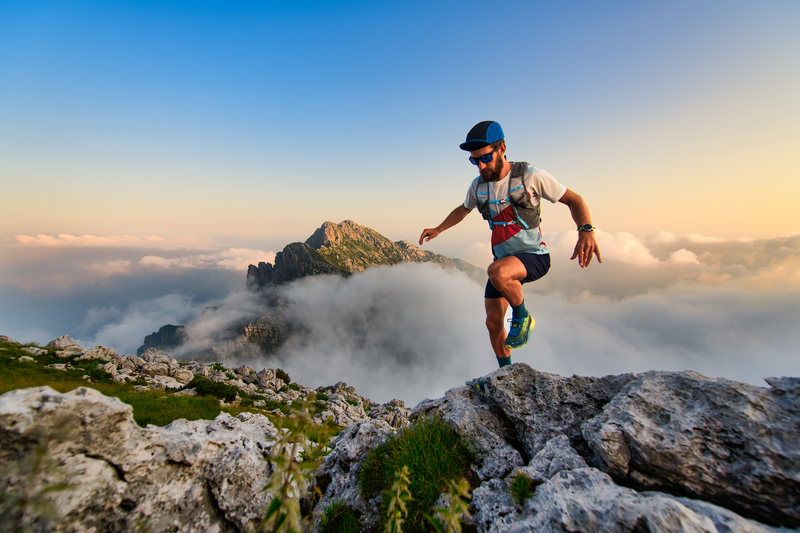
95% of researchers rate our articles as excellent or good
Learn more about the work of our research integrity team to safeguard the quality of each article we publish.
Find out more
ORIGINAL RESEARCH article
Front. Aging Neurosci. , 02 August 2024
Sec. Alzheimer's Disease and Related Dementias
Volume 16 - 2024 | https://doi.org/10.3389/fnagi.2024.1423725
Purpose: Alzheimer’s disease (AD) is a common neurodegenerative disease, which can lead to cognitive impairment and dementia. Since AD is tightly associated with aging and cellular senescence, objective of this study was to investigate the association between senescence-related genes and proteins (SRGs and SRPs) and the development of AD.
Design: The whole study was based on transcriptomic analysis of control and AD brain tissues and Mendelian randomization (MR) analysis.
Methods: For transcriptomic analysis, GSE5281 dataset from GEO database contains the transcriptomic data of human brain tissues (n = 161) from control group and AD patients. The expression of SRGs in control and AD brain tissues were compared by Student’s t test. For MR analysis, the instrumental single-nucleotide polymorphisms (SNPs) associated with 110 SRPs were filtered and selected from a large genome-wide association study (GWAS) for plasma proteome. The causality between plasma levels of SRPs and AD was explored using GWAS data of AD from Lambert et al. (17,008 cases and 37,154 controls) and further validated by using data from FinnGen consortium (6,489 patients and 170,489 controls). MR estimate was performed using the inverse-variance weighted (IVW) method and the heterogeneity and pleiotropy of results were tested.
Results: Transcriptomic analysis identified 36 up-regulated (including PLAUR) and 8 down-regulated SRGs in AD brain tissues. In addition, the MR results at both discovery and validation stages supported the causality between plasma levels of PLAUR (IVW-p = 3.04E-2, odds ratio [OR] = 1.15), CD55 (IVW-p = 1.56E-3, OR = 0.86), and SERPINE2 (IVW-p = 2.74E-2, OR = 0.91) and the risk of AD.
Conclusion: Our findings identified that PLAUR, as an SRG, may take part in the development of AD and found that high plasma levels of PLAUR was associated with increased risk of AD, indicating that this gene was a risk factor for this disease and providing the rationale of existing drugs or new preventative and therapeutic strategies.
Worldwide, dementia has become a major public concern, whose prevalence is expected to reach 152 million by 2050 (Livingston et al., 2020), placing considerable health burden on affected individuals and economic burden on society. Notably, a significant proportion of dementia cases was caused in whole or in part by Alzheimer’s disease (AD) (Brookmeyer et al., 2011; Saez-Atienzar and Masliah, 2020). AD is a progressive neurodegenerative disease (NDD) leading to cognitive impairment, neuropsychiatric symptoms, disability, and even premature death (Demakis, 2007; Gillespie et al., 2013). Despite recent advances, the preventive or treatment options for AD remain limited.
Advancing age is a major risk factor of AD (Holloway et al., 2023). Cellular senescence is a hallmark of aging and a significant contributor to age-related diseases including AD (Holloway et al., 2023). The accumulation of senescent neurons and glial cells have been detected in the brains of AD patients and mouse models and that selective clearance of senescent cells has been reported to prevent tau pathology and improves cognition in AD mouse models (Bussian et al., 2018). However, the mechanisms underlying when and how cellular senescence contributes to AD pathogenesis remain unclear.
Importantly, a gene set of senescence-related genes (SRGs) was reported for predicting senescence-associated pathways and senescent status across tissues (Saul et al., 2022), used in many studies about age-related diseases (Doolittle et al., 2023; Farr, 2023; Yu et al., 2023; Lei et al., 2024). This list of SRGs contains 9 categories, including Metalloproteases, Cytokine/Chemokine, Growth factor, Intercellular signal molecule, Miscellaneous, Protease inhibitors, Protein modifying enzymes, Transcription factors and regulators, and Transmembrane signal receptors (Saul et al., 2022). Saul et al. (2022) also illustrated a dense interaction network encoded by there SRGs, where every gene was highly influenced by other members in this gene set in multiple styles such as ligand-receptor interactions (such as EGF and EGFR) and influence expression or secretion patterns (such as TNFA and IL6). This network of SRGs was considered to facilitate better characterization of senescent cells. Especially, Lei et al. (2024) used this gene set and identified potential biomarkers and drug targets for age-related macular degeneration (AMD), an NDD in the eye. However, the association between SRGs and the proteins encoded by them (senescence-related proteins, SRPs) and the development of AD has not been investigated and remains unclear.
In this study, by combing transcriptomic and Mendelian randomization (MR) analysis, we explored the expression of SRGs in control and AD brain tissues and the causality between the plasma levels of SRPs and the risk of AD (Figure 1). Mendelian randomization (MR) approaches have provided the possibility and opportunities for researchers to identify risk or protective factors for various diseases (Davey Smith and Hemani, 2014), by using genome-wide association studies (GWAS) data and genetic variants as instrument. Moreover, MR approaches have been used to explore risk factors of AD (Li et al., 2023; Ning et al., 2023; Zhu et al., 2023). As a results, the expression of PLAUR was up-regulated in AD brain and the plasma level of protein encoded by PLAUR gene were identified to be associated with increased risk of AD.
Figure 1. The summary of the study design. AD, Alzheimer’s disease; SRGs, senescence-related genes; MR, Mendelian randomization; GWAS, genome-wide association studies; SRPs, senescence-related proteins.
The RNA sequencing data of human brain tissues were obtained from GEO database. GSE5281 reported the transcriptomic atlas of human brain tissues (n = 161) from control group and AD patients (Newman et al., 2012). The list of SRGs was shown in Supplementary Table 1 (Saul et al., 2022). The expression of SRGs in control and AD brain tissues were compared by student t test via limma R package. The criteria for differentially expressed genes (DEGs) were adjusted p < 0.05 and |log (FC) | > 1.
In this study, we used a two-sample MR approach (inverse-variance weighted, IVW) to explore relationship between SRPs plasma levels and risk of AD. The summary-level GWAS data of SRPs were obtained from Ferkingstad et al. (2021), a large-scale GWAS project on plasma proteome involving 35,559 participants. Summary-level GWAS data of AD were obtained from a meta-analysis of previously published GWAS datasets and reported by Lambert et al. (2013), which includes 17,008 AD cases and 37,154 cognitively normal age-matched controls. GWAS data of AD from FinnGen consortium (6,489 cases and 170,489 controls) (Kurki et al., 2003) was used for replication and validation.
The flowchart of this study is presented in Figure 2. Following the acquisition of necessary GWAS data, we need to selected suitable genetic instrument for subsequent analysis. Genetic instrument for MR analysis, i.e., single-nucleotide polymorphisms (SNPs), were selected through the following process and criteria: (1) significantly associated with the exposures (p < 5 × 10−6); (2) linkage disequilibrium (LD) threshold (r2 < 0.01 and window size 10,000 kb); (3) F-statistic > 10.
Figure 2. Flowchart of the MR analyses. MR, Mendelian randomization; SRPs, senescence-related proteins; AD, Alzheimer’s disease; SNP, single nucleotide polymorphism; IVW, inverse-variance weighted.
The control of pleiotropy is important for the validity of MR results (Lawlor et al., 2008). Therefore, we used the random-effect IVW method (Burgess et al., 2016) as the method for MR estimates and performed Cochran’s Q test and MR-Egger intercept test to evaluate the heterogeneity and detect pleiotropy (Bowden et al., 2019). Moreover, the MR Pleiotropy RESidual Sum and Outlier (MR-PRESSO) (Verbanck et al., 2018) test was used to further assess the robustness of MR estimations and SNPs identified as outliers by MR-PRESSO were removed. The cutoff used in these three tests was set as 0.05, where p > 0.05 means there was no apparent heterogeneity or pleiotropy.
Potential drugs targeting the identified AD-associated plasma proteins were obtained from the Drug-Gene Interaction Database (DGIdb 5.0)1 (Cannon et al., 2024), which can output a list of potential drugs after you input a name of gene.
All analyses were performed in R (version 4.0.1) using the limma and TwoSampleMR (Hemani et al., 2018) R package. All statistical tests are two-sided. The cutoff for heterogeneity and pleiotropy tests was set as 0.05, where p > 0.05 means there was no apparent heterogeneity or pleiotropy. The code for MR analysis is accessible at https://mrcieu.github.io/TwoSampleMR/articles/index.html.
Figure 3 presented the heatmap of SRGs expression in control and AD brain tissues, showing that many SRGs were differentially expressed in these two groups. The 36 up-regulated SRGs in AD brain and the violin plot for them was shown in Figure 4, including PTBP1, SERPINE2, HMGB1, SEMA3F, TNFRSF1A, PGF, GEM, PECAM1, SPP1, IGFBP7, CSF1, IL6ST, JUN, TNFRSF1B, RPS6KA5, FGF2, EGFR, CXCL1, CXCL16, ANGPTL4, HGF, AXL, FGF1, ANGPT1, PTGES, ICAM1, LCP1, SPX, PLAUR, EDN1, SELPLG, CCL2, CXCL12, MMP14, IGFBP5, and CXCL10. The 8 down-regulated SRGs in AD brain and the violin plot for them was shown in Figure 5, including MIF, NRG1, TUBGCP2, IGF1, KITLG, ETS2, INHA, and VGF.
Figure 3. The heatmap showing the expression profiles of SRGs in control and AD brain tissues. SRGs, senescence-related genes; AD, Alzheimer’s disease.
Figure 4. The violin plot of the 36 up-regulated SRGs in AD brain tissue. SRGs, senescence-related genes; AD, Alzheimer’s disease.
Figure 5. The violin plot of the 8 down-regulated SRGs in AD brain tissue. SRGs, senescence-related genes; AD, Alzheimer’s disease.
Figure 2 presented the flowchart of MR analysis. In the discovery stage, 7 SRPs were associated with risk of AD (Figure 6A; Supplementary Table 2), including: CCL8 (odds ratio [OR] = 0.94, 95% confidence interval [CI]: 0.90–0.99, IVW-p = 1.14E-2), CD55 (OR = 0.86, 95% CI: 0.78–0.94, IVW-p = 1.56E-3), PLAUR (OR = 1.15, 95% CI: 1.01–1.30, IVW-p = 3.04E-2), PAPPA (OR = 0.93, 95% CI: 0.87–1.00, IVW-p = 4.43E-2), SERPINE2 (OR = 0.91, 95% CI: 0.84–0.99, IVW-p = 2.74E-2), TNFRSF1A (OR = 0.88, 95% CI: 0.79–0.99, IVW-p = 3.74E-2), VEGFA (OR = 0.95, 95% CI: 0.90–1.00, IVW-p = 4.21E-2). The p values of MR-Egger intercept test and Cochran’s Q test are all beyond 0.05, indicating no heterogeneity or pleiotropy in these results.
Figure 6. Forest plot of the MR results in discovery (A) and validation (B) stage. SNP, single nucleotide polymorphism; IVW, inverse-variance weighted; OR, odds ratio; AD, Alzheimer’s disease.
In this stage, 7 SRPs were associated with risk of AD (Figure 6B; Supplementary Table 3), including: AXL (OR = 0.91, 95% CI: 0.83–0.99, IVW-p = 2.78E-2), CCL5 (OR = 1.15, 95% CI: 1.02–1.30, IVW-p = 2.66E-2), CD55 (OR = 0.89, 95% CI: 0.82–0.97, IVW-p = 1.01E-2), KITLG (OR = 1.23, 95% CI: 1.03–1.46, IVW-p = 2.33E-2), MMP2 (OR = 0.83, 95% CI: 0.70–0.98, IVW-p = 2.85E-2); PLAUR (OR = 1.29, 95% CI: 1.04–1.60, IVW-p = 2.38E-2); SERPINE2 (OR = 0.90, 95% CI: 0.81–0.99, IVW-p = 3.84E-2). Also, the p values of MR-Egger intercept test and Cochran’s Q test are all beyond 0.05, indicating no heterogeneity or pleiotropy in these results. Notably, the effect of PLAUR, CD55, and SERPINE2 on the risk of AD was validated.
We obtained approved and not approved drugs potentially interacting with PLAUR from DGIdb database, including LENOGRASTIM, PHORBOL 12-MYRISTATE 13-ACETATE, RUXOLITINIB RECOMBINANT HUMAN MONOCYTE CHEMOATTRACTANT PROTEIN-2, RECOMBINANT INTERFERON GAMMA, ADENOVIRUS VECTOR, and DIPHTHERIA TOXIN (Supplementary Table 4).
AD is a neurodegenerative disease (NDD) predominantly affecting the elders, associated with cognitive impairment and decreased quality of life in affected individuals (Livingston et al., 2020). Identifying potential biomarker and drug targets for AD is important. Therefore, this study aimed to explore the association between SRGs and SRPs and the development of AD. As a results, MR analysis indicated that high plasma levels of 2 SRPs (CD55 and SERPINE2) were associated with decreased risk of AD. More importantly, PLAUR was found to be up-regulated in AD brain tissues and the high plasma level of PLAUR was associated with higher risk of AD, which may contribute to the development of preventive and treatment strategies for this disease.
In aging individuals, there is a decline in the homeostasis capacity, which contributes to the accumulation of harmful cellular stressors, such as oxidative stress and DNA damage and lead to aging-associated damage to cells (Yang et al., 2024). Cellular senescence has been recognized as a fundamental mechanism underlying aging in organisms. Aging-associated homeostatic alterations activates cellular senescence (Li et al., 2024) and triggers chronic inflammation if the senescent cells were not cleared in time and accumulated. Characteristics of senescent cells include profound chromatin, secretory phenotype changes, and increased expression of senescence markers (Tchkonia et al., 2013). Senescent cells can develop a senescence-associated secretory phenotype (SASP) (Coppé et al., 2008; Xu et al., 2015), which is associated with physical, metabolic, and cognitive decline (Boniewska-Bernacka et al., 2020; Diniz et al., 2021). In AD, the chronic inflammation caused by the accumulation of senescent cells induces synapse damage and contributes to cognitive decline (Saez-Atienzar and Masliah, 2020). Notably, the association between toxic Tau protein aggregation and cellular senescence has been reported (Musi et al., 2018). The accumulation of senescent cells could induce a harmful environment for neuronal cells, making them more susceptible to toxic protein aggregation, and induce proteinopathy, both contributing to neuron loss and cognitive decline in AD (Saez-Atienzar and Masliah, 2020). A previous study has showed that the formation of senescent cells precedes tau aggregation in the AD mouse model (Bussian et al., 2018). Cellular senescence, in combination with the toxic protein aggregation, contributes to a greater accumulation of harmful factor within the cells in the brain. PLAUR, firstly identified as an SRG by Amor et al. (2020) and further identified by Saul et al. (2022), was found to be up-regulated in AD brain and the plasma level of the protein encoded by this gene was associated with higher risk of AD, indicating the potential role of it in the development of AD.
PLAUR gene encodes a protein called uPAR (urokinase plasminogen activator receptor), which is involved in many biological processes (Ragno, 2006; Blasi and Sidenius, 2010). Notably, uPAR is involved in inflammation-associated pathways such as the migration of inflammatory cells to lesions in tissue. Besides the plasma membrane-binding form, uPAR has soluble forms generated and released by glycosyl-phosphatidyl-inositol (GPI)-directed phospholipase. The classical function of uPAR is binding its ligand uPA, leading to the activation of plasminogen and subsequent proteolysis. Besides its canonical roles, evidence has indicated the participation of uPAR in the development of brain diseases (Archinti et al., 2011). Changes in the expression of uPAR has been observed in multiple brain diseases (Bruneau and Szepetowski, 2011). For instance, expression analysis in epileptic conditions revealed increased expression of uPAR in hippocampal and frontal lobe neurons and other cell types including microglia and vascular endothelial cells (Iyer et al., 2010; Liu et al., 2010). The increased expression of uPAR in microglia has also been observed in various neurodegenerative conditions associated with inflammatory responses (Cunningham et al., 2009). In multiple sclerosis (MS), increased expression of uPAR on the inflammatory cells in the perivascular zones has been demonstrated (Gveric et al., 2001), which was considered to be associated with further infiltration of inflammatory cells into the lesion. Such an increase in uPAR expression in perivascular zone has also been reported in AD brain (Deininger et al., 2002). Additionally, Walker et al. reported the significantly increased uPAR protein levels in human brain tissues with AD compared with control cases (Walker et al., 2002). They further demonstrate that uPAR expression is up-regulated following incubation of microglia obtained from human post-mortem brain using Aβ peptide (Walker et al., 2002). These results provide a connection between microglial activation and the possible involvement of uPAR in the pathogenesis of AD. Collectively, the association between PLAUR and the development of AD observed in this study might be bridged by the pro-inflammatory effects (potentially associated with microglial activation) of it in the central nervous system (CNS). Notably, despite two SNPs located in the PLAUR gene were indicative of a trend toward association, Cetinsoy et al. (2024) reported no significant genetic association between polymorphisms across the PLAUR gene and AD by utilizing the DNA bank of the Brains for Dementia Research cohort. This conflicting result might be caused by the differences between the study design and participants and highlighted the importance of our findings to be confirmed by more larger and prospective studies.
CD55, also known as Complement decay-accelerating factor, belongs to complement regulatory proteins and can protect cells from systemic complement (Flückiger et al., 2018). The deficiency of CD55 can lead to the increased sensitivity of cells to complement-mediated destruction (Merrill and Brodsky, 2018; Gurnari et al., 2021). It has also been reported that CD55 can control the adverse immune responses and neuroinflammation in CNS (Hoarau et al., 2011; Hernangómez et al., 2014). Notably, previous study found that a somatic mutation influencing transcription factor binding upstream of CD55 gene contributes to AD pathogenesis by affecting the complement system (Helgadottir et al., 2019). Considering the identified causal effect of plasma CD55 on lower AD risk in this study, the mechanism underlying this effect might be bridged by the modulation of complement system and neuroinflammation.
SERPINE2 (Serpin Family E Member 2), also termed as Protease Nexin-1 (PN-1), is a member of the serpin family of proteins, which can inhibit serine proteases including thrombin, urokinase, plasmin, and trypsin (Madjene et al., 2021), whose role in AD has not been fully understood. It presents strong antithrombotic and antifibrinolytic properties (Madjene et al., 2021). It is demonstrated that SERPINE2 is constitutively secreted through small vesicles and plays a key role for synaptic plasticity in the developing and adult CNS (Giau et al., 2005). It has also been reported that SERPINE2 is highly expressed by many cell types, especially microglia, and loss of SERPINE2 can lead to behavioral changes as well as deficits in synaptic plasticity in CNS (Krawczyk et al., 2023). Studies have also noticed the neurotrophic properties of SERPINE2 (Winokur et al., 2017), which can promote neurite outgrowth and extension (Choi et al., 1995). In addition, SERPINE2 is associated with coagulation and cholesterol homeostasis, whose mutations show pleiotropic effects on blood-cell-related traits such as platelet count, metabolic traits such as levels of lipoprotein and lipid, and on disease traits such as coronary artery disease and type 2 diabetes (Nath et al., 2019). Collectively, the effects of SERPINE2 on neurotrophy, synaptic plasticity, and neurite extension, as well as its influences on coagulation and lipid metabolism, might be the mechanical basis of the association between plasma SERPINE2 and lower risk of AD.
There were some limitations in this MR-designed investigation. First, this study only included European-ancestry individuals, which suggests that our findings cannot be directly applied to other populations. Second, our findings only revealed the causality between plasma levels of several SRPs and AD, not the underlying mechanisms, which are required to be elucidated by further studies.
In conclusion, by combing transcriptomic and MR analysis, we provided the evidence that PLAUR, as an SRG, may play a role in the development of AD, which may provide the rationale of existing drugs or novel drugs for AD, such as LENOGRASTIM and RUXOLITINIB (Cannon et al., 2024). Still, the results require to be confirmed by further studies. Moreover, investigating the mechanism underlying the identified causality in our study would also help us understanding the pathogenesis of this disease.
The original contributions presented in the study are included in the article/Supplementary material, further inquiries can be directed to the corresponding author.
This study used only publicly available, deidentified summary statistics from previously published works, making it exempt according to the institutional review board (IRB) of Shengjing Hospital of China Medical University. Our research adhered to the tenets of the Declaration of Helsinki.
YL: Writing – original draft, Visualization, Project administration, Methodology, Investigation, Formal analysis, Data curation, Conceptualization. JC: Writing – review & editing, Visualization, Supervision, Project administration, Methodology, Investigation, Formal analysis, Data curation, Conceptualization.
The author(s) declare that no financial support was received for the research, authorship, and/or publication of this article.
The authors declare that the research was conducted in the absence of any commercial or financial relationships that could be construed as a potential conflict of interest.
All claims expressed in this article are solely those of the authors and do not necessarily represent those of their affiliated organizations, or those of the publisher, the editors and the reviewers. Any product that may be evaluated in this article, or claim that may be made by its manufacturer, is not guaranteed or endorsed by the publisher.
The Supplementary material for this article can be found online at: https://www.frontiersin.org/articles/10.3389/fnagi.2024.1423725/full#supplementary-material
AD, Alzheimer’s disease; SRGs, Senescence-related genes; SRPs, Senescence-related proteins; MR, Mendelian randomization; SNP, Single nucleotide polymorphism; GWAS, Genome-wide association study; IVW, Inverse variance-weighted; OR, Odds ratio; CI, Confidence interval
Amor, C., Feucht, J., Leibold, J., Ho, Y. J., Zhu, C., Alonso-Curbelo, D., et al. (2020). Senolytic CAR T cells reverse senescence-associated pathologies. Nature 583, 127–132. doi: 10.1038/s41586-020-2403-9
Archinti, M., Britto, M., Eden, G., Furlan, F., Murphy, R., and Degryse, B. (2011). The urokinase receptor in the central nervous system. CNS Neurol. Disord. Drug Targets 10, 271–294. doi: 10.2174/187152711794480393
Blasi, F., and Sidenius, N. (2010). The urokinase receptor: focused cell surface proteolysis, cell adhesion and signaling. FEBS Lett. 584, 1923–1930. doi: 10.1016/j.febslet.2009.12.039
Boniewska-Bernacka, E., Pańczyszyn, A., and Klinger, M. (2020). Telomeres and telomerase in risk assessment of cardiovascular diseases. Exp. Cell Res. 397:112361. doi: 10.1016/j.yexcr.2020.112361
Bowden, J., Del Greco, M. F., Minelli, C., Zhao, Q., Lawlor, D. A., Sheehan, N. A., et al. (2019). Improving the accuracy of two-sample summary-data Mendelian randomization: moving beyond the NOME assumption. Int. J. Epidemiol. 48, 728–742. doi: 10.1093/ije/dyy258
Brookmeyer, R., Evans, D. A., Hebert, L., Langa, K. M., Heeringa, S. G., Plassman, B. L., et al. (2011). National estimates of the prevalence of Alzheimer's disease in the United States. Alzheimers Dement. 7, 61–73. doi: 10.1016/j.jalz.2010.11.007
Bruneau, N., and Szepetowski, P. (2011). The role of the urokinase receptor in epilepsy, in disorders of language, cognition, communication and behavior, and in the central nervous system. Curr. Pharm. Des. 17, 1914–1923. doi: 10.2174/138161211796718198
Burgess, S., Dudbridge, F., and Thompson, S. G. (2016). Combining information on multiple instrumental variables in Mendelian randomization: comparison of allele score and summarized data methods. Stat. Med. 35, 1880–1906. doi: 10.1002/sim.6835
Bussian, T. J., Aziz, A., Meyer, C. F., Swenson, B. L., van Deursen, J. M., and Baker, D. J. (2018). Clearance of senescent glial cells prevents tau-dependent pathology and cognitive decline. Nature 562, 578–582. doi: 10.1038/s41586-018-0543-y
Cannon, M., Stevenson, J., Stahl, K., Basu, R., Coffman, A., Kiwala, S., et al. (2024). DGIdb 5.0: rebuilding the drug-gene interaction database for precision medicine and drug discovery platforms. Nucleic Acids Res. 52, D1227–d1235. doi: 10.1093/nar/gkad1040
Cetinsoy, O., Anyanwu, I., Krishnanand, H., Natarajan, G., Ramachandran, N., Thomas, A., et al. (2024). Gene Association study of the Urokinase plasminogen activator and its receptor gene in Alzheimer's disease. J. Alzheimers Dis. 99, 241–250. doi: 10.3233/JAD-231383
Choi, B. H., Kim, R. C., Vaughan, P. J., Lau, A., Van Nostrand, W. E., Cotman, C. W., et al. (1995). Decreases in protease nexins in Alzheimer's disease brain. Neurobiol. Aging 16, 557–562. doi: 10.1016/0197-4580(95)00060-R
Coppé, J. P., Patil, C. K., Rodier, F., Sun, Y., Muñoz, D. P., Goldstein, J., et al. (2008). Senescence-associated secretory phenotypes reveal cell-nonautonomous functions of oncogenic RAS and the p53 tumor suppressor. PLoS Biol. 6, 2853–2868. doi: 10.1371/journal.pbio.0060301
Cunningham, O., Campion, S., Perry, V. H., Murray, C., Sidenius, N., Docagne, F., et al. (2009). Microglia and the urokinase plasminogen activator receptor/uPA system in innate brain inflammation. Glia 57, 1802–1814. doi: 10.1002/glia.20892
Davey Smith, G., and Hemani, G. (2014). Mendelian randomization: genetic anchors for causal inference in epidemiological studies. Hum. Mol. Genet. 23, R89–R98. doi: 10.1093/hmg/ddu328
Deininger, M. H., Trautmann, K., Magdolen, V., Luther, T., Schluesener, H. J., and Meyermann, R. (2002). Cortical neurons of Creutzfeldt-Jakob disease patients express the urokinase-type plasminogen activator receptor. Neurosci. Lett. 324, 80–82. doi: 10.1016/S0304-3940(02)00168-4
Demakis, G. J. (2007). Disability in Alzheimer's disease: causes, consequences, and economic considerations. J. Health Hum. Serv. Adm. 30, 292–305. doi: 10.1177/107937390703000302
Diniz, B. S., Vieira, E. M., Mendes-Silva, A. P., Bowie, C. R., Butters, M. A., Fischer, C. E., et al. (2021). Mild cognitive impairment and major depressive disorder are associated with molecular senescence abnormalities in older adults. Alzheimers Dement 7:e12129. doi: 10.1002/trc2.12129
Doolittle, M. L., Saul, D., Kaur, J., Rowsey, J. L., Vos, S. J., Pavelko, K. D., et al. (2023). Multiparametric senescent cell phenotyping reveals targets of senolytic therapy in the aged murine skeleton. Nat. Commun. 14:4587. doi: 10.1038/s41467-023-40393-9
Farr, J. N. (2023). Skeletal senescence with aging and type 2 diabetes. Endocrinol. Metab. 38, 295–301. doi: 10.3803/EnM.2023.1727
Ferkingstad, E., Sulem, P., Atlason, B. A., Sveinbjornsson, G., Magnusson, M. I., Styrmisdottir, E. L., et al. (2021). Large-scale integration of the plasma proteome with genetics and disease. Nat. Genet. 53, 1712–1721. doi: 10.1038/s41588-021-00978-w
Flückiger, R., Cocuzzi, E., Nagaraj, R. H., Shoham, M., Kern, T. S., and Medof, M. E. (2018). DAF in diabetic patients is subject to glycation/inactivation at its active site residues. Mol. Immunol. 93, 246–252. doi: 10.1016/j.molimm.2017.06.036
Giau, R., Carrette, J., Bockaert, J., and Homburger, V. (2005). Constitutive secretion of protease nexin-1 by glial cells and its regulation by G-protein-coupled receptors. J. Neurosci. 25, 8995–9004. doi: 10.1523/JNEUROSCI.2430-05.2005
Gillespie, P., O'Shea, E., Cullinan, J., Lacey, L., Gallagher, D., Ni Mhaolain, A., et al. (2013). The effects of dependence and function on costs of care for Alzheimer's disease and mild cognitive impairment in Ireland. Int. J. Geriatr. Psychiatry 28, 256–264. doi: 10.1002/gps.3819
Gurnari, C., Nautiyal, I., and Pagliuca, S. (2021). Current opinions on the clinical utility of Ravulizumab for the treatment of paroxysmal nocturnal hemoglobinuria. Ther. Clin. Risk Manag. 17, 1343–1351. doi: 10.2147/TCRM.S273360
Gveric, D., Hanemaaijer, R., Newcombe, J., van Lent, N., Sier, C. F., and Cuzner, M. L. (2001). Plasminogen activators in multiple sclerosis lesions: implications for the inflammatory response and axonal damage. Brain 124, 1978–1988. doi: 10.1093/brain/124.10.1978
Helgadottir, H. T., Lundin, P., Wallén Arzt, E., Lindström, A. K., Graff, C., and Eriksson, M. (2019). Somatic mutation that affects transcription factor binding upstream of CD55 in the temporal cortex of a late-onset Alzheimer disease patient. Hum. Mol. Genet. 28, 2675–2685. doi: 10.1093/hmg/ddz085
Hemani, G., Zheng, J., Elsworth, B., Wade, K. H., Haberland, V., Baird, D., et al. (2018). The MR-base platform supports systematic causal inference across the human phenome. eLife 7:7. doi: 10.7554/eLife.34408
Hernangómez, M., Carrillo-Salinas, F. J., Mecha, M., Correa, F., Mestre, L., Loría, F., et al. (2014). Brain innate immunity in the regulation of neuroinflammation: therapeutic strategies by modulating CD200-CD200R interaction involve the cannabinoid system. Curr. Pharm. Des. 20, 4707–4722. doi: 10.2174/1381612820666140130202911
Hoarau, J. J., Krejbich-Trotot, P., Jaffar-Bandjee, M. C., das, T., Thon-Hon, G. V., Kumar, S., et al. (2011). Activation and control of CNS innate immune responses in health and diseases: a balancing act finely tuned by neuroimmune regulators (NIReg). CNS Neurol. Disord. Drug Targets 10, 25–43. doi: 10.2174/187152711794488601
Holloway, K., Neherin, K., Dam, K. U., and Zhang, H. (2023). Cellular senescence and neurodegeneration. Hum. Genet. 142, 1247–1262. doi: 10.1007/s00439-023-02565-x
Iyer, A. M., Zurolo, E., Boer, K., Baayen, J. C., Giangaspero, F., Arcella, A., et al. (2010). Tissue plasminogen activator and urokinase plasminogen activator in human epileptogenic pathologies. Neuroscience 167, 929–945. doi: 10.1016/j.neuroscience.2010.02.047
Krawczyk, M. C., Godoy, M., Vander, P., Zhang, A. J., and Zhang, Y. (2023). Loss of serpin E2 alters antimicrobial gene expression by microglia but not astrocytes. Neurosci. Lett. 811:137354. doi: 10.1016/j.neulet.2023.137354
Kurki, M. I., Karjalainen, J., Palta, P., Sipilä, T. P., Kristiansson, K., Donner, K., et al. (2003). FinnGen: unique genetic insights from combining isolated population and national health register data. doi: 10.1101/2022.03.03.22271360 [Preprint].
Lambert, J. C., Ibrahim-Verbaas, C. A., Harold, D., Naj, A. C., Sims, R., Bellenguez, C., et al. (2013). Meta-analysis of 74,046 individuals identifies 11 new susceptibility loci for Alzheimer's disease. Nat. Genet. 45, 1452–1458. doi: 10.1038/ng.2802
Lawlor, D. A., Harbord, R. M., Sterne, J. A., Timpson, N., and Davey Smith, G. (2008). Mendelian randomization: using genes as instruments for making causal inferences in epidemiology. Stat. Med. 27, 1133–1163. doi: 10.1002/sim.3034
Lei, S., Hu, M., and Wei, Z. (2024). Identification of systemic biomarkers and potential drug targets for age-related macular degeneration. Front. Aging Neurosci. 16:1322519. doi: 10.3389/fnagi.2024.1322519
Li, D., Yu, Q., Wu, R., Tuo, Z., Wang, J., Ye, L., et al. (2024). Interactions between oxidative stress and senescence in cancer: mechanisms, therapeutic implications, and future perspectives. Redox Biol. 73:103208. doi: 10.1016/j.redox.2024.103208
Li, G. S., Yang, Y. Z., Ma, G. R., Li, P. F., Cheng, Q. H., Zhang, A. R., et al. (2023). Rheumatoid arthritis is a protective factor against Alzheimer's disease: a bidirectional two-sample Mendelian randomization study. Inflammopharmacology 32, 863–871. doi: 10.1007/s10787-023-01397-5
Liu, B., Zhang, B., Wang, T., Liang, Q. C., Jing, X. R., Zheng, J., et al. (2010). Increased expression of urokinase-type plasminogen activator receptor in the frontal cortex of patients with intractable frontal lobe epilepsy. J. Neurosci. Res. 88, 2747–2754. doi: 10.1002/jnr.22419
Livingston, G., Huntley, J., Sommerlad, A., Ames, D., Ballard, C., Banerjee, S., et al. (2020). Dementia prevention, intervention, and care: 2020 report of the lancet commission. Lancet 396, 413–446. doi: 10.1016/S0140-6736(20)30367-6
Madjene, C., Boutigny, A., Bouton, M. C., Arocas, V., and Richard, B. (2021). Protease Nexin-1 in the cardiovascular system: wherefore art thou? Front. Cardiovasc. Med. 8:652852. doi: 10.3389/fcvm.2021.652852
Merrill, S. A., and Brodsky, R. A. (2018). Complement-driven anemia: more than just paroxysmal nocturnal hemoglobinuria. Hematology Am. Soc. Hematol. Educ. Program 2018, 371–376. doi: 10.1182/asheducation-2018.1.371
Musi, N., Valentine, J. M., Sickora, K. R., Baeuerle, E., Thompson, C. S., Shen, Q., et al. (2018). Tau protein aggregation is associated with cellular senescence in the brain. Aging Cell 17:e12840. doi: 10.1111/acel.12840
Nath, A. P., Ritchie, S. C., Grinberg, N. F., Tang, H. H. F., Huang, Q. Q., Teo, S. M., et al. (2019). Multivariate genome-wide association analysis of a cytokine network reveals variants with widespread immune, Haematological, and Cardiometabolic pleiotropy. Am. J. Hum. Genet. 105, 1076–1090. doi: 10.1016/j.ajhg.2019.10.001
Newman, A. M., Gallo, N. B., Hancox, L. S., Miller, N. J., Radeke, C. M., Maloney, M. A., et al. (2012). Systems-level analysis of age-related macular degeneration reveals global biomarkers and phenotype-specific functional networks. Genome Med. 4:16. doi: 10.1186/gm315
Ning, P., Guo, X., Qu, Q., and Li, R. (2023). Exploring the association between air pollution and Parkinson's disease or Alzheimer's disease: a Mendelian randomization study. Environ. Sci. Pollut. Res. Int. 30, 123939–123947. doi: 10.1007/s11356-023-31047-w
Ragno, P. (2006). The urokinase receptor: a ligand or a receptor? Story of a sociable molecule. Cell. Mol. Life Sci. 63, 1028–1037. doi: 10.1007/s00018-005-5428-1
Saez-Atienzar, S., and Masliah, E. (2020). Cellular senescence and Alzheimer disease: the egg and the chicken scenario. Nat. Rev. Neurosci. 21, 433–444. doi: 10.1038/s41583-020-0325-z
Saul, D., Kosinsky, R. L., Atkinson, E. J., Doolittle, M. L., Zhang, X., LeBrasseur, N. K., et al. (2022). A new gene set identifies senescent cells and predicts senescence-associated pathways across tissues. Nat. Commun. 13:4827. doi: 10.1038/s41467-022-32552-1
Tchkonia, T., Zhu, Y., van Deursen, J., Campisi, J., and Kirkland, J. L. (2013). Cellular senescence and the senescent secretory phenotype: therapeutic opportunities. J. Clin. Invest. 123, 966–972. doi: 10.1172/JCI64098
Verbanck, M., Chen, C. Y., Neale, B., and do, R. (2018). Detection of widespread horizontal pleiotropy in causal relationships inferred from Mendelian randomization between complex traits and diseases. Nat. Genet. 50, 693–698. doi: 10.1038/s41588-018-0099-7
Walker, D. G., Lue, L. F., and Beach, T. G. (2002). Increased expression of the urokinase plasminogen-activator receptor in amyloid beta peptide-treated human brain microglia and in AD brains. Brain Res. 926, 69–79. doi: 10.1016/S0006-8993(01)03298-X
Winokur, P. N., Subramanian, P., Bullock, J. L., Arocas, V., and Becerra, S. P. (2017). Comparison of two neurotrophic serpins reveals a small fragment with cell survival activity. Mol. Vis. 23, 372–384
Xu, M., Palmer, A. K., Ding, H., Weivoda, M. M., Pirtskhalava, T., White, T. A., et al. (2015). Targeting senescent cells enhances adipogenesis and metabolic function in old age. eLife 4:e12997. doi: 10.7554/eLife.12997
Yang, J., Luo, J., Tian, X., Zhao, Y., Li, Y., and Wu, X. (2024). Progress in understanding oxidative stress, aging, and aging-related diseases. Antioxidants 13:394. doi: 10.3390/antiox13040394
Yu, S., Chen, M., Xu, L., Mao, E., and Sun, S. (2023). A senescence-based prognostic gene signature for colorectal cancer and identification of the role of SPP1-positive macrophages in tumor senescence. Front. Immunol. 14:1175490. doi: 10.3389/fimmu.2023.1175490
Keywords: Alzheimer’s disease, senescence, transcriptomic analysis, plasma proteome, Mendelian randomization
Citation: Liu Y and Chen J (2024) Senescence-related genes and proteins in the development of Alzheimer’s disease: evidence from transcriptomic and Mendelian randomization analysis. Front. Aging Neurosci. 16:1423725. doi: 10.3389/fnagi.2024.1423725
Received: 26 April 2024; Accepted: 23 July 2024;
Published: 02 August 2024.
Edited by:
Nibaldo C. Inestrosa, Pontificia Universidad Católica de Chile, ChileReviewed by:
Jolanta Dorszewska, Poznan University of Medical Sciences, PolandCopyright © 2024 Liu and Chen. This is an open-access article distributed under the terms of the Creative Commons Attribution License (CC BY). The use, distribution or reproduction in other forums is permitted, provided the original author(s) and the copyright owner(s) are credited and that the original publication in this journal is cited, in accordance with accepted academic practice. No use, distribution or reproduction is permitted which does not comply with these terms.
*Correspondence: Jiao Chen, Y2NjMjAyNGN1bUAxNjMuY29t
Disclaimer: All claims expressed in this article are solely those of the authors and do not necessarily represent those of their affiliated organizations, or those of the publisher, the editors and the reviewers. Any product that may be evaluated in this article or claim that may be made by its manufacturer is not guaranteed or endorsed by the publisher.
Research integrity at Frontiers
Learn more about the work of our research integrity team to safeguard the quality of each article we publish.