- 1Department of Anesthesiology, The First Affiliated Hospital of Wenzhou Medical University, Wenzhou, Zhejiang, China
- 2Department of Obstetrics, The First Affiliated Hospital of Wenzhou Medical University, Wenzhou, Zhejiang, China
Background: Cognitive function (CF) deterioration is a pressing concern in geriatric research. This study aimed to explore the relationship between physical activity (PA) and CF in older adults.
Methods: This study adopted a dual approach, employing both observational and genetic approaches through data from the National Health and Nutrition Examination Survey (NHANES) 2011–2014 and Mendelian Randomization (MR) analysis. For the NHANES component, PA levels were evaluated using the Global Physical Activity Questionnaire, and CF was assessed via standardized tests. Multivariate regression, threshold effect analysis, smoothing curve fitting, and subgroup analyses were conducted to examine the association between PA and CF. In parallel, MR methods, using genetic variants as instrumental variables, assessed the causal impact of PA on CF and related conditions such as Alzheimer’s disease and dementia.
Results: Observational findings from NHANES demonstrated a positive correlation between PA and CF, notably among female participants. The detailed analysis identified specific thresholds of PA that correlate with cognitive enhancements. However, MR results did not support a significant causal relationship between PA and CF or dementia-related outcomes, indicating an absence of a direct genetic basis for the observational associations.
Conclusion: Although observational data from NHANES suggest that PA is positively associated with CF in older adults, particularly among women, MR analysis did not confirm these findings as causally related. The discrepancy highlights the complexity of the PA-CF relationship and underscores the need for further research. These results emphasize the potential of PA as a modifiable risk factor for CF, though causal effects remain to be definitively established.
1 Introduction
The demographic trend toward a more aged global population has intensified the urgency to address cognitive decline and dementia among older adults (Petersen et al., 2014; Rabin et al., 2017; Chowdhary et al., 2021). As cognitive function (CF) is integral to maintaining autonomy, decision-making, and overall quality of life, the escalation in cognitive impairments presents a critical public health challenge (Rabin et al., 2017; Dolphin et al., 2024). This growing prevalence not only profoundly affects individuals but also places a considerable burden on families, healthcare systems, and societal resources (GBD 2015 Neurological Disorders Collaborator Group, 2017; Nandi et al., 2022). Therefore, effective interventions to combat the adverse effects of aging on CF and preserve mental acuity in the elderly are urgently needed.
Physical activity (PA) emerges as a promising non-pharmacological intervention with the potential to significantly enhance cognitive health (Raji et al., 2024). Beyond general health promotion, PA has been identified as a key factor in combating cognitive decline (Palta et al., 2019; Saint-Maurice et al., 2020; Raji et al., 2024). The extant research underscores that regular PA not only improves mental well-being but also acts as a preventive measure against the deterioration of CF (Li et al., 2023; Wang H, et al., 2024). However, comprehensive exploration of the relationship between PA and CF is limited, mostly due to the limited number of studies with large sample sizes and a relative dearth of research on causal correlations. Therefore, this necessitates further investigation to understand the underlying mechanisms and effects.
Although prior studies have recognized the positive impact of PA on cognitive well-being (Jehu et al., 2024; Lim et al., 2024; Mellow et al., 2024; Wang W, et al., 2024), the specific association by which PA influences CF and the causal relationships remain insufficiently understood. To address these limitations, our study adopts a pioneering approach by combining comprehensive cross-sectional data from the National Health and Nutrition Examination Survey (NHANES) 2011–2014 with Mendelian Randomization (MR) data from the UK Biobank. This innovative integration leverages NHANES’s extensive demographic, health, and nutritional data, and MR’s capacity to provide causality insights using genetic variants as instrumental variables (George and Shah, 2003; Md, 2009). Furthermore, Mendelian Randomization (MR) can elucidate causal relationships that are challenging to ascertain in traditional observational studies due to potential confounding factors and reverse causation (Md, 2009).
By amalgamating the detailed, population-wide insights from NHANES with the robust, causality-inferring framework of MR, our study aims to provide a more nuanced understanding of how PA influences CF. This combined approach allows us to transcend the limitations of traditional cross-sectional studies, potentially leading to more precise public health recommendations and the development of targeted, effective cognitive preservation strategies for the aging population. Our study hypothesizes that: Higher levels of PA are positively associated with better CF in older adults. MR analysis will provide evidence on the causality of this relationship.
2 Materials and methods
The NHANES dataset provides comprehensive cross-sectional data, including demographic, health, and nutritional information, which allows us to examine the association between PA and CF in a broad, real-world context. This dataset is used to assess the correlation between PA and CF among different demographic groups, providing valuable insights into the potential public health impact of PA. On the other hand, the MR analysis leverages genetic variants as instrumental variables to explore the causal relationship between PA and CF. By using data from the British Biobank, MR analysis helps to infer causality and reduce the bias inherent in observational studies. This approach allows us to address the limitations of NHANES, such as confounding factors and reverse causation, by providing a robust framework for causal inference. The combination of these two datasets enables a more comprehensive investigation into the relationship between PA and CF. While NHANES offers extensive observational data, MR provides the tools to establish causality. Together, they complement each other, enhancing our understanding of how PA impacts CF and potentially leading to more precise public health recommendations and effective cognitive preservation strategies for the aging population.
2.1 Methods of NHANES
2.1.1 Inclusion and exclusion criteria of participants
In the NHANES 2011–2014, the initial cohort comprised 19,931 participants. Exclusions were made for participants with incomplete PA records (N = 6,203) and those with incomplete CF data (N = 10,981), resulting in a study population of 2,927 individuals (Figure 1).
2.1.2 Physical activity
The assessment of PA levels among participants was conducted utilizing data derived from the NHANES for the years 2011–2014. This period’s data collection employed the Global Physical Activity Questionnaire (GPAQ), encompassing a comprehensive range of questions designed to capture various dimensions of PA (Cleland et al., 2014). Specifically, the questionnaire solicited detailed information on daily activities, leisure time PA, and sedentary behaviors, facilitating a holistic evaluation of participants’ PA profiles (Cleland et al., 2014).
To quantify PA, we adopted methodologies consistent with those utilized in prior research, treating PA both as a continuous variable for analytical purposes and as a categorical variable for ease of interpretation and application to public health guidelines (You et al., 2023; Lei et al., 2024; Wang N, et al., 2024). The categorization of PA levels was grounded in the recommendations set forth by the United States Physical Activity Guidelines (Health USDo, 2008). Accordingly, a high level of PA was operationally defined as achieving 600 metabolic equivalent (MET)-minutes per week or more. Conversely, a low level of PA was defined as accumulating less than 600 MET-minutes per week (Health USDo, 2008). This dichotomous classification enables the examination of PA levels in relation to CF outcomes, aligning with established benchmarks for health-enhancing PA.
2.1.3 Cognitive function
In this study, a series of standardized cognitive evaluations from the NHANES 2011–2014 data set were applied to gage CF in participants. These included the Consortium to Establish a Registry for Alzheimer’s Disease Word List utilized for evaluating both immediate recall over three trials and delayed recall after a set interval, aiming to measure short-term memory and learning retention (Morris et al., 1993). Additionally, verbal fluency and semantic memory were assessed through the Animal Fluency test, wherein participants were tasked with enumerating as many animal names as possible within a minute, reflecting on their capacity for rapid word generation within a specific category (Canning et al., 2004; Brody et al., 2019). The Digit Symbol Substitution Test was incorporated to examine executive function and processing speed, through a symbol-number matching task that tests attention, visual-motor coordination, and cognitive agility (Amaresha et al., 2014; Jaeger, 2018). To normalize the diverse cognitive domain scores for comparative analysis, Z-scores were calculated for each cognitive assessment. A composite score, representing the aggregate mean of these Z-scores, was subsequently computed to encapsulate an overarching measure of CF (Wilson, 2002).
2.1.4 Covariate
In our study, we conscientiously incorporated a selection of covariates to control for potential confounding effects on the association between PA and CF (Jia et al., 2024; Wang X L, et al., 2024). These covariates encompassed key demographic factors (gender, race, age, education level), socio-economic status (Poverty Income Ratio, PIR), health and lifestyle indicators (body mass index, BMI; alcohol consumption frequency; waist circumference; sleep disorders), and health conditions (smoking status, diabetes, depressive symptoms). This careful selection was aimed at ensuring a comprehensive adjustment for variables known to influence cognitive outcomes, thereby enhancing the validity and reliability of our results.
2.1.5 Statistical analysis
In our analysis, we focused on elucidating the relationship between PA and CF (including IR, DR, AF, and DSST), with a particular interest in gender-specific associations. Our investigation into the linear association between PA levels and CF was structured around three models of weighted multiple linear regression to accommodate varying degrees of adjustment. The initial model (Model 1) remained unadjusted. Model 2 introduced adjustments for a set of demographic variables, including race, age, Poverty Income Ratio (PIR), and education level. The most comprehensive model (Model 3) expanded upon this by adjusting for gender, race, age, education level, PIR, BMI, alcohol consumption frequency; waist circumference; sleep disorders, smoking status, diabetes, depressive symptoms. In addition, we employed smoothing curves and Generalized Additive Models (GAMs) to probe for potential nonlinear relationships between PA and CF. Lastly, threshold effect analysis was utilized in our study to enhance the analysis of the specific variations observed between PA and CF.
Statistical analyses were conducted using R software (version 4.2.3) and EmpowerStats (version 2.0), considering p < 0.05 as statistically significant. We represented categorical variables by percentages and continuous variables by their mean values ± standard deviations.
2.2 Mendelian randomization study
2.2.1 Study design
In our investigation, we adopted a two-sample MR framework to elucidate the causal relationships between genetically inferred exposures (average physical activity (AVPA), moderate to vigorous physical activity (MVPA), and vigorous physical activity (VPA)) and health outcomes (CF, Alzheimer’s disease, and dementia). To uphold the integrity of our MR analysis, we strictly adhered to the established triangulation principles: (i) The instrumental genetic variants must exhibit robust associations with the exposures under consideration; (ii) These variants must remain unassociated with potential confounding variables to avoid spurious correlations; and (iii) The influence of these variants on the health outcomes should be mediated exclusively through the stipulated exposures (Emdin et al., 2017).
Our methodology, depicted in Figure 2, utilizes single nucleotide polymorphisms (SNPs) associated with PA levels as instrumental variables (IVs), thus leveraging comprehensive data from genome-wide association studies (GWAS). This strategy mitigates biases inherent in conventional observational studies, offering a more reliable inference of causal relationships.
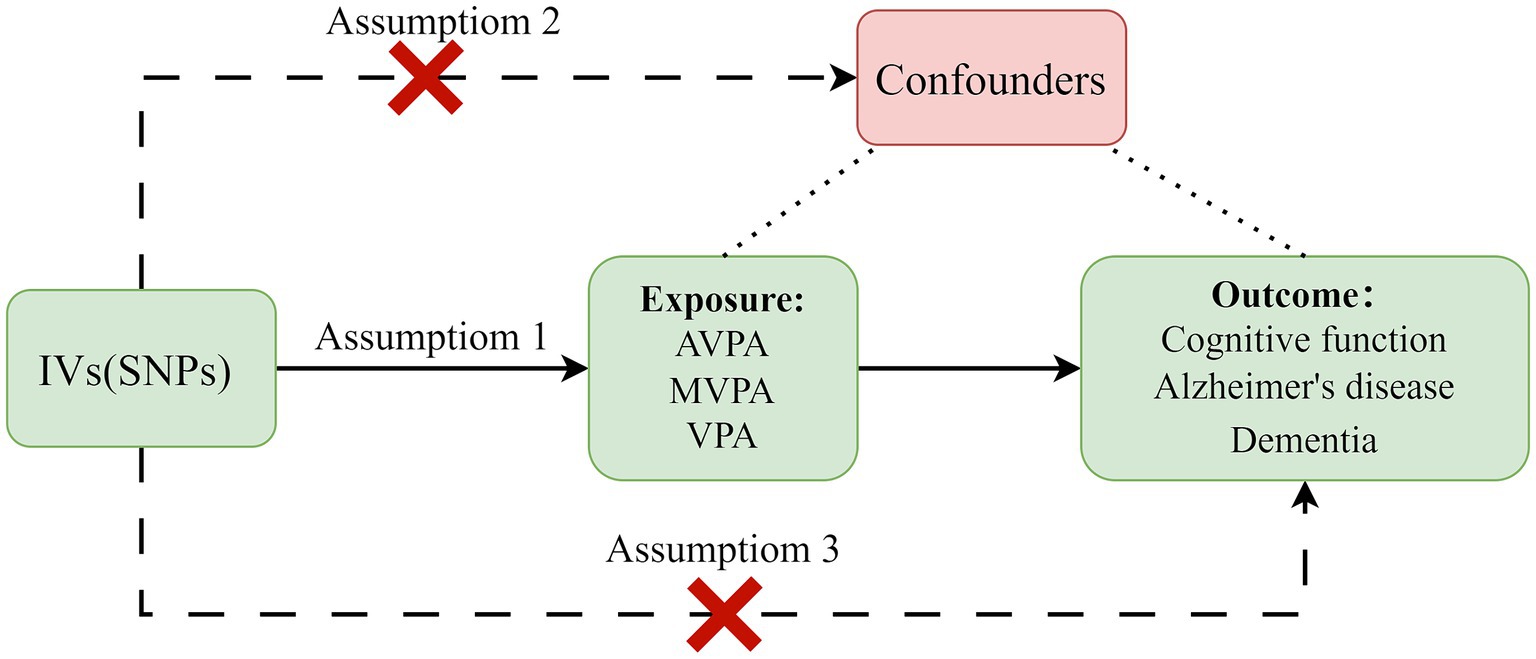
Figure 2. Principles of Mendelian randomization and assumptions. Assumption 1: The instrumental variables must be significantly associated with exposures. Assumption 2: They should not be correlated with any confounders. Assumption 3: Their impact on outcomes should be exclusively mediated through exposures. IVs, instrumental variables; SNPs, single nucleotide polymorphisms; AVPA, average physical activity; MVPA, moderate to vigorous physical activity; VPA, vigorous physical activity.
2.2.2 Genetic instruments selection
AVPA was evaluated using summary statistics from a GWAS analyzing accelerometer-derived PA metrics from approximately 100,000 UK Biobank participants (Klimentidis et al., 2018). Participants were equipped with the Axivity AX3, a wrist-worn triaxial accelerometer, programmed to collect data over a continuous seven-day period. Inclusion criteria mandated a minimum of 3 days (72 h) of data, with requisite activity recorded across all 24-h intervals. Exclusion criteria were applied to participants with insufficient data, uncalibrated devices, or those missing comprehensive daily activity cycles. Additionally, periods of non-wear, identified by stationary episodes exceeding 60 min with minimal deviation across all axes (<13 mg), were algorithmically imputed (Doherty et al., 2017). Unlike NHANES, which assesses PA based on self-reported questionnaires, MR analysis utilizes data from accelerometers (Chudyk et al., 2017).
The analytical model considered three primary metrics derived from the accelerometer data, encompassing a spectrum from 3 to 7 days of wear: Rabin et al. (2017). Average physical activity (AVPA): This metric accounts for all non-zero values of acceleration (Chowdhary et al., 2021). Moderate to vigorous physical Activity (MVPA): This is defined as the proportion of accelerations within the range of >100 mg to <425 mg (Petersen et al., 2014). Vigorous physical activity (VPA): This is defined as the proportion of accelerations exceeding 425 mg, up to 2000 mg (Klimentidis et al., 2018).
These metrics were refined through additional GWAS using the BGENIE software, focusing on decomposed acceleration signals from the UK Biobank (Bycroft et al., 2018). For the MR study, SNPs with strong associations with PA (genome-wide significance threshold p < 5×10−8) were utilized as IVs (Vaucher et al., 2018). We ensured IV independence by setting the linkage disequilibrium correlation coefficient to r2 < 0.001 and selecting a clumping window size of more than 10,000 kb.
To harmonize exposure and outcome data, SNPs that were missing, palindromic, incompatible, or directly related to outcomes were removed. To mitigate weak instrument bias, we calculated the F-statistic for each IV using the formula: to evaluate IV strength (Lawlor et al., 2008). IVs with F-statistics under 10 were excluded to avoid biases from weak IVs, ensuring robustness in our MR methodology (Pierce et al., 2011).
2.2.3 Summary dataset of outcome
Our study’s outcome variables were sourced from the comprehensive 2022 CF and Alzheimer’s disease datasets, under the GWAS identifiers ieu-b-4837 and ieu-b-5067, respectively, as provided by the IEU OpenGWAS project (2023a, 2023b). The dataset includes information from 9,997 and 488,285 European participants, respectively. Additionally, dementia-related outcomes were extracted from the 2021 FinnGen consortium dataset, which includes data from 7,284 cases (n case) and 209,487 controls (n control), offering extensive insights into the genetic underpinnings of dementia and related pathologies (FinnGen consortium dataset, 2023).
2.2.4 Statistical analysis
In our MR analysis, we used the Inverse Variance Weighted (IVW) method as the primary analytical tool (Burgess et al., 2013). The selection between the fixed effect IVW (IVW-FE) and random effect IVW (IVW-RE) models was determined based on the Cochrane Q heterogeneity test outcomes. The IVW-RE model, known for its more conservative estimations, was utilized in scenarios where significant heterogeneity was present (p < 0.05). In contrast, the IVW-FE model was adopted in cases lacking such heterogeneity (Greco et al., 2015).
To bolster the credibility of our findings, three additional analytical methods were used: weighted median, simple mode, and weighted mode. The weighted median approach assumes that fewer than 50% of the IVs are affected by horizontal pleiotropy (Bowden et al., 2016). Crucial sensitivity analyses included MR-Egger regression intercept tests to evaluate pleiotropy (Burgess et al., 2017). Additionally, a leave-one-out analysis was implemented to ensure the stability of our MR findings. This involved sequentially removing each SNP from the instrumental variables and recalculating the causal effect without that specific SNP, aiming to identify any single SNP’s disproportionate influence on the MR estimate (Burgess et al., 2017). Only exposure-outcome pairs that showed a consistent direction across all MR methods and a significant result in all analysis were confirmed to be causal.
Statistical significance was set at p < 0.05, with odds ratio (OR), standard error (SE), and 95% confidence intervals (95% CI) presenting the results of causal associations. These analyses were conducted using the “TwoSampleMR” package (version 0.5.6; Liang et al., 2018) in R software (version 4.2.3).
3 Results
3.1 Results of NHANES
3.1.1 Basic information on the study participants
Table 1 illustrates the demographic characteristics of the study participants. Among the 2,927 participants, 1,330 were males and 1,597 were females, with an average age of 69.20 ± 6.65 years. Significant demographic disparities were observed between genders in terms of age, education level, and the ratio of family income to poverty. Additionally, there were notable differences between males and females regarding the prevalence of diabetes, symptoms of depression, waist circumference, frequency of alcohol consumption, smoking status, and levels of PA. Cognitive performance also significantly differed between participants with high and low levels of PA.
3.1.2 Association between PA and CF stratified by gender
As illustrated in Table 2, PA was analyzed as both a continuous and categorical variable (Low level and High level) in relation to CF, with stratification by gender through multivariate regression analysis. Overall, a positive correlation between PA and CF was identified (p = 0.0248), with the High-level PA group exhibiting significantly superior CF compared to the Low-level PA group (p < 0.001). Upon gender stratification, a positive correlation between PA and CF was observed exclusively in females (p < 0.0142).
Additionally, when analyzing PA against individual cognitive assessments (IR, DR, AF, DSST) stratified by gender (see Supplementary Tables S1–S4 for detailed results), we found varied correlations. Generally, PA showed no significant correlation with IR, DR, and DSST, except for AF. However, participants in the High PA group scored significantly higher on all cognitive tests compared to those in the Low PA group.
Upon gender stratification, both male and female PA levels were positively correlated with AF. Additionally, female PA levels were positively correlated with DSST. Specifically, females with high PA levels significantly outperformed those with low PA levels in all four cognitive tests. In contrast, males with high PA levels only showed significant improvement in AF and DSST.
3.1.3 Smoothing curves and threshold effect analysis
As demonstrated in Table 3 and Figure 3, threshold effect analysis and smoothing curve fitting were applied to explore the relationship between PA and CF. The analysis identified inflection points at 2100 for male PA, and 120 for both female PA and overall PA. For males, PA exhibited a positive correlation with CF at levels below 2,100, with no significant correlation observed above this threshold. In females, PA positively correlated with CF when below 120, but not at higher levels. Similarly, for the general analysis, PA showed a positive correlation with CF when under 120, with no significant relationship beyond this point. The detailed smoothing curve fittings for PA and CF interactions are provided in Figure 3.
Additionally, threshold effect analyses and smoothing curve fittings were conducted for PA against the cognitive assessments of Immediate Recall, Delayed Recall, Animal Fluency, and the Digit Symbol Substitution Test. The results indicated significant nonlinear relationships between PA and all cognitive tests across participants, except for a non-significant relationship between PA and Immediate Recall in male participants. Detailed findings are provided in Supplementary Tables S5–S8 and Supplementary Figures S1, S2.
3.2 Results of MR analysis
In our research, 5 to 18 SNPs were chosen as IVs for exposures. For an in-depth overview of these IVs utilized in MR analysis, please refer to Supplementary Table S9. The F-statistics of each IV varied, ranging from 29.972 to 55.256. Given the heterogeneity observed in MVPA and dementia as determined by Cochrane’s Q test (p < 0.05; detailed in Table 4), the IVW-RE model was chosen as the primary method for MR analysis. The MR results indicated no causal link of PA (AVPA, MVPA, and VPA) on CF, Alzheimer’s disease, and dementia. Specifically, for AVPA, the odds ratio varied from 0.973 (95%CI: 0.909,1.042, p = 0.436) for dementia to 1.014 (95%CI: 0.961, 1.070, p = 0.618) for CF; for MVPA, the odds ratio varied from 0.882 (95%CI: 0.561, 1.385, p = 0.585) for CF to 1.002 (95%CI: 0.998, 1.005, p = 0.388) for Alzheimer’s disease; for VPA, the odds ratio varied from 0.420 (95%CI: 0.133, 1.328, p = 0.140) for CF to 1.801 (95%CI: 0.344, 9.433, p = 0.486) for dementia (Table 4). Other MR methods were finding no causal link either.
Sensitivity analyses, including MR-Egger intercept tests, indicated that horizontal pleiotropy did not significantly impact the MR analysis (all p > 0.05; Table 4). Leave-one-out sensitivity analysis confirmed the robustness of the MR results, showing no significant distortion of outcomes after the removal of any single SNP (Supplementary Figures S3–S11).
4 Discussion
Our study aimed to investigate the relationship between PA and CF using both cross-sectional and MR analysis. The findings from our cross-sectional study indicate that, as a continuous variable, PA is positively associated with CF across the general cohort and specifically within the female subgroup, but not in males. Conversely, when analyzed as a categorical variable, higher levels of PA were consistently associated with superior CF compared to lower levels, across both genders. However, the absence of a causal relationship from our MR analysis between PA levels and CF challenges straightforward interpretations and signals that additional variables may be at play in cognitive health. This divergence emphasizes the intricacies of PA’s effects on cognition and underlines the necessity for broader exploratory efforts in this field.
Our NHANES cross-sectional analysis echoes existing studies in highlighting the link between PA and improved CF in older adults, reinforcing the protective role of PA against cognitive deterioration (Jung et al., 2023; Werneck et al., 2023; You et al., 2023; Zheng et al., 2023). Our study extends these findings by illustrating a gender-specific effect, particularly noting enhanced benefits in older women. Although estrogen, known for its neuroprotective properties, is considered an important hormone for cognitive health (Bagit et al., 2021; Raciti et al., 2023), postmenopausal women experience a natural decline in estrogen levels. Therefore, the improvement in cognitive health in older women through PA may be achieved via other pathways. To delve deeper into the potential mechanisms, some study consider the role of neurotrophic factors, particularly brain-derived neurotrophic factor (BDNF; Peng et al., 2010; Kim et al., 2011; Aaron and Piepmeier Jennifer, 2015). BDNF is a key player in neuroplasticity, neuroprotection, and neurogenesis, all of which are crucial for maintaining cognitive function (Peng et al., 2010; Aaron and Piepmeier Jennifer, 2015). PA has been shown to significantly increase BDNF levels, thereby enhancing cognitive health (Peng et al., 2010; Kim et al., 2011; Aaron and Piepmeier Jennifer, 2015). This effect is especially important for postmenopausal women who experience a decline in estrogen levels (Farrukh et al., 2023). Moreover, the intensity and type of PA can differentially impact BDNF levels. High-intensity interval training (HIIT) has been found to induce more significant and immediate increases in BDNF compared to aerobic exercise, likely due to the intense, intermittent nature of HIIT, which stimulates greater neurotrophic responses (Boyne et al., 2019). However, while single sessions of aerobic exercise may not elevate BDNF levels as dramatically as HIIT, regular aerobic exercise is believed to be more beneficial for BDNF over time, especially in older adults (Boyne et al., 2019; Farrukh et al., 2023). Given that older women tend to engage more in moderate-intensity physical activities (Li et al., 2017; Liao et al., 2021), they seem to experience more sustained BDNF improvement benefits. Additionally, the metabolic and inflammatory responses to PA might differ between genders (Ansdell et al., 2019; Gado et al., 2024). As inflammation and metabolism are closely linked to cognitive health, the more substantial reductions in inflammatory markers and improvements in metabolic profiles resulting from PA could translate into more pronounced cognitive benefits for women (Montero-Odasso et al., 2018; Tan et al., 2023). Furthermore, considering that women generally have higher body fat percentages than men, the metabolic benefits of PA, such as improved insulin sensitivity and lipid profiles, may play a more critical role in maintaining cognitive health in older women (Kullmann et al., 2022; Heni, 2024; Vicente-Gabriel et al., 2024). Further research is needed to explore and validate the underlying effect mechanisms in more detail.
On the other hand, our MR analysis did not demonstrate a causal relationship between PA and CF, diverging from some previous causal findings (Zhang et al., 2022; Cheval et al., 2023). Previous studies, such as those by Zhang et al. (2022) and Cheval et al. (2023), found significant causal links between PA and CF or Alzheimer’s disease using different methodologies and populations. Zhang et al. (2022) found that genetically predicted walking was associated with a reduced risk of Alzheimer’s disease, while no significant association was observed for overall activity, sedentary behavior, or moderate-intensity activity. The protective effect of walking on Alzheimer’s disease risk highlights the potential benefits of specific types of physical activity (Zhang et al., 2022). Cheval et al. (2023) reported significant associations suggesting that MVPA positively influenced cognitive function. However, no causal effect was found for average physical activity (Cheval et al., 2023).
This variance could be attributed to differences in data sources among MR studies. MR analysis rely heavily on the genetic variants chosen as instrumental variables for PA. For instance, genetic instruments derived from one population may not be entirely applicable to another due to differences in lifestyle, environmental exposures, or gene–environment interactions, leading to disparate outcomes (Wang N, et al., 2024). Furthermore, our study’s MR analysis relied on specific sets of SNPs as instrumental variables, which may differ from those used in previous studies. The selection of genetic instruments and the statistical power of the analysis can significantly impact the results. Zhang et al. (2022) and Cheval et al. (2023) employed different MR methodologies and sensitivity analyses, which could account for the discrepancies (Zhang et al., 2022; Cheval et al., 2023). Additionally, time and geographical differences within the datasets should be considered, especially in studies of cognition. The NHANES dataset provides cross-sectional data from the United States, while the MR analysis utilizes data from the UK Biobank. These datasets represent different populations with distinct lifestyle factors, environmental exposures, and healthcare systems, which could influence cognitive outcomes. Furthermore, advancements in technology and data collection methods over the years may also contribute to differences in findings. Ancestry and population mismatch are critical factors that cannot be dismissed, as genetic instruments may not have the same relevance or effect across diverse populations.
The principal strength of our research lies in the innovative use of NHANES 2011–2014 cross-sectional methods combined with MR methods, offering a comprehensive insight into the complex relationship between PA and CF. By leveraging the strengths of both methods, we can explore the association from different analytical perspectives. This innovative approach allows us to explore the PA-CF relationship at a lower cost, significantly reducing the time and financial resources typically required for large-scale randomized controlled trials or longitudinal studies. However, our study is not without limitations. First, the generalizability of our findings is limited. While NHANES is representative of the population in the US, MR study individuals are European. Thus, our results may not be applicable to populations in other countries or regions with different lifestyles, healthcare systems, or genetic backgrounds. Additionally, the multiracial nature of the NHANES database could contribute to the lack of causal associations observed. Differences in genetic backgrounds and environmental exposures across diverse populations may introduce variability that impacts the results. Moreover, one limitation of our study is the variation in PA measurement methods between the datasets used. NHANES assesses PA based on self-reported questionnaires, which may introduce recall bias and subjective interpretation, potentially making PA assessment less accurate compared to accelerometer measurements. Furthermore, the possibility of unidentified SNPs relevant to the study may affect the strength and clarity of the causal relationships we aimed to explore. Lastly, while we have attempted to control for various confounding factors, there may be unmeasured confounders that could affect the observed relationship between PA and CF. Factors such as diet, socioeconomic status, or other lifestyle habits could play a significant role in cognitive health and may not be fully accounted for in our analysis.
5 Conclusion
Our study reveals a positive association between PA and CF in older adults, particularly among women, based on data from NHANES 2011–2014. Despite these associations, the lack of a causal link established through MR suggests the complexity of this relationship and the need for further investigation. These findings underscore the potential of PA as a modifiable factor for enhancing cognitive health in the aging population, highlighting the importance of continued research in this area.
Data availability statement
The original contributions presented in the study are included in the article/Supplementary material, further inquiries can be directed to the corresponding author.
Ethics statement
All data included in this study obtained ethical approval from the respective institutions. Written informed consent was obtained in accordance with the national legislation and the institutional requirements.
Author contributions
H-yL: Data curation, Formal analysis, Writing – original draft, Writing – review & editing. Y-JZ: Formal analysis, Investigation, Methodology, Writing – original draft, Writing – review & editing. W-yZ: Conceptualization, Supervision, Writing – original draft, Writing – review & editing.
Funding
The author(s) declare that no financial support was received for the research, authorship, and/or publication of this article.
Acknowledgments
We extend our gratitude to the NHANES study and the IEU OpenGWAS Project for making their summary statistics publicly available for use in our study.
Conflict of interest
The authors declare that the research was conducted in the absence of any commercial or financial relationships that could be construed as a potential conflict of interest.
Publisher’s note
All claims expressed in this article are solely those of the authors and do not necessarily represent those of their affiliated organizations, or those of the publisher, the editors and the reviewers. Any product that may be evaluated in this article, or claim that may be made by its manufacturer, is not guaranteed or endorsed by the publisher.
Supplementary material
The Supplementary material for this article can be found online at: https://www.frontiersin.org/articles/10.3389/fnagi.2024.1418455/full#supplementary-material
References
IEU OpenGWAS project. (2023a). Cognitive function. Available at: https://gwas.mrcieu.ac.uk/datasets/ieu-b-4838/. (Accessed May 1, 2023).
IEU OpenGWAS project. (2023b). Alzheimer’s disease. Available at: https://gwas.mrcieu.ac.uk/datasets/ieu-b-5067/. (Accessed May 1, 2023).
FinnGen consortium dataset. (2023). Dementia. Available at: https://www.finngen.fi/en/access_results. (Accessed May 1, 2023).
Aaron, T., and Piepmeier Jennifer, L. (2015). Brain-derived neurotrophic factor (BDNF) as a potential mechanism of the effects of acute exercise on cognitive performance. Sports Heal Sci. 4, 14–23. doi: 10.1016/j.jshs.2014.11.001
Amaresha, A. C., Danivas, V., Shivakumar, V., Agarwal, S. M., Kalmady, S. V., Narayanaswamy, J. C., et al. (2014). Clinical correlates of parametric digit-symbol substitution test in schizophrenia. Asian J. Psychiatr. 10, 45–50. doi: 10.1016/j.ajp.2014.03.010
Ansdell, P., Brownstein, C. G., Škarabot, J., Hicks, K. M., Howatson, G., Thomas, K., et al. (2019). Sex differences in fatigability and recovery relative to the intensity-duration relationship. J. Physiol. 597, 5577–5595. doi: 10.1113/JP278699
Bagit, A., Hayward, G. C., and MacPherson, R. E. K. (2021). Exercise and estrogen: common pathways in Alzheimer's disease pathology. Am. J. Physiol. Endocrinol. Metab. 321, E164–E168. doi: 10.1152/ajpendo.00008.2021
Bowden, J., Davey Smith, G., Haycock, P. C., and Burgess, S. (2016). Consistent estimation in Mendelian randomization with some invalid instruments using a weighted median estimator. Genet. Epidemiol. 40, 304–314. doi: 10.1002/gepi.21965
Boyne, P., Meyrose, C., Westover, J., Whitesel, D., Hatter, K., Reisman, D. S., et al. (2019). Exercise intensity affects acute neurotrophic and neurophysiological responses poststroke. J. Appl. Physiol. 126, 431–443. doi: 10.1152/japplphysiol.00594.2018
Brody, D. J., Kramarow, E. A., Taylor, C. A., and Mcguire, L. C. (2019). Cognitive performance in adults aged 60 and over: National Health and nutrition examination survey, 2011-2014. Natl Health Stat Report 126, 1–23.
Burgess, S., Bowden, J., Fall, T., Ingelsson, E., and Thompson, S. G. (2017). Sensitivity analyses for robust causal inference from Mendelian randomization analyses with multiple genetic variants. Epidemiology 28, 30–42. doi: 10.1097/EDE.0000000000000559
Burgess, S., Butterworth, A., and Thompson, S. G. (2013). Mendelian randomization analysis with multiple genetic variants using summarized data. Genet. Epidemiol. 37, 658–665. doi: 10.1002/gepi.21758
Bycroft, C., Freeman, C., Petkova, D., Band, G., Elliott, L. T., Sharp, K., et al. (2018). The UK biobank resource with deep phenotyping and genomic data. Nature 562, 203–209. doi: 10.1038/s41586-018-0579-z
Canning, S. J., Leach, L., Stuss, D., Ngo, L., and Black, S. E. (2004). Diagnostic utility of abbreviated fluency measures in Alzheimer disease and vascular dementia. Neurology 62, 556–562. doi: 10.1212/WNL.62.4.556
Cheval, B., Darrous, L., Choi, K. W., Klimentidis, Y. C., Raichlen, D. A., Alexander, G. E., et al. (2023). Genetic insights into the causal relationship between physical activity and cognitive functioning. Sci. Rep. 13:5310. doi: 10.1038/s41598-023-32150-1
Chowdhary, N., Barbui, C., Anstey, K. J., Kivipelto, M., Barbera, M., Peters, R., et al. (2021). Reducing the risk of cognitive decline and dementia: WHO recommendations. Front. Neurol. 12:765584. doi: 10.3389/fneur.2021.765584
Chudyk, A. M., McAllister, M. M., Cheung, H. K., McKay, H. A., and Ashe, M. C. (2017). Are we missing the sitting? Agreement between accelerometer non-wear time validation methods used with older adults' data. Cogent Med. 4:1313505. doi: 10.1080/2331205X.2017.1313505
Cleland, C. L., Hunter, R. F., Kee, F., Cupples, M. E., Sallis, J. F., and Tully, M. A. (2014). Validity of the global physical activity questionnaire (GPAQ) in assessing levels and change in moderate-vigorous physical activity and sedentary behaviour. BMC Public Health 14:1255. doi: 10.1186/1471-2458-14-1255
Doherty, A., Jackson, D., Hammerla, N., Plötz, T., Olivier, P., Granat, M. H., et al. (2017). Large scale population assessment of physical activity using wrist worn accelerometers: the UK biobank study. PLoS One 12:e0169649. doi: 10.1371/journal.pone.0169649
Dolphin, H., Dyer, A. H., Morrison, L., Shenkin, S. D., Welsh, T., and Kennelly, S. P. (2024). New horizons in the diagnosis and management of Alzheimer's disease in older adults. Age Ageing 53:afae005. doi: 10.1093/ageing/afae005
Emdin, C. A., Khera, A. V., and Kathiresan, S. (2017). Mendelian randomization. JAMA 318, 1925–1926. doi: 10.1001/jama.2017.17219
Farrukh, S., Habib, S., Rafaqat, A., Sarfraz, A., Sarfraz, Z., and Tariq, H. (2023). Association of exercise, brain-derived neurotrophic factor, and cognition among older women: a systematic review and meta-analysis. Arch. Gerontol. Geriatr. 114:105068. doi: 10.1016/j.archger.2023.105068
Gado, M., Tsaousidou, E., Bornstein, S. R., and Perakakis, N. (2024). Sex-based differences in insulin resistance. J. Endocrinol. 261:e230245. doi: 10.1530/JOE-23-0245
George, D. S., and Shah, E. (2003). 'Mendelian randomization': can genetic epidemiology contribute to understanding environmental determinants of disease? Int. J. Epidemiol. 32, 1–22. doi: 10.1093/ije/dyg070
Greco, M. F., Minelli, C., Sheehan, N. A., and Thompson, J. R. (2015). Detecting pleiotropy in Mendelian randomisation studies with summary data and a continuous outcome. Stat. Med. 34, 2926–2940. doi: 10.1002/sim.6522
Health USDo (2008). Physical activity guidelines for Americans, vol. 2008. Washington: ODPHP publication.
Heni, M. (2024). The insulin resistant brain: impact on whole-body metabolism and body fat distribution. Diabetologia 67, 1181–1191. doi: 10.1007/s00125-024-06104-9
Jaeger, J. (2018). Digit symbol substitution test: the case for sensitivity over specificity in neuropsychological testing. J. Clin. Psychopharmacol. 38, 513–519. doi: 10.1097/JCP.0000000000000941
Jehu, D. A., Pottayil, F., Dong, Y., Zhu, H., Sams, R., and Young, L. (2024). Exploring the association between physical activity and cognitive function among people living with dementia. J. Alzheimers Dis., 1–11. doi: 10.3233/JAD-230594
Jia, W., Wang, H., Li, C., Shi, J., Yong, F., and Jia, H. (2024). Association between dietary vitamin B1 intake and cognitive function among older adults: a cross-sectional study. J. Transl. Med. 22:165. doi: 10.1186/s12967-024-04969-3
Jung, M., Lee, S., Kang, M., and Allen, H. K. (2023). Age-varying association between depression symptoms and executive function among older adults: moderation by physical activity. J. Psychiatr. Res. 165, 115–122. doi: 10.1016/j.jpsychires.2023.07.025
Kim, J. M., Stewart, R., Bae, K. Y., Kim, S. W., Yang, S. J., Park, K. H., et al. (2011). Role of BDNF val66met polymorphism on the association between physical activity and incident dementia. Neurobiol. Aging 32, 551.e5–551.e12. doi: 10.1016/j.neurobiolaging.2010.01.018
Klimentidis, Y. C., Raichlen, D. A., Bea, J., Garcia, D. O., Wineinger, N. E., Mandarino, L. J., et al. (2018). Genome-wide association study of habitual physical activity in over 377,000 UK biobank participants identifies multiple variants including CADM2 and APOE. Int. J. Obes. 42, 1161–1176. doi: 10.1038/s41366-018-0120-3
Kullmann, S., Goj, T., Veit, R., Fritsche, L., Wagner, L., Schneeweiss, P., et al. (2022). Exercise restores brain insulin sensitivity in sedentary adults who are overweight and obese. JCI Insight 7:e161498. doi: 10.1172/jci.insight.161498
GBD 2015 Neurological Disorders Collaborator Group. (2017). Global, regional, and national burden of neurological disorders during 1990-2015: a systematic analysis for the global burden of disease study 2015. Lancet Neurol. 16, 877–897. doi: 10.1016/S1474-4422(17)30299-5
Lawlor, D. A., Harbord, R. M., Sterne, J. A., Timpson, N., and Davey, S. G. (2008). Mendelian randomization: using genes as instruments for making causal inferences in epidemiology. Stat. Med. 27, 1133–1163. doi: 10.1002/sim.3034
Lei, L., Li, J., Wang, W., Yu, Y., Pu, B., Peng, Y., et al. (2024). The associations of "weekend warrior" and regularly active physical activity with abdominal and general adiposity in US adults. Obesity (Silver Spring) 32, 822–833. doi: 10.1002/oby.23986
Li, X., Peng, A., Li, L., and Chen, L. (2023). Association between walking and square dancing-oriented leisure-time physical activity and cognitive function among middle-aged and elderly people in Southwest China. BMC Geriatr. 23:28. doi: 10.1186/s12877-023-03737-0
Li, W., Procter-Gray, E., Churchill, L., Crouter, S. E., Kane, K., Tian, J., et al. (2017). Gender and age differences in levels, types and locations of physical activity among older adults living in Car-dependent neighborhoods. J. Frailty Aging 6, 129–135. doi: 10.14283/jfa.2017.15
Liang, Y., Wu, D., Ledesma, D., Davis, C., Slaughter, R., and Guo, Z. (2018). Virtual tai-chi system: a smart-connected modality for rehabilitation. Smart Health. 9-10, 232–249. doi: 10.1016/j.smhl.2018.07.021
Liao, Y. H., Kao, T. W., Peng, T. C., and Chang, Y. W. (2021). Gender differences in the association between physical activity and health-related quality of life among community-dwelling elders. Aging Clin. Exp. Res. 33, 901–908. doi: 10.1007/s40520-020-01597-x
Lim, S. T., Kwak, H. B., Kang, J. H., Chang, E., Joa, K. L., Park, H. J., et al. (2024). Effects of physical activity participation on cognitive impairment in older adults population with disabilities. Front. Public Health 12:1293023. doi: 10.3389/fpubh.2024.1293023
Mellow, M. L., Dumuid, D., Olds, T., Stanford, T., Dorrian, J., Wade, A. T., et al. (2024). Cross-sectional associations between 24-hour time-use composition, grey matter volume and cognitive function in healthy older adults. Int. J. Behav. Nutr. Phys. Act. 21:11. doi: 10.1186/s12966-023-01557-4
Montero-Odasso, M., Almeida, Q. J., Burhan, A. M., Camicioli, R., Doyon, J., Fraser, S., et al. (2018). SYNERGIC TRIAL (SYNchronizing exercises, remedies in gait and cognition) a multi-Centre randomized controlled double blind trial to improve gait and cognition in mild cognitive impairment. BMC Geriatr. 18:93. doi: 10.1186/s12877-018-0782-7
Morris, J. C., Edland, S., Clark, C., and Galasko, D. (1993). The consortium to establish a registry for Alzheimer's disease (CERAD). Part IV. Rates of cognitive change in the longitudinal assessment of probable Alzheimer's disease. Neurology 43, 1159–1165.
Nandi, A., Counts, N., Chen, S., Seligman, B., Tortorice, D., Vigo, D., et al. (2022). Global and regional projections of the economic burden of Alzheimer's disease and related dementias from 2019 to 2050: a value of statistical life approach. EClinicalMedicine. 51:101580. doi: 10.1016/j.eclinm.2022.101580
Palta, P., Sharrett, A. R., Deal, J. A., Evenson, K. R., Gabriel, K. P., Folsom, A. R., et al. (2019). Leisure-time physical activity sustained since midlife and preservation of cognitive function: the atherosclerosis risk in communities study. Alzheimers Dement. 15, 273–281. doi: 10.1016/j.jalz.2018.08.008
Peng, S., Wuu, J., Mufson, E. J., and Fahnestock, M. (2010). Precursor form of brain-derived neurotrophic factor and mature brain-derived neurotrophic factor are decreased in the pre-clinical stages of Alzheimer's disease. J. Neurochem. 93, 1412–1421. doi: 10.1111/j.1471-4159.2005.03135.x
Petersen, R. C., Caracciolo, B., Brayne, C., Gauthier, S., Jelic, V., and Fratiglioni, L. (2014). Mild cognitive impairment: a concept in evolution. J. Intern. Med. 275, 214–228. doi: 10.1111/joim.12190
Pierce, B. L., Ahsan, H., and Vanderweele, T. J. (2011). Power and instrument strength requirements for Mendelian randomization studies using multiple genetic variants. Int. J. Epidemiol. 40, 740–752. doi: 10.1093/ije/dyq151
Rabin, L. A., Smart, C. M., and Amariglio, R. E. (2017). Subjective cognitive decline in preclinical Alzheimer's disease. New York: Social Science Electronic Publishing.
Raciti, L., Formica, C., Raciti, G., Quartarone, A., and Calabrò, R. S. (2023). Gender and Neurosteroids: implications for brain function, neuroplasticity and rehabilitation. Int. J. Mol. Sci. 24:4758. doi: 10.3390/ijms24054758
Raji, C. A., Meysami, S., Hashemi, S., Garg, S., Akbari, N., Ahmed, G., et al. (2024). Exercise-related physical activity relates to brain volumes in 10,125 individuals. J. Alzheimers Dis. 97, 829–839. doi: 10.3233/JAD-230740
Saint-Maurice, P. F., Troiano, R. P., Bassett, D. R. Jr., Graubard, B. I., Carlson, S. A., Shiroma, E. J., et al. (2020). Association of Daily Step Count and Step Intensity with Mortality among US adults. JAMA 323, 1151–1160. doi: 10.1001/jama.2020.1382
Tan, L. F., Chan, Y. H., Seetharaman, S., Denishkrshna, A., Au, L., Kwek, S. C., et al. (2023). Impact of exercise and cognitive stimulation therapy on physical function, cognition and muscle mass in pre-frail older adults in the primary care setting: a cluster randomized controlled trial. J. Nutr. Health Aging 27, 438–447. doi: 10.1007/s12603-023-1928-7
Vaucher, J., Keating, B. J., Lasserre, A. M., Gan, W., Lyall, D. M., Ward, J., et al. (2018). Cannabis use and risk of schizophrenia: a Mendelian randomization study. Mol. Psychiatry 23, 1287–1292. doi: 10.1038/mp.2016.252
Vicente-Gabriel, S., Lugones-Sánchez, C., Tamayo-Morales, O., Vicente Prieto, A., González-Sánchez, S., Conde Martín, S., et al. (2024). Relationship between addictions and obesity, physical activity and vascular aging in young adults (EVA-Adic study): a research protocol of a cross-sectional study. Front. Public Health 12:1322437. doi: 10.3389/fpubh.2024.1322437
Wang, X. L., Feng, H. L., Xu, X. Z., Liu, J., and Han, X. (2024). Relationship between cognitive function and weight-adjusted waist index in people ≥ 60 years old in NHANES 2011-2014. Aging Clin. Exp. Res. 36:30. doi: 10.1007/s40520-023-02649-8
Wang, H., Pei, Z., and Liu, Y. (2024). Effects of square dance exercise on cognitive function in elderly individuals with mild cognitive impairment: the mediating role of balance ability and executive function. BMC Geriatr. 24:156. doi: 10.1186/s12877-024-04714-x
Wang, W., Sun, P., Lv, T., and Li, M. (2024). The impact of modifiable health metrics on mortality for older adults with low cognitive function. Front. Public Health 12:1304876. doi: 10.3389/fpubh.2024.1304876
Wang, N., Wang, L., Wang, J., Chen, R., Shi, M., Liu, H., et al. (2024). Effects of physical activity and depressive symptoms on cognitive function in older adults: National Health and nutrition examination survey. Neurol. Sci. 45, 299–308. doi: 10.1007/s10072-023-07250-5
Werneck, A. O., Araujo, R. H. O., Silva, D. R., and Vancampfort, D. (2023). Handgrip strength, physical activity and incident mild cognitive impairment and dementia. Maturitas 176:107789. doi: 10.1016/j.maturitas.2023.107789
Wilson, R. S. (2002). Participation in cognitively stimulating activities and risk of incident Alzheimer disease. JAMA 287:742. doi: 10.1001/jama.287.6.742
You, Y., Chen, Y., Chen, X., Wei, M., Yin, J., Zhang, Q., et al. (2023). Threshold effects of the relationship between physical exercise and cognitive function in the short-sleep elder population. Front. Aging Neurosci. 15:1214748. doi: 10.3389/fnagi.2023.1214748
Zhang, B., Huang, X., Wang, X., Chen, X., Zheng, C., Shao, W., et al. (2022). Using a two-sample mendelian randomization analysis to explore the relationship between physical activity and Alzheimer's disease. Sci. Rep. 12:12976. doi: 10.1038/s41598-022-17207-x
Zheng, P., Pleuss, J. D., Turner, D. S., Ducharme, S. W., and Aguiar, E. J. (2023). Dose-response association between physical activity (daily MIMS, peak 30-minute MIMS) and cognitive function among older adults: NHANES 2011-2014. J. Gerontol. A Biol. Sci. Med. Sci. 78, 286–291. doi: 10.1093/gerona/glac076
Keywords: physical activity, cognitive function, older adults, NHANES, Mendelian randomization
Citation: Liu H-y, Zhang Y-J and Zhang W-y (2024) Exploring the association of physical activity on cognitive function in older adults from observational and genetic insights: a combined NHANES and Mendelian randomization study. Front. Aging Neurosci. 16:1418455. doi: 10.3389/fnagi.2024.1418455
Edited by:
Takao Yamasaki, Minkodo Minohara Hospital, JapanReviewed by:
Srinivasa Rao Pachava, Guru Nanak Dev University, IndiaRola Angga Lardika, Riau University, Indonesia
Copyright © 2024 Liu, Zhang and Zhang. This is an open-access article distributed under the terms of the Creative Commons Attribution License (CC BY). The use, distribution or reproduction in other forums is permitted, provided the original author(s) and the copyright owner(s) are credited and that the original publication in this journal is cited, in accordance with accepted academic practice. No use, distribution or reproduction is permitted which does not comply with these terms.
*Correspondence: Wen-you Zhang, zwywzmu@outlook.com