD and 11 oxidative stress biomarkers. Figure 2 presents the forest plots of the associations between Zn and PD. The IVW method shows that genetically determined Zn was strongly associated with PD (OR = 1.107, 95% CI 1.013–1.211; p = 0.025), which is consistent with results from the WM method (OR = 1.136, 95% CI 1.003–1.286; p = 0.044) (Table 2; Figure 2). However, there were no significant associations of catalase, glutathione peroxidases, superoxide dismutase, vitamin A, vitamin C, vitamin E, vitamin B12, folate, copper, or iron with PD (Table 2).
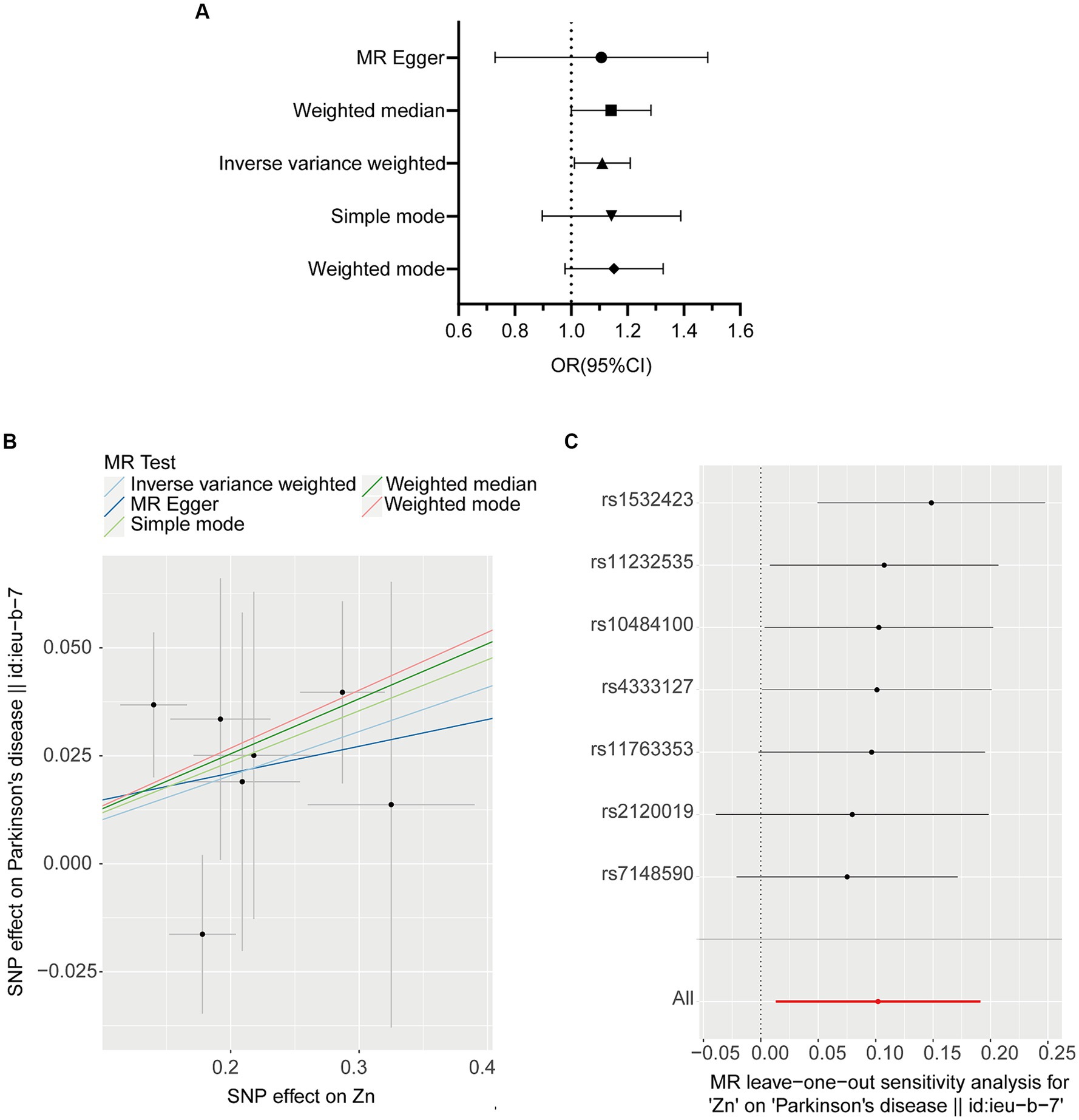
Figure 2. MR plots for the relationship of Zinc (N = 2,603) with PD (N = 482,730). (A) Forest plot of individual and combined SNP MR-estimated effect sizes. The effect estimates represent the odds that each S.D. increase in PD incidence increases odds of appendicular lean mass, and the error bars represent 95% CIs. (B) Scatter plot of SNP effects on Zinc vs. PD, with the slope of each line corresponding to the estimated MR effect per method. The x-axis represents the genetic association with Zinc risk; the y-axis represents the genetic association with the risk of PD. The data are expressed as raw β values with 95% Cis. (C) Leave one out plot, which detect outlier SNPs in Zinc and PD.
3.3 Sensitivity analysis
No heterogeneity was detected by MR–Egger and IVW tests (Supplementary Table S1). Additionally, no evidence of between-SNP directional and horizontal pleiotropy was detected by the MR-PRESSO global test and MR–Egger regression (Supplementary Table S1). We further performed a leave-one-out analysis to detect whether the causal estimate was driven by any single SNP, which revealed a consistent inverse association between genetically predicted Zn levels and the risk of PD (Figure 2C).
3.4 Causal effect of PD on oxidative stress biomarkers
Additionally, reverse MR analysis was conducted to investigate the potential causal effects of PD on 11 OS biomarkers. In these studies, we used 10–22 PD-associated SNPs, which explained 0.075–0.201 of the variances in PD risk and had an F-statistic of more than 3,650. The IVW test results showed no statistically significant associations of PD with OS. The null findings were supported by other MR methods (Table 3). The Egger intercept did not identify any pleiotropic SNP (p of Egger intercept from 0.327 to 0.930). Moreover, the MR-PRESSO global test did not identify outlier SNPs. Significant heterogeneity was apparent in our IVs for PD (only when Vitamin E and Vitamin B12 is the outcome) (IVW and MR Egger, Q pvalue < 0.1) (Supplementary Table S2). Similarly, there was no apparent sign of significant heterogeneity as assessed by leave-one-out analysis.
4 Discussion
This study represents the inaugural bidirectional investigation into the potential involvement of OS in PD. Our research delves into the causal connections between 11 OS biomarkers and the risk of PD, offering fresh perspectives on the correlation between OS status and PD. Notably, among the 11 OS biomarkers studied, we discovered genetic evidence supporting the causal link between Zn and PD. Our findings exhibited considerable robustness across various MR methods, each built upon different assumptions regarding horizontal pleiotropy. These findings may inform prevention strategies and interventions directed toward OS and PD.
Although many observational studies have highlighted the link between OS and PD, there have been few relevant MR studies. These MR studies usually focus on iron. Only one one-way MR study that examined causality between serum iron levels and PD suggested a causal relationship between elevated iron levels (instrumental variables, HFE rs1800562, HFE rs1799945, and TMPRSS6 rs855791) and a reduced risk of PD (OR 0.88, 95% CI 0.82–0.95; p = 0.001; Pichler et al., 2013). However, in our study, we did not find a causal relationship between iron levels and PD.
For other trace elements, suggestive causal associations were found between Zn and PD risk in this work (OR = 1.107, CI = 1.013–1.211, p = 0.025). In our previous study, they showed that alterations in zinc homeostasis have long been implicated in PD. Zinc exposure has been identified as an environmental risk factor for PD, and PD patients were clearly shown to have higher zinc exposure than those without PD (OR = 11.6, 95% CI: 1.51–90.90) (Pals et al., 2003). In addition, postmortem of idiopathic PD patients and PD mouse models showed Zn2+ depositions in dopaminergic neurons (Wang et al., 2023). Zn2+ chelation attenuated the loss of nigrostriatal dopaminergic neurons and the associated motor deficits induced by 6-OHDA or paraquat. The evidence suggests that endogenous Zn2+ plays a key role in the pathophysiology of PD. Zinc homeostasis (balance) is important for OS. On the one hand, Zn2+ can influence the activity of antioxidant enzymes and signaling pathways. On the other hand, overload of intracellular Zn2+ can lead to OS (Sikora and Ouagazzal, 2021). However, previous studies have found that lower serum and plasma zinc levels are associated with a higher risk of PD (Finkelstein et al., 2013; Du et al., 2017), which contradicts our findings. This discrepancy may stem from differences in study design and population sample sizes. Our study utilizes a robust genetic approach, distinct from the observational and experimental designs employed in other research. Genetic evidence offers a more direct assessment of causal relationships, thereby reducing potential confounding factors and biases. Additionally, variations in the populations studied, including differences in genetic backgrounds, environmental exposures, and dietary habits, might influence the results. Further research is needed to elucidate the specific mechanisms by which Zn affects PD and to identify potential therapeutic targets for intervention. Cu2+ is another common redox-active metal in addition to Zn and iron, but in this study, the causal effect of Cu2+ on PD was not significant. Redox-active metals are common cofactors that promote amyloid aggregation in neurodegenerative diseases, such as PD (Ben-Shushan and Miller, 2021). Overall, we speculate that redox-active metals do not entirely mediate the occurrence of PD through OS.
Enzymatic antioxidants, such as GPx, CAT, and SOD, are a critical component of the body’s defense mechanisms against OS (Emamzadeh and Surguchov, 2018). It could inhibit the formation of peroxide and remove free radicals. These enzymatic antioxidants work in concert to maintain the delicate balance between ROS production and neutralization, helping to prevent oxidative damage and maintain cellular health. Studies found that there were lower levels of antioxidant activity of SOD, CAT, and GPx in the PD group compared to controls (Khan and Ali, 2018; Duarte-Jurado et al., 2021). For example, decreased levels of GPx in the substantia nigra pars compacta (SNpc) are one of the earliest biochemical changes observed in PD (Pearce et al., 1997; Tobón-Velasco et al., 2010; Smeyne and Smeyne, 2013). GPx is an important antioxidant enzyme that helps protect cells from oxidative stress by neutralizing harmful ROS and peroxides. One of the biochemical alterations that has been detected in the postmortem brains of PD patients is the selective GPx reduction in the SNpc. In vivo and in vitro experiments have shown that replenishing intracellular GPx levels can prevent oxidative damage and maintain mitochondrial function in dopaminergic cells (Smeyne and Smeyne, 2013; Duarte-Jurado et al., 2021). However, these findings cannot resolve the previous debate on whether these enzymatic antioxidants are the cause of PD or a consequence of it. To date, there are no other MR studies that have assessed the causal association of enzymatic antioxidants and PD risk. In our MR study, it appeared likely that there is no causality between enzymatic antioxidants and PD risk.
Several studies have identified an association of nonenzymatic antioxidants, vitamins (vitamin A, E, C, B12, and folate) with PD (Hughes et al., 2016). Low plasma levels of exogenous antioxidants (including vitamin C) have been reported in patients with neurodegenerative diseases such as PD. In addition, a higher prevalence of PD with subclinical vitamin C deficiencies has also been reported (Duarte-Jurado et al., 2021). It has been found that vitamin E protects dopaminergic neurons against MPTP-mediated toxicity. A cross-sectional study reported that vitamin E status was inversely associated with PD risk (Miyake et al., 2011). However, our results do not support the hypothesis that vitamins substantially affect the risk of PD, which is in line with other case–control studies (Logroscino et al., 1996; Scheider et al., 1997; Powers et al., 2003). Furthermore, when antioxidant vitamins were tested in clinical trials, their effects were not reproduced. A prospective cohort study found that the intake of antioxidant vitamins does not reduce the risk of PD (Hughes et al., 2016). Our updated results suggest that causality causation is unlikely. Therefore, these results support the hypothesis that antioxidant supplementation is unlikely to be of clinical benefit in the prevention of PD. It is important to note that our null findings do not invalidate the role of oxidative stress in PD. Larger epidemiological studies and directed laboratory studies are needed to determine the biochemical and chemical biological bases for these associations.
The advantages of the present study include the following aspects. First, 11 OS biomarkers were included, which made it the most comprehensive MR study between PD and the OS system. Second, we used several essential methods to verify the accuracy of the results, such as calculating the F statistic and power, and conducting heterogeneity and pleiotropy tests. There were some limitations as well. First, in order to extract more SNPs showing a significant association with OS biomarkers to maintain the study power in MR, the threshold of the p value was set as p < 5 × 10−6, meaning that the proportion of variance explained for the associations between some IVs and OS biomarkers was relatively small. Additionally, this is a European based study, and our findings cannot be generalized to other populations.
5 Conclusion
In conclusion, this study used large exposure and outcome GWASs to conduct MR analysis to infer a causal relationship between OS and PD. We found robust genetic evidence for an association between Zn levels and higher PD risk, and the other antioxidants do not affect the risk of PD. This finding contrasts with preclinical studies, where antioxidants play a significant role in maintaining neuronal survival and activity in PD models (Duarte-Jurado et al., 2021). However, such effects have not been observed in clinical trials, as antioxidants have failed to modify the disease in terms of clinical symptoms or the onset of PD (Logroscino et al., 1996). This has given rise to the hypothesis that circulating antioxidant levels might not be representative of antioxidant capacity, and that increasing the levels of antioxidant in blood (either by nutritional intake or supplements) does not necessarily result in additional antioxidative effects. Our study offers novel insights into the complex interplay between antioxidant status and PD.
Data availability statement
The original contributions presented in the study are included in the article/Supplementary material; further inquiries can be directed to the corresponding authors.
Author contributions
LL: Writing – original draft, Data curation, Formal analysis, Visualization. ZL: Writing – review & editing, Data curation. XT: Writing – review & editing. LQ: Writing – review & editing, Funding acquisition. WY: Writing – review & editing, Funding acquisition. HZ: Writing – review & editing. FR: Writing – review & editing, Methodology. CW: Writing – review & editing, Conceptualization.
Funding
The author(s) declare that financial support was received for the research, authorship, and/or publication of this article. This research is supported by grant of National Natural Science Foundation of China (No. 82101342) and the National Science Foundation of Hunan Province (2023JJ40865).
Conflict of interest
The authors declare that the research was conducted in the absence of any commercial or financial relationships that could be construed as a potential conflict of interest.
Publisher’s note
All claims expressed in this article are solely those of the authors and do not necessarily represent those of their affiliated organizations, or those of the publisher, the editors and the reviewers. Any product that may be evaluated in this article, or claim that may be made by its manufacturer, is not guaranteed or endorsed by the publisher.
Supplementary material
The Supplementary material for this article can be found online at: https://www.frontiersin.org/articles/10.3389/fnagi.2024.1423773/full#supplementary-material
References
Abdelhamid, R. F., and Nagano, S. (2023). Crosstalk between oxidative stress and aging in neurodegeneration disorders. Cells 12, 1–16. doi: 10.3390/cells12050753
Anandhan, A., Jacome, M. S., Lei, S., Hernandez-Franco, P., Pappa, A., Panayiotidis, M. I., et al. (2017). Metabolic dysfunction in Parkinson's disease: bioenergetics, redox homeostasis and central carbon metabolism. Brain Res. Bull. 133, 12–30. doi: 10.1016/j.brainresbull.2017.03.009
Ben-Shushan, S., and Miller, Y. (2021). Neuropeptides: roles and activities as metal Chelators in neurodegenerative diseases. J. Phys. Chem. B 125, 2796–2811. doi: 10.1021/acs.jpcb.0c11151
Benyamin, B., Esko, T., Ried, J. S., Radhakrishnan, A., Vermeulen, S. H., Traglia, M., et al. (2014). Novel loci affecting iron homeostasis and their effects in individuals at risk for hemochromatosis. Nature Communications 5:4926. doi: 10.1038/ncomms5926
Blauwendraat, C., Nalls, M. A., and Singleton, A. B. (2020). The genetic architecture of Parkinson's disease. Lancet Neurol. 19, 170–178. doi: 10.1016/s1474-4422(19)30287-x
Bloem, B. R., Okun, M. S., and Klein, C. (2021). Parkinson's disease. Lancet 397, 2284–2303. doi: 10.1016/S0140-6736(21)00218-X
Bowden, J., Hemani, G., and Davey Smith, G. (2018). Invited commentary: detecting individual and global horizontal pleiotropy in Mendelian randomization—a job for the humble heterogeneity statistic? Am. J. Epidemiol. 187, 2681–2685. doi: 10.1093/aje/kwy185
Burgess, S., Davey Smith, G., Davies, N. M., Dudbridge, F., Gill, D., Glymour, M. M., et al. (2019). Guidelines for performing Mendelian randomization investigations. Wellcome Open Res 4:186. doi: 10.12688/wellcomeopenres.15555.1
Chang, K. H., and Chen, C. M. (2020). The role of oxidative stress in Parkinson's disease. Antioxidants 9:597. doi: 10.3390/antiox9070597
Devos, D., Moreau, C., Devedjian, J. C., Kluza, J., Petrault, M., Laloux, C., et al. (2014). Targeting chelatable iron as a therapeutic modality in Parkinson’s disease. Antioxid. Redox Signal. 21, 195–210. doi: 10.1089/ars.2013.5593
Du, K., Liu, M.-Y., Zhong, X., and Wei, M.-J. (2017). Decreased circulating zinc levels in Parkinson’s disease: a meta-analysis study. Sci. Rep. 7:3902. doi: 10.1038/s41598-017-04252-0
Duarte-Jurado, A. P., Gopar-Cuevas, Y., Saucedo-Cardenas, O., Loera-Arias, M. J., Montes-de-Oca-Luna, R., Garcia-Garcia, A., et al. (2021). Antioxidant therapeutics in Parkinson’s disease: current challenges and opportunities. Antioxidants 10:453. doi: 10.3390/antiox10030453
Emamzadeh, F. N., and Surguchov, A. (2018). Parkinson’s disease: biomarkers, treatment, and risk factors. Front. Neurosci. 12:612. doi: 10.3389/fnins.2018.00612
Evans, D. M., Zhu, G., Dy, V., Heath, A. C., Madden, P. A., Kemp, J. P., et al. (2013). Genome-wide association study identifies loci affecting blood copper, selenium and zinc. Hum. Mol. Genet. 22, 3998–4006. doi: 10.1093/hmg/ddt239
Fahn, S. (1992). A pilot trial of high-dose alpha-tocopherol and ascorbate in early Parkinson's disease. Ann. Neurol. 32, S128–S132.
Finkelstein, D. I., Zhao, H.-W., Lin, J., Wang, X.-B., Cheng, X., Wang, J.-Y., et al. (2013). Assessing plasma levels of selenium, copper, Iron and zinc in patients of Parkinson’s disease. PLoS One 8:e83060. doi: 10.1371/journal.pone.0083060
Floor, E., and Wetzel, M. G. (1998). Increased protein oxidation in human substantia Nigra pars compacta in comparison with basal ganglia and prefrontal cortex measured with an improved Dinitrophenylhydrazine assay. J. Neurochem. 70, 268–275. doi: 10.1046/j.1471-4159.1998.70010268.x
Group, P. S. (1993). Effects of tocopherol and deprenyl on the progression of disability in early Parkinson’s disease. N. Engl. J. Med. 328, 176–183. doi: 10.1056/NEJM199301213280305
Group, P. S. (1998). Mortality in DATATOP: a multicenter trial in early Parkinson’s disease. Parkinson study group. Ann. Neurol. 43, 318–325. doi: 10.1002/ana.410430309
Pichler, I., Del Greco, M. F., Gögele, M., Lill, C. M., Bertram, L., Do, C. B., et al. (2013). Serum Iron levels and the risk of Parkinson disease: a Mendelian randomization study. PLoS Med. 10:e1001462. doi: 10.1371/journal.pmed.1001462
Hughes, K. C., Gao, X., Kim, I. Y., Rimm, E. B., Wang, M., Weisskopf, M. G., et al. (2016). Intake of antioxidant vitamins and risk of Parkinson’s disease. Mov. Disord. 31, 1909–1914. doi: 10.1002/mds.26819
Jimenez-Moreno, N., and Lane, J. D. (2020). Autophagy and redox homeostasis in Parkinson’s: a crucial balancing act. Oxidative Med. Cell. Longev. 2020, 1–38. doi: 10.1155/2020/8865611
Kalia, L. V., and Lang, A. E. (2015). Parkinson's disease. Lancet 386, 896–912. doi: 10.1016/s0140-6736(14)61393-3
Khan, Z., and Ali, S. A. (2018). Oxidative stress-related biomarkers in Parkinson’s disease: a systematic review and meta-analysis. Iran J. Neurol. 17, 137–144
Logroscino, G., Marder, K., Cote, L., Tang, M. X., Shea, S., and Mayeux, R. (1996). Dietary lipids and antioxidants in Parkinson's disease: a population-based, case-control study. Ann. Neurol. 39, 89–94. doi: 10.1002/ana.410390113
Maiti, P., Manna, J., and Dunbar, G. L. (2017). Current understanding of the molecular mechanisms in Parkinson's disease: targets for potential treatments. Transl. Neurodegen. 6:28. doi: 10.1186/s40035-017-0099-z
Medeiros, M. S., Schumacher-Schuh, A., Cardoso, A. M., Bochi, G. V., Baldissarelli, J., Kegler, A., et al. (2016). Iron and oxidative stress in Parkinson's disease: an observational study of injury biomarkers. PLoS One 11:e0146129. doi: 10.1371/journal.pone.0146129
Miyake, Y., Fukushima, W., Tanaka, K., Sasaki, S., Kiyohara, C., Tsuboi, Y., et al. (2011). Dietary intake of antioxidant vitamins and risk of Parkinson's disease: a case-control study in Japan. Eur. J. Neurol. 18, 106–113. doi: 10.1111/j.1468-1331.2010.03088.x
Nalls, M. A., Blauwendraat, C., Vallerga, C. L., Heilbron, K., Bandres-Ciga, S., Chang, D., et al. (2019). Identification of novel risk loci, causal insights, and heritable risk for Parkinson's disease: a meta-analysis of genome-wide association studies. Lancet Neurol. 18, 1091–1102. doi: 10.1016/S1474-4422(19)30320-5
Nguyen, H. N., Byers, B., Cord, B., Shcheglovitov, A., Byrne, J., Gujar, P., et al. (2011). LRRK2 mutant iPSC-derived DA neurons demonstrate increased susceptibility to oxidative stress. Cell Stem Cell 8, 267–280. doi: 10.1016/j.stem.2011.01.013
Palmer, T. M., Lawlor, D. A., Harbord, R. M., Sheehan, N. A., Tobias, J. H., Timpson, N. J., et al. (2011). Using multiple genetic variants as instrumental variables for modifiable risk factors. Stat. Methods Med. Res. 21, 223–242. doi: 10.1177/0962280210394459
Pals, P., Van Everbroeck, B., Grubben, B., Viaene, M. K., Dom, R., van der Linden, C., et al. (2003). Case–control study of environmental risk factors for Parkinson’s disease in Belgium. Eur. J. Epidemiol. 18, 1133–1142. doi: 10.1023/b:ejep.0000006639.05690.92
Parkinson Study Group (1998). Effects of tocopherol and deprenyl on the progression of disability in early Parkinson's disease. N. Engl. J. Med. 328, 176–183. doi: 10.1056/NEJM199301213280305
Pearce, R. K., Owen, A., Daniel, S., Jenner, P., and Marsden, C. D. (1997). Alterations in the distribution of glutathione in the substantia nigra in Parkinson's disease. J. Neural Transm. 104, 661–677. doi: 10.1007/BF01291884
Poewe, W., Seppi, K., Tanner, C. M., Halliday, G. M., Brundin, P., Volkmann, J., et al. (2017). Parkinson disease. Nat. Rev. Dis. Primers 3:17013. doi: 10.1038/nrdp.2017.13
Powers, K. M., Smith-Weller, T., Franklin, G. M., Longstreth, W. T., Swanson, P. D., and Checkoway, H. (2003). Parkinson's disease risks associated with dietary iron, manganese, and other nutrient intakes. Neurology 60, 1761–1766. doi: 10.1212/01.WNL.0000068021.13945.7F
Puspita, L., Chung, S. Y., and Shim, J. W. (2017). Oxidative stress and cellular pathologies in Parkinson's disease. Mol. Brain 10:53. doi: 10.1186/s13041-017-0340-9
Scheider, W. L., Hershey, L. A., Vena, J. E., Holmlund, T., and Marshall, J. R. Freudenheim (1997). Dietary antioxidants and other dietary factors in the etiology of Parkinson's disease. Mov. Disord. 12, 190–196. doi: 10.1002/mds.870120209
Shin, S.-Y., Fauman, E. B., Petersen, A.-K., Krumsiek, J., Santos, R., Huang, J., et al. (2014). An atlas of genetic influences on human blood metabolites. Nat. Genet. 46, 543–550. doi: 10.1038/ng.2982
Sikora, J., and Ouagazzal, A. M. (2021). Synaptic zinc: an emerging player in Parkinson's disease. Int. J. Mol. Sci. 22:4724. doi: 10.3390/ijms22094724
Smeyne, M., and Smeyne, R. J. (2013). Glutathione metabolism and Parkinson's disease. Free Radic. Biol. Med. 62, 13–25. doi: 10.1016/j.freeradbiomed.2013.05.001
Sun, B. B., Maranville, J. C., Peters, J. E., Stacey, D., Staley, J. R., Blackshaw, J., et al. (2018). Genomic atlas of the human plasma proteome. Nature 558, 73–79. doi: 10.1038/s41586-018-0175-2
Tobón-Velasco, J. C., Carmona-Aparicio, L., Ali, S. F., and Santamaría, A. (2010). Biomarkers of cell damage induced by oxidative stress in Parkinson’s disease and related models. Cent. Nerv. Syst. Agents Med. Chem. 10, 278–286. doi: 10.2174/187152410793429719
Umeno, A., Biju, V., and Yoshida, Y. (2017). In vivo ROS production and use of oxidative stress-derived biomarkers to detect the onset of diseases such as Alzheimer’s disease, Parkinson’s disease, and diabetes. Free Radic. Res. 51, 413–427. doi: 10.1080/10715762.2017.1315114
Vinish, M., Anand, A., and Prabhakar, S. (2011). Altered oxidative stress levels in Indian Parkinson’s disease patients with PARK2 mutations. Acta Biochim. Pol. 58, 165–169. doi: 10.18388/abp.2011_2260
Wang, B., Fang, T., and Chen, H. (2023). Zinc and central nervous system disorders. Nutrients 15:2140. doi: 10.3390/nu15092140
Glossary
Keywords: Parkinson’s disease, oxidative stress, Mendelian randomization study, causal relationship, zinc
Citation: Lv L, Long Z, Tan X, Qin L, Yan W, Zhang H, Ren F and Wang C (2024) Bidirectional two-sample Mendelian randomization analysis identifies causal associations between oxidative stress and Parkinson’s disease. Front. Aging Neurosci. 16:1423773. doi: 10.3389/fnagi.2024.1423773
Edited by:
Yildiz Degirmenci, Istanbul Health and Technology University, TürkiyeReviewed by:
Ling Long, Third Affiliated Hospital of Sun Yat-sen University, ChinaAndrei Surguchov, University of Kansas Medical Center, United States
Copyright © 2024 Lv, Long, Tan, Qin, Yan, Zhang, Ren and Wang. This is an open-access article distributed under the terms of the Creative Commons Attribution License (CC BY). The use, distribution or reproduction in other forums is permitted, provided the original author(s) and the copyright owner(s) are credited and that the original publication in this journal is cited, in accordance with accepted academic practice. No use, distribution or reproduction is permitted which does not comply with these terms.
*Correspondence: Feng Ren, renfeng@csu.edu.cn; Chunyu Wang, wangchunyu@csu.edu.cn